- 1Atlantic Oceanographic and Meteorological Laboratory, National Oceanic and Atmospheric Administration, Miami, FL, United States
- 2Centre for Ocean and Atmospheric Sciences, School of Environmental Sciences, University of East Anglia, Norwich, United Kingdom
- 3Norwegian Research Centre (NORCE), Bergen, Norway
- 4Pacific Marine Environmental Laboratory, National Oceanic and Atmospheric Administration, Seattle, WA, United States
- 5Research and Development Center for Global Change, Japan Agency for Marine-Earth Science and Technology, Yokosuka, Japan
- 6Geophysical Institute, University of Bergen, Bergen, Norway
- 7National Center for Atmospheric Research, Boulder, CO, United States
- 8Commonwealth Scientific and Industrial Research Organisation, Oceans and Atmosphere, Hobart, TAS, Australia
- 9Cooperative Institute for Research in Environmental Sciences, University of Colorado, Boulder, Boulder, CO, United States
- 10Cooperative Institute for Marine and Atmospheric Studies, University of Miami, Miami, FL, United States
- 11Leibniz Institute for Baltic Sea Research Warnemünde, Rostock, Germany
- 12Instituto de Oceanografía y Cambio Global, Universidad de Las Palmas de Gran Canaria, Las Palmas de Gran Canaria, Spain
- 13Technological Research Institute, University of Santiago de Compostela, Santiago de Compostela, Spain
- 14Ocean Observing and Monitoring Division (NOAA), Silver Spring, MD, United States
- 15Joint Institute for the Study of the Atmosphere and Ocean, NOAA/PMEL/SDIG, University of Washington, Seattle, WA, United States
- 16National Institute of Water and Atmospheric Research, Dunedin, New Zealand
- 17International Ocean Carbon Coordination Project, Institute of Oceanology of Polish Academy of Sciences, Sopot, Poland
- 18Alfred Wegener Institute, Helmholtz Centre for Polar and Marine Research, Bremerhaven, Germany
- 19Japan Meteorological Agency, Meteorological Research Institute, Tsukuba, Japan
- 20Bermuda Institute of Ocean Sciences, St. George’s, Bermuda
- 21CNRS, IRD, MNHN, LOCEAN/IPSL Laboratory, Sorbonne Universités (UPMC, Univ Paris 06), Paris, France
- 22National Institute for Environmental Studies, Tsukuba, Japan
- 23Lamont-Doherty Earth Observatory, Columbia University, Palisades, NY, United States
- 24GEOMAR – Helmholtz Centre for Ocean Research Kiel, Kiel, Germany
- 25College of Life and Environmental Sciences, University of Exeter, Exeter, United Kingdom
The Surface Ocean CO2 NETwork (SOCONET) and atmospheric Marine Boundary Layer (MBL) CO2 measurements from ships and buoys focus on the operational aspects of measurements of CO2 in both the ocean surface and atmospheric MBLs. The goal is to provide accurate pCO2 data to within 2 micro atmosphere (μatm) for surface ocean and 0.2 parts per million (ppm) for MBL measurements following rigorous best practices, calibration and intercomparison procedures. Platforms and data will be tracked in near real-time and final quality-controlled data will be provided to the community within a year. The network, involving partners worldwide, will aid in production of important products such as maps of monthly resolved surface ocean CO2 and air-sea CO2 flux measurements. These products and other derivatives using surface ocean and MBL CO2 data, such as surface ocean pH maps and MBL CO2 maps, will be of high value for policy assessments and socio-economic decisions regarding the role of the ocean in sequestering anthropogenic CO2 and how this uptake is impacting ocean health by ocean acidification. SOCONET has an open ocean emphasis but will work with regional (coastal) networks. It will liaise with intergovernmental science organizations such as Global Atmosphere Watch (GAW), and the joint committee for and ocean and marine meteorology (JCOMM). Here we describe the details of this emerging network and its proposed operations and practices.
Introduction
Rising carbon dioxide (CO2) levels in the atmosphere and ocean are major issues of our time. Historically, the main focus in carbon cycle research has been on understanding the flow and partitioning of the excess carbon dioxide in the earth system components of atmosphere, ocean and terrestrial biosphere. Revelle and Suess (1957) stated “Human beings are now carrying out a large scale geophysical experiment of a kind that could not have happened in the past nor be reproduced in the future.” Roger Revelle subsequently wrote that “People’s attitude toward the rise of CO2 should probably contain more curiosity than apprehension.” (Weart, 2008). The basic understanding of processes and impacts remains a priority in carbon cycle research but concerns and societal implications of the impacts of rising CO2 have surpassed mere curiosity. Increasing emphasis in carbon cycle research is placed on monitoring and quantifying the sources and sinks of atmospheric CO2, and the interplay between the anthropogenic CO2, that is, CO2 released by human activities such as fossil fuel burning and land-use changes, and the natural carbon cycle. This requires a systematic and sustained observational approach, well served by a closely coordinated network. The ocean is a significant sink of anthropogenic CO2 capturing about 25% of the anthropogenic carbon from 1870–2017 (Le Quéré et al., 2018). Once sequestered by the ocean, the retention time is on the order of centuries to millennia, compared to decades for terrestrial systems. The uptake of CO2 by the ocean is thus a critical element in understanding carbon dynamics and future trajectories of atmospheric CO2 growth.
Accurate measurements of CO2 concentrations in the surface ocean and atmospheric marine boundary layer (MBL) are critical factors to quantify the air-sea flux of CO2, along with the forcing function, called the gas transfer velocity, k. The air-sea CO2 flux, FCO2 [mol m-2 yr-1] is commonly expressed in terms of a bulk formulation as:
where k [m yr-1] is parameterized with wind (Wanninkhof, 2014), s is the solubility [mol m-3 atm-1], pCO2w is the partial pressure of CO2 in water [atm], pCO2a is the partial pressure of CO2 in air [atm], and ΔpCO2 is the difference. The units for k, s, and pCO2 are often reported as cm hr-1, mol l-1 atm-1, and μatm, respectively, and appropriate conversions need to be applied. The quantities measured are the mole fractions of CO2 in water, xCO2w, and air, xCO2a, and these are converted to partial pressure with knowledge of the total pressure and water vapor pressure (Pierrot et al., 2009). While ΔpCO2 over the open ocean can vary in time and space by about ± 100 μatm, the average disequilibrium needed to sequester the current annual ocean uptake of 2.5 billion tons of anthropogenic carbon (2.5 Pg C yr-1) (Le Quéré et al., 2018) is only 7–14 μatm, requiring accurate measurements of pCO2w and pCO2a with high spatiotemporal resolution. Due to the small average disequilibrium, measurements must be accurate. Bias, in particular, can be a major issue and thus well-calibrated measurements are a must. Of note is that in Eq. 1 the concentrations right at the interface are of relevance. The measurements, typically at 0.2–8 m depth and 1–20 m height, need to be corrected to surface conditions requiring adjustments for temperature, pressure, and chemical effects. The corrections are largest and most uncertain on the water-side of the interface.
The sequestration of anthropogenic CO2 emissions by the ocean is of benefit as it curtails increasing atmospheric CO2 level and its associated greenhouse effect, but the corresponding CO2 increase in ocean surface waters also leads to ocean acidification (OA), which is detrimental to many marine organisms. Knowledge of the rate of CO2 uptake and changes thereof are of importance for socio-economic assessments related to the fate of anthropogenic CO2 and to ocean health.
Systematic measurement of atmospheric CO2 concentrations began in the late 1950s (Keeling, 1958) to investigate the long-term atmospheric trend of this important greenhouse gas. The discovery of seasonal variability, resulting from terrestrial biosphere CO2 uptake and release, prompted a small global network of measurements to assess the global distribution of the seasonal and long-term features in CO2 (Keeling, 2008). As such, initial CO2 measurements were made from locations where well-mixed MBL air could be sampled, usually coastal or island sites with prevailing onshore winds, so that the data were representative of the regional background CO2 concentration, and not unduly influenced by localized sources and sinks.
Today, there are more than 100 sites where atmospheric scientists make sustained high-accuracy measurements of atmospheric CO2. However, the open ocean MBL remains undersampled. Many of these oceanic regions are visited by research vessels and commercial ships of opportunity (SOOP) equipped with underway pCO2w systems that also make routine measurements of CO2 in the MBL. The atmospheric CO2 data from these ocean community CO2 systems do not, however, typically meet the rigorous standards of the atmospheric CO2 measurement community, as set out in the World Meteorological Organization (WMO) Global Atmosphere Watch (GAW). Much of this data is currently not quality controlled. If the MBL CO2 data from these ocean community measurement systems can be validated, and where necessary improved, this could lead to mutual benefits for both oceanographers and atmospheric scientists. As described below, based on initial comparisons and analyzer performance on underway systems, an accuracy of 0.2 ppm can be reached with these systems. While this is less accurate than the targets of global atmospheric CO2 measurements, such calibrated measurements can be used effectively for constraining air-sea CO2 fluxes, and in inverse models.
Surface ocean CO2 measurements have been performed onboard ships for over 50 years (Takahashi, 1961; Keeling, 1965) using approaches that are similar to current measurements, but the observations have become increasingly more automated. Unattended measurements referenced against compressed air standards traceable to atmospheric CO2 standards are now done routinely on ships and, since the 2000s, on moorings (Sutton et al., 2014). The measurements cover much of the global ocean, and allow regular access to regions of economic and environmental importance such as upwelling regions (González-Dávila et al., 2017). Many of the measurements are performed following standard operating procedures (e.g., Pierrot et al., 2009) and much of the data are submitted to global datasets and undergo independent secondary quality control. However, there is no global coordinated effort at the operational level for the data acquisition from ships and moorings as is proposed here for SOCONET.
This paper outlines the ongoing efforts to use established assets to create a reference network for high-quality surface ocean CO2 observations from SOOP and moorings. As part of the effort we will assess current accuracy and develop protocols for improvement of MBL measurements. The effort is focused on the operational aspect, that is, the operations and tracking of the platforms; acquisition of the data; and their validation. The scientific justifications and resulting products are briefly described. While the need of global coordination has been highlighted over the last decade (Bender et al., 2002; Monteiro et al., 2010; Wanninkhof et al., 2012), the description and justification of doing so in a systems/network approach has been lacking. SOCONET is its developmental stages, and details have not been worked out and implemented. This community white paper was developed from two abstracts for the OceanObs’19 conference, one focused on MBL and the other on the surface ocean CO2 measurements. The ideas described should be considered in a conceptual framework. The high-level scientific output and socioeconomic motivations are described first, followed by a discussion of the distributed network design, deliverables and challenges to establish the reference network. Table 1 provides a list of the acronyms and abbreviations used in this work.
Societal and Scientific Imperatives for Soconet
CO2 is an important anthropogenic greenhouse gas, and a major driver of climate change that has, and will continue to have, far reaching consequences for our society. Its relevance is highlighted as an Essential Climate Variable (ECV) in the atmosphere and the ocean (as part of the inorganic carbon system), as well as a biogeochemical Essential Ocean Variable (EOV). CO2 is produced by, for instance, the burning of fossil fuels, aerobic respiration, and oxidation of organic matter. At the most basic valuation this byproduct, or waste product, has an economic cost/value associated with it. Its cost/value has depended on speculation and has been affected by failures in the dedicated commodity markets. It currently is mostly traded as an “emission allowance” as part of a cap and trade system (re)instituted after the Paris Agreement. The largest trading system currently is the European Union (EU) emission trading scheme (ETS). The emission allowances in the EU ETS are equivalent to the right to emit one ton of CO2 (or 270 kg of C). While ocean carbon uptake is currently not part of the trading scheme, at the valuation listed it would have an annual value of 170 billion US dollars ($) (D’Maris and Andrew, 2017). This is based on a 2.5 Pg C yr-1 ocean uptake and a price of $19 per ton CO2.
While the uptake of CO2 by the ocean is not included in ETS, its value is recognized as an ecosystem service. The sequestration comes at a cost though in that the resulting elevated CO2 levels cause ocean acidification which impact ocean biota (see Appendix A). This, in turn, can have major effects on fisheries, tourism and other activities contributing to the marine economy. There are no estimates of the current dollar cost of the global impact of ocean acidification but an economic assessment of the impact of a future “OA catastrophe” ranges from a total cost of $97 billion to $301 billion (Colt and Knapp, 2016). While from an economic perspective the possible benefits of CO2 uptake, expressed per annum above, are greater than the total ecosystem service losses, such an analysis is overly simplistic and does not take the significant societal impacts into account. The socio-economic take-home message is that the anthropogenic component of the carbon cycle translates into many billions of dollars, and impacts ecosystem health and human well-being. It thus requires thorough investigation and monitoring.
Following the adage that anything of significant value needs to be tracked, many aspects of the global carbon cycle require monitoring. In particular, the stocks (inventories) of the major reservoirs and flows (fluxes) at the interfaces between the atmospheric, oceanic, and terrestrial boundaries need to be quantified. Many parts of the systems are monitored following well-developed network principles and data acquisition. The data from these networks are the cornerstone of increasingly sophisticated products benefitting from robust modeling frameworks. Of particular interest in developing SOCONET and MBL CO2 monitoring has been the development of the European Integrated Carbon Observation System (ICOS) which is a distributed network primarily based on established research entities incorporating oceanic, atmospheric and terrestrial components. This approach of going from measurements in research projects to a sustained monitoring network following clear protocols can guide development of SOCONET.
Surface Ocean CO2 NETwork will be a major contributor of reference quality observations to quantify air-sea CO2 fluxes on seasonal to interannual scales, and to determine trends in pCO2w levels over time. To deliver the global products on a regular and anticipated basis, it must be a global effort of sustained nature, and a network approach is most practical (Table 2). Networks are best established through a single source of funding/agency, with strong oversight and leadership, and uniform instrumentation. However, this is rarely achievable for global ocean networks focused on climate and environmental issues. The closest example in oceanography is the successful Argo profiling float network. SOCONET will be a distributed network involving many groups. It will provide coordination and homogenization of nationally funded efforts on a global level. The execution of the primary objectives rely on several other components and additional measurements. Besides accurate air and ocean water measurements provided by the SOCONET partners, data from other sources needs to be included through activities such as the Surface Ocean CO2 Atlas, SOCAT (Bakker et al., 2016) and mapping efforts such as SOCOM (Rödenbeck et al., 2015; Figure 1).
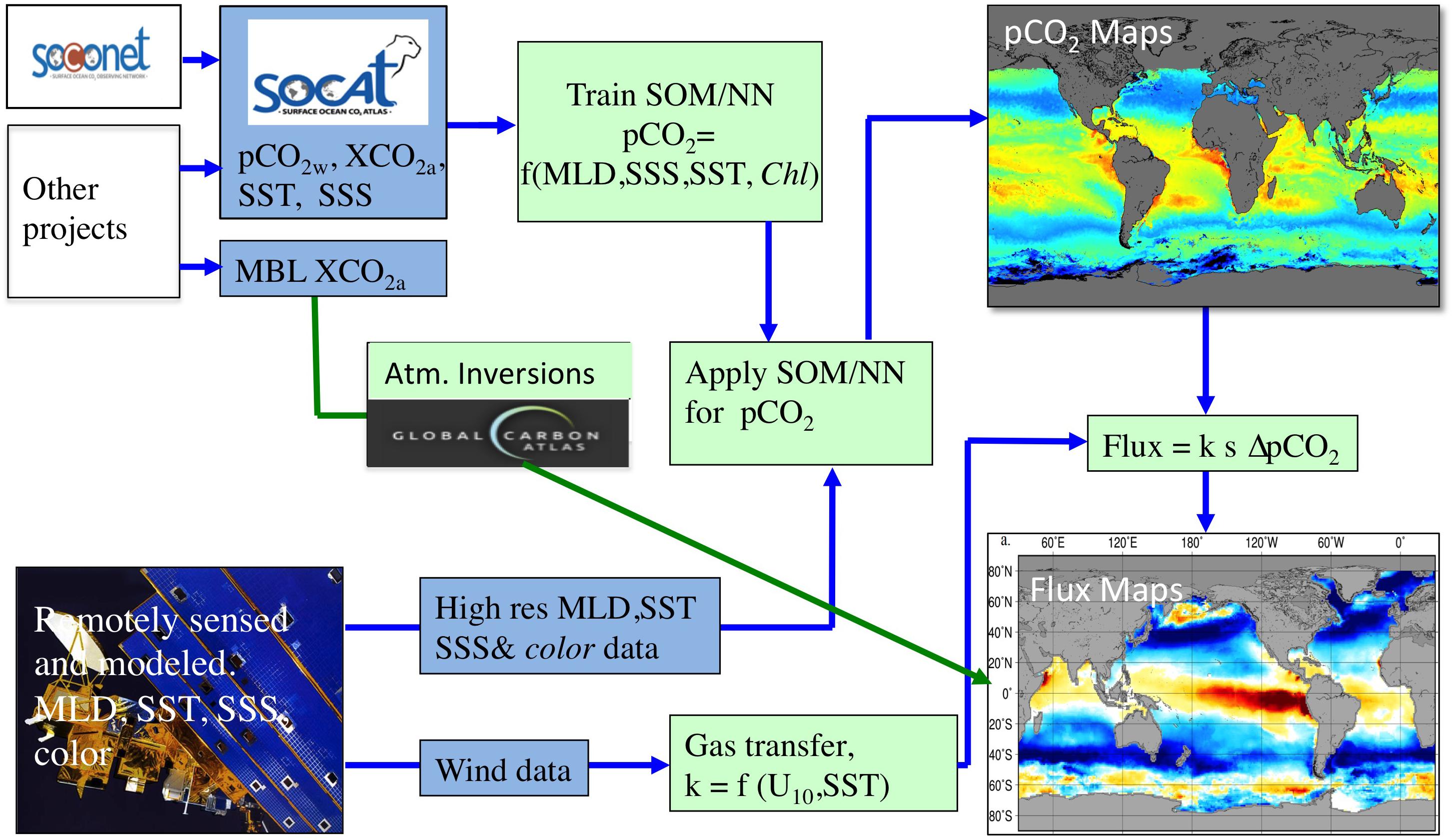
Figure 1. Schematic how SOCONET and MBL CO2 data will contribute to the creation of surface ocean pCO2 maps and CO2 flux maps. The blue boxes indicate data products and the light green boxes indicate the manipulations/calculations to the maps. This conceptual drawing indicates the many steps necessary to go from observations to products.
Surface Ocean CO2 NETwork is largely an operational entity but must be justified through delivery of (improved) products of scientific and socio-economic value. The major products that SOCONET will contribute to are surface ocean pCO2 maps and air-sea CO2 fluxes on monthly scales and with spatial resolution of 1°. The data need to be interpolated in time and space, and combined with other environmental parameters to create such maps (Figure 1). These maps rely on high-density data, often from satellite remote sensing (Shutler et al., 2019) and increasingly more sophisticated regression approaches, including machine learning such as neural networks (NN), and self-organizing maps (SOM) (Rödenbeck et al., 2015). Furthermore, possibilities of utilizing artificial intelligence (AI) approaches are being considered. Aside from application to determine the air-sea concentration difference (Eq. 1), the atmospheric CO2 measurements will be used by atmospheric inverse modeling teams to generate improved estimates of CO2 fluxes over oceans and adjacent continents (Jacobson et al., 2007; Gaubert et al., 2019).
These products and inputs are the cornerstones of derivatives, such as estimates of trends in uptake. The FCO2 estimates are currently used to test and benchmark carbon sink estimates derived from “bottom-up” ocean process models, many of which are used to predict future scenarios of global and regional climate change. The creation of surface pH maps using pCO2w as a primary variable, as part of the verifying targets of Sustainable Development Goal 14.3 is another important product. The needs for the products are articulated at high levels, such as the Global Carbon Project (GCP) that produces annual data-based estimates of fluxes between the major carbon reservoirs (Le Quéré et al., 2018), and the Global Climate Observing System (GCOS) that has called ocean acidification a headline indicator of changes in biogeochemistry in the ocean due to climate change.
The Establishment of Soconet
Network Principles
The SOCONET network development follows the network attributes proposed by the Observation Coordination Group (OCG) of JCOMM. This will facilitate incorporation of SOCONET within the JCOMM construct (Table 2). From an operational network perspective, a multi-PI distributed international network is challenging but benefits from human capital including expertise, innovation and oversight. The development of SOCONET relies heavily on established interactions in SOCAT. SOCAT is a well-designed data collation, quality check and distribution system of surface ocean pCO2 measurements (Bakker et al., 2016). SOCAT is not directly involved in the operational aspects of data acquisition that is the focus of SOCONET. A schematic of the interaction of SOCONET and SOCAT and the more informal product development efforts, such as the surface ocean pCO2 mapping intercomparison project, SOCOM is shown in Figure 2. Admission to SOCONET is selective based on meeting the network criteria. SOCONET will initially only include platforms that meet the data quality and release schedule as outlined in Table 3. The full details of SOCONET, that is focused on the operations of surface ocean CO2 measurements, can be found in the SOCONET prospectus (Wanninkhof et al., 2018) with a brief summary below.
Surface Ocean CO2 NETwork will cover key regions of the ocean (Figure 3) with data of specified quality. It will perform measurements following documented procedures and network practices including: common protocols, similar instrumentation, and standardization. It will provide standard operating procedures (SOPs) for acquiring the data. Data will be appropriately documented with metadata compliant with international protocols, and accuracy and precision requirements. Surface water pCO2 data from SOCONET will be submitted through the established SOCAT data system. The platforms will be tracked through the JCOMMOPS platform management system and tagged as SOCONET reference network data. The network will be constructed within the Framework for Ocean Observing (FOO) of the Global Ocean Observing System (GOOS) and in accord with FOO mission statement:
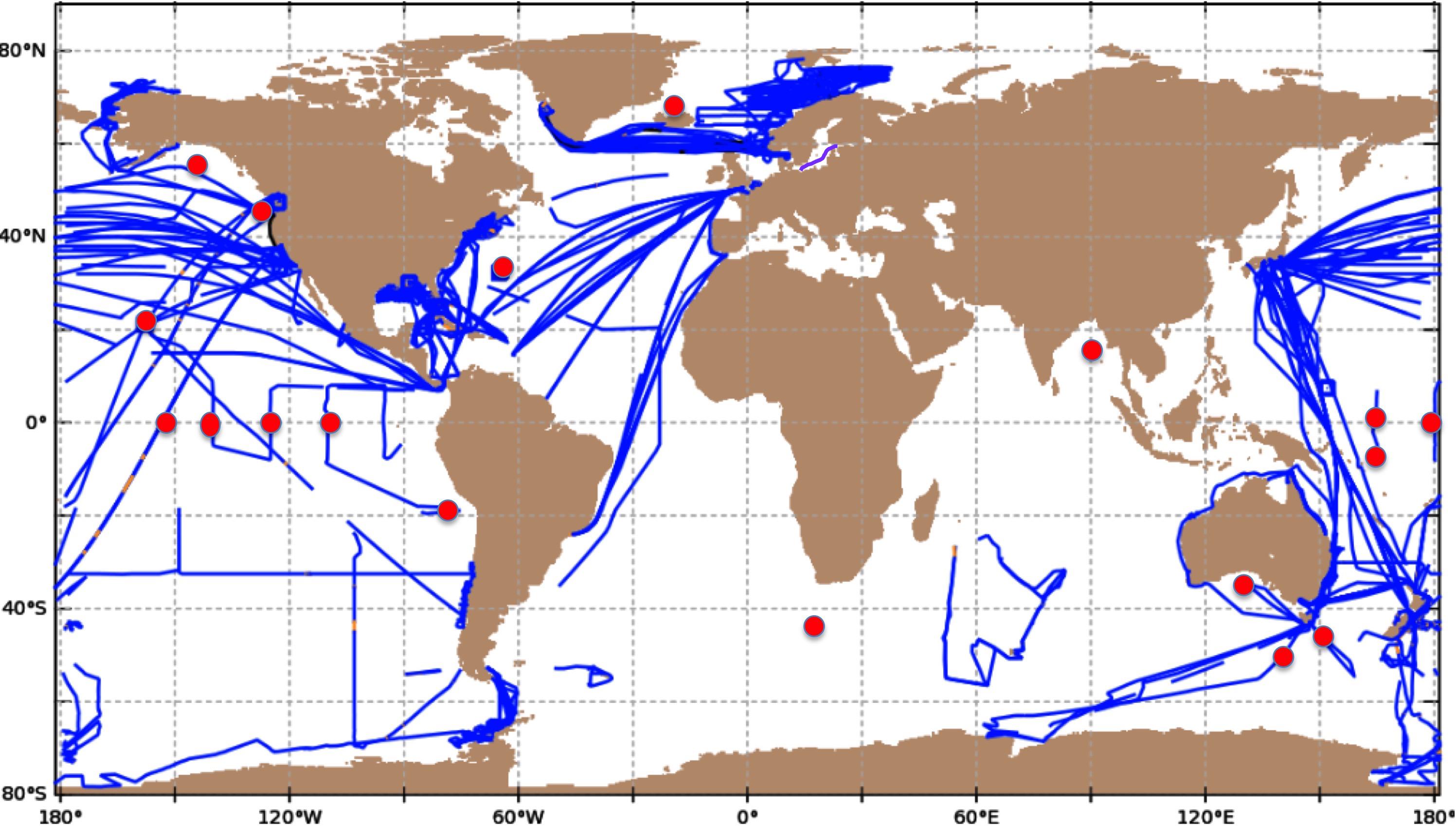
Figure 3. Ship lines and moorings that currently meet SOCONET data quality and are potential contributors to the SOCONET effort. Lines are based on the SOCAT holdings from 2017 to 2018 with pCO2 data that are believed to be accurate to within 2 μatm. The mooring sites with systems meeting the data quality standards are indicated by red circles.
“A framework for moving global sustained ocean observations forward in the next decade, integrating feasible new biogeochemical, ecosystem, and physical observations while sustaining present observations, and considering how best to take advantage of existing structures.”
The objectives and criteria of the SOCONET reference network are provided in Table 3.
Platform Types
Surface Ocean CO2 NETwork is envisioned as a multi-platform EOV-based network, but currently only includes instruments on moorings and ships. The differences and attributes of the platforms are shown in Table 4. The strengths and weaknesses of each platform listed are generalities, and vary for each individual platform, but it serves to show issues and challenges that require further attention. There are several other autonomous platforms and instruments that could be part of SOCONET in the future. However, each needs to be fully vetted in meeting the criteria specified in Table 3. Of particular use in this respect are instrument intercomparison exercises, and side-by-side comparisons to assure new platforms and instruments meet the requirements. Regular intercomparison activities are envisioned in collaboration with national and regional efforts, and coordination groups such as the alliance for coastal technologies (ACT) and the International Ocean Carbon Coordination Project (IOCCP).
Data Management, Access and Quality Control
The data management framework developed under SOCAT (Pfeil et al., 2013; Bakker et al., 2016) will also serve as the data depository for SOCONET surface water CO2 data (Figure 2), and likely for the MBL CO2 taken in conjunction with surface ocean pCO2. Over the last several years, the SOCAT data team has improved the submission, quality control, access and archival processes that support the annual releases of the SOCAT data products. These data products are available to the public through the web site, www.socat.info and are archived with persistent identifiers (doi’s) provided. In addition, the SOCAT data products are made available through the ERDDAP data platform, providing interoperable access to the datasets through a wide variety of tools and machine-to-machine services. Discovery and visualization services are provided for the SOCAT data through NOAA’s Live Access Server. By leveraging this framework, SOCAT, and therefore SOCONET, supports the FAIR (Findable, Accessible, Interoperable and Reusable) data principles for improved levels of data interoperability and reuse. The automated system used by SOCAT demonstrates a method to efficiently manage the larger volumes of data expected with the future of new ocean observing efforts and can support the emerging SOCONET.
Contributions of Soconet
Improved Understanding, Basic Research
Surface Ocean CO2 NETwork is, in part, a research network that delivers data for basic discovery and understanding of processes and mechanisms. Thus, the network will be used for more than the operational production of maps. This is important as there is a lack of understanding of the effect of variations and change in climate and ocean condition on CO2 levels, including the possibility of thresholds, tipping points, and feedbacks. The high quality needs and challenges of making the exacting measurements require extensive basic understanding, instrumental expertise and manual quality control requires a firm knowledge of the processes and instrumental analysis.
Research questions relating to climate and ecosystem changes benefit from sustained observations. There are a series of research questions that can, in part, be addressed with data from SOCONET platforms including quantifying the physical parameters impacting air-sea CO2 exchange (e.g., Zappa et al., 2004); the impact of the biological pump on surface ocean CO2 levels (e.g., Merlivat et al., 2015); feedbacks of calcifying organisms on surface water CO2 (e.g., Frankignoulle et al., 1994); the control and changes of biogeochemical process (e.g., Schneider and Müller, 2018); and the response of surface ocean CO2 levels to changes in atmospheric forcing (e.g., Arora et al., 2013). The latter is of great importance in the socio-economic arena to assess the efficacy of fossil fuel CO2 reductions in meeting climate accords (Peters et al., 2017) that will require observational validation.
The data from SOCONET platforms will be used to improve the quantification of air-sea CO2 fluxes through timely updates to algorithms such as those established in SOCOM (Rödenbeck et al., 2015). The observations can also be used in data withholding exercises that provide an independent estimate of the accuracy of the results. The rapid release of data can inform and serve as an early warning to changing patterns and trends, in particular those that are not fully captured in the regression approaches. The data will be critical to validate the results of new sensors and new platforms. Of note is the validation of pCO2 derived from pH sensors from profiling floats to estimate CO2 values (Williams et al., 2017). While the derived pCO2 data from pH provide good precision, the accuracy of the derived pCO2 is not well constrained and this can be uniquely addressed by validation with accurate in situ pCO2 data.
Network Design
To date there has not been a formal design of a global surface ocean CO2 network. Bender et al. (2002) provide a broad view of network needs based on de-correlation analyses which were fine-tuned by Li et al. (2005). Regional observing requirements for the Southern Ocean are described in Majkut et al. (2014), and an observing system design for biogeochemistry for this region is described in Kamenkovich et al. (2017). A global surface ocean CO2 network design has been lacking, in part because there have been no formal collaborations between operators of systems. Moreover, because of the paucity of data, and their many applications, any new data is considered a significant contribution.
Instrument deployment for accurate CO2 measurements is currently limited to platforms such as ships and moorings, but autonomous surface vehicles (ASV) have the potential to expand the means to obtain data. Data, particularly from the ASVs and research ships that often visit remote ocean regions, will be useful in observing system design. Several approaches such as observing system simulation experiments (OSSE), and observing system experiments (OSE) are available that utilize a priori knowledge of the global fields to optimize sampling strategy. These network design approaches, as well as approaches using mapping and data denial experiments will be necessary to justify and implement a comprehensive SOCONET network.
Using pCO2w to Estimate Other Inorganic Carbon Parameters and Develop Products
In addition to using pH to estimate pCO2w (Williams et al., 2017), the reverse needs to be investigated as well (Appendix A). The utilization of surface ocean pCO2 to aid in creating surface ocean pH maps will be an important use of SOCONET data (Lauvset et al., 2015). This is of particular relevance to determine longer-term trends in surface OA that need high accuracy data as called out in UN Sustainable Development Goal (SDG) 14.3 Ocean acidification and climate change. Much of the dedicated OA data are of lower quality focused on larger excursions of pH on sub-seasonal and local scales. These measurements are generally not suited for determining longer-term trends in OA. The Global Ocean Acidification Observing Network, GOA-ON will rely, in part, on SOCONET observations to estimate global patterns and trends. Figure 4 is an example of a high-resolution monthly pCO2 map based on a SOM/NN approach. The pCO2w data, along with measurements or estimates of TAlk or DIC, can be used to calculate pH from which surface ocean pH maps can be created applying similar mapping approaches (Takahashi et al., 2014). A major deliverable of SOCONET will be data for improved near-term estimates of air-sea CO2 fluxes. As described above, there are several other data streams required to determine air-sea CO2 fluxes, such as remotely sensed winds for estimating the gas transfer velocity, and different parameters to aid interpolation, most notably sea surface temperature (SST) (Figure 1).
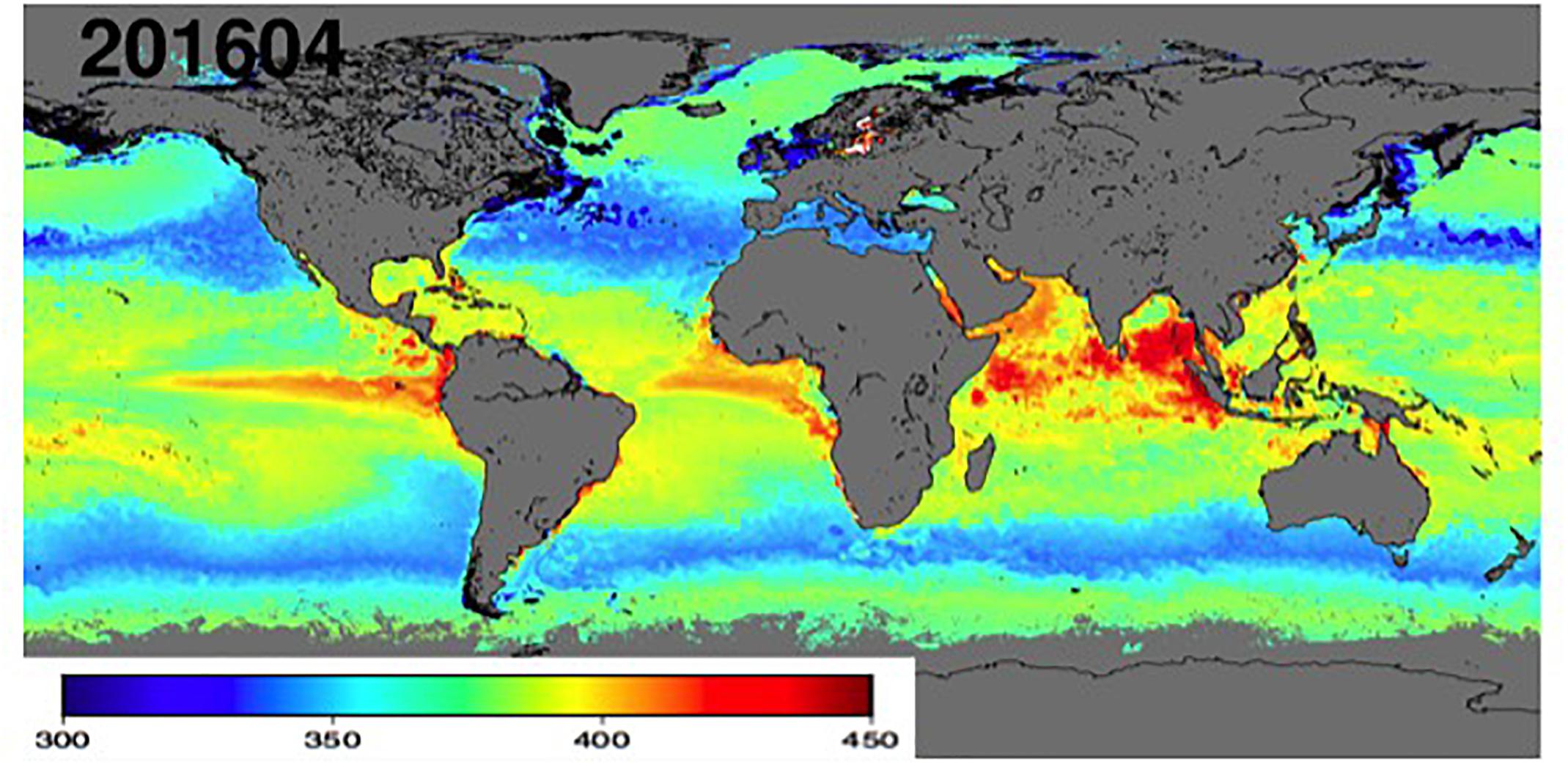
Figure 4. Monthly map of pCO2w for April 2016 created by a NN/SOM method showing the high fidelity of the output taking advantage of high-resolution remote sensing data. This example uses SOCAT data as the training set (units: μatm) (J. Triñanes, pers. com.).
Contribution of Mbl Co2 Observations
Surface Ocean CO2 NETwork has a strong focus on accurate pCO2w measurements (Table 3), but offers a unique opportunity to contribute to (air) MBL CO2 data, which are undersampled over the open ocean. Most of the underway pCO2 systems used in SOCONET take 5 air measurements, 1-min apart, from an intake at the bow or bridge of the ship, at intervals of about 3 h.
Moored pCO2 systems in SOCONET take an air measurement every 4 h from 0.5 to 1 m on the buoy tower. By developing proper measurement protocols and quality control procedures, these data will be useful for improved MBL and air-sea CO2 flux products. Here we focus on these measurements and means to verify their accuracy. In addition, there are dedicated instruments on some ships that meet GAW accuracy requirements. These efforts should be expanded, and having both types of instruments on select ships will provide critical information on the quality of the air data from the systems measuring surface water pCO2. Since the accuracy of MBL CO2 data from underway CO2 systems has not been fully investigated, and dedicated MBL systems meeting GAW accuracy requirements are costly, the air MBL requirements for SOCONET are under discussion and development. Below we describe the justification and current status.
Justification for Making Calibrated Accurate MBL CO2 Observations From Ships
Here, calibrated accurate CO2 measurements are those that are compatible to within ± 0.2 ppm of the global CO2 scale maintained by the WMO/GAW Central Calibration Laboratory (CCL). We propose that this is the quality standard to which ocean community MBL CO2 measurements should strive. The term accuracy is used instead of precision/repeatability in recognition that imprecise measurement systems can still be sufficiently accurate if the noise in the data is randomly distributed around the “true” value and therefore does not bias the mean values. The MBL CO2 variability over the ocean interior is smaller than atmospheric CO2 variability over land, and MBL CO2 from the relatively imprecise measurements from systems focused on pCO2w should be able to achieve the needed levels through averaging if these systems are appropriately optimized for atmospheric CO2 measurement and kept well-maintained, but this has not been fully tested. It should be noted that the WMO/GAW ± 0.1 ppm compatibility goal (±0.05 over the Southern Hemisphere) will likely not be attained by the systems measuring pCO2w. Moored air CO2 measurements have not been validated to yet meet the ± 0.2 ppm goal and this should be an area of focus for improving accuracy of existing moorings. Data of such accuracy from sparsely sampled oceanic regions will be beneficial to atmospheric inverse modelers as long as their accuracy is quantified and described in the metadata. Moreover, this level of accuracy will not introduce a significant error in the air-sea fluxes where the uncertainty in the concentration gradient is dominated by the pCO2w measurements that are good to within ± 2 μatm.
Validating and improving the quality of oceanic MBL CO2 measurements is mutually beneficial to both the ocean and atmospheric research communities. One of the key advantages for the ocean community is the improvement of air-sea CO2 fluxes (FCO2). While most ships make in situ MBL CO2 measurements, FCO2 is not usually calculated using these data. Instead, values for pCO2a (from Eq. 1) are most commonly derived from the MBL reference data product provided by the Global Monitoring Division (GMD) of NOAA/ESRL. This data product is generated from a subset of NOAA atmospheric CO2 measurement sites near the coast that predominantly experience MBL air. These data are filtered, interpolated, and smoothed prior to being fit at latitudinal intervals of 0.05 sine of latitude from 90°S to 90°N and joined to create a 2-dimensional matrix (time versus latitude) of weekly CO2 values (Conway et al., 1994; EW Team, 2005). Thus, while this data product is useful for identifying large-scale trends, it does not reflect the full spatial or temporal variability of MBL CO2 that exists in the atmosphere, as explained in the online documentation and demonstrated previously (Pickers et al., 2017). The implications for FCO2 calculated using this product are that in some regions, particularly coastal margins where the effects of continental airflow on MBL CO2 are not included in the NOAA MBL data product, biases will arise in the air-sea CO2 fluxes.
Comparing FCO2 calculated using different sources of MBL CO2 data is useful for demonstrating the potential impacts of using inaccurate atmospheric data to calculate fluxes. Figure 5 shows that air-sea CO2 fluxes calculated using the observed MBL CO2 values at the Martha’s Vineyard site in Massachusetts, United States (41.3°N, 70.6°W) can differ by up to 15% compared to those calculated using the NOAA MBL product. Mean annual differences between atmospheric CO2 from the CarbonTracker 2017 modeling system (Peters et al., 2007) and the NOAA MBL reference product can be as high as 20 ppm within coastal seas near industrial centers, which translates into flux differences for these regions that can exceed 0.5 mol m-2 yr-1 (Figure 5). Moored pCO2 systems, which measure air CO2, also show that these measurements can differ from the MBL reference data product in annual mean and seasonal variability due to local and regional effects (Northcott et al., 2019; Sutton et al., 2019). Although the uncertainty associated with pCO2a is often not considered to be significant compared to other sources of uncertainty in Eq. 1, Figures 5, 6 indicate that inaccurate atmospheric CO2 values can lead to significant biases in FCO2 at both local and regional scales. Using the in situ atmospheric CO2 data from ships and moorings will likely eliminate these FCO2 biases, provided that the MBL CO2 data are sufficiently accurate and devoid of ship contamination.
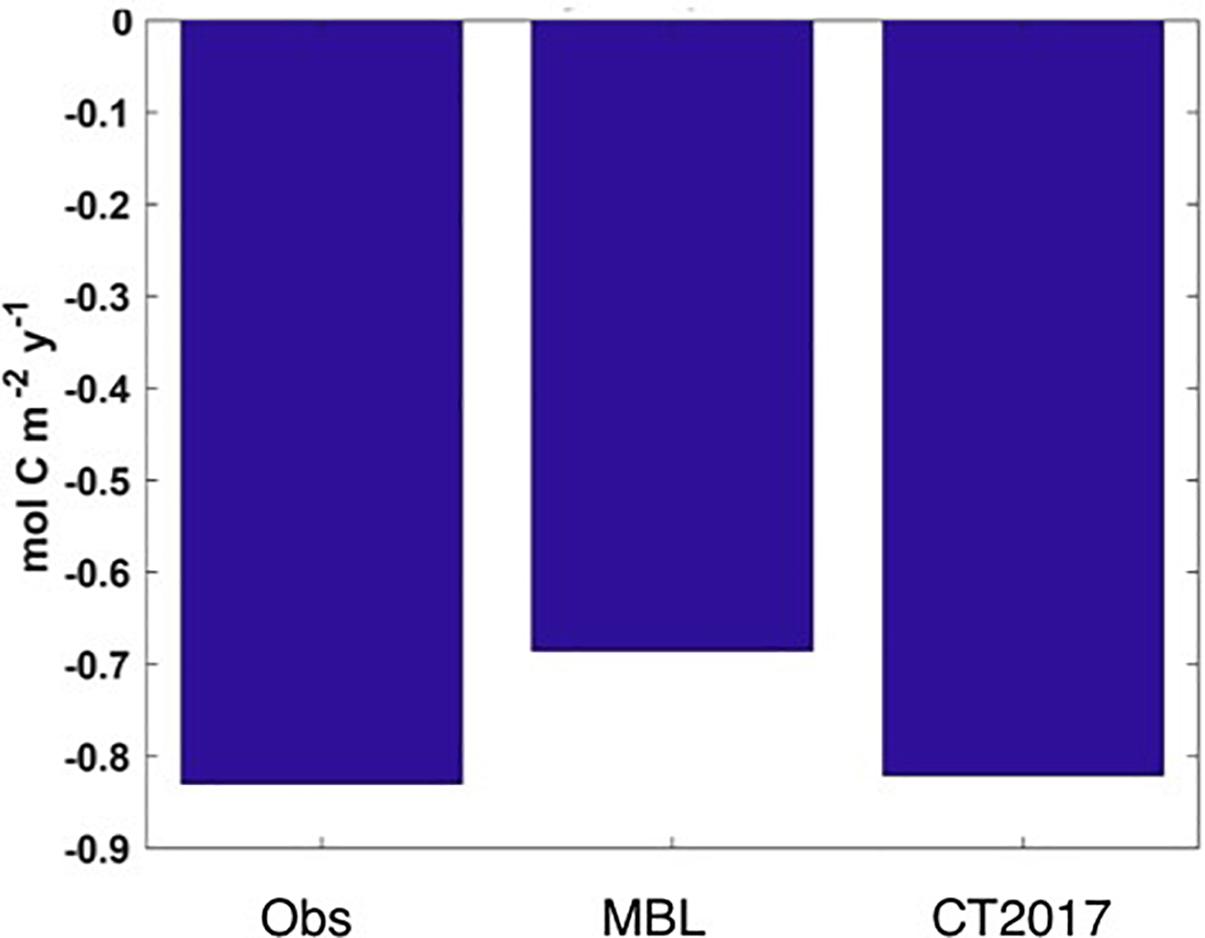
Figure 5. Air-sea CO2 fluxes at Martha’s Vineyard, calculated using three different sources of atmospheric CO2: in situ observations at the site (obs); the NOAA MBL reference product (MBL) and CarbonTracker 2017 (CT2017).
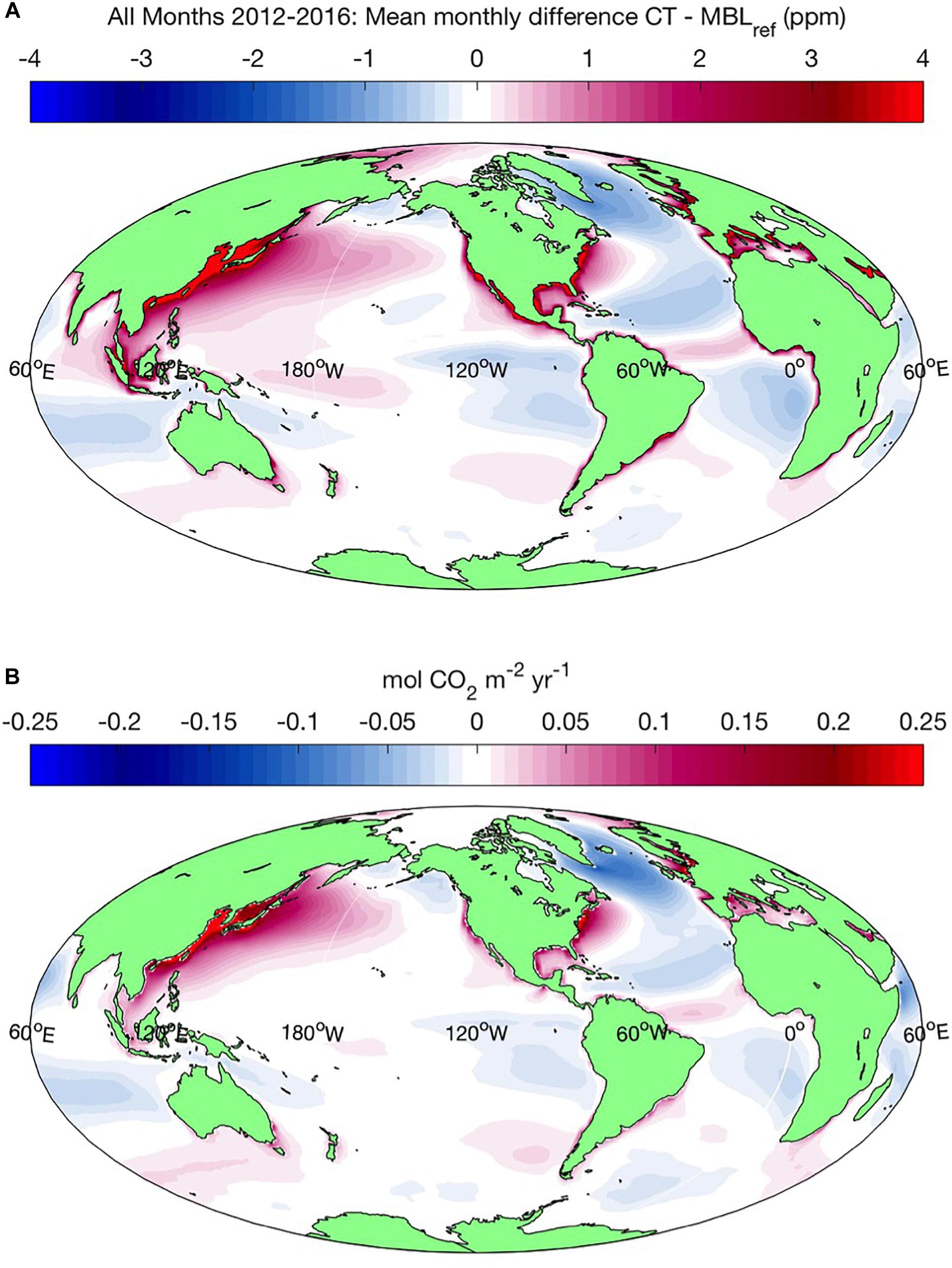
Figure 6. (A) Mean monthly difference in atmospheric CO2 over the oceans between CT2017 and the NOAA MBL CO2 reference product, for the period 2012–2016. Note the differences downwind of the northern hemisphere continental land masses; (B) annual mean difference in FCO2 that arises from using atmospheric CO2 from CT2017 compared to the NOAA MBL reference product.
Other benefits to the oceanic community from improving or validating shipboard and mooring MBL CO2 data include increased confidence in CO2 flux data products that include data from multiple different ships/measurement platforms, and better traceability of pCO2 data to the Central Calibration Laboratory (CCL) of WMO/GAW currently housed at NOAA/ESRL. The process of upgrading current shipboard CO2 measurement systems and protocols to facilitate high-accuracy atmospheric CO2 data from oceanic regions has an associated financial cost. This will require a significant oceanic community effort that should be supported by the collaboration of the atmospheric measurement community.
High-accuracy MBL CO2 data from ships will benefit the atmospheric research community by substantially augmenting the atmospheric CO2 measurement network in regions that are currently undersampled. Such data will be of value to the atmospheric inverse modeling community, who estimate surface CO2 fluxes using a “top-down” approach, an alternative methodology for the calculation of global air-sea CO2 fluxes to the bulk flux approach (Eq. 1) that utilizes surface ocean pCO2 measurements (e.g., Takahashi et al., 2009; Landschützer et al., 2013, 2014). The “top-down” approach combines measurements of atmospheric CO2 (e.g., provided by the surface sampling network of NOAA-GMD) and other global contributors together with information on atmospheric transport (usually from atmospheric transport models), process-based prior flux estimates, and an inverse Bayesian optimization methodology (e.g., Rodgers, 2000). The current generation of such top-down inverse analyses often employ data assimilation or variational methods (e.g., Peters et al., 2007; Chevallier et al., 2010; Kang et al., 2011) and can provide grid-resolved flux-estimates at spatial-scales of ∼10 km to 100 km (e.g., Broquet et al., 2013; Babenhauserheide et al., 2015). While top-down methods provide valuable alternative constraints on surface CO2 fluxes, they are subject to significant uncertainties in regions of sparse sampling, most notably, in open ocean regions with few fixed sites (Rödenbeck et al., 2006), as well as significant uncertainties relating to atmospheric transport and the data assimilation methodology.
Given the additional cost involved in improved MBL CO2 data from ships and moorings, interaction with the inverse modeling and observing system design communities will be used to identify regions where the added data have highest impact on uncertainty reduction. Within the European ICOS Network, pilot studies for the acquisition of MBL CO2 data matching the standards of the atmospheric community are currently underway. SOCONET can make use of these investigations for the design of a network of high-accuracy MBL CO2 measurement platforms with the aim to maximize the scientific return of investment.
High-Accuracy Atmospheric CO2 Measurement Approaches and Data Validation
The task of improving oceanic community MBL CO2 measurements will be approached in two ways: by upgrading existing measurement systems that are not currently optimized for atmospheric CO2 measurements; and, investment in new, purpose designed measurement systems that employ more modern technologies such as laser-based techniques. It is likely that some ocean community MBL CO2 data are already sufficiently accurate to be used in FCO2 calculations and in inverse modeling studies where highest-accuracy is not required.
However, without validation this cannot be determined at present. Two approaches for improving MBL CO2 are discussed, as well as the importance of data validation and quality control. Detailed technical information regarding atmospheric CO2 measurement can be found in WMO/GAW Report 229 (2016) and in the ICOS atmospheric stations specifications document (Laurent, 2017).
Most existing underway pCO2 measurements are currently made using instrumentation following ocean surface water pCO2 community design (Pierrot et al., 2009). The systems have been built in-house at different laboratories and are currently available from General Oceanics Ltd. They have both seawater and atmospheric CO2 measurements capabilities using a non-dispersive infrared (NDIR) analyzer (typically those manufactured by LI-COR Inc.), the traditional method for continuous atmospheric CO2 measurement. Ocean community MBL CO2 measurements are typically only required to be accurate to about ± 1 ppm in order to calculate air-sea CO2 fluxes to specifications (Bender et al., 2002); hence, these measurement systems are not designed for atmospheric CO2 measurement, with the priority instead focused upon ensuring the highest possible quality of near-continuous pCO2w measurements. As such, the setups of these measurement systems are not optimized for obtaining high-accuracy MBL CO2. For example, the wetted parts (i.e., the surfaces of components, such as pumps, valves and tubing, that are in contact with the sample air stream) might not be suitable for precise atmospheric CO2 measurement, sample air drying might not be sufficient (insufficient drying can lead to CO2 dilution, pressure broadening effects, and surface effects with tubing walls, all of which can bias CO2 measurement), and there may be small undetected leaks, which can cause non-negligible CO2 biases owing to the rigorous precision requirements of atmospheric CO2 measurement. Furthermore, calibration protocols are currently not sufficiently rigorous to meet the compatibility standards aspired to by the atmospheric CO2 measurement community as outlined in WMO/GAW report no. 229. Nevertheless, with careful adherence to established protocols and procedures, it appears possible to obtain well-calibrated, accurate atmospheric CO2 data using these existing systems.
Moored pCO2 measurements in SOCONET are made using an equilibrator- and NDIR-based methodology similar to the underway systems described above. The detector is spanned using WMO-traceable CO2 reference gas and zeroed using air stripped of CO2, prior to every measurement. The sample air is not as completely dried as in the underway pCO2 method (Sutton et al., 2014). Current development efforts are focused on improving accuracy through incorporation of a higher-quality NDIR or other CO2 analyzer, further drying of air sample, and incorporation of a CO2 reference/target gas.
The advent of commercial CO2 analyzers that employ laser-based spectroscopic technology, such as off-axis integrated cavity output spectroscopy (Baer et al., 2002), Fourier transform infrared spectroscopy (Esler et al., 2000), and cavity ring-down spectroscopy (Crosson, 2008) have opened up new opportunities for high-accuracy CO2 measurement on ships. These spectroscopic analyzers are typically stable for longer periods of time compared to NDIR-based analyzers, thus significantly reducing reference gas (required for differential analyzers) and calibration gas demands. Spectroscopic analyzers usually also have the provision to make sufficiently accurate water vapor corrections compared to NDIR-based analyzers that are not very accurate for H2O, which can allow for the relaxation of sample air drying requirements. It is important to note, however, that partial drying is normally still required with spectroscopic analyzers, as maintaining a high-accuracy water correction in the field over the full range of ambient atmospheric H2O concentrations is challenging.
The use of ships for MBL measurements using the new technology is gaining traction with the WMO recognizing the first mobile research station in the GAW in May 2018 on the Australian ship, RV Investigator. This ship is equipped with a purpose-built atmospheric monitoring laboratory that reports 1-min measurements of atmospheric CO2 using a cavity ring down spectrometer. The ship is also equipped with an array of meteorological, radon and carbon particulate sensors that are useful for identifying land-based or ship-stack sources of CO2. These newer spectroscopic analyzers are much more expensive than NDIR analyzers; they can, however, be used for pCO2w measurement as well as MBL CO2 measurement, preventing the need to double up on equipment, as demonstrated by Becker et al. (2012). Depending on the model, they are also capable of other underway measurements of interest to the carbon cycle community, such as the stable carbon isotope ratio of CO2 (13C/12C) in water and air (Cheng et al., 2019).
To make an informed decision about how best to obtain high-accuracy MBL CO2 data (i.e., using existing equipment or investing in new instrumentation), one needs to take into consideration both the scientific goals and logistical constraints (such as space, power requirements, and frequency of maintenance). It is also necessary to address the following question: just how good are the existing data? Verifying the quality of MBL CO2 data is an important and on-going part of making such measurements, and there are several approaches that can be employed. A highly recommended way is the use of a Target Tank (TT). A TT is a cylinder of dry, natural air that has been measured for CO2 against the CCL maintained scale before and after it is deployed in the field. The TT is not used to calibrate the system, but is run periodically as a quality control check (e.g., Kozlova and Manning, 2009), to check if the TT CO2 value obtained from the shipboard measurement system matches the CCL declared value, thus enabling the compatibility of the pCO2 system to be quantified relative to the laboratory where the TT CO2 value was declared. The main limitation of TTs is that they usually do not pass through the whole gas handling system (it is generally not practical to feed TT gas through the inlet lines, for example), and so only provide a partial test of the system. The TT can also be used to assess drift of the onboard calibration cylinders.
Other methods that provide a more independent check consist of comparisons with co-located measurements, either from flask samples, which are collected in situ and sent to a laboratory for subsequent analysis, or by making use of a “traveling instrument”: a completely independent, high-precision continuous measurement system that is installed alongside the existing measurement system for a limited time. The latter approach is used as part of the WMO/GAW station audits in the atmospheric measurement community (Zellweger et al., 2016). Using the flask approach is logistically much easier and can be continued periodically, but does not necessarily help to identify the source of discrepancy in cases where measurements do not agree. Conversely, a traveling instrument can be impractical to implement for a shipboard system and is usually a one-time operation lasting only a few weeks, but is more likely to be able to assist in diagnosing CO2 offsets.
Employing at least one of the methods mentioned above to regularly validate MBL CO2 measurements is fundamental to maintaining good data quality, regardless of whether an investigator uses existing equipment or new instrumentation.
A separate issue is that ships are moving platforms that generate their own CO2 emissions; thus, shipboard CO2 measurement differs from land-based CO2 measurement, where stations are typically located remotely from local sources of pollution to avoid data contamination. While efforts are made to locate measurement system inlets as far away as possible from ship exhaust stacks, it is usually unavoidable that some CO2 emissions from the ship itself will be observed and will need to be filtered out of the dataset, or “flagged,” during post-processing. Even if exhaust CO2 emissions are not often detected (as on some of the larger container ships), any data that is deemed to be “non-background,” such as when ships are close to the coast, will also need to be identified. Moorings and wind- or wave-powered ASVs avoid this CO2 contamination, except when in proximity to a ship or to the coast.
A simple and effective method for flagging non-background values in a MBL CO2 dataset is to assess the ± 1σ standard deviation (sd) of the CO2 values over a specific time period, often an hour (but sometimes a shorter or longer time period is used, depending on the measurement frequency). Other, more sophisticated statistical flagging methods also exist, such as the “REBS” method from El Yazidi et al. (2018), but are not necessarily any better than the sd approach. For ships, it is also often prudent to combine a statistical flagging method with meteorological flagging, whereby data that are measured when the relative wind direction originates from the exhaust stack of the ship and when the absolute wind speed is low are flagged as polluted (e.g., Chapter 3 of Pickers, 2016).
Regardless of the automated flagging method used, some manual quality control/validation of shipboard MBL CO2 measurements is desirable if these data are to be made available to the wider scientific community via online databases such as SOCAT. Details on quality control activities and who would be responsible are currently being worked out.
Perspective and Status of Product Development
The need for ocean carbon networks was articulated in a previous Ocean Observing Conference, OceanObs09 paper (Monteiro et al., 2010) and in an Integrated Ocean Observing System (IOOS) Summit manuscript (Wanninkhof et al., 2012). SOCONET is a refinement of the concepts discussed in these proceedings with more focus on network design, required instrumental accuracy and deliverables. SOCONET aims to contribute data of known high-quality and at regular intervals for three main products: surface ocean CO2 maps; the global air-sea CO2 fluxes at monthly resolution and 1° by 1° grid that will be served annually; and MBL CO2 data to constrain inverse models. These products are in development in research mode by different groups. The inverse models and assimilation approaches such as CarbonTracker are quasi-operational but results can be improved with quality MBL CO2 data.
Surface maps of ocean acidification can by created in a similar fashion as surface ocean CO2 maps, utilizing surface ocean pCO2 data, and estimates or measurements of DIC or TAlk.
These include pH maps but also carbonate ion concentrations and aragonite/calcite saturation state maps. A synopsis of the interrelationships between pCO2 and inorganic carbon parameters as they pertain to OA are provided in Appendix A. The global climatological maps of pH by Jiang et al. (2015) were produced from measurements and interpolation of the relevant ocean acidification parameters calculated from total alkalinity (TAlk) and total dissolved inorganic carbon (DIC). SOCONET will provide data for products that more closely follow the approach of Takahashi et al. (2014) and Lauvset et al. (2015). It uses surface ocean pCO2 data together with estimates of TAlk based on salinity to determine climatological OA products. The Takahashi et al. (2014) effort includes interpolation and is on monthly resolution and 4° by 5° spacing, and is based on a climatology referenced to year 2010 excluding the Pacific. By creating pCO2 fields using remotely sensed sea surface temperature (SST) and sea surface salinity (SSS) fields and other high-resolution data, the OA products derived from SOCONET can be created at higher temporal and spatial resolution (Salisbury et al., 2015; Shutler et al., 2019). The approach of assessing OA from pCO2 measurements may be hindered in coastal settings, such as the Baltic Sea where TAlk and TAlk-SSS relationships may change on similar timescales as pCO2 (Müller et al., 2016).
There are several efforts to create air-sea CO2 flux maps. Monthly climatologies at 4° by 5° grids referenced to a particular year are provided in Takahashi et al. (2009). The temporal and spatial gap filling, which is a major consideration in the production of maps, was aided by using a surface velocity field from an ocean circulation model. Lee et al. (1998) and Park et al. (2010) used these pCO2w climatologies to determine changes in time and space by establishing correlations between pCO2 and SST for each 4° × 5° pixel. This provided the first observation-based estimate of interannual changes in air-sea CO2 fluxes. More sophisticated approaches have been developed in the last decade, most notably NN and SOM approaches, and data constrained inverse methods. Eleven of the pCO2 products have been evaluated in a project called SOCOM (Rödenbeck et al., 2015). The detail and complexity of interpolation schemes differ significantly between the various approaches but they all aim to create pCO2 fields at high resolution from relatively sparse data (Figure 4).
Advances in collation of data from groups worldwide have aided the product development. First initiated by Taro Takahashi of LDEO, Columbia University, largely as a single investigator effort, it was communalized under the auspices of IOCCP as the Surface Ocean CO2 Atlas (SOCAT) effort that provides annual releases of data voluntarily submitted and quality controlled by groups around the globe (Bakker et al., 2016). The value added to the collated dataset is that the data undergo secondary quality control, and pertinent external parameters are added. Standardized metadata and common methods of data acquisition are encouraged, in part, through a ranking of datasets from A through F. Since data sets rated as A and B meet the accuracy standards for SOCONET pCO2w data (Table 3), the SOCAT data can be used as an initial screening of platforms. Data products averaged at 1° by 1° for the open ocean and ¼° by ¼° for the coastal ocean are provided by SOCAT as well.
A challenge in producing accurate global surface ocean CO2 and flux maps is that the magnitudes of longer-term trends in pCO2w are small compared to spatial and temporal variability but their assessments are critical in evaluating the trends of the flux on decadal time-scales (Schuster and Watson, 2007; Landschützer et al., 2014; Iida et al., 2015). Ocean acidification and long term changes in air-sea CO2 fluxes are driven by increases in atmospheric CO2 and the resulting disequilibrium between marine air and surface ocean, which is small. This small disequilibrium is difficult to discern. Atmospheric CO2 values that are currently increasing by 2.4 ppm yr-1 and seasonal changes in pCO2w that can be greater than 150 μatm. Regional annual mean differences are over 50 μatm (Figure 7). Moreover, near-surface gradients in CO2 caused by temperature and other physical and chemical effects can influence the CO2 gradient and flux across the interface. This requires more investigation and could influence operational aspects of SOCONET in the future. An underappreciated fact in view of the large variability is that small systematic biases in pCO2 measurements and biases caused by the interpolations over time will have a large impact on quantification of uptake.
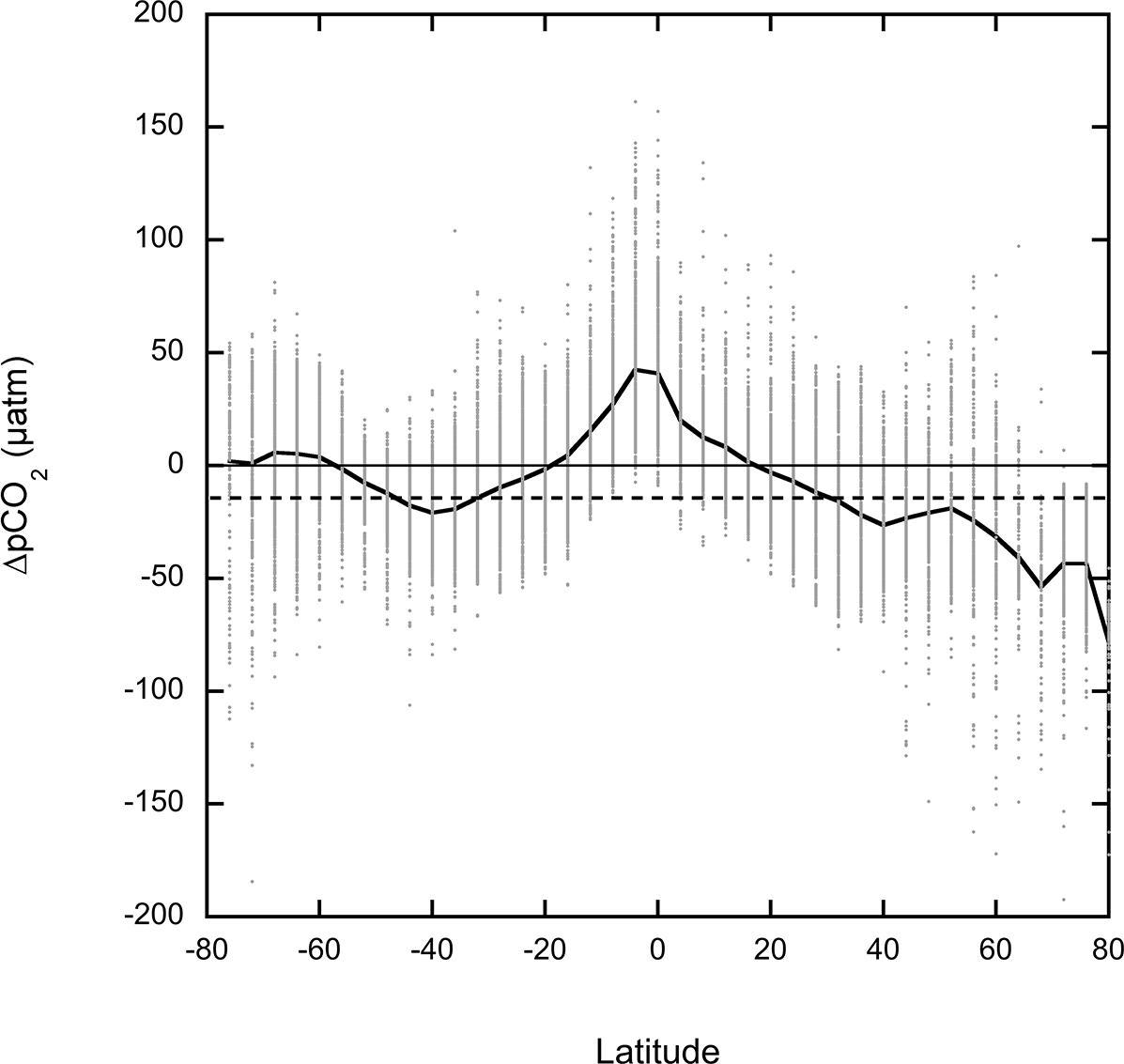
Figure 7. Meridional cross section of the ΔpCO2 (=pCO2w – pCO2a) climatology of Takahashi et al. (2014). The black line is the annual mean ΔpCO2 per 4° interval and the gray squares are the monthly averages for the 4° by 5° circumglobal grid boxes for the particular interval. Points above the solid horizontal line (ΔpCO2 = 0) mean that the area is a CO2 source to the atmosphere. The dashed line is the average disequilibrium needed for the global ocean to sequester 2.5 Pg C yr-1.
It is envisioned that the production of near real-time surface ocean CO2 maps and CO2 flux maps will rely heavily on the SOCONET effort. Currently the maps are not created in an operational fashion but tools to do so are under development. The closest to an operational product are the SOM/NN approaches. Summaries of the methodologies to determine surface CO2 fields and CO2 fluxes are provided in Rödenbeck et al. (2015) and Zeng et al. (2017). In these efforts the fidelity of the different approaches are critically and objectively investigated, and visualized through, for example, Taylor diagrams such that a concise statistical summary is obtained of how well patterns match each other in terms of their correlation, their root-mean-square difference, and the ratio of their variances (Taylor, 2001; National Center for Atmospheric Research Staff [NCAR], 2013).
All current surface ocean CO2 mapping efforts rely on interpolation and/or creating algorithms of pCO2 with environmental fields that are available with high space/time coverage. The ability to create realistic, near real-time maps will depend on the amount of pCO2 data available, its timeliness, and, because the fluxes are greatly influenced by bias, on the accuracy of pCO2w and pCO2a values. The MBL and surface ocean CO2 values are systematically changing with time due to emission of anthropogenic CO2 into the atmosphere, such that obtaining values in a timely fashion is critical.
The need for up-to-date CO2 values for accurate and timely products is emphasized as current approaches rely on creating relationships of pCO2 with variables that can be obtained in near real-time through remote sensing, models or from autonomous platforms. The NN and SOM methods that are increasingly used are based on machine learning of patterns and correlations. The relationships are created with different input parameters but generally include SST, location, mixed layer depth, and sometimes SSS, and ocean color. In some approaches there is partitioning based on biogeographic provinces that are effective for the changing ocean (Oliver and Irwin, 2008; Fay and McKinley, 2014). The independent variables change with time, and can change in a different fashion than surface ocean CO2, such that continued updates using recent pCO2w data are important in order to produce accurate products. Once the correlations in machine learning approaches are established, the approach can be used in absence of actual pCO2w data. However, the products can become biased over time if the algorithms are not updated.
Maps can be created as soon as the independent variables are available; this is in near real-time and within a year with quality control. It is a priori assumed that over annual time period the relationship between pCO2w and independent variables is invariant. If pCO2 data are available in a prompt fashion, these can be used for validation and for updating the parameterizations. A proper collation and quality control mechanisms of recent SOCONET data, and an approach to easily ingest the SOCONET data into algorithms will be essential. Being able to provide up-to-date information of anticipated data through real-time data tracking will facilitate the routine development of products.
Connectivity to Other Scientific Efforts and Networks
Surface Ocean CO2 NETwork will contribute to other surface ocean networks and the MBL measurements can contribute to atmospheric efforts. This includes the full surface ocean pCO2 network, whose data are largely captured by SOCAT, and contains pCO2 data obtained by different types of instruments. Networks that focus on other carbon variables, often associated with OA and ocean health under the GOA-ON purview, will benefit from the SOCONET effort. In addition, the SOCONET effort is closely aligned with GO-SHIP, executed on research ships. Accurate surface ocean and air CO2 values can be used to constrain CO2 fluxes in a similar fashion as heat and momentum fluxes (Edson et al., 2004). The MBL CO2 measurements are part of a broader effort of greenhouse gas measurements over the ocean including nitrous oxide and methane in ICOS.
The network is focused on the infrastructure to deliver accurate pCO2 data. It is envisioned that the JCOMM Observations Program Area (OPA) structure will facilitate the operational interactions with other networks. The interactions are largely synergistic, and include the needs for implementing SOCONET, and benefits of SOCONET to other efforts.
Efforts and Networks That Are of Direct Benefit to SOCONET
The surface ocean thermosalinograph (TSG) network and data management by the Global Ocean Surface Underway Data project, GOSUD provide sea surface temperature and salinity data. TSGs are integral support instruments for surface ocean CO2 observations and interpretation, and are often critical for their transformation to OA parameters. All underway and mooring CO2 systems have TSGs but these data do not undergo quality control as part of the pCO2 data reduction. While TSG data are captured in the pCO2 files, it is at lower temporal resolution congruent with the pCO2 measurements. Interactions with JCOMM/SOT/SOOP should facilitate that the TSG data on SOOP-CO2 and Mooring-CO2 are quality controlled and served to the community. The quality control of salinity data would be coordinated through GOSUD. Automated routines for TSG data are available but access and flagging routines are cumbersome.
The Volunteer Observing Ship (VOS) Meteorological observations and moorings under the Data Buoy Cooperation Panel (DBCP) benefit SOCONET as barometric pressure is a key variable to calculate pCO2 in air and water. These measurements are made routinely on VOS for weather applications, and barometers are calibrated by the national weather services. Wind speeds used to calculate air-sea CO2 fluxes are generally obtained from remote sensing or numerical weather models but anemometer on ships or buoys are useful for comparison or validation of wind products.
Contributions of SOCONET to Other Research and Network Efforts
Measurements of pCO2a from SOCONET platforms can be used to improve the NOAA/GMD MBL CO2 reference product; to validate of MBL CO2 in support of remote sensing (Chatterjee et al., 2017); and ground-based networks, such as TCCON (the Total Carbon Column Observing Network). An example of current satellite capacity to obtain synoptic global column XCO2 based on the OCO2 mission values on global scales is provided in Figure 8.
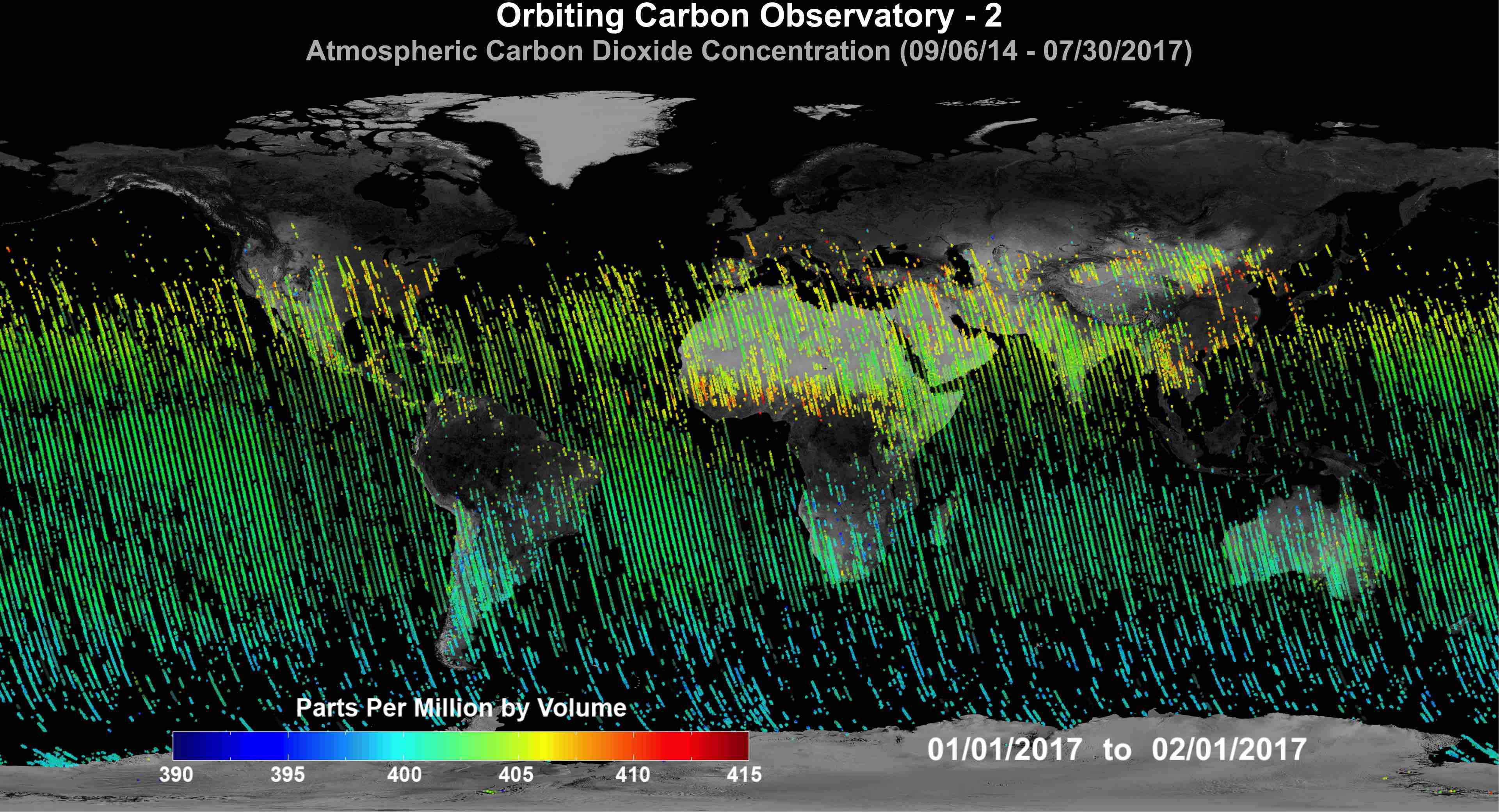
Figure 8. Column xCO2 measurement from the Observations from the Orbiting Carbon Observatory-2 (OCO-2) for January 2017. These data were produced by the OCO-2 project at the Jet Propulsion Laboratory, California Institute of Technology, and obtained from the OCO-2 data archive maintained at the NASA Goddard Earth Science Data and Information Services Center.
The value of underway pCO2 system MBL CO2 measurements with inaccuracies of up to 0.2 ppm still needs to be fully investigated; although these data would not meet the WMO CCL compatibility goal of +/- 0.1 ppm, but they still offer potential to be included in the collation/distribution efforts of the atmospheric measurement community because they help to fill gaps in the atmospheric measurement network. The World Data Centre for Greenhouse Gases (WDCGG), operated by the Japan Meteorological Agency (JMA) under GAW/WMO, and NOAA ObsPack products are two such atmospheric measurement community data distribution efforts that MBL CO2 data from pCO2 systems on ships could potentially contribute to. The atmospheric inverse modeling community as potential users of MBL CO2, for example, those involved in the TransCom and IG3IS initiatives, is another way the underway pCO2 community could forge and strengthen links with other scientists looking a similar carbon cycle issues from different angles.
The data from the SOCONET effort can be used to validate pCO2 estimates from BGC (biogeochemical) Argo floats. The development of biogeochemical sensors for Argo floats will greatly enhance our observational capabilities of the ocean, including the possibility of using the pH data from Argo to calculate surface ocean pCO2. The current estimated accuracy for pCO2w values derived from pH is about 7 μatm (Williams et al., 2017; Gray et al., 2018). However, the pH sensors cannot be calibrated once deployed, and pCO2w estimates need to be validated to evaluate how systematic errors evolve with time. This can be accomplished with ships in SOCONET. For example, cross-overs between SOCCOM BGC floats and the ARSV Laurence M. Gould have been evaluated by Fay et al. (2018). Strategies for targeting of BGC floats with SOCONET ships could enhance validation efforts by increasing the number and quality of cross-overs. As the reference network includes research ships that deploy the BGC Argo floats, co-located measurements are also possible at the site and time of deployment. A rapid return of quality-controlled data is desirable for this application.
Surface Ocean CO2 NETwork could be used to build out of a BGC network in the essential ocean variable (EOV) framework. Inorganic carbon is an EOV and pCO2 is a key component of the inorganic carbon system. Monitoring pCO2 from surface platforms will provide key insights on ocean acidification. It is a core measurement that can be used in conjunction with other developing BGC observations to study biological productivity in the ocean. The SOCONET reference network and its infrastructure have the potential to be the backbone of the surface ocean BGC observing system.
Outlook and Recommendations
Surface Ocean CO2 NETwork is a partnership of many investigators that have as major goal measuring surface ocean CO2 and MBL CO2 levels on an operational basis following agreed upon procedures. The accurate measurements will be disseminated within a year of measurement. Platform and instrument metadata tracking would occur in near-real time. The current list of platforms and participants that expressed interest in being part of SOCONET can be found at www.aoml.noaa.gov/ocd/gcc/SOCONET. The measurements are key inputs to products addressing important social, policy, and economic issues of our time as they pertain to marine health and anthropogenic carbon sequestration. The SOCONET activities are not the sole effort of most partners who are involved in a variety of related research activities. This will facilitate interactions with other networks and research efforts. While the surface ocean and MBL measurements are automated, the data reduction and quality control for the level of accuracy required for SOCONET are labor intensive, adding to the challenges of timeliness and cost of operation of the network. From an organizational perspective, securing and maintaining resources in these international distributed networks is critical, and means need to be explored to accomplish this. This holds true particularly for the communal aspects, including network design, data tracking, and coordination. A procedure of securing equitable national contributions must be developed for SOCONET (and many other network activities as described in this volume). Working through intergovernmental entities such as JCOMM and GOOS will be of benefit. SOCONET can serve as an example how networks will transition from platform-based to EOV-based entities addressing stakeholder needs.
The recommendations evolve around the establishment of the network for accurate pCO2w and MBL CO2 measurements following GOOS/JCOMM network principles that include utilizing the approaches of technical readiness level and addressing current impediments for execution. The following recommendations for implementing SOCONET and MBL measurements are the general steps necessary to develop and maintain a sustained network of surface ocean observations:
Resource Requirements
Determine the cost and agency contributions for a sustained reference network and develop strategies to maintain such a network including common operational facilities. Sustained support for technical coordination through JCOMMOPS needs to be sought.
Labeling of All Platforms in SOCONET
Labeling is a term used in ICOS referring to a station providing the required metadata and readiness of the measurements. For SOCONET the labeling and tracking of platforms would occur through JCOMMOPS.
Protocols for Quality Control and Verification of SOCONET Data
Surface Ocean CO2 NETwork instruments should be operated with a means to verify quality though a series of steps including shoreside checks, side-by-side comparisons, crossover checks, traceable gas standards and periodic calibration or calibration checks of system components.
Network Performance Checks
The network performance would be evaluated based on number of platforms acquiring data, initial quality assessment, data loss and causes thereof, and network stability based on number of platforms and location of measurements. Implementing these checks will require creating a set of metrics based on the delivery surface ocean and MBL CO2 data to specified accuracy and density.
MBL Air Measurements From SOCONET Platforms
Verify accuracy of current systems and determine protocols to determine quality of data including use of target gases and intercomparisons. Determine if accuracy meets community needs, and assess alternative arrangements such as different sampling frequency, sensors or stand-alone systems.
Utility of Measurements
Determine and track users and uses of measurements. This includes outreach and new applications focused on societal importance. In particular determine new customers of the reference data such as those who are involved in greenhouse gas verification schemes.
Surface Ocean CO2 NETwork and associated MBL CO2 measurements is an emerging network utilizing established instruments and platforms. It is focused on applying best practices for reference quality measurements, rapid data delivery, and platform tracking. The coordinated effort should aid development of timely and routine data products delivery in support of quantifying air-sea CO2 fluxes, trends and variability in MBL and surface ocean pCO2. It should lead to positive exposure and stability of funding for all the participants who rely on national resources for operation of their systems.
Author Contributions
All authors listed have made direct and intellectual contributions to the work, and approved it for publication. RW and PP were the lead on the surface ocean and MBL components, respectively. MH performed a thorough proofreading of the galley proofs.
Funding
RW, AS, LB, DP, JT, NB, TT, RF, KO’B, and KT were supported by the NOAA office of Oceanic and Atmospheric Research, including the Ocean Observations and Monitoring Division (OOMD), funding reference #100007298 and PMEL contribution #4893. AO and SL were supported by the Norwegian Research Council (ICOS-Norway 245927). MT acknowledges support from the United States National Science Foundation grant OCE-1840868 to the Scientific Committee on Oceanic Research (SCOR, United States). US was supported by the European AtlantOS project (Grant Agreement #633211), RINGO project (Grant Agreement #730944), the United Kingdom NERC SONATA project (Grant No. NE/P021417/1), and RAGNARoCC project (Grant No. NE/K002473/1). PP was supported by the NERC SONATA project. MH was partly supported by the German Federal Ministry of Education and Research (grant no. 01LK1224I; ICOS-D). BT was supported by the Australia’s Integrated Marine Observing System. NCAR was sponsored by the United States National Science Foundation.
Conflict of Interest Statement
The authors declare that the research was conducted in the absence of any commercial or financial relationships that could be construed as a potential conflict of interest.
The reviewer WA declared a shared affiliation, with no collaboration, with one of the authors, KO’B, to the handling Editor at the time of review.
Acknowledgments
The community white manuscript is the result of merging two submitted topics for the OceanObs’19: “establishing a global surface ocean CO2 reference observing network”, and “toward including atmospheric CO2 data from the oceanic community into the global high-accuracy atmospheric CO2 network of the surface ocean”. The manuscript attempts to discuss the efforts as one entity from a scientific perspective but combining these operationally is an ongoing discussion and will depend, in part, as to what accuracy the MBL CO2 values can be determined reliably from systems measuring pCO2w. The conceptual development of a surface ocean CO2 reference network, SOCONET, and associated marine boundary layer CO2 measurements were aided by discussions at the 10th International Carbon Dioxide Conference (ICDC-10) and the 19th WMO/IAEA Meeting on Carbon Dioxide, Other Greenhouse Gases, and Related Measurement Techniques (GGMT-2017 (August 2017), and a dedicated workshop sponsored by the International Ocean Carbon Coordination Project (IOCCP) and the NOAA Ocean Observation and Monitoring Division in Portland, OR, United States (January 2018).
Supplementary Material
The Supplementary Material for this article can be found online at: https://www.frontiersin.org/articles/10.3389/fmars.2019.00400/full#supplementary-material
References
Arora, V. K., Boer, G. J., Friedlingstein, P., Eby, M., Jones, C. D., Christian, J. R., et al. (2013). Carbon–concentration and carbon–climate feedbacks in CMIP5 earth system models. J. Clim. 26, 5289–5314. doi: 10.1175/jcli-d-12-00494.1
Babenhauserheide, A., Basu, S., Houweling, S., Peters, W., and Butz, A. A. (2015). Comparing the carbon tracker and TM5-4DVar data assimilation systems for CO2 surface flux inversions. Atmos. Chem. Phys. 15, 9747–9763. doi: 10.5194/acp-15-9747-2015
Baer, D. S., Paul, J. B., Gupta, M., and O’Keefe, A. (2002). “Sensitive absorption measurements in the near-infrared region using off-axis integrated cavity output spectroscopy. In: Diode Lasers and Applications in Atmospheric Sensing,” in Proceedings of the Society of Photo-Optical Instrumentation Engineers (SPIE), ed. A. Fried (Bellingham: Spie-Int Soc Optical Engineering).
Bakker, D. C. E., Pfeil, B., Landa, C. S., Metzl, N., Olsen, A., Smith, K., et al. (2016). A multi-decade record of high-quality fCO2 data in version 3 of the surface ocean CO2 Atlas (SOCAT). Earth Syst. Sci. Data 8, 383–413. doi: 10.5194/essd-8-382016
Bates, N. R., Astor, Y. M., Church, M. J., Currie, K. I, Dore, J. E., Gonzalez–Davila, M., et al. (2014). Changing ocean chemistry: a time–series view of ocean uptake of anthropogenic CO2 and ocean acidification. Oceanography 27, 121–141. doi: 10.5670/oceanog.2014.03
Becker, M., Andersen, B., Fiedler, B., Fietzek, P., Körtzinger, A., Steinhoff, T., et al. (2012). Using cavity ringdown spectroscopy for continuous monitoring of δ13C(CO2) and fCO2 in the surface ocean. Limnol. Oceanogr. Methods 10, 752–766. doi: 10.4319/lom.2012.10.752
Bender, M., Doney, S., Feely, R. A., Fung, I., Gruber, N., Harrison, D. E., et al. (2002). A Large-Scale Carbon Observing Plan: in Situ Oceans and Atmosphere (LSCOP). Springfield: Nat. Tech. Info. Services.
Broquet, G., Chevallier, F., Bréon, F.-M., Kadygrov, N., Alemanno, M., Apadula, F., et al. (2013). Regional inversion of CO2 ecosystem fluxes from atmospheric measurements: reliability of the uncertainty estimates. Atmos. Chem. Phys. 13, 9039–9056. doi: 10.5194/acp-13-9039-2013
Chatterjee, A., Gierach, M. M., Sutton, A. J., Feely, R. A., Crisp, D., Eldering, A., et al. (2017). Influence of El Niño on atmospheric CO2 budget over the tropical Pacific Ocean: findings from NASA’s OCO-2 mission. Science 358:6360. doi: 10.1126/science.aam5776
Cheng, L., Normandeau, C., Bowden, R., Doucett, R., Gallagher, B., Gillikin, D. P., et al. (2019). An international intercomparison of stable carbon isotope composition measurements of dissolved inorganic carbon in seawater. Limnol. Oceanogr. Methods 17, 200–209. doi: 10.1002/lom3.10300
Chevallier, F., Ciais, P., Conway, T. J., Aalto, T., Anderson, B. E., Bousquet, P., et al. (2010). CO2 surface fluxes at grid point scale estimated from a global 21- year reanalysis of atmospheric measurements. J. Geophys. Res. 115:D21307. doi: 10.1029/2010JD013887
Colt, S. G., and Knapp, G. P. (2016). Economic effects of an ocean acidification catastrophe. Am. Econom. Rev. 106, 615–619. doi: 10.1257/aer.p20161105
Conway, T. J., Tans, P. P., Waterman, L. S., Thoning, K. W., Kitzis, D. R., Masarie, K. A., et al. (1994). Evidence for interannual variability of the carbon cycle from the NOAA/CMDL global air sampling network. J. Geophys. Res. 99, 22831–22855.
Crosson, E. R. (2008). A cavity ring-down analyzer for measuring atmospheric levels of methane, carbon dioxide, and water vapour. Appl. Phys. B-Lasers Opt. 92, 403–408. doi: 10.1007/s00340-008-3135-y
D’Maris, C., and Andrew, L. (2017). Carbon dioxide removal and the futures market. Environ. Res. Lett. 12:015003. doi: 10.1088/1748-9326/aa54e8
Edson, J. B., Zappa, C. J., Ware, J. A., McGillis, W. R., and Hare, J. E. (2004). Scalar flux profile relationships over the open ocean. J. Geophys. Res. 109:C08S09. doi: 10.1029/2003JC001960
El Yazidi, A., Ramonet, M., Ciais, P., Broquet, G., Pison, I., Abbaris, A., et al. (2018). Identification of spikes associated with local sources in continuous time series of atmospheric CO, CO2 and CH4. Atmos. Meas. Techn. 11, 1599–1614. doi: 10.5194/amt-11-1599-2018
Esler, M. B., Griffith, D. W. T., Wilson, S. R., and Steele, L. P. (2000). Precision trace gas analysis by FT-IR spectroscopy. 1. Simultaneous analysis of CO2, CH4, N2O, and CO in air. Anal. Chem. 72, 206–215. doi: 10.1021/ac9905625
EW Team (2005). Marine Boundary Layer Reference - NOAA Earth System Research. Available at: https://www.esrl.noaa.gov/gmd/ccgg/mbl/ doi: 10.1021/ac9905625 (accessed September 25, 2018).
Fay, A. R., and McKinley, G. A. (2014). Global open-ocean biomes: mean and temporal variability. Earth Syst. Sci. Data 6, 273–284. doi: 10.5194/essd-6-273-2014
Fay, A. R., Lovenduski, N. S., McKinley, G. A., Munro, D. R., Sweeney, C., Gray, A., et al. (2018). Utilizing the drake passage time-series to understand variability and change in subpolar Southern Ocean pCO2. Biogeosciences 15, 3841–3844. doi: 10.5194/bg-15-3841-2018
Field, C. B., Barros, V., Stocker, T. F., Dahe, Q., Mach, K. J., Plattner, G.-K., et al. (2011). IPCC WGII/WGI Workshop on Impacts of Ocean Acidification on Marine Biology and Ecosystems. Stanford CA: IPCC Working Group II Technical Support Unit, Carnegie Institution.
Frankignoulle, M., Canon, C., and Gattuso, J.-P. (1994). Marine calcification as a source of carbon dioxide: positive feedback of increasing atmospheric CO2. Limnol. Oceanogr. 39, 458–462. doi: 10.4319/lo.1994.39.2.0458
Gaubert, B., Stephens, B. B., Basu, S., Chevallier, F., Deng, F., Eric, A., et al. (2019). Global atmospheric CO2 inverse models converging on neutral tropical land exchange, but disagreeing on fossil fuel and atmospheric growth rate. Biogeosciences 16, 117–134. doi: 10.5194/bg-16-117-2019
González-Dávila, M., Santana-Casiano, J. M., and Machín, F. (2017). Changes in the partial pressure of carbon dioxide in the Mauritanian-Cap Vert upwelling region between 2005 and 2012. Biogeosciences 14:2017. doi: 10.5194/bg-14-3859-2017
Gray, A. R., Johnson, K. S., Bushinsky, S. M., Riser, S. C., Russell, J. L., Talley, L. D., et al. (2018). Autonomous biogeochemical floats detect significant carbon dioxide outgassing in the high-latitude Southern Ocean. Geophys. Res. Lett. 45, 9049–9057. doi: 10.1029/2018GL078013
Iida, Y., Kojima, A., Takatani, Y., Nakano, T., Sugimoto, Y., Midorikawa, T., et al. (2015). Trends in pCO2 and sea–air CO2 flux over the global open oceans for the last two decades. J. Oceanogr. 71:637. doi: 10.1007/s10872-015-0306-4
Jacobson, A. R., Mikaloff Fletcher, S. E., Gruber, N., Sarmiento, J. S., and Gloor, M. (2007). A joint atmosphere-ocean inversion for surface fluxes of carbon dioxide: 1. Methods and global- scale fluxes. Glob. Biogeochem. Cycles 21:GB1019. doi: 10.1029/2005GB002556
Jiang, L.-Q., Feely, R. A., Carter, B. R., Greeley, D., and Arzayus, K. M. (2015). Climatological distribution of aragonite saturation state in the global oceans. Glob. Biogeochem. Cycles 29, 1656–1673. doi: 10.1002/2015GB005198
Kamenkovich, I., Haza, A., Gray, A. R., Dufour, C. O., and Garraffo, Z. (2017). Observing system simulation experiments for an array of autonomous biogeochemical profiling floats in the Southern Ocean. J. Geophys. Res. Oceans 122, 7595–7611. doi: 10.1002/2017JC012819
Kang, J. S., Kalnay, E., Liu, J. J., Fung, I., Miyoshi, T., and Ide, K. (2011). “Variable localization” in an ensemble Kalman filter: Application to the carbon cycle data assimilation. J. Geophys. Res. Atmos. 116:D09110. doi: 10.1029/2010jd014673
Keeling, C. D. (1958). The concentration and isotopic abundances of atmospheric carbon dioxide in rural areas. Geochim. Cosmochim. Acta 13, 322–334. doi: 10.1016/0016-7037(58)90033-4
Keeling, C. D. (1965). Carbon dioxide in surface waters of the Pacific Ocean 2. Calculation of the exchange with the atmosphere. J. Geophys. Res. 70, 6099–6102. doi: 10.1029/jz070i024p06099
Keeling, R. F. (2008). Atmospheric science: recording earth’s vital signs. Science 319, 1771–1772. doi: 10.1126/science.1156761
Kozlova, E. A., and Manning, A. C. (2009). Methodology and calibration for continuous measurements of biogeochemical trace gas and O2 concentrations from a 300-m tall tower in central Siberia. Atmos. Meas. Tech. 2, 205–220. doi: 10.5194/amt-2-205-2009
Landschützer, P., Gruber, N., Bakker, D. C. E., Schuster, U., Nakaoka, S., Payne, M. R., et al. (2013). A neural network-based estimate of the seasonal to inter-annual variability of the Atlantic Ocean carbon sink. Biogeosciences 10, 7793–7815. doi: 10.5194/bg-10-7793-2013
Landschützer, P., Gruber, N., Bakker, D. C. E., and Schuster, U. (2014). Recent variability of the global ocean carbon sink. Glob. Biogeochem. Cycles 28, 927–949. doi: 10.1002/2014gb004853
Laurent, O. (2017). ICOS Atmospheric Station Specifications. Version 1.3. Available at: https://icos-atc.lsce.ipsl.fr/filebrowser/download/69422 (accessed July, 2019).
Lauvset, S. K., Gruber, N., Landschützer, P., Olsen, A., and Tjiputra, J. (2015). Trends and drivers in global surface ocean pH over the past 3 decades. Biogeosci. Discuss. 12, 1285–1298. doi: 10.5194/bg-12-1285-2015
Le Quéré, C., Andrew, R. M., Friedlingstein, P., Sitch, S., Hauck, J., Pongratz, J., et al. (2018). Global carbon budget 2018. Earth Syst. Sci. Data Discuss. 2018, 1–3. doi: 10.5194/essd-2018-120
Lee, K., Wanninkhof, R., Takahashi, T., Doney, S., and Feely, R. A. (1998). Low interannual variability in recent oceanic uptake of atmospheric carbon dioxide. Nature 396, 155–159. doi: 10.1038/24139
Li, Z., Adamec, D., Takahashi, T., and Sutherland, S. C. (2005). Global autocorrelation scales of the partial pressure of oceanic CO2. J. Geophys. Res. 110:C08002. doi: 10.1029/2004JC002723
Majkut, J. D., Carter, B. R., Frölicher, T. L., Dufour, C. O., Rodgers, K. B., and Sarmiento, J. L. (2014). An observing system simulation for Southern Ocean carbon dioxide uptake. Philos. Trans. R. Soc. A 372:20130046. doi: 10.1098/rsta.2013.0046
Merlivat, L., Boutin, J., and d’Ovidio, F. (2015). Carbon, oxygen and biological productivity in the Southern Ocean in and out the Kerguelen plume: CARIOCA drifter results. Biogeosci. Discuss. 12, 3513–3524. doi: 10.5194/bg-12-3513-2015
Monteiro, P., Schuster, U., Hood, M., Lenton, A., Metzl, N., Olsen, A., et al. (2010). “A global sea surface carbon observing system: Assessment of changing sea surface CO2 and air-sea CO2 fluxes,” in Sustained Ocean Observations and Information for Society, Vol. 2, eds J. Hall and D. Stammer (Venice: ESA Publication).
Müller, J. D., Schneider, B., and Rehder, G. (2016). Long-term alkalinity trends in the Baltic Sea and their implications for CO2-induced acidification. Limnol. Oceanogr. 61, 1984–2002. doi: 10.1002/lno.10349
Northcott, D., Sevadjian, J., Sancho-Gallegos, D. A., Wahl, C., Friederich, J., and Chavez, F. P. (2019). Impacts of urban carbon dioxide emissions on sea-air flux and ocean acidification in nearshore waters. PLoS One 6:115. doi: 10.1371/journal.pone.0214403
National Center for Atmospheric Research Staff [NCAR] (ed.) (2013). The Climate Data Guide: Taylor Diagrams. Available at: http//climatedataguide.ucar.(edu)/climate-data-tools-and-analysis/taylor-diagrams (accessed July 23, 2013).
Oliver, M. J., and Irwin, A. J. (2008). Objective global ocean biogeographic provinces. Geophys. Res. Let. 35:L15601. doi: 10.1029/2008GL034238
Park, G.-H., Wanninkhof, R., Doney, S. C., Takahashi, T., Richard, K. L., Feely, A., et al. (2010). Variability of global net sea-air CO2 fluxes over the last three decades using empirical relationships. Tellus 62B, 352–368. doi: 10.1111/j.1600-0889.2010.00498.x
Peters, W., Jacobson, A. R., Sweeney, C., Andrews, A. E., Conway, T. J., Masarie, K., et al. (2007). An atmospheric perspective on North American carbon dioxide exchange: carbonTracker. Proc. Natl. Acad. Sci. U.S.A. 104, 18925–18930. doi: 10.1073/pnas.0708986104
Peters, G. P., Le Quéré, C., Andrew, R. M., Canadell, J. G., Friedlingstein, P., Ilyina, T., et al. (2017). Towards real-time verification of CO2 emissions. Nat. Clim. Change 7, 848–850. doi: 10.1038/s41558-017-0013-9
Pfeil, B., Olsen, A., Bakker, D. C. E., Hankin, S., Koyuk, H., Kozyr, A., et al. (2013). A uniform, quality controlled Surface Ocean CO2 Atlas (SOCAT). Earth Syst. Sci. Data 5, 125–143.
Pickers, P. A. (2016). New Applications of Continuous Atmospheric O2 Measurements: Meridional Transects Across the Atlantic Ocean, and Improved Quantification of Fossil Fuel- Derived CO2. Ph.D. thesis, School of Environmental Sciences, University of East Anglia, Norwich. Available at: http://cramlab.uea.ac.uk/Documents/Pickers_Penelope_PhD_Thesis_2016.pdf
Pickers, P. A., Manning, A. C., Sturges, W. T., Quéré, C., Fletcher, S. E. M., Wilson, P. A., et al. (2017). In situ measurements of atmospheric O2 and CO2 reveal an unexpected O2 signal over the tropical Atlantic Ocean. Glob. Biogeochem. Cycles 31, 1289–1305. doi: 10.1002/2017gb005631
Pierrot, D., Neil, C., Sullivan, K., Castle, R., Wanninkhof, R., Lueger, H., et al. (2009). Recommendations for autonomous underway pCO2 measuring systems and data reduction routines. Deep Sea Res. II 56, 512–522. doi: 10.1016/j.dsr2.2008.12.005
Revelle, R., and Suess, H. (1957). Carbon dioxide exchange between atmosphere and ocean and the question of an increase of atmospheric CO2 during the past decades. Tellus 9, 18–27. doi: 10.3402/tellusa.v9i1.9075
Rödenbeck, C., Conway, T. J., and Langenfelds, R. L. (2006). The effect of systematic measurement errors on atmospheric CO2 inversions: a quantitative assessment. Atmos. Chem. Phys. 6, 149–161. doi: 10.5194/acp-6-149-2006
Rödenbeck, C. D., Bakker, C. E., Gruber, N., Iida, Y., Jacobson, A. R., Jones, S., et al. (2015). Data-based estimates of the ocean carbon sink variability –first results of the Surface Ocean pCO2 Mapping intercomparison (SOCOM). Biogeosciences 12, 7251–7278. doi: 10.5194/bg-12-7251-2015
Salisbury, J., Vandemark, D., Jönsson, B., Balch, W., Chakraborty, S., Lohrenz, S., et al. (2015). How can present and future satellite missions support scientific studies that address ocean acidification? Oceanography 28, 108–121. doi: 10.5670/oceanog.2015.35
Schneider, B., and Müller, J. D. (2018). Biogeochemical Transformations in the Baltic Sea: Observations Through Carbon Dioxide Glasses. Berlin: Springer.
Schuster, U., and Watson, A. J. (2007). A variable and decreasing sink for atmospheric CO2 in the North Atlantic. J. Geophys. Res. 112:C11006.
Shutler, J. D., Wanninkhof, R., Nightingale, P. D., Woolf, D. K., Bakker, D. C. E., and Watson, A. J., et al. (2019). Rediscovering the ocean carbon sink: satellites will enable us to watch the global oceans breathe. Front. Ecol. Environ.
Sutton, A. J., Sabine, C. L., Maenner-Jones, S., Lawrence-Slavas, N., Meinig, C., Feely, R. A., et al. (2014). A high-frequency atmospheric and seawater pCO2 data set from 14 open-ocean sites using a moored autonomous system. Earth Syst. Sci. Data 6, 353–366. doi: 10.5194/essd-6-353-2014
Sutton, A. J., Feely, R. A., Maenner-Jones, S., Musielwicz, S., Osborne, J., Dietrich, C., et al. (2019). Autonomous seawater pCO2 and pH time series from 40 surface buoys and the emergence of anthropogenic trends. Earth Syst. Sci. Data 11, 421–439. doi: 10.5194/essd-11-421-2019
Takahashi, T. (1961). Carbon dioxide in the atmosphere and in Atlantic Ocean water. J. Geophys. Res. 66, 477–494. doi: 10.1029/JZ066i002p00477
Takahashi, T., Steawart, C. S., Rik, W., Colm, S., Richard, A. F., David, W. C., et al. (2009). Climatological mean and decadal change in surface ocean pCO2, and net sea-air CO2 flux over the global oceans. Deep Sea Res. II 2009, 554–577. doi: 10.1016/j.dsr2.2008.12.009
Takahashi, T., Sutherland, S. C., Chipman, D. W., Goddard, J. C., Ho, C., Newberger, T., et al. (2014). Climatological distributions of pH, pCO2, total CO2, alkalinity, and CaCO3 saturation in the global surface ocean, and temporal changes at selected locations. Mar. Chem. 164, 95–125. doi: 10.1016/j.marchem.2014.06.004
Taylor, K. E. (2001). Summarizing multiple aspects of model performance in a single diagram. J. Geophys. Res. 106, 7183–7192. doi: 10.1029/2000JD900719
Wanninkhof, R., Barbero Pierrot, D., Shuster, U., Tedesco, K., Sutton, A., and Telszewski, M. (2018). A Surface Ocean CO2 Observing Network, SOCONET, Prospectus. Available at: www.aoml.noaa.gov/ocd/gcc/SOCONET/SOCONET_prospectus.pdf (asccessed February 12, 2019).
Wanninkhof, R. (2014). Relationship between wind speed and gas exchange over the ocean revisited. Limnol. Oceanogr. Methods 12, 351–362. doi: 10.4319/lom.2014.12.351
Wanninkhof, R., Feely, R., Sutton, A., Sabine, C., Tedesco, K., Gruber, N., et al. (2012). “An integrated ocean carbon observing system (IOCOS),” in Proceedings, U.S. Integrated Ocean Observing System (IOOS) Summit, Interagency Ocean Observation Committee (IOOC). Herndon, VA (accessed November 13–16, 2012).
Weart, S. R. (2008). The Discovery of Global Warming, Revised and Expanded Edition. Cambridge: Harvard University Press.
Williams, N. L., Juranek, L. W., Feely, R. A., Johnson, K. S., Sarmiento, J. L., Talley, L. D., et al. (2017). Calculating surface ocean pCO2 from biogeochemical Argo floats equipped with pH: An uncertainty analysis. Glob. Biogeochem. Cycles 31, 591–604. doi: 10.1002/2016GB005541
WMO/GAW Report 229 (2016). Report from the 18th WMO/IAEA Meeting on Carbon Dioxide, Other Greenhouse Gases and Related Measurement Techniques (GGMT-2015). La Jolla, CA: WMO.
Zappa, C. J., Asher, W. E., Jessup, A. T., Klinke, J., and Long, S. R. (2004). Microbreaking and the enhancement of air-water transfer velocity. J. Geophys. Res. 109:C08S16. doi: 10.1029/2003JC001897
Zellweger, C., Emmenegger, L., Firdaus, M., Hatakka, J., and Heimann, M. (2016). Assessment of recent advances in measurement techniques for atmospheric carbon dioxide and methane observations. Atmos. Meas. Tech. 9, 4737–4757. doi: 10.5194/amt-9-4737-2016
Zeng, J., Matsunaga, T., Saigusa, N., Shira, T., Nakaoka, S.-I., and Tan, Z.-H. (2017). Technical note: Evaluation of three machine learning models for surface ocean CO2 mapping. Ocean Sci. 13, 303–313. doi: 10.5194/os-13-303-2017
Appendix A: Ocean Acidification and Pco2
A direct impact of increasing pCO2 levels in the ocean is the phenomenon of ocean acidification. While the definitions of OA vary to some extent most are in line with the following: “Ocean acidification refers to a reduction in the pH of the ocean over an extended period of time, typically decades or longer, which is caused primarily by uptake of carbon dioxide (CO2) from the atmosphere.” (Field et al., 2011). With increasing emphasis on changes in ocean inorganic carbon chemistry in addition to pH decrease, the definition is broadened to: “reduction of seawater pH and changes ocean chemistry that are collectively referred to as ocean acidification.”
Surface Ocean CO2 NETwork thus addresses the key forcing components of OA involving the uptake of anthropogenic CO2 and changing surface ocean CO2. There is a strong correlation between pCO2 and pH as can be seen from the hydration reaction and dissociation of CO2 summarized by the chemical equation:
The changes in inorganic carbon speciation can impact the biogeochemical and biological responses. In particular, increasing CO2 concentrations lower carbonate ion concentrations through the major net buffering reaction in the oceanic inorganic carbon system that can be summarized as:
An example of the correlation between pCO2 and pH for surface water is shown in Supplementary Figure S1 using surface ocean measurements from a GO-SHIP cruise P18 in the SE Pacific. The strong correspondence is apparent, and deviations from a singular relationship are due to differences in the buffering of the seawater that will impact the equilibria in A1 and A2.
Increasing CO2 leads to a decrease in carbonate levels and resulting decrease of calcium carbonate saturation state (Bates et al., 2014). This is of concern for calcifying organisms that are abundant in the ocean. The biological production of corals, as well as calcifying phytoplankton and zooplankton will be inhibited or slowed. The dissolution of biotic and abiotic calcium carbonate in the water column and the ocean floor will be enhanced.
Species containing aragonite, and meta-stable forms of calcium carbonate produced by corals and plankton, such as pteropods will be particularly susceptible to a reduction of CO32- in seawater.
Ocean acidification also impacts organisms that do not fix calcium carbonate. Increasing seawater CO2 levels and lower pH can weaken metabolic processes for organisms, from feeding to respiration to reproduction and change the chemical speciation of trace metals essential for their needs. While predicting the precise response of ocean ecosystems is challenging for scenarios of increased CO2 levels, it is likely the ecosystems will be less productive, less diverse and less resilient. In addition, the synergistic impacts of other climate and human impacts on the ocean, including ocean warming and de-oxygenation, will exacerbate the impacts of elevated CO2 levels and associated acidification. SOCONET data will provide critical input on the trends of the major factors influencing OA and air-sea CO2 fluxes, and resulting decreases in pH and carbonate levels.
Keywords: carbon dioxide, network, oceanography, fluxes, best practices
Citation: Wanninkhof R, Pickers PA, Omar AM, Sutton A, Murata A, Olsen A, Stephens BB, Tilbrook B, Munro D, Pierrot D, Rehder G, Santana-Casiano JM, Müller JD, Trinanes J, Tedesco K, O’Brien K, Currie K, Barbero L, Telszewski M, Hoppema M, Ishii M, González-Dávila M, Bates NR, Metzl N, Suntharalingam P, Feely RA, Nakaoka S-i, Lauvset SK, Takahashi T, Steinhoff T and Schuster U (2019) A Surface Ocean CO2 Reference Network, SOCONET and Associated Marine Boundary Layer CO2 Measurements. Front. Mar. Sci. 6:400. doi: 10.3389/fmars.2019.00400
Received: 21 November 2018; Accepted: 27 June 2019;
Published: 12 July 2019.
Edited by:
Laura Lorenzoni, University of South Florida, United StatesReviewed by:
William Asher, University of Washington, United StatesRodrigo Kerr, Fundação Universidade Federal do Rio Grande, Brazil
Copyright © 2019 Wanninkhof, Pickers, Omar, Sutton, Murata, Olsen, Stephens, Tilbrook, Munro, Pierrot, Rehder, Santana-Casiano, Müller, Trinanes, Tedesco, O’Brien, Currie, Barbero, Telszewski, Hoppema, Ishii, González-Dávila, Bates, Metzl, Suntharalingam, Feely, Nakaoka, Lauvset, Takahashi, Steinhoff and Schuster. This is an open-access article distributed under the terms of the Creative Commons Attribution License (CC BY). The use, distribution or reproduction in other forums is permitted, provided the original author(s) and the copyright owner(s) are credited and that the original publication in this journal is cited, in accordance with accepted academic practice. No use, distribution or reproduction is permitted which does not comply with these terms.
*Correspondence: Rik Wanninkhof, cmlrLndhbm5pbmtob2ZAbm9hYS5nb3Y=