- 1National Institute of Water and Atmospheric Research, Hamilton, New Zealand
- 2Environmental and Marine Biology, Åbo Akademi University, Turku, Finland
- 3Department of Statistics, The University of Auckland, Auckland, New Zealand
- 4Institute of Marine Science, The University of Auckland, Auckland, New Zealand
Research on disturbance-recovery dynamics has facilitated understanding of species recovery rates, and the likely consequences of human disturbances that operate at large spatial and temporal scales. Benthic Protected Areas (BPA) are a management tool used to protect seafloor communities from disturbance. However, few studies have investigated the benefits of BPAs for benthic communities. A spatially explicit seafloor disturbance model (represented by a 128 × 128 cell grid) was used to simulate recovery dynamics over time in a conceptual benthic community [consisting of eight functional groups (FG)] and investigate interactions between disturbance magnitude and protected area size. The response to disturbance varied between FGs driven by differences in life-history; opportunistic early colonists increased in occupancy whereas other FGs declined, and at high levels of disturbance were extirped. Increasing disturbance accentuated the speed of declines or increases in FG proportional occupancy and increased the recovery times to pre-disturbed levels (if these were not extirped). The inclusion of protected areas lowered the required time for recovery in disturbed areas, with areas adjacent to protected areas recovering faster compared to those further away from the protected areas. Model results suggest that the size of BPAs affects the resilience of the ecosystem, but equally that the effectiveness of protection is heavily dependent on the magnitude of the disturbance in unprotected areas. At high levels of disturbance the permanent loss of the most sensitive FGs occurred in protected areas, resulting in a less functionally diverse and more homogenous conceptual seafloor community. Despite the relatively simple conceptual representation of a benthic community, this heuristic model provides a cost-effective way to explore implications of different management decisions on seafloor communities and highlight model uncertainties for further empirical validation as part of an ecosystem-based management approach.
Introduction
Natural disturbances to the seafloor are an important driver of benthic structure and function. Varying scales of disturbance, species interactions and differing abilities of species to recolonize create over time a mosaic of patches in varying degrees of recovery (Johnson, 1970; Dayton et al., 1974; Sousa, 1984; Thrush et al., 2005). Research on disturbance-recovery dynamics has facilitated understanding of species recovery rates, and the likely consequences of human disturbances (such as trawling) that operate at larger spatial and temporal scales. High rates of disturbance can result in homogenization and a reduction in species diversity and ecosystem function (Thrush and Dayton, 2002; Thrush et al., 2013). This homogenization can disrupt species interactions, simplify the 3-dimensional structure of the seafloor, or change sediment characteristics and biogeochemical processes, which may further inhibit recovery dynamics after disturbance (Cranfield et al., 2004; James et al., 2004; Kaiser et al., 2006; Zajac, 2008; Howarth et al., 2015; Stephenson et al., 2017a). Recovery dynamics are also dependent on dispersal and settling characteristics of species, the connectivity of the disturbed area to source populations for recolonization, the relative size of disturbed and undisturbed areas, and the temporal scale of disturbance (Thrush et al., 2013; Edgar et al., 2014; Marco et al., 2015; Mellin et al., 2016).
One management tool for protection of the seafloor from disturbance are Marine Protected Areas (MPAs), including both fully protected no-take marine reserves as well as partially protected areas through no trawl zones, also commonly called benthic protected areas (BPAs) or bottom trawl closures (BTC). Both fully and partially protected MPAs are common tools used to conserve marine species and habitats, and their associated ecosystem functions and services (Jennings, 2009). There is substantial evidence suggesting MPAs facilitate recovery of fished species, with increases in fish abundance associated with strong enforcement, full protection (i.e., no-take marine reserves), reserve age (>10 years), reserve size (>100 km2), and reserve isolation from human populations (Edgar et al., 2014; D’agata et al., 2016). However, fewer studies have compiled information to determine if predictions of MPA benefits for non-target species such as benthic organisms are realized, or if MPAs increase ecosystem resilience to disturbance (e.g., Mellin et al., 2016). BPAs, areas that solely protect against benthic disturbance, are increasingly used in the management of bottom trawl fisheries (Holland, 2003; Denderen et al., 2016), but there is little understanding of how the spatial and temporal scales of benthic protection affect recovery dynamics or resilience to high levels of fishing pressure (Denderen et al., 2016). For example, the minimum proportion of area protected required to maintain source populations of colonists is likely to vary between different macrofaunal species based on biological characteristics such as larval life history and dispersal strategy (Grantham et al., 2003). The spatial distribution of protected and disturbed patches is also likely to affect connectivity to patches that serve as colonist sources (Cowen and Sponaugle, 2009; Moffitt et al., 2011).
Maximizing the use of BPAs as a management tool thus requires understanding the complexity of responses of benthic habitats to different temporal and spatial scales of seafloor protection (Holland, 2003; Tillin et al., 2006), extrapolating beyond the multitude of studies that elucidate short temporal scale responses of benthic communities to one or few individual disturbances from fishery trawls, dredges or seafloor mining (Sciberras et al., 2013; Miller et al., 2018; Muntadas et al., 2018). Responses to, and recovery from, seafloor disturbances are often measured in the context of changes in easily sampled species (e.g., highly visible) or simple community measures (species richness, total abundance) (Lindholm et al., 2001; James et al., 2004; Howarth et al., 2015). However, many studies have demonstrated that species loss is not random and that species with particular biological traits have different disturbance-recovery dynamics (Pearson and Rosenberg, 1978; Thrush et al., 2006; Sciberras et al., 2018). Specific combinations of biological traits (functional groups) have also been used to derive indicators of, or sensitivity to, stressors, predict ecosystem functions and services and predict recovery dynamics of specific species (de Juan et al., 2007; Ellis et al., 2017; Hewitt et al., 2018; Lundquist et al., 2018). Functional group approaches facilitate evaluation of general predictions about seafloor communities without the need to model each species individually and enable comparisons over large scales (e.g., different regions), despite differences in species composition, to determine the impact of environmental drivers or disturbance (e.g., Bremner et al., 2003; Tillin et al., 2006; de Juan et al., 2007, 2009; Lundquist et al., 2018).
Empirical research on the effectiveness of protected area size is difficult; experiments can rarely be conducted at the appropriate temporal scales and meta-analysis of existing protected areas is limited by their low number and how they are distributed along both environmental and size gradients (Willis et al., 2003; Edgar et al., 2014). Heuristic modeling approaches provide an advantage to predict potential responses to protection across a range of management scenarios (e.g., Fulton et al., 2015), particularly when logistics make it impractical or expensive to empirically evaluate different protection scenarios. Models can be used to test management strategies across a range of different spatial and temporal scales, and to explore the direct and indirect impacts of marine protection or disturbance (Moffitt et al., 2011; Fulton et al., 2015).
Here, we use a spatially explicit seafloor disturbance model to estimate the effects of BPA extent on disturbance and recovery dynamics of a conceptual seafloor community using eight functional groups (FGs). Previous iterations of this model have explored responses of marine ecosystems to varying magnitudes and intensities of disturbance (Lundquist et al., 2010, 2013). This dynamic is expanded upon in this study by including model options to simulate BPAs to evaluate how seafloor protection affects resilience to disturbance. We hypothesize that the introduction of protected areas within the seafloor disturbance model would provide increased resilience to all functional groups even under high levels of disturbance, and that edge effects will result in faster rates of recovery of FGs in areas adjacent to protected areas compared to those further away from the protected areas. We tested these hypotheses by simulating disturbance at various intensities within the seafloor disturbance model (2.5 to 30% of the model extent each year over the course of 35 years) and in models with varying levels of benthic protection selected to emulate real world examples of protected areas (10, 25, 50, 75% of the model extent protected from disturbance over the 35 years of the disturbance regime).
Materials and Methods
Model Structure
The spatially explicit seafloor disturbance model presented here expands upon prior model iterations by Thrush et al. (2005) and Lundquist et al. (2010, 2013) to incorporate eight interacting functional groups with life history characteristics selected to simulate typical biological components of soft-sediment seafloor communities. The model consists of a 128 × 128 cell grid coded using MATLAB (R2017, v. 9.3.07), where cells consist of individual patches of habitat that are large enough (i.e., ∼100 m × 100 m) to sustain a seafloor soft-sediment community. Each cell within the model grid may be potentially populated by up to eight co-occurring FGs, representing the mosaic of species and functional groups found within soft-sediment marine ecosystems. The model is iterated as a series of discrete time steps (Δt = 3 months), with seasonality allowing the model to simulate differences in the availability of colonists based on FG life history. At the end of each timestep, the occupancy state and the age (i.e., the number of timesteps since cell colonization) of each FG is recorded within each cell. During each timestep, the model evaluates cell occupancy, colonization by juvenile life stages, growth, disturbance, and mortality of each FG within each cell based on a set of deterministic and stochastic rules based on life history traits and proximity to neighboring occupied cells (Figure 1, see following sections for details). For simplicity, we do not include adult movement or migration, though we recognize the potential for recolonization by adults from neighboring cells such as seastars or crabs. The model grid consisted of periodic boundary conditions, such that disturbance areas and dispersal by FGs can be “wrapped” around the grid to remove edge effects of cells near the boundary of a finite grid. The model was initialized with all FGs present at randomly chosen ages (from settled juvenile (age = 1) to a maximum age which varied for each FG) in each cell of the model seascape. The model was then run for 200-time steps (50 years) in order to reach a patch mosaic with consistent proportions of each FG occupying cells within the model seascape; proportions of each FG in this pre-disturbance state were validated using datasets from the Chatham Rise and the Challenger Plateau (Lundquist et al., 2013). All disturbance scenarios were subsequently initialized from the same pre-disturbance state to reduce the influence of random model variability.
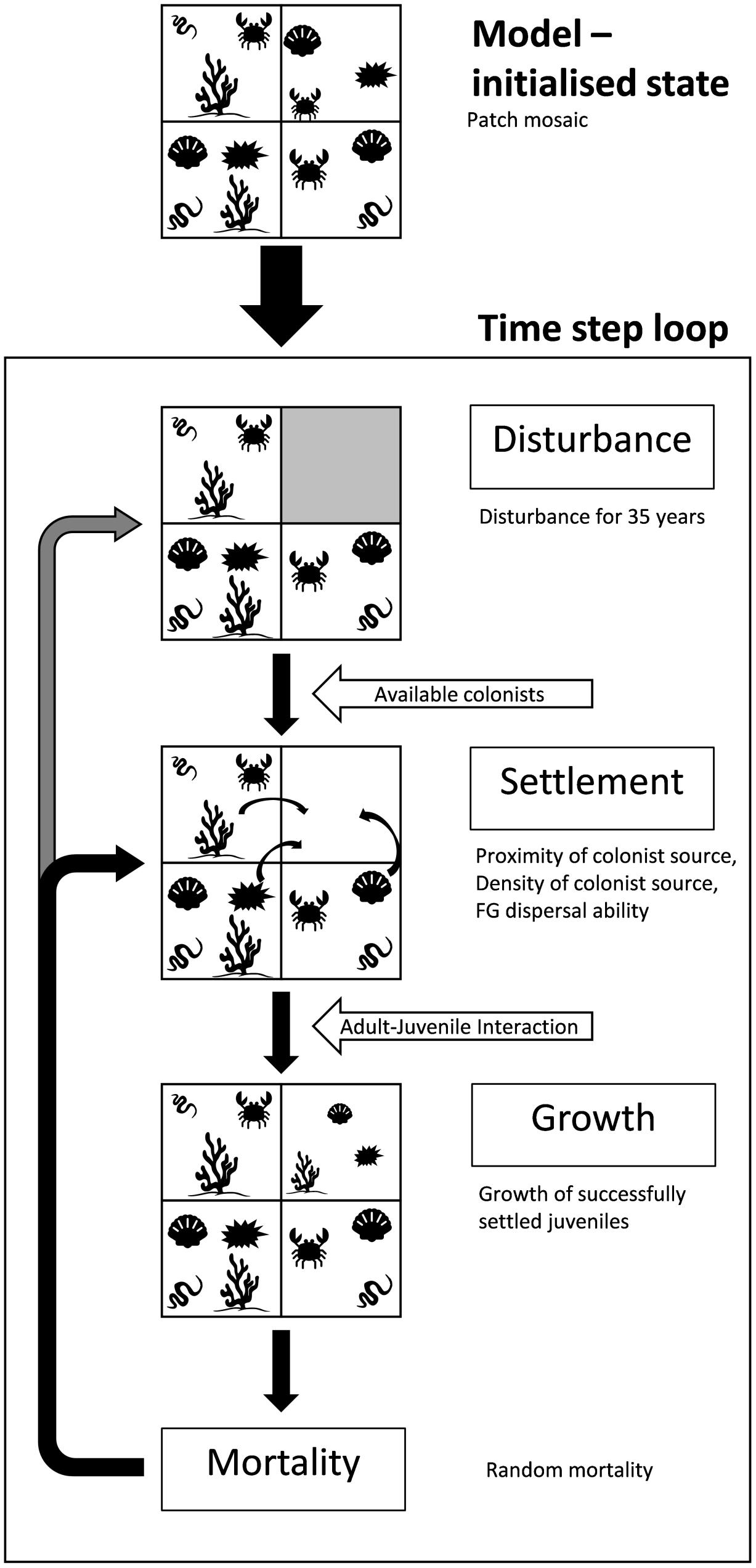
Figure 1. Flow chart of the spatially explicit seafloor disturbance model and schematic of conceptual seafloor patches undergoing disturbance and recovery (adapted from Lundquist et al., 2010; Lundquist et al., 2013).
Functional Groups
Eight FGs were defined iteratively during a series of workshops attended by a group of experts in soft-sediment benthic systems, and validated using datasets from the Chatham Rise and the Challenger Plateau, two important commercial fishing grounds in New Zealand (Lundquist et al., 2013, 2018). The FGs range from early to late colonists, surface dwellers to burrowers, substrate stabilizers to de-stabilizers, scavengers, predators and filter feeders; biological traits and life history determine how sensitive each FG will be to physical disturbance of the seafloor and how FGs interact within the model (Table 1). Life history parameters for each FG were determined from published studies on representative taxa within each group; each FG was assigned a specific age of maturity following a juvenile period where the FG does not reproduce, a dispersal length (which could represent larval, post-settlement or adult dispersal from a source cell), and a reproductive seasonality ranging from those with limited seasonal reproduction, to FGs with ongoing reproduction (Table 2). Once a cell is successfully settled by a FG, the age of the FG increases by one unit per timestep, as long as the cell is not impacted by either a disturbance event or natural mortality. Each FG has a specified maximum life span to allow the model to explore implications of longevity and age on species interactions. Natural mortality was simulated as a series of stochastic events applied separately to all FGs at each time step at a random mortality rate of 1% of the seascape occupied by each FG at that timestep.
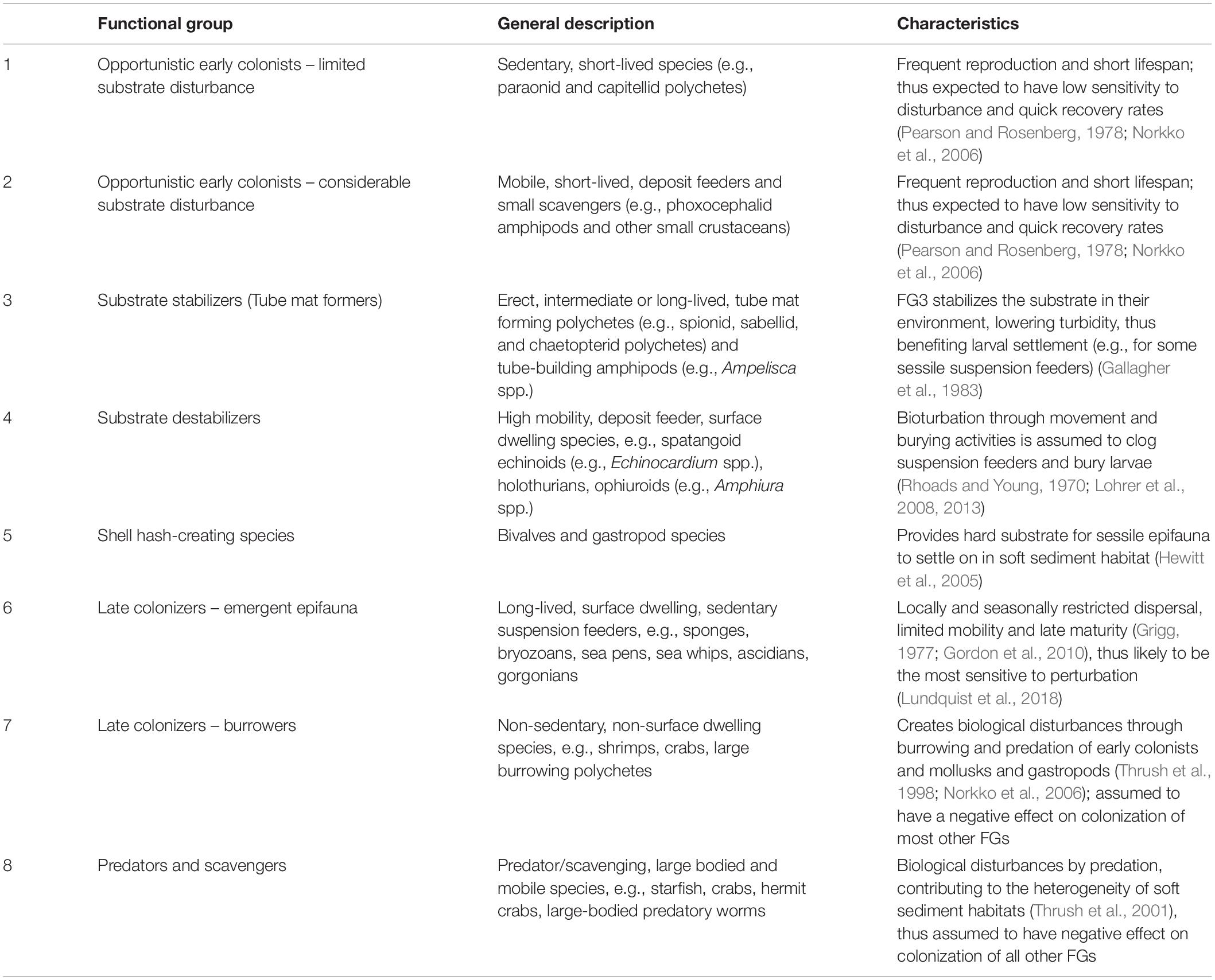
Table 1. General description of fauna classified under functional groups (FG) included in the model simulations.
Dispersal and Colonization
During each time step, dispersal and colonization rules are iterated to determine if any unoccupied cells are colonized by juveniles. The source neighborhood for colonists is FG-specific, based on pre-defined life history parameters to simulate local to long-distance dispersal of colonists (Table 2). Production of colonists occurs only from cells occupied by adults of each FG, and each FG only produces colonists during reproductively active seasons (Table 2). Connectivity between cells is incorporated within the model through approximated spatially explicit dispersal, where the potential distance traveled from adults by colonists is represented by a square-shaped neighborhood around the central dispersing cell, with the dimensions of the square being approximately twice the FG-specific dispersal distance (Table 2). For simplicity, dispersal was assumed to be uniform in all directions, with no influence of physical oceanic processes or colonist dispersal behavior on directionality or distance traveled. The number of potential colonists within the source neighborhood was modeled using a simple linear decay function, e.g., the closer an unoccupied cell is to a source adult, the higher the probability that colonists reach this cell. Reproduction occurred concurrently within all cells in the model seascape, and the cumulative number of colonists reaching a cell (ncol) was calculated from all source cells within the dispersal range of an unoccupied cell. Successful colonization was determined stochastically, where colonization occurred if a random number was less than the ratio of ncol divided by the maximum potential number of colonists that could reach a cell if all neighboring cells were occupied by adults of that FG.
Adult-Juvenile Interactions on Settlement Success
Adult-juvenile interactions were included in the model to further evaluate whether successful colonization (i.e., colonists reaching an unoccupied cell) resulted in successful settlement. Ecological interactions between adult and juveniles of all FGs were defined iteratively during a series of workshops attended by a group of experts in soft-sediment benthic systems (Table 3; Lundquist et al., 2013). Adult-juvenile interactions were evaluated simply as detrimental (−1), neutral (0) or beneficial (+1) scores for a potential juvenile settler within that cell based on the occupancy of adults of other FGs within the cell. For example, substrate stabilizers (FG 3) and the habitat providing groups (FG 5 and 6) were assumed to facilitate colonist success of most other FGs, whereas bioturbation by mobile opportunists (FG 2), substrate destabilizers (FG 4), and burrowers (FG 7) as well as the consumption of larvae by the predators (FG 8), were assumed to deter larval settlement of other functional groups (Table 3).
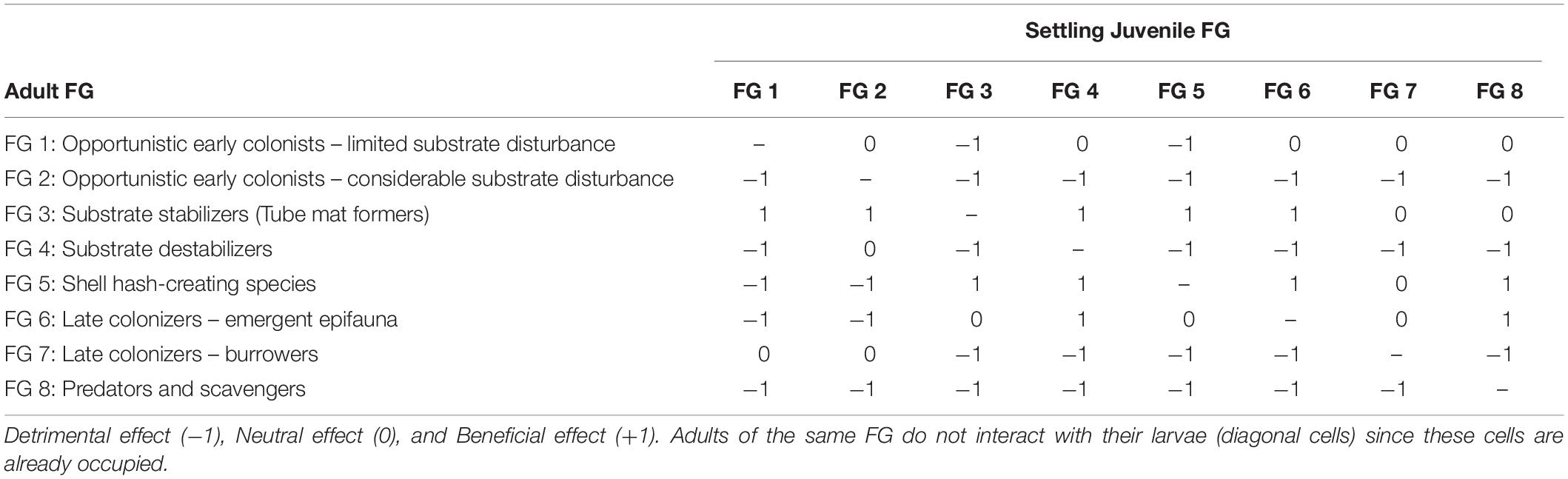
Table 3. Adult-Juvenile interaction matrix used to score functional group interaction in cells of the landscape.
To determine settlement success of each colonized cell at each time step, adult-juvenile interaction scores (Table 3) were summed based on the cumulative impact of the scores of all FG interactions in each cell based on whether a cell was occupied by adults of each FG. Settlement success was ultimately determined by comparing the cumulative adult-juvenile interaction score to an array of randomized score values that lie within the range of maximum-minimum score obtainable for the colonizing FG. Settlement occurred if interaction scores were less than or equal to the random array score value.
A further model complexity was included to represent the facilitation that shell hash of species such as bivalves and gastropods provides as the primary substratum for sessile epifaunal species to settle on soft sediment benthic habitats (Cummings et al., 1998; Commito et al., 2008). The fragmentation and removal of this hard substrate has been suggested as one possible reason for a lack of recovery in some structure-forming communities following disturbance events (e.g., Thrush et al., 2001; Cranfield et al., 2003). Here, the model was parameterized with the explicit assumption that the presence of shell hash was obligate for epifauna group (FG 6) to successfully settle. The presence and death of FG 5, consisting of shell-hash and carbonate-creating species such as bivalves and gastropods, was assumed to produce shell hash. Thus, successful settlement of FG 6 required either a cell to be currently occupied by FG 5, or if natural mortality to FG 5 had occurred, shell-hash was assumed to still be present in that cell. However, disturbance was assumed to completely remove all the shell debris in the corresponding disturbed area and thus leave these cells uninhabitable for epifauna until these were recolonized by FG 5 in subsequent time steps and new shell hash created.
Disturbance
Disturbance within the spatially explicit seafloor disturbance model was simulated as discrete, randomly located 4 × 4 cell square blocks within “unprotected” model areas (see section “Benthic Protected Areas” for description of protected area extents). Randomly distributed 4 × 4 cell disturbances could overlap in order to represent the often-overlapping nature of many seafloor disturbances (e.g., mobile bottom fishing, sedimentation, etc.). Five disturbance rates (2.5, 5, 10, 20, and 30% of the impacted area) were simulated. Disturbances occurred over a 35 year period in the model and was assumed to remove all FG present in the disturbed cells, i.e., disturbance events resulted in 100% mortality. Following disturbance, unoccupied cells were subject to dispersal, colonization and settlement rules in the following timestep (Figure 1).
Benthic Protected Areas
Four “protection” scenarios were simulated (10, 25, 50, and 75% of the total model area; Figure 2) to investigate effects of varying extents of benthic protected areas on disturbance and recovery dynamics. No disturbances occurred within the protected area extent, and model outputs at each timestep included occupancy and age of cells in different locations within the model seascape to evaluate effects within the: (a) protected area extent, blue areas in Figure 2; (b) the edges of disturbed areas (first 10 cells inward from the disturbance boundary, orange areas in Figure 2); and (c) inner disturbed areas (red areas in Figure 2). For simplicity, protected areas were represented by squares, maximizing our ability to investigate the recovery dynamics in the edges of the disturbed areas. A total of 20 scenarios were run, representing combinations of four protection extents and five disturbance rates. To investigate whether protection conferred resilience to the FGs, separate seafloor disturbance models, with the same number of grid cells as the individual impacted areas of each scenario (as per Figure 2), were run over the same number of time steps but without protected areas resulting in a further 20 “no protection” scenarios.
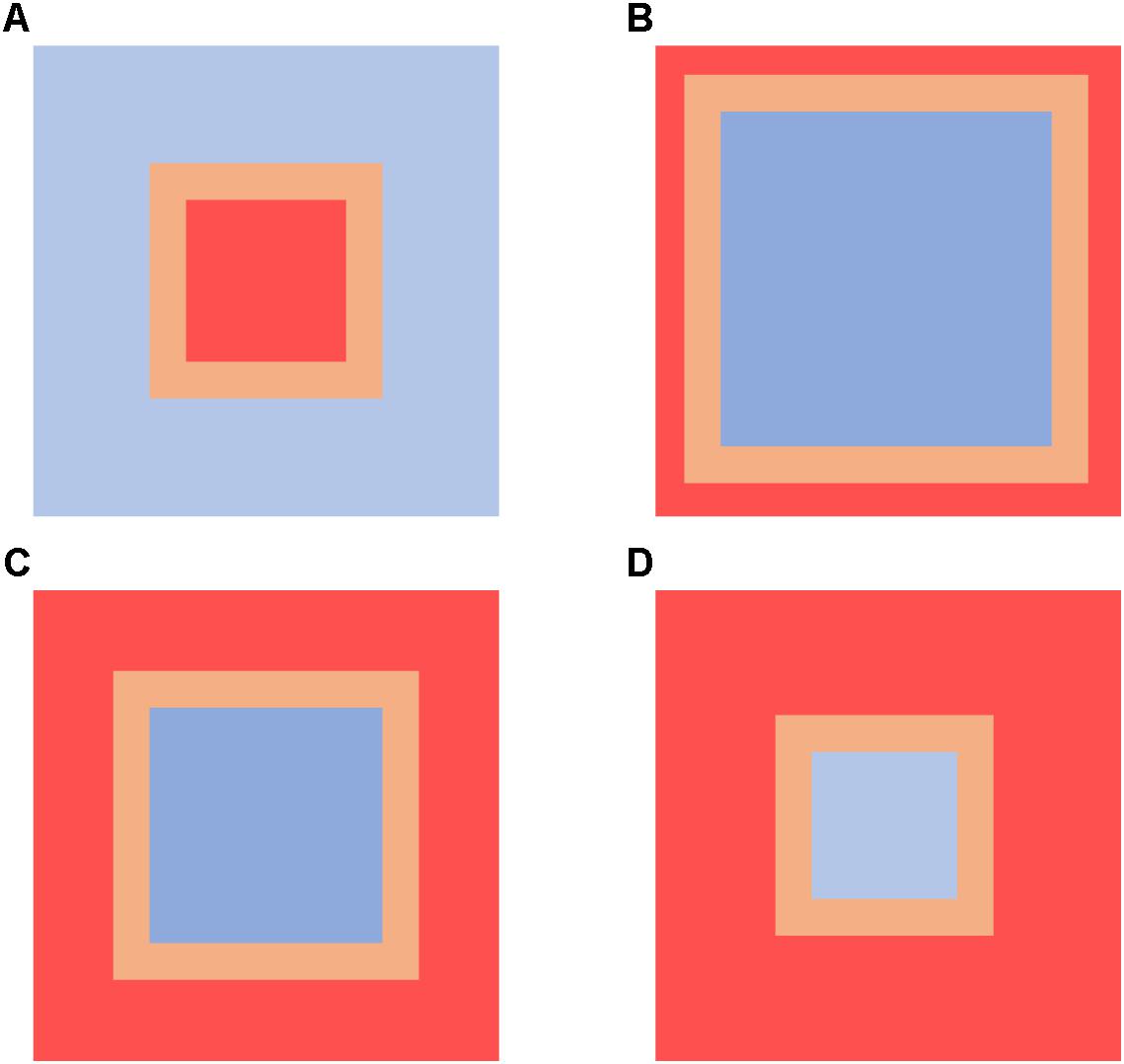
Figure 2. 128 × 128 cell grid seafloor disturbance model with varying extents of benthic protection (blue cells, protected areas; orange cells, impacted areas classified as edge; red cells, impacted areas). (A) 75% of model protected; (B) 50% of model protected; (C) 25% of model protected; (D) 10% of model protected.
Results
Changes in Magnitude of Disturbance on FG Presence
In “no protection” simulations, proportion of FG occupancy across the conceptual disturbance seafloor model when at equilibrium (i.e., year 0, in Figure 3) differed between FGs. FG 5 and 6 had the highest proportion occupancy (mean ± SD: 0.83 ± 0.02 and 0.82 ± 0.02, respectively), followed by FG8 (0.56 ± 0.01), FG3 (0.53 ± 0.06), FG7 (0.42 ± 0.06) and FG4 (0.38 ± 0.06); FG 1 and 2 had the lowest proportion occupancy (0.30 ± 0.02 and 0.21 ± 0.01, respectively) (Figure 3). The response to disturbance varied between functional groups driven by differences in colonization potential (dispersal, reproductive seasonality, age of maturity). All FGs declined in proportion occupancy during disturbance (shaded area, Figure 3), though FG 1 and 2 exhibited a rapid opportunistic response following an initial brief decline (Figure 3). FGs 4 and 6 were the most sensitive to disturbance, experiencing the largest decline in occupancy of the model seascape, and extirpation under the highest disturbance scenarios (red and orange lines, Figure 3). As disturbance increased from 2.5 to 30% of the model seascape disturbed each year, the declines in proportion of seascape occupied were accentuated for all FGs except FG 1 and FG 2. Recovery times to pre-disturbance levels of seascape occupancy increased for FGs 4, 6 and 7, for example, FG 6 took ca. 10 years to recover to pre-disturbance levels at 5% disturbance, but ca. 45 years to recover to pre-disturbance levels at 10% disturbance (Figure 3). FGs 3, 5 and 8 recovered quickly regardless of increasing disturbance, albeit, the proportion occupancy became more variable post-disturbance; increased variability lasted up to 90 years for FG 5 at 30% disturbance (Figure 3).
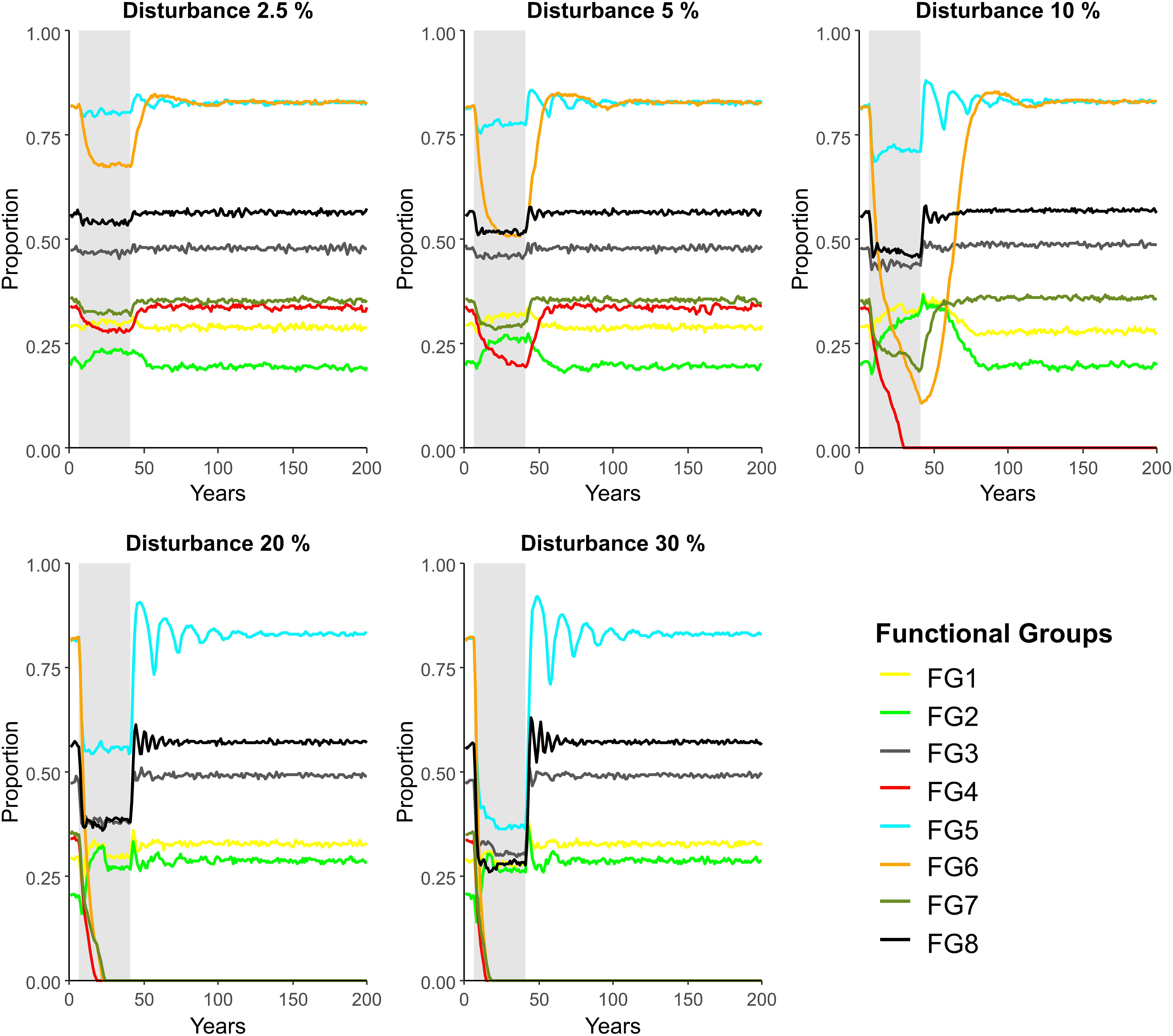
Figure 3. Proportion of cells occupied by adults of eight functional groups over time in a seafloor disturbance model parametrized with no protected areas and subject to increasing disturbance per year (2.5–30%) for 35 years (gray column). FG1, Opportunistic early colonists – limited substrate disturbance; FG2, Opportunistic early colonists – considerable substrate disturbance; FG3, Substrate stabilizers; FG 4, Substrate destabilizers; FG 5, Shell hash-creating species; FG 6, Late colonizers – emergent epifauna; FG 7, Late colonizers – burrowers; FG 8, Predators and scavengers.
Effect of Protection Within the Seabed Disturbance Model
In contrast to “no protection” scenarios, recovery to pre-disturbance levels occurred for all FGs in scenarios with high disturbance (20% per year) and high protection (75%) (Figure 4) since protected areas had a suitable number of adults allowing these to export juveniles which successfully recruited into the disturbed areas. For example, FGs 4, 6, and 7 recovered to pre-disturbance levels within 75 years (Figure 4) compared to extirpation in the “no protection” scenario (red line in Figure 4), showcasing the increased resilience conferred by this particular protection extent (albeit in scenarios with a high level of protection).
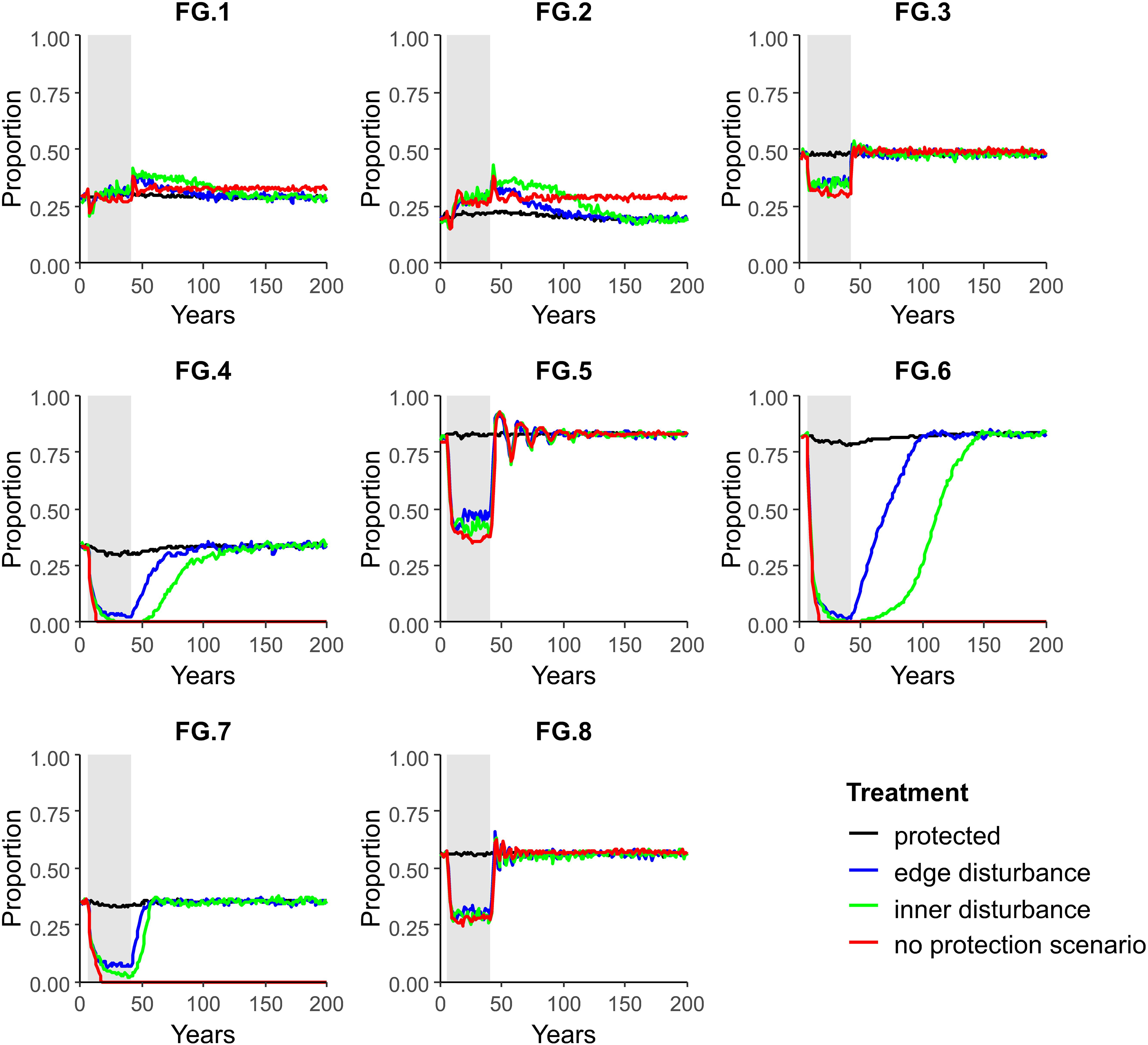
Figure 4. Proportion of seascape occupied by adults of each FG over time in four regions within the model seascape (“inner disturbed” and “edge disturbed” where disturbances were simulated; “protected” where no disturbances were simulated; and “no protection” which was a separate model, with the same dimensions as the impact area but included no protected areas). Scenario were parameterized with a 20% disturbance per year for 35 years within an impact area of 25% of the total model area (75% of the area in benthic protected area).
There were marked differences between regions within the model (inner, edge and protected, Figure 4); in inner and edge regions, FGs 3, 4, 5, 6, 7, and 8 declined in proportion occupancy of cells and FGs 1 and 2 increased in proportion occupancy of cells during disturbance (green and blue, respectively, Figure 4). For all FGs recovery time to pre-disturbance proportion of occupied cells was faster in edge areas compared to inner areas (Figure 4). This difference was largest for FGs 4, 6, and 7, e.g., FG 6 took ca. 25 years longer to recover to pre-disturbance proportion of occupied cells in inner areas compared to edge areas (Figure 4). No changes in proportion of occupied cells were observed for FGs 1, 2, 3, 5, and 8 within protected areas during disturbance; a very small decline in proportion of occupied cells were observed for FGs 4, 6 and 7 within protected areas (black line, Figure 4). Resettlement of FGs post-disturbance from the protected areas to inner areas is illustrated in the video files provided in the Supplementary Material and in a selection of snapshots provided in Figure 6A.
In contrast to scenarios with high protection (e.g., 75% as in Figure 4), recovery to pre-disturbance levels did not occur for all FGs in scenarios with high disturbance (20% per year) and low protection (25%) (Figure 5); FGs 4, 5 and 6 were rapidly extirped in impacted areas (“inner disturbance” and “edge disturbance”) as well as at a slower rate in “protected” areas (Figure 5). Changes in FG composition in both the impacted areas (“inner disturbance” and “edge disturbance”) and “protected” areas in the model through indirect interactions and community changes are illustrated in snapshots at various times taken from video files in the Supplementary Material (Figure 6B). The proportion of seascape occupied by adults of each FG over time in four regions within the model seascape for all other scenarios are presented in the Supplementary Material.
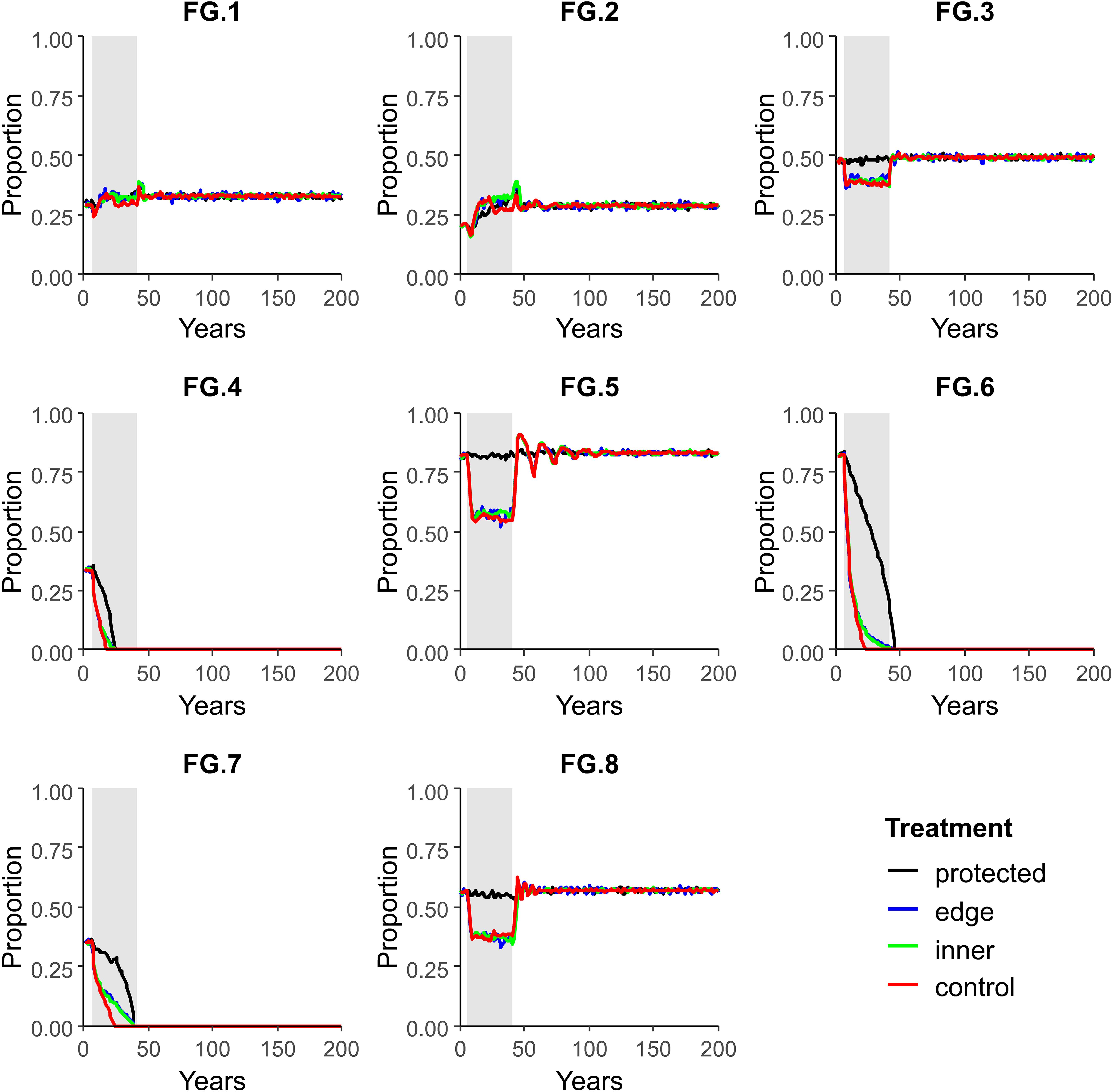
Figure 5. Proportion of seascape occupied by adults of each FG over time in four regions within the model seascape (“inner disturbed” and “edge disturbed” where disturbances were simulated; “protected” where no disturbances were simulated; and “no protection” which was a separate model, with the same dimensions as the impact area but included no protected areas). Scenario parameterized with a 20% disturbance per year for 35 years within an impact area of 75% of the total model area (25% of the area in benthic protected area).
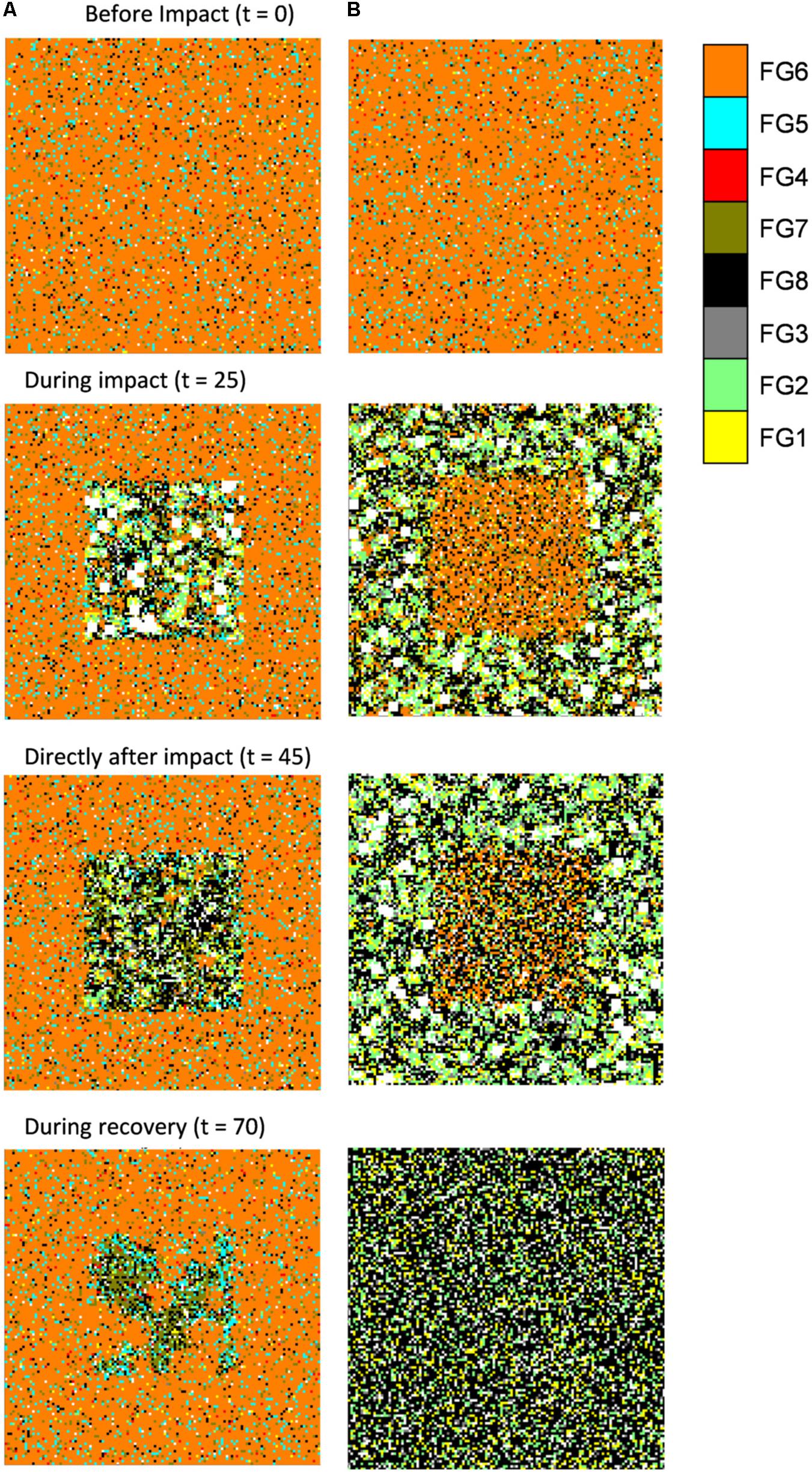
Figure 6. Visual output of the disturbance recovery model parameterized with (A) 20% disturbance per year for 35 years within an impact area equaling 25% of the total model area (75% of the area is protected); (B) 20% disturbance per year for 35 years within an impact area equaling 75% of the total model area (25% of the area is protected). Timesteps presented are: Before impact (t = 0); During impact (t = 25); Directly after impact (t = 45); During recovery (t = 70). FGs were assigned colors by a subjectively defined hierarchical order of importance (order shown in legend), e.g., if FG 6 was present then cell was colored orange, if FG 6 was not present but FG 5 was present this was colored blue, and so on. If no FGs were present (as is the case directly after disturbance) the cell was colored white.
Effect of Protection Extent and Increasing Disturbance on FG Occupancy
The rates of recovery and resilience both inside and outside the protected area were directly related to the interaction between proportion of the protected area in the model and disturbance magnitude; Figure 7 shows most combinations of the seafloor disturbance model scenarios for representative responses of behaviors illustrated by the FGs in the model (FGs 2, 4, 6, and 8 – columns in Figure 7). Protection extent of 75, 50, and 25% are shown in rows (Figure 7; scenarios with 10% protection extent were omitted because these were similar to scenarios with 25% protection).
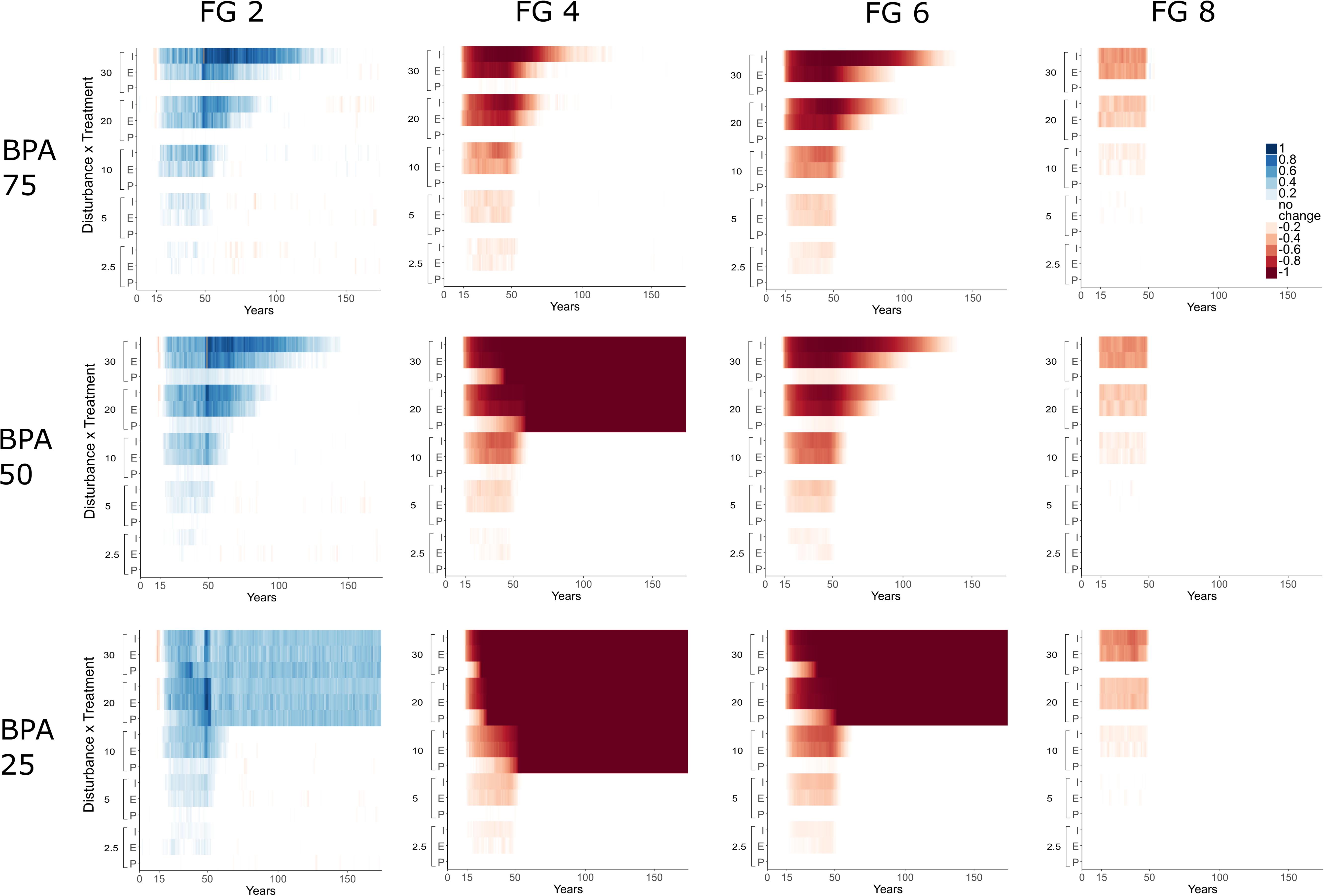
Figure 7. Proportion significant increase/decrease of adults compared to baseline pre-disturbance levels (mean ± 95% confidence interval) [shown as a color ranging from dark blue (1; proportion has doubled); no significant change (0; white); dark red (–1; FG has been extirped)] over time for four functional groups (FG 2, 4, 6, and 8 – columns) across models with varying BPA protection extent (25, 50, and 75% of the total model area – results for 90% were excluded because these did not differ substantially with those shown with 75% impact extent). Model seafloor areas [protected (P), Edge disturbance (E), and Inner disturbance (I)] are shown grouped by disturbance (2.5, 5, 10, 20 and 30% disturbance per year for 35 years – starting at year 15 and finishing at year 50) on the y-axis of each figure.
With large protection extents, recovery to pre-disturbance levels can occur even at high levels of disturbance, e.g., 30% disturbance for FG 6 with protection extent of 75%, top figure for FG 6 (Figure 7). However, as protection extent declines (moving down vertically in Figure 7), higher levels of disturbance resulted in changes in protected areas; FG 6 maintains resilience and has similar times to recovery for all disturbance levels in models with 75 and 50% protected areas, but for models with 25 and 10% protected areas and disturbances greater than 20% FG 6 is extirped including in protected areas (Figure 7).
FG 4 was the most sensitive and a tipping point in the proportion occurrence occurred even with relatively high levels of protection (e.g., 50% protected areas with 20% disturbance); equally, at lower levels of protection still (25 and 10% protected areas) it was more sensitive to disturbance magnitude, becoming extirped at 10% disturbance.
In contrast, FG 1 and FG 2 benefited from higher disturbance regimes and lower levels of protection. FG 2 (and FG 1 not shown here) increased in proportion occupancy of cells and remained at these high levels in scenarios where FG 4 and 6 were extirped (Figure 7). FG 8 declined only slightly during disturbances at differing levels of protection but recovered quickly; relative to FG 8’s baseline these declines were small. The increases in FG 2 and the lower relative declines of FG 8 during higher disturbance and lower protection resulted in changes in community structure across the model due to changes in the available space and changes in species interactions (Figure 6B).
Discussion
In this study, we simulate recovery dynamics across multiple functional groups simultaneously to provide insights into the interaction between disturbance magnitude and proportion of the protected area in the model. Many of the observed results were consistent with disturbance and recovery dynamics highlighted in empirical studies. However, we also demonstrate non-linear responses to disturbances, with clear disturbance thresholds (or tipping points) in benthic communities, and indirect effects of disturbance affecting benthic communities in protected areas.
The inclusion of protection not only resulted in protection of functional groups within protected areas, but also lowered the required time for recovery in disturbed areas, with the edges of the disturbed areas recovering faster than the inner areas (e.g., as observed in Forcada et al., 2009). This faster recovery was driven by spawning adults of each functional group in protected areas recruiting to the disturbed areas (e.g., Kough et al., 2017). Increasing the proportion of the protected area in the model had a marked impact on disturbance response and recovery within disturbed areas, however, as protection extent declined, disturbance also resulted in indirect effects on benthic communities in protected areas. Critically, by increasing the magnitude of disturbance and reducing protected area proportion in the model we were able to observe declines in occupancy of those most vulnerable functional groups within both the impacted and protected areas (Denderen et al., 2016).
Changes in Patch Mosaics: Winners and Losers
Three functional groups, substrate destabilizers (FG 4), late colonizers – emergent epifauna (FG 6), and late colonizers – burrowers (FG 7), showed the least tolerance to disturbance (as also observed in an empirical study using functional groups to investigate the benthic responses to fishing disturbance by Lundquist et al., 2018). The large and immediate declines in presence of these functional groups within the seafloor disturbance model at low to moderate rates of disturbance (e.g., >10% disturbance), imply sensitivity to disturbance as evidenced throughout the literature for these functional groups (Turner et al., 1999; Jennings et al., 2001; Thrush et al., 2001). While sensitive functional groups are often considered in the disturbance recovery context, our study also predicts changes to other functional groups. Under increasing disturbance intensities, the proportional occupancy of more tolerant functional groups increased (opportunistic early colonists and predators and scavengers, FGs 1, 2 and 8) resulting in homogenization of the seafloor communities. This is consistent with Norkko et al. (2006) who argued that the spatial scale and magnitude of disturbance is key to the development of opportunistic responses, possibly related to changes in the levels of resources made available and release from competitive interactions. Similar patterns have also been observed in studies of disturbance and recovery (e.g., see Bremner et al., 2003; Thrush et al., 2006; Hewitt et al., 2010; Lundquist et al., 2018).
These changes not only result in homogenization but also affect ecological functioning and ecosystem services. For example, the loss of substrate destabilizers and burrowers (FG 4) has significant biogeochemical implications (Lohrer et al., 2005); these groups play an important role mixing and displacing the sediment, destabilizing interstitial pore water and influencing the flux of inorganic nutrients from sediments to the bottom water column (Lohrer et al., 2005). Emergent epifauna, such as sponges and bryozoans (i.e., FG 6), enhance habitat complexity and potentially influence species interactions, such as through the provision of settlement substrates and refugia (Thrush et al., 1998). Non-sedentary, non-surface dwelling species, such as crabs and large burrowing polychetes (i.e., FG 7) are key in increasing the oxygen flow into the sediment and extending the total zone of denitrification and stimulating nutrient cycling by their action of burrowing (Beaumont et al., 2007; Norling et al., 2007). Collectively, these relatively long-lived, large-bodied groups (FGs 4, 6 and 7) are crucial in supporting ecosystem productivity and food production services (Jennings et al., 2001; Beaumont et al., 2007).
Non-linear Effects of Disturbance: Tipping Points
Disturbance can result in sudden and catastrophic non-linear ecological changes, which often manifest as surprises, sometimes referred to as tipping points or regime shifts. These events are particularly problematic because they are often in response to cumulative stressors and display little signs of early warning which makes response and avoidance extremely difficult (Scheffer et al., 2001; Hewitt and Thrush, 2010; Biggs et al., 2015). Here, we were able to simulate tipping points in functional group occupancy in response to differing magnitudes of disturbance and proportion of the protected area in the model. For the most sensitive species to disturbance (e.g., relatively long-lived, large-bodied groups – FGs 4 and 6), recovery time exponentially increased with increasing disturbance, and at critical levels resulted in permanent loss of those functional group from the system. However, the relationships between disturbance and functional group occupancy were not confined to disturbed areas, and tipping points within protected areas were also observed in our study (Denderen et al., 2016). Unique to our model, the indirect effects of disturbance in protected areas resulted in sudden changes at specific impact levels and protection extents, with the permanent loss of the most sensitive species in our conceptual seafloor disturbance model from both protected and non-protected areas (e.g., FGs 4, 6, and 7 at ≥20% disturbance per year and ≤25% protected area). The resulting less functionally diverse and more homogenous seafloor community persisted over time (driven by the loss of reproductive adult populations and associated reproduction). Similar observations have been made in a long-term monitoring study of benthic species in the Caribbean; Kough et al. (2017) found that the abundance of populations of queen conch, Lobatus gigas, progressively declined within a well-enforced, no-take, old, and large MPA due to low local retention and a lack of exogenous larval sources, associated with heavy fishing pressure outside of the MPA.
Critical Appraisal of the Seafloor Disturbance Model
The use of a seafloor disturbance model enabled the testing of a large number of management strategies, across large spatial and temporal scales, on the disturbance and recovery dynamics of a conceptual seafloor community based on ecological principles about the modeled organisms (Alexandridis et al., 2018). However, several assumptions were made to ensure a parsimonious model. The definition of the functional groups used, and parameterization of their life histories, were developed iteratively during a series of workshops and validated using datasets for the New Zealand region (Lundquist et al., 2013, 2018) but we acknowledge that the functional groups used here are not exhaustive and that values representing their life histories are simplified and may differ for other locations. In addition, we acknowledge that the shape and proportion of “protected area scenarios” in the conceptual model were simplified representations of management areas which may not represent all protected area shapes or extents.
The habitat type in the benthic seafloor disturbance model was assumed to be a circalittoral (below the photic zone) soft sediment bryozoan reef community (i.e., alga and other substrate types were not included). Although the soft sediment bryozoan reef community is a common habitat on the continental shelfs in some parts of the world, e.g., New Zealand (Cranfield et al., 2003), this does not encompass all possible functional groups or benthic subtidal habitat types. The effects of disturbance and subsequent recovery of communities, for example from the physical damage of the fishing gear on the seafloor, vary with habitat type (Kaiser et al., 2006; Lambert et al., 2014, 2017). In addition, recovery can be highly dependent on habitat type, for example, commercially important fish species such as the common dentex (Dentex dentex), striped red mullet (Mullus surmuletus), forkbeard (Phycis phycis), brown meager (Sciaena umbra) and black scorpionfish (Scorpaena porcus) were only found to increase in abundance and spillover to areas adjacent to protected areas when seagrass (Posidonia oceanica) meadows were present in a study of three Mediterranean MPAs (Forcada et al., 2009). Further, species and functional groups have been shown to have differing sensitivities to a variety of disturbances (Lambert et al., 2017; Lundquist et al., 2018). Here, the type of disturbance was not specified, and it was assumed that in the locations subject to disturbance the functional groups were removed. A spatial structure of the disturbance was not accounted for in the model. That is, disturbances were randomly distributed in the impact area; however, disturbances are often highly localized in space and time [e.g., fishing activity (Witt and Godley, 2007; Stephenson et al., 2017b)]. The incorporation of the different disturbance types, spatial structures of disturbance and differing functional group sensitivities would be of interest in future iterations of the seafloor disturbance model.
The functional group approach used here provided a practical way of reducing the number of interacting organisms (underpinned by well-known ecological principles derived from empirical studies) ensuring a parsimonious model. Closely related models have been shown to accurately reflect trends in benthic community over time (e.g., Lundquist et al. (2010), Alexandridis et al. (2018). Further, the use of functional groups has the added benefit that they allow the disturbance and recovery dynamics to be generalized to other geographical locations (Lundquist et al., 2018) and possibly other habitat types with similar recovery times [e.g., other biogenic habitats (Lundquist et al., 2010)]. Future model iterations have the scope to incorporate a greater number of functional groups or habitat types as well as incorporate within cell functional richness as a further measure of functional resilience.
Conclusion
The seafloor disturbance model presented here provides a heuristic tool to develop hypotheses of how a conceptual soft sediment community responds to disturbance. Results from our disturbance scenarios provide insight into possible benthic community changes and highlight important risks and threats to benthic ecosystems, and how management tools can mitigate against these threats. For example, the model suggests that the size of marine protected areas affects the resilience of the ecosystem, but equally that protection effectiveness is heavily dependent on the magnitude of the disturbance in unprotected areas. Despite the relatively simple representation of the conceptual benthic community this model provides a cost effective first step for environmental managers to explore potential effects of management decisions on seafloor communities as part of an ecosystem-based management approach (Fulton et al., 2015).
Data Availability Statement
The datasets generated for this study are available on request to the corresponding author.
Author Contributions
FS, MM-Y, and CL conceived the ideas. FS, MM-Y, LM, and CL analyzed the data. FS, RB, JH, and CL led the writing. All authors contributed to the writing.
Funding
This work was funded by the NIWA Coasts and Oceans Programme (Project: COME1901) and aligned funding from the New Zealand Sustainable Seas National Science Challenge (Project: SUSS16203 and UOA17202).
Conflict of Interest
The authors declare that the research was conducted in the absence of any commercial or financial relationships that could be construed as a potential conflict of interest.
Acknowledgments
While a number of people have participated in the development of this model over the years, we particularly acknowledge Simon Thrush for the initial ideas and funding and for his participation in expert workshops. We also thank Alex Vincent for advice on coding of the seafloor disturbance model.
Supplementary Material
The Supplementary Material for this article can be found online at: https://www.frontiersin.org/articles/10.3389/fmars.2019.00607/full#supplementary-material
References
Alexandridis, N., Bacher, C., Desroy, N., and Jean, F. (2018). Individual-based simulation of the spatial and temporal dynamics of macroinvertebrate functional groups provides insights into benthic community assembly mechanisms. PeerJ 6:e5038. doi: 10.7717/peerj.5038
Beaumont, N. J., Austen, M. C., Atkins, J. P., Burdon, D., Degraer, S., Dentinho, T. P., et al. (2007). Identification, definition and quantification of goods and services provided by marine biodiversity: implications for the ecosystem approach. Mar. Pollut. Bull. 54, 253–265. doi: 10.1016/j.marpolbul.2006.12.003
Biggs, R. O., Peterson, G. D., and Rocha, J. C. C. (2015). The regime shifts database: a framework for analyzing regime shifts in social-ecological systems. bioRxiv, [preprint]. doi: 10.1101/018473
Bremner, J., Rogers, S. I., and Frid, C. L. J. (2003). Assessing functional diversity in marine benthic ecosystems: a comparison of approaches. Mar. Ecol. Prog. Ser. 254, 11–25. doi: 10.3354/meps254011
Commito, J. A., Como, S., Grupe, B. M., and Dow, W. E. (2008). Species diversity in the soft-bottom intertidal zone: biogenic structure, sediment, and macrofauna across mussel bed spatial scales. J. Exp. Mar. Biol. Ecol. 366, 70–81. doi: 10.1016/j.jembe.2008.07.010
Cowen, R. K., and Sponaugle, S. (2009). Larval dispersal and marine population connectivity. Annu. Rev. Mar. Sci. 1, 443–466. doi: 10.1146/annurev.marine.010908.163757
Cranfield, H., Manighetti, B., Michael, K., and Hill, A. (2003). Effects of oyster dredging on the distribution of bryozoan biogenic reefs and associated sediments in Foveaux Strait, southern New Zealand. Cont. Shelf Res. 23, 1337–1357. doi: 10.1016/s0278-4343(03)00122-5
Cranfield, H. J., Rowden, A. A., Smith, D. J., Gordon, D. P., and Michael, K. P. (2004). Macrofaunal assemblages of benthic habitat of different complexity and the proposition of a model of biogenic reef habitat regeneration in Foveaux Strait. New Zealand. J. Sea Res. 52, 109–125. doi: 10.1016/j.seares.2003.12.003
Cummings, V., Thrush, S., Hewitt, J., and Turner, S. (1998). The influence of the pinnid bivalve Atrina zelandica (Gray) on benthic macroinvertebrate communities in soft-sediment habitats. J. Exp. Mar. Biol. Ecol. 228, 227–240. doi: 10.1016/s0022-0981(98)00028-8
D’agata, S., Mouillot, D., Wantiez, L., Friedlander, A. M., Kulbicki, M., and Vigliola, L. (2016). Marine reserves lag behind wilderness in the conservation of key functional roles. Nat. Commun. 7:12000. doi: 10.1038/ncomms12000
Dayton, P. K., Robilliard, G. A., Paine, R. T., and Dayton, L. B. (1974). Biological accommodation in the benthic community at McMurdo Sound. Antarctica. Ecol. Monogr. 44, 105–128. doi: 10.2307/1942321
de Juan, S., Demestre, M., and Thrush, S. F. (2009). Defining ecological indicators of trawling disturbance when everywhere that can be fished is fished: a Mediterranean case study. Mar. Policy 33, 472–478. doi: 10.1016/j.marpol.2008.11.005
de Juan, S., Thrush, S. F., and Demestre, M. (2007). Functional changes as indicators of trawling disturbance on a benthic community located in a fishing ground (NW Mediterranean Sea). Mar. Ecol. Prog. Ser. 334, 117–129. doi: 10.3354/meps334117
Denderen, P. D., Rijnsdorp, A. D., and Kooten, T. (2016). Using marine reserves to manage impact of bottom trawl fisheries requires consideration of benthic food-web interactions. Ecol. Appl. 26, 2302–2310. doi: 10.1002/eap.1360
Edgar, G. J., Stuart-Smith, R. D., Willis, T. J., Kininmonth, S., Baker, S. C., Banks, S., et al. (2014). Global conservation outcomes depend on marine protected areas with five key features. Nature 506:216. doi: 10.1038/nature13022
Ellis, J. I., Clark, M. R., Rouse, H. L., and Lamarche, G. (2017). Environmental management frameworks for offshore mining: the New Zealand approach. Mar. Policy 84, 178–192. doi: 10.1016/j.marpol.2017.07.004
Forcada, A., Valle, C., Bonhomme, P., Criquet, G., Cadiou, G., Lenfant, P., et al. (2009). Effects of habitat on spillover from marine protected areas to artisanal fisheries. Mar. Ecol. Prog. Ser. 379, 197–211. doi: 10.3354/meps07892
Fulton, E. A., Bax, N. J., Bustamante, R. H., Dambacher, J. M., Dichmont, C., Dunstan, P. K., et al. (2015). Modelling marine protected areas: insights and hurdles. Philos. Trans. R. Soc. B 370:17. doi: 10.1098/rstb.2014.0278
Gallagher, E. D., Jumars, P. A., and Trueblood, D. D. (1983). Facilitation of soft-bottom benthic succession by tube builders. Ecology 64, 1200–1216. doi: 10.2307/1937829
Gordon, D. P., Beaumont, J., Macdiarmid, A., Robertson, D. A., and Ahyong, S. T. (2010). Marine biodiversity of Aotearoa New Zealand. PLoS One 5:e10905. doi: 10.1371/journal.pone.0010905
Grantham, B. A., Eckert, G. L., and Shanks, A. L. (2003). Dispersal potential of marine invertebrates in diverse habitats. Ecol. Appl. 13, 108–116. doi: 10.1890/1051-0761(2003)013%5B0108:dpomii%5D2.0.co;2
Grigg, R. W. (1977). Population dynamics of two gorgonian corals. Ecology 58, 278–290. doi: 10.2307/1935603
Hewitt, J., Thrush, S., Lohrer, A., and Townsend, M. (2010). A latent threat to biodiversity: consequences of small-scale heterogeneity loss. Biodivers. Conserv. 19, 1315–1323. doi: 10.1007/s10531-009-9763-7
Hewitt, J. E., Lundquist, C. J., and Ellis, J. (2018). Assessing sensitivities of marine areas to stressors based on biological traits. Conserv. Biol. 33, 142–151. doi: 10.1111/cobi.13181
Hewitt, J. E., and Thrush, S. F. (2010). Empirical evidence of an approaching alternate state produced by intrinsic community dynamics, climatic variability and management actions. Mar. Ecol. Prog. Ser. 413, 267–276. doi: 10.3354/meps08626
Hewitt, J. E., Thrush, S. F., Halliday, J., and Duffy, C. (2005). The importance of small-scale habitat structure for maintaining beta diversity. Ecology 86, 1619–1626. doi: 10.1890/04-1099
Holland, D. S. (2003). Integrating spatial management measures into traditional fishery management systems: the case of the Georges Bank multispecies groundfish fishery. ICES J. Mar. Sci. 60, 915–929. doi: 10.1016/s1054-3139(03)00097-3
Howarth, L. M., Roberts, C. M., Hawkins, J. P., Steadman, D. J., and Beukers-Stewart, B. D. (2015). Effects of ecosystem protection on scallop populations within a community-led temperate marine reserve. Mar. Biol. 162, 823–840. doi: 10.1007/s00227-015-2627-7
James, L., Peter, A., and Page, V. (2004). Role of a large marine protected area for conserving landscape attributes of sand habitats on Georges Bank (NW Atlantic). Mar. Ecol. Prog. Ser. 269, 61–68. doi: 10.3354/meps269061
Jennings, S. (2009). The role of marine protected areas in environmental management. ICES J. Mar. Sci. 66, 16–21. doi: 10.1093/icesjms/fsn163
Jennings, S., Dinmore, T. A., Duplisea, D. E., Warr, K. J., and Lancaster, J. E. (2001). Trawling disturbance can modify benthic production processes. J. Anim. Ecol. 70, 459–475. doi: 10.1046/j.1365-2656.2001.00504.x
Johnson, R. G. (1970). Variations in diversity within benthic marine communities. Am. Nat. 104, 285–300. doi: 10.1086/282662
Kaiser, M. J., Clarke, K. R., Hinz, H., Austen, M. C. V., Somerfield, P. J., and Karakassis, I. (2006). Global analysis of response and recovery of benthic biota to fishing. Mar. Ecol. Prog. Ser. 311, 1–14. doi: 10.3354/meps311001
Kough, A. S., Cronin, H., Skubel, R., Belak, C. A., and Stoner, A. W. (2017). Efficacy of an established marine protected area at sustaining a queen conch Lobatus gigas population during three decades of monitoring. Mar. Ecol. Prog. Ser. 573, 177–189. doi: 10.3354/meps12163
Lambert, G., Murray, L., Hiddink, J., Hinz, H., Lincoln, H., Hold, N., et al. (2017). Defining thresholds of sustainable impact on benthic communities in relation to fishing disturbance. Sci. Rep. 7:5440. doi: 10.1038/s41598-017-04715-4
Lambert, G. I., Jennings, S., Kaiser, M. J., Davies, T. W., and Hiddink, J. G. (2014). Quantifying recovery rates and resilience of seabed habitats impacted by bottom fishing. J. Appl. Ecol. 51, 1326–1336. doi: 10.1111/1365-2664.12277
Lindholm, J. B., Auster, P. J., Ruth, M., and Kaufman, L. (2001). Modeling the effects of fishing and implications for the design of marine protected areas: juvenile fish responses to variations in seafloor habitat. Conserv. Biol. 15, 424–437. doi: 10.1046/j.1523-1739.2001.015002424.x
Lohrer, A. M., Chiaroni, L. D., Hewitt, J. E., and Thrush, S. F. (2008). Biogenic disturbance determines invasion success in a subtidal soft-sediment system. Ecology 89, 1299–1307. doi: 10.1890/07-0421.1
Lohrer, A. M., Rodil, I. F., Townsend, M., Chiaroni, L. D., Hewitt, J. E., and Thrush, S. F. (2013). Biogenic habitat transitions influence facilitation in a marine soft-sediment ecosystem. Ecology 94, 136–145. doi: 10.1890/11-1779.1
Lohrer, A. M., Thrush, S. F., Hunt, L., Hancock, N., and Lundquist, C. (2005). Rapid reworking of subtidal sediments by burrowing spatangoid urchins. J. Exp. Mar. Biol. Ecol. 321, 155–169. doi: 10.1016/j.jembe.2005.02.002
Lundquist, C. J., Bowden, D., Cartner, K., Stephenson, F., Tuck, I., and Hewitt, J. E. (2018). Assessing benthic responses to fishing disturbance over broad spatial scales that incorporate high environmental variation. Front. Mar. Sci. 5:405. doi: 10.3389/fmars.2018.00405
Lundquist, C. J., Pritchard, M., Thrush, S., Hewitt, J. E., Greenfield, B. L., Halliday, J., et al. (2013). Bottom disturbance and seafloor community dynamics: Development of a model of disturbance and recovery dynamics for marine benthic ecosystems. New Zealand Aquatic Environment and Biodiversity Report No. 118. New Zealand: Ministry for Primary Industries
Lundquist, C. J., Thrush, S. F., Coco, G., and Hewitt, J. E. (2010). Interactions between disturbance and dispersal reduce persistence thresholds in a benthic community. Mar. Ecol. Prog. Ser. 413, 217–228. doi: 10.3354/meps08578
Marco, A., David, M., Samuel, S., Wilfried, T., and Stéphanie, M. (2015). Additive effects of climate change on connectivity between marine protected areas and larval supply to fished areas. Divers. Distrib. 21, 139–150. doi: 10.1111/ddi.12250
Mellin, C., Aaron MacNeil, M., Cheal, A. J., Emslie, M. J., and Julian Caley, M. (2016). Marine protected areas increase resilience among coral reef communities. Ecol. Lett. 19, 629–637. doi: 10.1111/ele.12598
Miller, K. A., Thompson, K. F., Johnston, P., and Santillo, D. (2018). An overview of seabed mining including the current state of development, environmental impacts, and knowledge gaps. Front. Mar. Sci. 4:418. doi: 10.3389/fmars.2017.00418
Moffitt, E. A., Wilson White, J., and Botsford, L. W. (2011). The utility and limitations of size and spacing guidelines for designing marine protected area (MPA) networks. Biol. Conserv. 144, 306–318. doi: 10.1016/j.biocon.2010.09.008
Muntadas, A., Lample, M., Demestre, M., Ballé-Béganton, J., De Juan, S., Maynou, F., et al. (2018). A knowledge platform to inform on the effects of trawling on benthic communities. Estuarine Coastal Shelf Sci. 201, 223–233. doi: 10.1016/j.ecss.2017.01.001
Norkko, A., Rosenberg, R., Thrush, S. F., and Whitlatch, R. B. (2006). Scale- and intensity-dependent disturbance determines the magnitude of opportunistic response. J. Exp. Mar. Biol. Ecol. 330, 195–207. doi: 10.1016/j.jembe.2005.12.027
Norling, K., Rosenberg, R., Hulth, S., Gremare, A., and Bonsdorff, E. (2007). Importance of functional biodiversity and species-specific traits of benthic fauna for ecosystem functions in marine sediment. Mar Ecol. Prog. Ser. 332, 11–23. doi: 10.3354/meps332011
Pearson, T., and Rosenberg, R. (1978). Macrobenthic succession in relation to organic enrichment and pollution of the marine environment. Oceanogr. Mar. Biol. Annu. Rev. 16, 229–311.
Rhoads, D. C., and Young, D. K. (1970). The influence of deposit-feeding organisms on sediment stability and community trophic structure. J. Mar. Res. 28, 150–178.
Scheffer, M., Carpenter, S., Foley, J. A., Folke, C., and Walker, B. (2001). Catastrophic shifts in ecosystems. Nature 413, 591–596. doi: 10.1038/35098000
Sciberras, M., Hiddink, J. G., Jennings, S., Szostek, C. L., Hughes, K. M., Kneafsey, B., et al. (2018). Response of benthic fauna to experimental bottom fishing: a global meta-analysis. Fish Fish. 19, 698–715. doi: 10.1111/faf.12283
Sciberras, M., Hinz, H., Bennell, J. D., Jenkins, S. R., Hawkins, S. J., and Kaiser, M. J. (2013). Benthic community response to a scallop dredging closure within a dynamic seabed habitat. Mar. Ecol. Prog. Ser. 480, 83–98. doi: 10.3354/meps10198
Sousa, W. P. (1984). The role of disturbance in natural communities. Annu. Rev. Ecol. Syst. 15, 353–391. doi: 10.1146/annurev.es.15.110184.002033
Stephenson, F., Mill, A. C., Scott, C. L., Polunin, N. V., and Fitzsimmons, C. (2017a). Experimental potting impacts on common UK reef habitats in areas of high and low fishing pressure. ICES J. Mar. Sci. 74, 1648–1659. doi: 10.1093/icesjms/fsx013
Stephenson, F., Polunin, N. V., Mill, A. C., Scott, C., Lightfoot, P., and Fitzsimmons, C. (2017b). Spatial and temporal changes in pot-fishing effort and habitat use. ICES J. Mar. Sci. 74, 2201–2212. doi: 10.1093/icesjms/fsx051
Thrush, S. F., and Dayton, P. K. (2002). Disturbance to marine benthic habitats by trawling and dredging: implications for marine biodiversity. Annu. Rev. Ecol. Syst. 33, 449–473. doi: 10.1146/annurev.ecolsys.33.010802.150515
Thrush, S. F., Gray, J. S., Hewitt, J. E., and Ugland, K. I. (2006). Predicting the effects of habitat homogenization on marine biodiversity. Ecol. Appl. 16, 1636–1642. doi: 10.1890/1051-0761(2006)016%5B1636:pteohh%5D2.0.co;2
Thrush, S. F., Hewitt, J. E., Cummings, V. J., Dayton, P. K., Cryer, M., Turner, S. J., et al. (1998). Disturbance of the marine benthic habitat by commercial fishing: impacts at the scale of the fishery. Ecol. Appl. 8, 866–879. doi: 10.1890/1051-0761(1998)008%5B0866:dotmbh%5D2.0.co;2
Thrush, S. F., Hewitt, J. E., Funnell, G. A., Cummings, V. J., Ellis, J., Schultz, D., et al. (2001). Fishing disturbance and marine biodiversity: the role of habitat structure in simple soft-sediment systems. Mar. Ecol. Prog. Ser. 223, 277–286. doi: 10.3354/meps223277
Thrush, S. F., Hewitt, J. E., Lohrer, A. M., and Chiaroni, L. D. (2013). When small changes matter: the role of cross-scale interactions between habitat and ecological connectivity in recovery. Ecol. Appl. 23, 226–238. doi: 10.1890/12-0793.1
Thrush, S. F., Lundquist, C. J., and Hewitt, J. E. (2005). “Spatial and temporal scales of disturbance to the seafloor: a generalised framework for active habitat management,” in Benthic habitats and the effects of fishing. American Fisheries Society, Symposium Series, eds W. Barnes, and J. P. Thomas, (Bethesda, MD: American Fisheries Society).
Tillin, H. M., Hiddink, J. G., Jennings, S., and Kaiser, M. J. (2006). Chronic bottom trawling alters the functional composition of benthic invertebrate communities on a sea-basin scale. Mar. Ecol. Prog. Ser. 318, 31–45. doi: 10.3354/meps318031
Turner, S. J., Thrush, S. F., Hewitt, J. E., Cummings, V. J., and Funnell, G. (1999). Fishing impacts and the degradation or loss of habitat structure. Fish. Manag. Ecol. 6, 401–420. doi: 10.1046/j.1365-2400.1999.00167.x
Willis, T. J., Millar, R., Babcock, R., and Tolimieri, N. (2003). Burdens of evidence and the benefits of marine reserves: putting Descartes before des horse? Environ. Conserv. 30, 97–103. doi: 10.1017/s0376892903000092
Witt, M. J., and Godley, B. J. (2007). A step towards seascape scale conservation: using vessel monitoring systems (VMS) to map fishing activity. PLoS One 2:e1111. doi: 10.1371/journal.pone.0001111
Keywords: protected areas, conceptual seafloor community, soft-sediment, disturbance, recovery, heuristic model, spatial-temporal community dynamics
Citation: Stephenson F, Bulmer RH, Meredyth-Young M, Meysick L, Hewitt JE and Lundquist CJ (2019) Effects of Benthic Protection Extent on Recovery Dynamics of a Conceptual Seafloor Community. Front. Mar. Sci. 6:607. doi: 10.3389/fmars.2019.00607
Received: 13 June 2019; Accepted: 11 September 2019;
Published: 27 September 2019.
Edited by:
Alberto Basset, University of Salento, ItalyReviewed by:
Antonio Terlizzi, University of Trieste, ItalyMarina R. Cunha, University of Aveiro, Portugal
Copyright © 2019 Stephenson, Bulmer, Meredyth-Young, Meysick, Hewitt and Lundquist. This is an open-access article distributed under the terms of the Creative Commons Attribution License (CC BY). The use, distribution or reproduction in other forums is permitted, provided the original author(s) and the copyright owner(s) are credited and that the original publication in this journal is cited, in accordance with accepted academic practice. No use, distribution or reproduction is permitted which does not comply with these terms.
*Correspondence: Fabrice Stephenson, RmFicmljZS5TdGVwaGVuc29uQG5pd2EuY28ubno=