- 1Environmental Epigenetics Laboratory, Department of Biological Sciences, Center for Coastal Oceans Research, Institute of Water and Environment, Florida International University, North Miami, FL, United States
- 2Department of Biological Sciences, Florida International University, North Miami, FL, United States
- 3Chicago Zoological Society’s Sarasota Dolphin Research Program, c/o Mote Marine Laboratory, Sarasota, FL, United States
Age constitutes a critical parameter for the study of animal populations, providing information about development, environmental effects, survival, and reproduction. Unfortunately, age estimation is not only challenging in large, mobile and legally protected species, but often involves invasive sampling methods. The present work investigates the association between epigenetic modifications and chronological age in small cetaceans. For that purpose, DNA methylation at age-linked genes was characterized in an extensively studied, long-term resident common bottlenose dolphin (Tursiops truncatus) community from Sarasota Bay (FL, United States) for which sampled individuals have a known age. Results led to the identification of several CpG sites that are significantly correlated to chronological age in this species with the potential for sex to play a role in the modulation of this correlation. These findings have allowed for the development and validation of the “Bottlenose dolphin Epigenetic Age estimation Tool” (BEAT), improving minimally-invasive age estimation in free-ranging small cetaceans. Overall, the BEAT proved to be accurate in estimating age in these organisms. Given its minimally-invasive nature and potential large-scale implementation using skin biopsy samples, this tool can be used to generate age data from free-ranging small cetacean populations.
Introduction
Dolphins and porpoises are small cetaceans that occur in all marine habitats around the globe, and are threatened by a wide range of human activities (Pompa et al., 2011; Davidson et al., 2012). Common bottlenose dolphins (Tursiops truncatus), the model organism used in this study, are wide-ranging animals (Dans et al., 2010; Wells and Scott, 2018) occurring in estuarine, coastal, shelf, and oceanic habitats in temperate, subtropical, and tropical waters around the globe. Unfortunately, because small cetaceans often associate in coastal or near coastal areas, they often are exposed to multiple concurrent anthropogenic stressors (e.g., pollution, boat traffic, bycatch in fishing gears, etc.) with potentially significant impacts on populations. These species are often difficult to study, and access to carcasses to collect biological data is relatively limited, particularly for wild migratory populations. Such problem has been alleviated by the progressive implementation of remote collection biopsies over the past two decades, improving our understanding of the population structure, feeding ecology, and ecotoxicology of free-ranging small cetacean populations from around the world (Fossi et al., 2000; Torres et al., 2003; Kiszka et al., 2011). Yet, the accurate estimation of chronological age, a critical population parameter, remains elusive from biopsy samples in these organisms.
Age constitutes a critically important parameter for population studies. Within a given population, several aspects of the biology of species can be investigated, including reproductive success, number of individuals of reproductive age, and the age composition of the population (Campana, 2001; Westgate and Read, 2007). In addition, age can be correlated to a variety of parameters, including pollutant loads, foraging tactics, and social structure. However, it is notoriously difficult to determine the age of bottlenose dolphins (as well as many other marine organisms), partly because they do not have conclusive external phenotypic traits that can be visually associated with age (Wilson et al., 1997; Miller et al., 2010; Hartel et al., 2015).
Current methods to estimate age in dolphins and other marine mammals include the use of body size, although this generally provides a rough estimation of the age group in which the animal may be included [e.g., adolescent versus adult (Baker et al., 2018)]. Another very accurate method includes removal of a tooth by a veterinarian, under local anesthesia, longitudinal sectioning, and quantification of growth layer groups in the tooth (International Whaling Commission, 1980; Hohn et al., 1989). This approach requires capture and handling of animals, which may not be feasible or desirable in some cases such as with endangered populations. Cost-effective, efficient, less-invasive alternative methods for obtaining accurate age estimates for large numbers of animals, without the need for capture, would be very useful. Biopsy dart sampling is a well-established technique for obtaining small tissue samples from cetaceans (Barrett-Lennard et al., 1996; Krutzen et al., 2002), and efforts have been made to use such samples for age estimation. Initial work has included the analysis of chromosomal telomere length and lipid accumulation in skin samples of cetaceans (Herman et al., 2009; Olsen et al., 2012). These techniques are limited by the strong influence of environmental conditions and for displaying a very wide range of values across individuals of similar chronological age. More specifically, telomere analyses can also be biased by the high variability in telomere lengths displayed by cetaceans (Olsen et al., 2012), as well as by its correlation with paternal age (Kappei and Arturo Londoño-Vallejo, 2008; Broer et al., 2013).
Complementary to traditional molecular analyses, the study of epigenetics [i.e., the study of phenomena and mechanisms that cause chromosome-bound, heritable (mitotically and/or meiotically) changes to gene expression that are not dependent on changes to DNA sequence (Deans and Maggert, 2015)] is emerging as a very promising framework to estimate chronological and biological age from DNA samples. Epigenetic age estimation (hereinafter referred to as aging) is based on the analysis of DNA methylation (addition of a methyl group to a Cytosine in the DNA sequence to form 5-methylcytosine). This modification is found at 60–80% of CpG sites (where a cytosine, C, is next to a guanine, G, in the DNA sequence) across vertebrate genomes (Jones, 2001, 2012) both at gene bodies and promoters (Deaton and Bird, 2011), participating in the regulation of gene expression in response to developmental and environmental signals (Eirin-Lopez and Putnam, 2018). Most importantly, it has been shown that the %DNA methylation at specific positions of certain genes correlates linearly with chronological age, making age prediction reliable as demonstrated in the case of mammals including humans (Grönniger et al., 2010; Polanowski et al., 2014), as well as in other vertebrates (Paoli-Iseppi et al., 2019). This property has boosted the development of epigenetic aging tools that are now being successfully used in human forensics (Horvath, 2013; Shabani et al., 2018) as well as in marine organisms (Polanowski et al., 2014; Eirin-Lopez and Putnam, 2018).
In the present study we investigate the relationship between epigenetic modifications in age-responsive genes and chronological age in mammals, and their potential to develop an age evaluation tool for bottlenose dolphins and potentially other small cetacean species. For that purpose, genes displaying age-influenced changes in DNA methylation (GRIA2, TET2, and CDKN2A) were examined, in agreement with previous reports on humpback whales (Polanowski et al., 2014). In addition, we explored the potential for sex-specific differences in aging.
Materials and Methods
Sample Collection
Skin samples from 39 bottlenose dolphins collected from June 2004 through May 2009 by the Sarasota Dolphin Research Program (Sarasota, FL, United States), as part of capture-release health assessments of the long-term resident Sarasota Bay dolphin community (Wells et al., 2004, 2005) were used. This community has been studied since 1970, through at least six generations, and at any given time includes up to five concurrent generations within a single maternal lineage, and individuals up to 67 years of age (Irvine and Wells, 1972; Wells, 2014). As of 2015, the community was composed of 157.53 ± 43.05 SE dolphins (Tyson and Wells, 2016). In combination, health assessments, tagging and tracking, and monthly systematic photographic identification surveys have provided background information on the members of the resident community, including age, sex, genetic relationships, morphometrics, health, tissue concentrations of environmental contaminants, and reproductive histories (Wells, 2009). Skin samples were chosen as model biological material in the present study given the ability to perform samplings in large numbers of individuals in a minimally-invasive manner. This is further supported by previous reports describing age-related changes in DNA methylation in mammals including humans and humpback whales using skin tissue (Grönniger et al., 2010; Polanowski et al., 2014). Skin samples were obtained as subsamples of wedge-shaped surgical biopsies of blubber and skin collected under local anesthesia. The samples were frozen immediately in liquid nitrogen. Samples were stored at −80°C. Overall, the samples analyzed were chosen to represent a group consisting of 15 females and 24 males with an even age distribution between sexes ranging from 2 to 36 years of age. However, for both males and females, fewer older animals were sampled than younger. Sex was determined by observation during capture-release efforts (see Figure 1 and Supplementary File S1).
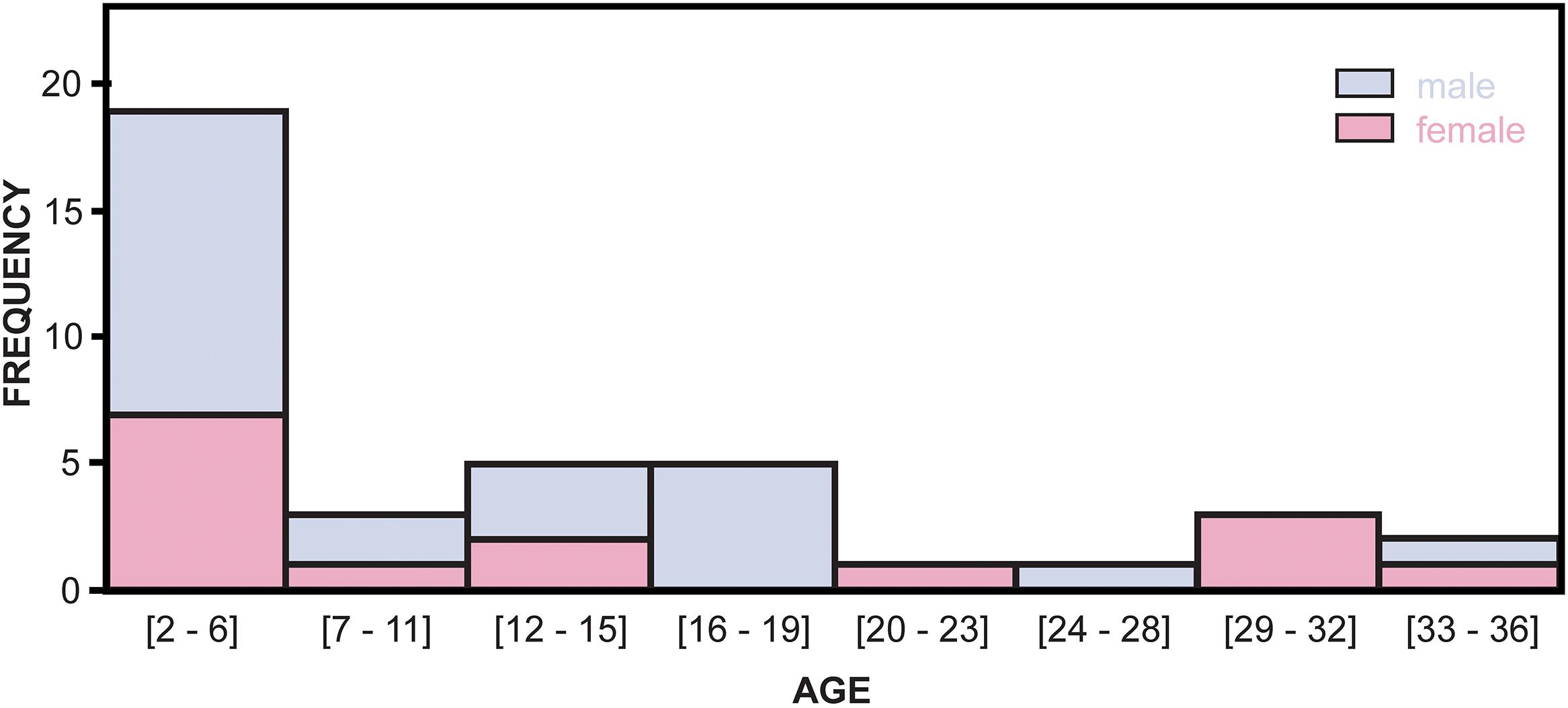
Figure 1. Age distribution of male and female dolphin individuals used for the development of the BEAT tool.
DNA Extraction
DNA was extracted from skin samples using a phenol/chloroform extraction protocol, adapted from Sambrook et al. (1989). Accordingly, a small piece of skin (<2 mm) was cut into small pieces and placed in 500 μL of CTAB lysis buffer (100 mM Tris, 20 mM EDTA, 1.2M NaCl, 2% CTAB, pH 8.0) with 10 μL of proteinase K and 40 μL of Dithiothreitol (DTT). Samples were digested overnight at 50°C in a water bath, and subsequently vortexed and mixed with 600 μL of 4°C phenol/chloroform/isoamyl alcohol (25:24:1 mixture, Fisher BioReagents). Samples were vortexed again and centrifuged at 12,000 g, 4°C for 5 min. Approximately, 90% of the supernatant was removed and transferred to a new tube. Two additional cycles of extraction with phenol/chloroform/isoamyl alcohol were performed, placing the resulting supernatant fraction on a new tube and precipitating the DNA using 50 μL of ammonium acetate and 980 μL of 99% isopropyl alcohol at 4°C. After an overnight incubation on ice, samples were vortexed and centrifuged at 12,000 g, 4°C for 30 min to form a pellet. The isopropyl alcohol was subsequently removed and the pellet was subject to two consecutive washes using ice-cold 70% ethanol. The precipitate was completely dried using a vacuum centrifuge, DNA quality was assessed using agarose gel electrophoresis, and DNA concentration was calculated using Qubit fluorometric quantitation (Thermo Fisher Scientific).
Quantification of %DNA Methylation at CpG Sites
Freshly extracted DNA was divided into 500 ng aliquots (20 ng/μL) and subsequently subject to bisulfite transformation followed by pyrosequencing (EpigenDx, Hopkinton, MA, United States) to identify the %DNA methylation at individual CpG sites, based on multiple DNA copies. Pyrosequencing yields approximately 20X coverage of each target sequence. Age-responsive candidate genes (TET2, GRIA2, and CDKN2A) were targeted. The selection of these genes was informed by previous reports identifying age-responsive genes in humans and particularly in a large cetacean species, the humpback whale (Polanowski et al., 2014).
Dolphin ortholog sequences for genes TET2, GRIA2, and CDKN2A were retrieved from the dolphin genome database (McGowen et al., 2012; Martinez-Viaud et al., 2019) and used to design primers using PyroMark software (Qiagen), specifically targeting most CpG sites present within the promoter sequences for these target genes (TET2, n = 4; GRIA2, n = 7; and CDKN2A, n = 6). Since CDKN2A is not annotated in the bottlenose dolphin genome, the killer whale (Orcinus orca) genome was used to verify this ortholog using the target sequence from the HEAA (Polanowski et al., 2014). The target sequences used and the CpG site location in relation to the start codon are detailed in Table 1 along with an explanation of CpG site labeling for this study. The %DNA methylation of gene promoters was calculated based upon the amount of fluorescence produced by PCR amplifications of pyrosequencing products at the targeted CpG sites. As a result, these analyses provide a final %DNA methylation for each of the samples at each of the CpG sites targeted for the three genes.
Statistical Analyses of BEAT Accuracy and Precision
Simple linear regression analyses were performed for each CpG site of each age-responsive gene using chronological age as the independent known variable and %DNA methylation for each CpG site at the different age-responsive genes as the dependent variable using R software (R Core Team, 2013, Version 3.5.2; R codes are included in Supplementary File S2). For each linear regression analysis, the coefficient of determination (R2) was calculated, representing the percentage of the variation in the dependent variable (%DNA methylation) that is explained by the independent variables. After simple linear regression was performed for all CpG sites, multiple regression analyses were conducted. All possible combinations of significantly correlated CpG sites were tested. To avoid the effect of concerted DNA methylation changes among CpG sites on the same gene regions (Grönniger et al., 2010) only one CpG site from each gene was used in the combinations. This approach follows up on the strategy currently used for developing epigenetic age assays in other mammalian species (Koch and Wagner, 2011; Polanowski et al., 2014). Best models were defined based on Akaike information criterion (AIC) and Bayesian information criterion (BIC). The contribution of sex-specific traits to the epigenetic estimation of chronological age was also studied by discriminating between male and female samples in the analysis of %DNA methylation as a function of age. Thus, simple linear regression analyses for male and female datasets were additionally performed (see Supplementary Files S2, S3). The differences in the best epigenetic aging models obtained for male and female only analyses were evaluated using an analysis of covariance (ANCOVA) using R software (α = 0.05). Lastly, the overall epigenetic aging model (including males + females) was validated through multiple regression analysis using the Leave-One-Out Cross Validation (LOOCV) method (Picard and Dennis Cook, 1984). This approach recurrently trains the model using all but one sample each time, until all samples had been left out once thereby predicting a value for each datapoint without using it to train the model.
Results
CpG Methylation at Age-Responsive Genes in Bottlenose Dolphins
Overall, a total of 17 CpG sites were obtained across the three candidate genes targeted (TET2, n = 4; GRIA2, n = 7; CDKN2A, n = 6) using pyrosequencing. The total %DNA methylation for all CpG sites at each of the genes analyzed showed a relatively low %DNA methylation, with gene average methylation falling between 1.6 and 12.2, with the highest percentage at a given site being 24.9 for an individual CpG site at the promoter of CDKN2A_4. This was for a 2 year old female. The lowest amount of methylation was the absence of DNA methylation observed at several different sites for some individuals (Supplementary File S1). In addition, average %DNA methylation levels were calculated for each individual across all CpG sites for each gene. The individual with the highest average methylation for each gene was as follows: GRIA2 = female 189 age 32 (10.85 ± 4.40); CDKN2A = female 189 age 32 (10.30 ± 2.80); TET2 = female 217 age 2 (12.25 ± 5.20). Similarly, the lowest averages were: GRIA2 = male 256 age 2 (1.60 ± 1.60); CDKN2A = male 252 age 3 (7.00 ± 3.30); TET2 = female 193 age 21 (4.30 ± 3.00). The average %DNA methylation was also calculated for each gene, using average values across individuals for each gene. In this case, CDKN2A displayed the highest overall average (8.45 ± 3.48; minimum: 4.35, maximum: 13.32), followed by TET2 (8.10 ± 3.32; minimum: 3.86, maximum: 11.23) and GRIA2 (3.96 ± 1.78; minimum: 2.12, maximum: 6.91; Supplementary File S1). Globally, bottlenose dolphins displayed %DNA methylation levels at CpG sites in GRIA2 and CDKN2A genes similar to those displayed by humpback whales (Polanowski et al., 2014). Oppositely, %DNA methylation at TET2 CpG sites were lower by almost an order of magnitude in bottlenose dolphins (8.03 ± 1.850, compared with humpback whales 14.630 ± 4.070; Table 2).

Table 2. Comparison between %DNA methylation levels at studied CpG sites in the BEAT and the HEAA models.
Correlation Between DNA Methylation and Chronological Age
Simple regression analyses of CpG methylation as a function of chronological age revealed that, out of the 17 CpG sites identified, only 4 of them (TET2_4, CDKN2A_2, CDKN2A_4, CDKN2A_6) were not significantly correlated with age, and therefore excluded from subsequent analyses (see Table 3 for all R2 values, see Figure 2 for top correlated CpG site graphs). Multiple regression analyses were then conducted for all possible combinations of CpG sites displaying significant correlation with age, using one CpG site from each of the genes to ensure that independent age estimates were being made. This approach was implemented to account for the observations made in other mammals that CpG sites within a gene tend to be strongly correlated to one another, more often than CpG sites from different genes possibly because of similar processes affecting multiple CpG sites across a single gene (Koch and Wagner, 2011; Polanowski et al., 2014).
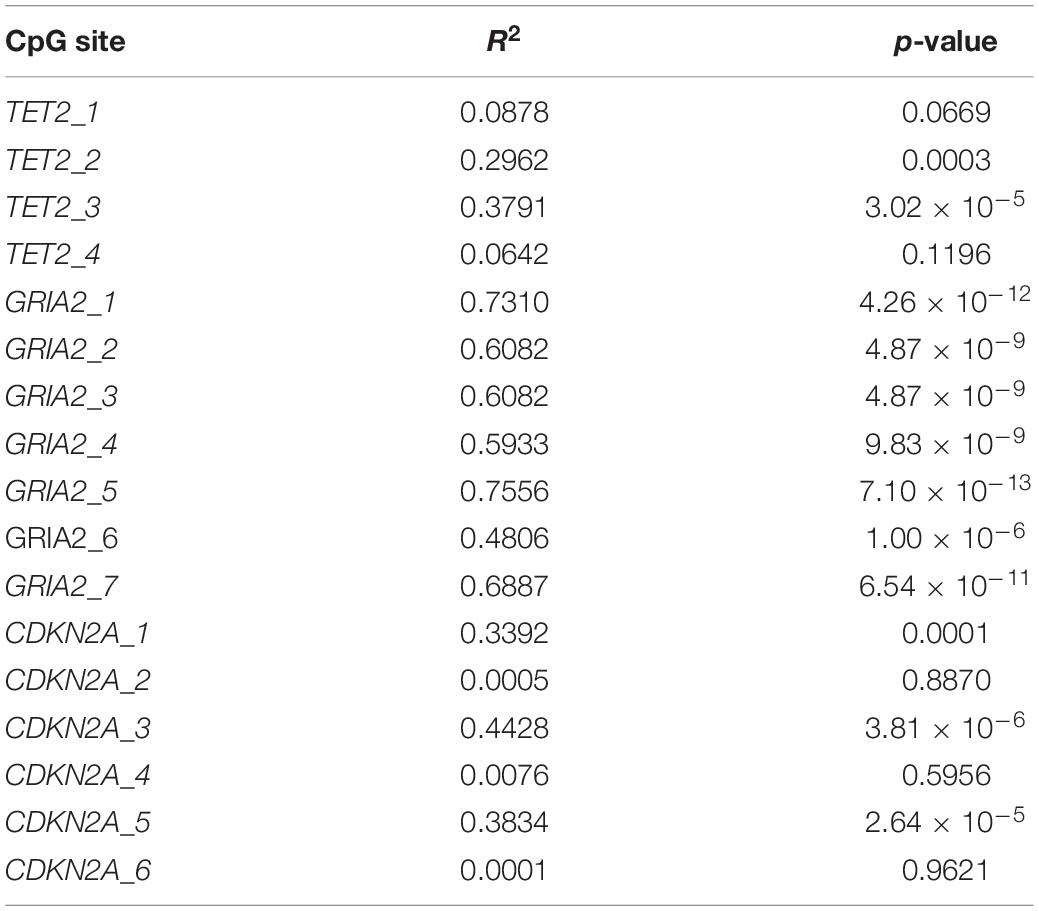
Table 3. CpG sites identified at TET2, GRIA2, and CDKN2A genes, along with R2 and p-values obtained in simple linear regression analyses.
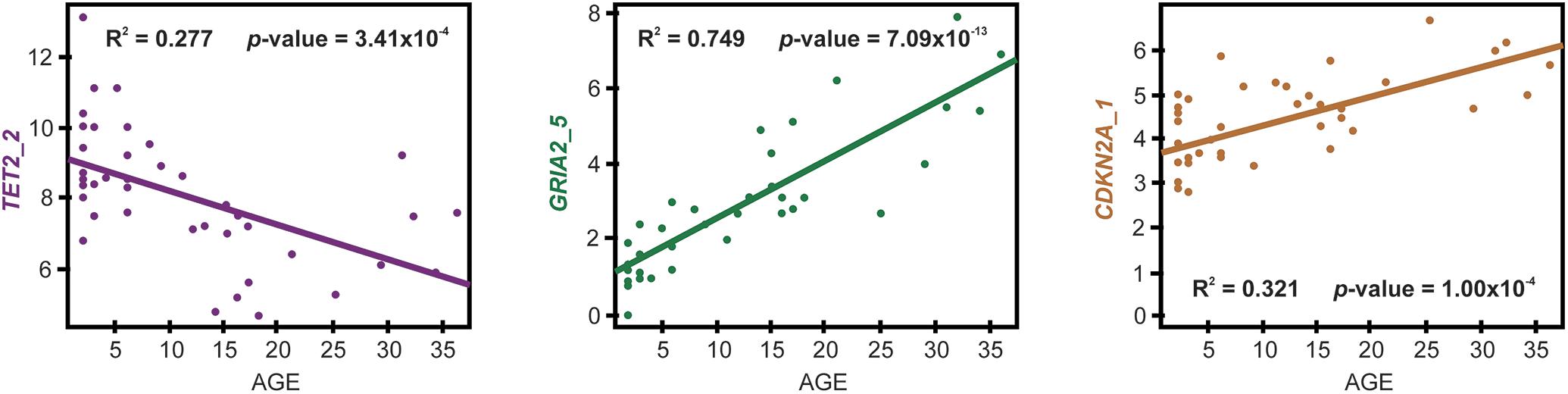
Figure 2. CpG sites displaying the strongest correlation with chronological age across dolphin individuals in each of the three genes studied.
Following multiple linear regression analysis, the top model (based on the lowest AIC and BIC and highest R2 values, R2 = 0.779, residual error = 4.83, p < 0.001; Table 4) included two genes, GRIA2 CpG site 5 (GRIA2_5) and TET2 CpG site 2 (TET2_2), and is hereafter referred to as the BEAT (Bottlenose dolphin Epigenetic Aging Tool). These two CpG sites displayed the strongest correlation with chronological age among all CpG sites studied in these two genes (Figure 2). Full details on the other top models from multiple regression analysis (along with AIC and BIC scores), are shown in Table 4. The validation of the best model using the LOOCV method yielded predicted points at R2 = 0.740 (root mean square error = 5.14) which were then plotted against the known age values, and had a strong correlation (R2 = 0.780; Figure 3). The following equation created from the multiple regression analysis is as follows: Age estimate = 8.602 + 4.392 (%DNA GRIA_5) − 1.149 (%DNA TET2_2).
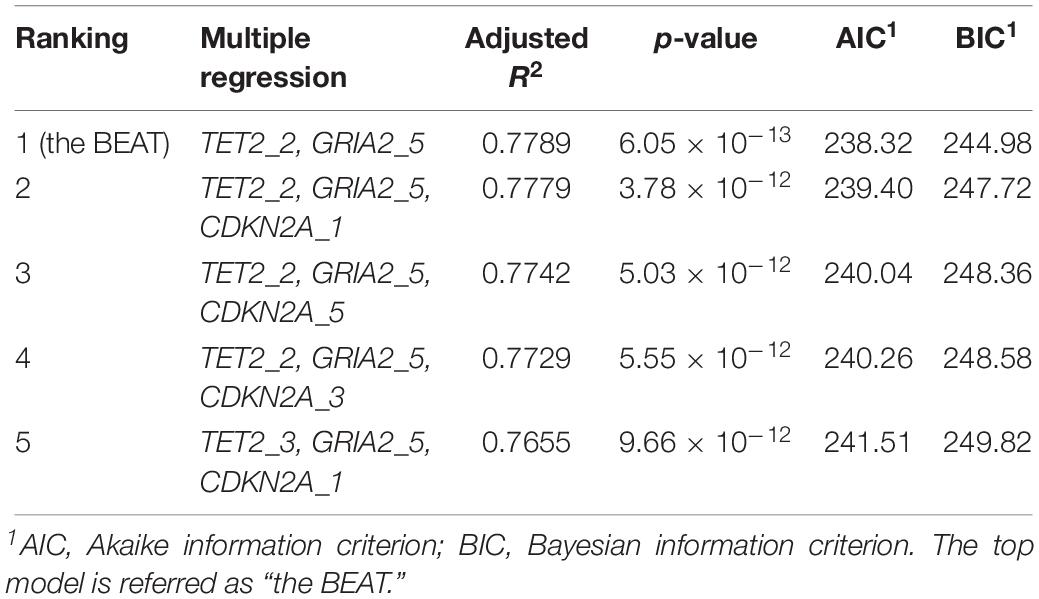
Table 4. Ranked list of the models best describing the relationship between %DNA methylation at CpG sites studied for TET2, GRIA2, and CDKN2A genes as a function of chronological age in bottlenose dolphins (male and female combined).
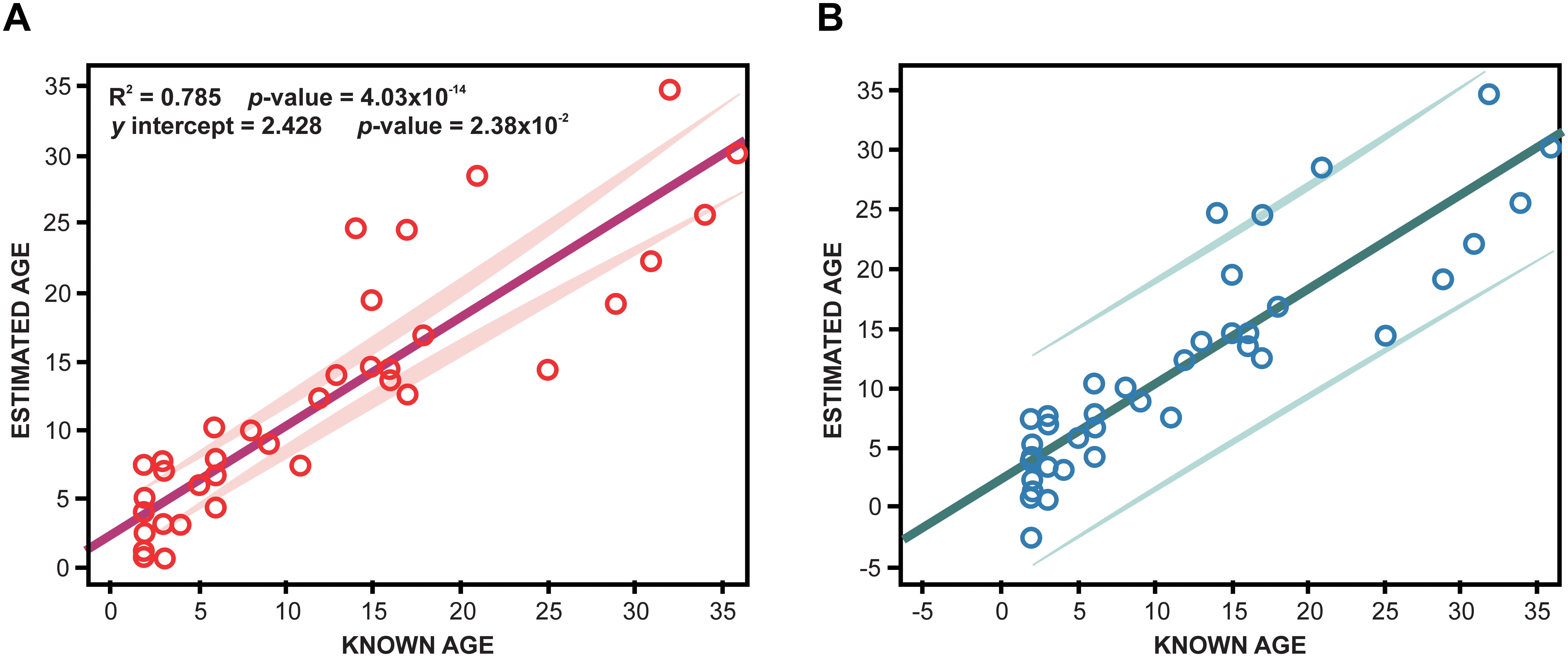
Figure 3. Analysis of the precision of the BEAT model using (A) multiple regression analysis (R2 = 0.785) and (B) its validation using LOOCV analysis (bottom graph, R2 = 0.740). In (A), the estimated age values were calculated for each individual using the equation generated by Multiple Regression analysis using all individuals to generate the model. In (B), estimated age values were calculated for each individual using the equation generated by multiple regression analysis with the individual being estimated left out (see section “Materials and Methods”).
The contribution of the sex of dolphin individuals to the epigenetic estimation of chronological age was evaluated using analysis of covariance (ANCOVA). While the results revealed a lack of significant differences between male and female changes in DNA methylation with age for the BEAT (p = 0.760), simple linear regression analyses performed using only female and male datasets revealed differences for the two CpG sites used in the BEAT model (Figure 4 and Supplementary File S3 for all other sites). Most importantly, for site GRIA2_5, the slopes for males and females deviate from one another at older ages. For site TET2_2 we see that both male and female slopes are similar but males tend to have much less methylation than females. ANCOVA analyses for simple linear regressions did not find significant differences except for TET2_2 (p = 0.006), however the methylation of CpG sites at this gene did not display interaction with the sex of the individuals analyzed (p = 0.713).
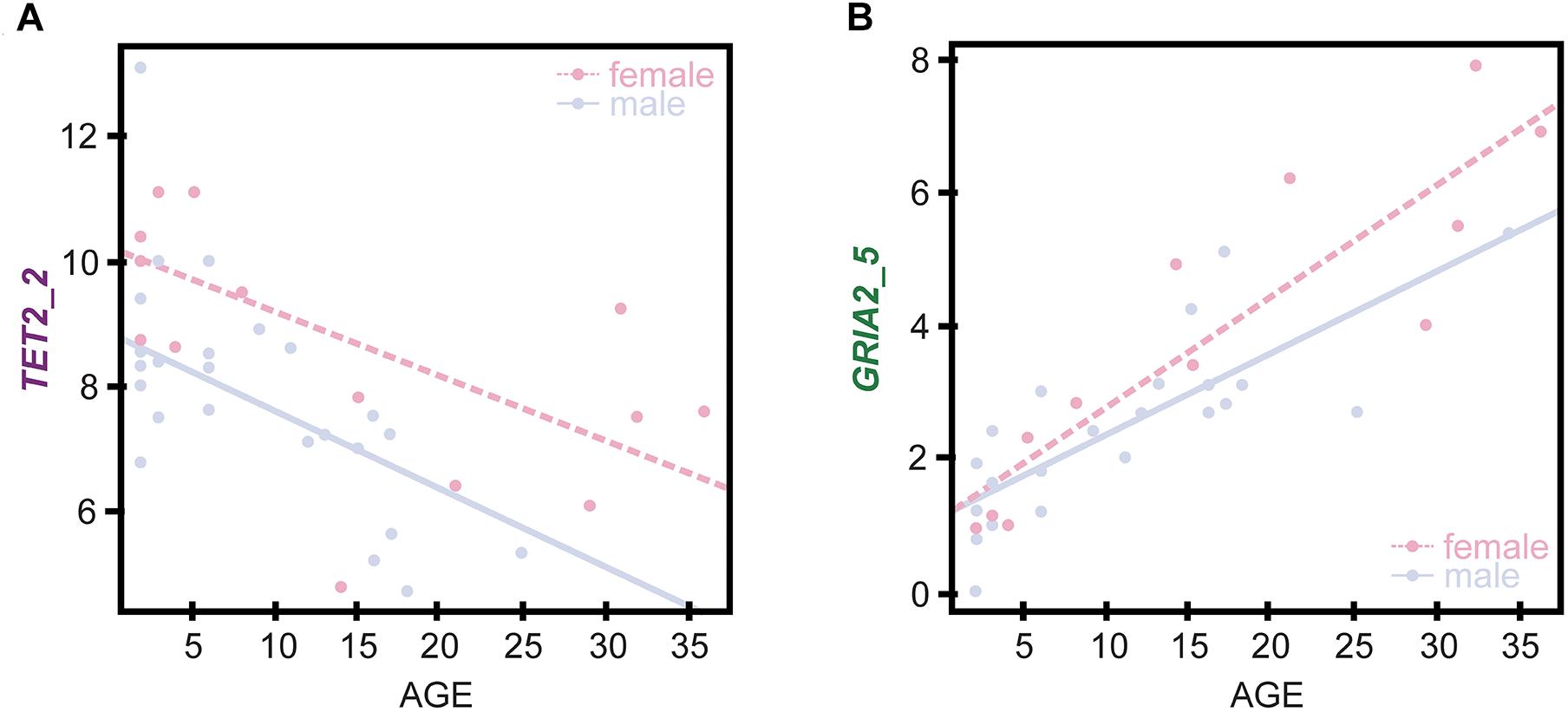
Figure 4. Sex-specific regression analyses between %DNA methylation at the CpG sites defined by the BEAT model in genes TET2 (A) and GRIA2 (B) and chronological age in females (open dots, dashed line) and males (solid dots and line).
Discussion
Dolphins, as well as many other marine mammals, show relatively little to no visible signs of aging, therefore requiring the use of alternative aging methods. Unfortunately, the current methods used to estimate age in dolphins are limited due to logistical constraints including the need to capture and handle individuals (International Whaling Commission, 1980). Such limitation hampers monitoring, assessing, and studying age-dependent effects of environmental stressors at the level of populations (Reeves, 2003; Westgate and Read, 2007; Agusa et al., 2008). Fortunately, studies in cetaceans can easily benefit from ongoing efforts creating more effective molecular aging tools in model systems such as humans or mice (Yi et al., 2014, 2015). In the present work, a similar experimental approach was used to investigate the relationship between epigenetic modifications in age-responsive genes and chronological age in mammals, and their potential to develop the BEAT (Bottlenose dolphin Epigenetic Aging Tool) to efficiently estimate chronological age using skin biopsy samples (Grönniger et al., 2010; Horvath, 2013; Polanowski et al., 2014). The BEAT is very similar to the HEAA [Humpback whale Epigenetic Aging Assay (Polanowski et al., 2014)] on its approach, but requiring only the analysis of CpG sites at two different genes, instead of the three genes required by the HEAA.
Age-Responsive Genes Display Different Levels of CpG Methylation Across Dolphin Age Groups
The BEAT model defined in the present study is based on %DNA methylation information from two age-responsive genes (GRIA2 and TET2) to determine chronological age. These genes were previously (and successfully) used to estimate age in other mammals, including North Atlantic humpback whales and humans (Koch and Wagner, 2011; Buscarlet et al., 2016). GRIA2 (glutamate receptor 2) belongs to a family of receptors that function in many neurophysiological processes (especially in the brain) and displays hypermethylation in older individuals (Koch and Wagner, 2011). TET2 (tet methylcytosine dioxygenase 2) encodes an epigenetic regulator mediating the transformation of 5-methylcytosine (5mC) into 5-hydroxymethylcytosine (5hmC) (Buscarlet et al., 2016), also functioning as a tumor suppressor that is heavily methylated in cancer tissues (Grönniger et al., 2010). Surprisingly, TET2 hypomethylation in older marine mammals contrasts with its hypermethylation in humans (Grönniger et al., 2010; Polanowski et al., 2014), underscoring the effects of the different constraints imposed by the regulatory role of DNA methylation on the function and subsequent long-term evolution of different genes across diverse ecosystems (Hernando-Herraez et al., 2015).
Overall, bottlenose dolphins displayed %DNA methylation levels at CpG sites in GRIA2 and CDKN2A genes similar to those displayed by humpback whales (Polanowski et al., 2014). Oppositely, %DNA methylation at TET2 CpG sites were lower by almost an order of magnitude in bottlenose dolphins (8.03 ± 1.850, compared with humpback whales 14.630 ± 4.070; Table 2). This difference underscores the importance of doing age assessment studies for different species and even developing species specific aging tools. Discriminating among the CpG sites used in the BEAT, the %DNA methylation at the GRIA2_5 site displayed a very high coefficient of determination for simple linear regression with age (R2 = 0.749), which contrasts with the lower value found for TET2_2 (R2 = 0.277), suggesting that other factors (besides age) may contribute to DNA methylation in these sites. Although the coefficient of determination obtained for the BEAT (R2 = 0.779) is not as high as in similar tools developed for human forensics using high-resolution methods [e.g., R2 = 0.91 (Yi et al., 2014)] this model can still be considered a massive improvement to previous methods used to estimate age in dolphins and small cetaceans, including additional room for improvement as the application of new methods becomes feasible (i.e., affordable) in non-model organisms.
The BEAT Tool Provides Epigenetic Age Estimations Based on Two Age-Responsive Genes
In total, 17 CpG sites located at three age-responsive genes (GRIA2, TET2, and CDKN2A) were studied to develop the BEAT. The best model correlating %DNA methylation with dolphin chronological age used a single CpG site in TET2 (CpG site 2) and GRIA2 (CpG site 5) genes (multiple regression R2 = 0.779; LOOCV validation R2 = 0.740, Figure 3) approximately 78% of the variation observed in %DNA methylation among dolphins is explained by their differences in chronological age. The remainder of the variation (22%) could be potentially due to other environmental factors (e.g., stress exposure) and/or be determined by genetic variability. Contrary to the HEAA assay, the BEAT tool did not require incorporating CpG sites from the CDKN2A gene into the model, although this gene was used by the second best correlation model (Table 4). Combined, these results suggest that the BEAT model might be less influenced on multiple age proxy markers to produce age estimates, reducing its dependence on genotypic or environmental variation, a key feature supporting its accuracy (Polanowski et al., 2014). Indeed, the methylation at the CpG site used in GRIA2 confers by itself a very strong correlation to dolphin chronological age (Figure 2), which is further improved by the incorporation of an additional CpG site from TET2.
The BEAT model displays a high correlation between %DNA methylation and age for the studied CpG sites (Figure 3 and Table 4). Yet, the accuracy of the model decreases slightly as dolphins age, as suggested by the increasing variance of estimated ages for older individuals [the same observation was made by studies attempting to create an age estimation tool for humans (Xu et al., 2015; Yi et al., 2015)]. Nonetheless, this effect does not seem to hamper the ability of the model to produce accurate estimations for a wide range of ages as validated by the LOOCV analysis (Figure 3) and by the fact that the error of the BEAT model is never higher than 5 years meaning that age estimates by the model will be within 5 years of the actual age. Obviously, as in every model, the error can be reduced by increasing the sample size (notably by adding older individuals). Nonetheless, its present form constitutes a very important advancement from traditional aging methods used on these organisms (Hohn et al., 1989; Olsen et al., 2012). Further validation of the model using unknown wild specimens was not conducted in this study (but are currently underway) due to the similarity of this model to the HEAA where this type of validation was conducted and was found to be successful (Polanowski et al., 2014). Two different and complementary explanations might account for the observed dispersion. First, in the present study the age distribution of the samples is skewed toward young individuals (2–4 years, n = 14), compared with older individuals (4–36 years, n = 25; Figure 1 and Supplementary File S1). Although such distribution was also a potential problem for the estimations made by the HEAA assay in humpback whales (Polanowski et al., 2014), it can be easily corrected by progressively incorporating older individuals in the dataset to better fit the model. In the present case, the oldest individual incorporated into the BEAT tool was 36 years old, making the model reliable for most dolphins within the boundaries of the lifespan of most individuals (Wells and Scott, 2018).
Sex-Specific Differences Do Not Affect the Age Estimations Made by the BEAT Tool
The second explanation for the wider spread of data points around the regression line for older individuals refers to the potential increasing contribution of sex-specific traits to DNA methylation after dolphins reach sexual maturity. Indeed, mammals display critical differences in gene regulation between females and males, including epigenetic mechanisms [e.g., X chromosome inactivation, imprinting, etc. (Bar et al., 2019; Mutzel et al., 2019)], and their role during aging (Kornack et al., 1991; Berchtold et al., 2008; Arslan-Ergul and Adams, 2014). In the present work, the analysis of younger individuals (either females or males) revealed a better correlation between %DNA methylation and age (Figures 2, 3) when compared with older, sexually mature dolphins (age > 15 years) (Wells and Scott, 2018).
There have been several published studies investigating differences between sexes in aging and many other life functions (Garm et al., 2013; Soares et al., 2014; Fischer and Riddle, 2018). Given the role of epigenetic mechanisms regulating gene expression, it is not surprising that a growing number of studies are reporting sex-specific changes in DNA methylation occurring with age (Horvath, 2013; Horvath et al., 2016), highlighting the importance of including sex in DNA methylation studies. Therefore, in order to ascertain if sex plays a significant role contributing to the BEAT model, additional analyses were performed, discriminating between females (n = 15) and males (n = 24), including similar numbers of 2–4 year old individuals (females n = 6, males n = 8), as well as additional individuals evenly representing all other age groups (see Figure 1). The results suggest that the addition of sex to the model does not improve the BEAT’s ability to estimate age (ANCOVA, p = 0.760, Figure 4). Interestingly, when simple and multiple regression analyses were performed separately between females and males, the results revealed the presence of sex-specific differences in the CpG sites displaying higher correlations with %DNA methylation (see Supplementary File S3). Similarly, sex-specific multiple regression analyses defined different best models for each sex. Accordingly, the best female-specific model used CpG sites at two different genes (GRIA2_5 and TET2_4), whereas the male-specific model used CpG sites from all three genes investigated (GRIA2_1, CDKN2A_5, and TET2_2, see Table 5).
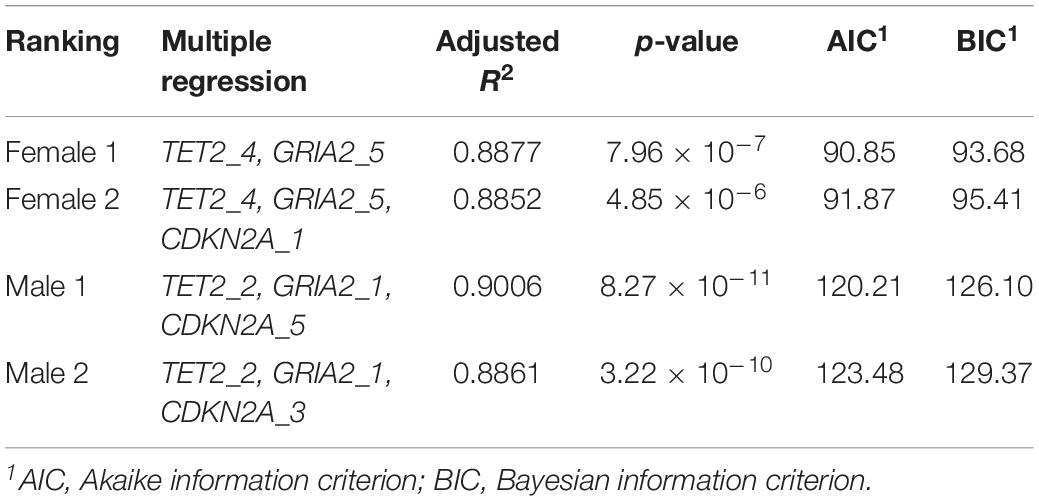
Table 5. Ranked models best describing the relationship between %DNA methylation at studied CpG sites as a function of chronological age discriminating between female and male bottlenose dolphins.
Overall, although the incorporation of sex does not seem to significantly improve the BEAT model, it does not discard its role in the rate of DNA methylation change between males and females. One possibility is that the small number of reproductive age individuals in the dataset may be hindering such contribution. Indeed, the simple linear regression models for GRIA2_5 and TET2_2 discriminating between females and males (Figure 4), show that regression lines share similar slopes, with the deviation being more pronounced for older individuals for both CpG sites. As samples from more known-age individuals of greater age become available, sex differences may become more evident and could possibly improve the accuracy of age estimation in cetaceans.
Conclusion
The present study investigates the relationship between epigenetic modifications in age-responsive genes and chronological age in bottlenose dolphins, using that information to develop an epigenetic age determination tool, the BEAT. The model underlying the BEAT is based on the %DNA methylation displayed by CpG sites in genes TET2 (CpG site 2) and GRIA2 (CpG site 5), estimating chronological age within 4.8 years of accuracy and with a high coefficient of determination (R2 = 0.779). Overall, this tool provides a much needed alternative for feasibly estimating ages for large numbers of individuals in cetacean populations of interest. Furthermore, the ability to train the model with additional samples, especially older individuals with equal representation of males and females, will foster the further development of the BEAT tool providing a finer estimation of age depending on sex and age-groups, and environmental factors that might influence biological age.
Data Availability
Data corresponding to dolphin age, sex, and %DNA methylation for the different CpG sites studied, R scripts and correlation analyses associated to the BEAT on which the present research is based, are deposited in the repository of the CREST-CAChE NSF Center at Florida International University and publicly available at github.com/eelabfiu/beat.
Ethics Statement
The animal study was conducted under National Marine Fisheries Service Scientific Research Permits, and approvals under Mote Marine Laboratory’s IACUC.
Author Contributions
AB designed the study, developed the experiments, analyzed the data, and wrote the manuscript. JK designed the study and wrote the manuscript. RW designed the study, provided the samples, and wrote the manuscript. JE-L designed the study, provided the materials, analyzed the data, and wrote the manuscript.
Funding
This work was supported by grants from the National Science Foundation (Grant IOS 1810981 awarded to JE-L, and Grant HRD 1547798 awarded to Florida International University as part of the Centers for Research Excellence in Science and Technology Program) and by awards from FIU’s CREST Center for Aquatic Chemistry and Environment (CREST-CAChE) and from FIU Tropics (AB).
Conflict of Interest Statement
The authors declare that the research was conducted in the absence of any commercial or financial relationships that could be construed as a potential conflict of interest.
Acknowledgments
Samples from Sarasota Bay dolphins were collected under National Marine Fisheries Service Scientific Research Permits issued to RW, under IACUC approvals through Mote Marine Laboratory. We are thankful to the staff, students, veterinarians, collaborators, and volunteers of the Sarasota Dolphin Research Program for collection of the samples, and to all members and volunteers in the Environmental Epigenetics Lab for their assistance during the development of this work. Primary support for sample collection was provided by Dolphin Quest, Inc. This is contribution #148 from the Center for Coastal Oceans Research and #915 from the Southeast Environmental Research Center in the Institute of Water and Environment at Florida International University.
Supplementary Material
The Supplementary Material for this article can be found online at: https://www.frontiersin.org/articles/10.3389/fmars.2019.00561/full#supplementary-material
FILE S1 | Bottlenose dolphin dataset used in the present work, including: age, sex, and %DNA methylation for the different CpG sites studied in genes TET2, GRIA2, and CDKN2A across all individuals analyzed.
FILE S2 | R codes used for the development of the BEAT and tests for normality including outlier analyses. Residual plots and Normality Q–Q plots are also included.
FILE S3 | Individual linear correlation analyses for CpG sites displaying a significant correlation between %DNA methylation and age, calculated from whole dataset and discriminating between females and males.
References
Agusa, T., Nomura, K., Kunito, T., Anan, Y., Iwata, H., Miyazaki, N., et al. (2008). Interelement relationships and age-related variation of trace element concentrations in liver of striped dolphins (Stenella coeruleoalba) from Japanese coastal waters. Mar. Pollut. Bull. 57, 807–815. doi: 10.1016/j.marpolbul.2008.01.039
Arslan-Ergul, A., and Adams, M. M. (2014). Gene expression changes in aging Zebrafish (Danio rerio) brains are sexually dimorphic. BMC Neurosci. 15:29. doi: 10.1186/1471-2202-15-29
Baker, I., O’Brien, J., McHugh, K., and Berrow, S. (2018). Female reproductive parameters and population demographics of bottlenose dolphins (Tursiops truncatus) in the Shannon Estuary, Ireland. Mar. Biol. 165:15. doi: 10.1007/s00227-017-3265-z
Bar, S., Seaton, L. R., Weissbein, U., Eldar-Geva, T., and Benvenisty, N. (2019). Global characterization of X chromosome inactivation in human pluripotent stem cells. Cell Rep. 27, 20.e3–29.e3. doi: 10.1016/j.celrep.2019.03.019
Barrett-Lennard, L., Smith, T. G., and Ellis, G. M. (1996). A cetacean biopsy system using lightweight pneumatic darts, and its effect on the behavior of killer whales. Mar. Mammal Sci. 12, 14–27. doi: 10.1111/j.1748-7692.1996.tb00302.x
Berchtold, N. C., Cribbs, D. H., Coleman, P. D., Rogers, J., Head, E., Kim, R., et al. (2008). Gene expression changes in the course of normal brain aging are sexually dimorphic. Proc. Natl. Acad. Sci. U.S.A. 105, 15605–15610. doi: 10.1073/pnas.0806883105
Broer, L., Codd, V., Nyholt, D. R., Deelen, J., Mangino, M., Willemsen, G., et al. (2013). Meta-analysis of telomere length in 19,713 subjects reveals high heritability, stronger maternal inheritance and a paternal age effect. Eur. J. Hum. Genet. 21, 1163–1168. doi: 10.1038/ejhg.2012.303
Buscarlet, M., Tessier, A., Provost, S., Mollica, L., and Busque, L. (2016). Human blood cell levels of 5-hydroxymethylcytosine (5hmC) decline with age, partly related to acquired mutations in TET2. Exp. Hematol. 44, 1072–1084. doi: 10.1016/j.exphem.2016.07.009
Campana, S. (2001). Accuracy, precision and quality control in age determination, including a review of the use and abuse of age validation methods. J. Fish Biol. 59, 197–242. doi: 10.1006/jfbi.2001.1668
Dans, S. L., Crespo, E. A., Koen-Alonso, M., Markowitz, T. M., Vera, B. B., and Dahood, A. D. (2010). “Dusky dolphin trophic ecology: their role in the food web,” in The Dusky Dolphin: Master Acrobat off Different Shores, eds B. Würsig, and M. Würsig, (Amsterdam: Elsevier), 49–74.
Davidson, A. D., Boyer, A. G., Kim, H., Pompa-Mansilla, S., Hamilton, M. J., Costa, D. P., et al. (2012). Drivers and hotspots of extinction risk in marine mammals. Proc. Natl. Acad. Sci. 109, 3395–3400. doi: 10.1073/pnas.1121469109
Deaton, A. M., and Bird, A. (2011). CpG islands and the regulation of transcription. Genes Dev. 25, 1010–1022. doi: 10.1101/gad.2037511
Eirin-Lopez, J., and Putnam, H. (2018). Marine environmental epigenetics. Annu. Rev. Mar. Sci. 11, 335–368.
Fischer, K. E., and Riddle, N. C. (2018). Sex differences in aging: genomic instability. J. Gerontol. A Biol. Sci. Med. Sci. 73, 166–174. doi: 10.1093/gerona/glx105
Fossi, M. C., Marsili, L., Neri, G., Casini, S., Bearzi, G., Politi, E., et al. (2000). Skin biopsy of Mediterranean cetaceans for the investigation of interspecies susceptibility to xenobiotic contaminants. Mar. Environ. Res. 50, 517–521. doi: 10.1016/s0141-1136(00)00127-6
Garm, C., Moreno-Villanueva, M., Bürkle, A., Petersen, I., Bohr, V. A., Christensen, K., et al. (2013). Age and gender effects on DNA strand break repair in peripheral blood mononuclear cells. Aging Cell 12, 58–66. doi: 10.1111/acel.12019
Grönniger, E., Weber, B., Heil, O., Peters, N., Stäb, F., Wenck, H., et al. (2010). Aging and chronic sun exposure cause distinct epigenetic changes in human skin. PLoS Genet. 6:e1000971. doi: 10.1371/journal.pgen.1000971
Hartel, E. F., Constantine, R., and Torres, L. G. (2015). Changes in habitat use patterns by bottlenose dolphins over a 10-year period render static management boundaries ineffective. Aquat. Conserv. Mar. Freshw. Ecosyst. 25, 701–711. doi: 10.1002/aqc.2465
Herman, D. P., Ylitalo, G. M., Robbins, J., Straley, J. M., Gabriele, C. M., Clapham, P. J., et al. (2009). Age determination of humpback whales Megaptera novaeangliae through blubber fatty acid compositions of biopsy samples. Mar. Ecol. Prog. Ser. 392, 277–293. doi: 10.3354/meps08249
Hernando-Herraez, I., Garcia-Perez, R., Sharp, A. J., and Marques-Bonet, T. (2015). DNA methylation: insights into human evolution. PLoS Genet. 11:e1005661. doi: 10.1371/journal.pgen.1005661
Hohn, A. A., Scott, M. D., Wells, R. S., Sweeney, J. C., and Blair Irvine, A. (1989). Growth layers in teeth from known-age, free-ranging bottlenose Dolphins. Mar. Mammal Sci. 5, 315–342. doi: 10.1111/j.1748-7692.1989.tb00346.x
Horvath, S. (2013). DNA methylation age of human tissues and cell types. Genome Biol. 14:R115. doi: 10.1186/gb-2013-14-10-r115
Horvath, S., Gurven, M., Levine, M. E., Trumble, B. C., Kaplan, H., Allayee, H., et al. (2016). An epigenetic clock analysis of race/ethnicity, sex, and coronary heart disease. Genome Biol. 17:171. doi: 10.1186/s13059-016-1030-0
International Whaling Commission, (1980). Age Determination of Toothed Whales and Sirenians. Cambridge: International Whaling Commission.
Irvine, B., and Wells, R. S. (1972). Results of attempts to tag Atlantic bottlenose dolphins (Tursiops truncatus). Cetology 13, 1–5. doi: 10.1093/mmy/myw011
Jones, P. A. (2001). The role of DNA methylation in mammalian epigenetics. Science 293, 1068–1070. doi: 10.1126/science.1063852
Jones, P. A. (2012). Functions of DNA methylation: islands, start sites, gene bodies and beyond. Nat. Rev. Genet. 13, 484–492. doi: 10.1038/nrg3230
Kappei, D., and Arturo Londoño-Vallejo, J. (2008). Telomere length inheritance and aging. Mech. Ageing Dev. 129, 17–26. doi: 10.1016/j.mad.2007.10.009
Kiszka, J., Simon-Bouhet, B., Martinez, L., Pusineri, C., Richard, P., and Ridoux, V. (2011). Ecological niche segregation within a community of sympatric dolphins around a tropical island. Mar. Ecol. Prog. Ser. 433, 273–288. doi: 10.3354/meps09165
Koch, C. M., and Wagner, W. (2011). Epigenetic-aging-signature to determine age in different tissues. Aging 3, 1018–1027.
Kornack, D. R., Lu, B., and Black, I. B. (1991). Sexually dimorphic expression of the NGF receptor gene in the developing rat brain. Brain Res. 542, 171–174. doi: 10.1016/0006-8993(91)91015-s
Krutzen, M., Barre, L. M., Moller, L. M., Heithaus, M. R., Simms, C., and Sherwin, W. B. (2002). A biopsy system for small cetaceans: darting success and wound healing in Tursiops spp. Mar. Mammal Sci. 18, 863–878. doi: 10.1111/j.1748-7692.2002.tb01078.x
Martinez-Viaud, K. A., Lawley, C. T., Vergara, M. M., Ben-Zvi, G., Biniashvili, T., Baruch, K., et al. (2019). New de novo assembly of the Atlantic bottlenose dolphin (Tursiops truncatus) improves genome completeness and provides haplotype phasing. Gigascience 8:giy168. doi: 10.1093/gigascience/giy168
McGowen, M. R., Grossman, L. I., and Wildman, D. E. (2012). Dolphin genome provides evidence for adaptive evolution of nervous system genes and a molecular rate slowdown. Proc. R. Soc. B Biol. Sci. 279, 3643–3651. doi: 10.1098/rspb.2012.0869
Miller, L. J., Solangi, M., and Kuczaj, S. A. II (2010). Seasonal and diurnal patterns of behavior exhibited by Atlantic Bottlenose Dolphins (Tursiops truncatus) in the mississippi sound. Ethology 116, 1127–1137. doi: 10.1111/j.1439-0310.2010.01824.x
Mutzel, V., Okamoto, I., Dunkel, I., Saitou, M., Giorgetti, L., Heard, E., et al. (2019). A symmetric toggle switch explains the onset of random X inactivation in different mammals. Nat. Struct. Mol. Biol. 26, 350–360. doi: 10.1038/s41594-019-0214-1
Olsen, M. T., Bérubé, M., Robbins, J., and Palsbøll, P. J. (2012). Empirical evaluation of humpback whale telomere length estimates; quality control and factors causing variability in the singleplex and multiplex qPCR methods. BMC Genet. 13:77. doi: 10.1186/1471-2156-13-77
Paoli-Iseppi, R. D., De Paoli-Iseppi, R., Deagle, B. E., Polanowski, A. M., McMahon, C. R., Dickinson, J. L., et al. (2019). Age estimation in a long-lived seabird (Ardenna tenuirostris) using DNA methylation-based biomarkers. Mol. Ecol. Resourc. 19, 411–425. doi: 10.1111/1755-0998.12981
Picard, R. R., and Dennis Cook, R. (1984). Cross-validation of regression models. J. Am. Stat. Assoc. 79, 575–583. doi: 10.2307/2288403
Polanowski, A. M., Robbins, J., Chandler, D., and Jarman, S. N. (2014). Epigenetic estimation of age in humpback whales. Mol. Ecol. Resour. 14, 976–987. doi: 10.1111/1755-0998.12247
Pompa, S., Ehrlich, P. R., and Ceballos, G. (2011). Global distribution and conservation of marine mammals. Proc. Natl. Acad. Sci. U.S.A. 108, 13600–13605. doi: 10.1073/pnas.1101525108
R Core Team, (2013). R: A Language and Environment for Statistical Computing. Vienna: R Foundation for Statistical Computing.
Reeves, R. R. (2003). Dolphins, Whales and Porpoises: 2002-2010 Conservation Action Plan for the World’s Cetaceans. Gland: IUCN.
Sambrook, J., Fritsch, E. F., and Maniatis, T. (1989). Molecular Cloning: A Laboratory Manual. Cold Spring Harbor, NY: Cold Spring Harbor Laboratory Press.
Shabani, M., Borry, P., Smeers, I., and Bekaert, B. (2018). Forensic epigenetic age estimation and beyond: ethical and legal considerations. Trends Genet. 34, 489–491. doi: 10.1016/j.tig.2018.03.006
Soares, J. P., Cortinhas, A., Bento, T., Leitão, J. C., Collins, A. R., Gaivão, I., et al. (2014). Aging and DNA damage in humans: a meta-analysis study. Aging 6, 432–439.
Torres, L. G., Rosel, P. E., D’Agrosa, C., and Read, A. J. (2003). Improving management of overlapping bottlenose dolphin ecotypes through spatial analysis and genetics. Mar. Mammal Sci. 19, 502–514. doi: 10.1111/j.1748-7692.2003.tb01317.x
Tyson, R. B., and Wells, R. S. (2016). Sarasota Bay/Little Sarasota Bay Bottlenose Dolphin Abundance Estimates: 2015. Prepared for National Marine Fisheries Service Northern Gulf of Mexico Bay, Sound and Estuary Bottlenose Dolphin Stock Blocks B20 and B35, Combined. Southeast Fisheries Science Center Reference Document PRBD-2016-02. Silver Spring, MD: NOAA.
Wells, R., Rhinehart, H., Hansen, L., Sweeney, J., Townsend, F., Stone, R., et al. (2004). Bottlenose Dolphins as marine ecosystem sentinels: developing a health monitoring system. EcoHealth 1, 246–254. doi: 10.1007/s10393-004-0094-6
Wells, R. S. (2009). Learning from nature: bottlenose dolphin care and husbandry. Zoo Biol. 28, 1–17. doi: 10.1002/zoo.20252
Wells, R. S. (2014). “Social structure and life history of common bottlenose dolphins near Sarasota Bay, Florida: insights from four decades and five generations,” in Primates and Cetaceans: Field Research and Conservation of Complex Mammalian Societies, Primatology Monographs, eds J. Yamagiwa, and L. Karczmarski, (Tokyo: Springer), 149–172. doi: 10.1007/978-4-431-54523-1_8
Wells, R. S., and Scott, M. D. (2018). “Bottlenose dolphin: common bottlenose dolphin (Tursiops Truncatus),” in Encyclopedia of Marine Mammals, 3rd Edn, eds B. Würsig, J. G. M. Thewissen, and K. Kovacs (San Diego, CA: Academic Press/Elsevier), 118–125.
Wells, R. S., Tornero, V., Borrell, A., Aguilar, A., Rowles, T. K., Rhinehart, H. L., et al. (2005). Integrating life-history and reproductive success data to examine potential relationships with organochlorine compounds for bottlenose dolphins (Tursiops truncatus) in Sarasota Bay, Florida. Sci. Total Environ. 349, 106–119.
Westgate, A. J., and Read, A. J. (2007). Reproduction in short-beaked common dolphins (Delphinus delphis) from the western North Atlantic. Mar. Biol. 150, 1011–1024. doi: 10.1007/s00227-006-0394-1
Wilson, B., Thompson, P. M., and Hammond, P. S. (1997). Habitat use by bottlenose dolphins: seasonal distribution and stratified movement patterns in the Moray Firth, Scotland. J. Appl. Ecol. 34, 1365–1374.
Xu, C., Qu, H., Wang, G., Xie, B., Shi, Y., Yang, Y., et al. (2015). A novel strategy for forensic age prediction by DNA methylation and support vector regression model. Sci. Rep. 5:17788. doi: 10.1038/srep17788
Yi, S. H., Jia, Y. S., Mei, K., Yang, R. Z., and Huang, D. X. (2015). Age-related DNA methylation changes for forensic age-prediction. Int. J. Legal Med. 129, 237–244. doi: 10.1007/s00414-014-1100-3
Keywords: DNA methylation, population parameter, sex, correlation – regression analysis, pyrosequencing
Citation: Beal AP, Kiszka JJ, Wells RS and Eirin-Lopez JM (2019) The Bottlenose Dolphin Epigenetic Aging Tool (BEAT): A Molecular Age Estimation Tool for Small Cetaceans. Front. Mar. Sci. 6:561. doi: 10.3389/fmars.2019.00561
Received: 04 June 2019; Accepted: 27 August 2019;
Published: 26 September 2019.
Edited by:
Andrew Stanley Mount, Clemson University, United StatesReviewed by:
Charles Rice, Clemson University, United StatesDaniel Rittschof, Duke University, United States
Copyright © 2019 Beal, Kiszka, Wells and Eirin-Lopez. This is an open-access article distributed under the terms of the Creative Commons Attribution License (CC BY). The use, distribution or reproduction in other forums is permitted, provided the original author(s) and the copyright owner(s) are credited and that the original publication in this journal is cited, in accordance with accepted academic practice. No use, distribution or reproduction is permitted which does not comply with these terms.
*Correspondence: Jose M. Eirin-Lopez, amVpcmlubG9AZml1LmVkdQ==