- Faculty of Biosciences, Fisheries and Economics, Norwegian College of Fishery Science, UiT-The Arctic University of Norway, Tromsø, Norway
Fisheries are complex adaptive social-ecological systems (SES) that consist of interlinked human and ecosystems. They have mainly been studied by the natural sciences and focused on the ecosystem. However, rising concerns about sustainability and increasing complexity of societal challenges often require an understanding of fisheries in a SES context. For this purpose, the study of the human system should be expanded within fisheries science. Models are currently the most common method used in the field and these need to include the human dimension, alongside the ecosystem, when addressing fisheries systems as SES. The human dimension is an umbrella term for the complex web of human processes and it is captured by disciplines from the social sciences and the humanities. Consequently, capturing and synthesizing the variety of disciplines involved in the human dimension, and integrating them into fisheries models, requires an interdisciplinary approach. This study attempts to assess the presence of the human dimension in fisheries models applied to a European Union context and to evaluate interdisciplinarity within modeled human dimension aspects through a systematic review and qualitative analysis. Within 31 modeling publications, 20 different human dimension aspects could be identified within the categories of social phenomena, social processes, and individual attributes. Most of the human dimension aspects were modeled in an interdisciplinary manner in mathematical, statistical, simulation, or conceptual models. Yet, predominantly through the use of economic and environmental variables. We conclude that there is potential for the expansion of the human dimension and interdisciplinarity in fisheries models. To reach this potential, one should consider early involvement of all relevant disciplines in the formulation of theories, identification of data, and in the model development. We provide recommendations for interdisciplinary model development, communication, and documentation to increase our understanding of fisheries as SES.
Introduction
Fisheries have been recognized as social-ecological systems (SES), that couple a human system with a natural one (Ostrom, 2009). These two subsystems are connected and intertwined, and have a two-way feedback relationship, where a change in one of the subsystems can impact the other, and vice-versa (Berkes, 2011). SES also have the characteristics of complex adaptive systems, such as non-linearity, uncertainty, and self-organization (Levin et al., 2012; Leenhardt et al., 2015). Thus, fisheries can be understood as social-ecological complex adaptive systems.
The field of fisheries science has been traditionally dominated by natural scientists (Link, 2010). Their research efforts have focused mainly on topics relating to the natural subsystem (Syed et al., 2018). As a result, the lack of consideration of the human subsystem has in some cases led to management and policy failures (Freire and Garcia-Allut, 2000; Österblom et al., 2011). Yet, rising concerns about sustainability and the increasing complexity of societal challenges, e.g., fisheries under climate change, require an understanding of the interconnections of fisheries in a broader context (Starfield and Jarre, 2011; Elsevier SciDev.Net, 2015; Rissman and Gillon, 2017; Marshall et al., 2018). Therefore, to better understand and address such societal and sustainability concerns, fisheries science will have to expand to a SES perspective by including considerations of the human subsystem alongside the natural one (Ostrom, 2009; Hicks et al., 2016).
Fisheries science commonly uses modeling approaches to assess and explore fisheries systems for knowledge production and to provide management advice. Models are the most commonly used method in this field (Jarić et al., 2012). Models are a common way to integrate various data and additional considerations on, for example, theory or indicators (Link, 2010, p. 89). Models can provide an inspiring point of departure and a guiding principle for interdisciplinarity (e.g., Heemskerk et al., 2003), and models have a high potential to be used as an integrative research method in itself. Consequently, including considerations of the human subsystem into these models could provide a better understanding of fisheries as SES. However, the human subsystem is not easily captured, as it is a broad and diverse field of study.
The umbrella term “human dimension” in relation to fisheries has been used in order to refer to the diversity within the human subsystem and to highlight its importance (OECD, 2007; Charnley et al., 2017). The human dimension (HD) can be understood as a complex web of human processes that relate to natural resources (Spalding et al., 2017). It can be categorized into social phenomena, social processes, and individual attributes (Bennett et al., 2017). To study the HD, human dimension aspects (HDA) (i.e., smaller components within an HD category) are often analyzed, such as compliance or trust. Due to the diversity of the human subsystem, the HD and its HDAs are addressed by many different disciplines, ranging across the social sciences and the humanities (Bennett et al., 2017; Spalding et al., 2017).
Fisheries models commonly use environmental, fisheries and economic data, because these data are available from surveys, log-books, landing-, and sales statistics. Economic and environmental considerations are also very prominent in frameworks for a comprehensive approach to fisheries management (Stephenson et al., 2018). Consideration of the HD and the collection of HD data has been falling short and social data is often unavailable or lacking (Hatchard and Gray, 2014). Social information is also more difficult to collect as social issues range from individual to global concerns (Bennett et al., 2017), additionally hindering the quantification of HDAs (Symes and Phillipson, 2009; Hatchard and Gray, 2014). In cases where social science data has been provided, information is usually presented in the form of descriptive text, which is often neither read, nor integrated into fisheries assessments in a meaningful way (Hall-Arber et al., 2009).
While the inclusion of HD in models is generally driven by the research question, sustainability and societal challenges create a need for fisheries models to capture the HD and its diversity. Through multi- and interdisciplinary efforts, the necessary support for the inclusion of the broad concept of HD can be provided. However, it remains unclear to what extend the HD has been integrated into fisheries models and how interdisciplinary the field of HD in fisheries models is at present, and into what areas and approaches it could be expanded.
The aim of this study is to assess the presence of HD in fisheries models, to evaluate interdisciplinarity within modeled HDAs and to explore the modeling approaches used to model different HDAs. These objectives were translated into the following research questions: How interdisciplinary is the field of the human dimension in fisheries modeling? Is there a gap between the HDAs that are modeled and those that could be modeled? Are HDAs modeled in an interdisciplinary manner? Is there a relation between the modeled HDAs and the modeling approaches?
Conceptual Framework
Interdisciplinarity
In this study, we understand interdisciplinarity as an attempt at mutual interaction between disciplinary components that involves crossing the boundaries of several academic disciplines with contrasting research paradigms in order to create new theories and knowledge (Tress et al., 2005). Interdisciplinary activities and studies apply, synthesize, integrate, or transcend parts of two or more disciplines with a common goal (Tress et al., 2005; Huutoniemi et al., 2010; Chiu et al., 2013). To make the distinction, multidisciplinarity involves several academic disciplines that have multiple parallel goals, often with the purpose of comparison, but does not cross subject boundaries or aim for any form of integration. Transdisciplinarity combines interdisciplinarity with a participatory approach by involving non-academic participants and knowledge bodies to create new knowledge and theory (Tress et al., 2005).
To assess interdisciplinarity within the field of the HD in fisheries models, we used the typology and indicators for interdisciplinarity developed by Huutoniemi et al. (2010). This typology considers interdisciplinarity on three dimensions: (1) the scope of interdisciplinarity, i.e., what is being integrated; (2) the type of interdisciplinary interaction, i.e., how it is being done; and (3) the types of goals, i.e., why an interdisciplinary approach is being used.
The scope of interdisciplinarity refers to the conceptual and cultural distance between the participating disciplines or research fields. It is understood as narrow if the participating fields are conceptually close to each other (e.g., life sciences and biological sciences), whereas it is considered broad when the fields are conceptually diverse (e.g., law and engineering). The type of interdisciplinary interaction describes how interdisciplinarity is being carried out, and three different approaches can be distinguished: empirical, methodological, and theoretical. Empirical interdisciplinarity integrates different types of empirical data (e.g., qualitative and quantitative data). Methodological interdisciplinarity implies the integration of different methodological approaches. As we chose to explore only models as a fisheries research methodology, this dimension of interdisciplinarity has not been assessed in this study. Theoretical interdisciplinarity occurs when concepts, models, or theories from more than one field or discipline are synthesized in order to develop new theoretical tools (Huutoniemi et al., 2010). By considering only empirical and theoretical interdisciplinarity, we assumed that the HD should be fit into fisheries models and did not consider potential other methodological approaches that could be suitable for studying fisheries as SES and providing science advice to management.
The types of interdisciplinary goals can be epistemologically oriented to increase knowledge, or instrumentally oriented to achieve an extra-academic goal or solve a societal problem. The types of interdisciplinary goals can also have a mixed orientation when they have both, an epistemological and an instrumental orientation.
Methodology
In order to address our research questions, we employed a systematic literature review (SLR) approach that consisted of three consecutive steps: (1) relevant literature was collected and selected in a systematic, reproducible manner; (2) the selected literature was analyzed in a qualitative way through content analysis and hierarchical coding, which was followed by (3) the design of data visualizations. Subsequently, we applied a typology and indicators to assess interdisciplinarity within the data. In the SLR, we classified models as any method that the authors of the publication referred to as a model, which included qualitative and quantitative models, process and conceptual models and frameworks. All the applied methods are explained in detail in the following sections, followed by their limitations.
Literature Collection and Selection
In order to select a large enough sample of papers on fisheries models to study the practices being used to model the HD, we decided to use a systematic approach. This provides transparency and replicability and makes the choice of the publications under review comprehensible by determining: 1. a set of keywords to be used as search terms in an unbiased academic search engine, and 2. clear inclusion and exclusion criteria by which the resulting literature will be evaluated. These steps are described in sections Search Terms and Inclusion and Exclusion Criteria.
This methodology is commonly referred to as a Systematic Literature Review (SLR) and is an effective approach for sampling the literature in a systematic and reproducible way. SLRs are commonly applied in fields such as medical science (e.g., Weitzen et al., 2004) and software engineering (e.g., Kitchenham et al., 2009), and they are an emerging method in fields such as organizational studies (Maier et al., 2016), education (e.g., Hainey et al., 2016), and marine and coastal studies (e.g., Liquete et al., 2013).
Search Terms
The literature search was conducted using the scientific search engine Scopus (www.scopus.com), where the search terms “fisheries,” “model*,” and “common fisheries policy” were employed to select for peer-reviewed publications on fisheries models. All subject areas as identified by Scopus (i.e., life sciences, health sciences, physical sciences, social sciences, and humanities) and all possible publication years were selected. The precise search string used in Scopus can be found in Appendix S1 (Supplementary Material). The search was conducted on 25/08/2015.
We used the term “fisheries” in order to select for models with a system perspective, rather than select for models only considering the environmental components (e.g., fish), and therefore we did not use the search term “fish*.” To achieve a general perspective on the field of fisheries modeling, we chose not to limit this study to a particular modeling technique (e.g., Bayesian belief networks) or a particular model type (e.g., stock assessment). Thus, we sampled models created for a large variety of fisheries that are performing under similar managerial assumptions. Among the multitude of possible managerial assumptions, we chose the Common Fisheries Policy of the European Union (EU), a common set of rules that applies to all EU fishing fleets and EU fish stocks. This decision was driven mainly by the fact that the EU fisheries are among the most extensively studied in the world (Jarić et al., 2012), therefore presumably offering a large, but still manageable, sample for qualitative analysis. It also allowed us to narrow down the number of publications to a number feasible for qualitative analysis.
Inclusion and Exclusion Criteria
The full text of all publications was downloaded, and the publication metadata was exported from Scopus, including authors, title, year, journal, and journal subject areas. All articles were screened for relevance to the study objectives and included or excluded based on the criteria listed in Table 1. Throughout this process, we followed the guidelines for systematic reviews in conservation and environmental management (Pullin and Stewart, 2006), and the PRISMA reporting guidelines (Moher et al., 2009; see Appendix S2). These guidelines ensure a thorough execution of the sampling and analysis of the literature while carrying out the SLR.
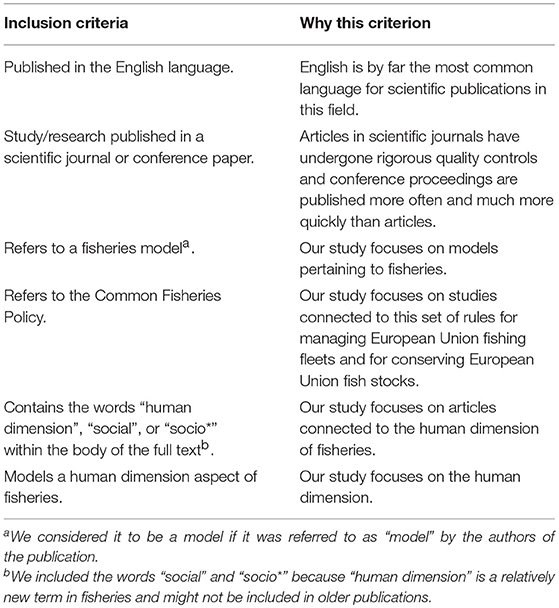
Table 1. Inclusion criteria used to select publications for the systematic literature review of modeling the human dimension in fisheries models.
Content Analysis
The SLR process was followed by a qualitative analysis and synthesis through content analysis, which is a research methodology for making valid inferences from texts in a replicable manner (Krippendorff, 2013). This study followed a problem-driven approach to content analysis, which means that it was motivated by epistemic questions about currently inaccessible information that the text is assumed to be able to answer (Krippendorff, 2013). During our content analysis, coding categories and recording instructions were developed, and an analytical procedure was selected. These steps are explained in detail in sections Model Classification and Coding of the Human Dimension Aspects.
Model Classification
Information on the types of models e.g., Bayesian belief network, bioeconomic model, etc., were extracted from the publications and recorded in Microsoft Excel 2016. In order to classify the models, we applied a general model typology based on Hanneman (1995), which differentiates between mathematical, statistical, and simulation models. In addition, we added the model category “conceptual” based on Miles and Huberman (1994, p. 18). The applied typology distinguished between models as follows:
Mathematical
A mathematical model translates the relations among concepts in a theory into explicit dynamic formalism, using mathematical concepts and formulas.
Statistical
A statistical model describes the probabilistic estimation of parameters from empirical data in such a way as to choose the values of parameters that are most likely to have produced the data.
Simulation
A simulation model consists of a number of states, i.e., actors or variables, or both, and a set of explicit hypotheses about how change occurs in these states over time. In computer simulations, these states are expressed as systems of equations using mathematical or logical operators.
Conceptual
A conceptual model or framework explains, either graphically or in narrative form, the main things to be studied—the key factors, constructs or variables, and the presumed relationships among them. The models and frameworks can be rudimentary or elaborate, theory-driven or commonsensical, descriptive or causal.
Coding of the Human Dimension Aspects
The content of the selected publications, i.e., the information relevant to the research questions of this study, was analyzed through coding and the development of a category system. Coding is the process of categorizing and organizing information into a meaningful framework (Johnson, 2007) to empower and speed up systematic qualitative data analysis (Lofland et al., 2006). The term coding refers to the process of reading the data and dividing it into meaningful analytical units, also known as segmenting the data. Once a meaningful unit has been identified, it is coded, which means that the unit is marked with a descriptive word or a category name. During coding, a master list is maintained in order to keep track of all previously coded units, so that codes can be reapplied to new data segments each time an appropriate unit or segment is discovered within the text (Johnson, 2007). We developed an indicative code, which means that it was created by the researcher whilst directly inspecting the data, in contrast to, for example, using a pre-existing set of codes that had been developed a priori to the analysis.
We coded the data according to a hierarchical category system. This enables organization of the data into different levels or categories based on the idea that some themes are more general than others, and that codes are therefore related vertically (Johnson, 2007). We used the term “function” to describe the categorical relationship between the codes. A functional relationship between two variables essentially means: X is used for Y (Johnson, 2007).
In the code developed for this study the main aspect modeled by a publication, or the main subject of the model, was coded as the first hierarchical unit representing the general theme and overall goal. The main aspect modeled was identified based on what the authors themselves stated in the title, the abstract, or the introduction to the article (e.g., “…we modeled the exploitation of a fishery…”). The theme identified as the overall goal or main aspect of the model was categorized into one of three dimensions: human/social, economic, or environmental, or a combination of these (see section Assigning the Dimensions Identified in the Fisheries Models to the Human Dimension Aspects).
Only studies whose main aspect was identified as the HD were analyzed in depth via further hierarchical coding to determine through which variables they had been modeled. Two more descending hierarchies were introduced into the coding, which resulted in a three-level code hierarchy: Level 1—the main HDA; Level 2—variables that were used to model Level 1 and the functional relationship between them; Level 3—variables that were employed to model Level 2 and the functional relationship between them. In more mathematical terms, this can be described as follows:
where HDA is the main HDA, (Level 1), which is modeled as a function F of the variables b and c, and where b is modeled as a function G of the variables d and e.
All these variables were coded in NVivo 11 (QSR International Pty Ltd, 2015). The codes, which are represented as nodes in NVivo, were assigned to hierarchical categories in order to distinguish between Level 1, Level 2, and Level 3 variables (Figure 1).
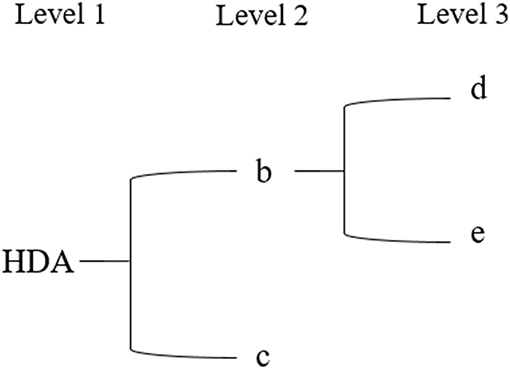
Figure 1. A conceptual display of the hierarchy of variables used to model the main Human Dimension Aspects (HDA) of the human dimension fisheries models. Level 1 represents the main HDA, and Levels 2 and 3 represent the variables (b,c and d,e) that were used in a functional relationship to model the HDA.
Assigning the Dimensions Identified in the Fisheries Models to the Human Dimension Aspects
The identified HDAs and other variables were assigned to the dimensions described previously (human/social, economic, and environmental) based on the indicators for sustainable development of marine capture fisheries developed by the Food and Agriculture Organization (FAO) of the United Nations (FAO Fishery Resources Division, 1999). We included the FAO's governance dimension in the social one and renamed the latter as the human dimension. The economic dimension was treated as a dimension in its own, as the tradition of treating it separately in fisheries science seems to be very strong (Haapasaari et al., 2012). We found the FAO framework appropriate given its global penetration level and authority in fisheries science, but we are aware that other categorizations and divisions of fisheries systems exist (e.g., Charles, 2000). The HDAs were categorized into three topics as described by Bennett et al. (2017): social phenomena, social processes, and individual attributes.
Enumeration and Visualization of the Qualitative Data
The qualitative coding analysis of the publications was followed by enumeration, which refers to the quantification of the qualitative data and coding results, for example, the number of HDAs and the human/social, economic, and environmental variables for each HDA were counted. The enumeration of the qualitative data was conducted using the software NVivo 11 (QSR International Pty Ltd, 2015) because computer-aided qualitative data analysis allows for the automated enumeration while enabling all data to be exported into other formats (e.g., csv, excel, etc.). Visualizations were created using Gephi (Version 0.9.1), which is an open source visualization tool for graph and network analysis (Bastian et al., 2009). A detailed description of this approach is provided in Appendix S3 (Supplementary Material).
Assessment of Interdisciplinarity
Interdisciplinarity was assessed based on the typology and indicators described by Huutoniemi et al. (2010), as explained in section Conceptual Framework. We assessed interdisciplinarity in the modeling of the HD in fisheries through: (1) indicators of the scope of interdisciplinarity (narrow or broad, i.e., what is being integrated), and we assessed interdisciplinarity within the modeled HDAs through (2) the types of interdisciplinary interaction (empirical or theoretical, i.e., how the integration is done). The former was determined by an inspection of the diversity of the journals in which the papers were published, and their subject areas, and as well as the diversity of the types of models. The latter was determined by inspecting the diversity of the HDAs found within the models (theoretical interdisciplinarity), and examining the diversity of the fisheries dimensions (human, economic, environmental) within the variables used to model the HDAs (empirical interdisciplinarity). It is important to emphasize that we assessed the interdisciplinarity of the sample as a whole (based on the aggregated empirical data we had collected), rather than looking at each individual model separately. We did not asses the types of goals because this was not the primary purpose of our study.
Limitations of the Applied Methodology
One limitation of the SLR approach, as with any keyword-based study, is that the choice of keywords is prone to human subjectivity and that relevant literature can be potentially excluded if the keywords are not present in the searchable fields, e.g., abstract, title, or keywords of the item. Also, the similar managerial assumptions introduced through the keyword search term “common fisheries policy” potentially limit the scope of the search for the incorporation of the HD into fisheries models in the European Union. Additionally, the number of publications reviewed is often much smaller than in, for example, computational approaches such as topic modeling (Syed and Weber, 2018).
Another limitation of the SLR approach is the exclusion of gray literature. Gray literature is not indexed in the same manner as scientific publications, and therefore cannot be sampled in the same way. On the other hand, gray literature does not undergo the same rigorous peer-review process as scientific journal publications, which gave us a good enough reason to exclude it and focus our interest on peer-reviewed scientific publications. We are aware that due to the limitations of this approach, relevant documents might have been excluded and are therefore absent from our sample. Our work reflects the academic contributions to the incorporation of HD into fisheries models, but not the fisheries science contributions as a whole (including modeling of stock assessments and advice) to this domain. However, since the aim of this study was to select a large sample of the literature in a transparent manner, rather than to identify all of the literature in the field, the methodological approach described above was considered sufficient.
Another limitation of the SLR approach is inherent to qualitative analysis and synthesis: it is an interpretative process, and the results can vary between human coders. Therefore, to ensure coding consistency, the coding was conducted by only one of the authors.
Interdisciplinarity is difficult to assess (Huutoniemi et al., 2010) and the approach applied here is therefore another limitation of this study. The measures used to assess interdisciplinarity (journal subject areas, model diversity, HD categories, and diversity of variables used to model HD) are indicators and thus not direct measures of interdisciplinarity because they do not measure actual integration. This is due to the fact that the exact form and degree of integration in interdisciplinary research are often difficult to identify within a publication if it is not made explicit (e.g., whether the theories underlying the model were integrated and which theories they were). However, we assume interdisciplinarity (and not multidisciplinarity) since various variables and data from HD and natural sciences were integrated into the models.
Results and Discussion
How Interdisciplinary Is the Field of Modeling the Human Dimension?
The Scopus search generated a total of 211 publications, out of which 131 were excluded based on the inclusion and exclusion criteria in Table 1. This left 80 publications that were eligible for further qualitative analysis. Within these 80 publications, we identified 31 papers as modeling an HDA, based on our coding criteria of the content analysis (see Appendix S4 in the Supplementary Material for a full list of these papers). These 31 articles had been published in 20 different journals, which were listed in eight different subject areas in Scopus (Table 2). While some of the subject areas can be considered relatively similar from a conceptual point of view (e.g., environmental sciences and agricultural and biological sciences), other subject areas were conceptually diverse and crossed the boundaries of broad intellectual areas (e.g., social science and computer science). At the same time, many of these journals were registered in more than one field (e.g., Marine Policy is listed in three fields, Land Economics is listed in two fields). This spread of journals and subject areas, together with the presence of the same journals in multiple fields, could indicate the potential for both narrow and broad interdisciplinarity in the modeling of the HD in fisheries. At the same time, it is interesting to note that, even though the models we analyzed were about HD, and one would expect these to be published mainly in journals in the field of social sciences, the most highly-represented subject field was environmental science, with social sciences being only half the size. This result is in line with the fact that fisheries science has been traditionally dominated by natural scientists (Link, 2010).
The journal with the highest frequency of appearance in the dataset was Marine Policy, accounting for almost one-third of the articles on modeling an HDA in fisheries. This is not surprising, considering that the journal describes its contributions as a “unique combination of analyses in the principal social science disciplines relevant to the formulation of marine policy” (Elsevier, 2018), while the main topics published by this journal are fisheries management, conservation, fishing gear, and models (Syed et al., 2018).
A total of 27 different model types were identified within the publications, where the model categorization showed that statistical models were the most common models (n = 14), followed by conceptual models (n = 8), simulation models (n = 7), and mathematical models (n = 5) (Table 3). The 27 different model types range from classic economics models (e.g., econometrics models) to conceptual frameworks (Table 3). As is the case for publication outlets and subject areas, this spread of model types could indicate the potential for both narrow and broad interdisciplinarity in the field being analyzed. The application of various modeling approaches could be a potential first step toward an integration of the HD into fisheries assessments (Schlüter et al., 2012). Almost one-fifth of the publications included in this analysis used a bioeconomic model. The greater use of these models is likely related to their long-term use in fisheries, dating back to Gordon (1954) and Clark (1973). It might also indicate the interdisciplinary practice of borrowing methods and tools from across the disciplines in an effort to address the needs dictated by the specific problem at hand (Huutoniemi et al., 2010). It is also possible that the uptake of models more suitable for modeling the HD, e.g., agent-based models (Schlüter et al., 2012), and social network analysis (Scott, 2017), is rather slow.
Is There a Gap Between the Human Dimension Aspects That Are Modeled and Those That Could Be Modeled?
A total of 20 different main HDAs (Table 4) were identified within the 31 publications. These aspects cover all three of the categories of topics relating to the HD described by Bennett et al. (2017), which could be taken as a sign of theoretical interdisciplinarity at the field level. However, the number of specific aspects that have been modeled is rather small compared with the wealth of HDAs that could be modeled. As stated in Syed et al. (2018), the HD in fisheries in particular, or in any similar social-ecological system in general, could be explored by addressing topics such as: “institutional aspects (enforcement and compliance, policy interactions etc.), social aspects (gender, religion/beliefs, welfare, social cohesion, social networks, education and learning, human agency, health, safety and security at sea, food security, perception, attitudes, social norms, compliance, mental models of various actors involved in fisheries etc.), economic aspects (poverty, innovation, distribution of benefits, spiritual, inspirational, and aesthetic services of fisheries etc.), political aspects (power structures, transparency etc.), and cultural aspects (traditional/local ecological knowledge, history, cultural dimensions, culinary choices, heritage, blue humanities, fisheries literacy etc.).” Note that this list is not exhaustive and the items are listed in random order.
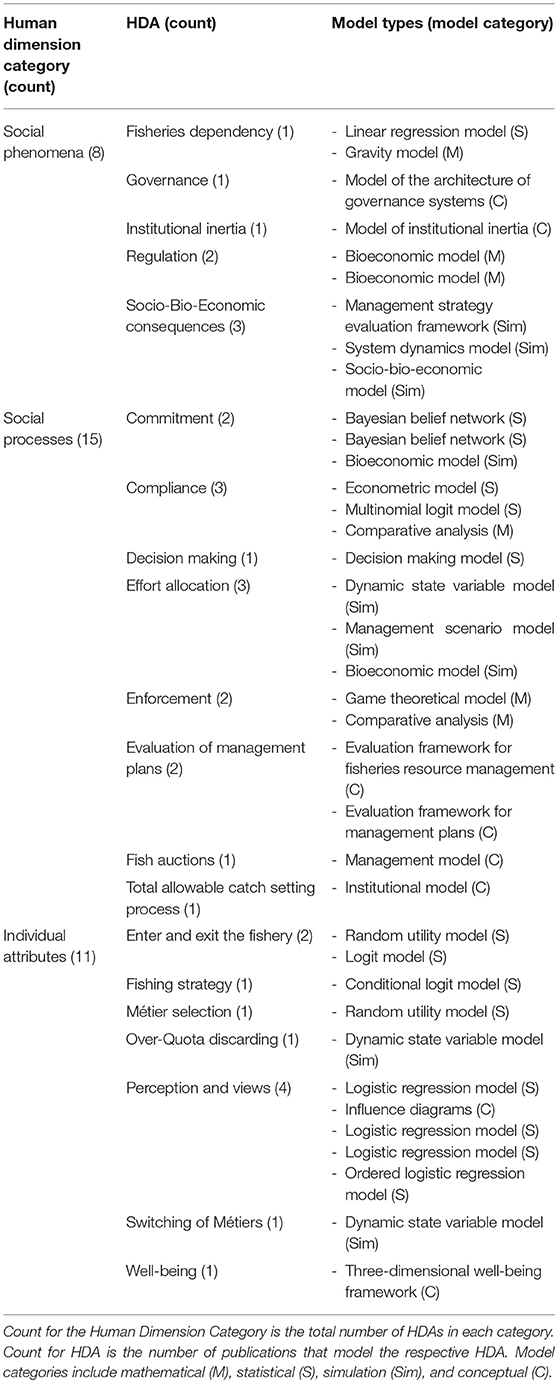
Table 4. List of human dimension aspect (HDAs) identified within the publications, mapped against the general human dimension topics of study proposed by Bennett et al. (2017) and the model types.
Compared to this list, the results for the 31 papers reviewed in this study reveal a significant gap between the HDAs that were modeled and the ones that could be modeled. However, considering the small sample size, this gap does not necessarily reflect the situation in the Common Fisheries Policy area.
A theory describes our understanding of the components and aspects of reality, and their interactions. Once developed, a theory guides modelers in their decisions regarding what elements, relationships, and processes to include into their models. It is therefore the case that a model itself and the generalizability of its results can be judged by the validity and quality of the theories incorporated (Raser, 1972). Moreover, when studying complex systems, a single theory taken in isolation is rarely sufficient (Orcutt et al., 1961). From this perspective, achieving theoretical interdisciplinarity is a pre-requisite for integrative theories and/or theories from more than one field, assuming that these theories are suitable for integration. The low number of HDAs that result from our systematic literature review may be due to a shortage of adequate theories, to a lack of appropriate HDA data, or to both.
Are Human Dimension Aspects Modeled in an Interdisciplinary Manner?
The 20 Level 1 HDAs were modeled using a total of 43 different Level 2 variables and 137 different Level 3 variables (see Appendices S5, S6 in the Supplementary Material). All visual representations of the HDAs are presented in Figure 3 and Appendix S7 (Supplementary Material). Perception and views has the most Level 2 variables. Fish auctions has the smallest number of Level 3 variables, with only three (Figure 3), whereas socio-bio-economic consequences has the largest number of Level 3 variables, with 37. Fish auctions also has the smallest number of variables overall, with a total of five across Level 2 and Level 3. Other HDAs with generally low numbers of Level 2 and Level 3 variables are fisheries dependency (n = 6) and decision making (n = 6). The majority of the HDAs have a total number of variables between 10 and 20. The HDA socio-bio-economic consequences has the largest number of variables overall, with a total of 41. This variety of Level 2 and Level 3 variables might indicate the existence of several theories around the same aspect of Level 1, something which contributes to theoretical interdisciplinarity of the field.
The number of aspects modeled and the variables assigned to each dimension are shown in Figure 2. A close inspection of this figure reveals that the proportion of each of the three fisheries dimensions changes with an increase in the depth of analysis. Thus, at Level 2, the count and usage of human dimension variables are higher, compared to the economic variables. Whereas, at Level 3, human dimension variables' usage is much lower compared to economic variables' usage. This diversification might indicate an empirical interdisciplinary nature to the modeling of the HD. However, it might also indicate a lack of suitable operationalisation of human dimension variables and, consequently, a lack of suitable data to use in modeling. At the same time, this highlights how the HD can be modeled using economic and environmental variables, and the entanglement of the dimensions.
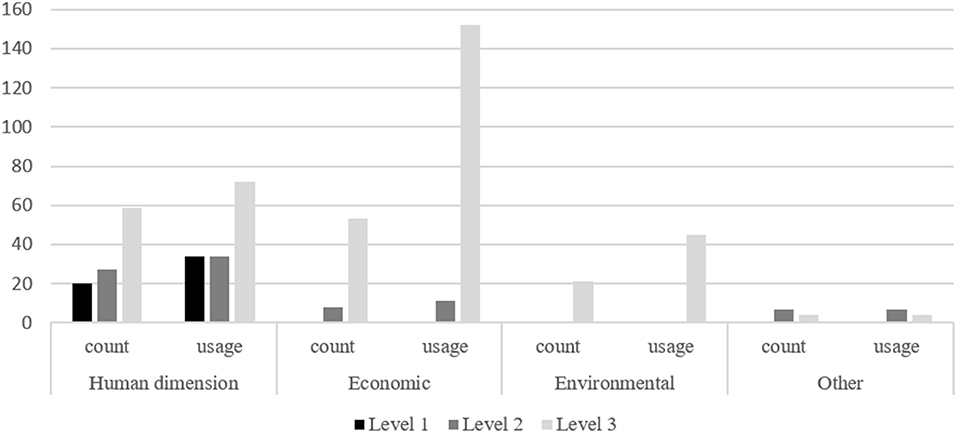
Figure 2. Occurrence and usage of variables for all three levels, from the four different dimensions human, economic, environmental, and other. Count indicates the number of different variables identified for each level and each dimension. Usage indicates the number of times that variables from each dimension were used. Other includes variables that could not be categorized within the three dimensions, human, economic, and environmental, such as time.
Only one HDA, governance, was modeled entirely through human dimension variables on all levels. Fish auctions was the only HDA where all Level 2 and Level 3 factors were economic (Figure 3). The two HDAs fishing strategy and institutional inertia were modeled using Level 2 and Level 3 variables from only two different dimensions, whereas fishing strategy was modeled using factors from the economic and environmental dimensions, and institutional inertia was modeled using factors from the economic and human dimensions (see Appendix S7 in the Supplementary Material). Thirteen HDAs were modeled using Level 2 and Level 3 variables from three different dimensions (n = 12) and five HDAs were modeled using Level 2 and Level 3 variables from all dimensions. These were: socio-bio-economic consequences, compliance, evaluation of management plans, perception and views, and TAC setting process.
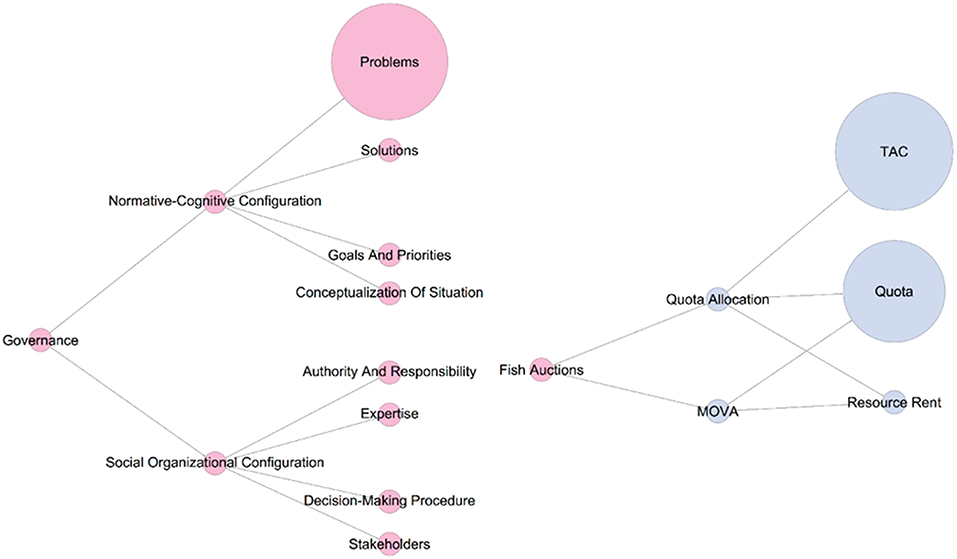
Figure 3. A visual representation of the Human Dimension Aspects (HDAs) governance (left) and fish auctions (right) and the Level 2 and Level 3 variables that were used to model these HDAs. The size of each node represents the relative importance of the variable (i.e., the number of publications using it) and the color indicates its dimension (pink: human; blue: economic; green: environmental; white: other/more than one dimension). The position of each node (left—middle—right) indicates its level (Level 1—Level 2—Level 3).
Overall, variables from the economic dimension were used the most often (Figure 2); in particular, cost (n = 13), effort (n = 13), and price (n = 12) were the most used economic variables in Level 3. The variables from the human dimension that were used most often in Level 3 were demography (n = 4), regulation (n = 4), and employment (n = 3), whereas the most frequently used variables from the environmental dimension in Level 3 were stock (n = 13), area (n = 6), and fishing mortality (n = 4). This study suggests that HDAs are modeled using mainly economic and partly environmental variables, which represents the data typically available for fisheries assessments. Some of the HDAs, such as governance, might be very difficult (if not maybe impossible) to be expressed in numerical terms.
Is There a Relation Between the Modeled HDAs and the Modeling Approaches?
The HD categories “social phenomena” and “social processes” contain modeling approaches from all four model categories (mathematical, statistical, simulation, and conceptual), while the HD category “individual attribute” contains only statistical, simulation, and conceptual models (Table 4). This indicates that the modeling of HD categories is independent from the modeling approach and that each HD category can be captured through a range of mathematical, statistical, simulation and conceptual models. The lack of mathematical models in the individual attributes HD category is likely due to our small sample size.
Most of the HDAs were modeled using only one of the model categories, such as the HDAs regulation (only mathematical), effort allocation (only simulation), evaluation of management plans (only conceptual), and enter and exit the fishery (only statistical). In some cases, an HDA was modeled using two different modeling categories, e.g., fisheries dependency has been modeled in both a mathematical and a statistical way. The same can be found for compliance. Commitment was modeled using statistical models and a simulation model. Perception and views was modeled using statistical models and a conceptual model (Table 4).
In the modeling categories, some differences in the interdisciplinarity of how HDAs were modeled can be oberserved by inspecting the diversity of the variables used (see Table 4 and Figures in Appendix S7 in the Supplementary Material). Mathematical models commonly included more economic variables, as apparent in enforcement and regulation. Whereas, most of the statistical models included variables from all three dimension (human, economic, environmental), and appeared to allow for a more interdisciplinary modeling approach. Some of the statistical models used more human dimension variables than others, such as commitment, while other statistical models included more economic variables, such as fishing strategy and enter and exit the fishery. Simulation models included mostly economic variables but were still interdisciplinary as they included variables from all dimension, even if only few variables from the human dimension were present. Conceptual models allowed for the inclusion of most social variables but were the least interdisciplinary model category as they often included variables from only one dimension. For example, governance contained only human dimension variables and fish auctions contained only economic variables, while institutional inertia contained only two dimensions, which made these conceptual HDA models the least interdisciplinary,
Regarding the different model types used to model the HDAs, only few model types were used for more than one HDA. For example, bioeconomic models were used to model three different HDAs, i.e., regulation, commitment, and effort allocation; while random utility models were used to model the HDAs enter and exit of the fishery and métier selection.
Overall, our study shows how HDAs can be explored through all four modeling approaches, from mathematical, statistical, simulation, to conceptual, independent from what HDA category the HDA is. It also highlights how the same model type (e.g., bioeconomic model) can be used to model different HDAs from different HD categories. In addition, whether a model is interdisciplinary or not appears to be independent of the HD category and modeling approach, as interdisciplinarity (based on the diversity of variables used from the different dimensions, see Figures in Appendix S7 in the Supplementary Material) can be found to different degrees within each of the mathematical, statistical, simulation, and conceptual model categories, as well as the different model types.
How to Advance the Interdisciplinarity of the Field
As a first step to advance the interdisciplinarity of the field, we suggest a protocol based on Huutoniemi et al. (2010) that succinctly describes the elements necessary for assessing various interdisciplinary typologies, shown in Table 5. Such a protocol could guide scientists on how to take an interdisciplinary approach during model development and implementation. It is also paramount for the advancement of the field that HD models are reproducible. Many of the descriptions of models in published articles are incomplete, which makes it impossible to re-implement them or replicate their results (Railsback and Grimm, 2012). As we have ourselves encountered when carrying out this study, model descriptions are often “a wordy mixture of factual descriptions and lengthy justifications, explanations, and discussions of all kinds” (Railsback and Grimm, 2012, p. 36). Therefore, we also suggest that this protocol is used as a documentation tool in order to help modelers to express the interdisciplinary characteristics of their models clearly. This would also aid model communication, in-depth model comprehension, model assessment, model replication, model comparison, theory building, and code generation (Müller et al., 2014).
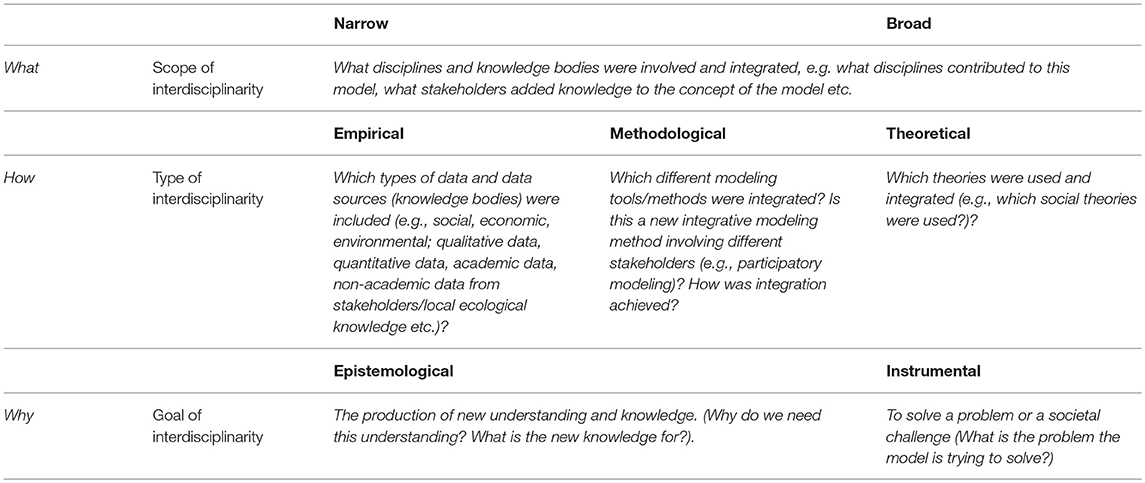
Table 5. An overview of the protocol for assessing the interdisciplinarity of models, based on Huutoniemi et al. (2010).
Social issues are often complex and understanding these issues from SES perspective will require interdisciplinary efforts from the natural and social sciences, as well as the humanities (Urquhart et al., 2014). This assertion is backed by this empirical study, which brings evidence on how entangled the HD is when viewing fisheries as SES. Multi- and interdisciplinarity would entail the transfer of knowledge, tools, and methods from a multitude of disciplines into the field of fisheries science, making it possible to integrate various data inputs (e.g., quantitative and qualitative data). The outlined empirical results in our study demonstrate through which model categories and model types the HD was addressed. These approaches could be expanded through other existing methods, such as agent-based models, systems analysis, and social network analysis from domains ranging from political science to business organization. Such methods could be integrated into fisheries science and used to study societies, social interactions, and people's behavior in fisheries (Libre et al., 2015; Scott, 2017).
Through an expansion of current practices, a wider range of the HDAs could be considered in fisheries models to better reflect the diversity of the HD. This endeavor could be fostered further through the inclusion of scientists from the social sciences and the humanities right from the start of a project (Criddle, 2016). In this way, they can contribute to the formulation of the research questions that ought to be answered by a model, which could lead to a more diversified investigation of the HD.
The challenges of performing interdisciplinary research are not new, as they have been already identified 20 years ago (see for example Volume 2, Issue 4, 1999 of the journal Ecosystems). Thus, in order to address the issues identified by the above analysis, it might be that fisheries science will require new types of experts, besides biologists, mathematicians, and statisticians: (1) scientists from the social sciences and the humanities; (2) scientists with interdisciplinary backgrounds who can address fisheries from a more holistic perspective and apply the concept of SES to multi- and interdisciplinary fisheries workgroups and research; and (3) modelers with the latest skillset who are trained to use tools that can reflect fisheries as SES, and include the HD in an interdisciplinary way. This would potentially lead to the rise and also the recognition of a new kind of natural resources expert: interdisciplinary individuals with the flexibility required to move between fields and explore various SES, e.g., sustainability science (Haider et al., 2018), conservation science, and complexity science.
Researchers putting aside their differences and finding better ways to communicate could support the practice of interdisciplinary science and disciplinary cross-fertilization (Arlinghaus et al., 2014), whilst the interdisciplinary development of conceptual models could support communication between social and natural scientists (Hall-Arber et al., 2009). Some things in the culture of science might have to change, e.g., arrogances and the way we speak to each other, but we also need to rethink our assumptions, values, and institutional structures (Degnbol et al., 2006). Researchers from cross-disciplinary research programs, as well as innovative graduate training programs, would have to become more involved. In addition, interdisciplinary career choices would have to be rewarded instead of generating a fear of risking one's career (Rhoten and Parker, 2004; Fischer et al., 2012).
Besides experts and scientists from different disciplines, the insight of stakeholders should also be taken into account. Stakeholders and practitioners, such as management authorities and non-governmental organizations, can contribute to the modeling process through co-creation (Santiago et al., 2015; Wood et al., 2015). Co-creation could highlight the importance of HD components and lead to assurances that managers and policy makers will take the behavior of individuals and organizations into consideration within their fishing communities. This would make models of the HD more relevant for societal challenges, management and decision making, while contributing to the scientific knowledge production and supporting local and global policies and sustainability goals, such as the EU's Common Fisheries Policy and the United Nations' Sustainable Development Goals (United Nations, 2015).
Furthermore, with this study we wish to stimulate the discussion on how best to model the HD of SES. Based on our empirical results, the HD is largely modeled using statistical models, while all model types are using mostly economic and environmental variables. One could argue that the field of HD modeling needs to diversify modeling approaches and requires more operationalisable social theories and more data relevant to these theories. At the same time, using more easily available economic and environmental data is a more practical short-term approach. In contrast, some argue for extreme caution in modeling the HD, and social phenomena in general (ní Aodha and Edmonds, 2017). These decisions will likely be made on an individual level, but we hope that researchers from all fields can engage in these discussions and share their experiences as well as the reasons for the approaches they have taken and their lessons learned.
Conclusions
This study identifies a variety of HDAs that have been investigated in the context of fisheries models. HDAs can be modeled in an interdisciplinary manner through a wide diversity of modeling types, and this study can potentially provide some useful guidance for those seeking to include HDA into fisheries models. However, there is broad potential for the expansion of the HD in fisheries models, as there is potential for diversification of the types of models. This expansion is important in order to increase our understanding of fisheries systems in general, and to better reflect the interdisciplinarity of the HD field in order to understand and support sustainable fisheries.
In the support of modeling the HD in a SES context, interdisciplinary approaches are required. Such efforts need to focus on several aspects, including: acknowledging that exploring the HD requires interdisciplinarity; early involvement of all relevant disciplines and stakeholders in model development through co-creation; expansion and improved development and integration of tools for the modeling of HDAs; the formulation of operationalisable theories and the collection and inclusion of more data from the HD. To further improve and advance the interdisciplinarity of HD modeling in the long term, model transparency, documentation, and communication will be key. A model publication should be easy for the reader to understand and follow, and it should make the HDAs and levels of interdisciplinarity explicit. Clear model descriptions will enable interested readers and modelers to understand how interdisciplinarity and HD modeling was achieved, thus facilitating model uptake and re-use by scientists, managers, and policy makers.
Author Contributions
CW collected and analyzed the data. CW and MB interpreted the data. CW, MB, and MA wrote the manuscript.
Funding
This research was funded by the project SAF21—Social science aspects of fisheries for the 21st century. This is a project financed under the EU Horizon 2020 Marie Skłodowska-Curie (MSC) ITN-ETN Program; project number: 642080.
Conflict of Interest Statement
The authors declare that the research was conducted in the absence of any commercial or financial relationships that could be construed as a potential conflict of interest.
Acknowledgments
We wish to thank the reviewers for their constructive comments that helped improve earlier versions of this paper.
Supplementary Material
The Supplementary Material for this article can be found online at: https://www.frontiersin.org/articles/10.3389/fmars.2019.00369/full#supplementary-material
The Scopus search string (Appendix S1), the PRISMA flow diagram (Appendix S2), a description of the methodological approach for creating the visualizations of the HDAs (Appendix S3), a table listing all publications included in analysis and synthesis phase (Appendix S4), a table for all Level 2 variables (Appendix S5), a table for all Level 3 variables (Appendix S6), and all remaining visualizations (Appendix S7) are available in the Supplementary Material.
References
Arlinghaus, R., Hunt, L. M., Post, J. R., and Allen, M. S. (2014). “Not fish, not meat: some guidance on how to study fisheries from an interdisciplinary perspective,” in Future of Fisheries: Perspectives for Emerging Professionals, eds W. Taylor, A. Lynch, and N. Leonard (Bethesda, MD: American Fisheries Society), 223–230.
Bastian, M., Heymann, S., and Jacomy, M. (2009). “Gephi: an open source software for exploring and manipulating networks,” in International AAAI Conference on Weblogs and Social Media (San Jose, CA), 361–362.
Bennett, N. J., Roth, R., Klain, S. C., Chan, K., Christie, P., Clark, D. A., et al. (2017). Conservation social science: understanding and integrating human dimensions to improve conservation. Biol. Conserv. 205, 93–108. doi: 10.1016/j.biocon.2016.10.006
Berkes, F. (2011). “Restoring unity,” in World Fisheries, eds R. E. Omner, R. I. Perry, K. Cochrane, and P. Cury (Oxford: Wiley-Blackwell, 9–28.
Charnley, S., Carothers, C., Satterfield, T., Levine, A., Poe, M. R., Norman, K., et al. (2017). Evaluating the best available social science for natural resource management decision-making. Environ. Sci. Policy. 73, 80–88. doi: 10.1016/j.envsci.2017.04.002
Chiu, C. Y., Kwan, L. Y.-Y., and Liou, S. (2013). Culturally motivated challenges to innovations in integrative research: theory and solutions. Social Issues Policy Rev. 7, 149–172. doi: 10.1111/j.1751-2409.2012.01046.x
Clark, C. W. (1973). The economics of overexploitation. Science 181, 630–634. doi: 10.1126/science.181.4100.630
Criddle, K. (2016). MSEAS 2016: Understanding Marine Socio-Ecological Systems: Including the Human Dimension in Integrated Ecosystem Assessments. PICES Press.
Degnbol, P., Gislason, H., Hanna, S., Jentoft, S., Raakjær Nielsen, J., Sverdrup-Jensen, S., et al. (2006). Painting the floor with a hammer: technical fixes in fisheries management. Marine Policy 30, 534–543. doi: 10.1016/j.marpol.2005.07.002
Elsevier (2018). Marine Policy. Retrieved from https://www.journals.elsevier.com/marine-policy (accessed October 11, 2018).
FAO Fishery Resources Division (1999). Indicators for Sustainable Development of Marine Capture Fisheries. Rome: FAO.
Fischer, E. V., Mackey, K. R. M., Cusack, D. F., DeSantis, L. R. G., Hartzell-Nichols, L., Lutz, J. A., et al. (2012). Is pretenure interdisciplinary research a career risk? Eos Trans. Am. Geophys. Union 93, 311–312. doi: 10.1029/2012EO320004
Freire, J., and Garcia-Allut, A. (2000). Socioeconomic and biological causes of management failures in European artisanal fisheries: the case of Galicia (NW Spain). Marine Policy 24, 375–384. doi: 10.1016/S0308-597X(00)00013-0
Gordon, H. S. (1954). The economic theory of a common-property resource: the fishery. J. Polit. Econ. 62, 124–142. doi: 10.1086/257497
Haapasaari, P., Kulmala, S., and Kuikka, S. (2012). Growing into interdisciplinarity: how to converge biology, economics, and social science in fisheries research? Ecol. Soc. 17:art6. doi: 10.5751/ES-04503-170106
Haider, L. J., Hentati-Sundberg, J., Giusti, M., Goodness, J., Hamann, M., Masterson, V. A., et al. (2018). The undisciplinary journey: early-career perspectives in sustainability science. Sustain. Sci. 13, 191–204. doi: 10.1007/s11625-017-0445-1
Hainey, T., Connolly, T. M., Boyle, E. A., Wilson, A., and Razak, A. (2016). A systematic literature review of games-based learning empirical evidence in primary education. Comp. Educ. 102, 202–223. doi: 10.1016/j.compedu.2016.09.001
Hall-Arber, M., Pomeroy, C., and Conway, F. (2009). Figuring out the human dimensions of fisheries: illuminating models. Marine Coast. Fish. 1, 300–314. doi: 10.1577/C09-006.1
Hanneman, R. A. (1995). Simulation modeling and theoretical analysis in sociology. Sociol. Perspect. 38, 457–462. doi: 10.2307/1389267
Hatchard, J. L., and Gray, T. S. (2014). From RACs to advisory councils: lessons from North Sea discourse for the 2014 reform of the European Common Fisheries Policy. Marine Policy 47, 87–93. doi: 10.1016/j.marpol.2014.02.015
Heemskerk, M., Wilson, K., and Pavao-Zuckerman, M. (2003). Conceptual models as tools for communication across disciplines. Conserv. Ecol. 7:8. doi: 10.5751/ES-00554-070308
Hicks, C. C., Levine, A., Agrawal, A., Basurto, X., Breslow, S. J., Carothers, C., et al. (2016). Engage key social concepts for sustainability. Science 352, 38–40. doi: 10.1126/science.aad4977
Huutoniemi, K., Klein, J. T., Bruun, H., and Hukkinen, J. (2010). Analyzing interdisciplinarity: typology and indicators. Res. Policy 39, 79–88. doi: 10.1016/j.respol.2009.09.011
Jarić, I., Cvijanović, G., Knežević-Jarić, J., and Lenhardt, M. (2012). Trends in fisheries science from 2000 to 2009: a bibliometric study. Rev. Fish. Sci. 20, 70–79. doi: 10.1080/10641262.2012.659775
Johnson, B. (2007). Educational Research : Quantitative, Qualitative, and Mixed Approaches. London: SAGE Publishers.
Kitchenham, B., Pearl Brereton, O., Budgen, D., Turner, M., Bailey, J., and Linkman, S. (2009). Systematic literature reviews in software engineering – a systematic literature review. Inform. Softw. Technol. 51, 7–15. doi: 10.1016/j.infsof.2008.09.009
Krippendorff, K. (2013). Content Analysis: An Introduction to Its Methodology, 3rd Edn. Thousand Oaks, CA: SAGE Publications.
Leenhardt, P., Teneva, L., Kininmonth, S., Darling, E., Cooley, S., and Claudet, J. (2015). Challenges, insights and perspectives associated with using social-ecological science for marine conservation. Ocean Coast. Manag. 115, 49–60. doi: 10.1016/j.ocecoaman.2015.04.018
Levin, S., Xepapadeas, T., Crépin, A.-S., Norberg, J., de Zeeuw, A., Folke, C., et al. (2012). Social-ecological systems as complex adaptive systems: modeling and policy implications. Environ. Dev. Econ. 18, 111–132. doi: 10.1017/S1355770X12000460
Libre, S. V. D., van Voorn, G. A. K., ten Broeke, G. A., Bailey, M., Berentsen, P., and Bush, S. R. (2015). Effects of social factors on fishing effort: the case of the Philippine tuna purse seine fishery. Fish. Res. 172, 250–260. doi: 10.1016/j.fishres.2015.07.033
Link, J. S. (2010). Ecosystem-Based Fisheries Management: Confronting Tradeoffs. Cambridge: Cambridge University Press.
Liquete, C., Piroddi, C., Drakou, E. G., Gurney, L., Katsanevakis, S., Charef, A., et al. (2013). Current status and future prospects for the assessment of marine and coastal ecosystem services: a systematic review. PLoS ONE 8:e67737. doi: 10.1371/journal.pone.0067737
Lofland, J., Snow, D., Anderson, L., and Lofland, L. H. (2006). Analyzing Social Setting. A Guide to Qualitative Observation and Analysis, 4th Edn. Belmon, WA: Wadsworth Cengage Learning.
Maier, F., Meyer, M., and Steinbereithner, M. (2016). Nonprofit Organizations becoming business-like. Nonprofit Volunt. Sect. Q. 45, 64–86. doi: 10.1177/0899764014561796
Marshall, K. N., Levin, P. S., Essington, T. E., Koehn, L. E., Anderson, L. G., Bundy, A., et al. (2018). Ecosystem-based fisheries management for social-ecological systems: renewing the focus in the united states with next generation fishery ecosystem plans. Conserv. Lett. 11:e12367. doi: 10.1111/conl.12367
Miles, M. B., and Huberman, A. M. (1994). Qualitative Data Analysis: An Expanded Sourcebook. Los Angeles, CA: Sage Publications.
Moher, D., Liberati, A., Tetzlaff, J., Altman, D. G., and Group, T. P. (2009). Preferred reporting items for systematic reviews and meta-analyses: the PRISMA statement. PLoS Med. 6:e1000097. doi: 10.1371/journal.pmed.1000097
Müller, B., Balbi, S., Buchmann, C. M., de Sousa, L., Dressler, G., Groeneveld, J., et al. (2014). Standardised and transparent model descriptions for agent-based models: current status and prospects. Environ. Model. Softw. 55, 156–163. doi: 10.1016/j.envsoft.2014.01.029
ní Aodha, L., and Edmonds, B. (2017). “Some pitfalls to beware when applying models to issues of policy relevance,” in Simulating Social Complexity, Understanding Complex Systems, eds B. Edmonds and R. Meyer (Cham: Springer, 801–822.
OECD (2007). Structural Change in Fisheries Dealing with the Human Dimension. Paris: OECD Publishing.
Orcutt, G. H., Greenberger, M., Korbel, J., and Rivlin, A. M. (1961). Microanalysis of Socioeconomic Systems: A Simulation Study. New York, NY: Harper and Row.
Österblom, H., Sissenwine, M., Symes, D., Kadin, M., Daw, T., and Folke, C. (2011). Incentives, social-ecological feedbacks and European fisheries. Marine Policy 35, 568–574. doi: 10.1016/j.marpol.2011.01.018
Ostrom, E. (2009). A general framework for analyzing sustainability of social-ecological systems. Science 325, 419–422. doi: 10.1126/science.1172133
Pullin, A. S., and Stewart, G. B. (2006). Guidelines for systematic review in conservation and environmental management. Conserv. Biol. 20, 1647–1656. doi: 10.1111/j.1523-1739.2006.00485.x,
Railsback, S. F., and Grimm, V. (2012). Agent-Based and Individual-Based Modeling: A Practical Introduction. Oxford: Princeton University Press.
Rhoten, D., and Parker, A. (2004). Risks and rewards of an interdiciplinary research path. Science 306:2046. doi: 10.1126/science.1103628
Rissman, A. R., and Gillon, S. (2017). Where are ecology and biodiversity in social-ecological systems research? A review of research methods and applied recommendations. Conserv. Lett. 10, 86–93. doi: 10.1111/conl.12250
Santiago, J. L., Ballesteros, M. A., Chapela, R., Silva, C., Nielsen, K. N., Rangel, M., et al. (2015). Is Europe ready for a results-based approach to fisheries management? The voice of stakeholders. Marine Policy 56, 86–97. doi: 10.1016/j.marpol.2015.02.006
Schlüter, M., Mcallister, R. R. J., Arlinghaus, R., Bunnefeld, N., Eisenack, K., Hölker, F., et al. (2012). New horizons for managing the environment: a review of coupled social-ecological systems modeling. Nat. Res. Model. 25, 219–272. doi: 10.1111/j.1939-7445.2011.00108.x
Spalding, A. K., Biedenweg, K., Hettinger, A., and Nelson, M. P. (2017). Demystifying the human dimension of ecological research. Front. Ecol. Environ. 15, 119–119. doi: 10.1002/fee.1476
Starfield, A. M., and Jarre, A. (2011). “Interdisciplinary modeling for an ecosystem approach to management in marine social-ecological systems,” in World Fisheries: A Social-Ecological Analysis, eds R. E. Ommer, R. I. Perry, K. Cochrane, and P. Cury (Oxford: Wiley-Blackwell, 105–119.
Stephenson, R. L., Paul, S., Wiber, M., Angel, E., Benson, A. J., Charles, A., et al. (2018). Evaluating and implementing social-ecological systems: a comprehensive approach to sustainable fisheries. Fish Fish. 19, 853–873. doi: 10.1111/faf.12296
Syed, S., Borit, M., and Spruit, M. (2018). Narrow lenses for capturing the complexity of fisheries: a topic analysis of fisheries science from 1990 to 2016. Fish Fish. 19, 643–661. doi: 10.1111/faf.12280
Syed, S., and Weber, C. T. (2018). Using machine learning to uncover latent research topics in fishery models. Rev. Fish. Sci. Aquacult. 26, 319–336. doi: 10.1080/23308249.2017.1416331
Symes, D., and Phillipson, J. (2009). Whatever became of social objectives in fisheries policy? Fish. Res. 95, 1–5. doi: 10.1016/j.fishres.2008.08.001
Tress, B., Tress, G., and Fry, G. (2005). “Defining concepts and the process of knowledge production in integrative research,” in From Landscape Research to Landscape Planning: Aspects of Integration, Education and Application, eds B. Tress, G. Tress, G. Fry, and P. Opdam (Wageningen: Springer), 13–26.
United Nations (2015). Transforming Our World: The 2030 Agenda for Sustainable Development. UN Doc. A/70/L.1 of 18, United Nations General Assembly.
Urquhart, J., Acott, T. G., Symes, D., and Zhao, M. (eds.). (2014). Social Issues in Sustainable Fisheries Management, Vol. 9:355. Dordrecht: Springer Netherlands.
Weitzen, S., Lapane, K. L., Toledano, A. Y., Hume, A. L., and Mor, V. (2004). Principles for modeling propensity scores in medical research: a systematic literature review. Pharmacoepidemiol. Drug Saf. 13, 841–853. doi: 10.1002/pds.969
Keywords: social science, integration, social model, reproducibility, model documentation
Citation: Weber CT, Borit M and Aschan M (2019) An Interdisciplinary Insight Into the Human Dimension in Fisheries Models. A Systematic Literature Review in a European Union Context. Front. Mar. Sci. 6:369. doi: 10.3389/fmars.2019.00369
Received: 13 July 2018; Accepted: 14 June 2019;
Published: 02 July 2019.
Edited by:
Simone Libralato, Istituto Nazionale di Oceanografia e di Geofisica Sperimentale (OGS), ItalyReviewed by:
Alida Bundy, Bedford Institute of Oceanography (BIO), CanadaSebastian Villasante, University of Santiago de Compostela, Spain
Copyright © 2019 Weber, Borit and Aschan. This is an open-access article distributed under the terms of the Creative Commons Attribution License (CC BY). The use, distribution or reproduction in other forums is permitted, provided the original author(s) and the copyright owner(s) are credited and that the original publication in this journal is cited, in accordance with accepted academic practice. No use, distribution or reproduction is permitted which does not comply with these terms.
*Correspondence: Charlotte Teresa Weber, charlotte.t.weber@uit.no