- 1Department of Biology, University of Padova, Padua, Italy
- 2Functional Genomics Center, Department of Biotechnology, University of Verona, Verona, Italy
- 3Consorzio Nazionale Interuniversitario per le Scienze del Mare, Rome, Italy
- 4Department of Hydrobiology, Fisheries Faculty, Sinop University, Sinop, Turkey
- 5Institut National Agronomique de Tunisie, Université de Carthage, Tunis, Tunisia
- 6Laboratoire de Biodiversité, Biotechnologie et Changement Climatique, Faculté des Sciences de Tunis, Université de Tunis El Manar, Tunis, Tunisia
- 7USR 3278 CNRS-EPHE, CRIOBE, Université de Perpignan, Perpignan, France
- 8Department of Biological and Environmental Sciences and Technologies, University of Salento, Lecce, Italy
- 9Department of Ecology, Agronomy and Aquaculture, University of Zadar, Zadar, Croatia
- 10Institute of Marine Biology, University of Montenegro, Kotor, Montenegro
- 11Romanian Waters National Authority, Dobrogea-Black Sea Basin Administration, Constanta, Romania
- 12Institute of Marine Biological Research, Sevastopol, Russia
- 13Institute of Oceanology (IO-BAS), Varna, Bulgaria
- 14Faculty of Biology, Alexandro Ioan Cuza University of Iaşi, Iaşi, Romania
- 15Institute of Oceanography, Hellenic Centre for Marine Research, Anavyssos, Greece
Connectivity between populations shapes the genetic structure of species being crucial for an effective management of environmental resources. Genetic approaches can provide indirect measures of connectivity, allowing the identification of genetically differentiated – unconnected – populations. In this study, we applied a 2b-RAD approach based on hundreds of polymorphic loci to provide the first detailed insight into the population genomics of the Mediterranean mussel Mytilus galloprovincialis in part of its native geographical range. We sampled 19 localities within the Mediterranean and Black Seas, and analyzed a total of 478 samples. We detected strong differences between the two seas, whereas no differences were found between samples from the Western and Central Mediterranean and within Western Mediterranean samples. In the Central Mediterranean a significant differentiation emerged comparing Central Adriatic samples with those from South Adriatic and Ionian Seas. Furthermore, an East-to-West genetic structuring was found in the Central Adriatic Sea, which was not present in the Southern Adriatic and Ionian Seas. These results possibly reflect the local oceanography, with a Middle Adriatic gyre unable to prevent genetic differentiation in this species, and a Southern Adriatic gyre that effectively mixes propagules in Southern areas. In the Black Sea, no signal of genetic structure was found, although samples were spaced at similar distances as in the Adriatic-Ionian area. Genetic connectivity patterns of M. galloprovincialis reveal peculiar species-specific features respect to other species with similar larval duration, suggesting caution in using genetic connectivity data of single species in defining conservation units. We recommend of using genetic connectivity data of many species representing a variety of life history traits, and we call for new investigations using high resolution population genomics, particularly in the Black Sea, to understand if areas separated by hundreds of kilometers can be considered genetically connected as mussels’ data suggest. This information will be critical to ensure “a well-connected system of protected areas” according to Aichi Target 11 of the Convention on Biological Diversity.
Introduction
Understanding the distribution of genetic variability is a keystone for environmental resources management and conservation biology of marine species (Moritz, 1994; Palumbi, 2003; Cowen et al., 2006). Population connectivity plays a crucial role in local and metapopulation dynamics, genetic structure and population resiliency, e.g., in response to human exploitation (Hastings and Harrison, 1994; Cowen et al., 2007; Weersing and Toonen, 2009; Puckett et al., 2014). Most marine species release planktonic larvae which disperse over days up to months with the currents and thereby constitute the primary source of the dispersal capacity (Mileikovsky, 1971; Ward et al., 1994; Gilg and Hilbish, 2003). Direct labeling and tracking of larvae is rarely a possibility (Levin, 1990; but see Becker et al., 2007), so genetic data are widely used for the indirect inference of population connectivity (Hellberg et al., 2002; Thorrold et al., 2002; Palumbi, 2003; Broquet and Petit, 2009; Cowen and Sponaugle, 2009; Lowe and Allendorf, 2010).
The FP7 CoCoNET European project aimed at an in-depth analysis of connectivity among present and future Marine Protected Areas (MPAs) in the Mediterranean and Black Seas to instruct the placement of future networks of MPAs (Boero et al., 2016). A major task was the exploration of the population genetic structure of species from different taxonomic and functional groups having different ecological roles and dispersal modes. Specific traits related to the biology of each species, e.g., pelagic larval duration (PLD), reproductive timing, behavioral traits can determine recruitment success and result in different connectivity outcomes (Boissin et al., 2016; Jahnke et al., 2016, 2017; Carreras et al., 2017; Paterno et al., 2017; Boscari et al., 2019). Multispecies genetic analysis is needed to obtain a “collection of networks of genetic variation of all species within a community” (Fortuna et al., 2009).
Mussels of the genus Mytilus occur worldwide in all oceans and major seas in both northern and southern hemispheres (Gerard et al., 2008; Kijewski et al., 2011; Zbawicka et al., 2012) showing a wide distribution range. Specifically, in Europe, the presence of 3 distinct Mytilus species (M. galloprovincialis; M. edulis Linnaeus, 1758; M. trossulus Gould, 1850) and 2 hybrids (M. edulis/M. trossulus and M. edulis/M. galloprovicialis) has been traditionally reported (McDonald et al., 1991; Rawson et al., 1996).
The Mediterranean mussel Mytilus galloprovincialis (Lamarck, 1819) was selected in the project CoCoNET as model organism for species with a strong dispersal potential to typify patterns of genetic structure expected within scenarios of high connectivity. M. galloprovincialis is a sessile filter-feeder with pelagic larvae very common in both Mediterranean and Black Seas. The species undergoes multiple spawning events per season (Da Ros et al., 1985) with high fecundity per event. In addition, this mussel has long-lived pelagic larvae (i.e., more than a month; Cáceres-Martínez and Figueras, 1998; Bierne et al., 2002) able to settle onto rafts of natural and artificial substrates, thus to arrive at the final recruitment sites even as postlarval stages (Miller et al., 2018; see also Lane et al., 1985). This mussel is therefore considered a species with an exceptionally high potential for dispersion over time and space (Chicaro and Chicaro, 2000; Salinas-de-Leòn et al., 2012). The species is also economically important (Astorga, 2014) since largely utilized in aquaculture, where production is based on natural recruitment, which can further increase genetic mixing, at least at the spatial scale of spat collection. Being a sessile filter-feeder, M. galloprovincialis also serves as bioindicator of environmental conditions (Gosling, 1992).
Mytilus galloprovincialis originated in the Mediterranean Sea around 2 million years ago (Daguin and Borsa, 2000) and, at present, it occurs as a pure taxon in the latter and in the Black Sea (Śmietanka et al., 2004; Kijewski et al., 2011). Few studies describe its genetic structuring along the coasts of Mediterranean and Black Seas, and they are characterized by restricted numbers of sampling sites, low within-site replication and by the molecular markers used. High resolution, hypervariable nuclear markers such as microsatellites are typically of low densities and of difficult isolation in molluscs (Cruz et al., 2005; McInerney et al., 2011) and the use of mitochondrial markers is complicated by the doubly uniparental inheritance (DUI) of these organelles in mussels (Skibinski et al., 1994; Zouros et al., 1994). To overcome these limits, single nucleotide polymorphisms (SNPs) have started to be developed as markers in Mytilus spp., and they have been successfully used to differentiate species and populations within taxa in native and non-native areas (Zbawicka et al., 2012, 2018; Gardner et al., 2016; Larraín et al., 2018; Wilson et al., 2018).
Regardless of these findings, a consistent and reliable SNPs-based population structure of M. galloprovincialis across its native range is still missing. A restricted gene flow was found for this species throughout the Atlantic, Mediterranean and Black Seas (the native range), by using allozyme loci, mtDNA and RFLPs (Quesada et al., 1995; Sanjuan et al., 1996, 1997, Ladoukakis et al., 2002). At a finer geographical scale including only the Aegean Sea and the Central-Eastern Mediterranean Sea, genetic homogeneity was found in contrast to an evident genetic heterogeneity within the Adriatic-Ionian basins (microsatellites, mtDNA F and M; Ladoukakis et al., 2002; Giantsis et al., 2014a, b). Genetic data about this species are still scarce in the Adriatic region because of the restricted number of locations considered (up to 3; Giantsis et al., 2014a, b), while in the Black Sea the genetic diversity of M. galloprovincialis is essentially unknown.
The Next Generation Sequencing (NGS) population genomics approach applied in the present study is based on hundreds of polymorphic loci and takes advantage of the wide scale sampling design within the CoCoNET network, with analyses on 8 localities in the Central Mediterranean (Adriatic-Ionian basins), 2 in the Western Mediterranean and 9 in the Black Sea, providing the first insight into the M. galloprovincialis population genomics across a substantial part of its native distribution range. We used the RADseq 2b-RAD protocol (Wang et al., 2012) for the isolation and genotyping of SNPs with a genome-wide coverage.
Specifically, through a big effort of samples collection across both Mediterranean and Black basins, the present study aims to: (1) provide the overall genetic structure of M. galloprovincialis at the large scale (i.e., Mediterranean and Black Seas); (2) investigate the presence of genetic differentiation at a smaller scale within basins (i.e., Central Mediterranean and Black Seas); (3) compare our findings to those obtained for other species investigated in the same CoCoNET project. We anticipate that the outcomes provide useful insights for the conservation management and in particular for the design of connected MPAs, within the target areas, but potentially exportable to other geographic regions.
Materials and Methods
Sampling and Genomic DNA Extraction
Totally, 478 individuals of M. galloprovincialis were collected from 2013 to 2014 in the Mediterranean and Black Seas at 19 sampling locations (9 sites in the Black Sea, 8 sites in the Central Mediterranean, i.e., Adriatic and Ionian Seas), and 2 sites in the Western Mediterranean Sea (see Table 1 and Figure 1 for details). At each location, the shell of each sample was opened, the body removed and preserved in absolute ethanol until the genomic DNA (gDNA) extraction from gills (Eurogold Tissue DNA mini kit, EuroClone). For all the samples, the quality of extracted gDNA in terms of fragments size (1% agarose gel electrophoresis), concentration and purity ratio (NanoDrop UV-Vis spectrophotometer) was checked.
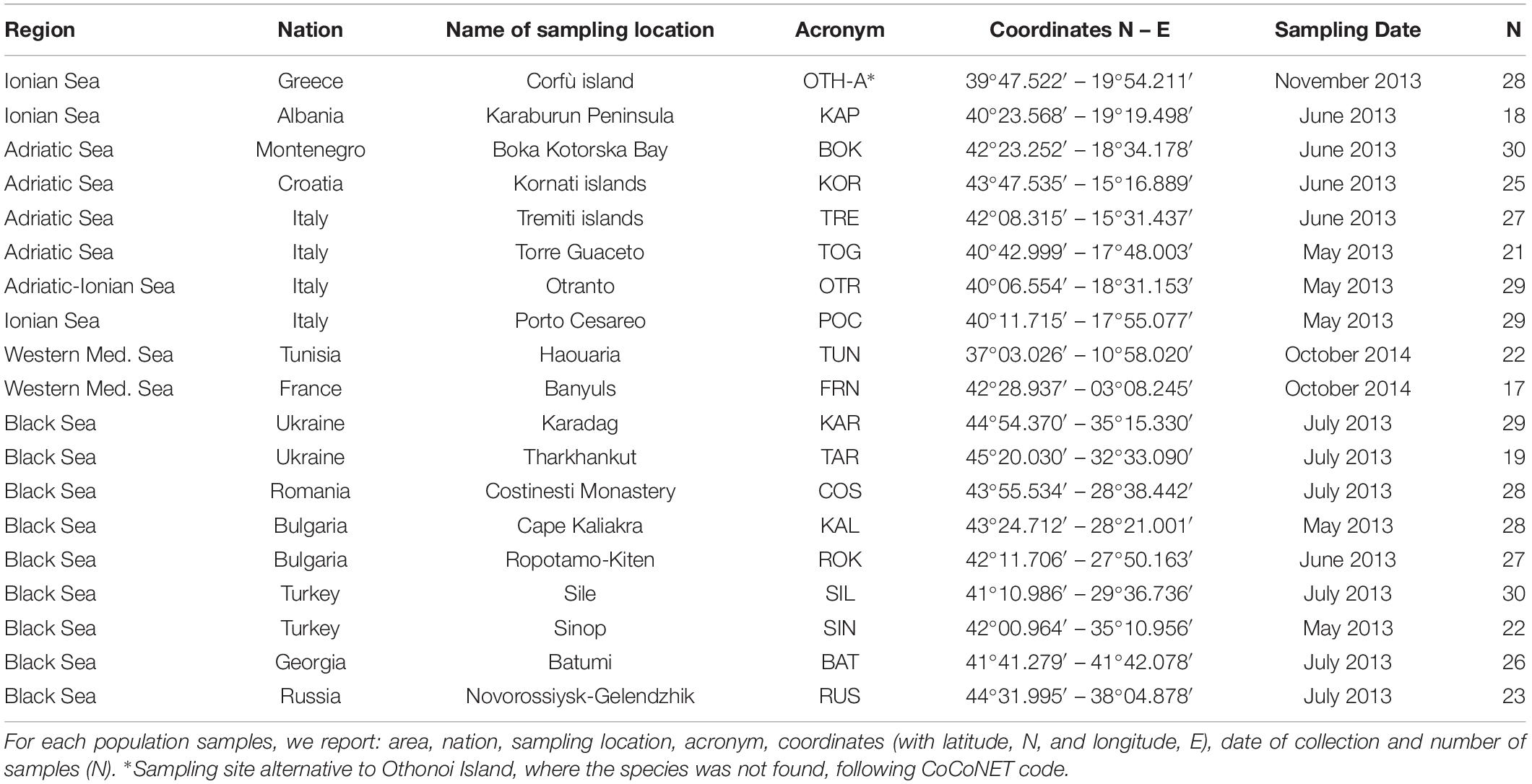
Table 1. Sampling information about the 19 Mytilus galloprovincialis population samples collected from the Mediterranean and Black Seas.
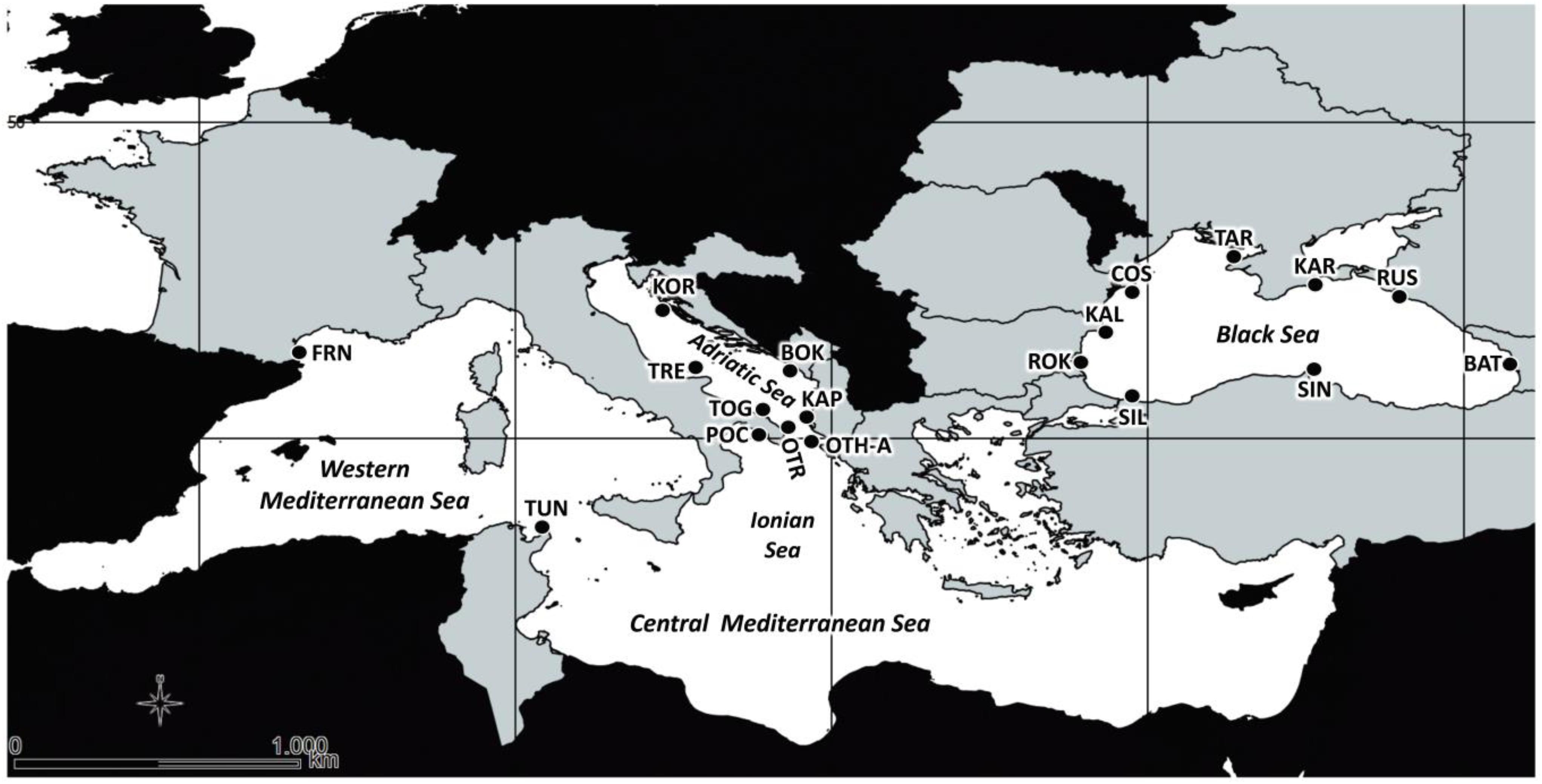
Figure 1. Map of sampling sites of the 19 Mytilus galloprovincialis population samples in Mediterranean and Black Seas. See Table 1 for acronyms.
Construction and Next Generation Sequencing of 2b-RAD Libraries
The concentration of high-quality RNA-free gDNA obtained from M. galloprovincialis samples was optimized for the 2b-RAD protocol (Wang et al., 2012) at about 250–400 ng for each individual. All the collected samples (N = 478) were processed following the 2b-RAD protocol steps described in Paterno et al. (2017), except for (i) the ligation of adaptors with partially (not fully) degenerated overhangs to the restriction fragments and (ii) the 2b-RAD tags amplification for a smaller number of cycles (14). Three pools (about 160 barcoded-samples each) were assembled and the high-molecular weight fragments and primer-dimers removed in two steps as in Paterno et al. (2017). The Next Generation Sequencing (NGS) of each pool was performed on Illumina HiSeq platforms with a SR50 High Output mode by UC Davis Genome Center (CA, United States) and Genomix4Life S.r.l. (Baronissi, SA, Italy); the sequencing services performed also the data demultiplexing and quality-filtering. In order to increase the read depth and ensure an equal number of reads for each sample, each pool was sequenced twice, adjusting the relative volumes of barcoded-samples on the second run (Paterno et al., 2017).
De novo Analysis: In silico Identification of Loci and Genotyping
The quality of the raw demultiplexed and quality-filtered reads was verified by using FASTQC 0.11.51. Later, custom-made PythonTM scripts were used for trimming the adaptors and filtering the reads for the presence of the IIB restriction enzyme (CspCI) recognition site, producing trimmed high-quality reads of uniform 32 bp-length.
STACKS 1.42 (Catchen et al., 2013) was used to carry out the assembly of loci and the individual genotyping (denovo_map.pl pipeline). Two technical replicates (one Mediterranean and one Black Sea specimen) were included for the optimization of the de novo assembly parameters in STACKS. Parameters were set as follows: m = 15 (minimum stack depth per allele in a sample), M = 4 (number of mismatches allowed between stacks to build a locus in an individual), n = 4 (maximum distance between loci from distinct individuals to be merged in the population catalog), SNP bound = 0–0.1 (error rate to call SNP), alpha = 0.05 (significance level to call a heterozygote or homozygote).
The employment of SNPs as RAD-Seq markers in population genomics typically implicates a certain level of missing data in the dataset because not all the genotyped markers are shared by all the samples (Chattopadhyay et al., 2014). This implies the need to retain only those loci shared among a reasonable, yet arbitrary, fraction of individuals for downstream analysis. However, if the missing data are not evenly scattered among the samples, a dataset of many individuals collected from distinct areas could lose power in detecting a fine genetic differentiation within areas because of the decrease of the number of shared polymorphic markers usable for the analyses.
For this reason, we performed three independent STACKS runs in order to achieve the best power of resolution according to the geographical scale considered: the wide scale (the entire study area) on one side, and the basin scale (Adriatic-Ionian basins or Black Sea) on the other side. First, an overall catalog of loci for the exploration of the global population structure of the species throughout the entire study area was obtained by running together all 478 samples (with two additional technical replicates) from the 19 population samples. Later, the 207 samples (plus one technical replicate) from the 8 Adriatic-Ionian sites and the 232 samples (plus one technical replicate) from the 9 Black Sea’s sites were run separately to obtain basin-specific datasets for the evaluation of the genetic structuring of the species at a small spatial scale.
For each of the three STACKS runs, we selected a final dataset consisting of all the polymorphic loci shared by at least 80% of the individuals with up to 3 SNPs and 6 alleles. When more than one SNP was found at a locus, only the SNP with the highest expected heterozygosity across each dataset was retained (Phillips, 2005). The threshold of missing loci per individual was set to 30%. Since the parameter m (minimum read depth for allele) was set to 15, no additional filter for sequencing depth was applied (Paterno et al., 2017). At the end, we obtained 3 distinct datasets (i.e., the overall dataset, the Adriatic-Ionian dataset, and the Black Sea one). The package Populations in STACKS was used to generate input file in GENEPOP format, and later CREATE (Coombs et al., 2008) and PGDSPIDER 2.1.0.3 (Lischer and Excoffier, 2012) were used for the conversion in several formats for genetic analyses.
Statistical Power of the Basin-Specific Datasets
The statistical power to detect true levels of genetic differentiation was evaluated and compared for the Adriatic-Ionian and Black Sea’s datasets by the simulation method of Ryman and Palm (2006) implemented in POWSIM 4.1 (SNPs executable Powsim_b). POWSIM simulates sampling from populations at various levels of expected divergence under a classical Wright-Fisher model without migration or mutation. Simulations were run using default parameter values for dememorizations (1000), batches (100), and iterations per batch (1000) for a scenario involving 8 and 9 subpopulations, respectively, using a range of effective population size (Ne = 1000–10,000) and of generations of drift (t = 10–100). The statistical power to detect an expected divergence as small as FST = 0.005 was estimated after 200 replicates as the proportion of statistically significant test (P < 0.05).
Population Genomics
The genetic variability within population samples, population structure and genetic differentiation were evaluated separately for the three datasets (overall, Adriatic-Ionian, Black Sea). The observed heterozygosity (Ho), unbiased expected heterozygosity (He) and the inbreeding coefficient (FIS) of each population sample were assessed on the polymorphic loci (GENETIX 4.05.2; Belkhir et al., 2000); the allelic richness (AR) was calculated on the smallest population sample size of each dataset (HP-RARE; Kalinowski, 2005). Non-hierarchical and hierarchical analysis of molecular variance (AMOVA; Excoffier et al., 1992) as well as the pairwise genetic distances between population samples (FST) were calculated (ARLEQUIN 3.5; Excoffier and Lischer, 2010); due to the presence of missing data, the locus by locus option was set. Benjamini and Hochberg (1995) correction for multiple tests was applied for adjusting the significance level in multiple comparisons. The Discriminant Analysis of Principal Components (DAPC; ADEGENET for R, Jombart, 2008) was used (1) to find the best number of clusters of genetically related individuals (based on the Bayesian Information Criterion, BIC) without the reference of the original populations and (2) for the visual assessment of the between-population differentiation of the original population samples. Bayesian clustering (STRUCTURE 2.3.4; Pritchard et al., 2000) was also performed to infer the most likely genetic clusters. After some trials, a burn-in of 100,000 followed by one million steps, K = 1–8 (depending on the datasets) and 5 iterations per each K value was set; sampling locations as prior (model LOCPRIOR, Hubisz et al., 2009) was used; admixture model and correlated allelic frequencies were assumed. The most probable number of clusters was identified based on delta K (STRUCTURE HARVESTER online; Earl and VonHoldt, 2012).
Detection of Loci Under Selection (Outliers)
For the identification of putative loci under directional selection, two neutrality tests were employed. The first test is the Bayesian method implemented in BAYESCAN 2.01 (Foll and Gaggiotti, 2008), and the second one is the FST-outlier method implemented in LOSITAN (Antao et al., 2008). Both software were run separately with the three datasets. LOSITAN parameters were set as follows: 1 million simulations under neutral mean FST, confidence interval of 0.95% and a false discovery rate (FDR) of 0.01; the infinite allele model was applied. For each run, three replicates were performed. BAYESCAN parameters were: burn in = 50,000; thinning interval = 30; sample size = 5000; numbers of pilot runs = 50; length of pilot runs = 5000 and the same false discovery rate (FDR) threshold set in LOSITAN (0.01). The loci detected by both methods were considered outliers under selection.
Results
Sequencing Results, Filtering and Selection of Loci for Genetic Analysis
For all the collected samples (N = 478), high-quality RNA-free gDNA was successfully extracted from the gills. The Illumina sequencing of the three 2b-RAD pools (N = 480; 2 technical replicates included) produced 1,032,760,048 de-multiplexed and quality-filtered reads (mean quality score per base > 37) and more than 92% was retained after trimming and filtering for CspCI restriction site (about 1,990,000 reads for each sample). The three independent STACKS runs identified 113,494, 77,459, and 83,000 2b-RAD tags considering, respectively, all the 19 population samples, the 8 Adriatic-Ionian ones alone, or the 9 Black Sea’s collections; about the 33% of loci were polymorphic in all 3 runs.
After filtering and the exclusion of the two technical replicates and of those individuals showing more than 30% of missing loci, we obtained three datasets as follows: (1) the overall dataset: 461 individuals, 512 polymorphic loci; (2) the Adriatic-Ionian dataset: 201 individuals, 811 polymorphic loci; and (3) the Black Sea’s dataset: 228 individuals, 998 polymorphic loci.
Genetic Analyses
Statistical Power of the Basin-Specific Datasets
The 200 simulations performed by POWSIM showed that the population sample size, number of loci and their allele frequencies have enough statistical power to detect FST values as small as 0.005 with a power approaching 100% under all the tested conditions of effective population sizes and number of generations of drift.
Genetic Variability
For the three datasets, similar values were obtained among the population samples for Ho, unbiased He and AR, confirmed by one-way ANOVA detecting no significant differences among samples (P > 0.05; Supplementary Tables S1–S3). A generalized heterozygote deficit was detected, as evident from the positive values obtained for the inbreeding coefficients (FIS) in all the populations (Supplementary Tables S1–S3). Locus-by-locus analysis showed that the distribution of FIS values is skewed toward positive values, though the majority of the loci show FIS values very close to 0 and few of them negative values (Supplementary Figures S1–S3).
Genetic Structure at the Large Scale
The non-hierarchical AMOVA carried out on the 19 population samples (the overall dataset, 512 polymorphic loci) provided a clear indication of genetic structuring at the large spatial scale, with a statistically significant global multilocus FST (FST = 0.03243, P < 0.0001). Considering the 171 pairwise FST (Table 2), all 90 comparisons involving a location from the Mediterranean Sea and a location from the Black Sea were statistically significant after correction for multiple tests (FST range = 0.04208–0.08079; P < 0.0001), indicating a clear genetic differentiation between the 2 seas. In addition, among Mediterranean samples 14 comparisons out of 45 were significant after multitest correction (FST range = 0.00809–0.02075; P < 0.05); in this case, pairwise differences were mainly due to the deviation of the sample from Montenegro (BOK, 9 significant comparisons). The North-Western (FRN) and the South-Western (TUN) samples were not genetically different despite the geographic distance, and they differed only weakly from the Central Mediterranean ones (3 significant comparisons, 2 of them involving the BOK sample). No significant pairwise FST was detected within the Black Sea.
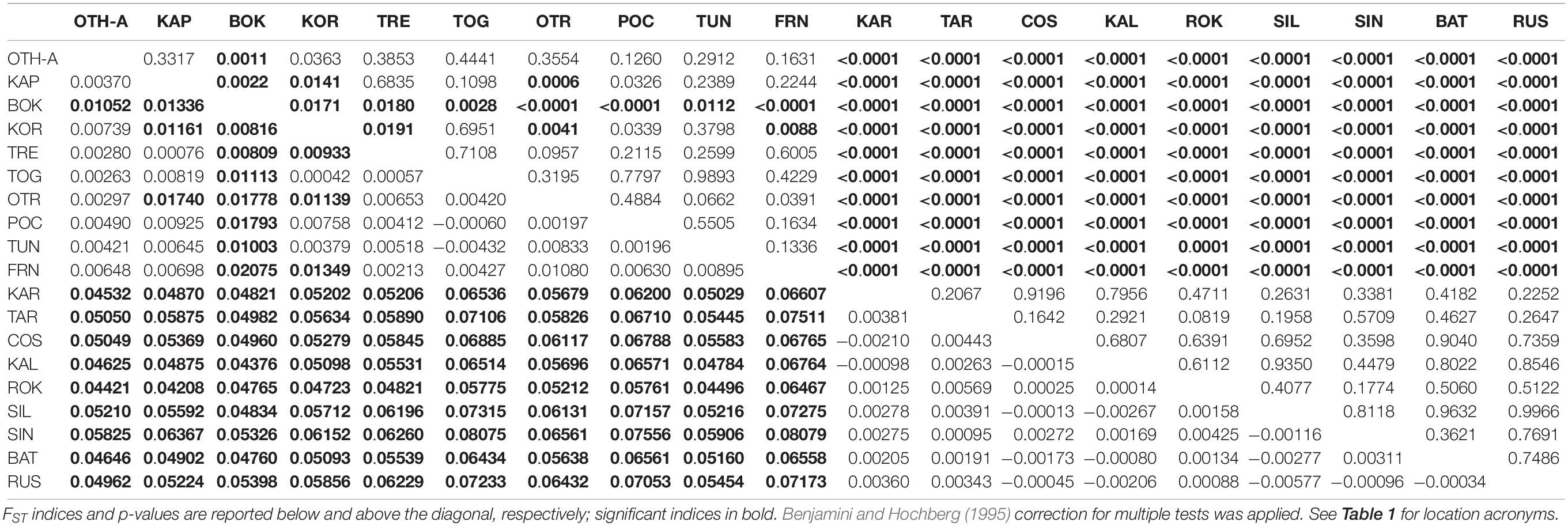
Table 2. Pairwise genetic distances (FST) between all the 19 population samples based on the overall dataset of 512 polymorphic loci.
Hierarchical AMOVA showed that the genetic variation of the dataset can be best partitioned into 2 groups, one including the 10 Mediterranean population samples, and the other one the 9 Black Sea’s population samples (FCT = 0.05286, P < 0.0001), maximizing the genetic variance between groups. Similarly, the DAPC identified two genetic clusters as the optimal clustering solution based on the lowest BIC (Figure 2A), with the 2 clusters corresponding to the Mediterranean Sea and the Black Sea population samples (Figure 2B). Interestingly, the graphs plotting the density of the inferred clusters and the original populations on the single discriminant function described the same trend (Figures 2C,D). The Bayesian clustering carried out by STRUCTURE was consistent with the DAPC, in fact the most probable number of clusters was identified for K = 2 with the same groupings (Figure 3).
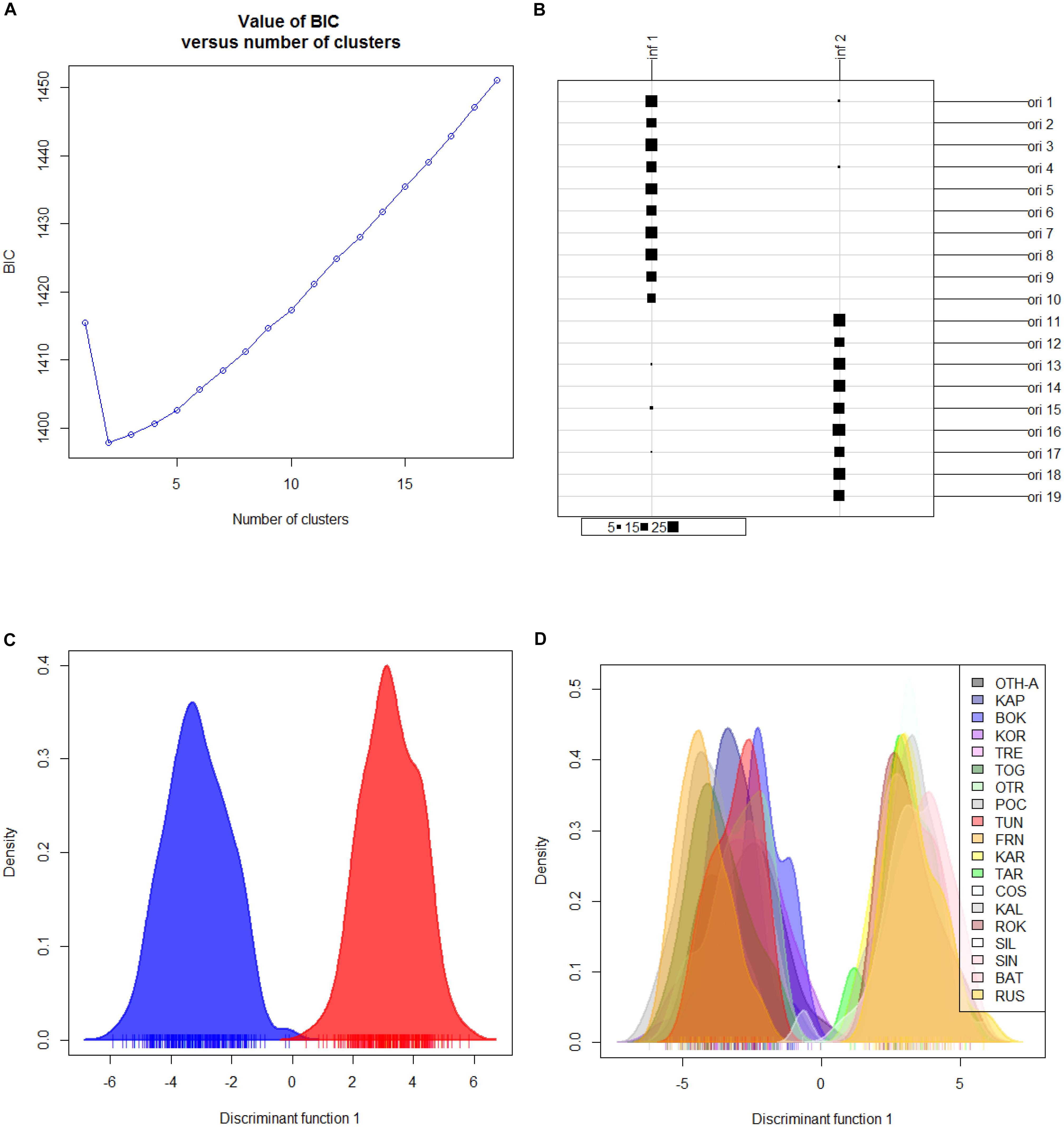
Figure 2. Discriminant analysis of principal components (DAPC) for the overall dataset of 19 population samples from Mediterranean and Black Seas. (A) BIC value. Graph of BIC values for increasing value of number of clusters (k). (B) Graphical table showing the number of original populations (ori) VS the number of inferred clusters (inf) and the group sizes of each correspondence. Original populations legend: ori 1: Corfù Island, Greece (OTH-A); ori 2: Karaburun Peninsula, Albania (KAP); ori 3: Boka Kotorska Bay, Montenegro (BOK); ori 4: Kornati islands, Croatia (KOR); ori 5: Tremiti islands, Italy (TRE); ori 6: Torre Guaceto, Italy (TOG); ori 7: Otranto, Italy (OTR); ori 8: Porto Cesareo, Italy (POC); ori 9: Tunisia (TUN); ori 10: France (FRN); ori 11: Karadag MPA, Ukraine (KAR); ori 12: Tharkhankut, Ukraine (TAR); ori 13: Costinesti Monastery, Romania (COS); ori 14: Cape Kaliakra, Bulgaria (KAL); ori 15: Ropotamo-Kiten, Bulgaria (ROK); ori 16: Sile, Turkey (SIL); ori 17: Sinop, Turkey (SIN); ori 18: Batumi, Georgia (BAT); ori 19: Novorossiysk-Gelendzhik, Russia (RUS). (C) 2D scatterplot representing the density of the inferred numbers of clusters (k = 2) on the single discriminant function. (D) 2D scatterplot representing density of the 19 original populations on the single discriminant function.

Figure 3. Bayesian clustering performed with STRUCTURE on the overall dataset of 19 population samples from Mediterranean and Black seas. Legend: 1: Corfù Island, Greece (OTH-A); 2: Karaburun Peninsula, Albania (KAP); 3: Boka Kotorska Bay, Montenegro (BOK); 4: Kornati islands, Croatia (KOR); 5: Tremiti islands, Italy (TRE); 6: Torre Guaceto, Italy (TOG); 7: Otranto, Italy (OTR); 8: Porto Cesareo, Italy (POC); 9: Tunisia (TUN); 10: France (FRN); 11: Karadag MPA, Ukraine (KAR); 12: Tharkhankut, Ukraine (TAR); 13: Costinesti Monastery, Romania (COS); 14: Cape Kaliakra, Bulgaria (KAL); 15: Ropotamo-Kiten, Bulgaria (ROK); 16: Sile, Turkey (SIL); 17: Sinop, Turkey (SIN); 18: Batumi, Georgia (BAT); 19: Novorossiysk-Gelendzhik, Russia (RUS).
Genetic Structure at the Basin Scale
The lack of genetic structure of the Black Sea and the signal of genetic differentiation in the Central Mediterranean found with the overall dataset were confirmed by the more powerful basin-specific datasets.
The non-hierarchical AMOVA conducted on Adriatic-Ionian dataset (811 polymorphic loci, 8 population samples) pointed out that most of the genetic variation (>99%) arose from the within-populations level and only 0.69% from the among-populations level, with, however, a statistically significant global FST (P < 0.0001). A number of pairwise FST values were statistically significant after correction for multi-test (9/28; Table 3) and, once again, most of these comparisons (6/9) involved the Montenegro sample (BOK); 2 significant values were obtained comparing the Croatia sample (KOR) with the Tremiti islands (TRE) and with Otranto (OTR), and the last significant value was between TRE and OTR. Both the genetic clustering methods found out a single cluster including all the population samples (BIC values of the DAPC in Figure 4; STRUCTURE plot not provided). Interestingly, a hierarchical AMOVA found the best clustering solution for three groups: the first group with the population sample from Tremiti islands (TRE) alone, the second group including BOK sample and the Croatian sample (KOR), and the last including the three other Italian samples (OTR, POC, and TOG), the Greek (OTH-A) and Albanian (KAP) samples (FCT = 0.00312, P < 0.05). The scatterplot of the discriminant analysis set on the original populations reflected the weak genetic differentiation detected among these population samples (Figures 4B,C).
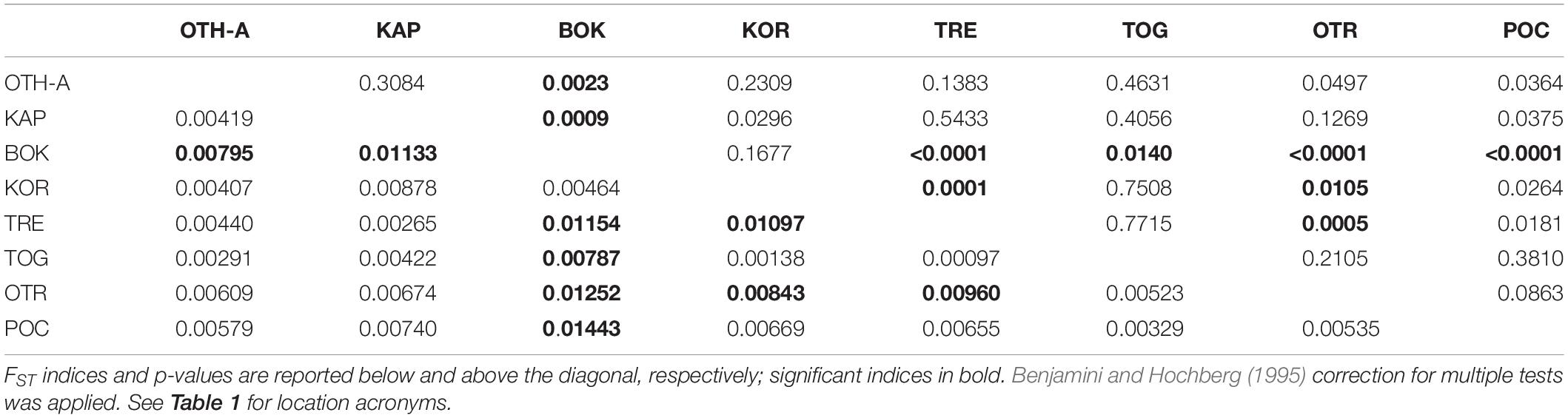
Table 3. Pairwise genetic distances (FST) between the 8 Adriatic-Ionian population samples based on Adriatic-Ionian dataset (811 polymorphic loci).
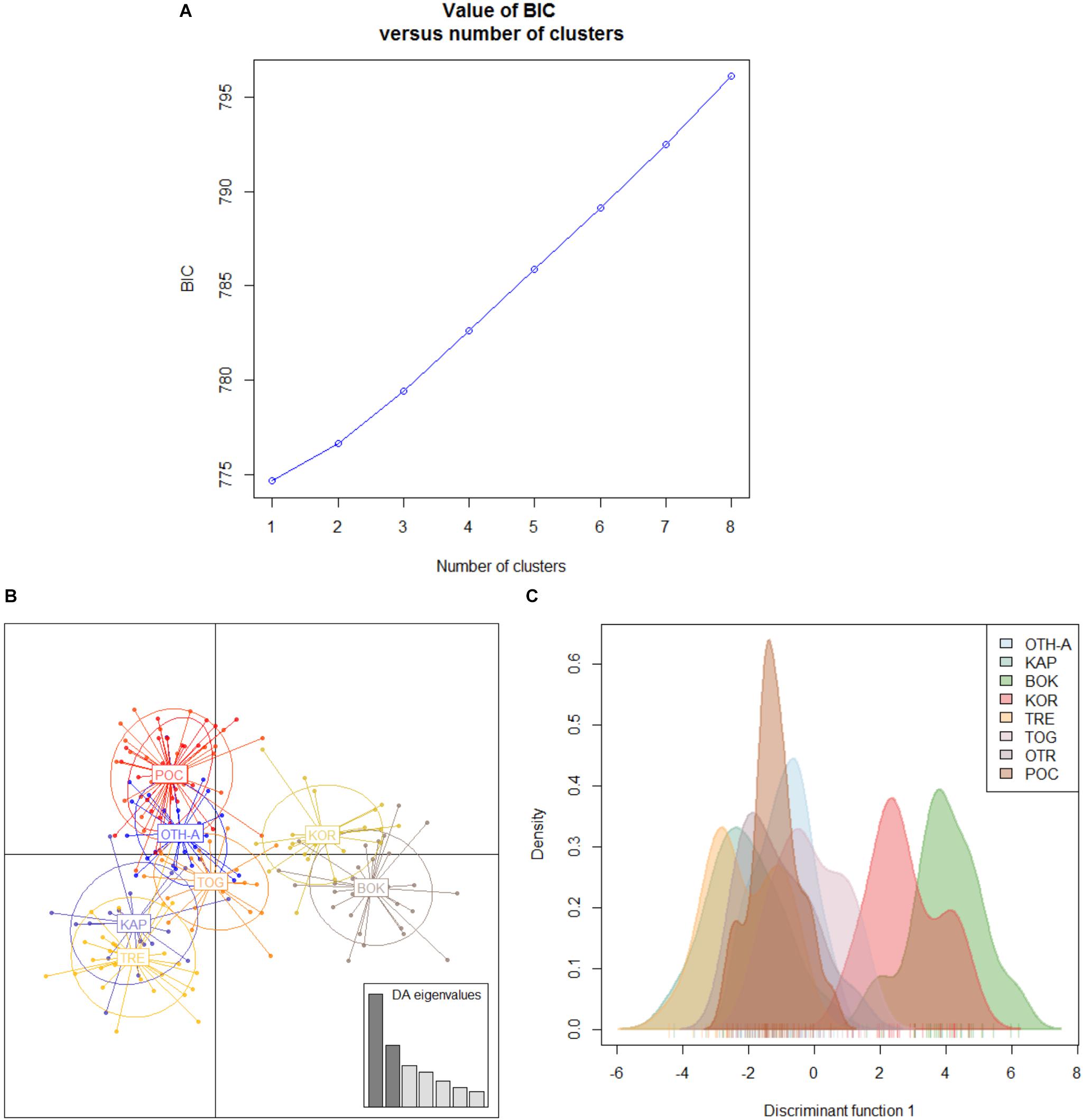
Figure 4. Discriminant analysis of the principal components (DAPC) for the Adriatic-Ionian dataset of 8 population samples. (A) Graph of BIC values for increasing value of number of clusters (k). (B) Scatterplot of the discriminant analysis set on the original populations. (C) 2D scatterplot showing the density of the original populations plotted on the single discriminant. For the acronym locations see Table 1.
The Black Sea dataset (998 polymorphic loci, 9 population samples) revealed a small and not significant global multilocus FST value (FST = 0.00278, P = 0.59584) and no pairwise FST value resulted to be significant within this basin (Table 4). Both Bayesian clustering methods conducted by ADEGENET and STRUCTURE found only one genetic cluster (BIC values of the DAPC in Figure 5; STRUCTURE plot not reported). As expected due to the lack of genetic structuring, the visual assessment of the between-population differentiation of the original populations showed all the samples strongly overlapping (DAPC plots, Figures 5B,C).
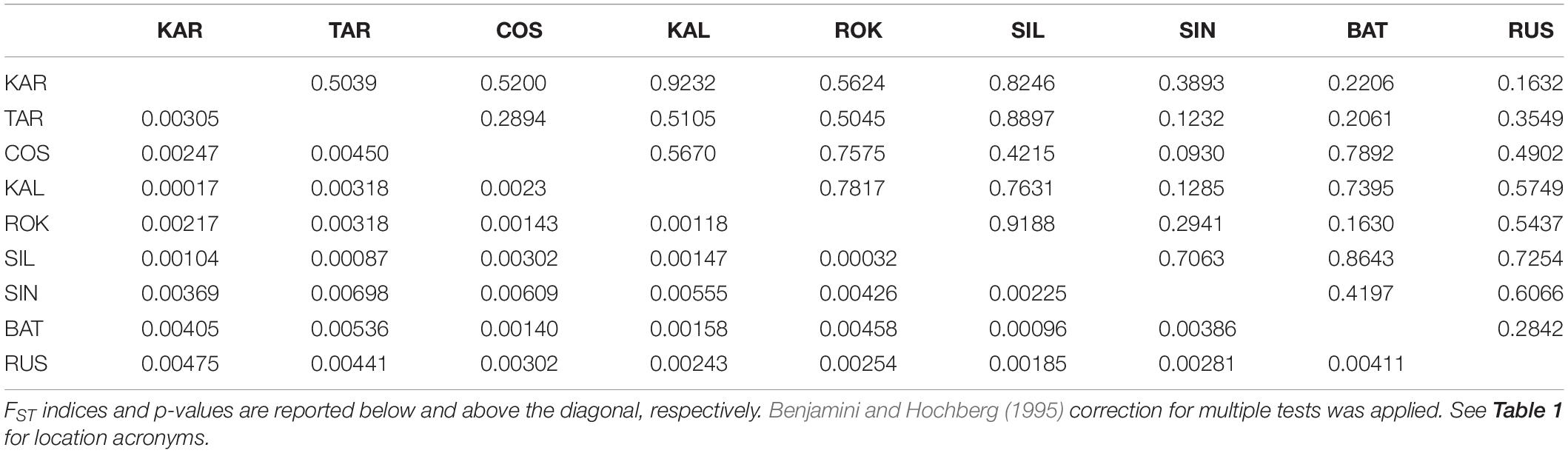
Table 4. Pairwise genetic distances (FST) between the 9 population samples from the Black Sea based on the Black Sea’s dataset (998 polymorphic loci).
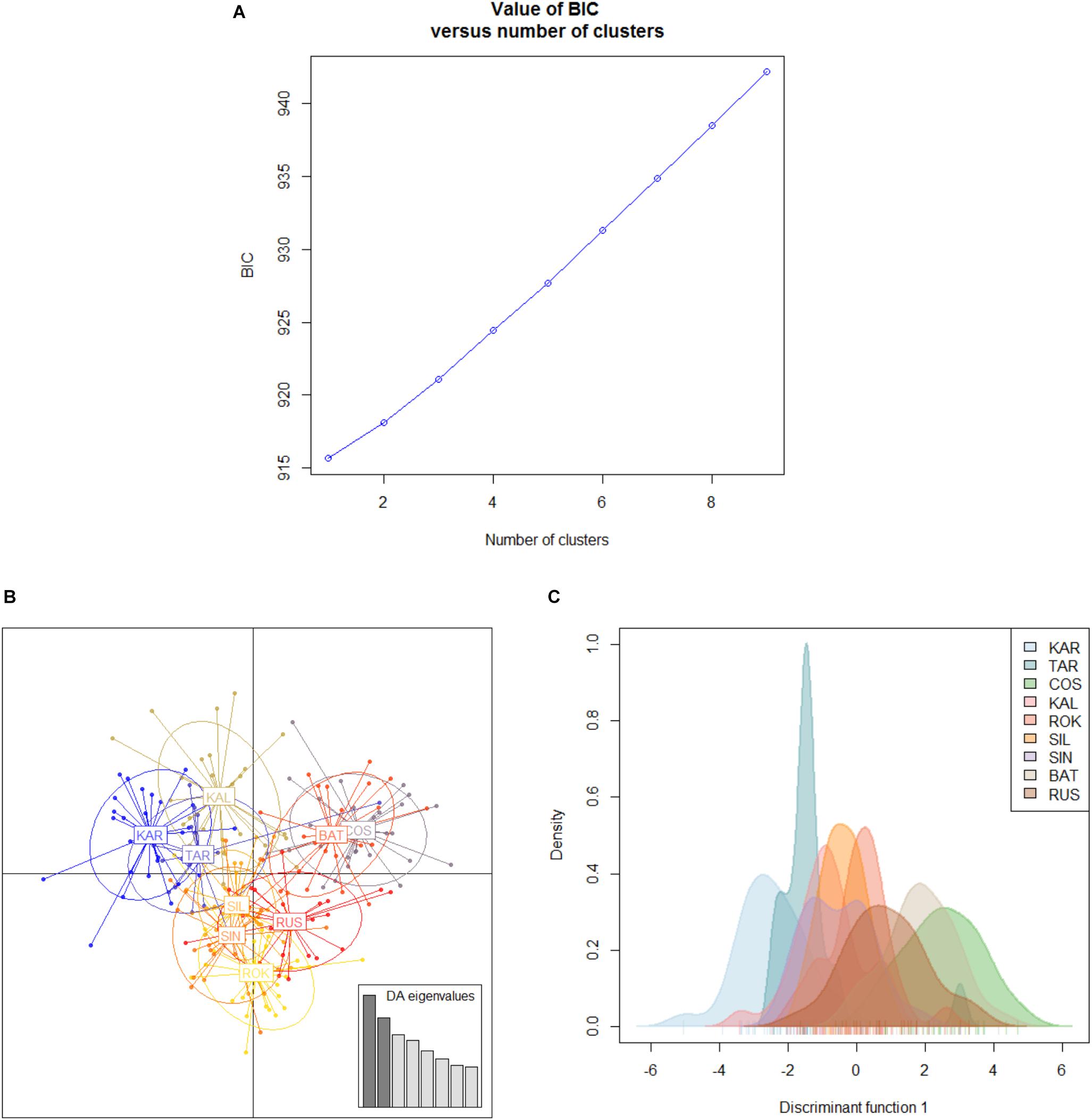
Figure 5. Discriminant analysis of the principal components (DAPC) for the Black Sea’s dataset of 9 population samples. (A) Graph of BIC values for increasing value of number of clusters (k). (B) Scatterplot of the discriminant analysis set on the original populations. (C) 2D scatterplot showing the density of the original populations plotted on the single discriminant. For the acronym locations see Table 1.
Outliers Detection
The outliers detection conducted on the overall dataset identified 25 loci putatively under positive selection. Fourteen out of these 25 loci were detected by both software, and 11 only by BAYESCAN. The exclusion of these loci resulted in a substantial reduction of the global genetic variance of the dataset (FST = 0.01152; P < 0.0001), but the general pattern of structuring was maintained, with the best clustering solution for two groups (Mediterranean population samples against Black Sea’s population samples; FCT = 0.01565; P < 0.0001) and with 86/90 significant pairwise FST distances between the Mediterranean and the Black Sea.
The scan of the Adriatic-Ionian dataset by both software detected 2 putative outliers, whose exclusion caused a small decrease of the molecular variance (FST = 0.00591; P < 0.0001) and the loss of 3 significant pairwise FST, all involving the population sample from Montenegro (BOK). As expected due to the complete lack of genetic differentiation, the scan of the Black Sea’s dataset did not identify any locus putatively under selection within the Black Sea.
Discussion
The genomic analysis of the 19 M. galloprovincialis populations sampled across different locations: (1) provided the first detailed analysis of the metapopulation structure of this species throughout part of its native range at the large scale; (2) allowed to investigate the presence of genetic differentiation at a smaller scale within basins; (3) enabled the comparisons with other CoCoNET species.
Using hundreds SNPs identified and genotyped with the 2b-RAD protocol (Wang et al., 2012), we detected a clear differentiation between the Mediterranean and the Black Sea samples, the existence of weak genetic structuring within the Adriatic-Ionian Sea and a complete genetic homogeneity in the Black Sea. We also detected a generalized heterozygote deficit caused by an excess of loci showing positive FIS values. This result could be explained by an extensive mixing at the local scale, expected for a species with an exceptionally high potential for dispersion such as M. galloprovincialis, leading to a Wahlund effect at all the analyzed locations. However, technical factors linked to genotyping can also lead to loss of heterozygotes, particularly when dealing with Next Generation Sequencing (NGS) approaches (Andrews et al., 2016). Thus, further investigations, with a specific experimental design, are needed to understand the potential biological relevance of the detected heterozygote deficit.
Genetic Structure at the Large Scale
A marked difference in genetic structure comparing the Mediterranean and the Black Seas was found. This result confirms and strengthens the limited genetic data obtained for this species (Ladoukakis et al., 2002), which hinted at reduced gene flow between the two seas. A similar differentiation was previously reported for other species (including anchovies, Magoulas et al., 2006; sticklebacks, Mäkinen and Merilä, 2008; sprats, Limborg et al., 2012; bluefishes, Miralles et al., 2014). The effect of oceanographic features like the narrow Dardanelles and Bosphorus straits and the existence of environmental barriers (salinity, temperature) limit the exchange of water and species between the two seas and are suspected as a probable cause. The extent of isolation of the Black Sea makes it a potential refuge for temperate and cold water species under future warm conditions. The current absence of the harbor porpoise (Phocoena phocoena), a cold-water adapted species, from the Mediterranean Sea and its presence in the Atlantic Ocean and in the Black Sea (the latter colonized not before 5000 years ago) seems to confirm such refuge potential (Fontaine et al., 2014). A post-glacial timing of Black Sea colonization has been recently suggested for the black scorpionfish (Scorpaena porcus Linnaeus, 1758), another species analyzed in the CoCoNET project, facilitated by the reopening of the connection between the Black Sea and the Mediterranean Sea about 8000 years ago (Boissin et al., 2016). Both time estimates are consistent with timing of the Black Sea postglacial transition from a fresh/brackish-water ecosystem to a fully marine ecosystem allowing new colonizations from the Aegean Sea. This shift followed a period of isolation from the Mediterranean due to glacial advance and sea level retreat, when water salinity was strongly reduced leading to the eradication of the preexisting marine fauna (Aksu et al., 1999; Çağatay et al., 2000). It is worth noting that, besides being obtained with different genetic markers, the level of differentiation found between Mediterranean and Black Seas samples (average SNPs FST = 0.058, range 0.042–0.081, our study) is comparable to that found for the black scorpionfish (average microsatellites FST = 0.057, range 0.011–0.126; Boissin et al., 2016), suggesting that a recent colonization of the Black Sea occurred in mussels as well, also considering that microsatellites tend to underestimate genetic differentiation (O’Reilly et al., 2004), due to their higher heterozygosity compared to SNPs.
Within the Mediterranean Sea, no signal of differentiation was found between Western and Central Mediterranean based on mussel population samples. This result is unexpected since the Sicily Channel is known to act as a barrier and seems to prevent the gene flow in many other species (Borsa et al., 1997; De Innocentiis et al., 2004; Zitari-Chatti et al., 2009; Serra et al., 2010; Pascual et al., 2017). The lack of differentiation, however, again agrees with the genetic structure observed for the black scorpionfish (Boissin et al., 2016). A similar lack of differentiation within Western Mediterranean samples from France and Tunisia was found in the present study on mussels despite a geographic separation of more than 1000 km.
Genetic Structure at the Basin Scale
In contrast to the Western Mediterranean, the Central Mediterranean, represented by Adriatic-Ionian mussel population samples, showed a weak but significant genetic differentiation, which was not affected by the presence of a few outliers. Our results confirm and complement previous reports that indicated, despite a limited sampling effort, genetic heterogeneity in the Adriatic for this species (Giantsis et al., 2014a, b). In our study, differences were weak, e.g., Bayesian clustering failed to detect genetically differentiated clusters, and significant pairwise FST comparisons were found mostly in pairing involving BOK sample (Montenegro). However, hierarchical AMOVA clearly indicated that genetic variation of the dataset can be best partitioned into three groups: a first group consisting of TRE sample (Tremiti Islands) alone, a second including BOK and KOR (i.e., Montenegrin and Croatian) samples, and a last group comprising the remaining three Italian samples (POC, OTR, TOG) together with OTH-A and KAP samples (i.e., Greek and Albanian samples respectively). This pattern suggests an East–West differentiation in the Central Adriatic Sea and a further North–South differentiation between the Central Adriatic and the South Adriatic and Ionian Seas. A general homogeneity was found among localities within the latter ones (i.e., South Adriatic-Ionian area). Considering that the adult stage of mussels species is sessile, their dispersal ability (and thus the gene flow) is mostly due to the passive transport of larvae driven by the complex water circulation of the Adriatic and Ionian Seas (i.e., characterized by the presence of minor gyres in addition to the main currents). Indeed, the differentiation found here seems to reflect the general surface circulation of the area and the need to support this hypothesis with further sampling. The Adriatic is characterized by a large-scale cyclonic meander, with a northerly flow along the East coast and a southerly flow along the West one (Orlić et al., 1992). Within this large system, three cyclonic gyres further divide the basin into three regions named North, Central and South Adriatic Sea (hereafter NAd, Cad, and SAd respectively). The NAd gyre is defined mainly in autumn and located at the surface, while the middle MAd and SAd gyres become more intense in summer and autumn (Artegiani et al., 1997). According to this, the genetic subdivision detected by AMOVA suggests that the boundary between the MAd and SAd gyres can represent an area of genetic discontinuity, with the MAd unable to prevent East to West differentiation in the Central Adriatic and the SAd promoting an effective mixing of the genetic pool in the Southern Adriatic, further extending to the Ionian Sea. Interestingly, also the population of shore crab Carcinus aestuarii follow this pattern as highlighted with genetic markers and biophysical models provided by Schiavina et al. (2014).
As far as in the Black Sea we found a complete lack of genetic structure, the current patterns combined with the long PLD probably ensure a good connectivity among the sampled locations. Unfortunately, the lack of baseline information regarding M. galloprovincialis population genetic structure in the Black Sea impair us to make comparisons. Further insights can be obtained exclusively by comparison with the black scorpionfish Scorpaena porcus populations (Boissin et al., 2016) also showing a remarkably homogeneous genetic structure in the basin Black Sea.
Comparison With Other CoCoNET Species
Striking similarities occur between M. galloprovincialis and the black scorpionfish analyzed in the CoCoNET project. Both species show Mediterranean/Black Sea weak but significant differentiation, no differences between Western and Central Mediterranean samples, genetic heterogeneity among Adriatic-Ionian samples and genetic homogeneity in the Black Sea (Boissin et al., 2016). However, when the comparison is extended to other species analyzed in the CoCoNET project in the Adriatic-Ionian basins, the picture becomes more nuanced.
Black scorpionfish are benthic, with a pelagic larval stage life duration of about 4 weeks (Raventós and Macpherson, 2001). Within the Adriatic-Ionian basins they show a clear East to West genetic break between population samples (Boissin et al., 2016), extending to southern localities the pattern found in the Central Adriatic in M. galloprovincialis. In this case, the East to West differentiation seems to prevail on the Adriatic sub-basin regionalization based on oceanography, suggesting that both the MAd and the SAd gyres are not able to effectively connect eastern and western localities across the sea, whereas dispersal alongshore and nearby islands (TRE in the West or KOR and OTH-A in the East) is sufficient to prevent differentiation.
The east Atlantic peacock wrasse Symphodus tinca (Linnaeus, 1758) is a demersal fish with a larval duration of about 10 days (Raventós and Macpherson, 2001). The evaluation of the population structure of this species in the Adriatic-Ionian basins identified the existence of 2 barriers to gene flow separating as before the eastern shore localities from the western ones, but also differentiating the Tremiti islands’s sample (TRE) from all the others (Carreras et al., 2017). Thus, in this case, the Tremiti islands sample seems not connected with the samples collected from the mainland Italian coast, which might be tentatively explained with its shorter larval duration and nearshore larval distribution.
For the marble crab Pachygrapsus marmoratus (Fabricius, 1787), a rocky shore crab species with a PLD of about 4 weeks, population samples collected at the same Adriatic-Ionian localities showed a fairly genetic homogeneity, with a weak differentiation only due to a single locus putatively under selection (Marino et al., personal communication). This result could be explained with the existence of random fluctuations of allele frequencies depending on variability in fecundity, mortality or reproductive success, that can lead to a scenario of genetic patchiness in a general context of genetic homogeneity, as was previously reported by different studies on this species (Silva et al., 2009; Fratini et al., 2011, 2013, 2016).
At the extremes of this differentiation range, still considering a propagule duration of about one month, the habitat former Posidonia oceanica (Jahnke et al., 2017) and the sea urchin Paracentrotus lividus (Lamarck, 1816) (Paterno et al., 2017) showed completely different patterns. The seagrass P. oceanica (Linnaeus Delile, 1813) showed a marked genetic structuring within and between Adriatic-Ionian basins, even between the closest localities, with a possible north-to-south subdivision (Jahnke et al., 2017). On the other side, the analysis of P. lividus samples indicated a clear genetic homogeneity in the same area, with a broadly consistent prediction of the potential larval exchange among the same sites (obtained with Lagrangian simulations). This latter outcome supports the view of a relatively persistent flux of propagules from each sites to the closest neighbor, mostly in a counterclockwise direction (Paterno et al., 2017).
Implications for MPAs
MPAs have been increasingly considered the most effective tool for conservation and management of marine environment, able to mitigate the alteration of ecosystems and the negative effect of human activities (Gaines et al., 2010; Gabrié et al., 2012). The implementation of networks of MPAs, where the protected areas can cooperatively operate, is the new conservation target, because it is supposed that networks could be more effective and offer more protection than the individual sites alone (Wood et al., 2008; Wabnitz et al., 2010; Fenberg et al., 2012; Grorud-Colvert et al., 2014). Connectivity data obtained so far in the CoCoNET study area, highlighted species-specific connectivity patterns, ranging from the total absence of genetic differentiation (P. lividus) to a strong structuring (P. oceanica). In the marine environment, historical and evolutionary processes as well as temperature and ocean currents strongly contribute in shaping species distribution at large biogeographical scale and thus determine the different connectivity patterns observed. Altogether, the framework developed in CoCoNET highlighted the importance of evaluating and discussing the genetic data of population structure in the light of those biological, physical and ecological factors that could have a significant role in shaping the genetic variability of these species. In a management and conservation perspective, the implementation of ecological effective networks of MPAs should rely on the knowledge of connectivity patterns of a representative panel of species living in the selected areas.
The different connectivity outcomes so far obtained (i) indicate the importance of evaluating the genetic distribution of the species under a seascape genomics framework (Di Franco et al., 2012; Pujolar et al., 2013; Paterno et al., 2017); (ii) warn against any generalization in defining conservation units based on the results of a single species because they cannot represent the entire community (Melià et al., 2016); (iii) suggest to take into account connectivity data for different species with a variety of life history traits to improve the efficacy of the management and conservation strategy of the marine ecosystem (Pascual et al., 2017). Besides these limitations, on the basis of the study outcomes, we can anticipate that the delineation of Cells of Ecosystem Functioning, ecologically coherent units of management based on connectivity (Boero et al., 2016), will likely result in much smaller units of conservation in the Adriatic-Ionian area than in the well-connected Black Sea, this latter being particularly promising for the establishment of “a well-connected system of protected areas” according to Aichi Target 11 of the Convention on Biological diversity (Brooks, 2014).
Data Availability
The datasets for this study can be found in Dryad at https://doi.org/10.5061/dryad.4177rd5. Short read data are deposited in SRA with BioProject accession number PRJNA564770.
Author Contributions
MPat and LZ conceived and designed the experiments, analyzed the data, and wrote the manuscript. MPat performed the experiments. LZ contributed reagents, materials, and analysis tools. All authors equally contributed in reviewing and approving the final version of the manuscript. LB, JS, AC, GG, CK, CP, VM, DM, NM, MPan, SP, VT, and EV contributed to the sampling and logistics.
Conflict of Interest Statement
The authors declare that the research was conducted in the absence of any commercial or financial relationships that could be construed as a potential conflict of interest.
Funding
This research was supported by the European Community’s 7th Framework Programme (FP7/2007-2013) under Grant Agreement No. 287844 for the project “Towards COast to COast NETworks of marine protected areas (from the shore to the high and deep sea), coupled with sea-based wind energy potential (CoCoNet, http://www.coconet-fp7.eu/)”, and by the University of Padua grant CPDA148387/14.
Acknowledgments
We are thankful to the following people for their precious scientific inputs, and for their help for logistics and collecting samples on the field: F. Boero and S. Fraschetti (University of Naples Federico II, Italy) and S. Bevilacqua and A. Terlizzi (University of Trieste, Italy), S. Reizopoulou and M. A. Pancucci-Papadopoulou (HCMR, Greece), S. Beqiraj (Department of Biology, Faculty of Natural Sciences, University of Tirana), E. Hajdëri (Catholic University “Our Lady of Good Counsel,” Tirana), and V. Neglia and N. Tolou (CRIOBE, Université de Perpignan).
Supplementary Material
The Supplementary Material for this article can be found online at: https://www.frontiersin.org/articles/10.3389/fmars.2019.00566/full#supplementary-material
Footnotes
References
Aksu, A. E., Hiscott, R. N., and Yasar, D. (1999). Oscillating Quaternary water levels of the Marmara Sea and vigorous outflow into the Aegean Sea from the Marmara Sea–Black Sea drainage corridor. Mar. Geol. 153, 275–302. doi: 10.1016/S0025-3227(98)00078-4
Andrews, K. R., Good, J. M., Miller, M. R., Luikart, G., and Hohenlohe, P. A. (2016). Harnessing the power of RADseq for ecological and evolutionary genomics. Nat. Rev. Genet. 17, 81–92. doi: 10.1038/nrg.2015.28
Antao, T., Lopes, A., Lopes, R. J., Beja-Pereira, A., and Luikart, G. (2008). LOSITAN: a workbench to detect molecular adaptation based on a FST-outlier method. BMC Bioinformatics 9:323. doi: 10.1186/1471-2105-9-323
Artegiani, A., Bregant, D., Paschini, E., Pinardi, N., Raicich, F., and Russo, A. (1997). The Adriatic sea general circulation. Part I: air–sea interactions and water mass structure. J. Phys. Ocean 27, 1492–1514. doi: 10.1175/1520-04851997027<1492:TASGCP<2.0.CO;2
Astorga, M. P. (2014). Genetic considerations for mollusc production in aquaculture: current state of knowledge. Front. Genet. 5:435. doi: 10.3389/fgene.2014.00435
Becker, B. J., Levin, L. A., Fodrie, F. J., and MacMillan, P. A. (2007). Complex larval connectivity patterns among marine invertebrate populations. Proc. Natl. Acad. Sci. U.S.A. 104, 3267–3272. doi: 10.1073/pnas.0611651104
Belkhir, K., Borsa, P., Chikhi, L., Raufaste, N., and Bonhomme, F. (2000). GENETIX 4.02, Logiciel Sous Windows TM Pour La Génétique Des Populations. Laboratoire Génome, Populations Interactions, CNRS UMR 5000. Montpellier: Université de Montpellier II.
Benjamini, Y., and Hochberg, Y. (1995). Controlling the false discovery rate: a practical and powerful approach to multiple testing. J. R. Stat. Soc. Ser. B 57, 289–300. doi: 10.1111/j.2517-6161.1995.tb02031.x
Bierne, N., David, P., Boudry, P., and Bonhomme, F. (2002). Assortative fertilization and selection at larval stage in the mussels Mytilus edulis and M. galloprovincialis. Evolution 56, 292–298. doi: 10.1111/j.0014-3820.2002.tb01339.x
Boero, F., Foglini, F., Fraschetti, S., Goriup, P., Planes, S., Soukissian, T., et al. (2016). CoCoNet: towards coast to coast networks of Marine Protected Areas (From the shore to the high and deep sea), coupled with sea-based wind energy potential. SCIRES IT 6, 1–95. doi: 10.2423/i22394303v6SpI
Boissin, E., Micu, D., Janczyszyn-Le Goff, M., Neglia, V., Bat, L., Todorova, V., et al. (2016). Contemporary genetic structure and post-glacial demographic history of the black scorpionfish, Scorpaena porcus, in the mediterranean and the black seas. Mol. Ecol. 25, 2195–2209. doi: 10.1111/mec.13616
Borsa, P., Blanquer, A., and Berrebi, P. (1997). Genetic structure of the flounders Platichthys flesus and P. stellatus at different geographic scales. Mar. Biol. 129, 233–246. doi: 10.1007/s002270050164
Boscari, E., Abbiati, M., Badalamenti, F., Bavestrello, G., Cannas, R., Cau, A., et al. (2019). A population genomics insight by 2b-RAD reveals populations’ uniqueness along the Italian coastline in Leptopsammia pruvoti (Scleractinia, Dendrophylliidae). Div. Distrib. 25, 1101–1117. doi: 10.1111/ddi.12918
Broquet, T., and Petit, E. J. (2009). Molecular estimation of dispersal for ecology and population genetics. Annu. Rev. Ecol. Evol. Syst. 40, 193–216. doi: 10.1146/annurev.ecolsys.110308.120324
Cáceres-Martínez, J., and Figueras, A. (1998). Long-term survey on wild and cultured mussels (Mytilus galloprovincialis Lmk) reproductive cycles in the Ria de Vigo (NW Spain). Aquaculture 162, 141–156. doi: 10.1016/S0044-8486(98)00210-5
Çağatay, M. N., Görür, N., Algan, O., Eastoe, C., Tchapalyga, A., Ongan, D., et al. (2000). Late Glacial–Holocene palaeoceanography of the Sea of Marmara: timing of connections with the Mediterranean and the Black Seas. Mar. Geol. 167, 191–206. doi: 10.1016/S0025-3227(00)00031-1
Carreras, C., Ordóñez, V., Zane, L., Kruschel, C., Nasto, I., Macpherson, E., et al. (2017). Population genomics of an endemic Mediterranean fish: differentiation by fine scale dispersal and adaptation. Sci. Rep. 7:43417. doi: 10.1038/srep43417
Catchen, J., Hohenlohe, P. A., Bassham, S., Amores, A., and Cresko, W. A. (2013). Stacks: an analysis tool set for population genomics. Mol. Ecol. 22, 3124–3140. doi: 10.1111/mec.12354
Chattopadhyay, B., Garg, K. M., and Ramakrishnan, U. (2014). Effect of diversity and missing data on genetic assignment with RAD-Seq markers. BMC Res. Notes 7:841. doi: 10.1186/1756-0500-7-841
Chicaro, L. M. Z., and Chicaro, M. A. (2000). Short-term fluctuations in bivalve larvae compared with some environmental factors in a coastal lagoon (South Portugal). Sci. Mar. 64, 413–420. doi: 10.3989/scimar.2000.64n4413
Coombs, J. A., Letcher, B. H., and Nislow, K. H. (2008). Create: a software to create input files from diploid genotypic data for 52 genetic software programs. Mol. Ecol. Res. 8, 578–580. doi: 10.1111/j.1471-8286.2007.02036.x
Cowen, R. K., Gawarkiewicz, G., Pineda, J., Thorrold, S. R., and Werner, F. E. (2007). Population connectivity in marine systems: an overview. Oceanography 20, 14–21. doi: 10.5670/oceanog.2007.26
Cowen, R. K., Paris, C. B., and Srinivasan, A. (2006). Scaling of connectivity in marine populations. Science 311, 522–527. doi: 10.1126/science.1122039
Cowen, R. K., and Sponaugle, S. (2009). Larval dispersal and marine population connectivity. Annu. Rev. Mar. Sci. 1, 443–466. doi: 10.5670/oceanog.2007.26
Cruz, F., Montse, P., and Presa, P. (2005). Distribution and abundance of microsatellites in the genome of bivalves. Gene 346, 241–247. doi: 10.1016/j.gene.2004.11.013
Da Ros, L., Bressan, M., and Marin, M. G. (1985). Reproductive cycle of the mussel (Mytilus galloprovincialis Lmk) in Venice Lagoon (North Adriatic). Italian J. Zool. 52, 223–229. doi: 10.1080/11250008509440522
Daguin, C., and Borsa, P. (2000). “Genetic relationships of Mytilus galloprovincialis populations worldwide: evidence from nuclear-DNA markers,” in The evolutionary biology of the Bivalvia, Vol. 177, eds E. M. Harper, J. D. Taylor, and J. A. Crame, (London: Geological Society), 389–397. doi: 10.1144/gsl.sp.2000.177.01.26
De Innocentiis, S., Lesti, A., Livi, S., Rossi, A. R., Crosetti, D., and Sola, L. (2004). Microsatellite markers reveal population structure in gilthead sea bream Sparus auratus from the Atlantic Ocean and Mediterranean Sea. Fish. Sci. 70, 852–859. doi: 10.1111/j.1444-2906.2004.00879.x
Di Franco, A., Coppini, G., Pujolar, J. M., De Leo, G. A., Gatto, M., Lyubartsev, V., et al. (2012). Assessing dispersal patterns of fish propagules from an effective mediterranean marine protected area. PLoS One 7:e52108. doi: 10.1371/journal.pone.0052108
Earl, D. A., and VonHoldt, B. M. (2012). STRUCTURE HARVESTER: a website and program for visualizing STRUCTURE output and implementing the Evanno method. Cons. Genet. Res. 4, 359–361. doi: 10.1007/s12686-011-9548-7
Excoffier, L., and Lischer, H. E. L. (2010). Arlequin Suite ver 3.5: a new series of programs to perform population genetics analyses under Linux and Windows. Mol. Ecol. Res. 10, 564–567. doi: 10.1111/j.1755-0998.2010.02847.x
Excoffier, L., Smouse, P. E., and Quattro, J. M. (1992). Analysis of molecular variance inferred from metric distances among DNA haplotypes: application to human mitochondrial DNA restriction data. Genetics 131, 479–491.
Fenberg, P. B., Caselle, J. E., Claudet, J., Clemence, M., Gaines, S. D., García-Charton, J. A., et al. (2012). The science of European marine reserves: status, efficacy, and future needs. Mar. Policy 36, 1012–1021. doi: 10.1016/j.marpol.2012.02.021
Foll, M., and Gaggiotti, O. E. (2008). A genome scan method to identify selected loci appropriate for both dominant and codominant markers: a bayesian perspective. Genetics 180, 977–993. doi: 10.1534/genetics.108.092221
Fontaine, M. C., Roland, K., Calves, I., Austerlitz, F., Palstra, F. P., Tolley, K. A., et al. (2014). Postglacial climate changes and rise of three ecotypes of harbour porpoises, Phocoena phocoena, in western Palearctic waters. Mol. Ecol. 23, 3306–3321. doi: 10.1111/mec.12817
Fortuna, M. A., Albaladejo, R. G., Fernández, L., Aparicio, A., and Bascompte, J. (2009). Networks of spatial genetic variation across species. Proc. Natl. Acad. Sci. U.S.A. 106, 19044–19040. doi: 10.1073/pnas.0907704106
Fratini, S., Ragionieri, L., Cutuli, G., Vannini, M., and Cannicci, S. (2013). Pattern of genetic isolation in the crab Pachygrapsus marmoratus within the Tuscan Archipelago (Mediterranean Sea). Mar. Ecol. Prog. Sci. 478, 173–183. doi: 10.3354/meps10247
Fratini, S., Ragionieri, L., Deli, T., Harrer, A., Marino, I. A. M., Cannicci, S., et al. (2016). Unravelling population genetic structure with mitochondrial DNA in a notional panmictic coastal crab species: sample size makes the difference. BMC Evol. Biol. 16:150. doi: 10.1186/s12862-016-0720-2
Fratini, S., Schubart, C. D., and Ragionieri, L. (2011). “Population genetics in the rocky shore crab Pachygrapsus marmoratus from the western Mediterranean and eastern Atlantic: complementary results from mtDNA and microsatellites at different geographic scales,” in Phylogeography and Population Genetic in Crustacea, eds C. Held, S. Koenemann, and C. D. Schubart, (Boca Raton: CRC Press), 191–213. doi: 10.1201/b11113-12
Gabrié, C., Lagabrielle, E., Bissery, C., Crochelet, E., Meola, B., Webster, C., et al. (2012). The Status of Marine Protected Areas in the Mediterranean Sea. Marseille: MedPAN, 256.
Gaines, S. D., White, C., Carr, M. H., and Palumbi, S. R. (2010). Designing marine reserve networks for both conservation and fisheries management. Proc. Natl. Acad. Sci. U.S.A. 43, 18286–18293. doi: 10.1073/pnas.0906473107
Gardner, J. P. A., Zbawicka, M., Westfall, M., and Wenne, R. (2016). Invasive blue mussels threaten regional scale genetic diversity in mainland and remote offshore locations: the need for baseline data and enhanced protection in the Southern Ocean. Glob. Change Biol. 22, 3182–3195. doi: 10.1111/gcb.13332
Gerard, K., Bierne, N., Borsa, P., Chenuil, A., and Feral, J. P. (2008). Pleistocene separation of mitochondrial lineages of Mytilus spp. mussels from Northern and Southern Hemispheres and strong genetic differentiation among southern populations. Mol. Phylogenet. Evol. 49, 84–91. doi: 10.1016/j.ympev.2008.07.006
Giantsis, I. A., Abatzopoulos, T. J., Angelidis, P., and Apostolidis, A. P. (2014a). Mitochondrial control region variability in Mytilus galloprovincialis populations from the Central-Eastern Mediterranean Sea. Int. J. Mol. Sci. 15, 11614–11625. doi: 10.3390/ijms150711614
Giantsis, I. A., Mucci, N., Randi, E., Abatzopoulos, T. J., and Apostolidis, A. P. (2014b). Microsatellite variation of mussels (Mytilus galloprovincialis) in central and eastern Mediterranean: genetic panmixia in the Aegean and the Ionian Seas. J. Mar. Biol. Assoc. 94, 797–809. doi: 10.1017/S0025315414000174
Gilg, M. R., and Hilbish, T. J. (2003). The geography of marine larval dispersal: coupling genetics with fine-scale physical oceanography. Ecology 84, 2989–2998. doi: 10.1890/02-0498
Gosling, E. (1992). “Genetics of Mytilus,” in The Mussels Mytilus: Ecology, Physiology, Genetics and Culture ed. E. Gosling, (Amsterdam: Elsevier), 309–382.
Grorud-Colvert, K., Claudet, J., Tissot, B. N., Caselle, J. E., Carr, M. H., Day, J. C., et al. (2014). Marine protected area networks: assessing whether the whole is greater than the sum of its parts. PLoS One 9:e102298. doi: 10.1371/journal.pone.0102298
Hastings, A., and Harrison, S. (1994). Metapopulation dynamics and genetics. Annu. Rev. Ecol. Syst. 25, 167–188. doi: 10.1146/annurev.es.25.110194.001123
Hellberg, M. E., Burton, R. S., Neigel, J. E., and Palumbi, S. R. (2002). Genetic assessment of connectivity among marine populations. Bull. Mar. Sci. 70, 273–290.
Hubisz, M. J., Falush, D., Stephens, M., and Pritchard, J. K. (2009). Inferring weak population structure with the assistance of sample group information. Mol. Ecol. Res. 9, 1322–1332. doi: 10.1111/j.1755-0998.2009.02591.x
Jahnke, M., Casagrandi, R., Melià, P., Schiavina, M., Schultz, S. T., Zane, L., et al. (2017). Potential and realized connectivity of the seagrass Posidonia oceanica and their implication for conservation. Div. Distrib. 23, 1423–1434. doi: 10.1111/ddi.12633
Jahnke, M., Christensen, A., Micu, D., Milchakova, N., Sezgin, M., Todorova, V., et al. (2016). Patterns and mechanisms of dispersal in a keystone seagrass species. Mar. Environ. Res. 117, 54–62. doi: 10.1016/j.marenvres.2016.04.004
Jombart, T. (2008). Adegenet: a R package for the multivariate analysis of genetic markers. Bioinformatics 24, 1403–1405. doi: 10.1093/bioinformatics/btn129
Kalinowski, S. T. (2005). HP-RARE 1.0: a computer program for performing rarefaction on measures of allelic richness. Mol. Ecol. Notes 5, 187–189. doi: 10.1111/j.1471-8286.2004.00845.x
Kijewski, T., Śmietanka, B., Zbawicka, M., Gosling, E., Hummel, H., and Wenne, R. (2011). Distribution of Mytilus taxa in European coastal areas as inferred from molecular markers. J. Sea Res. 65, 224–234. doi: 10.1016/j.seares.2010.10.004
Ladoukakis, E. D., Saavedra, C., Magoulas, A., and Zouros, E. (2002). Mitochondrial DNA variation in a species with two mitochondrial genomes: the case of Mytilus galloprovincialis from the Atlantic, the Mediterranean and the Black Sea. Mol. Ecol. 11, 755–769. doi: 10.1046/j.1365-294X.2002.01473.x
Lane, D. J. W., Beaumont, A. R., and Hunter, J. R. (1985). Byssus drifting and the drifting threads of the young post-larval mussel Mytilus edulis. Mar. Biol. 84, 301–303. doi: 10.1007/BF00392500
Larraín, M. A., Zbawicka, M., Araneda, C., Gardner, J. P. A., and Wenne, R. (2018). Native and invasive taxa on the Pacific coast of South America: impacts on aquaculture, traceability and biodiversity of blue mussels (Mytilus spp.). Evol. Appl. 11, 298–311. doi: 10.1111/eva.12553
Levin, L. A. (1990). A review of methods for labeling and tracking marine invertebrate larvae. Ophelia 32, 115–144. doi: 10.1080/00785236.1990.10422028
Limborg, M. T., Hanel, R., Debes, P. V., Andrè, C., Tsigenopoulos, C. S., and Bekkevold, D. (2012). Imprints from genetic drift and mutation imply relative divergence times across marine transition zones in a pan-European small pelagic fish (Sprattus sprattus). Heredity 109, 96–107. doi: 10.1038/hdy.2012.18
Lischer, H. E. L., and Excoffier, L. (2012). PGDSpider: an automated data conversion tool for connecting population genetics and genomics programs. Bioinformatics 28, 298–299. doi: 10.1093/bioinformatics/btr642
Lowe, W. H., and Allendorf, F. W. (2010). What can genetics tell us about population connectivity? Mol. Ecol. 19, 3038–3051. doi: 10.1111/j.1365-294X.2010.04688.x
Magoulas, A., Castilho, R., Caetano, S., Marcato, S., and Patarnello, T. (2006). Mitochondrial DNA reveals a mosaic pattern of phylogeographical structure in Atlantic and Mediterranean populations of anchovy (Engraulis encrasicolus). Mol. Phylogenet. Evol. 39, 734–746. doi: 10.1016/j.ympev.2006.01.016
Mäkinen, H., and Merilä, J. (2008). Mitochondrial DNA phylogeography of the three-spined stickleback (Gasterosteus aculeatus) in Europe - Evidence for multiple glacial refugia. Mol. Phylogenet. Evol. 46, 167–182. doi: 10.1016/j.ympev.2007.06.011
McDonald, J. H., Seed, R., and Koehn, R. K. (1991). Allozymes and morphometric characters of three species of Mytilus in the Northern and Southern Hemispheres. Mar. Biol. 111, 323–333. doi: 10.1007/bf01319403
McInerney, C. E., Allcock, A. L., Johnson, M. P., Bailie, D. A., and Prodohl, P. A. (2011). Comparative genomic analysis reveals species-dependent complexities that explain difficulties with microsatellite marker development in Molluscs. Heredity 106, 78–87. doi: 10.1038/hdy.2010.36
Melià, P., Schiavina, M., Rossetto, M., Gatto, M., Fraschetti, S., and Casagrandi, R. (2016). Looking for hotspots of marine metacommunity connectivity: a methodological framework. Sci. Rep. 6:23705. doi: 10.1038/srep23705
Mileikovsky, S. A. (1971). Types of larval development in marine bottom invertebrates, their distribution and ecological significance: a re-evaluation. Mar. Biol. 10, 193–213. doi: 10.1007/BF00352809
Miller, J. A., Carlton, J. T., Chapman, J. W., Gellerd, J. B., and Ruize, G. M. (2018). Transoceanic dispersal of the mussel Mytilus galloprovincialis on Japanese tsunami marine debris: an approach for evaluating rafting of a coastal species at sea. Mar. Pollut. Bull. 32, 60–69. doi: 10.1016/j.marpolbul.2017.10.040
Miralles, L., Juanes, F., Pardiñas, A. F., and Garcia-Vazquez, E. (2014). Paleoclimate shaped bluefish structure in the Northern Hemisphere. Fisheries 39, 578–586. doi: 10.1080/03632415.2014.976701
Moritz, C. (1994). Defining ‘Evolutionarily Significant Units’ for conservation. Trends Ecol. Evol. 9, 373–375. doi: 10.1016/0169-5347(94)90057-4
O’Reilly, P. T., Canino, M. F., Bailey, K. M., and Bentzen, P. (2004). Inverse relationship between FST and microsatellite polymorphism in the marine fish, walleye pollock (Theragra chalcogramma): implications for resolving weak population structure. Mol. Ecol. 13, 1799–1814. doi: 10.1111/j.1365-294X.2004.02214.x
Orlić, M., Gačić, M., and La Violette, P. E. (1992). The currents and circulation of the Adriatic Sea. Oceanol. Acta 15, 109–124.
Palumbi, S. R. (2003). Population genetics, demographic connectivity, and the design of marine reserves. Ecol. Appl. 13, 146–158. doi: 10.1890/1051-0761(2003)013
Pascual, M., Rives, B., Schunter, C., and Macpherson, E. (2017). Impact of life history traits on gene flow: a multispecies systematic review across oceanographics barriers in the Mediterranean Sea. PLoS One 12:e0176419. doi: 10.1371/journal.pone.0176419
Paterno, M., Schiavina, M., Aglieri, G., Ben Souissi, J., Boscari, E., Casagrandi, R., et al. (2017). Population genomics meet Lagrangian simulations: oceanographic patterns and long larval duration ensure connectivity among Paracentrotus lividus populations in the Adriatic and Ionian seas. Ecol. Evol. 7, 2463–2479. doi: 10.1002/ece3.2844
Phillips, C. (2005). “Using Online Databases for Developing SNP Markers of Forensic Interest,” in Forensic DNA Typing Protocols, ed. A. Carracedo, (Totowa, NJ: Humana Press), 83–105.
Pritchard, J. K., Stephens, M., and Donnelly, P. (2000). Inference of population structure using multilocus genotype data. Genetics 155, 945–959.
Puckett, B. J., Eggleston, D. B., Kerr, P. C., and Luettich, R. A. Jr. (2014). Larval dispersal and population connectivity among a network of marine reserves. Fish. Ocean. 23, 342–361. doi: 10.1111/fog.12067
Pujolar, J. M., Schiavina, M., Di Franco, A., Melià, P., Guidetti, P., Gatto, M., et al. (2013). Understanding the effectiveness of marine protected areas using genetic connectivity patterns and Lagrangian simulations. Div. Distr. 19, 1531–1542. doi: 10.1111/ddi.12114
Quesada, H., Beynon, C. M., and Skibinski, D. O. (1995). A mitochondrial DNA discontinuity in the mussel Mytilus galloprovincialis Lmk: pleistocene vicariance biogeography and secondary intergradation. Mol. Biol. Evol. 12, 521–524. doi: 10.1093/oxfordjournals.molbev.a040227
Raventós, N., and Macpherson, E. (2001). Planktonic larval duration and settlement marks on the otoliths of Mediterranean littoral fishes. Mar. Biol. 138, 1115–1120. doi: 10.1007/s002270000535
Rawson, P. D., Joyner, K. L., Meetze, K., and Hilbis, T. J. (1996). Evidence for intragenic recombination within a novel genetic marker that distinguishes mussels in the Mytilus edulis species complex. Heredity 77, 599–607. doi: 10.1038/hdy.1996.187
Ryman, N., and Palm, S. (2006). POWSIM: a computer program for assessing statistical power when testing for genetic differentiation. Mol. Ecol. Notes 6, 600–602. doi: 10.1111/j.1471-8286.2006.01378.x
Salinas-de-Leòn, P., Jones, T., and Bell, J. J. (2012). Successful determination of larval dispersal distances and subsequent settlement for long-lived pelagic larvae. PLoS One 7:e32788. doi: 10.1371/journal.pone.0032788
Sanjuan, A., Comesaña, A. S., and De Carlos, A. (1996). Macrogeographic differentiation by mtDNA restriction site analysis in the S.W. European Mytilus galloprovincialis Lmk. J. Exp. Mar. Biol. Ecol. 198, 89–100. doi: 10.1016/0022-0981(95)00209-X
Sanjuan, A., Zapata, C., and Alvarez, G. (1997). Genetic differentiation in Mytilus galloprovincialis Lmk. throughout the World. Ophelia 47, 13–31. doi: 10.1080/00785326.1997.10433387
Schiavina, M., Marino, I. A. M., Zane, L., and Meliá, P. (2014). Matching oceanography and genetics at the basin scale. Seascape connectivity of the Mediterranean shore crab in the Adriatic Sea. Mol. Ecol. 23, 5496–5507. doi: 10.1111/mec.12956
Serra, I. A., Innocenti, A., Di Maida, G., Calvo, S., Migliaccio, M., and Zambianchi, E. (2010). Genetic structure in the Mediterranean seagrass Posidonia oceanica: disentangling past vicariance events from contemporary patterns of gene flow. Mol. Ecol. 19, 557–568. doi: 10.1111/j.1365-294X.2009.04462.x
Silva, A. C. F., Brazão, S., Hawkins, S. J., Thompson, R. C., and Boaventura, D. (2009). Abundance, population structure and claw morphology of the semi-terrestrial crab Pachygrapsus marmoratus (Fabricius, 1787) on shores of differing wave exposure. Mar. Biol. 156, 2591–2599. doi: 10.1007/s00227-009-1283-1
Skibinski, D. O. F., Gallagher, C., and Beynon, C. M. (1994). Sex-limited mitochondrial DNA transmission in the marine mussel Mytilus edulis. Genetics 138, 801–809.
Śmietanka, B., Zbawicka, M., Wołowicz, M., and Wenne, R. (2004). Mitochondrial DNA lineages in the European populations of mussels Mytilus. Mar. Biol. 146, 79–92. doi: 10.1007/s00227-004-1418-3
Thorrold, S. R., Jones, G. P., Hellberg, M. E., Burton, R. S., Swearer, S. E., Neigel, J. E., et al. (2002). Quantifying larval retention and connectivity in marine populations with artificial and natural markers. Bull. Mar. Sci. 70, 291–308.
Wabnitz, C. C. C., Andrefouet, S., and Muller-Karger, F. E. (2010). Measuring progress toward global marine conservation targets. Front. Ecol. Environ. 8:124–129. doi: 10.1890/080109
Wang, S., Meyer, E., McKay, J. K., and Matz, M. V. (2012). 2b-RAD: a simple and flexible method for genome-wide genotyping. Nat. Meth. 9, 808–810. doi: 10.1038/nmeth.2023
Ward, R. D., Woodwark, M., and Skibinski, D. O. F. (1994). A comparison of genetic diversity levels in marine, fresh-water, and anadromous fishes. J. Fish Biol. 44, 213–232. doi: 10.1111/j.1095-8649.1994.tb01200.x
Weersing, K., and Toonen, R. J. (2009). Population genetics, larval dispersal, and connectivity in marine systems. Mar. Ecol. Prog. Ser. 393, 1–12. doi: 10.3354/meps08287
Wilson, J., Matejusova, I., McIntosh, R. E., Carboni, S., and Bekaert, M. (2018). New diagnostic SNP molecular markers for the Mytilus species complex. PLoS One 13:e0200654. doi: 10.1371/journal.pone.0200654
Wood, L. J., Fish, L., Laughren, J., and Pauly, D. (2008). Assessing progress towards global marine protection targets: shortfalls in information and action. Oryx 42, 340–351. doi: 10.1017/S003060530800046X
Zbawicka, M., Drywa, A., Śmietanka, B., and Wenne, R. (2012). Identification and validation of novel SNP markers in European populations of marine Mytilus mussels. Mar. Biol. 159, 1347–1362. doi: 10.1007/s00227-012-1915-8
Zbawicka, M., Trucco, A. I., and Wenne, R. (2018). Single nucleotide polymorphisms in native South American Atlantic coast populations of smooth shelled mussels: hybridization with invasive European Mytilus galloprovincialis. Genet. Sel. Evol. 50:5. doi: 10.1186/s12711-018-0376-z
Zitari-Chatti, R., Chatti, N., Fulgione, D., Caiazza, I., Aprea, G., and Elouaer, A. (2009). Mitochondrial DNA variation in the caramote prawn Penaeus (Melicertus) kerathurus across a transition zone in the Mediterranean Sea. Genetica 136, 439–447. doi: 10.1007/s10709-008-9344-9
Keywords: connectivity, mussel, population genomics, 2b-RAD, SNP markers, Mediterranean, Black Sea
Citation: Paterno M, Bat L, Souissi JB, Boscari E, Chassanite A, Congiu L, Guarnieri G, Kruschel C, Mačći V, Marino IAM, Micu D, Milchakova N, Panayotova M, Papetti C, Planes S, Strungaru S, Todorova VR, Voutsinas E and Zane L (2019) A Genome-Wide Approach to the Phylogeography of the Mussel Mytilus galloprovincialis in the Adriatic and the Black Seas. Front. Mar. Sci. 6:566. doi: 10.3389/fmars.2019.00566
Received: 30 April 2019; Accepted: 27 August 2019;
Published: 01 October 2019.
Edited by:
Cemal Turan, Iskenderun Technical University, TurkeyReviewed by:
Carlos Vergara-Chen, Technological University of Panama, PanamaRoman Wenne, Institute of Oceanology (PAN), Poland
Sabrina Lo Brutto, University of Palermo, Italy
Copyright © 2019 Paterno, Bat, Souissi, Boscari, Chassanite, Congiu, Guarnieri, Kruschel, Mačić, Marino, Micu, Milchakova, Panayotova, Papetti, Planes, Strungaru, Todorova, Voutsinas and Zane. This is an open-access article distributed under the terms of the Creative Commons Attribution License (CC BY). The use, distribution or reproduction in other forums is permitted, provided the original author(s) and the copyright owner(s) are credited and that the original publication in this journal is cited, in accordance with accepted academic practice. No use, distribution or reproduction is permitted which does not comply with these terms.
*Correspondence: Lorenzo Zane, bG9yZW56by56YW5lQHVuaXBkLml0