- 1Demersal Fish Research Group, Institute of Marine Research, Bergen, Norway
- 2Ecosystem Processes Research Group, Institute of Marine Research, Bergen, Norway
- 3Marine Processes and Human Impacts Research Program, Institute of Marine Research, Bergen, Norway
Mass mortality events (MMEs) are a key concern for the management of marine ecosystems. Specific stages and species are at risk and the causes may be single or cumulative pressure from a range of sources including pollutants, anthropogenic climate change or natural variability. Identifying risk and quantifying effects of plausible scenarios including MMEs are key to stakeholders and a quest for scientists. MMEs affect the whole ecosystem, but are traditionally only studied in relation to specific species, disregarding ecological feedbacks. Here we use an end-to-end ecosystem model adapted to the Nordic and Barents seas to evaluate the species-specific and ecological impacts for 50 years following an MME. MMEs were modeled as 10, 50, or 90% reduced recruitment for cod, herring and haddock, individually or in combination. The MME scenarios were compared to a base case model run that includes the current fishing mortality. All species showed declines in population biomass following an MME, increasing in duration and severity with increasing mortality. Cod biomass rebounded to the base case level within 3–13 years post the MME independent of scenario, while neither haddock nor herring fully rebounded to base case levels within the considered time horizon. Haddock responded much more variably to the mortality scenarios than cod or herring, with some scenarios yielding much higher levels of biomass than the base case. Herring responded negatively to all scenarios, leading to lower herring biomass and a steeper decline of the species than seen in the base case due to persistent harvest pressure. Corresponding responses showed that the demersal guild biomass increased substantially, while the pelagic guild biomass declined. Few effects were observed on the other guilds, including the top predators. Ecosystem effects as measured by ecological indicators were greatest after 5 years, but persisted through the entire model run. Fishery indicators showed the same features, but the responses were stronger than for the ecosystem indicators. Taken together this indicates long-term, ecological response to MMEs that can be described as regime shifts, highlighting the importance of using ecosystem models when evaluating effects of MMEs.
Introduction
Rare events with large negative consequences aka. ‘Black Swans’ (Taleb, 2011) occur in natural ecosystems, human societies and economies (Sornette, 2002). Black Swans are caused by events like earthquakes, storms, oil spills, or disease outbreaks and their consequences on ecosystems can include severe die-offs or population crashes (Anderson et al., 2017), called mass mortality events (MMEs). In fact, catastrophic MMEs affecting entire populations have been increasing in frequency during the last decades (Fey et al., 2015). Events that severely affect only parts of the life-history. are also considered as Black Swans and may cause MMEs with severe short- or long-term effects on the populations and ecosystems (species examples in Lawrence, 1996; López et al., 2008), similar to the MMEs (Langangen et al., 2017). In the face of climate change and increases in other human-induced external pressures the effects of such events may be exacerbated through cumulative impact (Fey et al., 2015).
The acute effects of ‘Black Swan’ events, are often dramatic and extensive but the long-term effects also give great cause for concern as exemplified by the 1989 Exxon Valdez oil spill in Alaska (Peterson, 2001). There is also concern that they may lead to regime shifts (Mollmann et al., 2014), altering the structure of the ecosystem on a long-term or even permanent basis.
Several marine fish populations show sporadic recruitment patterns with many years passing between successful year-classes contributing substantially to the long-term reproductive part of the population. Therefore, there is concern that mortality events in the early life stages may have severe and long-lasting effects on the population (Ohlberger and Langangen, 2015; Langangen et al., 2017). Most long-term effect studies have focused on single species, whereas the wider effects on the entire marine ecosystem have been neglected, mainly because of a lack of adequate tools for such complex integrated analyses.
Offshore petroleum extraction is the largest export industry in Norway, having boosted the country’s economy since 1969 when the first oil deposit was discovered in the North Sea. The environmental consequences, especially of acute spills caused by accidents, are at the heart of the ongoing debate over whether regions should be opened to oil and gas extraction (Olsen et al., 2016). Environmental risk analysis has been used to evaluate the effects of potential oil spill and large research projects have focused on understanding and quantifying these (Carroll et al., 2018), although with a focus on a few key species and their interactions. Quantifying the full ecological effects of such accidental spills has so far not been done for any of the Norwegian marine ecosystems.
Complex models combining the ecology and human pressures of marine ecosystems are now being developed for many major sea and ocean areas, allowing researchers to explore the ecological effects as well as the species-specific responses of perturbations and changing pressures on marine ecosystems. Such models have been used e.g., to specifically explore the ecosystem-based fisheries management strategies for fisheries in Australia (Fulton et al., 2014) and the United States West coast (Kaplan et al., 2012; Kaplan and Marshall, 2016), but also the integrated effects of a range of human activities on the Great Australian Bight in general (Fulton et al., 2018), and future fisheries, protection and ocean acidification scenarios across a range of global ecosystems (Olsen et al., 2018). Ecosystem models have been used to evaluate the ecological effects of the Deepwater Horizon oil spill in the Gulf of Mexico (Ainsworth et al., 2018). Ainsworth et al. (2018) used the end-to-end ecosystem model Atlantis to simulate impacts from the Deepwater Horizon oil spill on fish guilds within the Gulf of Mexico. By using Atlantis, Ainsworth et al. (2018) were able to discern that the biomass of fish within areas most affected by the oil spill decreased between 25 and 70%, loss of prey caused starvation amongst predators, and recovery of stocks took 10–30 + years.
In the present, study we utilize an Atlantis end-to-end ecosystem model (Fulton et al., 2011) for the Norwegian – Barents sea ecosystem (NoBa, Figure 1; Hansen et al., 2019a) to explore the species specific as well as the wider ecosystem effects of MMEs leading to reduced recruitment of key commercial fish species through increased mortality of fish eggs and larvae.
Materials and Methods
In the present study, we analyzed the ecosystem effects of MMEs on key fish species in the Norwegian and Barents sea: Northeast Arctic (NEA) Cod (Gadus morhua), Northeast Arctic haddock (Melanogrammus aeglefinus) and Norwegian Spring Spawning (NSS) Herring (Clupea harengus) all of which have major overlapping spawning grounds in the Lofoten – Vesterålen – Senja area or early life stage (ELS) drifting by Olsen et al. (2010) and Misund and Olsen (2013). The MMEs could be caused by a number of factors. However, we assumed that the pressure only occurred in a single year, which is plausible e.g., for the acute phase of an oil spill, but not for ocean acidification. That is, oil may be enclosed in ecosystem compartments such as sediments and result in releases also in subsequent years (Peterson 2001), but at lower levels than those that are needed to cause a MME. Hence, we did not consider pressures lasting multiple years, but only direct mortality effects occurring in a single year in the model. While the study was motivated by risk analysis considering oil spill impacts the results are valid independent of the cause of MMEs in fish ELS. This approach allowed us to evaluate the long-term effects over a 50-year post-MME modeling period, both on the species directly affected by added mortality, but also the indirect ecological effects through trophic interactions.
The Nordic and Barents Seas Atlantis model (NoBa) is a deterministic end-to-end ecosystem model parameterized for the Nordic and Barents seas (Figure 1). The Atlantis framework (Fulton et al., 2005, 2011) includes a variety of modules ranging from sunshine to fisheries, socio-economy and possibilities for management strategy evaluations. NoBa includes 53 species and functional groups (hereafter components), representing the ecosystems of the areas. The components are connected through a diet matrix, where a prey availability is defined. The prey availability represents the overlap between the prey and its predator, but as Atlantis is spatially resolved, they do need to be overlapping in time and space to have any interactions. The size of prey compared to the size of the predator is also taken into consideration. Important life history processes such as growth rates, consumption rates, recruitment and mortality rates were all defined based on published literature and verified to reproduce observations of biomass and population structures (Hansen et al., 2016). Atlantis also requires daily input of sea water temperature, salinity and currents. NoBa was forced by a Regional Ocean Modeling System (Shchepetkin and McWilliams, 2005) covering the Nordic and Barents seas. The forcing was received from three different set-ups of this system, covering three different time slots; 1981–2001, 2002–2005, and 2006–2068. By using three different setups for forcing, we introduced uncertainties tied to the differences in these. However, in this study, we only analyze output from the model driven by the last forcing. This specific setup is based on emission scenario RCP 4.5, which has been shown to only include a weak climate signal during this period (Skogen et al., 2018).
The simulated biomass and catches of pelagic (including Norwegian Spring Spawning herring, mackerel and blue whiting) and demersal guilds (including Greenland halibut, Northeast Arctic cod, haddock, beaked redfish and golden redfish) were comparable to observations (Hansen et al., 2019b). The biomass levels were in good agreement, the timing of peaks and troughs though fitted better for the demersal guild than for the pelagic guild. Moreover, the model was able to reproduce the ratio found by Hansen et al. (2019a) that the individual weights in each age class in over 70% of the components (including the three key species in this study) was within 50% change of the initial values, a measure often applied for tuning of Atlantis models.
Enhanced mortality on the eggs and larvae of ecologically important fish species in the Lofoten – Barents Sea ecosystem due to oil spill has been assessed over the past decade through numerous risk assessments and fate-modeling synthesized in Carroll et al. (2018). Although Atlantis is capable of explicitly simulating an oil spill (Ainsworth et al., 2018), the coarse spatial structure of the NoBa Atlantis model (Figure 1) is not conducive for realistic modeling of oil spill drift. Instead, we refer to published values of significant enhanced mortality on ELSs following large oil spills and initiated scenarios where recruitment to year 1 of NEA Cod, NEA Haddock and NSS Herring span out a range of single-year recruitment failures. Recruitment to age 1 occurs in all spatial boxes where a species occur, representing the distribution of ELS. Hence an MME event occurring in limited spatial area was assumed to affect the entire population. The 43% ELS reduction reported in Carroll et al. (2018) corresponding to 12% reduction in recruitment to the adult stock was the most severe outcome of scenarios investigated in that study. However, as stated above there is significant uncertainty when projecting additional ELS mortality onto changes in recruitment. Hence, as discussed by Langangen et al. (2017), depending on how the precautionary principle is implemented the 43% loss of ELS might also be interpreted as a larger loss of recruits. We therefore investigated losses of 10, 50, and 90% of recruits, both individually and cumulatively. The 90% scenario was chosen to include a worst case MME higher than the max levels established in the Norwegian planning context.
The three mortality levels were imposed on each of the species in 12 different model runs, either by applying the added mortality to one, or to all three species simultaneously (Table 1). We also ran a base-case simulation without adding any mortality to serve as comparison for the MME scenarios. The mortality was applied in the model by first running a base case with no MME. Thereafter, the mortality to the first cohort of the species was applied by multiplying the number of that specific cohort by a scalar (0.9, 0.5, 0.1), e.g., by reducing the numbers of the specific cohort by 10, 50, or 90%. This was only the case for the single year where we assumed that the MME would happen. The simulated years after the MME was performed without any forcing. All runs included active fisheries, following historical fishing pressures until 2017, thereafter applying Fmsy levels (fishing pressures achieving maximum sustainable yield) for all commercial stocks (Hansen et al., 2019b).
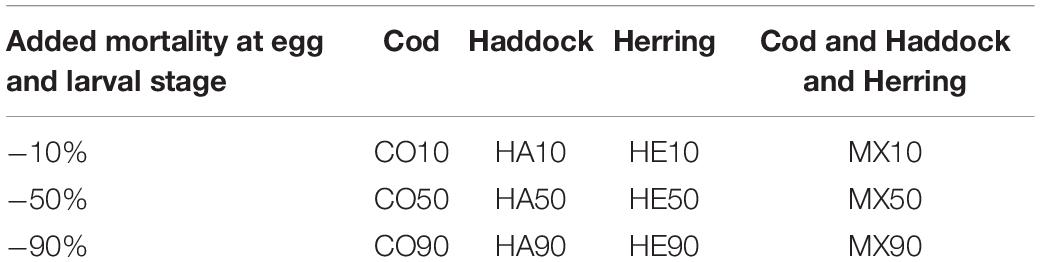
Table 1. Scenarios of reduced survival at the egg and larval stages of Northeast Arctic cod (Gadus morhua), Northeast Arctic haddock (Melanogrammus aeglefinus), Norwegian spring spawning herring (Clupea harengus), evaluated using the Norwegian – Barents Sea Atlantis model.
Each model run was 110 years with 24 years for spin-up followed by 36 year reflecting the 1982–2017 historical period before any perturbation was introduced. The spin-up period was forced by looping the physical forcing from ROMS representing 1981 24 times. The mortality was introduced in one time-step in January of year 62 (2018). The exact timing of the MME during a year would have little effect on model outputs due to the MME being modeled as a reduction of the incoming cohort, not a disruption of a spawning event. In the years after the event mortality was not adjusted as we assume the acute effects of the MME to be transient and out of the system well before the next annual recruitment event. This might not be true if there are additional environmental changes at spawning grounds that may affect future spawning. However, we currently do not have information indicating that this may be the case.
The effects of the 12 scenarios (Table 1) were evaluated at three levels: (1) the direct responses on an index of biomass (dividing all biomass values by 250 000) and age structure of the species affected in each scenario and the indirect effect on the other two of the three focus species, (2) the indirect effects on other ecosystem components as evaluated by functional guilds (Supplementary Table S1), and (3) the effects on ecosystem structure and emergent properties as measured through previously vetted indicators of ecosystem and fisheries status (Supplementary Table S2; Fulton et al., 2005; Olsen et al., 2018). The guild responses and ecosystem indicators were evaluated as averages of the first 5, 10, and 20 years post the MME.
Model and Code for Analysis
The scenarios were run with Atlantis version 6205. Model outputs were analyzed in R studio version 3.5.2. Scripts used to generate plots and the raw model output data are available upon request.
Results
Total Biomass
All scenarios for reduced recruitment (reduced survival of fish eggs and larvae) had clear effects on the total stock biomass of the species directly affected (Figure 2), as well as on all species included in the NoBa model (Supplementary Figure S1). Although the focal species of the present analysis has been cod, haddock and herring, the other species included in the NoBa Atlantis model also reacted to the MMEs indirectly through food-web interactions. In general, the magnitudes of their reactions were lower than for the study species (Supplementary Figure S1), with some notable exceptions like capelin, beaked redfish, killer whale, squid, large and medium zooplankton (Calanus finmarchicus) that all showed responses exceeding 10% for some of the scenarios.
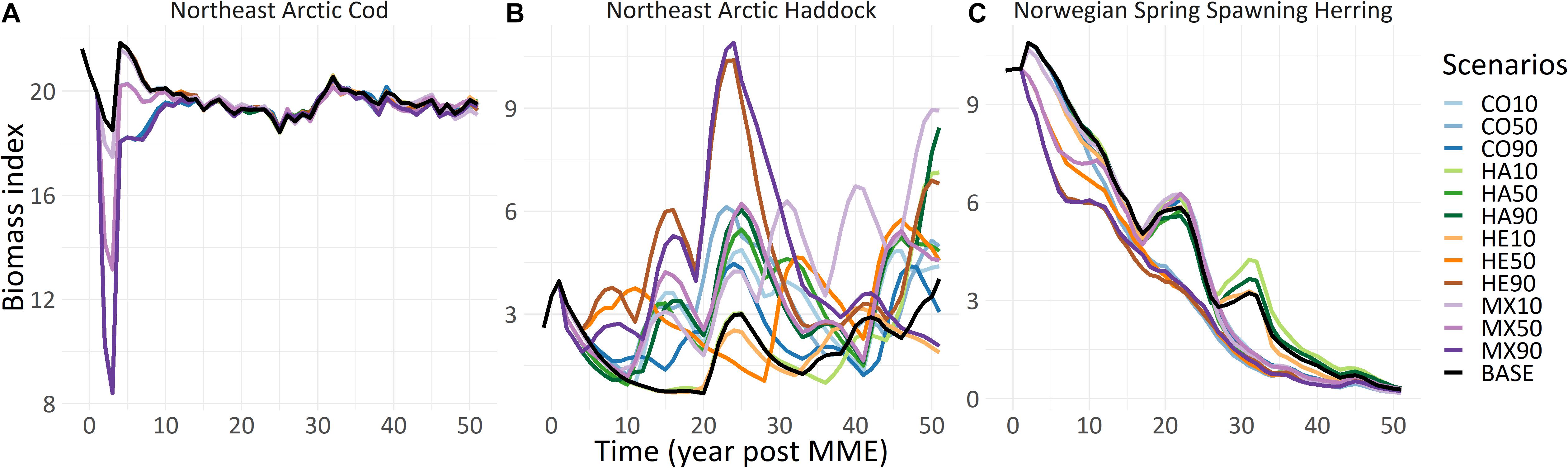
Figure 2. Changes in total biomass index for (A) Northeast Arctic cod (Gadus morhua), (B) Northeast Arctic haddock (Melanogrammus aeglefinus), and (C) Norwegian spring spawning herring (Clupea harengus) under twelve scenarios for reduced egg and larval survival (see Table 1) affecting one species at a time, or three species combined, compared to the baseline scenarios modeled using the NoBa Atlantis ecosystem model. Run “BASE” is the baseline, the same as the reduced survival scenarios up until year 0 of the simulation when the mortality event takes place.
There was an immediate drop in biomass for all three focus fish species, largest for cod and smaller for the haddock and herring. Cod rebounded fairly quickly from the mortality event, in 3 years for the CO10 scenario, 8 years for CO50 and 13 years for CO90, with cod total biomass stabilizing at base case levels (Figure 2A). None of the scenarios for haddock or herring had any discernible effect on the total biomass of cod (Figure 2A) as all lines overlapped completely with the base case.
The loss of juvenile fish due to a MME could affect dietary responses across the ecosystem, such as shifting predation pressures due to the loss of important sources of food or promoting the growth of other juvenile groups due to the reduction in competition for resources. The NOBA Atlantis model uses nitrogen to track the flow of nutrients through the marine ecosystem, so the condition of age-structured functional groups can be tracked through the energy allocation to structural (e.g., hard tissue; bone) and reserve (e.g., soft tissue; fat) nitrogen. To explore changes in organismal condition following a MME, we considered changes in residual nitrogen relative to the residual nitrogen just before a MME (RNt/RN0; where t is the annual time step) and structural nitrogen relative to the structural nitrogen just before a MME (SNt/SN0). Nitrogen ratios of cod were about (∼1.6) for all scenarios, both for residual and structural Nt/N0 (Supplementary Figures S2, S3), indicating increased individual growth and fewer individuals toward the end of the model run compared to the time of the MME.
The impacts of reduced recruitment to haddock had very different effects on the total biomass than for cod (Figure 2B). The HA90 and HA50 scenarios both led to immediate and discernable drops in biomass, although the relative changes from the base case and between scenarios were much lower than for cod. Interestingly, the HA10 scenario showed no immediate drop in biomass as compared to the base case, only starting to diverge from the base case 18 years after the MME, and staying below the base case until 33 post the MME, after which it fluctuated above and below the base case. Eight years past the MME both the HA90 and HA50 scenarios had rebounded to the base case level, and thereafter followed the base case trajectory for 10–18 years, when biomass became higher than the base case for the next 10–15 years. 10–28 years past the MME the HA50 and HA90 scenarios showed variable performance compared to the base case, but at the end of the model run both HA50 and HA90 showed biomass levels above the base case. Thus, the haddock scenarios were much more chaotic than the cod scenarios, possibly reflecting the naturally more stochastic recruitment events and stock fluctuations in this stock compared to the cod (Olsen et al., 2010). The haddock also showed clear responses to reduced recruitment in the other two species (Figure 2B), with all herring and cod scenarios leading to an increased haddock stock above the baseline for at least 20 years past the mortality event. Haddock nitrogen ratios at the end of the model run were lower than one for both residual and structural nitrogen for all scenarios except HA90 and MX90, indicating a reduced individual growth toward the end of the modeling period compared to the time of the MME (Supplementary Figures S2, S3).
The modeled herring population steadily declined under the present level of recruitment and harvest control rules (HCR), with or without a mortality event. Added mortality exacerbated this decline. There were however slightly higher levels of herring for some of the cod scenarios compared to the base case due to reduced predation by cod (see Figure 2C). The HE10 scenario showed no immediate drop in total biomass level and this scenario followed the baseline only with minor variability through the whole model run. In contrast, both the HE50 and HE90 showed an immediate drop in total biomass index relative to the baseline. Both the HE50 and HE90 scenarios had lower total herring biomass for the entire run, compared to the base case, but none had completely merged with the base case. Herring responded only slightly to reduced recruitment for the other two species, and only from 9 years after the MME. The exception was the CO50 scenario that led to lower total biomass of herring from nine years past the MME and onward. From 22 years after the MME the CO50 scenario showed the same levels of total biomass as the HE50 and HE90 scenarios. After 30 years the other non-herring scenarios diverged from the base case. The HA10 and HA90 scenarios led to slightly higher herring biomass while the CO10 and CO90 cod and HA50 haddock led to reduced total biomass compared to the base case for the rest of the run. Herring also had nitrogen ratios below 1 for both structural and residual nitrogen for all scenarios except HE90 and MX90, indicating a reduced individual growth toward the end of the modeling period compared to the time of the MME (Supplementary Figures S2, S3).
For cod the combined scenarios followed the same trajectory as the species-specific scenarios for the entire model run (Figure 2A). For herring most scenarios lead to generally lower biomasses depending on the imposed mortality, with a similar impact of both species-specific or combined MME scenarios. For haddock the response to the combined scenarios was more variable, although at the start all three showed declines comparable to the haddock species-specific scenarios (Figure 2B). Most haddock scenarios lead to distinctly higher biomasses, without any clear trend toward rebound to the base case.
Changes in Age Structure
Since all vertebrate components in the Atlantis models are represented in an age-structured way we could evaluate the changes to each specific age group under each scenario. In Figure 3, the age structure is shown for all combined scenarios: MX10, MX50, MX90. From Figure 3, a clear ripple-effect showing how an initial decline in recruits due to the mortality event could be followed as a decline in one-year-olds after one year, two-year-olds after two years and so on (see Figures 3A,B,H,I for the clearest examples).
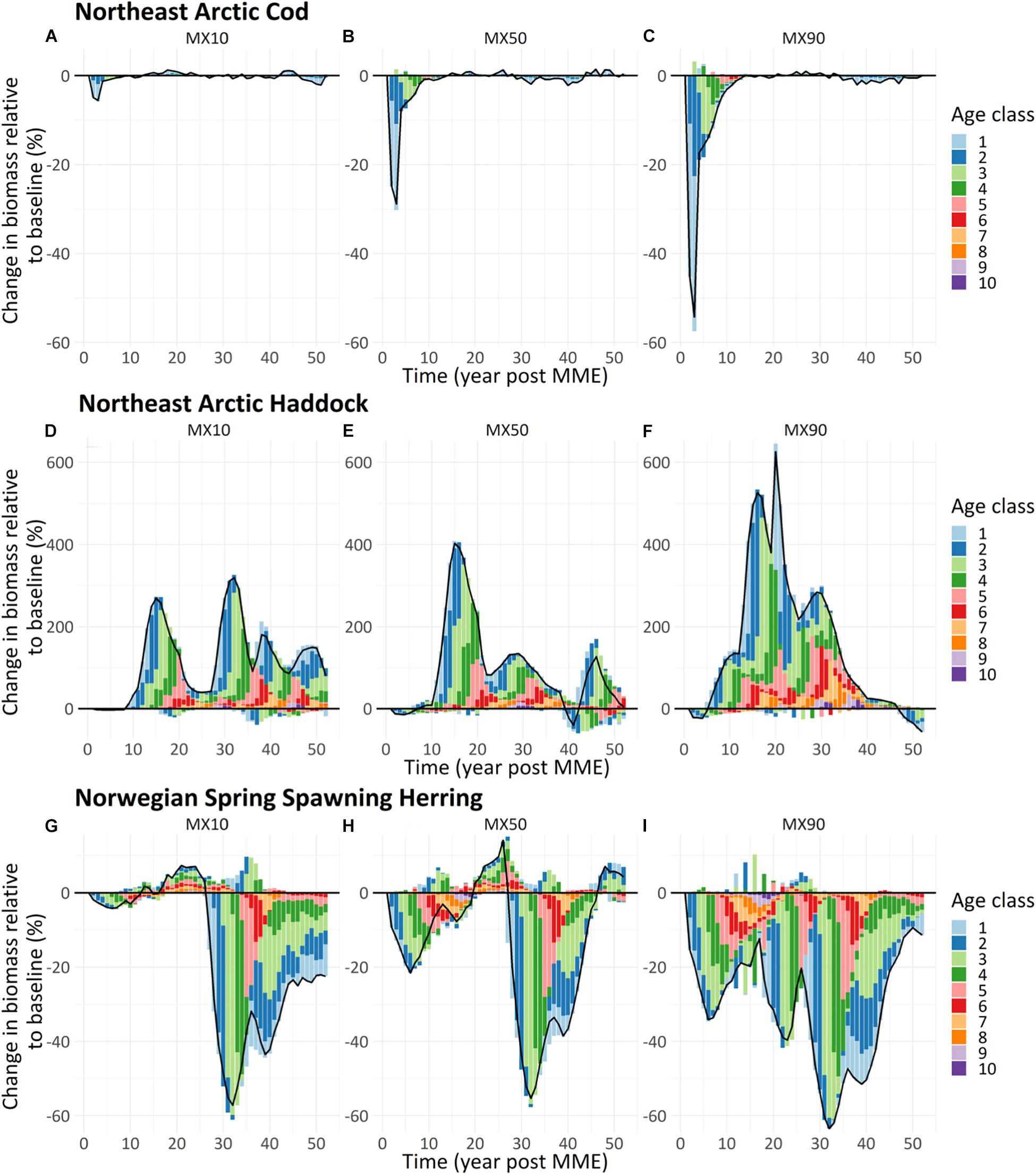
Figure 3. Change in age structure biomass (% relative to baseline run) of the modeled age classes (1–10) for (A–C) Northeast Arctic cod (Gadus morhua), (D–F) Northeast Arctic haddock (Melanogrammus aeglefinus), and (G–I) Norwegian spring spawning herring (Clupea harengus) following three reduced mortality events (Panels: A: –10%, B: –50%, C: –90%) modeled using the NoBa Atlantis ecosystem model. The black line shows the change in total biomass of the population. Year 0 is the year of the mortality event.
The increasingly negative effect when reducing recruitment was clearly seen for all three species with the cod rebounding to levels close to the base case 3–13 years post the mortality event (Figures 3A–C). For haddock the initial decline was much smaller, and there was no rebound – rather, the stock levels increase, although with large variability especially for the MX10 scenario (Figures 3D–F). For herring there was more variability around the base case, especially for the MX10 scenario that showed increasing fluctuations toward the end of the run (Figure 3G), while the MX50 and MX90 herring scenarios showed a steady decline compared to the base case (Figures 3H,I).
Ecological Responses
Trophic Guild Responses
We observed clear effects of the MME on the demersal fish and pelagic fish guilds, but varying with scenario and time after the MME. Only the effects of the most severe scenarios (CO90, HA90, HE90, MX10, MX50 and MX90) are shown in Figures 4A–C below, the trophic guild responses of the remaining six scenarios are shown in Supplementary Figure S4. Average guild effects were small (<5%) for the first 5 year period past the MME event (Figure 4 and Supplementary Figure S5). They increased over time for the demersal fish guild, while the average responses of the pelagic fish guild remained comparable for 5, 10, and 20 years past the MME. The average guild responses for the demersal and pelagic guilds were driven by increased responses in one species, while the other guild species remained at the base case, or in some cases even showed decreased responses. Responses of individual species increased for all guilds over time, and after 10 years one could start noticing effects on other guilds than those directly affected by the MME.
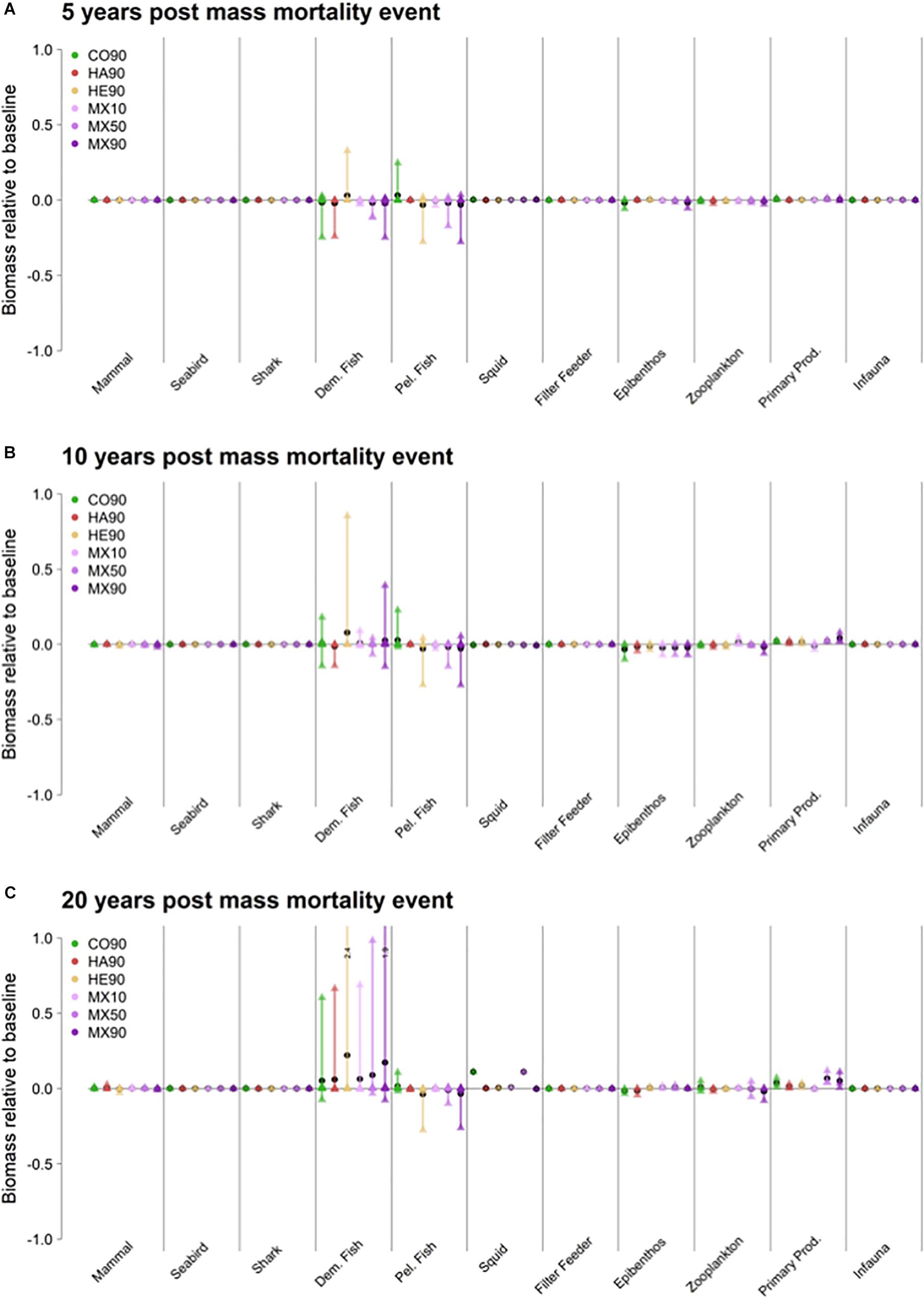
Figure 4. Averages of the responses of the 11 trophic guilds to mass mortality events reducing the survival of fish egg and larvae of Northeast Arctic cod (Gadus morhua), Northeast Arctic haddock (Melanogrammus aeglefinus) and Norwegian Spring Spawning herring (Clupea harengus) over the first 5 (A), 10 (B) and 20 years (C) post the mass mortality event (oil spill) modeled using the NoBa Atlantis ecosystem model. The colored bars cover the range of responses from minimum to maximum, the black circles are the mean response within a guild, while the triangles show the response of individual species (or species groups).
Some very minor effects could be seen on the epibenthos (food for demersal fish), and zooplankton (food for pelagic fish) for the 5-year period past the MME event, but these effects increased by 10 and 20 years. By year 20 there were some positive effects on squid for the CO90 and MX50 scenarios, possibly linked to the large increase in biomass of prey from the demersal guild.
The average effects on the demersal and pelagic guilds were slightly negative for all the combined scenarios (Figure 4A) in the first 5 years past the mortality event, except for the demersal fish guild which responded slightly positively to the HE90 scenario and the pelagic fish guild which responded positively to the CO90 scenario. For each scenario there was a spread in responses (triangles within the colored bars of Figure 4A) with only a single species showing a marked response (in either direction) while the rest remained at or close to the base case.
After 10 years (Figure 4B), the demersal fish guild still showed a positive response under the HE90 scenario, but now there was a slight positive response in the MX10 scenario. The average response for the CO90, MX50 and MX90 scenarios were the same as the base case, but for these three scenarios some species responded positively while others responded negatively.
In the pelagic guild the average guild and individual species responses were similar to the situation 5 years earlier. Epibenthos responded slightly negatively to all scenarios, while the primary production responded slightly positively to all scenarios.
Over the 20-year period past the MME event (Figure 4C), the demersal fish guild had responded positively under all scenarios, driven by a very strong positive response in haddock. In the demersal guild there were some slight negative responses of single species for the CO90, MX50 and MX90 scenarios although the average response was positive. The pelagic guild again showed a similar response as 5 and 10 years past the MME, although the extent of single species responses had been slightly dampened. Epibenthos showed less responses than after 10 years, while primary production was slightly higher for all scenarios. Zooplankton responses were also stronger than after 10 years, we saw increased biomass of squid for the CO90 and MX50 scenarios. It is also interesting to note the small average increase (<5%) in the primary producer guild.
Ecosystem and Fishery Indicators
There were stronger responses in the fishery indicators than the ecological indicators to the MME event at all three-periods (5, 10, and 20 years) past the event (Figures 5A–C and Supplementary Figure S6). Response levels for the ecological indicators were minor (<5%), while the fishery indicators showed some stronger responses (up to 25%, for the 5 year period past the MME). The response of the ecological indicators changed over time, with the strongest responses appearing in the 20 year period past the MME event (Figures 5A–C). However, yearly responses varied much more (Supplementary Figure S6), with the largest yearly deviations from the base case occurring more than 20 years after the MME. Contrary, the effects on the fishery indicators were dampened after the MME and lowest over the 20 year period post the MME. Still, even 20 years past the MME event there were up to 6% negative effects on some of the fisheries indicators Some of the fisheries indicators (Total Catch, Value of catch, Demersal catch) showed a similar pattern to the ecological indicators with increased deviation from the base case (with large variability between the scenarios) during the period from year 20 to the end of the model run (Supplementary Figure S6). These changes in both ecological and fisheries indicators can mainly be linked to the large changes in the haddock population during this period.
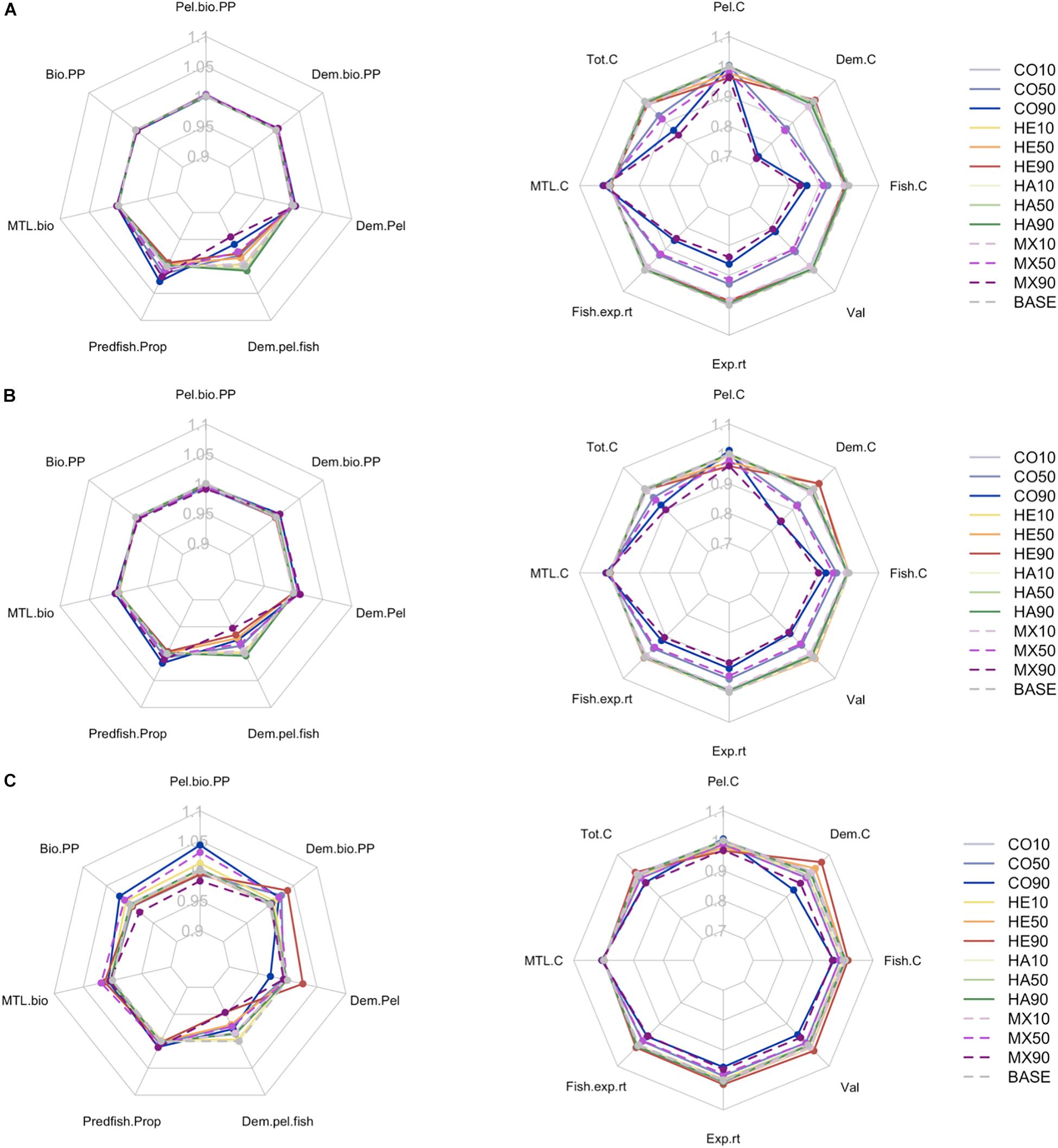
Figure 5. Averages of the ecological (left) and fisheries (right) indicators within the years following a mass mortality event. (A) 5 years post the mortality event, (B) 10 years post the mortality event, (C) 20 years post the mortality event. Displayed values are relative to the baseline run and are based on interannual snapshots of the indicator values. Pel.bio.PP: Pelagic biomass as ratio of primary production, Dem.bio.PP: Demersal biomass as ratio of primary production, Dem.Pel: Demersal – Pelagic biomass ratio, Dem.pel.fish: Demersal – Pelagic fish biomass ratio, Predfish.Prop: Proportion of predatory fish, MTL.bio: mean trophic level of biomass, Bio.pp: total biomass as ratio of primary production, Pel.C: catch of pelagics, Dem.C: catch of demersals, Fish.C: catch of fish, Val: value of catch, Exp.rt: exploitation rate of all, Fish.exp.rt: exploitation rate of fish, MTL.C: mean trophic level of catch, Tot.C: total catch (see Supplementary Table S2 for details).
The ecological indicator response was very similar for the 5 and 10 year periods past the MME (Figures 5A,B), with only the demersal – pelagic fish ratio (Dem.pel.fish) decreasing more than 2% compared to the base case in the CO90, HE50, HE90 and MX 90 scenarios. Five years past the MME the proportion of predatory fish increased above the base case for the CO90, MX90 and MX50 scenarios, while the demersal fish ratio decreased below the base case for the MX90, CO90, MX50, HE90, HE50 scenarios. This pattern remained 10 years past the MME, although less intense responses were observed for the CO90 and MX90 scenarios. Then, over the 20 year period past the MME the pattern changed dramatically, with all ecological indicators, except the proportion of predatory fish, showing divergences >1% for one or more of the scenarios (Figure 5C). Now the pelagic biomass – primary production ratio (Pel.bio.pp) showed the greatest variability around the base case with the CO90 scenario exceeding the base case by 4.3% while the MX90 was 2.1% below the base case. Similar diverging patterns of responses were seen for both the Bio.pp, Dem.pel and Dem.pel.fish indicators. It was also interesting to note that the various scenarios had different indicator responses. Whereas the CO90 scenario had the lowest response for the Dem.pel indicator, 3% below the base case, as noted above it exceeded the base for the Pel.bio.pp indicator.
All fisheries indicators, except the mean trophic level of the catch (MTL.C, Figures 5A–C) showed responses diverging >1% for one or more of the scenarios. Although the strongest responses were seen over the first 5 years past the MME, and then decreasing 10 and 20 years past the MME, the fisheries indicators never rebounded completely to the base case level for any scenario. Even 20 years after the MME the fisheries indicators of the CO90 and MX 90 scenarios were below the base case level (except for MTL.C).
Discussion
By using a vetted Atlantis end-to-end ecosystem-model of the Norwegian and Barents Sea (Hansen et al., 2019a) we were able to simulate the ecosystem effects of increased mortalities in fish egg and larvae of cod, haddock and herring. All species show immediate declines in biomass following the mortality event, from low, but measurable effects at 10% added mortality, increasing with the severity of the event, and highest for the 90% mortality scenarios affecting cod (CO90), or all three species (MX90). This immediate post-event decline was expected, both based on our understanding of species life cycles as implemented in the NoBA model, as well as on previous modeling studies (Ohlberger and Langangen, 2015; Langangen et al., 2017; Carroll et al., 2018). However, we see that the effects on cod persisted for longer than the previous studies have indicated, caused widely fluctuating biomass of haddock, and accelerated the population decline of herring (Figure 2). In the NoBa model, cod had a very limited response to increased mortality for the haddock or herring, while both herring and haddock (to the greatest degree) showed strong responses to changes in the mortality of the other two species. This clearly shows the importance of including species interactions when studying the effect of perturbations to ecologically important species. It also indicates differences in resilience between the three species to MMEs, with the cod population being resilient, returning to biomass levels similar to the base case 13 years after the mortality event. Herring and haddock showed no similar tendency to resilience, rather the haddock population showed a very fluctuating population biomass for all mortality scenarios, while the herring biomass under the mortality scenarios were consistently under the base case biomass for the entire model run. Our results correspond to the results of the sensitivity analysis of a different NoBa Atlantis model implementation (Hansen et al., 2019a), which showed that top predators such as whales and seabirds are less impacted by changes in other species, as they are generalists and therefore are able to switch prey if a prey group decreases while species that are at the center of the trophic web, in particular herring, are much more vulnerable to changes at both their own level, but also at other components.
Cod experienced an immediate decline in overall biomass, and we observed a clear ripple-effect of reduced survival of the affected year-classes (Figure 3) that varied with the intensity of the mortality. Although the immediate drop in biomass was compensated and the stock biomass returned to base case levels in 10–15 years, showing stability and indicating low internal variability of the cod stock. This was in stark contrast to the haddock which exhibited signs of large internal variability (i.e., sensitivity to initial conditions) making it a potential significant factor in the simulated biomass trajectory. The immediate decline compared to the base case ranged from ∼5% for the MX10 (and CO10) scenario to ∼55% for the MX90 scenario. The effect on year-classes subsided with time, but at age 3, when cod mature and start entering the fishery (Durant et al., 2008) they were ∼5% below the base case for the MX50 scenario and ∼12% below in the MX90 scenario. A decline of 5–12% of year-class 3 of cod translates to 9 570–22 969 tonnes, based on the 2018 NEA cod stock assessment (ICES, 2018). For the fishery the effects of a MME first become noticeable after the year class enters the fishery. Three years past the MME, the effect on cod would be marginal as few of the 3-year olds are caught in the fishery. However, the effect will increase as the fish grow and become more available to the fishery, and the affected year class becomes a more substantial part of the catch. We see clear effects on the demersal fishery (which is dominated by cod) of the scenarios including 50% or 90% mortality of cod (CO50, CO90, MX50 and MX90) when evaluating the fishery indicators (Figure 5) throughout the model run, although at the end (20 years past the event) the effects are less than 5% compared to the base case. Immediately after the event (5 years) we observed a reduction in demersal catch of up to 25% leading to a 10–20% reduction in the value of the catch for the 50 and 90% mortality scenarios. Such effects are substantial and dramatic for the fishing industry in Norway and Russia that dominate the demersal fisheries in the Barents Sea.
Since cod, haddock and herring are key species in the Norwegian Sea and Barents Sea ecosystems, it is no surprise that changes in the biomass of these species have strong effects on the total biomass of the demersal and pelagic fish guilds that they are part of Figure 4. In particular the increases in the demersal fish guild after 10 and 20 years was driven by the large increases in the haddock population caused by very good recruitment events at three to four periods after the MME (depending on the scenarios – see Figure 3B). Such sporadically good recruitment events are a characteristic of the Northeast Arctic haddock stock (Fogarty et al., 2001; Olsen et al., 2010), indicating that the NoBa model gives a realistic representation of the haddock recruitment potential. A sporadic recruitment potential also implies that the species reproduction is more opportunistic than species with a more stable recruitment, being able to take advantage optimal physical conditions or opportunities with more prey that may arise from perturbations to the system (even pulse perturbations as in the present analysis), potentially caused by a MME. It may be hypothesized that this is a more general characteristic of sporadic recruiting species – that they are better able to take advantage of perturbations than less opportunistic species. However, at the guild level the effects of the MMEs were most discernable at the 50 and 90% mortality levels, indicating a dampening effect of perturbations stemming from varying effect of different guild members, and increased resilience of ecological guilds comparable to what has previously been observed for similar ecosystem modeling studies (Olsen et al., 2018). Even so, the ecological effects that were observed continued for the entire model run, and for several of the guilds increased 10–20 years after the mortality event. The changes in guild biomass indicate changes in ecosystem structure, which was clearly seen in the ecosystem and fishery indicators (Figure 5).
Taken together the effects on species biomass, ecological guilds, ecosystem and fishery indicators point to complex ecological effects of MMEs, effects that may be temporary blips for some species, but that may lead to long-lasting or even permanent changes in the absolute population size and trophic role. The ecosystem indicators also point to long-term secondary ecological effects impacting guilds not directly affected by the event, but that are prey or predators of the species affected. Indicators of ecosystem state and fisheries outputs thus give insight into how interactions in the ecosystem respond to perturbations to single or combined components of the system. Here they indicate long-term and measurable changes in the dynamics of the ecosystem. The ecosystem becomes more dominated by the demersal fish guild under all mortality scenarios compared to the unperturbed base case run. Such changes are persistent alterations of the structure of the ecosystem, also apparent in the species trajectories of all species in the NoBa model (Supplementary Figure S1), and thus can be classified as regime shifts according to Mollmann et al. (2014).
Neither the marine mammal, seabird nor shark guilds showed any response to any of the mass mortality scenarios at any time step (Figure 4). These three guilds are the top-predators of the system and find much of their food in the pelagic guild, and to a less extent the demersal fish guild. It is therefore a bit surprising that there is no feedback effect on these guilds from changes in the biomass of their prey. Because the changes in the pelagic and demersal guilds mainly affected cod, haddock and herring, while the top predators remained at base case level the most probable explanation is that the top predators in the model changed their feeding on other members of their prey guilds when the biomass of their preferred prey changed. When the recruitment of NE Arctic cod experienced an MME, the predation upon cod reduced amongst all of the primary predators (Supplementary Figure S7A). However, there were not any other changes in predation from these predators (Supplementary Figure S7B). This was most likely because NE Arctic cod was not a key prey item for any of the indicated predators. A similar situation could be observed for NE Arctic haddock (Supplementary Figures S8A,B). When the recruitment of herring experienced an MME, decreases in predation on herring was observed immediately for boreal seabirds and after 25 years for killer whales (Supplementary Figure S9A). However, the direct impact from the MME appears to have little influence on the predation of these key predators on other prey groups (Supplementary Figure S9B).
For the historical period, NoBa showed reasonable biomasses and development for both the pelagic and demersal guilds, comparable to Hansen et al. (2019b) runs (Supplementary Figure S10). For the demersal guild, the correlation between observed and modeled biomass was particularly high (r = 0.89), whereas the correlation between observed and modeled pelagic biomass was not as high (r = 0.47). However, it needs to be kept in mind that these comparisons are not completely independent, as the harvest pressure for the historical period unquestionably had an impact on the development of the stocks/guilds. Although the model proved to be within reasonable bounds for these guilds, there are still large intrinsic uncertainties in complex end-to-end models (Lehuta et al., 2016; Hansen et al., 2019a).
Conclusion
MMEs on early life stages of fish have strong negative responses in the short term. In our modeled system some species rebounded, and some even to higher levels than before. Systemic effects could be traced in the ecosystem for the entire model run which lasted 50 years post the event, indicating that such events perturb the system into a new state. In the present scenarios this state is one where demersal fish species dominate more than in the base case without the mortality event. The main driver for the demersal dominance were the several successful recruitment events of haddock leading to large increases in this population, indicating that the sporadically recruiting haddock was better able to take benefit from the perturbations to the ecosystem caused by the MME. It may be hypothesized that the ability to take advantage of ecosystem perturbations is a characteristic shared by many marine teleost species with sporadic recruitment pattern.
Our model analysis supports the view that cod as a species is resilient to MMEs, but found a longer impact duration than that of Durant et al. (2008) and Ohlberger and Langangen (2015), and more similar to those found by Ainsworth et al. (2018) in the Gulf of Mexico. Our modeled impacts are stronger than those modeled for oil spills in the Great Australian Bight (Fulton et al., 2018), but then again, our scenarios of 50% and 90% reduction in recruitment were higher than what was predicted in the Australian oil spill scenario. Also, we found significant impact on the fishery following a 50% recruitment reduction, which is a lower level than that explored by Ohlberger and Langangen (2015). Our scenario of 50% reduced recruitment was chosen based on the worst case scenarios of 43% simulated by Carroll et al. (2018), and similar levels modeled by Ohlberger and Langangen (2015), that in turn lead to <3–12% decreases in adult cod (spawning stock) biomass (Figures 2, 3).
Our motivation for initiating this study was the ongoing discussion of the ecological effects of major oil spills on marine ecosystems, one potential cause of a MME. Oil spill environmental impact assessments are an integral part of the Norwegian Integrated management plan for the Lofoten – Barents sea areas (BSMP, Olsen et al., 2007), but the magnitudes of the spills assessed are debated. In the 2011 revision of the BSMP a maximum spill rate of 4500 m3/day was used (Hauge et al., 2014), which is low compared to oil spills like the Deepwater Horizon with a spill rate up to 8332 m3/day (McNutt et al., 2012). Limiting the analysis to 4500 m3/day would potentially underestimate the worst-case oil spill for the region. Concerned by the critique of the maximum spill size (Hauge et al., 2014), we therefore chose to include higher mortality levels (90%) than those used in the risk analysis of the management plan, and by Carroll et al. (2018) in their integrated modeling study.
Previous studies have limited their scope to a single species, i.e., cod, which seems to be more resilient to oil than its sibling species haddock (Sørensen et al., 2017). Adding to this the different life histories and recruitment patterns (Olsen et al., 2010) for cod, herring and haddock leads to overlooking species-specific and ecological responses that become apparent when investigating the effects of MMEs using end-to-end ecosystem models. One would have expected all species to respond like cod, to have an immediate effect of the MME, but then return to the base case level in a few years, and remain stable for the rest of the model run. Our model system did not behave like this neither for haddock, nor herring, and this is a very important result to be aware of when evaluating the consequences of human activities that can lead to MMEs, e.g., petroleum activities. Still, our results only show potential effects of an MME event given the limitations of the structure and parameterization of our Atlantis model. Thus, our results need to be checked with other models, as well as empirical and analytical studies, to verify the potential effects we have uncovered. Even so, under the precautionary principle, potential long-term ecosystem effects such as these we have discovered should be included in the considerations taken by managers of the Norwegian and Barents Sea ecosystems.
Understanding the effects of MMEs, be it on entire populations or the recruitment to the population, is vitally important in order to predict the possible changes to species and ecosystems from external perturbations ranging from pollution events such as off-shore oil spills, climate change leading to increased temperature and increased ocean acidification, or epidemics. In the Norwegian – Barents sea ecosystem the potential effects of oil spills are at present the most important to management as it is at the heart of the ongoing political discussions of what areas should be opened to the petroleum industry (Misund and Olsen, 2013). However, in the longer term (50–100 years) climate change impacts such as ocean acidification (Olsen et al., 2018) and temperature increases may overshadow the effects of direct anthropogenic activities. This makes our present ecological modeling approach of direct relevance to the ongoing and future management of the Norwegian and Barents Sea ecosystem.
Data Availability Statement
The datasets generated for this study are available on request to the corresponding author.
Author Contributions
EO, CH, HP, and FV conceived the idea for this study and defined the scenarios. CH, IN, and HP carried out the modeling of the scenarios and produced the results. All authors contributed to the analysis and writing of the manuscript.
Funding
This work was carried out as part and funded by the Institute of Marine Research Strategic Project ‘Reduced Uncertainty in Stock Assessment’ (2016–2020), project number 3680_14809.
Conflict of Interest
The authors declare that the research was conducted in the absence of any commercial or financial relationships that could be construed as a potential conflict of interest.
Supplementary Material
The Supplementary Material for this article can be found online at: https://www.frontiersin.org/articles/10.3389/fmars.2019.00669/full#supplementary-material
References
Ainsworth, C. H., Paris, C. B., Perlin, N., Dornberger, L. N., Patterson, W. F., III, Chancellor, E., et al. (2018). Impacts of the deepwater horizon oil spill evaluated using an end-to-end ecosystem model. PLoS One 13:e0190840. doi: 10.1371/journal.pone.0190840
Anderson, S. C., Branch, T. A., Cooper, A. B., and Dulvy, N. K. (2017). Black-swan events in animal populations. Proc. Natl. Acad. Sci. U.S. 114, 3252–3257. doi: 10.1073/pnas.1611525114
Carroll, J., Vikebø, F., Howell, D., Broch, O. J., Nepstad, R., Augustine, S., et al. (2018). Assessing impacts of simulated oil spills on the Northeast Arctic cod fishery. Mar. Pollut. Bull. 126, 63–73. doi: 10.1016/j.marpolbul.2017.10.069
Durant, J. M., Hjermann, D. O., Sabarros, P. S., and Stenseth, N. C. (2008). Northeast Arctic cod population persistence in the lofoten-barents sea system under fishing. Ecol. Appl. 18, 662–669. doi: 10.1890/07-0960.1
Fey, S. B., Siepielski, A. M., Nusslé, S., Cervantes-Yoshida, K., Hwan, J. L., Huber, E. R., et al. (2015). Recent shifts in the occurrence, cause, and magnitude of animal mass mortality events. Proc. Natl. Acad. Sci. U.S. 112, 1083–1088. doi: 10.1073/pnas.1414894112
Fogarty, M. J., Myers, R. A., and Bowen, K. G. (2001). Recruitment of cod and haddock in the North Atlantic: a comparative analysis. ICES J. Mar. Sci. 58, 952–961. doi: 10.1006/jmsc.2001.1108
Fulton, E., Smith, A., and Punt, A. (2005). Which ecological indicators can robustly detect effects of fishing? ICES J. Mar. Sci. 62, 540–551. doi: 10.1016/j.icesjms.2004.12.012
Fulton, E. A., Bulman, C. M., Pethybridge, H., and Goldsworthy, S. D. (2018). Modelling the great australian bight ecosystem. Deep Sea Res. Part II 157, 211–235. doi: 10.1016/j.dsr2.2018.11.002
Fulton, E. A., Link, J. S., Kaplan, I. C., Savina-Rolland, M., Johnson, P., Ainsworth, C., et al. (2011). Lessons in modelling and management of marine ecosystems: the Atlantis experience. Fish Fish. 12, 171–188. doi: 10.1111/j.1467-2979.2011.00412.x
Fulton, E. A., Smith, A. D. M., Smith, D. C., and Johnson, P. (2014). An integrated approach is needed for ecosystem based fisheries management: insights from ecosystem-level management strategy evaluation. PLoS One 9:e84242. doi: 10.1371/journal.pone.0084242
Hansen, C., Drinkwater, K. F., Jähkel, A., Fulton, E. A., Gorton, R., and Skern-Mauritzen, M. (2019a). Sensitivity of the norwegian and barents sea atlantis end-to-end ecosystem model to parameter perturbations of key species. PLoS One 14:e0210419. doi: 10.1371/journal.pone.0210419
Hansen, C., Nash, R., Drinkwater, K. F., and Hjøllo, S. S. (2019b). Management scenarios under climate change – a study of the nordic and barents seas. Front. Mar. Sci. 6:668. doi: 10.3389/fmars.2019.00668
Hansen, C., Skern-Mauritzen, M., van der Meeren, G., Jähkel, A., and Drinkwater, K. (2016). Set-Up of the Nordic and Barents Seas (NoBa) Atlantis Model. Available at: http://hdl.handle.net/11250/2408609 (accessed February 14, 2017).
Hauge, K. H., Blanchard, A., Andersen, G., Boland, R., Grøsvik, B. E., Howell, D., et al. (2014). Inadequate risk assessments – a study on worst-case scenarios related to petroleum exploitation in the Lofoten area. Mar. Policy 44, 82–89. doi: 10.1016/j.marpol.2013.07.008
Kaplan, I. C., Horne, P. J., and Levin, P. S. (2012). Screening California current fishery management scenarios using the Atlantis end-to-end ecosystem model. Prog. Oceanogr. 102, 5–18. doi: 10.1016/j.pocean.2012.03.009
Kaplan, I. C., and Marshall, K. N. (2016). A guinea pig’s tale: learning to review end-to-end marine ecosystem models for management applications. ICES J. Mar. Sci. 73, 1715–1724. doi: 10.1093/icesjms/fsw047
Langangen, Ø., Ohlberger, J., Stige, L. C., Durant, J. M., Ravagnan, E., Stenseth, N. C., et al. (2017). Cascading effects of mass mortality events in arctic marine communities. Glob. Change Biol. 23, 283–292. doi: 10.1111/gcb.13344
Lawrence, J. M. (1996). Mass mortality of echinoderms from abiotic factors. Echinoderm Stud. 5, 103–137.
Lehuta, S., Girardin, R., Mahévas, S., Travers-Trolet, M., and Vermard, Y. (2016). Reconciling complex system models and fisheries advice: practical examples and leads. Aquat. Living Resour. 29:208. doi: 10.1051/alr/2016022
López, R. A., Penchaszadeh, P. E., and Marcomini, S. C. (2008). Storm-Related strandings of mollusks on the northeast coast of buenos aires, argentina. J. Coast. Res. 24, 925–935. doi: 10.2112/05-0621.1
McNutt, M. K., Camilli, R., Crone, T. J., Guthrie, G. D., Hsieh, P. A., Ryerson, T. B., et al. (2012). Review of flow rate estimates of the deepwater horizon oil spill. Proc. Natl. Acad. Sci. U.S.A. 109, 20260–20267. doi: 10.1073/pnas.1112139108
Misund, O. A., and Olsen, E. (2013). Lofoten-vesteralen: for cod and cod fisheries, but not for oil? ICES J. Mar. Sci. 70, 722–725. doi: 10.1093/icesjms/fst086
Mollmann, C., Folke, C., Edwards, M., and Conversi, A. (2014). Marine regime shifts around the globe: theory, drivers and impacts. Philos. Trans. R. Soc. B Biol. Sci. 370:20130260. doi: 10.1098/rstb.2013.0260
Ohlberger, J., and Langangen, Ø. (2015). Population resilience to catastrophic mortality events during early life stages. Ecol. Appl. 25, 1348–1356. doi: 10.1890/14-1534.1
Olsen, E., Aanes, S., Mehl, S., Holst, J. C., Aglen, A., and Gjosaeter, H. (2010). Cod, haddock, saithe, herring, and capelin in the barents sea and adjacent waters: a review of the biological value of the area. ICES J. Mar. Sci. 67, 87–101. doi: 10.1093/icesjms/fsp229
Olsen, E., Gjøsaeter, H., Røttingen, I., Dommasnes, A., Fossum, P., and Sandberg, P. (2007). The norwegian ecosystem-based management plan for the barents sea. ICES J. Mar. Sci. 64, 599–602. doi: 10.1093/icesjms/fsm005
Olsen, E., Holen, S., Hoel, A. H., Buhl-Mortensen, L., and Røttingen, I. (2016). How integrated ocean governance in the barents sea was created by a drive for increased oil production. Mar. Policy 71, 293–300. doi: 10.1016/j.marpol.2015.12.005
Olsen, E., Kaplan, I. C., Ainsworth, C., Fay, G., Gaichas, S., Gamble, R., et al. (2018). Ocean futures under ocean acidification, marine protection, and changing fishing pressures explored using a worldwide suite of ecosystem models. Front. Mar. Sci. 5:64. doi: 10.3389/fmars.2018.00064
Peterson, C. H. (2001). The “Exxon Valdez” oil spill in alaska: acute, indirect and chronic effects on the ecosystem. Adv. Mar. Biol. 39, 1–103. doi: 10.1016/s0065-2881(01)39008-9
Shchepetkin, A. F., and McWilliams, J. C. (2005). The regional oceanic modeling system (ROMS): a split-explicit, free-surface, topography-following-coordinate oceanic model. Ocean Model. 9, 347–404.
Skogen, M. D., Hjøllo, S. S., Sandø, A. B., Tjiputra, J., and Travers-Trolet, H. E. M. (2018). Future ecosystem changes in the Northeast Atlantic: a comparison between a global and a regional model system. ICES J. Mar. Sci. 75, 2355–2369. doi: 10.1093/icesjms/fsy088
Sørensen, L., Sørhus, E., Nordtug, T., Incardona, J. P., Linbo, T. L., Giovanetti, L., et al. (2017). Oil droplet fouling and differential toxicokinetics of polycyclic aromatic hydrocarbons in embryos of atlantic haddock and cod. PLoS One 12:e0180048. doi: 10.1371/journal.pone.0180048
Sornette, D. (2002). Predictability of catastrophic events: material rupture, earthquakes, turbulence, financial crashes, and human birth. Proc. Natl. Acad. Sci. U.S.A. 99 (Suppl. 1), 2522–2529. doi: 10.1073/pnas.022581999
Keywords: mass mortality events, ecosystem model, Barents sea, Norwegian sea, oil spill, cod, haddock, herring
Citation: Olsen E, Hansen C, Nilsen I, Perryman H and Vikebø F (2019) Ecological Effects and Ecosystem Shifts Caused by Mass Mortality Events on Early Life Stages of Fish. Front. Mar. Sci. 6:669. doi: 10.3389/fmars.2019.00669
Received: 07 June 2019; Accepted: 14 October 2019;
Published: 29 October 2019.
Edited by:
Jeffrey William Krause, Dauphin Island Sea Lab, United StatesReviewed by:
Donald F. Boesch, University of Maryland Center for Environmental Science (UMCES), United StatesMoritz Mathis, Helmholtz-Zentrum Geesthacht, Germany
Copyright © 2019 Olsen, Hansen, Nilsen, Perryman and Vikebø. This is an open-access article distributed under the terms of the Creative Commons Attribution License (CC BY). The use, distribution or reproduction in other forums is permitted, provided the original author(s) and the copyright owner(s) are credited and that the original publication in this journal is cited, in accordance with accepted academic practice. No use, distribution or reproduction is permitted which does not comply with these terms.
*Correspondence: Erik Olsen, ZXJpa29AaGkubm8=