Molecular Level Analysis Reveals Changes in Chemical Composition of Dissolved Organic Matter From South Texas Rivers After High Flow Events
- Marine Science Institute, The University of Texas at Austin, Port Aransas, TX, United States
Riverine dissolved organic matter (DOM) is a major source of reduced carbon exported from land to marine environments, and the inflow of riverine organic matter greatly affects biogeochemical cycling in estuaries and bays. Thus, any change in DOM composition, such as changes caused by flood waters as a result of storms and hurricanes, can subsequently affect estuarine environments. To investigate the impact of high flow events on riverine DOM, multidimensional molecular level information on DOM from four South Texas Rivers (Aransas, Lavaca, Mission, and Nueces Rivers) was acquired using high-resolution Ion Mobility Quadrupole Time of Flight Liquid Chromatography Mass Spectrometry (IM Q-TOF LCMS). Base-flow samples were collected in May, July, and October of 2016, June of 2017, and March of 2018, while high-flow samples were collected in September of 2017, as well as June and September of 2018. Based on the molecular formulas assigned from IM Q-TOF LCMS, the H/C ratio decreased during high-flow events (1.52 to 1.51 in ESI+ and 1.19 to 1.07 in ESI−), while the O/C ratio increased (0.31 to 0.33 in ESI−). Furthermore, DOM shifted from a protein-like and lipid-like dominated community at base flow conditions, to a lignin, tannin and condensed aromatic structure dominated community during high flow events, based on MS and tandem MS data. These changes in high-flow riverine DOM indicate an increase of terrestrial signal, which is likely a result of mobilization of terrestrial organic matter from the watersheds by flooding. The mobilized DOM, though refractory at high-flow conditions in rivers, could be reactive in coastal regions when conditions change, and thus could potentially fuel microbial activities downstream. In addition, about 3.76–21.8% of DOM molecules contain structural isomers among different flow conditions. This low number of isomer percentages suggests that DOM, as the products of various enzymatic biochemical reactions, is constrained in the number of isomers. Taken together, results from our study provide insights into structural changes of riverine DOM in response to extreme climate events in subtropical regions and have implications in understanding biogeochemical changes in estuaries under a changing climate.
Introduction
Dissolved organic matter (DOM) represents a major pool of reduced carbon (C) and nitrogen (N) in aquatic systems. With ca. 6.6 × 1017 g of C, oceanic dissolved organic carbon (DOC) is the second largest bioreactive pool of C (Carlson and Hansell, 2015), and plays an important role in global carbon cycling (Hedges, 2002; Jiao et al., 2011; Ridgwell and Arndt, 2015). The steady state in age and concentration of oceanic DOM is dependent on riverine input (Raymond and Spencer, 2015). Even though the origin of oceanic DOM is still under debate, the flux of riverine DOM from land to ocean is nevertheless a major source of reduced carbon to marine environments (Meybeck, 1993; Hedges et al., 1997; Raymond and Bauer, 2001). Globally, rivers transport a total amount of roughly 0.2 × 1017 g of C to the ocean annually (Meybeck, 1993; Ludwig et al., 1996; Cauwet, 2002; Seitzinger et al., 2005; Dai et al., 2012), with the top 30 largest rivers in terms of discharge contributing to over 51% of the total global flux (Raymond and Spencer, 2015).
The quantity and composition of DOM exported to coastal and oceanic waters are affected by hydrologic events, as river discharge could increase several hundred folds during storm events. The strong positive correlation between DOC export and river discharge has been well documented (e.g., Buffam et al., 2001; McGlynn and McDonnell, 2003; Inamdar et al., 2004; Hood et al., 2006; Dalzell et al., 2007; Vidon et al., 2008). For example, during an extreme flooding event caused by Hurricane Matthew in 2016, the Yadkin-Pee Dee River basin alone could export over 474 million kg of DOC (Majidzadeh et al., 2017); and a single two-to-three-day storm event could account for ca. 31% of the DOC flux in an Ontario headwater stream in Canada (Hinton et al., 1997). A meta-analysis of 30 small eastern United States forested watersheds showed a power-law relationship between DOC concentration and discharges, with 86% of DOC exported during hydrologic events (Raymond and Saiers, 2010). Also acknowledged is that the increase in stream water DOM concentration during high-flow events is generally concurrent with a change in the DOM composition (Gremm and Kaplan, 1998; Hood et al., 2006; Zhang et al., 2007; Spencer et al., 2008). This shift is a result of mobilization of a different terrestrial DOM pool, which is not available under base flow scenarios (Vidon et al., 2008; Fellman et al., 2009). However, a quick search on Web of Science1 with the key words of (“dissolved organic matter” OR “DOM”) AND (“composition”) AND (“flood” OR “storm”) returns a total number of 77 publications, suggesting that data are still scarce in evaluating how the chemical composition of riverine DOM changes during high-flow events.
A better understanding of how the riverine DOM community changes during high-flow events is needed under a changing global climate, as increasing frequency of strong tropical cyclones was predicted based on high resolution models, along with an increase of 20% in the precipitation rate within 100 km of the storm center (Knutson et al., 2010). Particularly, the frequency of category 4 and 5 storms in Atlantic Ocean was projected to double by the end of 21st century (Knutson et al., 2008; Bender et al., 2010). In 2017 alone, a total of six major hurricanes (category >3) were formed in Atlantic Ocean, marking the highest number since 2005 and twice of the averaged major hurricane number in the past decade (2006–2016). Besides the direct impact on the landfall area (e.g., Han et al., 2018), high-flow events after hurricane could subsequently change the hydrology and water quality in subtropical regions (e.g., Nesbit and Mitsch, 2018). Characterized by a semiarid climate and sporadic rainfalls, South Texas is known for very intense precipitation events cropping up within relatively short period of time (Bomar, 1983, 2017; Mooney and McClelland, 2012; Bruesewitz et al., 2013; Reyna et al., 2017). For instance, Hurricane Harvey, a category four major hurricane that made landfall near University of Texas Marine Science Institute (UTMSI) on August 25, 2017, caused unprecedented flooding in nearby areas. The USGS monitor station at Mission River (28°17′30′′N, 97°16′44′′W; 0.31 m above sea level) showed that the discharge experienced an over-4000-fold increase within 72 h of the landfall. Similarly, the storm event in June of 2018 caused an over-7000-fold increase in river discharge within a week. These episodic flood events make the region of South Texas an ideal study area to investigate how riverine DOM community reacts to high-flow events in tropical and subtropical regions.
The chemical composition of DOM in riverine systems, represented by its molecular formula composition, functional groups, geometric configuration of DOM molecules, as well as its isomeric composition, strongly affects its fate, and role in aquatic environments. Due to the inherent complexity of DOM composition, previous studies were mostly focused on measuring the bulk concentration and fluxes of DOM (e.g., Raymond and Saiers, 2010), with only a few paying attention to how the chemical composition of DOM changes during the storm (Fellman et al., 2009). The riverine DOM can be characterized by parameters, including bulk C:N ratio, stable (δ13C, δ15N; Cai et al., 2013) and radioactive isotopes (Δ14C; Evans et al., 2007), optical properties (e.g., specific UV absorbance, SUVA254; Fellman et al., 2009; Majidzadeh et al., 2017; Osburn et al., 2019), as well as specific compounds and biomarkers (e.g., amino acids, carbohydrates, and lignins; Ward et al., 2012). High-resolution and multi-dimension molecular level analytical techniques of riverine DOM have only recently become available (Cauwet, 2002; Raymond and Spencer, 2015), and have not been widely applied to study how DOM composition reacts to high-flow events. Previous work has implied that isomeric information on DOM is crucial in understanding its chemical composition diversity (Zark et al., 2017; Wagner et al., 2019) and its reactivity. However, even with ultra-high-resolution mass spectrometry, isomeric information is often not accessible easily (Zark et al., 2017; Zark and Dittmar, 2018). To the best of our knowledge, a direct measurement of the isomers on riverine DOM across a temporal range has not yet been conducted.
The objective of this work is to investigate the impact of high-flow events on the composition of riverine DOM via molecular level analysis. Specifically, we aim to elucidate the change of DOM composition, including its molecular formulas, geometric configurations (i.e., the 3D structure), as well as isomeric information (i.e., the number of isomers), in response to high-flow events using a combination of liquid chromatography, ion mobility, and high-resolution quadrupole time-of-flight mass spectrometry (IM Q-TOF LCMS).
Materials and Methods
Sample Collection
Water samples were collected from four South Texas rivers in the lower-river region but without saltwater influence (Aransas River, AR: N 28.13°, W 97.43°; Lavaca River, LR: N 28.83°, W 96.58°; Mission River, MR: N 28.29°, W 97.28°; and Nueces River, NR: N 27.88°, W 97.63°; Figure 1). Gage and discharge data from the nearest USGS stations were used as a proxy to indicate the flow condition of each river. From 2016 to 2018, the median discharge rate of four rivers ranged from 0.06 to 43.25 feet3 s–1. In this study a high-flow event was defined as at least 10 times of the median discharge. The exception is NR, where the discharge is mainly affected by the water dam. Therefore, the flow condition in NR was determined based on weather and flow conditions of other rivers, given the rivers in this work share a very close drainage area. Base-flow samples were collected on May 27th, July 19th and October 7th of 2016, June 19th of 2017, and March 21st of 2018, and high-flow samples were collected on September 28th of 2017, and June 21st and September 19th of 2018 (Supplementary Figure S1). High-flow samples were all collected, either during a flooding event or within 2 days after the peak discharge/gage height.
Water samples were collected with a Van Dorn sampler and transferred to acid-washed 1-L polycarbonate bottles in a cooler with ice. Salinity, pH, dissolved oxygen (concentration and saturation level), and temperature were measured in situ at each site using a YSI Sonde. Upon returning to lab the same day, 1 L samples were filtered through 0.2 μm pore size Nylon membrane filters (WhatmanTM 47 mm 0.2 μm membrane filter; Catalog No 7402-004) or filter cartridges (WhatmanTM Polycap AS 75 Capsule filter, 0.2 μm; Catalog No 2706T) to remove suspended particles. Ca. 30 mL of the filtered water was preserved at −20°C until further DOC and amino acids analysis. DOM was isolated from the filtered water and prepared for LC/MS analysis by solid-phase extraction (SPE) using a PPL cartridge (Agilent Bond Elut PPL cartridge, P/N 12105006), following the established protocol (Dittmar et al., 2008). DOM extracts were eluted with four-cartridge volume of methanol and were re-concentrated to a final volume of 1 mL and stored at −20°C until further analysis. The extracts were diluted 10-fold with methanol prior to analysis. The carbon-based recovery rate of DOM extraction, calculated as DOC of extract/DOC of filtered water × 100%, ranged from 31 to 44% on a carbon basis. All solvents and chemicals were LC/MS grade (acquired from Fisher or Sigma-Aldrich).
DOC and Amino Acids Analysis
Bulk DOC concentration was measured with a total organic carbon/total dissolved nitrogen analyzer (Shimadzu TOC-V/TDM-1). The precision of DOC measurement between duplicate samples agreed within 6%, and the accuracy ranged from 102 to 111% compared with a 10 ppm DOC standard. Total dissolved amino acids (TDAA) were analyzed using high performance liquid chromatography (HPLC) with fluorescence detection. Briefly, the filtered water was hydrolyzed using 6 M HCl under nitrogen gas at 110°C for 20 h (Kuznetsova and Lee, 2002). Then, TDAA, including dissolved free and combined amino acids, in the water were measured using HPLC after pre-column o-phthaldialdehyde derivatization (Lee et al., 2000; Liu et al., 2013). The relative standard deviations of TDAA in replicate samples were within 10%.
Molecular Level Characterization of Riverine DOM
Molecular level characterization of riverine DOM was performed on an Agilent 6560 Ion Mobility Quadrupole Time of Flight Liquid Chromatography Mass Spectrometer (IM Q-TOF LC/MS). Electrospray ionization negative (ESI−) mode has been commonly applied in the molecular characterization of complex DOM mixtures (e.g., Kujawinski, 2002; Kim et al., 2003; Wagner et al., 2019), which primarily contain molecules with carboxyl groups that can be ionized efficiently in negative mode. However, the presence of high carboxyl-content aromatic compounds, especially in terrestrial DOM (Kramer et al., 2012), can lead to the suppressed ionization of aliphatic, and carbohydrate-like DOM (Ohno et al., 2016). Therefore, both ESI− and ESI+ modes were applied in this work, aiming at providing a more comprehensive picture of riverine DOM.
The instrument and data acquisition methods followed our previous study (Lu et al., 2018). Briefly, for ESI+ mode, mobile phase A was H2O with 0.1% (v/v) formic acid, and B was acetonitrile. One-μL of each sample was eluted through a StableBond C18 column (Poroshell 120 SB-C18; 2.7 μm, 2.1 × 100 mm; Agilent P/N 685775-902) at a flow rate of 0.5 mL⋅min–1. During the 21-min run, mobile phase B was increased from 3 to 90% during the first 15 min, and held at 90% from 15 to 20 min, and then dropped to 3% from 20 to 21 min. A post-run time of 4 min allowed the column to reach equilibrium. For ESI− mode, mobile phase A was H2O with 10 mM ammonium acetate, and mobile phase B was acetonitrile. One-μL of samples was eluted through a HILIC column (2.7 μm, 15 cm × 4.6 mm SUPELCO, P/N 53981-U) at a flow rate of 0.5 mL⋅min–1. During the 10-min run, mobile phase B was held at 98% during the first 1 min, and dropped to 95% from 1 to 10 min. A post-run period of 15 min allowed the column to reach equilibrium before the injection of next sample.
Mass spectrum (MS) data, non-targeted tandem MS (MS/MS) and ion mobility-mass spectrum (IM-MS) data were acquired with software MassHunter LC/MS Data Acquisition (Version B.07.00) in both ESI− and ESI+ modes. The estimated resolving power (calculated as t/Δt, in which t is drift time and Δt is the peak width measured in milliseconds at half height) of ion mobility exceeds 60 at drift time of 30 ms. The TOF MS alone has resolving power greater than 40 000 at m/z of 1200, while the whole instrument IM Q-TOF LC/MS can resolve up to 2 000 000 at m/z of 300. Samples were run in duplication. The acquisition parameters followed the published protocol (Lu et al., 2018). Briefly, the MS acquisition rate was 4 spectra/s. The collision gas for MS/MS was He. The MS/MS acquisition rate was 2 spectra/s, and acquisition time was 500 ms/spectrum. The resolution for the precursor ion was maintained at 40 000 at m/z of 1200, with a precursor window of ±2 m/z. The number of precursor ions in the precursor window was constrained by a preferred ID list created based on the MS results. Collision energy for ESI+ mode was 30 V, with a threshold to trigger MS/MS of at least 2000 counts for the precursor ion. Collision energy for ESI− mode was 40 V, with a threshold to trigger MS/MS of at least 6000 counts for the precursor ion.
Data analysis also followed the published protocol (Lu et al., 2018). Briefly, MS data and MS/MS data were analyzed with MassHunter Qualitative Analysis (Version B.07.00, Service Pack 2), and IM-MS data was analyzed with MassHunter IM-MS Browser (Version B.07.01). Detected peaks were filtered based on signal-to-noise (S/N) ratios of ≥8 for ESI+ mode and of ≥5 for ESI− mode. The elements allowed in formulas in this work were C, H, N, O, and S. +H and +Na were allowed in positive data, while −H, +HCOO, and +CH3COO were allowed in negative data. A neutral loss of H2O was enabled in both modes. The mass inaccuracy tolerance was set at ≤1.5 ppm. Formulas of DOM were then calculated and assigned with the following rules (Kujawinski and Behn, 2006; Stubbins et al., 2010; Liu et al., 2011; Hertkorn et al., 2013; Lu et al., 2018): (1) Double Bond Equivalent (DBE) = 1 + 1/2(2C-H + N) ≥ 0; (2) O:C ≤ 1; (3) N ≤ 4 and N:C ≤ 1; (4) 0.333 ≤ H/C ≤ 2.25; (5) Nitrogen Rule; (6) S ≤ 2; and (7) Correct isotopic spacing and abundance. On average, 1818 peaks per sample were detected, and 1006 peaks were assigned with a formula under ESI+. Under ESI−, 748 peaks per sample were detected, and 488 were assigned with a formula. Riverine DOM was further divided into eight sub-classes based on its element stoichiometries (Kim et al., 2003; Ohno and Ohno, 2013; Mangal et al., 2016, 2017): (1) Lipid-like structures with elements ratios of: 0.01 ≤ O/C ≤ 0.1; 1.5 ≤ H/C ≤ 2.0; (2) Unsaturated hydrocarbon-like structures: 0.01 ≤ O/C ≤ 0.1; 0.75 ≤ H/C < 1.5; (3) Condensed aromatic structures: 0.01 ≤ O/C ≤ 0.65; 0.25 ≤ H/C < 0.75; (4) Protein-like structures: 0.1 < O/C ≤ 0.65; 1.5 ≤ H/C ≤ 2.3; N ≥ 1; (5) Lignin-like structures: 0.1 < O/C ≤ 0.65; 0.75 ≤ H/C < 1.5; (6) Tannin-like structures: 0.65 < O/C ≤ 0.85; 0.75 ≤ H/C ≤ 1.5; (7) Carbohydrate-like structures: 0.65 < O/C ≤ 1.0; 1.5 < H/C ≤ 2.5; and (8) Others: those do not fall into any other category. Based on the Aromaticity Index (AI; Koch and Dittmar, 2006, 2016), which can be calculated from the number of C, H, O, N, and S in the molecular formula, the DOM can also be categorized into non-aromatic (AI < 0.5), aromatic (0.5 ≤ AI < 0.67), and condensed aromatic structures (AI ≥ 0.67). It has been demonstrated that ionization efficiencies among different molecules and compound classes are not equal (Oss et al., 2010), which means that the peak area of a specific molecular formula obtained from IM Q-TOF LCMS does not accurately represent its actual molecular concentration (the same is applied to all other ESI-MS). Therefore, in this work, the percentage of each compound group was calculated using the number of formulas, rather than peak area, following a previous study (e.g., Mangal et al., 2016).
Features obtained through non-targeted MS/MS were assigned with possible structures by comparing the product ion spectra with a standalone version of METLIN Metabolites database (Smith et al., 2005). MS/MS data were further examined in detail using Molecular Structure Correlator, which provided fragmentation information by comparing results with ChemSpider and METLIN Metabolites databases. Note that this assignment can only be used as a guide, not an identification, as there is no database for natural DOM and further evidence (e.g., direct comparison with a standard) is required for true identification. However, the product ion spectra of a DOM molecule can provide information on its fragmentation pathway and functional groups during the collision induced dissociation (CID) process.
IM-MS provides insights into structural isomers of DOM molecules and thus structural diversity (Lu et al., 2018; Leyva et al., 2019), which adds another level of information on the top of accurate molecular formulas. The IM-MS spectra were calibrated for mass and ion mobility measurements using the Agilent Tuning Mix mass calibration standard (Tune Mix, G2421A, Agilent Technologies, Santa Clara, CA, United States). Features with same m/z but different LC retention time (RT) and/or different collision cross section (CCS) are characterized as isomers following our previous study (Lu et al., 2018). Given the current resolving power (ca. 60; Lu et al., 2018), this drift time ion mobility instrument is able to differentiate isomers with difference in CCS as low as 2% (Nichols et al., 2018). Note that a majority of molecules can be lost in the drift tube during the IM mode due to interactions with nitrogen gas (Lu et al., 2018).
For statistical analyses, t-test and χ2 test were conducted using Excel (version 2016) and principal component analysis (PCA) was conducted using R Studio (version 1.1) with package “factoextra.” A significant level of 0.05 (i.e., p < 0.05 was considered significant) was used throughout this work.
Results
Changes in Hydrological Parameters in High Flow Events
Salinities at sampling sites were <1%, with the exception of NR and LR sites (Supplementary Table S1). Salinity at these two sites can reach over 8‰ occasionally due to the presence of a water dam and/or the high evaporation rate in South Texas. Dissolved oxygen (DO) concentrations and saturation percentages were high at all sampling sites at base flow (DO > 5 mg⋅L–1; DO% > 70%; Supplementary Table S1). However, after high-flow events (2017 September, 2018 June and September), both DO concentration and saturation percentage decreased significantly (p = 0.03 for DO%; p = 0.04 for DO). The averaged DO concentration during base-flow conditions was 6.4 mg⋅L–1 with an averaged saturation of ca. 83.1%, while after high-flow events, DO dropped to 4.4 mg⋅L–1 with an averaged saturation of 55.6%.
Concentrations of DOC and TDAA at Different Flow Conditions
Dissolved organic carbon concentrations in the four rivers ranged from 316 to 1089 μmol C L–1, and 328 to 1008 μmol C L–1 at base-flow and high-flow conditions, respectively, with MR varying the most (Supplementary Table S2). Taking all four rivers together, the DOC concentration at base flow was ca. 628 μmol C L–1, and ca. 653 μmol C L–1 after high-flow events, on average. However, no statistical difference was detected between different sampling times at base-flow and at high-flow (two-sample t-test; p = 0.76).
Total dissolved amino acids concentrations ranged from 2.23 to 25.5 μmol⋅L–1 and 1.00 to 37.0 μmol⋅L–1 at base-flow and high-flow conditions, respectively (Supplementary Table S2). TDAA at high-flow conditions was more variable, and no statistical difference was detected between different flow conditions (two-sample t-test; p = 0.78). TDAA-C yield (TDAA-C/DOC%) ranged from 1.67 to 18.5% and 0.90 to 24.6% at base-flow and high-flow conditions, respectively (Supplementary Table S2). Even though averaged TDAA-C yield at high-flow (9.86%) was higher than that of base-flow (6.71%), the difference again was not significant (t-test; p = 0.78). Compared with other large rivers such as Mississippi River (0.85–1.40%) and Atchafalaya River (0.94–2.95%) in subtropical regions (Shen et al., 2012), the four South Texas rivers generally had a higher TDAA-C yield, suggesting that DOM in this region may be more bioavailable than that of Mississippi and Atchafalaya Rivers, which have much longer flow paths.
Glycine (Gly) was the most abundant amino acid, on average accounting for 21.3% of TDAA in molecular composition, followed by aspartic acid (Asp; 11.3%), serine (Ser; 9.68%), and alanine (Ala; 9.46%; compositional data not shown). This pattern is consistent with other studies in riverine systems (e.g., Peter et al., 2012). We further applied PCA on amino acid composition (mol %) to explore any difference between base- and high-flow samples (Supplementary Figure S2). Principal component 1 (PC1) and principal component 2 (PC2) explained 50.0% and 17.8% of the data variance, respectively. The separation along the PC1 axis can be attributed to mainly ten variables, namely, β-alanine (BALA), γ-aminobutyric acid (GABA) and Gly with the most negative values, while glutamic acid (Glu), Ser, arginine (Arg), valine (Val), leucine (Leu) and isoleucine (Ile) with the most positive PC1 values. Therefore, PC1 provides preliminary separation of DOM samples based on their diagenetic characteristics, as an enrichment of Glu, Arg, Leu, and Ile suggests a fresh DOM source (Li et al., 2018), while a higher percentage of Gly, BALA, and GABA is indicative of a highly altered and degraded sample (Dauwe and Middelburg, 1998; Chen et al., 2004; Davis et al., 2009; Peter et al., 2012). Though no clear separation can be made, compared with base-flow samples, the trend that high-flow samples were positioned toward the positive or right end of PC1 is notable (Supplementary Figure S2).
Comparison of DOM Molecular Composition at Different Flow Conditions
Our previous study showed that the spatial difference in riverine DOM composition among these rivers is generally small (Lu et al., 2018), considering these rivers are spatially close (within 200 km), and have similar drainage basins (Mission and Aransas rivers from San Antonio-Nueces Coastal Basin, Nueces river from Nueces-Rio Grande Coastal Basin, and Lavaca river from Lavaca-Guadalupe Coastal Basin2). Furthermore, the difference among HPLC chromatographs across different rivers (Supplementary Figure S3) is much smaller than those from different sampling times at the same river (Supplementary Figure S4). Therefore, results will be more focused on temporal (among flow conditions) rather than spatial difference (among rivers).
High performance liquid chromatography is an important tool in molecular-level analysis of complex samples (Hertkorn et al., 2007), and has been used to isolate and identify biomolecules in many studies (Ogawa et al., 2001; Leenheer and Croué, 2003; Rathgeb et al., 2017). Using DOM samples collected from MR as an example, the HPLC chromatograms (i.e., the shape and the retention time of peaks) at base-flow were comparable (Supplementary Figure S4). Even though it is hard to extract a consistent trend from the HPLC chromatograms, it is clear that some peaks disappeared at high-flow conditions (e.g., peaks at ca. 5.8 min and ca. 11.0–12.0 min from ESI+; peaks at ca. 4.6–6.0 min, and peaks at ca. 8.0 min from ESI−; purple shades in Supplementary Figure S4), while other peaks emerged (e.g., peaks at ca. 6.0 and 14.0 min from ESI+; blue shades in Supplementary Figure S4), indicating changes in the DOM composition.
The averaged peak assignment percentage (Number of peaks assigned with formula/Number of peaks above S/N threshold × 100%) was 58.4% under ESI+, and 64.3% under ESI−, respectively. Element stoichiometry of a sample was calculated by averaging the H/C, O/C and N/C ratios of all assigned formulas. Element stoichiometry of one river at a certain sampling time (e.g., AR at-May 27, 2016) was calculated as the average of the duplicates (Supplementary Table S3), and element stoichiometry of a certain sampling time (e.g., May 27, 2016) was calculated as the average of all rivers on that sampling day (Table 1). Standard errors between duplicates were generally small (<0.01). Overall, under ESI+ mode, H/C ranged from 1.46 to 1.60 and O/C ranged from 0.21 to 0.27 at base flow condition, while H/C and O/C was in the range of 1.48–1.55 and 0.21–0.26, respectively, at high flow condition (Table 1). Under ESI+ mode, H/C decreased from 1.52 to 1.51 at high flow condition, but O/C remained constant (0.24). Statistical analysis showed that base and high flow samples were not different in terms of element stoichiometry under ESI+. However, under ESI− mode, H/C decreased from an average of 1.19 (1.07–1.26) at base flow, to an average of 1.07 (1.03–1.12) at high flow (t-test; p = 0.02), while O/C increased from 0.31 (0.29–0.33) at base flow, to 0.33 (0.32–0.34) at high flow (t-test; p = 0.03).
These changes in element ratios can be further visualized by van Krevelen diagrams (ESI+ mode, Supplementary Figure S5; ESI−, Supplementary Figure S6), in which H/C was plotted against O/C and each dot represents one formula. The van Krevelen diagrams of the four rivers, regardless of the flow condition, resembled published work (e.g., Tfaily et al., 2015), characterized by a large fraction of high H/C and low O/C molecules. Meanwhile, the change after high-flow events was clear, with a fraction of low H/C but high O/C molecules emerging in high-flow samples. This change is more evident when unique features were extracted from different flow conditions (Figure 2). Unique features at base flow were dominated by formulas with high H/C but low O/C ratios (Figure 2A), while those at high flow were mainly characterized by formulas with low H/C ratios (Figure 2B). The appearance of high-flow molecules (Figure 2B) and the loss of base-flow unique molecules (Figure 2A) shifted the element stoichiometry toward low H/C but high O/C (Table 1).
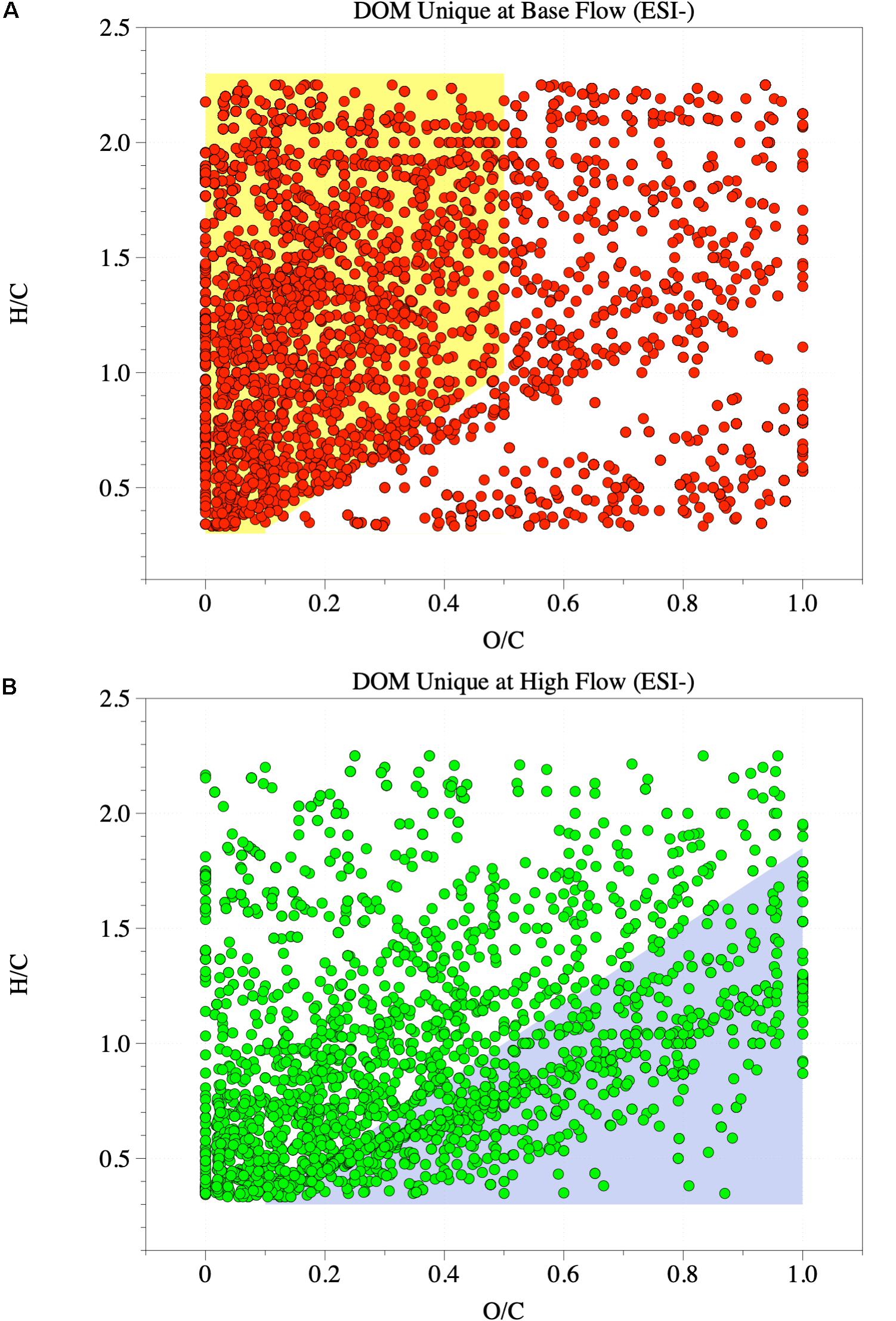
Figure 2. van Krevelen diagrams of riverine DOM under ESI– mode. (A) Unique features at base-flow (6725 unique formulas). (B) Unique features at high-flow (2622 unique formulas). Yellow shade in A indicates high H/C and low O/C formulas. Blue shade in B indicates low H/C formulas.
Dissolved organic matter was further categorized into different compound groups based on element stoichiometry. Again, changes in the proportions of these categories were more evident in ESI− mode (Table 2, Figure 3B, and Supplementary Figure S6) than those in ESI+ mode (Supplementary Table S4, Figure 3A, and Supplementary Figure S5). Regardless of the flow conditions, riverine DOM was dominated by lignin-like compounds (34.8%), followed by condensed aromatics (18.9%), and protein-like compounds (9.1%), under ESI−. From high-flow to low-flow, fractions of lignin-like compounds (34.7% to 35.1%; p = 0.42), tannin-like compounds (2.11% to 4.16%; p < 0.01), and condensed aromatics (16.2% to 23.3%; p = 0.04) all increased, while decreases were observed for unsaturated hydrocarbons (9.19% to 6.28%; p = 0.04), protein-like (9.92% to 7.71%; p = 0.06), lipid-like (3.23% to 1.59%; p = 0.03), and carbohydrate-like compounds (6.65% to 6.62%; p = 0.48). The distribution of compound classes under ESI− mode can be further visualized by PCA (percentages of each category as the PCA data input, and data were normalized before analysis; Figure 4), where base-flow samples were characterized by relative high fractions of protein- and lipid-like structures, while high-flow samples were characterized by elevated levels of carbohydrate-, lignin-, tannin-like compounds, and condensed aromatics. A similar pattern was observed under ESI+ mode, yet none of those trends was statistically significant (Figure 3A, Supplementary Figure S5, and Supplementary Table S4). Also noted were the much higher percentages (24–36%) of protein-like compounds under ESI+ mode, a pattern that was caused by preferential ionization of nitrogen containing molecules (Lin et al., 2012; Ohno et al., 2016).
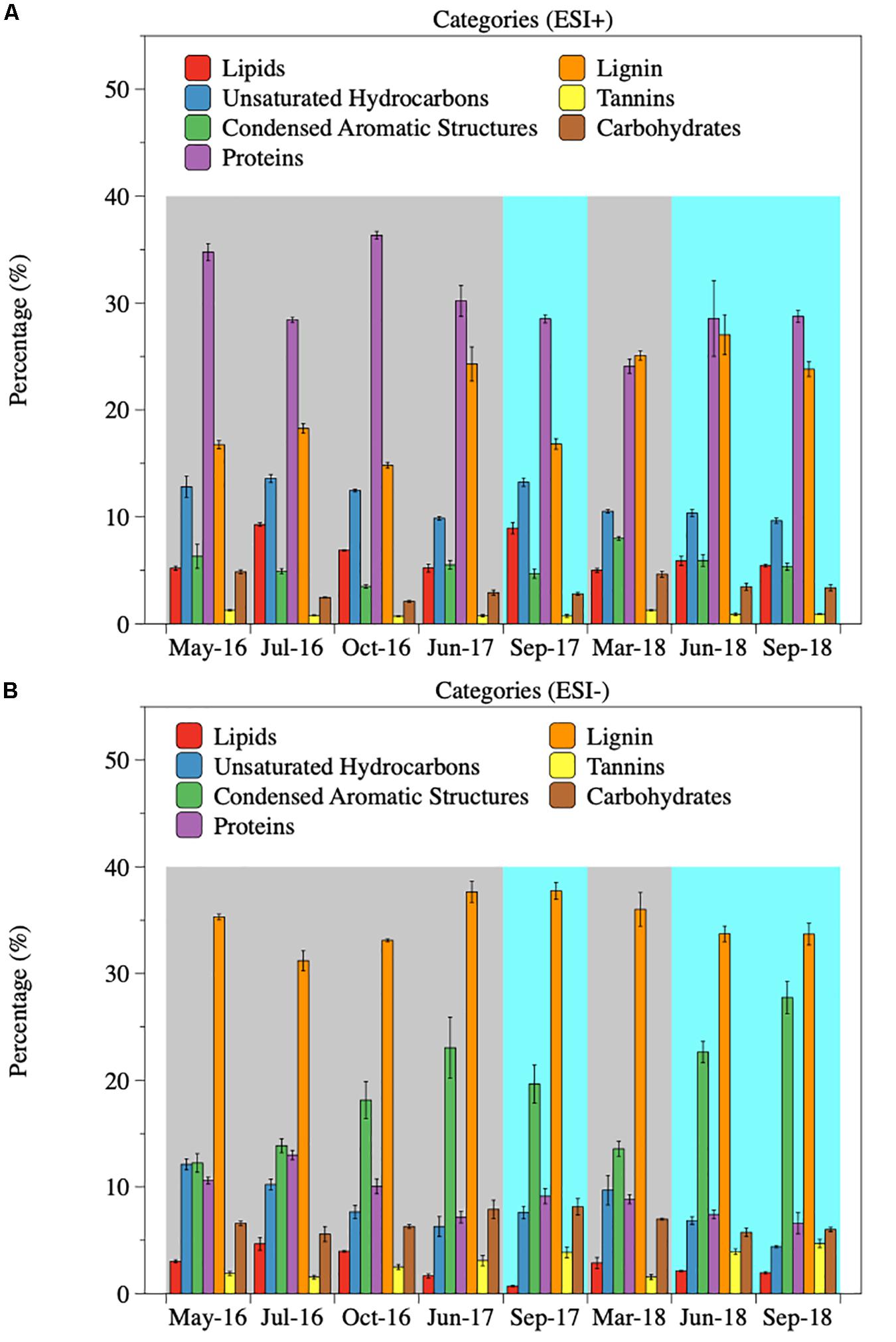
Figure 3. Percentages of different compound classes under ESI+ (A) and ESI– (B) modes. DOM was categorized into different compound classes based on the assigned formula and element stoichiometry. Gray shades indicate base-flow condition. Cyan shades indicate high-flow condition.
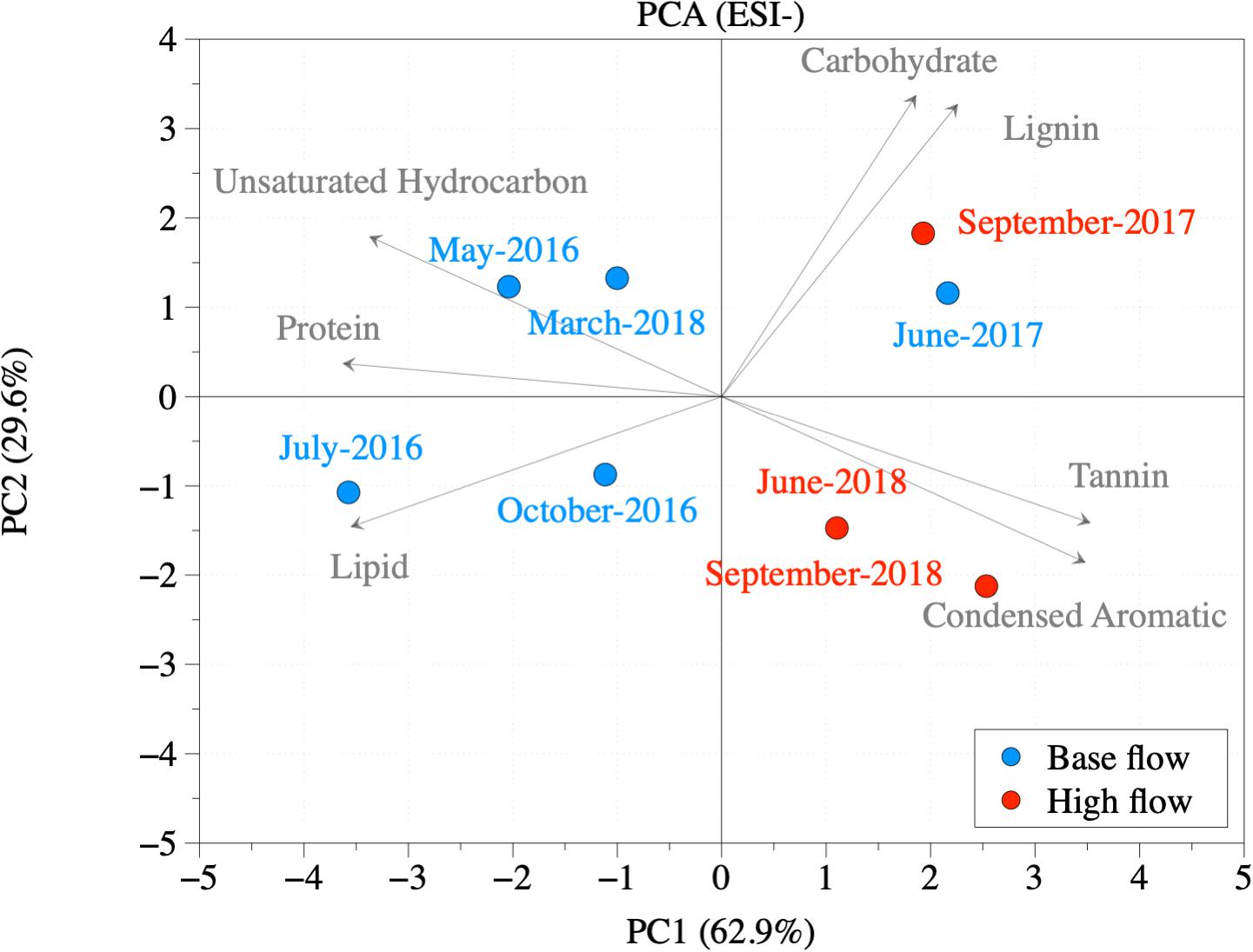
Figure 4. PCA based on the percentage of different compound classes under ESI– mode. Blue indicates samples at base-flow condition (May 2016, July 2016, October 2016, June 2017, and March 2018). Red indicates samples at high-flow condition (September 2017, June 2018, and September 2018).
The aromaticity of riverine DOM was calculated by AI (Koch and Dittmar, 2006, 2016). Based on AI, riverine DOM was dominated by non-aromatics under both ESI modes regardless of flow conditions (Table 3, Supplementary Table S5, and Figure 5). However, a significant increase in aromaticity was observed at high flow, as the proportion of aromatic and condensed aromatic formulas increased from 16.5 and 17.1% (p = 0.06) at base flow to 17.7 and 22.8% (p = 0.04) at high flow under ESI− mode, respectively (Table 3 and Figure 5B), consistent with the observed increase in condensed aromatics based on element stoichiometry (Table 1, Figure 3B, and Supplementary Figure S6). Under ESI+ mode, the changes in aromatic and condensed aromatic compounds were not significant (p = 0.18 and 0.23, respectively; Supplementary Table S5 and Figure 5A).
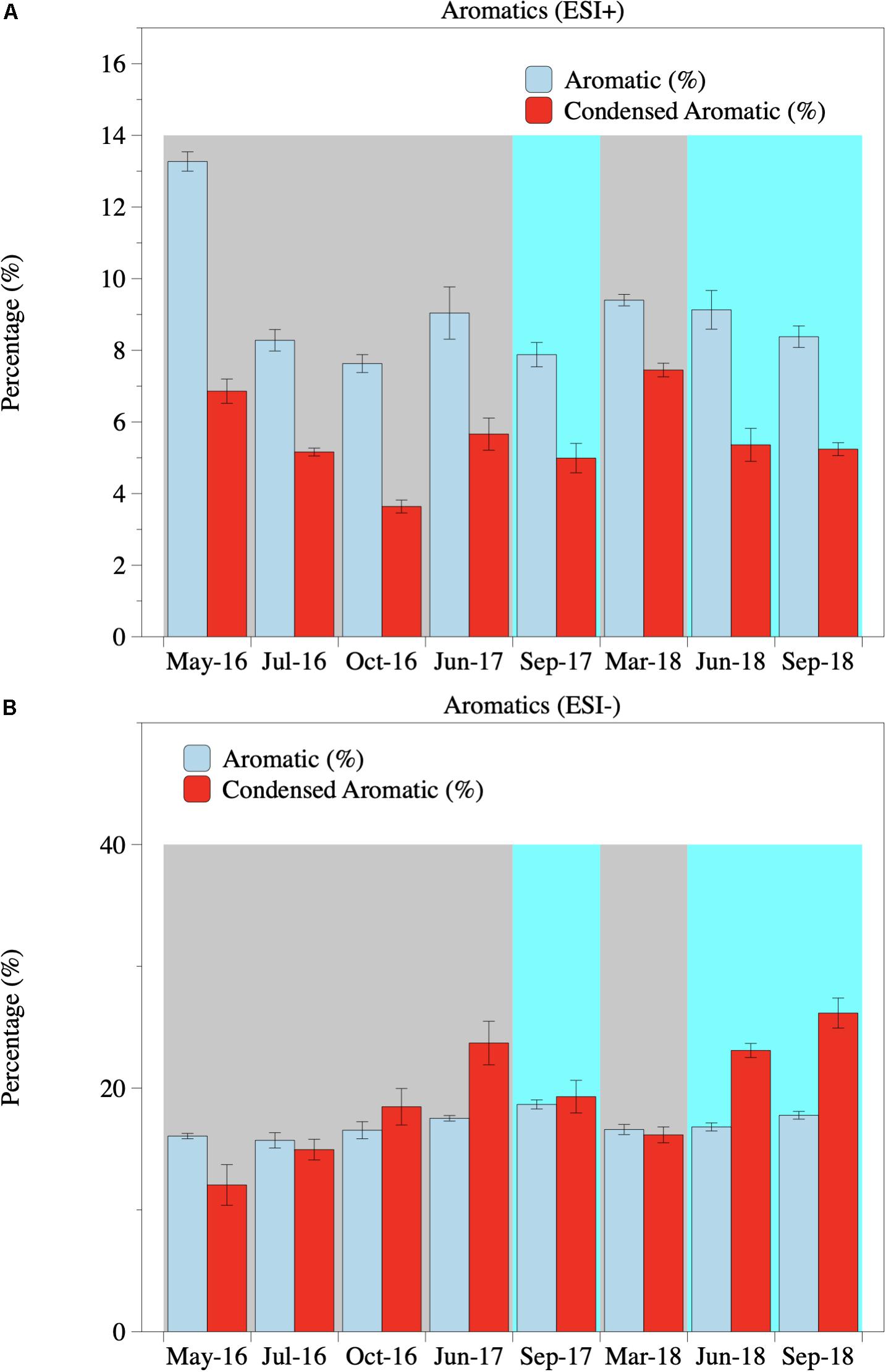
Figure 5. Percentages of different compound classes under ESI+ (A) and ESI– (B) modes. DOM was categorized into different aromatic classes based on the assigned formula and the calculated AI. Gray shades indicate base-flow condition. Cyan shades indicate high-flow condition.
Since changes in DOM composition were more evident in ESI− based on MS, the MS/MS analysis was focused on ESI−. On average, ca. 500 MS/MS features were obtained for each sample, and the non-targeted MS/MS assigned a total of 829 compounds based on the precursor and product ion spectra (ESI− mode), while the majority cannot be assigned due to the limitation of the database. Even though this assignment cannot guarantee an exact structure of the DOM compound, it provides insights into the possible structural moieties of DOM, and how these moieties changed during a high-flow event. When focused on the unique formulas found only at high-flow conditions, a total number of 73 compounds were found. These 73 compounds contained various metabolites from Loquatoside (C20H22O11), with a possible source from the fruit of loquat (Eriobotrya japonica; common in South Texas3), to Egonol (C19H18O5) from mushrooms Laetiporus sulphureus var. miniatu (also present in South Texas), with an average H/C of 1.22 and O/C of 0.40 (a list of compounds can be found in the Supplementary Data Sheet S1. The matches with metabolites in the database do not necessarily confirm the exact structures, which would need further work, such as running with known standards, but these results show the power of non-targeted MS/MS in seeking molecules of interest from an extremely complex DOM pool. The MS/MS fragments of precursor molecules can be assigned to exact structures with confidence due to their smaller m/z and the distribution of these fragments further facilitates the assignment of precursor ions with the database. Most of these assigned compounds have multiple aromatic rings and multiple O atoms in their structure, which can be further confirmed by their product ion spectra (e.g., the presence of C6H5 fragments). For instance, precursor ion (m/z of 473.0747) MS spectrum (Figure 6B) was first extracted from the HPLC chromatogram (Figure 6A). The precursor ion was selected by quadrupole mass filter and was further fragmented by collision to produce product ion spectra (Figure 6C). The product ion spectra were further compared with the database (Figure 6D), and the presence of several aromatic-ring structures after collision in the product ion spectra confirmed an oxygenated, aromatic-rich structure for the compound C22H18O12. Another example can be found in Supplementary Figure S7.
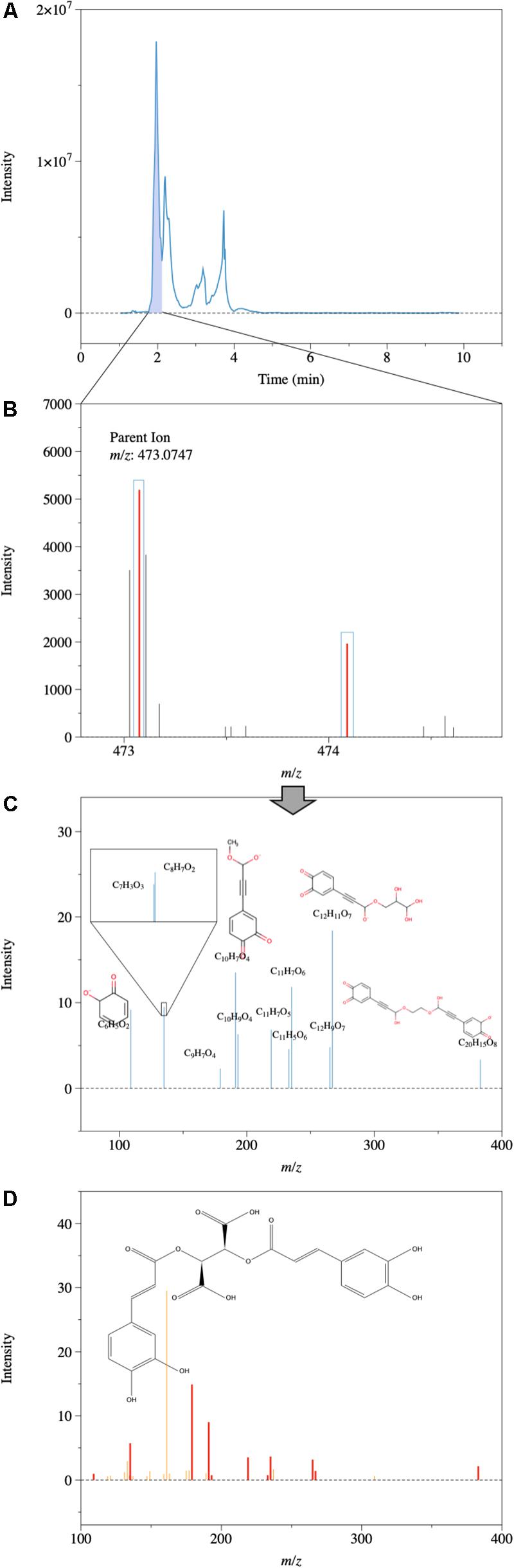
Figure 6. One example of assigned structures based on MS/MS spectra and a METLIN metabolites database. Note that the exact structures may not be right, but the product ions after collision proved the presence of aromatic ring structures and O atoms. (A) HPLC chromatogram; (B) Precursor ion (m/z of 473.0747) extracted from the HPLC chromatogram. (C) Detected product ion spectra after application of a collision energy of 40 V. (D) Predicted product ion spectrum of compound C22H18O12 based on database (METLIN and Human Metabolome Database at: http://www.hmdb.ca/) at the same collision energy of 40 V, matches between detected spectra and predicted spectra are shown in bold red.
Comparison of Geometric Configurations and Isomeric Percentage During Different Flow Conditions
In ion mobility, separation of different molecules is achieved by low-energy interactions between molecules and the inert buffer gas. The time (drift time) for each molecule to traverse the mobility tube is a function of its 3D structure, i.e., molecules with more stretched structures are slowed down more easily by the inert gas, and thus possess longer drift times. Therefore, the geometric configuration of DOM molecules, in the form of collision section area (collision cross section, CCS) values, can be quantified by calibrating with known standards (Tune Mix, G2421A, Agilent Technologies, Santa Clara, CA, United States). CCS values of the DOM molecules generally increased with increasing m/z (Figure 7 and Supplementary Figure S8). Compared with biomolecule standards (May et al., 2014), natural DOM molecules had more compacted geometric configurations, which was consistent with our previous findings (Lu et al., 2018). However, a group of DOM compounds possessed CCS values even higher than those of tetraalkylammonium standards. These compounds were generally in the m/z range of 100–400, and were mainly from high-flow DOM. Assigned molecular formula based on MS data indicate that these compounds had much higher O/C ratios (average of 0.51; Supplementary Table S6) compared with bulk O/C under ESI+. However, MS/MS analysis did not find any match in the metabolite database, suggesting that these compounds are likely natural and/or processed organic matter. Nevertheless, the similar CCS values between high- and base-flow DOM (Figure 7 and Supplementary Figure S8), suggest that the overall geometric configuration of DOM was not affected by flow conditions.
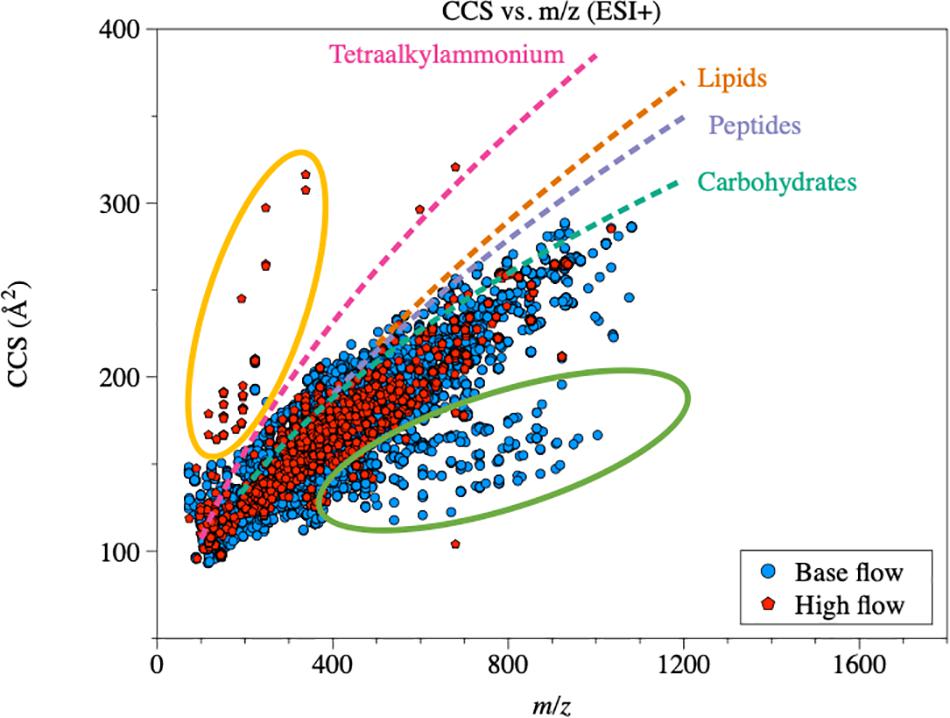
Figure 7. CCS vs. m/z under ESI+ mode. Trend lines of CCS vs. m/z for biomolecules were calculated based on May et al. (2014). CCS of riverine DOM was generally lower than biomolecules. The majority from different flow conditions cannot be clearly separated. Green oval indicates more compacted compounds (more diagenetically altered) from base-flow samples. Yellow oval indicates more extended compounds (less diagenetically altered) from high-flow samples.
Isomeric information on DOM was obtained via IM-MS (Lu et al., 2018). Structural isomers, though possessing a same molecular formula, have different three-dimensional structures. With a resolving power of ca. 60, drift time IM-MS can separate isomers with a 2% difference in their CCS values (Nichols et al., 2018). Therefore, isomer percentage was further defined as:
At base-flow condition, isomer percentages of riverine DOM molecules ranges from 5.71 to 17.3% across different rivers, with the highest isomer number of 8 in ESI+ mode, and 3.76 to 21.8%, with the highest isomer number of 10 in ESI− mode (Table 4), consistent with a previous study (Lu et al., 2018). At high-flow, isomer percentage under ESI+ mode ranges from 8.48 to 15.2%, while from 3.44 to 14.0% under ESI− mode (Table 4). The change in isomer percentage under ESI+ mode was not significant (p = 0.17). However, under ESI− mode, isomer percentage decreased significantly from 11.3% at base-flow to 7.72% at high-flow (p = 0.02). Further information on the change in isomeric percentage at different flow conditions can be found in the Supporting Information.
Discussion
Impact of Storm Events on Riverine DOM
Besides quantity, a high-flow event can affect the composition of DOM (Gremm and Kaplan, 1998; Hood et al., 2006; Zhang et al., 2007; Spencer et al., 2008), which is a result of mobilization of different terrestrial DOM pools that are not available under base flow conditions (Vidon et al., 2008; Fellman et al., 2009; Osburn et al., 2019). The appearance of a group of new compounds under high flow is consistent with this idea (Figure 2B). In terms of compound classes, high-flow DOM was more aromatic (Tables 2, 3 and Figure 5), and had elevated proportions of lignin- and tannin-like compounds (Table 2 and Figures 3, 4). During periods of high flow, elevated water level and faster flow can mobilize the DOM on the higher part of the riverbank and watershed. In these watersheds, DOM is mainly sourced from higher plants (Mooney and McClelland, 2012). This speculation is supported by the PCA (Figure 4), in which the high-flow DOM has positive PC1 loading, showing a strong terrestrial signal, and an elevated contribution from higher plants. Furthermore, the non-targeted MS/MS confirmed the terrestrial source, as the assigned structures from MS/MS are highly oxygenated (O/C of 0.40) and contain multiple aromatic structures.
However, the bioavailability of high flow DOM is controversial. Since no bioassay experiment was conducted to quantify the bioavailability of DOM from different flow conditions, we have to mainly rely on the chemical composition information obtained through MS and MS/MS. It has been suggested that hydrogen-deficient molecules with low H/C ratios are not easily metabolized by microbes (Kim et al., 2006), and molecules with a H/C ≤ 1.5 are considered to be recalcitrant (D’Andrilli et al., 2015). Therefore, a decrease in H/C at high-flow in both ESI modes (Table 1, Supplementary Table S3, and Figure 2) may suggest an increase in recalcitrance in DOM at high-flow. However, other studies have demonstrated that terrestrial DOM with high O/C and enriched aromatic structures are selectively degraded in fresh water systems (e.g., Kellerman et al., 2014, 2015). Furthermore, the amino acids analysis showed that the majority of base-flow DOM (except for May-2016 and October-2016 samples) had high mol% of Gly, BALA, and GABA, clearly suggesting a more diagenetically altered nature (Supplementary Figure S2). As a comparison, DOM at high-flow tended to be at the positive end of PC1, characterized by high mol% of Glu, Arg, Leu, and Ile, indicating that these DOM were less altered. This, again, suggests a decrease in recalcitrance of DOM at high flow.
The “apparent” conflicting results may be reconciled by the fact that high-flow DOM may contain both recalcitrant and highly labile fractions of DOM. In addition, at base-flow conditions, due to the low flow rate, high temperature, and strong irradiation in South Texas, riverine DOM may have been heavily processed due to strong microbial activities and photochemical reactions. This explains the fact that the base-flow DOM appears to be more diagenetically altered based on amino acids analysis. At high-flow conditions, the chemical composition of river DOM is mainly shaped by terrestrial DOM, as the most degraded base-flow DOM is carried away by elevated flow with less time for processing during the transit. The mobilized DOM, often enriched in O but depleted in H due to the presence of aromatic structures (e.g., Vidon et al., 2008; Majidzadeh et al., 2017; Osburn et al., 2019; Wagner et al., 2019), tends to resist biodegradation (Spencer et al., 2016), but can be selectively degraded with the presence of sunlight (Stubbins et al., 2010). However, photochemical degradation may be inhibited at high flow due to several reasons. First, the residence time of river water, as well as mobilized terrestrial DOM, is highly reduced during a high-flow event due to the increased flow rate (Supplementary Figure S1B). Hence, the exposure time of DOM to sunlight is reduced. Second, an increase in turbidity due to the resuspension of sediment at high flow would reduce the amount of light absorbed by DOM. Finally, the remaining aromatic DOM might be protected by the self-shading effect when the colored DOM (CDOM) concentration is high (Del Castillo et al., 1999; Köhler et al., 2002; Larson et al., 2007; Granskog, 2012; Wagner et al., 2019).
Overall, DOM at high-flow appears to contain relatively higher percentages of aromatic structures, which may represent a recalcitrant nature under certain conditions, but can be very reactive when conditions change. Therefore, the high-flow events can relocate a large amount of terrestrial photo-active DOM to coastal region, where it could be rapidly utilized by microbes and further fuel primary production in brackish water (e.g., Liu et al., 2011; Mooney and McClelland, 2012; Bruesewitz et al., 2013; Reyna et al., 2017). High flow DOM also seems to contain a fraction of highly bioavailable DOM in the form of hydrolyzable amino acids, which can further supply labile substrates to estuaries and bays. At the same time, the refractory components of the relocated terrestrial DOM may persist for a prolonged period of time (e.g., from weeks to months), and can cause lingering impact on estuarine C cycling (Osburn et al., 2019).
Changes in the Geometric Configuration and Isomeric Composition of Riverine DOM
The correlations between CCS and m/z in base-flow and high-flow conditions can be described adequately by a power-law relationship (base-flow ESI+: y = 18.7 x0.37, R2 = 0.72; high-flow ESI+: y = 16.0 x0.40, R2 = 0.80; base-flow ESI−: y = 23.1 x0.35, R2 = 0.20; high-flow ESI−: y = 38.9 x0.25, R2 = 0.12). χ2 tests were performed and no statistical difference was detected in CCS-m/z distribution between base-flow and high-flow samples (Figure 7 and Supplementary Figure S8; p-value > 0.05 for both ESI modes), suggesting that the flow condition did not affect the geometric configuration of riverine DOM. This pattern implies that DOM from different sources and processes (i.e., highly altered at base flow vs. terrestrial at high flow) has similar geometric structures. As natural DOM is originated from biopolymers, the fact that the majority of riverine DOM molecules possessed lower CCS values (i.e., more compacted geometric structures) compared with standard biomolecules suggests that environmental degradation tends to make natural DOM more compacted (Lu et al., 2018). Even though no statistical difference was detected, CCS values of some DOM at base flow were lower than the others (green oval in Figure 7), while high-CCS molecules appeared in high-flow samples (yellow oval in Figure 7). Based on the formula assigned, these high-CCS compounds generally have a relative high O/C ratio (average 0.51; 0.33–0.71), indicating a strong terrestrial signal. The presence of these two groups of features in IM-MS diagram supports the speculation that CCS can reflect the diagenetic status of DOM to an extent, as more diagenetically altered compounds (from base-flow, green oval) tend to have even more compacted structures, while less altered compounds (from high-flow, yellow oval) may have extended geometric structure as biomolecules. However, this is only the case for a small group of compounds in IM-MS diagram, as base-flow and high-flow samples were statistically similar. Also, IM-MS analysis can only represent a small fraction of molecules detected in MS (Lu et al., 2018). Therefore, more studies are needed to reveal the relationship between the CCS of DOM and its reactivity.
Although no direct measurement was conducted, a recent study suggested that riverine DOM after storm events could potentially have high structural diversity (Wagner et al., 2019), based on modeling results (Zark et al., 2017; Hawkes et al., 2018; Zark and Dittmar, 2018). Based on the central limit theorem, these modeling studies assumed a random combination of atoms in DOM molecules and suggested that each formula assigned in ultrahigh-resolution mass spectrometry potentially represents 10–100 different isomers (Zark et al., 2017; Hawkes et al., 2018). Our results, however, suggested an opposite pattern. The percentages of molecules that have isomers, across different sampling sites, sampling time and ESI modes, were highly constrained, ranging from ca. 3% to ca. 22%. Also highly constrained was the number of isomers for each formula, with most formula having only 2 isomers (one formula, two different molecules) and the largest of 10 (one formula, ten different molecules). This distribution pattern can be further visualized by overlying all isomers together, with isomers in DOM having separated, non-even peaks (Figure 8). The lower isomer percentages indicate that DOM formation may have gone through certain biochemical pathways, and that there are additional constraints on the bonds breaking and formation processes of natural DOM. This is in sharp contrast with crude oil, in which the isomers of a given hydrocarbon are more evenly distributed (Benigni et al., 2017). The formation of crude oil involves random chemical reactions under high pressure and heat (e.g., Tissot et al., 1974; Vandenbroucke and Largeau, 2007), while the biogeochemical pathways, key to DOM formation, may not be random. For example, odd- and even-numbered n-alkanes are evenly distributed in crude oil, while the odd-numbered dominates even-numbered n-alkanes in samples derived from high plants.
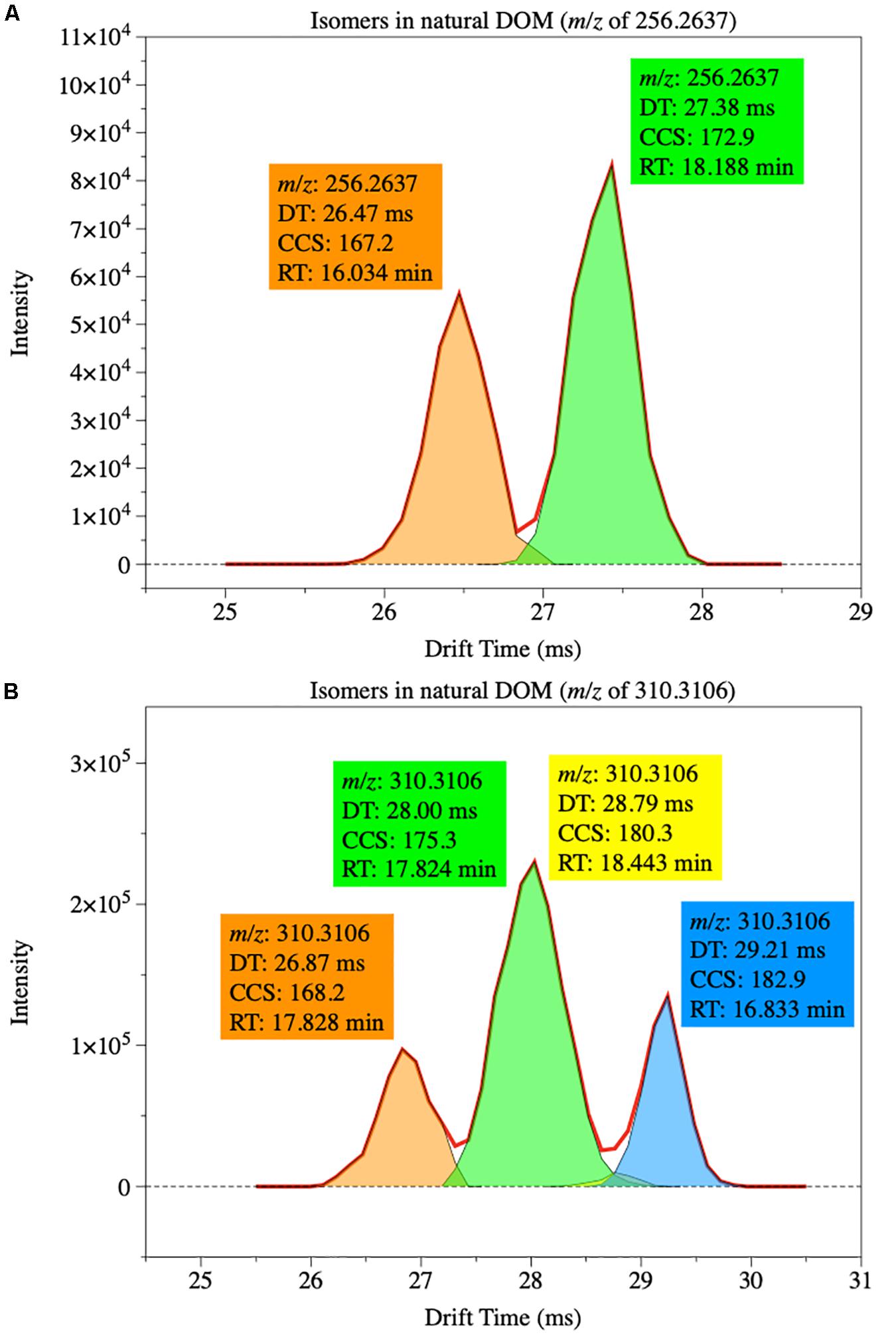
Figure 8. Examples of isomer distribution of two sets of compounds in riverine DOM. (A) Compound with a m/z of 256.2637 has two isomers. (B) Compound with a m/z of 310.3106 has four isomers.
While more work is needed to reconcile the discrepancy between our data and modeling results, this dataset provides one of the first few quantitative studies about DOM isomers based on actual measurements (Benigni et al., 2017; Lu et al., 2018; Leyva et al., 2019), and it opens a new direction in molecular-level DOM analysis for the future.
Conclusion
A multi-dimension molecular level analysis of riverine DOM via IM Q-TOF LCMS revealed molecular level changes in DOM at different flow conditions in the South Texas region. Our results showed that high-flow events driven by storm or hurricane, as well as the subsequent biotic and abiotic process, changed the chemical composition of riverine DOM. Even though the bulk concentrations of DOM were similar across the sampling period, DOM pool experienced an increase in the proportions of lignin-, tannin-, carbohydrate-like, and condensed aromatic formulas, showing a stronger terrestrial signal as expected. With LC and IM, we obtained a direct measurement of isomers in riverine DOM. With an isomer percentage ranging from 3 to 22%, our data indicated that the number of isomers in riverine DOM was highly constrained. This finding in the isomeric information on riverine DOM not only showed the impacts of high-flow events on DOM, but also provided crucial insights into molecular and structural details of riverine DOM under extreme weather events.
Data Availability Statement
All datasets generated for this study are available upon request and most of them can be found in the article/Supplementary Material.
Author Contributions
KL and ZL designed the experiments and wrote the manuscript. KL conducted the experiments.
Funding
This study was supported by the NOAA (NA15NOS4780185), an Institutional Grant (NA14OAR4170102) to the Texas Sea Grant College Program from the National Sea Grant Office, NOAA, and the NSF Chemical Oceanography program (OCE 1763167).
Conflict of Interest
The authors declare that the research was conducted in the absence of any commercial or financial relationships that could be construed as a potential conflict of interest.
Acknowledgments
We thank Dr. Ryan Hladyniuk from the UTMSI Core Facility Lab for helping assemble and maintain the equipment, and Drs. Carol Haney Ball, Dawn Stickle, Caroline S. Chu, and Daniel Cuthbertson from the Agilent Technologies for invaluable technical support. Special thanks to Drs. Robert Dickey and Ed Buskey for making the instrumentation available at UTMSI. We also thank Dr. Xianbiao Lin, Dr. Kai Wu, Jason Jenkins, John O’Connor, and Cory Staryk for their help in sampling trips.
Supplementary Material
The Supplementary Material for this article can be found online at: https://www.frontiersin.org/articles/10.3389/fmars.2019.00673/full#supplementary-material
Footnotes
- ^ https://www.webofknowledge.com/
- ^ http://www.twdb.texas.gov/
- ^ https://aggie-horticulture.tamu.edu/citrus/loquat.htm
References
Bender, M. A., Knutson, T. R., Tuleya, R. E., Sirutis, J. J., Vecchi, G. A., Garner, S. T., et al. (2010). Modeled impact of anthropogenic warming on the frequency of intense Atlantic Hurricanes. Science 327, 454–458. doi: 10.1126/science.1180568
Benigni, P., Sandoval, K., Thompson, C. J., Ridgeway, M. E., Park, M. A., Gardinali, P., et al. (2017). Analysis of photoirradiated water accommodated fractions of crude oils using tandem TIMS and FT-ICR MS. Environ. Sci. Technol. 51, 5978–5988. doi: 10.1021/acs.est.7b00508
Bomar, G. W. (2017). Weather in Texas: The Essential Handbook, Third Edn. Austin, TX: University of Texas Press.
Bruesewitz, D. A., Gardner, W. S., Mooney, R. F., Pollard, L., and Buskey, E. J. (2013). Estuarine ecosystem function response to flood and drought in a shallow, semiarid estuary: nitrogen cycling and ecosystem metabolism. Limnol. Oceanogr. 58, 2293–2309. doi: 10.4319/lo.2013.58.6.2293
Buffam, I., Galloway, J. N., Blum, L. K., and McGlathery, K. J. (2001). A stormflow/baseflow comparison of dissolved organic matter concentrations and bioavailability in an Appalachian stream. Biogeochemistry 53, 269–306. doi: 10.1023/A:1010643432253
Cai, Y., Guo, L., Wang, X., Lohrenz, S. E., and Mojzis, A. K. (2013). Effects of tropical cyclones on river chemistry: a case study of the lower Pearl River during Hurricanes Gustav and Ike. Estuar. Coast. Shelf Sci. 129, 180–188. doi: 10.1016/j.ecss.2013.05.019
Carlson, C. A., and Hansell, D. A. (2015). “DOM Sources, Sinks, Reactivity, and Budgets,” in Biogeochemistry of Marine Dissolved Organic Matter, Second Edn, eds D. A. Hansell, and C. A. Carlson, (Boston, MA: Academic Press), 65–126. doi: 10.1016/b978-0-12-405940-5.00003-0
Cauwet, G. (2002). “DOM in the coastal zone,” in Biogeochemistry of Marine Dissolved Organic Matter, First Edn, eds D. A. Hansell, and C. A. Carlson, (Boston, MA: Academic Press), 579–609. doi: 10.1016/b978-012323841-2/50014-2
Chen, J., Li, Y., Yin, K., and Jin, H. (2004). Amino acids in the Pearl River Estuary and adjacent waters: origins, transformation and degradation. Cont. Shelf Res. 24, 1877–1894. doi: 10.1016/j.csr.2004.06.013
Dai, M., Yin, Z., Meng, F., Liu, Q., and Cai, W.-J. (2012). Spatial distribution of riverine DOC inputs to the ocean: an updated global synthesis. Curr. Opin. Environ. Sustain. 4, 170–178. doi: 10.1016/j.cosust.2012.03.003
Dalzell, B. J., Filley, T. R., and Harbor, J. M. (2007). The role of hydrology in annual organic carbon loads and terrestrial organic matter export from a midwestern agricultural watershed. Geochim. Cosmochim. Acta 71, 1448–1462. doi: 10.1016/j.gca.2006.12.009
D’Andrilli, J., Cooper, W. T., Foreman, C. M., and Marshall, A. G. (2015). An ultrahigh-resolution mass spectrometry index to estimate natural organic matter lability. Rapid Commun. Mass Spectrom. 29, 2385–2401. doi: 10.1002/rcm.7400
Dauwe, B., and Middelburg, J. J. (1998). Amino acids and hexosamines as indicators of organic matter degradation state in North Sea sediments. Limnol. Oceanogr. 43, 782–798. doi: 10.4319/lo.1998.43.5.0782
Davis, J., Kaiser, K., and Benner, R. (2009). Amino acid and amino sugar yields and compositions as indicators of dissolved organic matter diagenesis. Org. Geochem. 40, 343–352. doi: 10.1016/j.orggeochem.2008.12.003
Del Castillo, C. E., Coble, P. G., Morell, J. M., López, J. M., and Corredor, J. E. (1999). Analysis of the optical properties of the Orinoco River plume by absorption and fluorescence spectroscopy. Mar. Chem. 66, 35–51. doi: 10.1016/S0304-4203(99)00023-7
Dittmar, T., Koch, B., Hertkorn, N., and Kattner, G. (2008). A simple and efficient method for the solid-phase extraction of dissolved organic matter (SPE-DOM) from seawater. Limnol. Oceanogr. Methods 6, 230–235. doi: 10.4319/lom.2008.6.230
Evans, C. D., Freeman, C., Cork, L. G., Thomas, D. N., Reynolds, B., Billett, M. F., et al. (2007). Evidence against recent climate-induced destabilisation of soil carbon from 14C analysis of riverine dissolved organic matter. Geophys. Res. Lett. 34:L07407. doi: 10.1029/2007GL029431
Fellman, J. B., Hood, E., Edwards, R. T., and D’Amore, D. V. (2009). Changes in the concentration, biodegradability, and fluorescent properties of dissolved organic matter during stormflows in coastal temperate watersheds. J. Geophys. Res. Biogeosci. 114:G01021. doi: 10.1029/2008JG000790
Granskog, M. A. (2012). Changes in spectral slopes of colored dissolved organic matter absorption with mixing and removal in a terrestrially dominated marine system (Hudson Bay, Canada). Mar. Chem. 134, 10–17. doi: 10.1016/j.marchem.2012.02.008
Gremm, T. J., and Kaplan, L. A. (1998). Dissolved carbohydrate concentration, composition, and bioavailability to microbial heterotrophs in stream water. Acta Hydrochim. Hydrobiol. 26, 167–171.
Han, X., Feng, L., Hu, C., and Kramer, P. (2018). Hurricane-induced changes in the everglades national park mangrove forest: landsat observations between 1985 and 2017. J. Geophys. Res. Biogeosci. 123, 3470–3488. doi: 10.1029/2018JG004501
Hawkes, J. A., Patriarca, C., Sjöberg, P. J. R., Tranvik, L. J., and Bergquist, J. (2018). Extreme isomeric complexity of dissolved organic matter found across aquatic environments. Limnol. Oceanogr. Lett. 3, 21–30. doi: 10.1002/lol2.10064
Hedges, J. I. (2002). “Why Dissolved Organics Matter,” in Biogeochemistry of Marine Dissolved Organic Matter, First Edn, eds D. A. Hansell, and C. A. Carlson, (Boston: Academic Press), 1–33. doi: 10.1016/b978-012323841-2/50003-8
Hedges, J. I., Keil, R. G., and Benner, R. (1997). What happens to terrestrial organic matter in the ocean? Org. Geochem. 27, 195–212. doi: 10.1016/S0146-6380(97)00066-1
Hertkorn, N., Harir, M., Koch, B. P., Michalke, B., and Schmitt-Kopplin, P. (2013). High-field NMR spectroscopy and FTICR mass spectrometry: powerful discovery tools for the molecular level characterization of marine dissolved organic matter. Biogeosciences 10, 1583–1624. doi: 10.5194/bg-10-1583-2013
Hertkorn, N., Ruecker, C., Meringer, M., Gugisch, R., Frommberger, M., Perdue, E. M., et al. (2007). High-precision frequency measurements: indispensable tools at the core of the molecular-level analysis of complex systems. Anal. Bioanal. Chem. 389, 1311–1327. doi: 10.1007/s00216-007-1577-4
Hinton, M. J., Schiff, S. L., and English, M. C. (1997). The significance of storms for the concentration and export of dissolved organic carbon from two Precambrian Shield catchments. Biogeochemistry 36, 67–88. doi: 10.1023/A:1005779711821
Hood, E., Gooseff, M. N., and Johnson, S. L. (2006). Changes in the character of stream water dissolved organic carbon during flushing in three small watersheds, Oregon. J. Geophys. Res. Biogeosci. 111:G01007. doi: 10.1029/2005JG000082
Inamdar, S. P., Christopher, S. F., and Mitchell, M. J. (2004). Export mechanisms for dissolved organic carbon and nitrate during summer storm events in a glaciated forested catchment in New York, USA. Hydrol. Process. 18, 2651–2661. doi: 10.1002/hyp.5572
Jiao, N., Herndl, G. J., Hansell, D. A., Benner, R., Kattner, G., Wilhelm, S. W., et al. (2011). The microbial carbon pump and the oceanic recalcitrant dissolved organic matter pool. Nat. Rev. Micro 9:555. doi: 10.1038/nrmicro2386-c5
Kellerman, A. M., Dittmar, T., Kothawala, D. N., and Tranvik, L. J. (2014). Chemodiversity of dissolved organic matter in lakes driven by climate and hydrology. Nat. Commun. 5:3804. doi: 10.1038/ncomms4804
Kellerman, A. M., Kothawala, D. N., Dittmar, T., and Tranvik, L. J. (2015). Persistence of dissolved organic matter in lakes related to its molecular characteristics. Nat. Geosci. 8, 454–457. doi: 10.1038/ngeo2440
Kim, S., Kaplan, L. A., and Hatcher, P. G. (2006). Biodegradable dissolved organic matter in a temperate and a tropical stream determined from ultra-high resolution mass spectrometry. Limnol. Oceanogr. 51, 1054–1063. doi: 10.4319/lo.2006.51.2.1054
Kim, S., Kramer, R. W., and Hatcher, P. G. (2003). Graphical method for analysis of ultrahigh-resolution broadband mass spectra of natural organic matter, the Van Krevelen Diagram. Anal. Chem. 75, 5336–5344. doi: 10.1021/ac034415p
Knutson, T. R., McBride, J. L., Chan, J., Emanuel, K., Holland, G., Landsea, C., et al. (2010). Tropical cyclones and climate change. Nat. Geosci. 3, 157–163. doi: 10.1038/ngeo779
Knutson, T. R., Sirutis, J. J., Garner, S. T., Vecchi, G. A., and Held, I. M. (2008). Simulated reduction in Atlantic hurricane frequency under twenty-first-century warming conditions. Nat. Geosci. 1, 359–364. doi: 10.1038/ngeo202
Koch, B. P., and Dittmar, T. (2006). From mass to structure: an aromaticity index for high-resolution mass data of natural organic matter. Rapid Commun. Mass Spectrom. 20, 926–932. doi: 10.1002/rcm.2386
Koch, B. P., and Dittmar, T. (2016). From mass to structure: an aromaticity index for high-resolution mass data of natural organic matter. Rapid Commun. Mass Spectrom. 30, 250–250. doi: 10.1002/rcm.7433
Köhler, S., Buffam, I., Jonsson, A., and Bishop, K. (2002). Photochemical and microbial processing of stream and soil water dissolved organic matter in a boreal forested catchment in northern Sweden. Aquat. Sci. 64, 269–281. doi: 10.1007/s00027-002-8071-z
Kramer, M. G., Sanderman, J., Chadwick, O. A., Chorover, J., and Vitousek, P. M. (2012). Long-term carbon storage through retention of dissolved aromatic acids by reactive particles in soil. Glob. Change Biol. 18, 2594–2605. doi: 10.1111/j.1365-2486.2012.02681.x
Kujawinski, E. B. (2002). Electrospray ionization fourier transform ion cyclotron resonance mass spectrometry (ESI FT-ICR MS): characterization of complex environmental mixtures. Environ. Forensics 3, 207–216. doi: 10.1006/enfo.2002.0109
Kujawinski, E. B., and Behn, M. D. (2006). Automated analysis of electrospray ionization fourier transform ion cyclotron resonance mass spectra of natural organic matter. Anal. Chem. 78, 4363–4373. doi: 10.1021/ac0600306
Kuznetsova, M., and Lee, C. (2002). Dissolved free and combined amino acids in nearshore seawater, sea surface microlayers and foams: influence of extracellular hydrolysis. Aquat. Sci. 64, 252–268. doi: 10.1007/s00027-002-8070-0
Larson, J. H., Frost, P. C., Lodge, D. M., and Lamberti, G. A. (2007). Photodegradation of dissolved organic matter in forested streams of the northern Great Lakes region. J. N. Am. Benthol. Soc. 26, 416–425. doi: 10.1899/06-097.1
Lee, C., Wakeham, S. G., and Hedges, J. I. (2000). Composition and flux of particulate amino acids and chloropigments in equatorial pacific seawater and sediments. Deep Sea Res. Part 1 Oceanogr. Res. Papers 47, 1535–1568. doi: 10.1016/S0967-0637(99)00116-8
Leenheer, J. A., and Croué, J.-P. (2003). Peer reviewed: characterizing aquatic dissolved organic matter. Environ. Sci. Technol. 37, 18A–26A. doi: 10.1021/es032333c
Leyva, D., Valadares, L., Porter, J., Wolff, J., Jaffè, R., and Fernandez-Lima, F. (2019). Understanding the structural complexity of dissolved organic matter: isomeric diversity. Faraday Discuss. 218, 431–440. doi: 10.1039/C8FD00221E
Li, X., Liu, Z., Chen, W., Wang, L., He, B., Wu, K., et al. (2018). Production and transformation of dissolved and particulate organic matter as indicated by amino acids in the Pearl River Estuary, China. J. Geophys. Res. Biogeosci. 123, 3523–3537. doi: 10.1029/2018JG004690
Lin, P., Rincon, A. G., Kalberber, M., and Yu, J. (2012). Elemental composition of HULIS in the Pearl River Delta region, China: results inferred from positive and negative electrospray high resolution mass spectrometric data. Environ. Sci. Technol. 46, 7454–7462. doi: 10.1021/es300285d
Liu, Z., Liu, S., Liu, J., and Gardner, W. S. (2013). Differences in peptide decomposition rates and pathways between hypoxic and oxic coastal environments. Mar. Chem. 157, 67–77. doi: 10.1016/j.marchem.2013.08.003
Liu, Z., Sleighter, R. L., Zhong, J., and Hatcher, P. G. (2011). The chemical changes of DOM from black waters to coastal marine waters by HPLC combined with ultrahigh resolution mass spectrometry. Estuar. Coast. Shelf Sci. 92, 205–216. doi: 10.1016/j.ecss.2010.12.030
Lu, K., Gardner, W. S., and Liu, Z. (2018). Molecular structure characterization of riverine and coastal dissolved organic matter with ion mobility quadrupole time-of-flight LCMS (IM Q-TOF LCMS). Environ. Sci. Technol. 52, 7182–7191. doi: 10.1021/acs.est.8b00999
Ludwig, W., Probst, J.-L., and Kempe, S. (1996). Predicting the oceanic input of organic carbon by continental erosion. Glob. Biogeochem. Cycles 10, 23–41. doi: 10.1029/95GB02925
Majidzadeh, H., Uzun, H., Ruecker, A., Miller, D., Vernon, J., Zhang, H., et al. (2017). Extreme flooding mobilized dissolved organic matter from coastal forested wetlands. Biogeochemistry 136, 293–309. doi: 10.1007/s10533-017-0394-x
Mangal, V., Shi, Y. X., and Guéguen, C. (2017). Compositional changes and molecular transformations of dissolved organic matter during the arctic spring floods in the lower Churchill watershed (Northern Manitoba, Canada). Biogeochemistry 136, 151–165. doi: 10.1007/s10533-017-0388-8
Mangal, V., Stock, N. L., and Guéguen, C. (2016). Molecular characterization of phytoplankton dissolved organic matter (DOM) and sulfur components using high resolution Orbitrap mass spectrometry. Anal. Bioanal. Chem. 408, 1891–1900. doi: 10.1007/s00216-015-9295-9
May, J. C., Goodwin, C. R., Lareau, N. M., Leaptrot, K. L., Morris, C. B., Kurulugama, R. T., et al. (2014). Conformational ordering of biomolecules in the gas phase: nitrogen collision cross sections measured on a prototype high resolution drift tube ion mobility-mass spectrometer. Anal. Chem. 86, 2107–2116. doi: 10.1021/ac4038448
McGlynn, B. L., and McDonnell, J. J. (2003). Role of discrete landscape units in controlling catchment dissolved organic carbon dynamics. Water Resour. Res. 39:1090. doi: 10.1029/2002WR001525
Meybeck, M. (1993). Riverine transport of atmospheric carbon: sources, global typology and budget. Water Air Soil Pollut. 70, 443–463. doi: 10.1007/BF01105015
Mooney, R. F., and McClelland, J. W. (2012). Watershed export events and ecosystem responses in the mission–aransas national estuarine research reserve, South Texas. Estuar. Coasts 35, 1468–1485. doi: 10.1007/s12237-012-9537-4
Nesbit, T. A., and Mitsch, W. J. (2018). Hurricane and seasonal effects on hydrology and water quality of a subtropical urban stormwater wetland. Ecol. Eng. 120, 134–145. doi: 10.1016/j.ecoleng.2018.05.041
Nichols, C. M., Dodds, J. N., Rose, B. S., Picache, J. A., Morris, C. B., Codreanu, S. G., et al. (2018). Untargeted molecular discovery in primary metabolism: collision cross section as a molecular descriptor in ion mobility-mass spectrometry. Anal. Chem. 90, 14484–14492. doi: 10.1021/acs.analchem.8b04322
Ogawa, H., Amagai, Y., Koike, I., Kaiser, K., and Benner, R. (2001). Production of refractory dissolved organic matter by bacteria. Science 292, 917–920. doi: 10.1126/science.1057627
Ohno, T., and Ohno, P. E. (2013). Influence of heteroatom pre-selection on the molecular formula assignment of soil organic matter components determined by ultrahigh resolution mass spectrometry. Anal. Bioanal. Chem. 405, 3299–3306. doi: 10.1007/s00216-013-6734-3
Ohno, T., Sleighter, R. L., and Hatcher, P. G. (2016). Comparative study of organic matter chemical characterization using negative and positive mode electrospray ionization ultrahigh-resolution mass spectrometry. Anal. Bioanal. Chem. 408, 2497–2504. doi: 10.1007/s00216-016-9346-x
Osburn, C. L., Rudolph, J. C., Paerl, H. W., Hounshell, A. G., and Dam, B. R. V. (2019). Lingering carbon cycle effects of Hurricane Matthew in North Carolina’s coastal waters. Geophys. Res. Lett. 46, 2654–2661. doi: 10.1029/2019GL082014
Oss, M., Kruve, A., Herodes, K., and Leito, I. (2010). Electrospray ionization efficiency scale of organic compounds. Anal. Chem. 82, 2865–2872. doi: 10.1021/ac902856t
Peter, S., Shen, Y., Kaiser, K., Benner, R., and Durisch-Kaiser, E. (2012). Bioavailability and diagenetic state of dissolved organic matter in riparian groundwater. J. Geophys. Res. Biogeosci. 117:G04006. doi: 10.1029/2012JG002072
Rathgeb, A., Causon, T., Krachler, R., and Hann, S. (2017). From the peat bog to the estuarine mixing zone: common features and variances in riverine dissolved organic matter determined by non-targeted analysis. Mar. Chem. 194, 158–167. doi: 10.1016/j.marchem.2017.06.012
Raymond, P. A., and Bauer, J. E. (2001). Riverine export of aged terrestrial organic matter to the North Atlantic Ocean. Nature 409, 497–500. doi: 10.1038/35054034
Raymond, P. A., and Saiers, J. E. (2010). Event controlled DOC export from forested watersheds. Biogeochemistry 100, 197–209. doi: 10.1007/s10533-010-9416-7
Raymond, P. A., and Spencer, R. G. M. (2015). “Riverine DOM,” in Biogeochemistry of Marine Dissolved Organic Matter, Second Edn, eds D. A. Hansell, and C. A. Carlson, (Boston, MA: Academic Press), 509–533. doi: 10.1016/b978-0-12-405940-5.00011-x
Reyna, N. E., Hardison, A., and Liu, Z. (2017). Influence of major storm events on the quantity and composition of particulate organic matter and the phytoplankton community in a subtropical estuary, Texas. Front. Mar. Sci. 4:43.
Ridgwell, A., and Arndt, S. (2015). “Why Dissolved Organics Matter: DOC in Ancient Oceans and Past Climate Change,” in Biogeochemistry of Marine Dissolved Organic Matter, Second Edn, ed. D. A. H. A. Carlson, (Boston, MA: Academic Press), 1–20.
Seitzinger, S. P., Harrison, J. A., Dumont, E., Beusen, A. H. W., and Bouwman, A. F. (2005). Sources and delivery of carbon, nitrogen, and phosphorus to the coastal zone: an overview of Global Nutrient Export from Watersheds (NEWS) models and their application. Glob. Biogeochem. Cycles 19:GB4S01. doi: 10.1029/2005GB002606
Shen, Y., Fichot, C. G., and Benner, R. (2012). Floodplain influence on dissolved organic matter composition and export from the Mississippi—Atchafalaya River system to the Gulf of Mexico. Limnol. Oceanogr. 57, 1149–1160. doi: 10.4319/lo.2012.57.4.1149
Smith, C. A., Maille, G. O., Want, E. J., Qin, C., Trauger, S. A., Brandon, T. R., et al. (2005). METLIN: a metabolite mass spectral database. Ther. Drug Monit. 27, 747–751.
Spencer, R. G. M., Aiken, G. R., Wickland, K. P., Striegl, R. G., and Hernes, P. J. (2008). Seasonal and spatial variability in dissolved organic matter quantity and composition from the Yukon River basin, Alaska. Glob. Biogeochem. Cycles 22:GB4002. doi: 10.1029/2008GB003231
Spencer, R. G. M., Mann, P. J., Dittmar, T., Eglinton, T. I., McIntyre, C., Holmes, R. M., et al. (2016). Detecting the signature of permafrost thaw in Arctic rivers. J. Geophys. Res. Oceans 42, 2830–2835. doi: 10.1002/2015GL063498
Stubbins, A., Spencer, R. G. M., Chen, H., Hatcher, P. G., Mopper, K., Hernes, P. J., et al. (2010). Illuminated darkness: molecular signatures of Congo River dissolved organic matter and its photochemical alteration as revealed by ultrahigh precision mass spectrometry. Limnol. Oceanogr. 55, 1467–1477. doi: 10.4319/lo.2010.55.4.1467
Tfaily, M. M., Chu, R. K., Toliæ, N., Roscioli, K. M., Anderton, C. R., Paša-Toliæ, L., et al. (2015). Advanced solvent based methods for molecular characterization of soil organic matter by high-resolution mass spectrometry. Anal. Chem. 87, 5206–5215. doi: 10.1021/acs.analchem.5b00116
Tissot, B., Durand, B., Espitalie, J., and Combaz, A. (1974). Influence of nature and diagenesis of organic matter in formation of petroleum. AAPG Bull. 58, 499–506.
Vandenbroucke, M., and Largeau, C. (2007). Kerogen origin, evolution and structure. Org. Geochem. 38, 719–833. doi: 10.1016/j.orggeochem.2007.01.001
Vidon, P., Wagner, L. E., and Soyeux, E. (2008). Changes in the character of DOC in streams during storms in two Midwestern watersheds with contrasting land uses. Biogeochemistry 88, 257–270. doi: 10.1007/s10533-008-9207-6
Wagner, S., Fair, J. H., Matt, S., Hosen, J. D., Raymond, P., Saiers, J., et al. (2019). Molecular hysteresis: hydrologically driven changes in riverine dissolved organic matter chemistry during a storm event. J. Geophys. Res. Biogeosci. 124, 759–774. doi: 10.1029/2018JG004817
Ward, N. D., Richey, J. E., and Keil, R. G. (2012). Temporal variation in river nutrient and dissolved lignin phenol concentrations and the impact of storm events on nutrient loading to Hood Canal, Washington, USA. Biogeochemistry 111, 629–645. doi: 10.1007/s10533-012-9700-9
Zark, M., Christoffers, J., and Dittmar, T. (2017). Molecular properties of deep-sea dissolved organic matter are predictable by the central limit theorem: evidence from tandem FT-ICR-MS. Mar. Chem. 191, 9–15. doi: 10.1016/j.marchem.2017.02.005
Zark, M., and Dittmar, T. (2018). Universal molecular structures in natural dissolved organic matter. Nat. Commun. 9:3178. doi: 10.1038/s41467-018-05665-9
Keywords: riverine dissolved organic matter, hurricane harvey, high flow, liquid chromatography mass spectrometry, South Texas, structural isomers
Citation: Lu K and Liu Z (2019) Molecular Level Analysis Reveals Changes in Chemical Composition of Dissolved Organic Matter From South Texas Rivers After High Flow Events. Front. Mar. Sci. 6:673. doi: 10.3389/fmars.2019.00673
Received: 31 July 2019; Accepted: 16 October 2019;
Published: 07 November 2019.
Edited by:
Christopher Osburn, North Carolina State University, United StatesReviewed by:
Sasha Wagner, Rensselaer Polytechnic Institute, United StatesElizabeth Minor, University of Minnesota Duluth, United States
Copyright © 2019 Lu and Liu. This is an open-access article distributed under the terms of the Creative Commons Attribution License (CC BY). The use, distribution or reproduction in other forums is permitted, provided the original author(s) and the copyright owner(s) are credited and that the original publication in this journal is cited, in accordance with accepted academic practice. No use, distribution or reproduction is permitted which does not comply with these terms.
*Correspondence: Zhanfei Liu, zhanfei.liu@utexas.edu