- Institute of Marine Research (IMR), Bergen, Norway
The effects of increasing fishing pressure in combination with temperature increases in the Nordic and Barents Seas have been evaluated using an end-to-end model for the area forced by a downscaled RCP 4.5 climate scenario. The scenarios that have been applied have used four different fractions of fisheries mortality at maximum sustainable yield (Fmsy); 0.6, 0.8, 1.0 and 1.1 × Fmsy. As it is highly likely that more ecosystem components will be harvested in the future, the four scenarios have been repeated with fishing on a larger number of ecosystem components, including harvesting of lower trophic levels (mesozooplankton and mesopelagic fish). The zooplankton biomass had an increasing trend, regardless of the increase in fishing pressure on their predators. However, when introducing harvest on the lower trophic levels, this increase was no longer evident. When harvesting more components, the negative response in biomass of pelagic and demersal fish to increasing harvest became more prominent, indicating an increasing vulnerability in the ecosystem structure to stressors. Although harvest on lower trophic level led to an immense increase in the total catch, it also resulted in a decrease in the total catches of pelagic and demersal fish, despite more species being harvested in these guilds.
Introduction
Facing climate change and a growing human population, the world’s ocean resources will be put under even higher pressures in the future than they already are. The increased demand for food from the oceans for human consumption has already led to declining stocks in several marine ecosystems (e.g., FAO, 2018a), and cumulative impacts of climate and increased fishing pressure may cause further declines (Halpern et al., 2015; Armstrong et al., 2019). There are however, strong spatial differences in how climate change affects ecosystems. Cheung et al. (2010) showed that high-latitude areas such as the Norwegian and Barents Seas are likely to experience an increase in total catch potential in the future, based mostly on calculations of future primary production, trophic level of the species and its geographic range. This is supported by observations from the Barents Sea over the last decades, where increasing temperatures have been beneficial for e.g., Northeast Arctic cod Gadus morhua (Kjesbu et al., 2014). However, changes in fisheries management strategies can be as or more important to the ecosystem as climate change (Groeneveld et al., 2018).
To meet the United Nations sustainability development goal (SDG) 2 (zero hunger), catches from marine ecosystems should likely increase. This has to occur along with SDG 14 (life below water), securing sustainable harvests of all marine resources. As seen in FAO (2018a), the commercial stocks being harvested above sustainable levels are currently at 33%, whereas only 7% are underfished. In the Norwegian and Barents Seas, most stocks are being harvested at or close to maximum sustainable yield (ICES, 2017, 2018a). Hence, a further increase of the fishing mortality would likely lead to declining stocks and decreasing catches.
Another possibility is to harvest more ecosystem components, including lower trophic levels such as zooplankton and mesopelagic fish. The production and biomass at this ecosystem level are considerably higher compared to pelagic fish and top-predators. However, by being the most important food source for juvenile and pelagic fish, they form the basis for nearly all life in the ocean. Therefore, harvest at this trophic level needs to be managed in a way that does not disrupt the balance in the system. In the Norwegian Sea, a trial fishery for the copepod Calanus finmarchicus has been active for the last decade or so (Grimaldo and Gjøsund, 2012). Recently, a commercial quota was set for this species but with area-based restrictions. However, the quota is low (165 000 tonnes) compared to the standing stock biomass (∼31 million tonnes), following a strict precautionary approach to the fishery (Broms et al., 2016).
Exploring the effect of changes in management strategies, including the number of ecosystem components being harvested, in combination with climate changes can be undertaken by applying end-to-end ecosystem models. End-to-end models include ‘everything’ from sunshine to fishing vessels and harvest control rules, and are built for studying ecosystem effects resulting from almost any kind of perturbation or disturbance (Plagányi and Food and Agriculture Organization of the United Nations [FAO], 2007). They also provide the only way of testing indirect and direct impact of increased harvest on multiple components in an ecosystem, without applying the change on the real system. Complex end-to-end models such as Atlantis (Fulton et al., 2011) give an overview of tradeoffs that need to be considered, especially when introducing harvest at lower trophic levels. However, their complexity and level of uncertainty makes them inappropriate for setting quotas (FAO, 2008; Link et al., 2010).
Future management regimes are very uncertain, and changes in harvest pressures or strategies can outweigh climate changes (Frank et al., 2016). The EU Climate change and European aquatic RESources (CERES) project approached the complex problem of investigating climate change effects using a scenario approach (CERES, 2016), and utilized the community-derived Shared Socio-economic Pathways (SSPs) from the Intergovernmental Panel on Climate Change (Riahi et al., 2017) which inspired a simplified version of four socio-political scenarios, including changing fishing pressure only. To examine the potential consequences of these simplified scenarios on the fisheries of the Barents and Norwegian Sea in a future climate, we utilized a complex end-to-end ecosystem model developed specifically for the Nordic and Barents Seas (Hansen et al., 2016). For the environment, we applied the results from a regional downscaled IPCC RCP4.5 scenario (Sandø et al., 2014; Skogen et al., 2018). Combined changes in climate, fishing pressure and the number of harvested components in the system are explored, and the uncertainties in this set of scenarios are discussed.
Methods and Models
Atlantis (Fulton et al., 2011) is a deterministic end-to-end ecosystem model consisting of multiple modules, including ecology, physics and fisheries. The Nordic and Barents seas Atlantis model represents the ecosystems of the respective areas based upon 53 species and functional groups (Hansen et al., 2019). These species and functional groups are biomass dominant, key species (e.g., predator–prey relationships), vulnerable and/or commercially important (Hansen et al., 2016). The model domain consists of 60 polygons that cover the Nordic and Barents seas, an area of close to 4 million km2 (Figure 1). Vertically, the model is relatively coarse, resolving the water column with seven depth levels and one sediment layer.
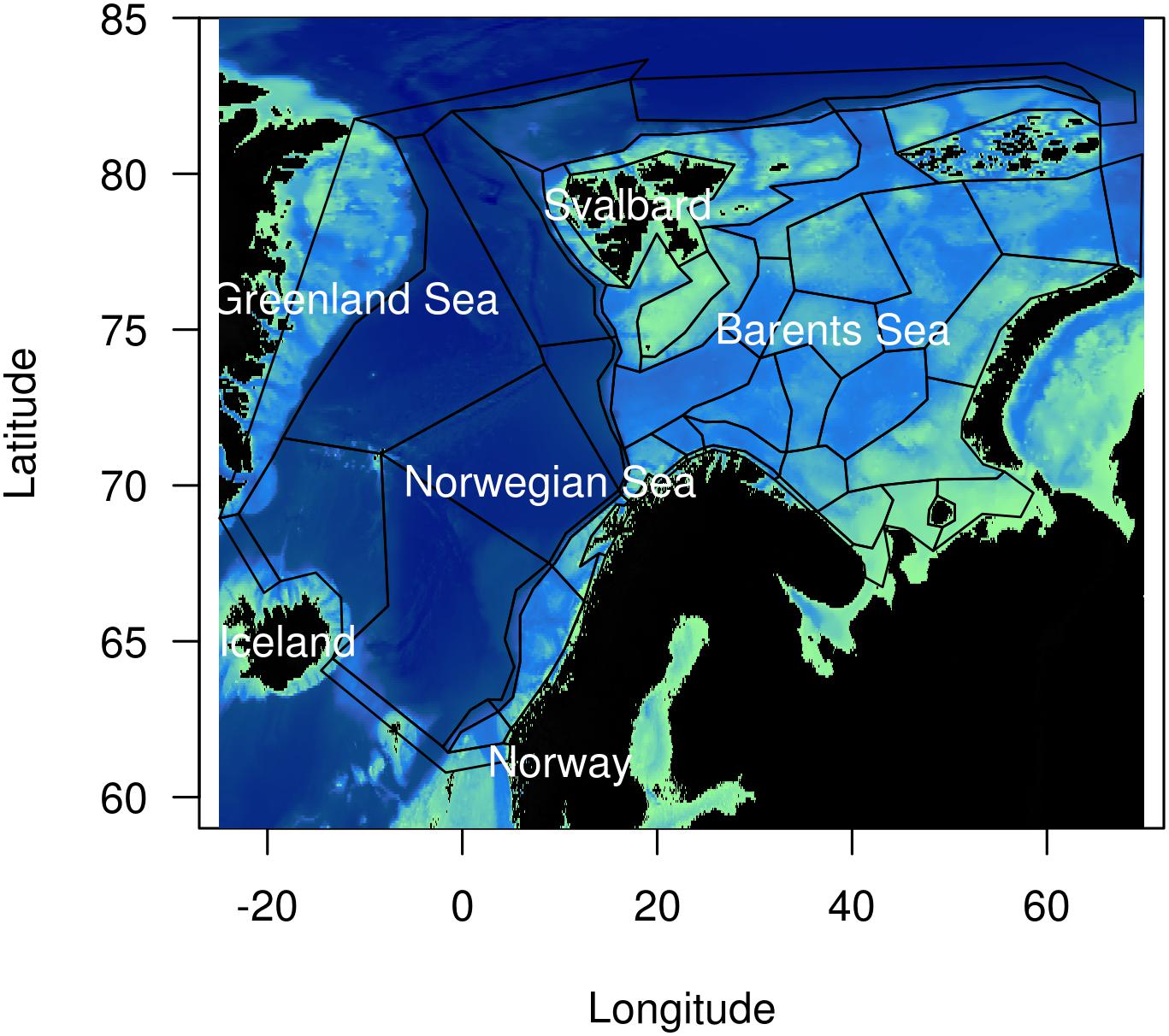
Figure 1. Topography of the Norwegian, Barents and Greenland Seas, and polygons in the NoBa model domain. Dark colors indicate deep areas.
The 53 species and functional groups (hereafter components) are connected through a flexible diet matrix, where the predator–prey interactions are defined as fractions of prey available for the predator. However, if the prey is an inadequate size for the predator, or not overlapping in time and space, the predator will switch to another prey. Likewise, the biomass of the prey will have a large impact on the diet of the predator. In Hansen et al. (2019), it was found that of the five most important key life history parameters (Pantus, 2006), the growth rates of particularly the lower trophic levels are important for the behavior and responses in the model.
As physical forcing, the Nordic and Barents Seas Atlantis model (hereafter NoBa) applied information on temperature, salinity and currents from three different set-ups of the Regional Ocean Model System model (Shchepetkin and McWilliams, 2005), covering the periods 1981–2000 (reference period), 2001–2005 (for comparison of observed and modeled present day biomass) and 2006–2068 (future climate; regional downscaled IPCC RCP4.5 scenario (Sandø et al., 2014; Skogen et al., 2018). The differences between the three configurations of the ROMS model are likely to introduce changes in the physical forcing, and we have therefore chosen to mainly focus on results from the latter part of the simulation (2006–2068). There are, however, comparisons between historical and model biomasses for the period 2001–2015. The temperature trend in the period 2006–2068 is relatively weak, ΔSST ∼0,02° year–1, although with regional differences. No trend in primary production is seen, but the timing of spring bloom is 1 month earlier at the end of the period (Skogen et al., 2018). Despite the limitations in using one single possible future climate evolution as forecasts of future conditions, we see this as a novel approach to enhance our understanding of marine species’ and systems responses to multiple climate drivers and pressures, which further can be fed into the development of climate adaptation strategies and actions.
The NoBa model has a spin-up time of 24 years, for which the model only applies the physical forcing from 1981. Thereafter, the simulation ran for 87 years, including a 36-year period following historical fishing levels for the main commercially important stocks (Table 1), from 1981 to 2016. The historical fishing levels were calculated using assessment catches and total stock biomass data for the larger commercial stocks (ICES 2017, 2018b). As opposed to using the total catches from the assessments directly in the model, we chose to apply time series of fishing mortality (yr–1). These were calculated by dividing total catch (t yr–1) by total stock biomass (t). The last part of the model simulations (2016–2068) applied the fishing mortality representing maximum sustainable yield (Table 1). The fishing mortality changes only once per year and transfers from the previous year’s mortality to current mortality over the course of 1 day. The fishing mortality was applied on the whole stock, evenly across the model domain. We only briefly present a model-observation comparison, as a full skill assessment of the model would be a paper in itself.
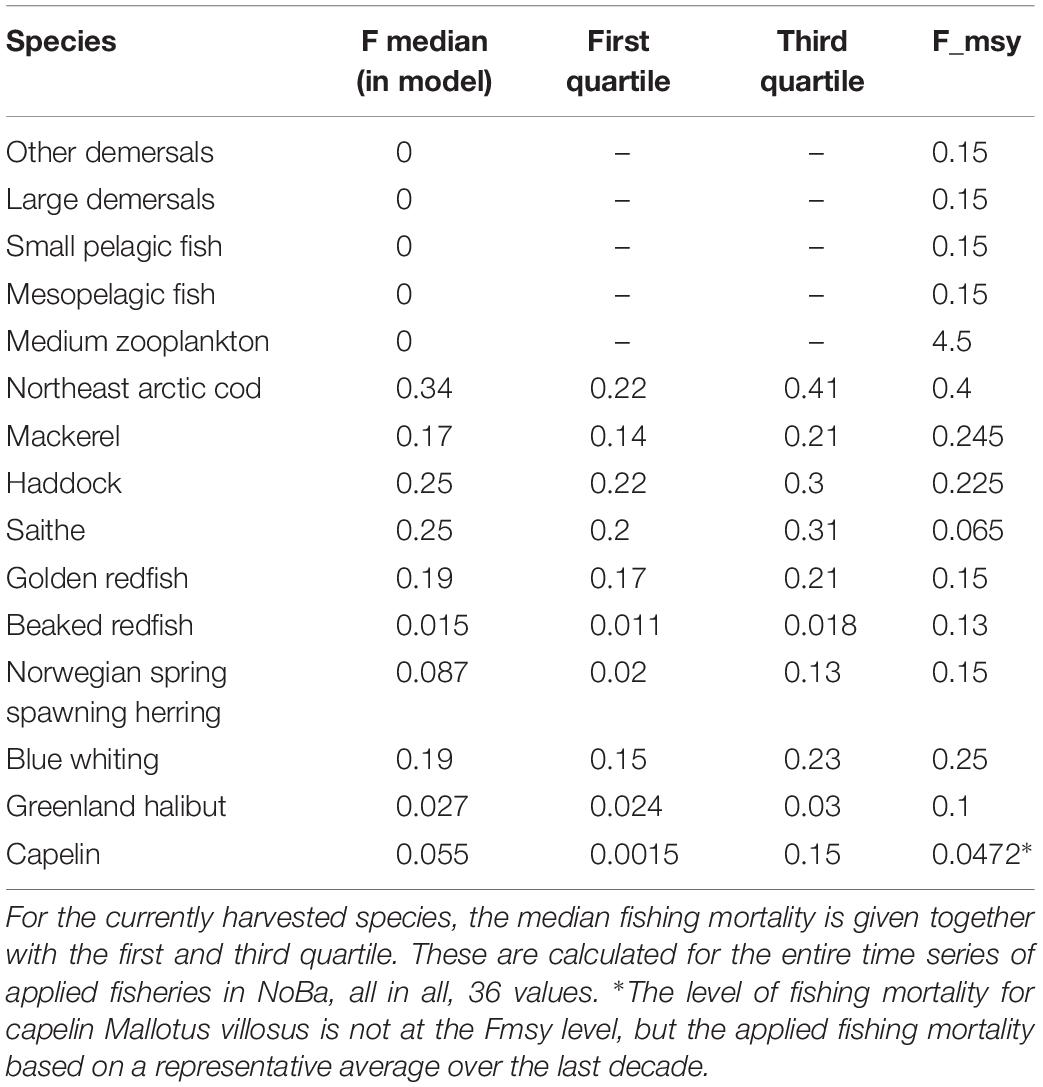
Table 1. Overview of harvested species, their current fishing mortality and their estimated Fmsy level in the model.
To identify additional new components for potential fishing, we used fisheries statistics from the period 1980–2010. These data were obtained from the Norwegian Directorate of Fisheries, and have a higher spatial resolution compared to ICES official areas (Directorate of Fisheries, 2019). Only areas overlapping with the model domain were included, all data collected in areas outside of the model domain were excluded. The data were resolved at species-level, thus the first step was sorting the species into their respective components (either species-specific or functional groups – Supplementary Table S1). The species where harvest was already implemented in the model was removed from the list, as the aim was to identify new groups for harvesting. The resulting list represented commercial species of less economic importance not currently being harvested in the model. Based on this, we ended up with 103 components/species not currently being harvested in the model. Based on their average catches, the functional groups, small pelagic fish, large demersal and other demersals, made up 67% of the total catch of the top six groups in terms of average catches over the 30-year period represented in the data. As these three functional groups were already present in the model, we decided to use these as three of our additional groups.
In addition to these three, mesopelagic fish and mesozooplankton were added to the list of additional components that should be harvested. The reason for the last two is the increased interest in these resources, and that they most likely will be fished at a larger scale in a not too distant future (e.g., Hidalgo and Browman, 2019 and references therein).
The fishing mortality at maximum sustainable yield (Fmsy; Table 1) was calculated for each of the harvested components by performing multiple simulations. In these simulations, the catches of other species were held constant at current levels, whereas the catch level of the species of interest was increased until the stock collapsed. Each simulation was run for a period of 55 years, and biomass and catches were averaged over the last 10 years of each simulation. Fmsy was then the fishing mortality that gave the highest average catches at the end of the simulation while at the same time avoiding biomass collapse. The high Fmsy for mesozooplankton (4.5; Table 1) emerges from the high zooplankton production and corresponds to productivity levels found in e.g., the Norwecom.e2e model (Morten D. Skogen, personal communication).
The scenarios were then classified as one of four scenarios (Global sustainability, World markets, Local stewardship, and National enterprise; Groeneveld et al., 2018), each involving a different fishing strategy. In the case of ‘Global sustainability’ the scenario includes lower fish and meat consumption, reductions in fishing areas and the introduction of lower impact fishing gears. The result is a reduced fishing pressure which is interpreted here as 0.6 × Fmsy. In the ‘World markets’ scenario, fish are obtained from the cheapest source, decommissioning reduced, few legal and technical restrictions on fishing with a greater competition for resources. This is manifested as a lower fishing pressure (0.8 × Fmsy) but not to the same extent as the previous scenario. The ‘Local stewardship’ scenario is considered, amongst other things, under local or regional governance of fish resources and a mosaic of different management measures. In this scenario, the result is that F occurs at MSY (1.0 × Fmsy). The last scenario (National enterprise) entails relying on national supplies with decreased imports thus a greater pressure on local stocks. This results in an increase in the fishing pressure above MSY (1.1 × Fmsy). These applications were discussed within the ICES community before being used (Katell Hamon, personal communication).
A future with both changed environmental forcing and harvesting strategies were simulated by using the downscaled RCP4.5 forcing and the four fishing pressure scenarios (0.6, 0.8, 1.0, 1.1 × Fmsy). Hence, the model was run with historical fishing pressure until 2017, from then on the Fmsy value and its multiplier was applied. The simulations were divided into two batches, those including Fmsy for commercial species only, and those including the additional five species mentioned above. For the five additional species, the fishing pressure changed from 0 to Fmsy in 2017. Capelin is a special case. This is a short-lived fish, fished almost exclusively on the spawning fraction of the stock and with total spawning mortality. The fishable biomass of capelin varies by an order of magnitude, and the concept of a “constant Fmsy” fits poorly to this stock. In high capelin years, the stock can sustain a high fishing mortality and still achieve full reproductive success, while in low capelin years any fishing level could impair future recruitment. The stock is managed with an escapement strategy for precisely this reason. Therefore, an average catch rate calculated over the last decade was applied for capelin. This fishing mortality level was multiplied with the fractions defined above. However, due to a recent collapse in the population, in addition to a large predator cod population, the fishing pressure applied to the capelin population was very low (Table 1).
The mesozooplankton does not have this total spawning mortality, and a fishery would likely harvest a greater variety of life stages. Furthermore, multiple species were combined into a single “mesozooplankton” stock within the model, which would make the level of specificity in the capelin case difficult to achieve. We therefore considered a simplified “constant Fmsy” appropriate for the mesozooplankton.
For each set of socio-political simulations (global sustainability, local stewardship, national enterprise, world market; Groeneveld et al., 2018), there are 28 different runs. 14 of these include ‘all’ species, 14 include only those currently harvested in the model system (Table 1). Each set of 14 simulations followed an individual pattern of mesozooplankton growth. The variability was based on a time series of mesozooplankton biomass in the Norwegian sea for the period 1995–2017 (Broms et al., 2016). The mesozooplankton growth was calculated by the mesozooplankton biomass pattern from this time series, twelve of these started in a different year (see example in Supplementary Figure S1), one had random variation based on the time series and the last replicate did not apply any zooplankton growth forcing at all. The reasoning for applying 13 different time series for mesozooplankton growth in the model was that complex end-to-end ecosystem models incorporate a large degree of uncertainty. Performing the simulations this way meant that an envelope of possible results was created for a larger part of the components, within which ranges we were confident. All in all, this gives us 112 simulations. We use the scenario with 1 × Fmsy as a base case run, all other runs will be compared to this one.
All results are presented using ecosystem guilds, where we have chosen to use pelagic fish, demersal fish, non-harvested lower trophic levels and harvested lower trophic levels. We also chose to split between harvested and non-harvested lower trophic levels. The reason for doing so was that these components have a tendency to increase when others at the same level decrease, hence disguising any changes.
Simple ecological and fisheries indicators were calculated for comparing the differences between the scenarios (Table 2). Pelagic and demersal catches, and the relationship between pelagic and demersal biomass are all indicators that also were used in the study by Olsen et al. (2018).
Results
The fourteen different time series of zooplankton applied for each set of management scenarios created an envelope of solutions for the components in the model. This was the case for both the pelagic and the demersal fish, which ended up by being almost as vulnerable to the bottom-up effects of mesozooplankton growth. Some top predators such as cod, who have a larger spectrum of prey, were not as dependent on one single prey source. The variability at lower trophic levels following the changes in mesozooplankton time series were larger than the effects of any of the changes in the harvest regimes (Figure 2).
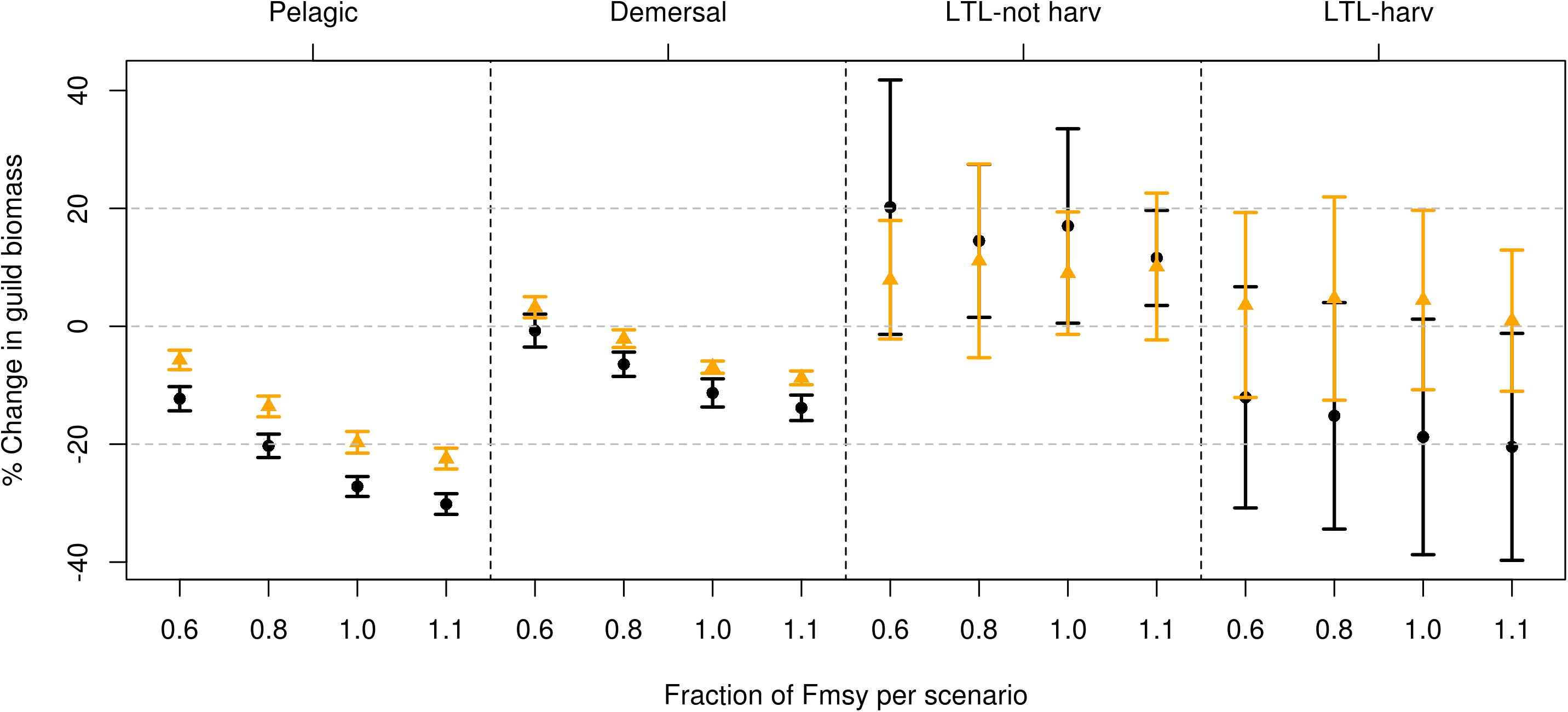
Figure 2. Change in guild biomass (%) between future (2055–2065) scenarios compared to historic scenarios (2005–2015). The dots represent the average difference for all components included in the guild, lines represent one standard deviation, resulting from the 14 replicates. There are eight sets of simulations within each guild, where orange dots and lines represent harvest of the large commercial components only and black includes harvest of the large commercial components plus the five additional components. The fraction of Fmsy for each scenario is indicated on the x-axis for each guild. The demersal guild includes cod, haddock, Greenland halibut, golden redfish, beaked redfish, and the functional groups “other demersals” and “large demersals.” The pelagic guild includes mackerel, blue whiting, herring, capelin and polar cod. LTL-harv are the lower trophic levels that are being harvested: mesozooplankton, prawns and mesopelagic fish, whereas LTL-not harv are the lower trophic levels not being harvested: jellyfish, small and large zooplankton.
Overall, the impact of changing the harvest in the model from historical levels to the fractions of Fmsy applied in the different scenarios, was more evident in the ‘all in’ scenarios compared to the ‘commercial’ scenarios (Figures 3, 4). This was the case both for the demersal and pelagic guilds. The time series of the guild shows no significant deviations between the eight management scenarios in time (Figures 3, 4).
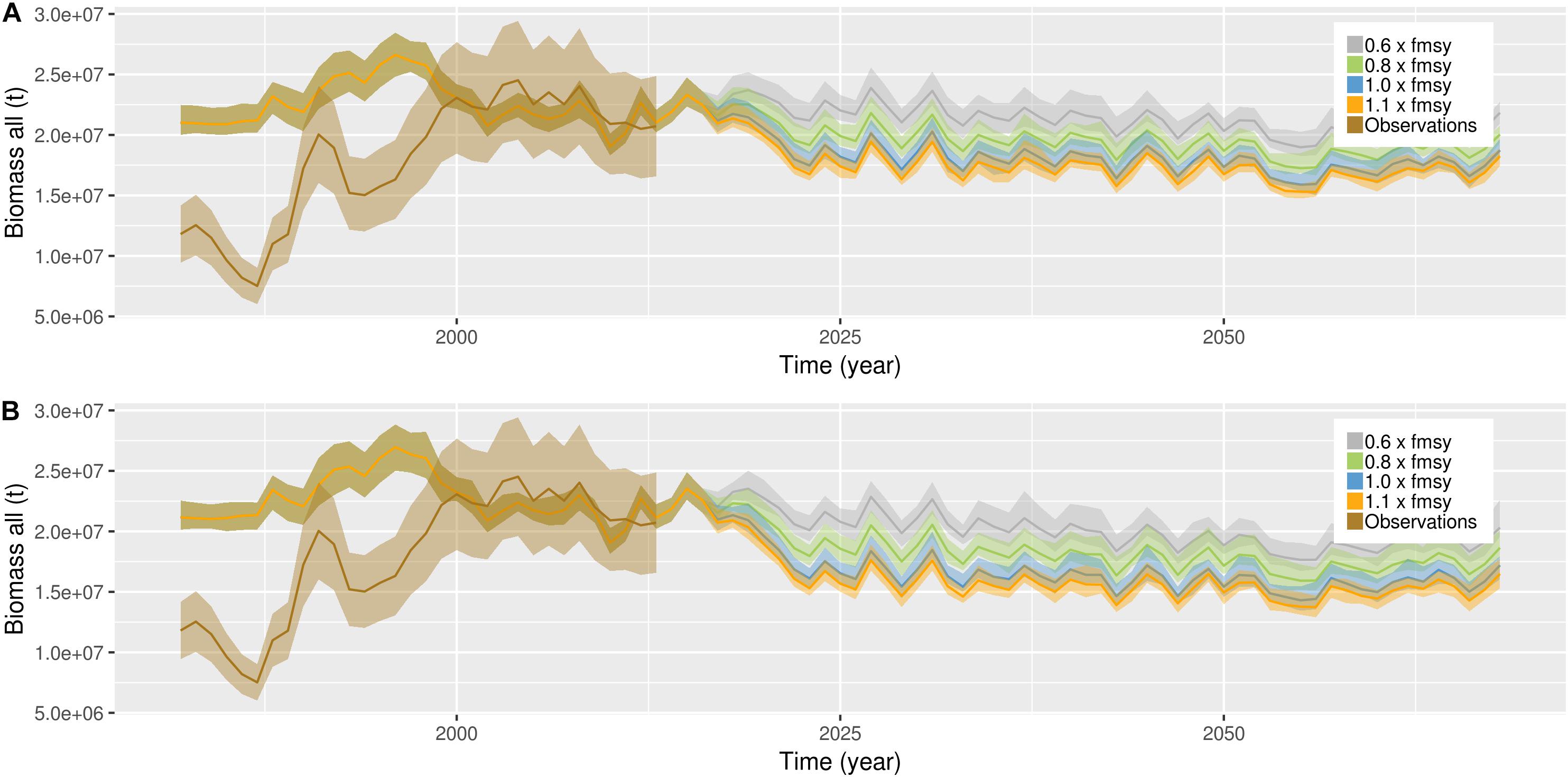
Figure 3. Temporal development of pelagic biomass (biomass of cohorts included in the assessment) in the four scenarios only including commercial fish (A) and in the four scenarios including additional harvest on mesopelagic fish, other demersals, large demersals, zooplankton and small pelagic fish (B). Brown (transparent) is observations of total biomass from assessment reports, including 20% uncertainty. Gray line represents global sustainability, green represents world market, blue represents local stewardship and orange represents national enterprise scenario. The shading represents one standard deviation from the mean of the 14 different simulations representing each scenario.
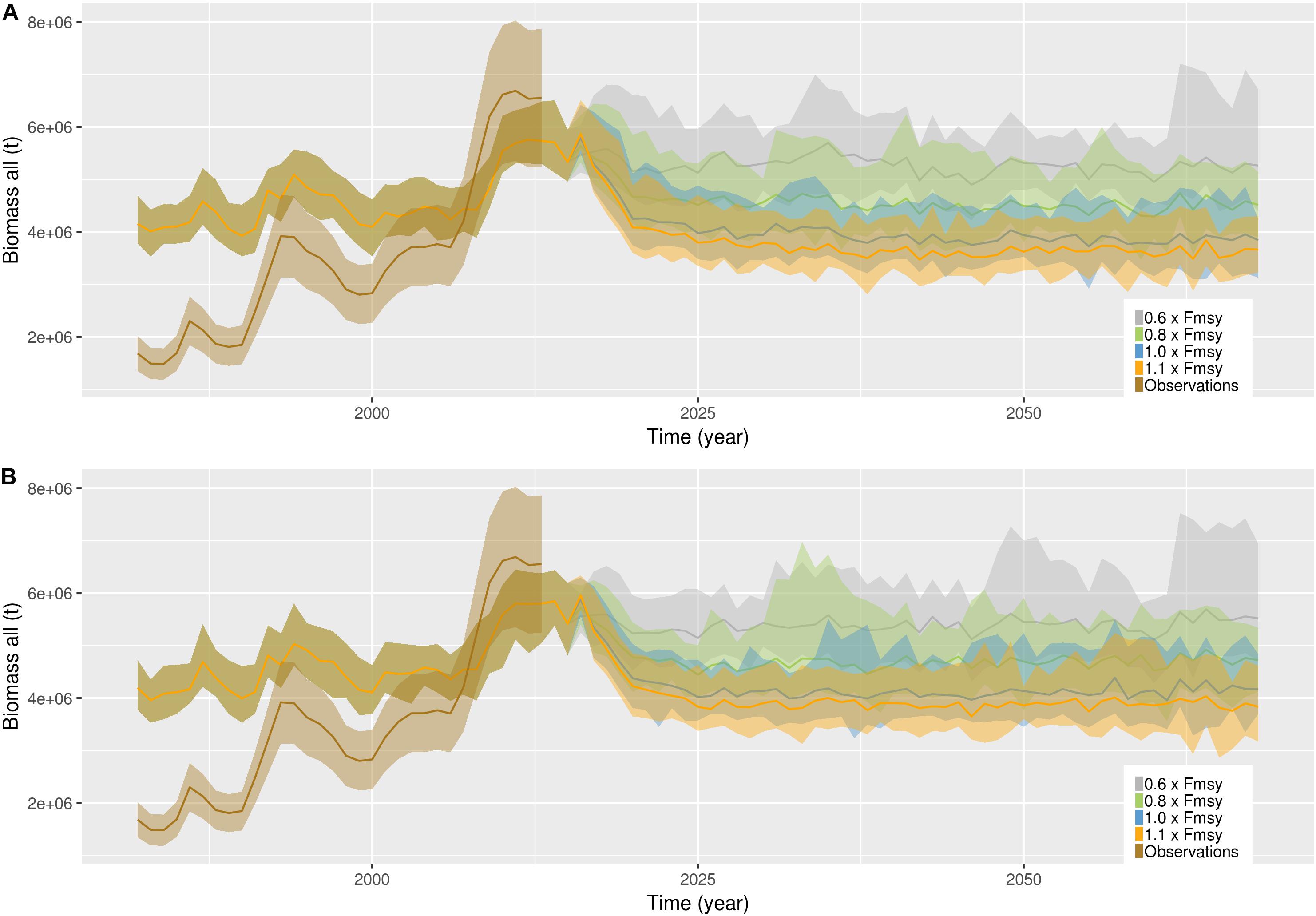
Figure 4. Temporal development of demersal biomass (biomass of cohorts included in the assessment) in the four scenarios only including commercial fish (A) and in the four scenarios including additional harvest on mesopelagic fish, other demersal, large demersal, zooplankton and small pelagic fish (B). Brown (transparent) is observations of total biomass from assessment reports, including 20% uncertainty. Gray line represents global sustainability, green represents world market, blue represents local stewardship and orange represents national enterprise scenario. The shading represents one standard deviation from the mean of the 14 different simulations representing each scenario.
The reader should be aware that we are only applying the fishing pressure described in the socio-political scenarios, and have for simplification chosen not to follow the other details given in Groeneveld et al. (2018). Also, as several of the stocks in Norwegian waters have been fished below Fmsy in recent decades, this change in harvest pressure will in itself lead to a decrease in biomass when compared to historical levels of the stocks.
Evaluation of Historical Biomass Levels
NoBa Atlantis was built using input information on biomasses, weights, abundances, distributions and other life history parameters from assessment reports, literature and gray literature (Hansen et al., 2016). When first initializing the model based on all available information, it was run toward equilibrium repeating a 1-year cycle (daily) of physical forcing (Hansen et al., 2016). After the spin-up of 24 years, the majority of the components were between 0.5 and 1.5 of the initial values (Hansen et al., 2019). Here, the set-up is changed to run the model with continuous daily physical forcing. In all simulations, we applied the historical harvest pressure for all the commercial stocks. The pelagic and demersal guilds were compared to observations from ICES assessments; WGWIDE and AFWG, respectively (Figures 3, 4). The observations from 1981–2000 was used for tuning, therefore only observations from 2001 to 2015 were used for comparison. For this period, the pelagic guild biomasses fit well (Figure 3), but not so well in terms of timing of events, resulting in a correlation of 0.49 (p = 0.07). The demersal biomass fit well and had a much better match in terms of timing (Figure 4, resulting in a correlation of 0.87 (p = 0). It has to be mentioned that the observations that were used for comparison were not entirely independent, as they were a result of the harvesting regime which was also applied in the model.
Guilds in a Future Climate and With Different Harvesting Regimes
Global Sustainability (0.6 × Fmsy)
For all guilds except the pelagic and the mammals, the reduced fishing pressure (0.6 × Fmsy) that was applied in all global sustainability simulations with harvesting of commercial species led to a positive response, compared to historical levels (Figures 2, 5). Introducing more components to the harvest had, however, a negative effect on the already established pelagic and demersal guilds, due to direct and indirect predator–prey effects. The decrease shown in the pelagic guild was both caused by a decline in Norwegian spring spawning herring, and an increased predation pressure from top predators. The biological indicators (Table 2) showed the same picture, with the biomasses of the global sustainability scenarios being highest compared to the other scenarios for the demersal, pelagic and lower trophic level harvested biomass (Figure 5; DemB, PelB, LTLhB, respectively). The fraction of pelagic to demersal biomass (Figure 5; PelDem parameter) was highest for both the ‘commercial’ simulations and the ‘all in’ simulations, compared to the three other scenarios. However, the historical period had a higher fraction than all the other projections. In the ‘commercial’ simulations, an increase in both the catches and biomass of the demersal guild (Figure 5; DemB and DemC, respectively) was seen, in contrast to a lower demersal biomass when more ecosystem components were harvested. Considering the lower trophic level biomass (Figure 5; LTLnhB and LTLhB parameter), this was at its lowest value for the commercial scenario, completely opposite of the ‘all in’ scenarios. This was a direct result of the removal of LTL, leaving a larger share of the phytoplankton for the non-harvested LTL components. This was seen in all scenarios. Compared to the historical levels, both the non-harvested and the harvested LTLs experienced an increase in the biomass in the ‘commercial’ scenario. The same was valid for catches of all guilds, including the lower trophic levels (Figure 5). Catches of demersal species were higher in the ‘all in’ simulations compared to the ‘commercial’ simulations, whereas the catches of pelagic fish decreased by 9% when more ecosystem components were harvested, despite the higher number of harvested groups included in the pelagic guild. The total catch biomass was 37 times higher in the ‘all in’ simulations compared to the commercial simulations (Figure 6).
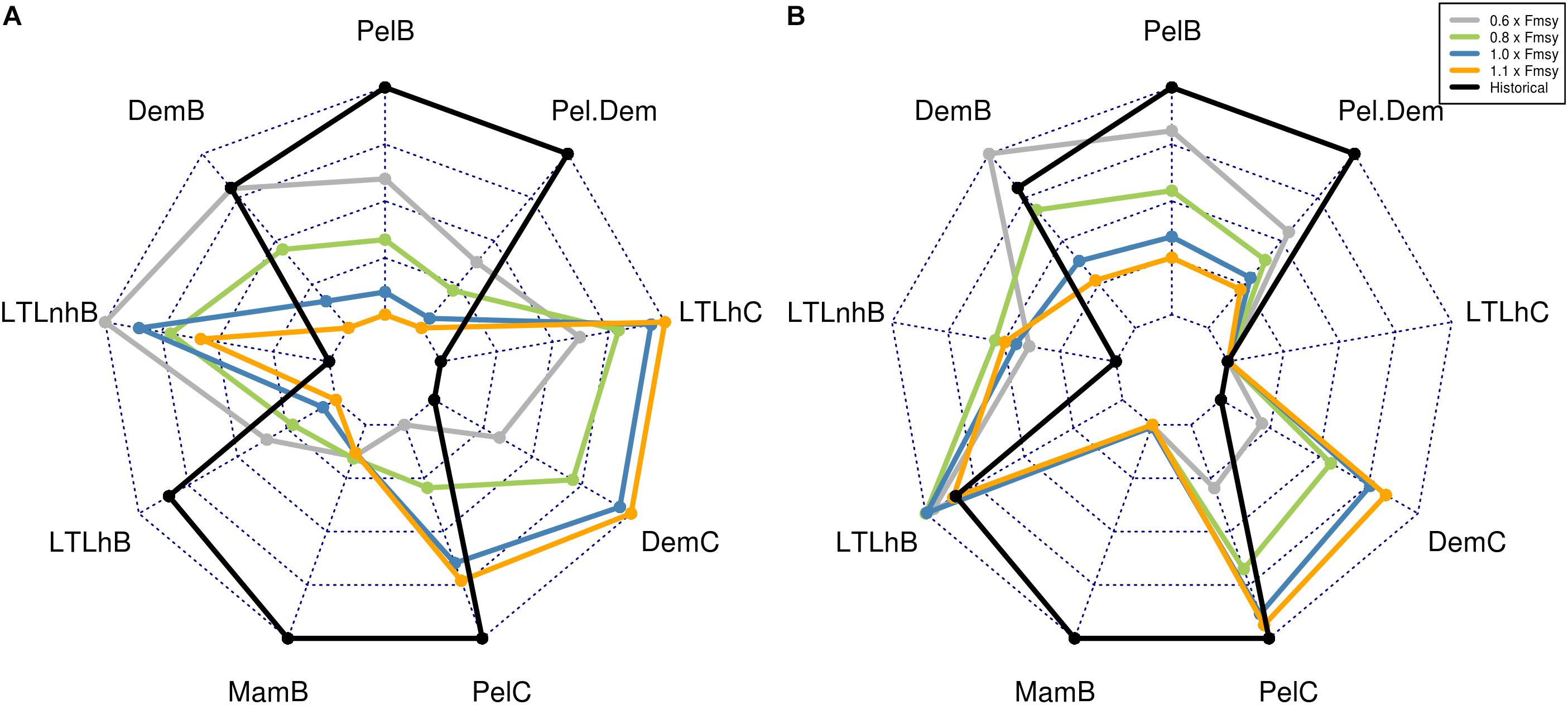
Figure 5. Ecological indicators for the four scenarios investigated. (A) Results from the scenarios when only commercial species are harvested. (B) Results from the scenarios when all components (commercial + five additional components) are being harvested. For the four scenarios, gs is the global sustainability (gray), ls is local stewardship (blue), ne is national enterprise (orange) and wm is world market (green). In addition, the indicators from the historical period of the simulations are added to the figures, for comparison between future projections and historical results. Biomass indicators are grouped on the left and catch indicators on the right side of the radar charts. All values increase from the center of the radar plot, to the edge.
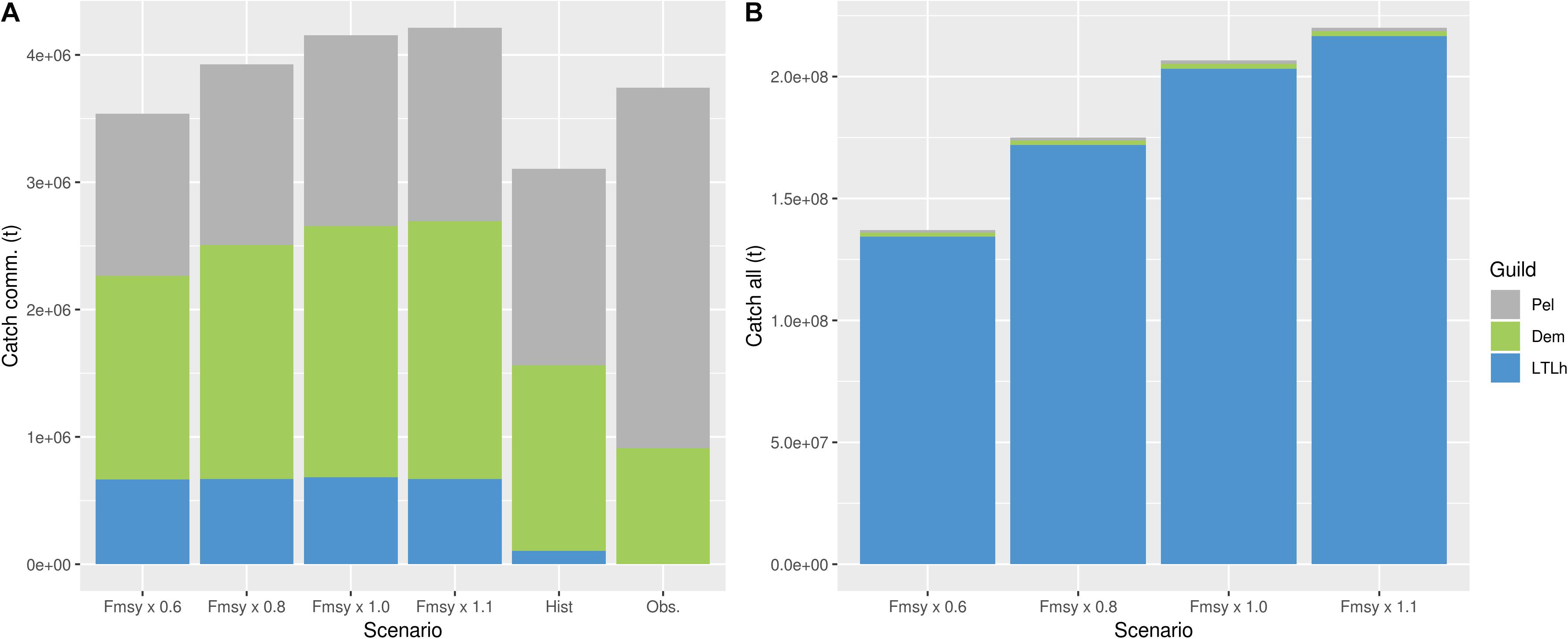
Figure 6. (A) Simulated average total catches per year for the period 2055–2065 for the four scenarios (Fmsy × 0.6, Fmsy × 0.8, Fmsy × 1.0, and Fmsy × 1.1), simulated average total catches per year for the period 2005–2015 (Hist) and observed (Obs.). (B) Simulated average catches for the period 2055–2065 for the four scenarios including the additional components. Both figures show catches for the same guilds; pelagic (Pel - gray), demersal (Dem - green) and harvested lower trophic levels (LTLh - blue). Notice the difference in catch levels between panels (A) and (B), and that lower trophic level is missing from the observed bar.
World Markets 0.8 × Fmsy
In a future climate, both demersal and pelagic guilds showed a decrease compared to historical levels (Figure 2), due to an increase in the fishing pressure compared to the global sustainability scenario (0.6 × Fmsy). The decrease in the demersal guild was around 10%, while the pelagic guild experienced a difference between 17 and 20% compared to historical levels. As in most of the other scenarios, the pelagic and demersal guild experienced a significant reduction in their biomass levels when more components were harvested (Figure 2). The lower trophic levels not harvested experienced their largest biomass for this scenario, and the variability was higher for the simulations that only included the currently harvested components (Figure 2). The variation in the lower trophic levels canceled out any significant differences between the two sets of scenarios, indicating that the forced variation in mesozooplankton growth was as important as changes at the harvest level. The marine mammals (Figure 5; MamB parameter) had their highest biomass in the ‘all in’ simulations, although the differences between the scenarios in total marine mammal biomasses were small. The same shift in the demersal catches compared to the pelagic catches as in the global sustainability scenario was seen here, with the ‘all in’ simulations providing a higher demersal catch compared to the ‘commercial’ simulations (Figure 5; DemC and PelC, respectively). Likewise, the demersal and pelagic biomass (Figure 5; DemB and PelB, respectively) was higher in the ‘commercial‘ simulations compared to the ‘all in’ simulations. In total, the pelagic catches decreased by 10% when additional species were being harvested, despite the pelagic guild now including more species. When comparing the total average catches, there is an increase of close to 49 times between the ‘commercial’ simulations and the ‘all in’ simulations (Figure 6).
Local Stewardship 1.0 × Fmsy
The local stewardship (1.0 × Fmsy) scenario can also be interpreted as representing the effect of environmental changes only. Here, for the future climate, the demersal and pelagic guilds experienced a decline compared to the two previous scenarios and compared to historical levels (Figure 2). The difference between the simulations only including currently harvested species and those including additional species increased for the demersal guild. There was no such significant difference between species/guilds for the lower trophic levels for the two sets of simulations. However, while the scenarios that include harvest on the additional components experienced a future decline in the non-harvested lower trophic levels, compared to the global sustainability scenario, the scenarios excluding the additional components showed a small increase. Local stewardship showed a surprisingly high biomass of lower trophic levels for the ‘all in’ scenario (Figure 5; LTLhB parameter). Apart from that, it scored low on the total biomass, and high on the total catches of multiple guilds, not unexpected (Figure 5). At the Fmsy level, the same pattern in the catches as in the scenarios applying lower harvest mortalities was seen, with a higher demersal catch in the ‘all in’ simulations compared to the ‘commercial’ (Figure 5; DemC). The pelagic catches, on the other hand, decreased by 6% when more species were harvested, despite slightly more pelagic components being harvested. The total catches in the ‘all in’ scenario was roughly 49 times higher than the total catches in the commercial scenario (Figure 6).
National Enterprise 1.1 × Fmsy
The difference between the ‘commercial’ simulations and the ‘all in’ simulations was at its highest in the demersal guild for this scenario. The demersal guild experienced a decrease of between 15 and 17% compared to the historical levels, much lower than the 20–30% decrease seen in the pelagic guild. Both lower trophic levels were at the lowest biomasses for this scenario, including the components not being harvested (Figure 2). The ‘commercial’ simulations showed a slight positive development in the lower trophic levels, whereas adding harvest at this magnitude on the lower trophic levels decreased their biomasses by close to 20% compared to historical levels (Figure 2). National enterprise showed by far the largest catches of all the harvested components of the ecosystem (Figure 5; DemC, PelC, LTLhC, respectively, Figure 6). It had a higher biomass at the lower trophic level (non-harvested) compared to both global sustainability and the local stewardship. This can be explained by the effect of both indirect and direct predator–prey interactions caused by the removal of top and mid-level predators, such as demersal and pelagic fish. There was a smaller difference between the local stewardship scenario and the national enterprise for the pelagic catches compared to the demersal catches when only the commercial species were harvested. This was not as clear in the ‘all in’ simulations. However, the pelagic catches decreased by 5% when additional species were harvested, even though the number of harvested pelagic species increased slightly. The average catches in the commercial simulations were more than 50 times lower than in the ‘all in’ simulations for this particular scenario (Figure 6).
Discussion
In this study, we explored the ecosystem effects of changes in management regimes for eight different scenarios in two environmental settings (historical and future climate). In each of the scenarios, multiple simulations were performed to introduce bottom-up variability by forcing the growth rate of mesozooplankton. All the scenarios applied different fractions of Fmsy mortality for the major commercial fisheries in the Norwegian and Barents seas. In four of the eight scenarios, additional components were harvested. The additional species were chosen based on catch statistics (small pelagics, large demersals and other demersals) and increased commercial interest in the component (mesopelagic fish and mesozooplankton).
Model Uncertainty
Structural uncertainties in large end-to-end ecosystem models can be quantified in different ways, for instance by running ensembles of models, or by testing multiple model set-ups within the same model framework (Lehuta et al., 2016). There are other end to end models representing the Norwegian and Barents seas, for example the NORWegian ECOlogical Model system End-To-End (NORWECOM.E2E), a coupled physical, chemical, biological model system (Skogen et al., 2007), covering primary and secondary producers as well as key fish species. The model has been validated by comparison with field data in the Nordic and Barents seas (Skogen et al., 2007; Hjøllo et al., 2012; Utne et al., 2012). The NORWECOM.E2E model has been run using the same physical forcing as in this study, and showed a slight future decline in some of the pelagic fish components (herring), using the present level of the fishery (S. S. Hjøllo, personal communication), and a slight increase in the zooplankton component. Having the same trend in two so different ecosystem model supports the findings in this study. Uncertainties within the model system originates from multiple sources, e.g., parameter settings, initial conditions, simplifications of ecological processes and the application of fisheries. Given the complexity and computational costs of running NoBa, we eventually ended up with the 14 simulations for each scenario. As we already were aware of the models sensitivity to mesozooplankton (Hansen et al., 2019), we were confident that adding this variability creates reasonable envelopes around the components in the model, something also shown in Figures 3, 4. For further studies, uncertainties connected to recruitment and/or biomass levels of the three potentially most important pelagic species in the systems (polar cod, capelin and Norwegian spring spawning herring) could be explored.
Ecosystem Effects of Changes in Management and Climate
The cumulative effect of fisheries and climate has, for a majority of studies, showed declines in catches and productivity in commercially important stocks (see e.g., Cheung et al., 2016; FAO, 2018b), although this also depends on the physical projection applied. While climate change might decrease the production in some regions of the oceans, the world is also facing a growing requirement for food (United Nations, Department of Economic and Social Affairs, Population Division, 2019). This is a direct result of a growing human population and the world health goal of zero hunger, indicating that there will be continued demand for increasing catches from the marine fisheries as the world population continues to grow. Enhancing the number of components and also spreading the harvest across multiple trophic levels has been suggested as one solution (Sethi et al., 2010; Garcia et al., 2012; Kolding et al., 2016). However, going to a fully balanced harvest might not be the preferred solution (Howell et al., 2016; Nilsen, 2018).
The Barents and Norwegian Seas ecosystems are among the more balanced harvested ecosystems of the world, harvesting on most trophic levels from copepods (commercial quota given from 2019) to marine mammals (Howell et al., 2016). Increasing the harvest on lower trophic levels can introduce unforeseen predator–prey effects, lowering the catches and/or economic yield at higher trophic levels (Smith et al., 2011). Despite not being a surprise based on previous sensitivity studies of the model system (Pantus, 2006; Hansen et al., 2019), it was interesting to note the strong effect of implementing an Fmsy fishery for the mesozooplankton and mesopelagic fish. Not only was the effect evident in the increase in total catches, but also in the decrease in biomass of other components in the system due to direct and indirect predator–prey interactions. Even though the sensitivity of the model to the zooplankton components might be a structural effect of the Atlantis framework (Hansen et al., 2019), the importance of mesozooplankton (in particular Calanus finmarchicus) as a food source for pelagic fish in the Norwegian Sea is unquestionable (Bachiller et al., 2016).
Contrary to (Smith et al., 2011), where harvesting on forage fish had a strong impact on parts of the ecosystem, the Norwegian and Barents seas ecosystems seem to handle fisheries targeting pelagic fish at Fmsy well. However, there is an important piece that is missing in Fmsy scenarios applied here, namely the capelin. For all eight scenarios, this component is fished rather lightly. Capelin, being short-lived and semelparous, does not translate into an Fmsy easily, and it can be debated whether or not it is possible, or at all correct, to calculate an Fmsy for this component. Based on this we chose to leave the catches at a representative average for the last decade. Due to the total catch allowances over recent years being low, corresponding to the high biomass of Northeast Arctic cod (Gjøsaeter et al., 2015), this resulted in a low harvest rate of the capelin, leaving it rather unresponsive to changes in the fishing pressure. Despite this missing piece, there is an increasingly negative response in catches of the demersal guild with increasing fishing pressure (from 0.6 to 1.1 × Fmsy) in the ‘all in’ simulations compared to the commercial simulations. This indicates a growing vulnerability in the whole system when a larger number of components are being harvested. It is possible that this increasing vulnerability would be even stronger if the capelin had been harvested at a higher rate. This is based on empirical observations showing severe ecosystem effects caused by simultaneously low biomasses of pelagic fish and zooplankton in the Barents Sea (Gjøsaeter et al., 2009). For future studies, we suggest that the harvest pressure on capelin should be implemented using a version of the escapement rule that leaves at least 200,000 t of capelin to spawn (Gjøsaeter et al., 2015).
With the large changes in the management strategies for the historical and future projections, it was particularly difficult to track down changes related to increasing temperatures alone. However, Figure 5 showed an increase in biomasses at the lower trophic levels for 2055–2065 compared to the historical time slot, regardless of fishing pressure at the higher trophic levels. This is in agreement with findings in other papers exploring climate change in the high latitudes (e.g., Steinacher et al., 2010), although model systems applying different physical forcing have reported declines in primary production (Slagstad et al., 2015). Both these studies explain their differences based on changes in the environment, such as reduced light and temperature limitation and advection. Unfortunately, RCP 4.5 was the only down-scaled scenario available for the Nordic and Barents Seas. Skogen et al. (2018) showed that downscaling of low-resolution global models to regional models is important for this area, which is the reason why we chose not to use a global model representing any of the other RCPs. For future work, we strongly recommend that multiple physical scenarios should be available, as this will provide the opportunity to differentiate between the effect of climate change alone compared to the impact of changes in management.
Impact on Catches Following Changes in Management and Climate
Calculating the Fmsy levels for the additional components resulted in the same value for several of the non-commercial stocks (Table 2). This might emerge from these components not being as thoroughly evaluated and parameterized as the large, commercially important stocks. For the commercially important stocks there are much more data available. These conclusions are also supported by Nilsen et al. (this issue), who found that the harvest levels of these components were generally lower than for the commercially important stocks, although the production levels one would expect from these components should not be very different. From Table 1, the Fmsy level for saithe, beaked redfish and Greenland halibut diverges from the historical levels of fishing mortalities.
Applying an Fmsy on the mesopelagic fish and mesozooplankton is relatively unrealistic, due to both the technical aspects with these fisheries, and the role of these species as key prey in the ecosystem. Based on the Commision for the Conservation of Antarctic Marine Living Resources work, a precautionary approach is applied to set the quota for the mesozooplankton in the Norwegian Sea. This gives a total allowable catch of 165 000 tonnes from a total biomass estimated to be above 30 million tonnes, and it also includes area restrictions on where the harvest can take place. The area restrictions are meant to prevent issues with bycatch of eggs and larvae of other species. The aspect of bycatch is a concern with both mesopelagic fish and copepods, and was thoroughly discussed when the management plan for Calanus finmarchicus in the Norwegian Sea was developed (Broms et al., 2016). The main concern in this sense is the bycatch due to mesh size and target areas. We consider the technical details on these fisheries outside the scope of our study and have therefore only considered the ecosystem effects of fishing mortality as such.
The Fmsy multipliers used in this study were taken from the predefined CERES scenarios (Groeneveld et al., 2018). Single-species assessments are, to a large degree, managed with the aim of applying a Fmsy (ICES, 2018a). However, there have been studies showing that applying multiple single-species Fmsys simultaneously is not ecosystem-friendly (e.g., Gaichas et al., 2012), as the cumulative pressure of each of these might result in a total harvest above the threshold of the system. This is explained by predator–prey interactions between the species, which are not taken into account in assessments for the majority of the stocks worldwide (Walters et al., 2005;. Skern-Mauritzen et al., 2016). Although we do not experience a collapse in the system when applying multiple Fmsy simultaneously, we do notice a decrease in both the pelagic and demersal guilds when the Fmsy’s are introduced (Figures 3, 4). We explain this by the combined effect of introducing a higher F for some of the species, in combination with the decrease in biomass at lower trophic levels for half of the scenarios. In addition, there is a significant difference between applying a flat Fmsy on a stock, compared to the harvest control rules (HCR) that presently are a part of a majority of the commercial fisheries in the Norwegian and Barents seas. While an HCR possibly could prevent the collapse of the herring stock (not shown) that was experienced here, this was not the case with the invariant Fmsy.
Extending the number of harvested components, increased the total catches significantly due to the biomass at the lower trophic levels. This resulted in decreasing catches of the traditionally targeted pelagic and demersal guilds. The decrease was not dramatic, 6–10%, but it must be kept in mind that for these scenarios, the number of harvested species also increased for these guilds. Isolated, we would therefore expect a total increase, not a decrease. However, the catches of mesozooplankton increase with several magnitudes, introducing strong bottom-up effects. For the demersal guild, there was a clear connection between increased harvest pressure, increased number of components being harvested, and a decline of demersal catches. Comparing the historical (2005–2015) and the future (2055–2065) biomass, we found a clear decline in the future biomass of pelagic, demersal and LTL-harvested (‘all-in’ scenarios) components, caused mainly by the change in harvest regimes.
Conclusion
There are multiple important tradeoffs being identified within and between the management scenarios described here. The most emergent ones are related to the fishing of mesozooplankton and mesopelagic fish. Here, the catches of pelagic and demersal guilds decrease whereas the new fisheries at lower trophic levels can increase a lot. It also has to be kept in mind that the majority of the catches from the pelagic and demersal guilds (in particular the demersal) are being used directly for human consumption, whereas the newer lower trophic level catches with present day consumer behavior cannot fill that role. However, their use for food in aquaculture might be important in terms of food security.
Large end-to-end models such as NoBa should never be used for tactical management (Link et al., 2010; Fulton et al., 2014). However, they can be useful and informative for exploring ecosystem responses to cumulative changes as, for example, in management regimes and fisheries and environmental conditions. As the oceans are facing increasing pressure from global warming and food production industry, we need to explore the possible effects of changes in our traditional fisheries management. Here, using one realization (NorESM RCP4.5 scenario downscaled to regional resolution with ROMS) out of a range of possible future climate projections, we conclude that increasing harvest on lower trophic levels could be beneficial in terms of total catches, but multiple trade-offs need to be considered and discussed across the different sectors involved in both the traditional fisheries and potential new fisheries, before such management changes are implemented.
Data Availability Statement
The datasets generated for this study are available on request to the corresponding author.
Author Contributions
CH performed the simulation, created the figures, and is main author of manuscript. SH provided invaluable discussions on results and significant contributions to the manuscript and figures. RN and KD provided invaluable discussions on results and significant contributions to the manuscript.
Funding
SH acknowledges support from the European Union’s Horizon 2020 Research and Innovation Programme under grant agreement no. 677038 (ClimeFish). CH, RN, and KD acknowledge support from the European Union’s Horizon 2020 Research and Innovation Programme under grant agreement no. 678193 (CERES).
Conflict of Interest
The authors declare that the research was conducted in the absence of any commercial or financial relationships that could be construed as a potential conflict of interest.
The reviewer NJ declared a past co-authorship with one of the authors CH to the handling Editor.
Acknowledgments
We thank Dr. Daniel Howell (Institute of Marine Research, Bergen, Norway) for comments on a draft of this manuscript. We would also like to thank the three reviewers and the editor for all their effort and their valuable critique of the manuscript.
Supplementary Material
The Supplementary Material for this article can be found online at: https://www.frontiersin.org/articles/10.3389/fmars.2019.00668/full#supplementary-material
FIGURE S1 | Normalized zooplankton biomass time series from (in black) the unforced replicate, and (in orange, whole line) one of the 13 replicates that is forced with the time series of zooplankton growth. The dotted orange line is the fraction that eventually was multiplied by zooplankton growth and applied in the model system to create variability for each scenario.
TABLE S1 | Overview over functional groups and species included in NoBa Atlantis.
References
Armstrong, C. W., Vondolia, G. K., Foley, N. S., Henry, L.-A., Needham, K., and Ressurreição, A. (2019). Expert assessment of risks posed by climate change and anthropogenic activities to ecosystem services in the deep North Atlantic. Front. Mar. Sci. 6:158. doi: 10.3389/fmars.2019.00158
Bachiller, E., Skaret, G., Nøttestad, L., and Slotte, A. (2016). Feeding ecology Northeast Atlantic mackerel, Norwegian spring-spawning herring and blue whiting in the Norwegian Sea. PLoS One 11:e0149238. doi: 10.1371/journal.pone.0149238
Broms, C., Strand, E., Utne, K. R., Hjøllo, S., Sundby, S., and Melle, W. (2016). Vitenskapelig Bakgrunnsmateriale for Forvaltningsplan for Raudåte. Available at: http://hdl.handle.net/11250/2440945 (accessed April 26, 2019).
CERES (2016). “Exploratory socio-political scenarios for the fishery and aquaculture sectors in Europe,” in Deliverable D1.1-Glossy ‘Report Card’ Aimed at Stakeholders, eds J. K. Pinnegar, and G. H. Engelhard (Lowestoft: Centre for Environment, Fisheries & Aquaculture Science (Cefas)), 8.
Cheung, W. W. L., Lam, V. W. Y., Sarmiento, J. L., Kearney, K., Watson, R., Zeller, D., et al. (2010). Large-scale redistribution of maximum fisheries catch potential in the global ocean under climate change: climate change impacts on catch potential. Glob. Chang. Biol. 16, 24–35. doi: 10.1111/j.1365-2486.2009.01995.x
Cheung, W. W. L., Reygondeau, G., and Frölicher, T. L. (2016). Large benefits to marine fisheries of meeting the 1.5°C global warming target. Science 354, 1591–1594. doi: 10.1126/science.aag2331
Directorate of Fisheries, (2019). Official Norwegian Sales Notes (Landings) Statistics. Guwahati: Directorate of Fisheries.
Food and Agriculture Organization of the United Nations [FAO], (2018a). Impacts of Climate Change on Fisheries and Aquaculture: Synthesis of Current Knowledge, Adaptation and Mitigation Options. FAO Fisheries And Aquaculture Technical Paper 627. Rome: FAO.
Food and Agriculture Organization of the United Nations [FAO], (2018b). The State of World Fisheries and Aquaculture 2018. Rome: FAO.
Food and Agriculture Organization of the United Nations [FAO], (2008). Best Practices in Ecosystem Modelling for Informing an Ecosystem Approach to Fisheries. Rome: FAO.
Frank, K. T., Petrie, B., Leggett, W. C., and Boyce, D. G. (2016). Large scale, synchronous variability of marine fish populations driven by commercial exploitation. Proc. Natl. Acad. Sci. U.S.A. 113, 8248–8253. doi: 10.1073/pnas.1602325113
Fulton, E. A., Link, J. S., Kaplan, I. C., Savina-Rolland, M., Johnson, P., Ainsworth, C., et al. (2011). Lessons in modelling and management of marine ecosystems: the atlantis experience. Fish Fish. 12, 171–188. doi: 10.1111/j.1467-2979.2011.00412.x
Fulton, E. A., Smith, A. D. M., Smith, D. C., and Johnson, P. (2014). An integrated approach is needed for ecosystem based fisheries management: insights from ecosystem-level management strategy evaluation. PLoS One 9:e84242. doi: 10.1371/journal.pone.0084242
Gaichas, S., Gamble, R., Fogarty, M., Benoît, H., Essington, T., Fu, C., et al. (2012). Assembly rules for aggregate-species production models: simulations in support of management strategy evaluation. Mar. Ecol. Prog. Ser. 459, 275–292. doi: 10.3354/meps09650
Garcia, S. M., Kolding, J., Rice, J., Rochet, M.-J., Zhou, S., Arimoto, T., et al. (2012). Conservation. Reconsidering the consequences of selective fisheries. Science 335, 1045–1047. doi: 10.1126/science.1214594
Gjøsaeter, H., Bogstad, B., and Tjelmeland, S. (2009). Ecosystem effects of the three capelin stock collapses in the barents sea. Mar. Biol. Res. 5, 40–53. doi: 10.1080/17451000802454866
Gjøsaeter, H., Bogstad, B., Tjelmeland, S., and Subbey, S. (2015). A retrospective evaluation of the Barents Sea capelin management advice. Mar. Biol. Res. 11, 135–143. doi: 10.1080/17451000.2014.928414
Grimaldo, E., and Gjøsund, S. H. (2012). “Commercial exploitation of zooplankton in the Norwegian Sea,” in The Functioning of Ecosystems, ed. M. Ali, (Rijeka: IntechOpen).
Groeneveld, R. A., Bosello, F., Butenschön, M., Elliott, M., Peck, M. A., and Pinnegar, J. K. (2018). Defining scenarios of future vectors of change in marine life and associated economic sectors. Estuar. Coast. Shelf Sci. 201, 164–171. doi: 10.1016/j.ecss.2015.10.020
Halpern, B. S., Frazier, M., Potapenko, J., Casey, K. S., Koenig, K., Longo, C., et al. (2015). Spatial and temporal changes in cumulative human impacts on the world’s ocean. Nat. Commun. 6:7615. doi: 10.1038/ncomms8615
Hansen, C., Drinkwater, K. F., Jähkel, A., Fulton, E. A., Gorton, R., and Skern-Mauritzen, M. (2019). Sensitivity of the Norwegian and Barents Sea Atlantis end-to-end ecosystem model to parameter perturbations of key species. PLoS One 14:e0210419. doi: 10.1371/journal.pone.0210419
Hansen, C., Skern-Mauritzen, M., van der Meeren, G., Jähkel, A., and Drinkwater, K. (2016). Set-up of the Nordic and Barents Seas (NoBa) Atlantis Model. Available at: http://hdl.handle.net/11250/2408609 (accessed September 5, 2019).
Hidalgo, M., and Browman, H. I. (2019). Developing the knowledge base needed to sustainably manage mesopelagic resources. ICES J. Mar. Sci. 76, 609–615. doi: 10.1093/icesjms/fsz067
Hjøllo, S. S., Huse, G., Skogen, M. D., and Melle, W. (2012). Modelling secondary production in the Norwegian Sea with a fully coupled physical/primary production/individual-basedcalanus finmarchicusmodel system. Mar. Biol. Res. 8, 508–526. doi: 10.1080/17451000.2011.642805
Howell, D., Hansen, C., Bogstad, B., and Skern-Mauritzen, M. (2016). Balanced harvesting in a variable and uncertain world: a case study from the Barents Sea. ICES J. Mar. Sci. 73, 1623–1631. doi: 10.1093/icesjms/fsw034
ICES, (2018a). Publication Reports - Introduction_to_Advice_2018.pdf. Available at: http://www.ices.dk/sites/pub/Publication%20Reports/Forms/DispForm.aspx?ID=34640 (accessed May 30, 2019).
Kjesbu, O. S., Bogstad, B., Devine, J. A., Gjøsaeter, H., Howell, D., Ingvaldsen, R. B., et al. (2014). Synergies between climate and management for Atlantic cod fisheries at high latitudes. Proc. Natl. Acad. Sci. U.S.A. 111, 3478–3483. doi: 10.1073/pnas.1316342111
Kolding, J., Bundy, A., van Zwieten, P. A. M., and Plank, M. J. (2016). Fisheries, the inverted food pyramid. ICES J. Mar. Sci. 73, 1697–1713. doi: 10.1093/icesjms/fsv225
Lehuta, S., Girardin, R., Mahévas, S., Travers-Trolet, M., and Vermard, Y. (2016). Reconciling complex system models and fisheries advice: practical examples and leads. Aquat. Living Resour. 29:208. doi: 10.1051/alr/2016022
Link, J. S., Fulton, E. A., and Gamble, R. J. (2010). The Northeast US application of Atlantis: a full system model exploring marine ecosystem dynamics in a living marine resource management context. Prog. Oceanogr. 87, 214–234. doi: 10.1016/j.pocean.2010.09.020
Nilsen, I. (2018). Exploring Balanced Harvesting by Using an Atlantis Ecosystem Model for the Nordic and Barents Seas. Available at: http://bora.uib.no/handle/1956/18087 (accessed October 21, 2019).
Olsen, E., Kaplan, I. C., Ainsworth, C., Fay, G., Gaichas, S., Gamble, R., et al. (2018). Ocean futures under ocean acidification, marine protection, and changing fishing pressures explored using a worldwide suite of ecosystem models. Front. Mar. Sci. 5:64. doi: 10.3389/fmars.2018.00064
Pantus, F. J. (2006). Sensitivity Analysis for Complex Ecosystem Models. PhD Thesis, University of Queensland, Brisbane QLD.
Plagányi, ÉE. Food and Agriculture Organization of the United Nations [FAO], (2007). Models for an Ecosystem Approach to Fisheries. Rome: FAO.
Riahi, K., van Vuuren, D. P., Kriegler, E., Edmonds, J., O’Neill, B. C., Fujimori, S., et al. (2017). The shared socioeconomic pathways and their energy, land use, and greenhouse gas emissions implications: an overview. Glob. Environ. Change 42, 153–168.
Sandø, A. B., Melsom, A., and Budgell, W. P. (2014). Downscaling IPCC control run and future scenario with focus on the Barents Sea. Ocean Dyn. 64, 927–949. doi: 10.1007/s10236-014-0731-8
Sethi, S. A., Branch, T. A., and Watson, R. (2010). Global fishery development patterns are driven by profit but not trophic level. Proc. Natl. Acad. Sci. U.S.A. 107, 12163–12167. doi: 10.1073/pnas.1003236107
Shchepetkin, A. F., and McWilliams, J. C. (2005). The regional oceanic modeling system (ROMS): a split-explicit, free-surface, topography-following- coordinate oceanic model. Ocean Model. 9, 347–404. doi: 10.1016/j.ocemod.2004.08.002
Skern-Mauritzen, M., Ottersen, G., Handegard, N. O., Huse, G., Dingsør, G. E., Stenseth, N. C., et al. (2016). Ecosystem processes are rarely included in tactical fisheries management. Fish Fish. 17, 165–175. doi: 10.1111/faf.12111
Skogen, M. D., Budgell, W. P., and Rey, F. (2007). Interannual variability in Nordic Seas primary production. ICES J. Mar. Sci. 64, 889–898. doi: 10.1093/icesjms/fsm063
Skogen, M. D., Hjøllo, S. S., Sandø, A. B., Tjiputra, J., and Travers-Trolet, H. E. M. (2018). Future ecosystem changes in the Northeast Atlantic: a comparison between a global and a regional model system. ICES J. Mar. Sci. 75, 2355–2369. doi: 10.1093/icesjms/fsy088
Slagstad, D., Wassmann, P. F. J., and Ellingsen, I. (2015). Physical constrains and productivity in the future Arctic Ocean. Front. Mar. Sci. 2:85. doi: 10.3389/fmars.2015.00085
Smith, A. D. M., Brown, C. J., Bulman, C. M., Fulton, E. A., Johnson, P., Kaplan, I. C., et al. (2011). Impacts of fishing low-trophic level species on marine ecosystems. Science 333, 1147–1150. doi: 10.1126/science.1209395
Steinacher, M., Joos, F., Frölicher, T., Plattner, G.-K., and Doney, S. (2010). Projected 21st century decrease in marine productivity: a multi-model analysis. Biogeosciences 7, 979–1005. doi: 10.5194/bg-7-979-2010
United Nations, Department of Economic and Social Affairs, Population Division (2019). World Population Prospects 2019: Highlights (ST/ESA/SER.A/423).
Utne, K. R., Hjøllo, S. S., Huse, G., and Skogen, M. (2012). Estimating the consumption ofcalanus finmarchicusby planktivorous fish in the Norwegian Sea using a fully coupled 3D model system. Mar. Biol. Res. 8, 527–547. doi: 10.1080/17451000.2011.642804
Keywords: ecosystem based management, Atlantis end-to-end ecosystem model, climate change, fisheries management, socio-political pathways
Citation: Hansen C, Nash RDM, Drinkwater KF and Hjøllo SS (2019) Management Scenarios Under Climate Change – A Study of the Nordic and Barents Seas. Front. Mar. Sci. 6:668. doi: 10.3389/fmars.2019.00668
Received: 02 June 2019; Accepted: 14 October 2019;
Published: 30 October 2019.
Edited by:
Paul E. Renaud, Akvaplan-niva, NorwayReviewed by:
Nis Sand Jacobsen, National Marine Fisheries Service (NOAA), United StatesMarta Coll, Superior Council of Scientific Investigations, Spain
Vidette McGregor, National Institute of Water and Atmospheric Research (NIWA), New Zealand
Copyright © 2019 Hansen, Nash, Drinkwater and Hjøllo. This is an open-access article distributed under the terms of the Creative Commons Attribution License (CC BY). The use, distribution or reproduction in other forums is permitted, provided the original author(s) and the copyright owner(s) are credited and that the original publication in this journal is cited, in accordance with accepted academic practice. No use, distribution or reproduction is permitted which does not comply with these terms.
*Correspondence: Cecilie Hansen, Y2VjaWxpZS5oYW5zZW5AaGkubm8=