- 1IRBIM – Institute for Marine Biological Resources and Biotechnologies, National Research Council, Mazara del Vallo, Italy
- 2School of Biological and Marine Sciences, Faculty of Science and Engineering, University of Plymouth, Plymouth, United Kingdom
- 3IAS – Institute of Anthropic Impacts and Sustainability in Marine Environment, National Research Council, Palermo, Italia
Marine ecosystems are being continually impacted by human activities and, among these, fisheries have been one of the most damaging. Fisheries modify the structure and functioning of food-webs through biomass removal and physical damage to the seabed, leading to loss of biodiversity and ecosystem services provided by the oceans. The ecosystem-based approach to fisheries is considered the most efficient way to achieve the goal of sustainable use of marine resources while allowing for biodiversity protection. The Strait of Sicily is a biologically important area of the central Mediterranean Sea characterized by high habitat complexity and rich biodiversity, however, due to the multispecific nature of local fisheries and weak implementation of the adopted management plans, this region is particularly vulnerable. We used fishery independent time series (1994–2016) to identify the main demersal assemblages and map their spatial distribution. The pressure of fishing effort on each of these defined assemblages was then quantified in order to evaluate the impact of bottom trawling on demersal communities. Our results showed four spatially distinct and temporally stable assemblages of the Strait of Sicily. These have a clear spatial distribution, different species composition and biodiversity values and are driven primarily by environmental gradients (i.e., mainly depth and, to a lesser extent, surface salinity). The demersal assemblages were subsequently grouped in homogeneous areas characterized by specific communities of commercial and non-commercial species and response to trawling impacts. These areas are proposed as Spatial Managements Units to evaluate and manage demersal mixed fisheries, while also considering biodiversity conservation in the central Mediterranean Sea.
Introduction
Marine ecosystems continue to be impacted significantly by human activities (e.g., pollution, overfishing, introduction of alien species, and global warming) which modify the marine food web and have drastic effects upon biodiversity (Worm et al., 2006). Among these, commercial fisheries represent potentially the most damaging activity and this pressure has increased over time (FAO, 2016). Fisheries generate impacts at the ecosystem level, by changing the structure and functioning of food webs through biomass removal, altering system productivity and destroying habitats through the physical impact of bottom-towed gear (Hinz, 2017). Consequently, fisheries have been responsible for biodiversity loss which compromises the oceans capacity to provide ecosystem services (e.g., food provisioning, maintaining water quality, carbon sequestration and recovery from perturbations) with detrimental impacts to people’s livelihoods and wellbeing (Worm et al., 2006; Liquete et al., 2013).
The ecosystem-based approach to fisheries (EAF) has been widely recognized as the most promising way to achieve the goal of sustainable resource exploitation while protecting biodiversity (Garcia and de Leiva Moreno, 2003). In Europe, the need to adopt this approach was highlighted during the most recent reform of the EU Common Fishery Policy in 2013 (European Commission, 2013). Nevertheless, the application of an ecosystem-based approach is strongly limited by appropriate management and policy measures (Cowan et al., 2012). In fact, there are relatively few case studies of successful implementation (Arkema et al., 2006), and often the original goals of sustainability and biodiversity conservation are not achieved due to conflicting political interests. Ecosystem models (Stecken and Failler, 2016) are mostly used to provide insights into the effects of fishing on the ecosystem, given that short-term advice on the status of stocks is still largely based on single species models (Hilborn, 2011; Fogarty, 2014). In this context, EAF considers the spatial dimension in assessing and managing fisheries that allows fishers to make a living while also targeting the conservation of marine resources (Bastardie et al., 2014; McGilliard et al., 2014; Khoukh and Maynou, 2018; Russo et al., 2019). The importance of including spatial aspects in resource management has been highlighted in the recent EU directive on Maritime Spatial Planning (MSP) (European Commission, 2014) that focuses on an ecosystem-based approach to promote the sustainable development and growth of maritime and coastal economies: known as “Blue Growth.” To achieve this goal, it is essential to have a detailed knowledge of the structure and function of marine ecosystems and the spatial distribution of the main commercial species in relation to the environment. This type of information represents a solid base from where the capacity of marine ecosystems to respond to human-induced changes can be understood and predicted. From this, an ad hoc approach to fisheries management that combines the principles of the EAF and MSP is feasible, identifying and classifying discrete areas characterized by distinct assemblages (in terms of species composition and environmental characteristics) and exposure to different fishing pressures. The identification of Spatial Management Units (SMUs) could be particularly important for supporting such fishery management policies, especially for regions characterized by mixed fisheries (i.e., where several species are caught in the same area with different gears), such as the Mediterranean Sea, but this requires robust data gathering on seabed habitats, assemblages and the associated fishing pressure.
The Strait of Sicily (Figure 1) is an ecologically important area of the central Mediterranean Sea that has high habitat complexity (Di Lorenzo et al., 2018). This region is key for biodiversity, defined as an Ecologically or Biologically Significant Area (EBSA) under the Convention on Biological Diversity (Bax et al., 2016). Here the main operating fishing fleets are the Italian bottom trawlers (395 vessels), with a production of about 13,000 tones and an economic value of €114 million in 2016 (Maiorano et al., 2019). The main demersal targeted species are deep water rose shrimp Parapenaeus longirostris (5,293 tones), giant red shrimp Aristaemorpha foliacea (1,490 tones) and hake Merluccius merluccius (1,373 tones); these three species alone were valued at €75.6 million in 2016 (Maiorano et al., 2019). Here some studies have shown that the spatial distribution of nursery grounds of commercial species seems quite stable over time, with juvenile fish favoring specific habitats (Garofalo et al., 2011a; Gristina et al., 2013; Colloca et al., 2015). In addition, the structure and composition of demersal assemblages appear to be impacted by different fisheries regimes (Gristina et al., 2006), but knowledge of the actual structure of the demersal assemblages and how these are shaped by natural and human-induced pressures is lacking. This information is necessary to regulate fishing effort spatially if there is to be a balance between the objectives of sustainable fisheries and biodiversity conservation, especially for a “blue growth” long-term strategy. The aims of the present study were twofold. First, we identified the main demersal assemblages in the Strait of Sicily, the major environmental drivers and tested for their temporal and spatial stability by using a long-term database covering 23 years (1994–2016). Second, we investigated the extent of fishing impact on each assemblage in terms of biodiversity. If different impacts are observed on each assemblage, it may be then possible to suggest them as Spatial Management Units for bottom trawling fisheries.
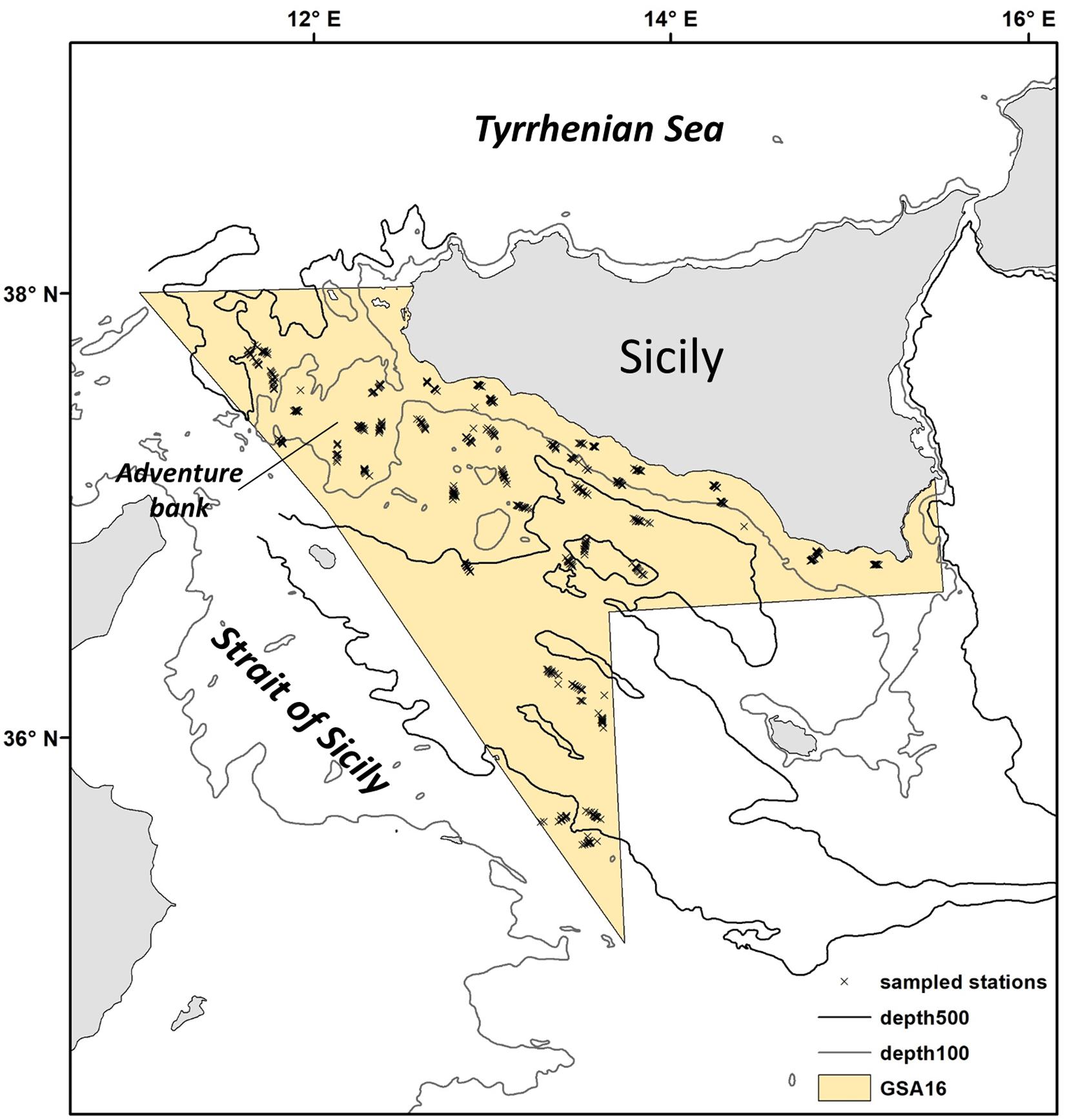
Figure 1. Location of the study region within the Strait of Sicily (Central Mediterranean Sea). This area corresponds to the Geographic Sub Area (GSA) 16. Trawl stations sampled during the MEDITS Survey are indicated with an x.
Materials and Methods
Ecological and Fishery Features of the Study Area
Our study area is situated in the central Mediterranean Sea and includes the northern side of the Strait of Sicily between 34°59′–38°00′N and 10°59′–15°18′E (Figure 1). This area corresponds to the Geographic Sub Area (GSA) 16 of the General Fisheries Commission for the Mediterranean (GCFM, 2007) and covers about 34,000 km2. It presents a varied seafloor morphology, including a shallow bank (Adventure Bank) in the western part (about 100 m depth), and deeper areas in the southeast (about 1800 m depth; Figure 2A). Bottom temperatures during spring/summer are warmer along the coast (13°C in average; Figure 2B), while the warmest surface waters (reaching about 24°C) are found in the southeast part of the region (Figure 2C). Highest primary productivity (Chla) is found along the southern coast of Sicily (Figure 2D). Sea surface salinity does not show a marked range in observed values during spring/summer, but higher values are apparent toward the east (Figure 2E). This region is influenced by a complex circulation pattern with several nutrient upwellings (cold deep-water that intrudes onto the shelf); these richer waters generate a large quantity of organic material that supports the food webs within coastal benthic and pelagic ecosystems (Agostini and Bakun, 2002; Di Lorenzo et al., 2018). All these environmental conditions contribute to an extremely highly productive region and a biodiversity hotspot for fish and invertebrates (Garofalo et al., 2007; Gristina et al., 2013; Lauria et al., 2015).
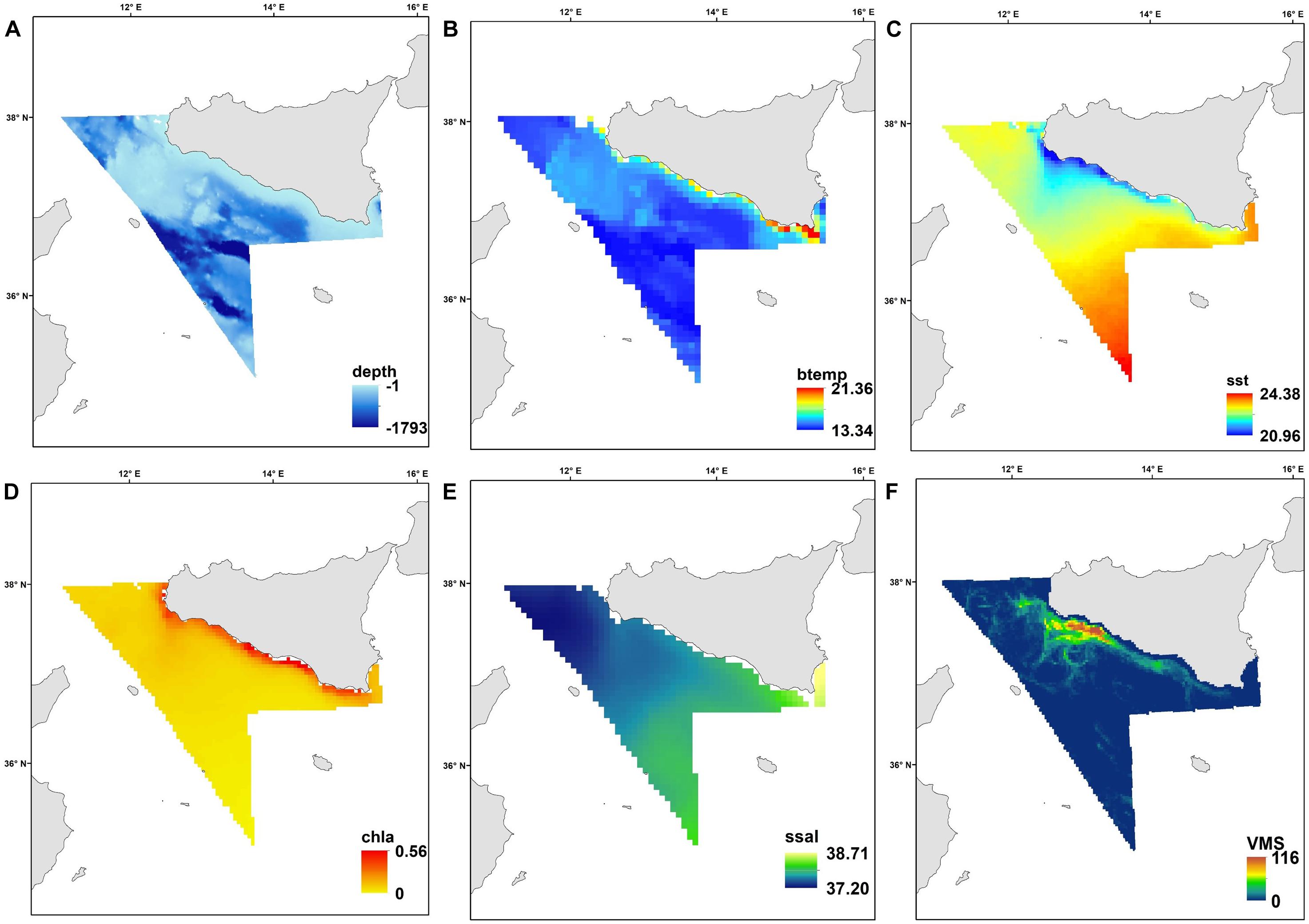
Figure 2. The spatial patterns of the environmental variables as average for the study period (1994–2016): (A) depth (m); (B) bottom temperature (°C); (C) sea surface temperature (°C); (D) chlorophyll a (mg/m3); (E) sea surface salinity (PSU), and (F) distribution of the fishing effort in terms of number of fishing hours/year (average 2009–2016).
The Strait of Sicily is important for bottom trawling and two main types of boats are identified (Fiorentino et al., 2013). Coastal trawlers (length overall -LOA between 12 and 24 m) undertake mainly short-distance fishing trips (which range from 1 to 2 days at sea) and exploit the grounds on the outer shelf/upper slope, while larger trawlers (LOA over 24 m) usually take longer voyages (up to 4 weeks) and go fishing further down in the Strait of Sicily. Usually, the smaller trawlers operating in more coastal waters follow a mixed fishery pattern, while large trawlers go to national and international waters within the Strait of Sicily, operating offshore both on the shallow banks and on the slope down to 700–800 m depth. During daytime, these larger trawlers traditionally target deep water rose shrimps and associated species, such as European hake (Merluccius merluccius), Norway lobster (Nephrops norvegicus), lesser flying squid (Todaropsis eblanae), white and black anglerfish (Lophius piscatorius and Lophius budegassa) and Rays. At night, the vessels move to shallow waters targeting mainly red mullet (Mullus spp.), sparids (Dentex spp., Pagrus spp. and Pagellus spp.) and cephalopods (octopuses, squids and cuttlefish). A fraction of these larger trawlers also specialized in fishing giant red shrimps at depths over 300 m. In recent years, the number of trawlers operating from Mazara del Vallo (the main fishing harbor of the south coast of Sicily) has decreased (see Supplementary Figure S1). Most of the larger trawlers targeting giant red shrimps have moved from Strait of Sicily to Aegean and Levant Sea (Eastern Mediterranean) (Garofalo et al., 2007) and the traditional shallow-water large trawler fishery that targeted mainly fish and cephalopods has progressively changed into a strictly shrimp-oriented fishery (Pinello et al., 2018).
Survey Design and Sampling
Since 1994, the Strait of Sicily has been surveyed under the Mediterranean International Trawl Survey program – MEDITS (Bertrand et al., 2002). Sampling is carried out annually in late spring/early summer and takes place in several areas of the Mediterranean Sea using a standardized methodology (Anonymous, 2013). It provides a long-term dataset of fishery-independent indices relating to abundance, demographic structure and spatial distribution of demersal species. Stations are sampled each year according to a stratified random sampling design based on five depth strata: 10–50 m, 51–100 m, 101–200 m, 200–500 m, 500–800 m. The number of hauls is proportional to the area of each stratum (Figure 1). A total of 34–120 stations (haul duration 30–60 min hauls; trawl speed 5.6 kmh–1) was sampled each year (Figure 1) on board the commercial trawler Sant’Anna. The gear was the GOV 73 bottom trawl net with a vertical opening ranging between 2 and 2.5 m and 20 mm side diamond stretched mesh in the cod-end. At each trawl station, the collected organisms were identified to species, weighed, counted and measured. A set of 114 species of bony fish, cephalopod, crustacean and elasmobranch was selected for analysis based on their ecological or commercial importance and the catchability of the sampling gear. Species density was calculated as the number of individuals per km2 (N/km2) (Table 1).
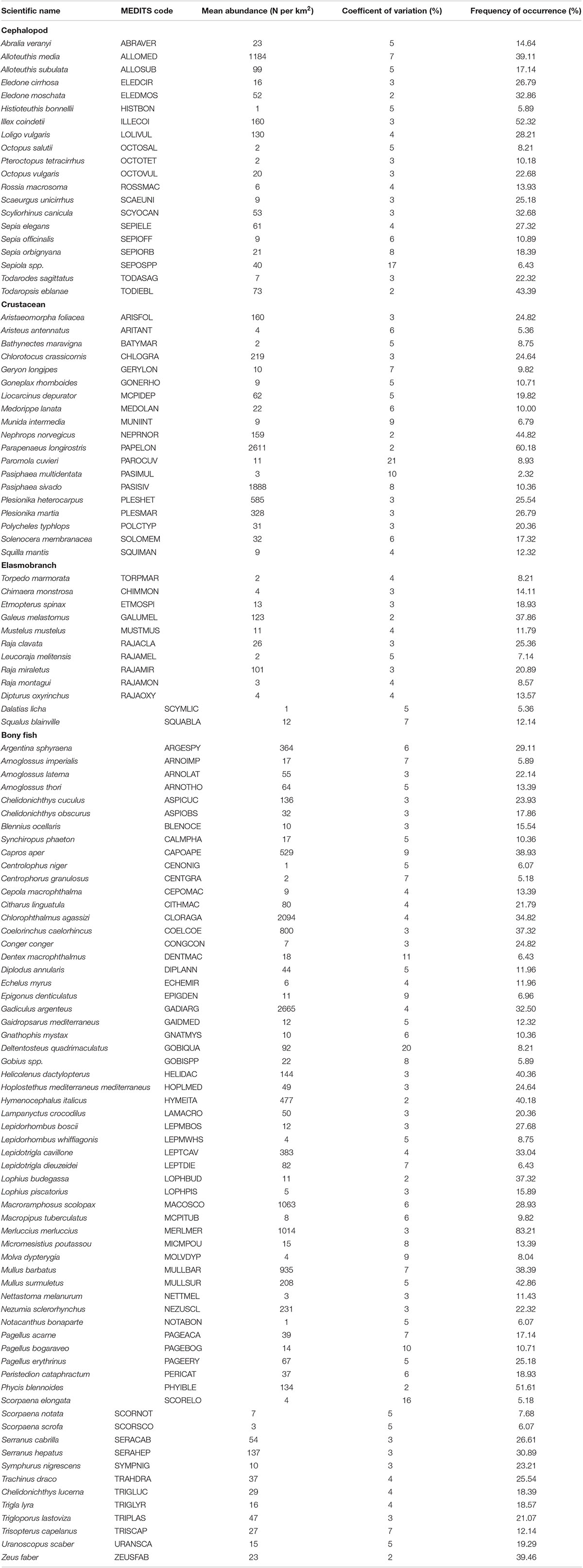
Table 1. Species list of the studied community including average species abundance, coefficient of variation and frequency of occurrence (as % of total number of observation n = 560) for the period 2002–2016.
Environmental and Fishery Data
Environmental variables included physical descriptors (depth) and oceanographic variables (sea surface temperature, sea bottom temperature, sea surface salinity and chlorophyll-a; Figure 2); these were collected from several sources (Table 2). Depth is one of the environmental gradients, linked to light and hydrodynamism, that mainly governs species spatial patterns (Tserpes et al., 2008; Lauria et al., 2011). For this study, bathymetric data were extracted from a re-projection of the MARSPEC database, a world ocean dataset developed for marine spatial ecology (Sbrocco and Barber, 2013). Data on primary productivity (Chl-a) and Sea Surface Temperature (sst) were obtained from existing Satellite imagery data. Monthly data were extracted from EOWEB1 (see Table 2 for details) and a seasonal average was calculated for each year from 1994 to 2016 (Figure 2). When the survey was undertaken across two seasons (e.g., spring-summer), the season that had the highest number of sampling days was chosen. Sea Surface salinity (ssal) and bottom temperature monthly values were extracted from the Ocean General Circulation Model (OGCM; database MyOceans)2 implemented for the Mediterranean Sea and a seasonal average was calculated for each year (Figures 2B,E). Maps of fishing pressure exerted by trawlers in the area were constructed using the data provided by the vessel monitoring system (VMS), the main geo-positioning device currently used to monitoring the spatio-temporal behavior of fishing vessels. The VMS data covering the years 2009–2016 were processed following the methodology described in Russo et al. (2013, 2014) and used to assess the spatial distribution of the fishing effort in terms of number of fishing hours per year (Figure 2F).
Analysis of Community Structure and Stability
As the number of sampled stations in GSA 16 during the MEDITS survey has steadily increased over time (from 34 sampled stations in 1994 to 120 in 2016), to study the temporal dynamics of the demersal assemblage it was necessary to identify the stations that have been regularly sampled every year for the entire study period. A total of 15 stations has been consistently sampled from 1994 to 2016 (22 years; Figure 3A), while from 2002 to 2016 (15 years) the number of repeatedly sampled stations was 59 (Figure 3B). The year 2014 was excluded from the analysis as the survey was conducted in autumn with a reduced number of hauls due to logistic constrains. Multivariate statistics were performed on each dataset (1994–2016 and 2002–2016) separately using the PRIMER v7 software (Clarke and Warwick, 2001). The underlying rationale of using both datasets is that, as they have different spatial and time scales, they can provide comparative information on the trajectory (evolution over time) of the demersal assemblage in the Strait of Sicily. Despite the 1994–2016 time series only having 15 constantly sampled stations, it still provides 22 years of data; such long time series are very valuable in ecological studies, and can capture changes that are not detectable by using shorter time series.
Abundance data were root-transformed to down-weight the importance of highly abundant species in analyses. For each year, a similarity matrix (Bray and Curtis, 1957) was constructed and Cluster analysis (visualized using a dendrogram) and SIMPROF applied to verify if different sites have similar species composition and whether assemblages could be defined. SIMPROF is a permutation test of the null hypothesis that a specified set of samples (which are not divided a priori into groups) do not differ from each other in a multivariate structure. Non-metric Multi-Dimensional Scaling (nMDS) was used to graphically represent the ordination in space of the sampled stations based on the Bray-Curtis similarity matrix. Finally, the SIMPER routine was applied to determine the species that explain most of the dissimilarity between the identified assemblages.
To evaluate stability of the assemblages over time (annual trend) in both time series, the similarity matrices were compared in pairs using the RELATE test. This seriation test assumes that adjacent years tend to be closest together in terms of their assemblage similarity (matching coefficient ρ) than years which are further apart in time. Under the null hypothesis that there is no relation whatsoever between the two similarity matrices, ρ will be approximately zero; conversely, if ρ is close to 1 the two resemblance matrices are highly related. Finally, the interannual trajectory of the demersal assemblage over the two study periods was examined by using a second stage MDS analysis. This routine allows comparison of the MDS plots constructed for each year (Clarke et al., 2006). Points that are close together on this plot indicate higher similarity between years while more distant points indicate a marked difference.
Community Relationship With Environment and Fisheries
To understand the relationship between assemblage composition and potential environmental drivers, further multivariate statistics were used. These were applied only to the 2002–2016 timeseries as it includes the highest number of sampled stations and it is spatially representative of our study area (Figure 3B). First, the five environmental variables were tested for collinearity (Variance Inflation Factor < 2; Zuur et al., 2007).
The overall spatial pattern of the demersal assemblages identified from the MEDITS survey data was correlated to the pattern of environmental data by using the BIOENV routine (Clarke et al., 2006). This routine permits the selection of the abiotic variable subset that maximizes the rank correlation (rho) between biotic and abiotic similarity matrices (illustrated by MDS analysis). This allows the identification of which environmental variable, or combination, provided the best correlation with the overall spatial pattern of the demersal assemblages (rho values close to 1 indicate strong agreement between environmental and biota patterns). Additionally, the DistLM routine (Clarke et al., 2006) was used to quantify the assemblage composition variation that is explained by the environmental variables (on a normalized scale; Warfe et al., 2013). The routine allows predictor variables to be fit individually or together in specified sets. P-values for testing the null hypothesis of no relationship (either for individual variables alone or conditional on other variables) are obtained using appropriate permutation methods. The most parsimonious model was selected using a stepwise regression and Akaike Information Criterion corrected for small sampling size (AICc). The distance-based redundancy analysis (dbRDA) plot was then applied to visualize the relative contributions of each of the predictor variables on the assemblage composition (Legendre and Anderson, 1999). To also test for a possible combined effect of fisheries and environment as potential drivers for assemblage structure and composition, the same analysis was applied to a shorter timeseries (2009–2016). This is because reliable VMS data were available only from 2009; these analyses were performed in PERMANOVA + (Anderson et al., 2008).
Pressure of Fisheries on the Assemblages and Identification of Spatial Management Units
Indicator kriging (Goovaerts, 1997) was used to create a probability map of assemblage distribution in the Strait of Sicily using the 2002–2016 dataset (as this one has the highest number of sampled stations and because the assemblages identified in this timeseries were comparable to the ones of the 1994–2016 timeseries). Each sampled station was converted to binary variables (1 for presence of a given assemblage and 0 for absence), each representing one assemblage. These data were used to fit theoretical models of spatial continuity and compute the parameters of experimental semivariograms (Table 1 and Supplementary Material). Hence, for each indicator variable (assemblage), ordinary kriging was applied to estimate values at non-sampled locations and mapping the probability of occurrence of community type at any given location (Garofalo et al., 2004; Vaz et al., 2007). Grids were constructed using 981 × 981 m cells and the projection used was the Albers Equal area conic. Cross-validation was undertaken to determine the difference between the measured and estimated values and to check the goodness of fit of several variogram models and the choice of kriging parameters. The best-fitting parameters were finally chosen to obtain the most accurate estimation. The distribution maps for the defined assemblages were then combined by multiplying each map by using raster calculator, selecting at each location the community type displaying the greatest probability of occurrence (Garofalo et al., 2004; Vaz et al., 2007). The final map of the spatial distribution of the demersal assemblages was overlain with the map of fishery effort in order to quantify the impact of bottom trawling on each assemblage.
To measure the diversity of each assemblage two indices were utilized: species richness and the Hill number N(1), or exponential of Shannon diversity, for each year of the 2002–2016 dataset. Species richness is an intuitive and universal applied index to measure biodiversity, but because it describes the community only in terms of number of species, it weights rare species the same of common ones. The Hill number (N1) also accounts for species abundance and have several advantages (e.g., they are all expressed in units of effective numbers of species, allow a comparison of the species diversity of different assemblages in time or space (Chao et al., 2013) and provide a unified framework for measuring biodiversity (Jost, 2006, 2007). ANOVA was used to test for significant differences between the diversity indices calculated individually for each assemblage. Any differences in fisheries and biodiversity were used to identify the Spatial Management Units.
Results
Analysis of Community Structure
The results of the cluster analysis and the SIMPROF test indicated that four assemblages can be identified in both datasets (1994–2016 and 2002–2016) as shown in Figure 4. These are defined as follows: Assemblage 1 between 10 and 70 m depth; Assemblage 2 from 70 to 250 m depth; Assemblage 3 between 250 and 450 m depth and Assemblage 4 from 400 to 800 m depth. The lists of the species that contribute to 60% of the composition of each assemblage are presented in Table 3 for both datasets (1994–2016 and 2002–2016).
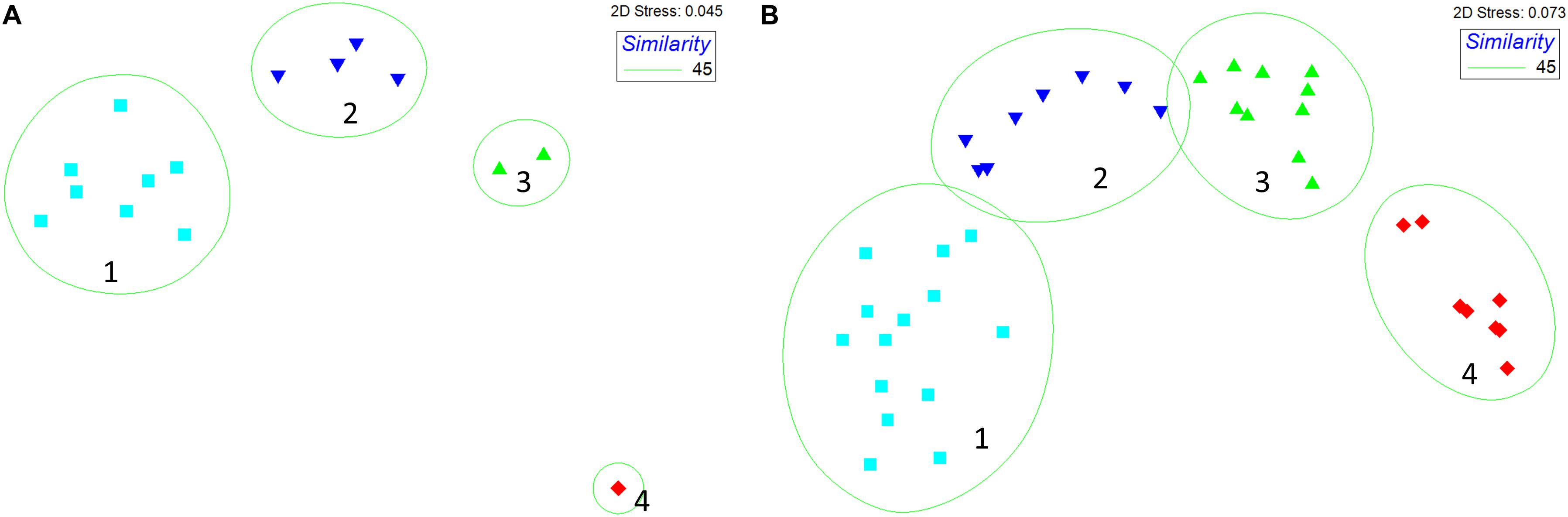
Figure 4. MDS ordination for both time periods (A) 1994–2016 (15 sites) and (B) 2002–2016 (59 sites). The green lines show the results of the cluster analysis overlaid to the MDS ordination. Abundance data were square root transformed Bray-Curtis similarity was calculated. For simplicity we are presenting only the plots relative to the average similarity matrix for each time series (that was constructed by averaging species abundances at each sampling stations across all years).
In general, it was observed that the composition of the assemblages was highly similar for the two time series as shown in Table 3. However, because the 2002–2016 timeseries has the highest number of sampled stations, we believe that this dataset provides the most thorough description of the species composition of each assemblage; therefore we will focus only on this in the following section.
The main species defining Assemblage 1 are those associated with the continental shelf. Among the fisheries target species we found here were red mullets (Mullus barbatus and M. surmuletus) which together contributed about 16% to similarities, followed by the squid Loligo vulgaris (about 6%) and European Hake (Merluccius merluccius) that contributes less than 5%. Other important species, but not exploited by fisheries are: Large-scaled Gurnard (Lepidotrigla cavillone; about 6%), the squid Alloteuthis media (5.5%) and the Brown Comber (Serranus hepatus; 4.4%) as shown in Table 3. The most important commercial species in terms of abundance in the Assemblage 2 is the deep-water rose shrimp (Parapenaeus longirostris), which contributes nearly 20%, followed by European Hake (M. merluccius; 12%) and the squid Illex coindetii (4.6%). Other species that are found well represented in this assemblage, but are not commercially important, are the squid A. media (9%), Longspine Snipefish (Macroramphosus scolopax; 8%), followed by the silvery pout (Gadiculus argenteus; 3%) and boarfish (Capros aper; 3%). Assemblage 3 is mainly formed by species that are not targeted by fisheries, for example the gadoid Silvery Pout (Gadiculus argenteus) that contributes 10%, the Hollowsnout grenadier (Coelorinchus caelorhincus) and the green shrimp (Chlorotocus crassicornis) that account for about 19% together. Within this assemblage are also found the valuable shrimp P. longirostris (9%) and squid (I. coindetii; about 5%). In contrast, the most important species of Assemblage 4 are the non-commercial fish Rough-tip Grenadier (Nezumia sclerorhynchus; 13%), Glasshead grenadier (Hymenocephalus italicus; 10%) and the elasmobranch Blackmouth Catshark (Galeus melastomus) that contributes 8%. Assemblage 4 is, however, also important for the highly prized giant red shrimp (Aristaeomorpha foliacea, 7%) and the shrimp Plesionika martia (about 11%), both species are the target of bottom trawling fisheries.
Temporal Evolution of the Community and Relationship With the Environment
The composition of the demersal community in the Strait of Sicily appears quite stable over time, as confirmed from the results of the RELATE test (Table 4), which show that the observed ρ is greater than any of the 999 simulated values, so that the null hypothesis (no tendency to seriation at all ρ = 0) is rejected. The trajectory analysis for the whole demersal community for the period 1994–2016 showed high similarity among all years, except for 1994 and 2002 (Figure 5A). Similarly, in the 2002–2016 time series all years appear to be similar with exception of 2002 (Figure 5B).
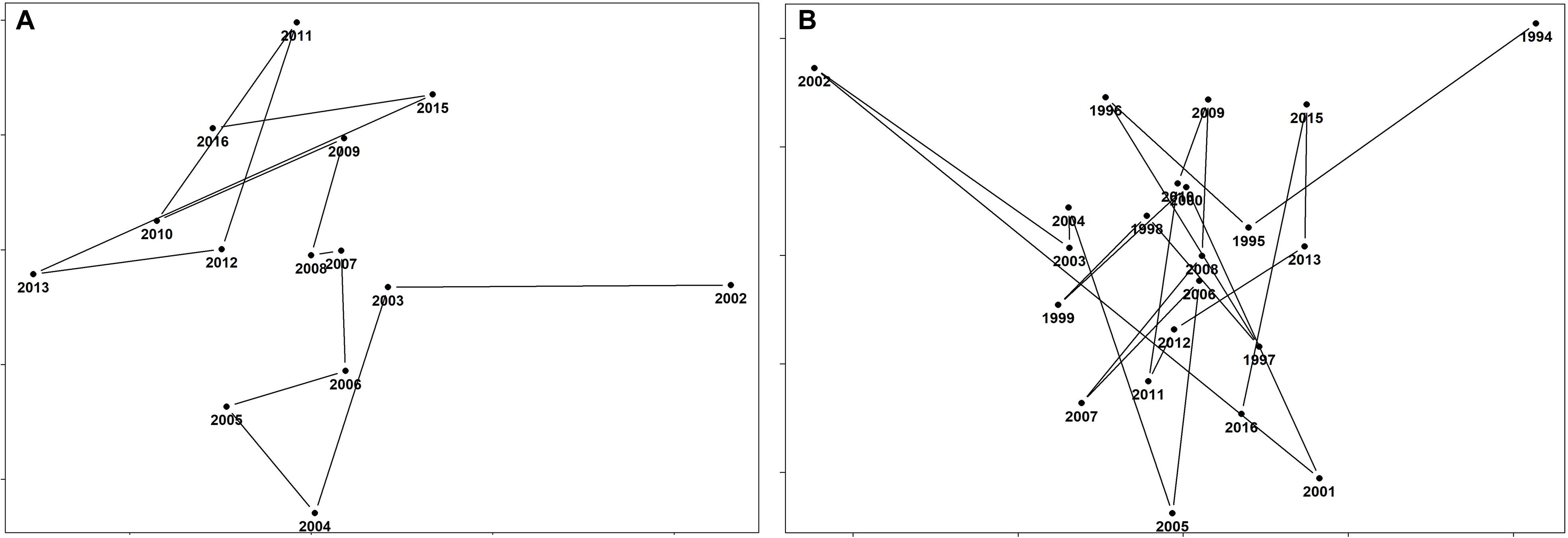
Figure 5. The temporal evolution of the demersal community for both time periods (A) 1994–2016 and (B) 2002–2016 for the Strait of Sicily. Years which are anomalous in terms of their spatial pattern stand out as outliers, while years with similar structure are clustered together.
When the composition of the demersal community was correlated to the pattern of the environmental variables using the BIOENV routine, perhaps unsurprisingly the results showed that the highest-rank correlation was found with depth (rho = 0.854). Similarly, the results of the DistLM analysis identified that 46.80% of the total variation observed in the spatial distribution of the demersal assemblage was explained by two environmental predictors: depth (p = 0.001) and sea surface salinity (p = 0.05) (Best model with AICc = 298.25). This result was reinforced by the two axes of the dbRDA plot, revealing that 45.5% of the total variation observed in the composition of the demersal assemblage of the Strait of Sicily is explained by depth and sea surface salinity (Figure 6). Depth therefore represents the most important environmental factor that determines the structure of the four assemblages (first axes explains 42.5% of the total variance; Figure 6). The effect of sea surface salinity is comparatively marginal and explains only 3% of the variance, probably because there is not a marked gradient of this environmental variable in the Strait of Sicily (Figure 2E). When the DistLM analysis was used for testing the combined effect of environment and fisheries on the spatial pattern of the demersal assemblages, still only two environmental variables (i.e., depth and surface salinity) appear to play an important role, thus excluding the role of fishing activities as a main driving factor (see Supplementary Figure S2).
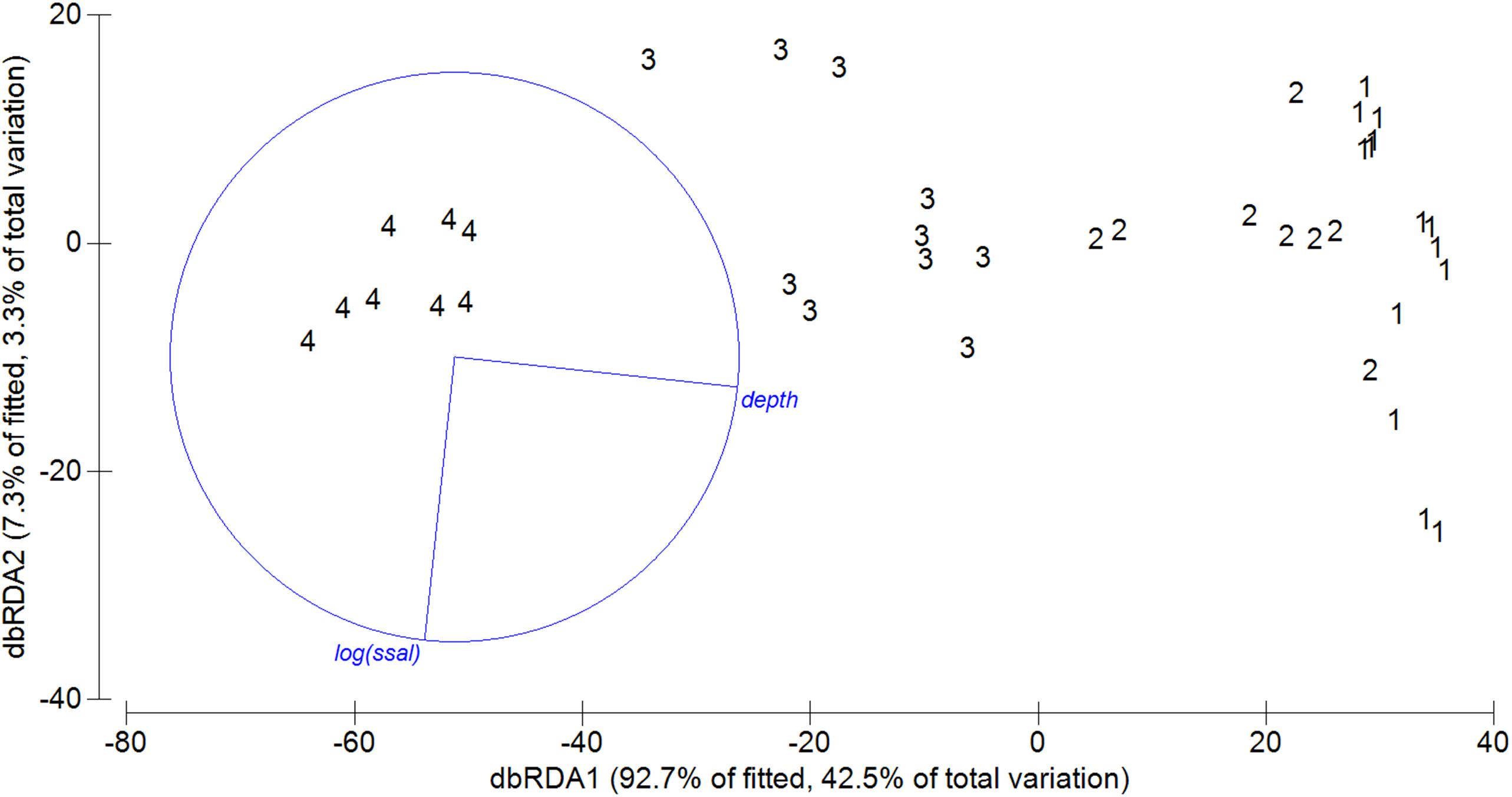
Figure 6. Distance based redundancy analyses (dbRDA) of demersal community abundance and environmental variables based on Bray Curtis similarity. Vectors indicate direction of the parameter effect in the ordination plot. Significant environmental variables of sequential test are shown in blue (p < 0.05).
Diversity Indices and Spatial Pattern of SMUs
The map presented in Figure 7A illustrates the spatial distribution of assemblages, including transition boundaries. As we expected when the fishing effort was quantified on each area (defined as the spatial distribution of each assemblage), marked differences emerged among them (Figure 7B). Fishing effort was higher on Assemblage 1 and Assemblage 2, with the latter showing a sharp increase since 2011. When biodiversity indices were compared among the assemblages, species richness was reasonably similar among them with exception of Assemblage 3 that showed a significant higher value (Table 5 and Figure 8A). Conversely, the lowest Hills number N1 was found for Assemblage 2, with the highest for the Assemblage 1 (Table 5 and Figure 8B). Because of these differences in fishery effort and biodiversity among assemblages, we identified four Spatial Management Units as shown in Figure 9.
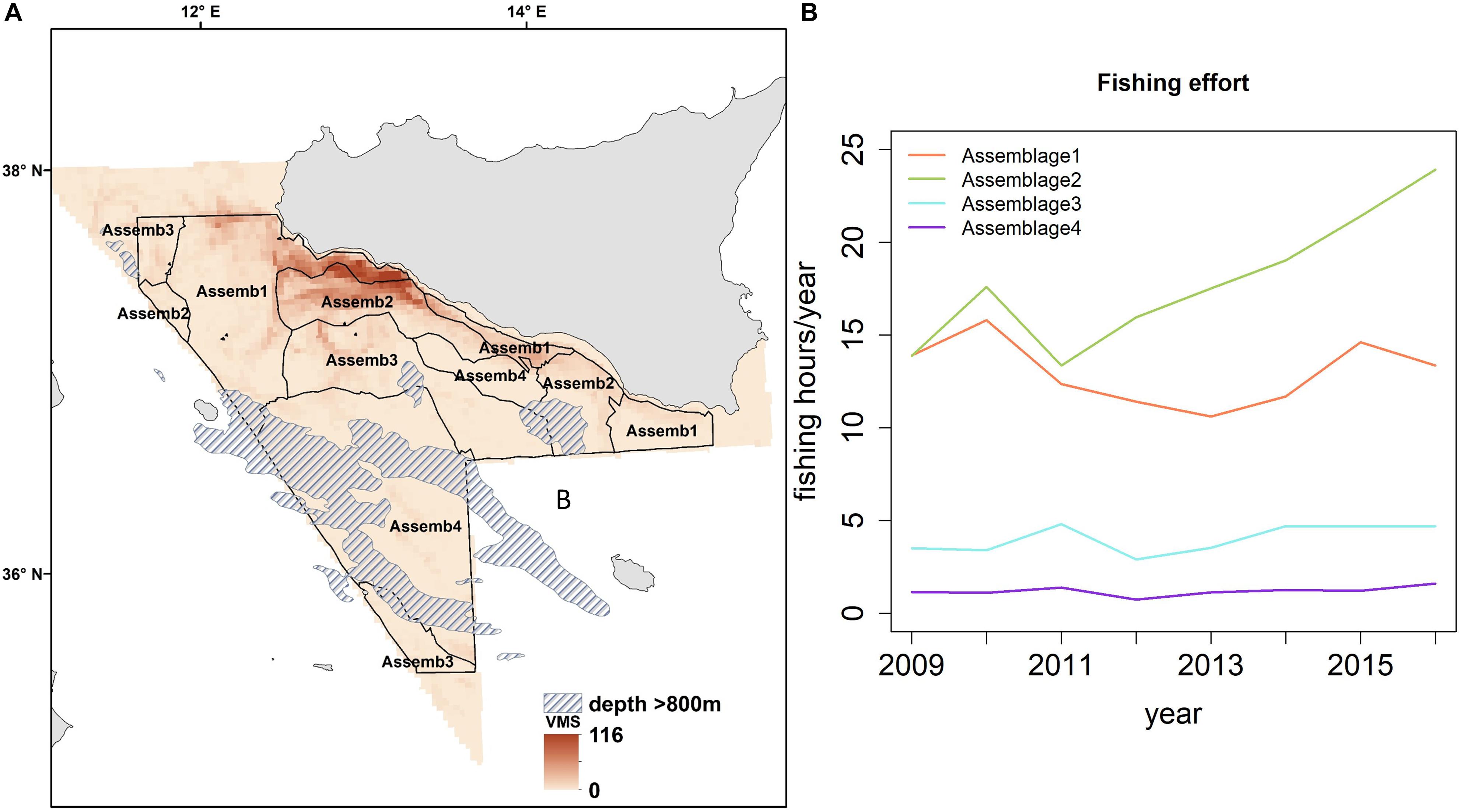
Figure 7. (A) Spatial distribution of the demersal assemblages in the central Mediterranean Sea based on data from 2002 to 2016. (B) Temporal trend of the fishing effort between 2009 and 2016 in terms of number of fishing hours/year in each of them.
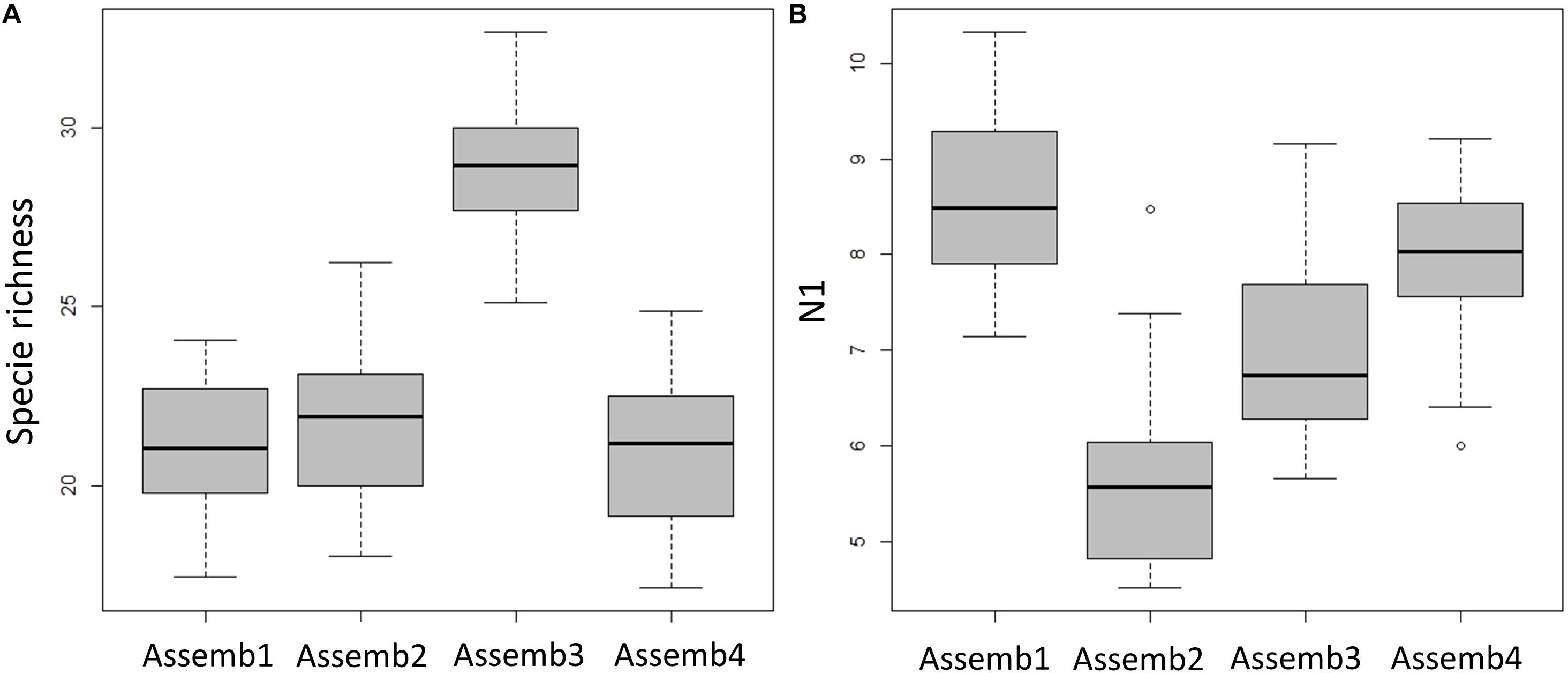
Figure 8. Diversity indices for each demersal assemblage are showed as boxplot. (A) Species richness (number of species) and (B) Hill’s number N1 (modified Shannon diversity).
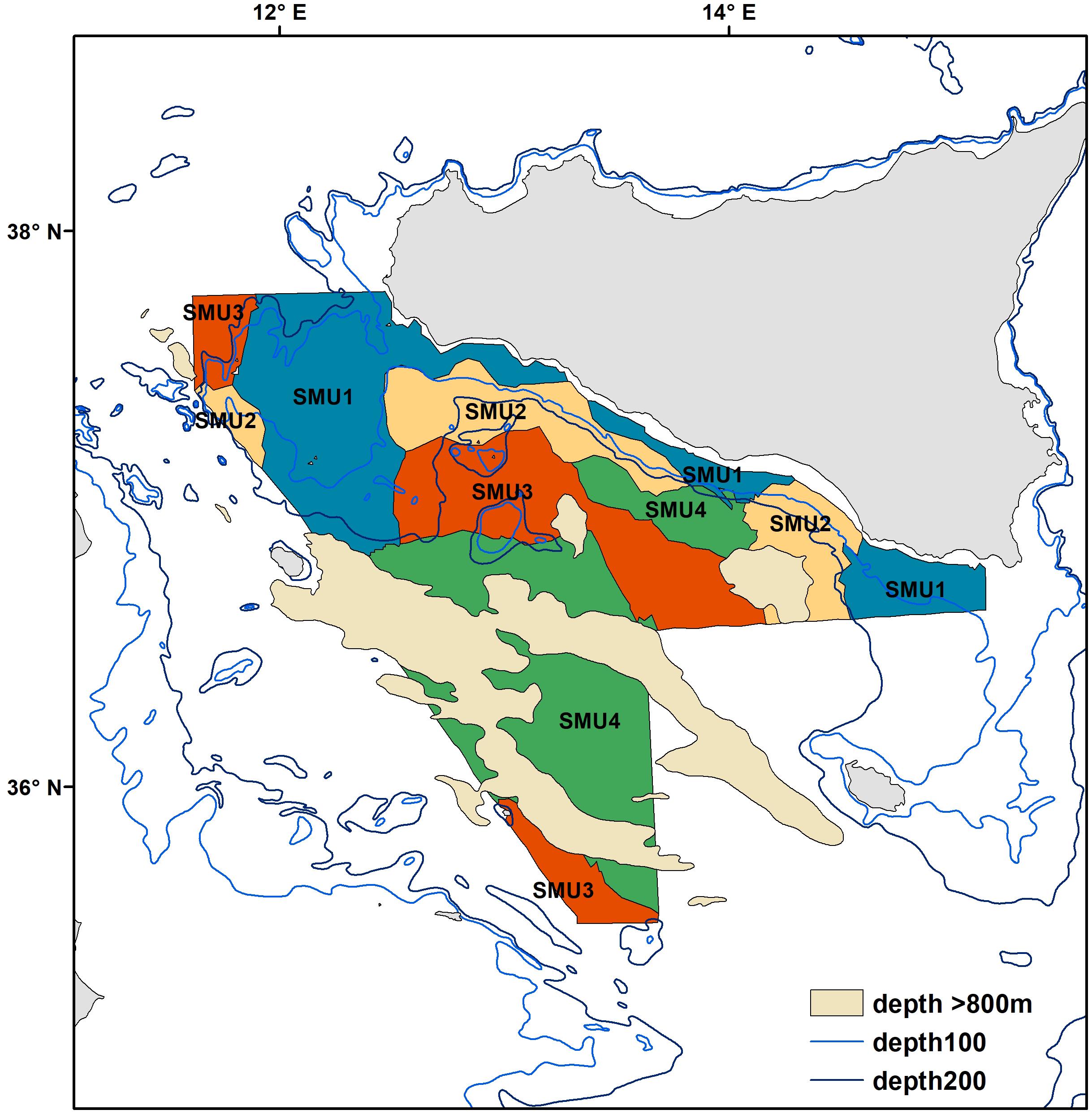
Figure 9. Spatial distribution of the proposed Spatial Management Units for the Strait of Sicily. The areas where depths are over 800 m are shaded in light yellow.
Discussion
The demersal community of the Strait of Sicily appears to be stable over time, which agrees with other long-term studies in the northeast Atlantic that have shown temporal stability of fish assemblages in terms of composition and spatial distribution (Sousa et al., 2005; Vaz et al., 2007). The main environmental drivers that are related to demersal assemblage structure are depth and, to a lesser extent, sea surface salinity (Figure 6). This is similar to several marine ecosystems from the northeast Atlantic (i.e., North Sea and Iceland) to the Mediterranean Sea where spatial variation in bathymetry and hydrographical regimes influences the structure and distribution of the demersal fish community (Ehrich et al., 2009; Stefansdottir et al., 2010; García-Rodríguez et al., 2011). Usually demersal assemblages are temporally stable when a specific spatial distribution that is closely linked to depth is apparent (Gaertner et al., 2002; Colloca et al., 2003; Massutí and Renones, 2005; Garces et al., 2006; Labropoulou and Papaconstantinou, 2008). A marginal effect of sea surface salinity was also detected, but because of its narrow range in our study area (Figure 2E) we believe that, in our case, this environmental factor is not a main driving force, though it has been suggested to be locally important for the eastern Mediterranean fish community (i.e., North Aegean Sea; Labropoulou and Papaconstantinou, 2008). The analysis of the temporal evolution (or trajectory) of the demersal community suggests a high similarity among years (from 1994 to 2016). This implies that there have not been major changes in the community, despite multidecadal variability in oceanography (Marbà et al., 2015). However, our results for both the time periods (1994–2016 and 2002–2016) evidenced that 2002 seems to be marginally different from the other years (Figure 5); this might be explained by biotic interactions between species that are often challenging to predict (Kraft et al., 2015) as there is no explanatory environmental variation. Despite our results indicate the stability of demersal assemblages during the 1994–2016 period, it is worth acknowledging that previous studies have showed that demersal fisheries in the Strait of Sicily were not sustainable during the decades prior our study period (Levi and Andreoli, 1989). In particular, this region experienced a strong increase of fishing capacity from the 1970s to the early 1980s, with main target species being fished over the Maximum Sustainable Yield (Levi et al., 1993, 1995; Ragonese and Bianchini, 1996). This overfishing, varying in severity among species, has been confirmed by the GFCM working groups for the main target species up in recent years.
Our results identify four spatially distinct and temporally persistent demersal assemblages (Figure 4). Because of their diverse composition of target species of high economic value, and the varied fisheries pressure and impacts (Figures 7, 8), these are suggested as Spatial Management Units for bottom trawling in the central Mediterranean Sea (Figure 9). Our results show that SMU1 (10–70 m depth) is found from the shallow waters of south coast of Sicily from the Adventure bank to the Malta bank. Here the main commercial species are the ones associated with the continental shelf (see Table 3 for details). This SMU is particularly important because it includes the main nurseries of coastal species (such as M. barbatus and Pagellus erythrinus; Garofalo et al., 2011a; Gristina et al., 2013; Colloca et al., 2015). SMU1 has been historically exploited by bottom-towed fisheries and fishing effort remains quite high compared to other parts of the Strait of Sicily (Figure 7B). In terms of biodiversity, SMU1 has a species richness comparable to other parts of our study area, but the highest values of the Hills N1 index that accounts for both number of species and equitability (Figure 8). This suggests that the impact of fisheries is not significantly modifying the community structure over the time period studied. The high diversity found in SMU1 agrees with Garofalo et al. (2007), who related the patterns of diversity to the specific hydrographic conditions and habitat complexity of the Strait of Sicily, resulting in a persistent area of high fish diversity over the Adventure Bank. Other studies have shown that the spatial patterns of demersal fish diversity associated to the continental shelf and upper/lower slope in the Mediterranean are dependent on differences in the distribution of cumulative threats to marine biodiversity, which are mainly concentrated in coastal areas and on the continental shelf of the Mediterranean (Danovaro et al., 2010; Coll et al., 2012; Farriols et al., 2019). A generally stable, or a slight recovering, trend has been highlighted for some areas of the Mediterranean Sea (see Farriols et al., 2019), which further confirms our findings for SMU1.
Moving away from land toward the continental slope, SMU2 is found between 70 and 250 m depth (Figure 9); here the species composition is dominated by the valuable deep-water rose shrimp that shows a persistent spawning ground located on the south-eastern side of the Adventure Bank (Fortibuoni et al., 2010). A recent study showed that this fishery produces lower level of discards compared to other local areas (Milisenda et al., 2017), probably because the intense exploitation of these grounds reduced the abundance of other species with biological traits more sensitive to the fishing pressure than shrimps, such as many large fish. SMU2 is also characterized by another commercially important species – the European Hake (M. merluccius; Table 3). Here recruits generally are distributed over a wide bathymetric range (50–600 m), with a concentration peak at 250 m depth (Garofalo et al., 2011b). In this region, both hake and deep-water rose shrimp show a full overlap of their nurseries which is persistent over time (Garofalo et al., 2011a). Because of these productive shrimp grounds, the trawling effort in SMU2 has sharply increased over time (Figure 7B). This is likely to have had a negative impact on the biodiversity of SMU2 that shows the lowest value within the whole study area (Figure 6B and Table 4). In the Mediterranean, marine ecosystem species richness decreases with depth because of a drop in system productivity, but in some cases a peak can be observed at intermediate depths (around 1000 m depth; Coll et al., 2010). In our case, we suggest that the differences in diversity among the SMUs of the Strait of Sicily (Figure 8) are most likely influenced by changes in fishing effort and fisher behavior. This sharp decline of biodiversity recorded in intermediate waters between 70 and 250 m depth could be related to the increased pressure of trawlers targeting deep water rose shrimp in SMU2.
SMU3 is identified from 250 to 450 m depth (Figure 9), with the main commercial species being crustaceans: the deep water rose shrimp (P. longirostris) and the Norway lobster (Nephrops norvegicus). This area is particularly important because of the presence of Norway lobster nurseries and high density of juvenile fish (Garofalo et al., 2011a; Gristina et al., 2013). In terms of diversity patterns, SMU3 has the highest number of species (Figure 8A). This result could be explained by the fact that most large trawlers that used to fish the giant red shrimps in this part of the Strait of Sicily have moved out toward more productive fishing grounds (outside the GSA 16) ranging from the Sardinia Channel to the Gulf of Sirte, Aegean and Levant Sea (Pinello et al., 2018). This spatial re-distribution of the trawlers has resulted in a reduction of fishing pressure on deep water grounds of the Sicily area, which probably explains the higher biodiversity observed. Increasing levels of disturbance have generally been considered to decrease diversity, species richness and evenness, and this has been observed in many demersal communities exposed to intense fishing effort (Rijnsdorp et al., 1996; Jennings and Kaiser, 1998; Jennings et al., 1999). This negative relationship between biodiversity and fishing pressure seems to explain the results observed for SMU3. This area is particularly important because is associated with the deep-sea pennatulid Funiculina quadrangularis, an indicator of Vulnerable Marine Ecosystems (Lauria et al., 2017). This is considered one of the most sensitive sea pen species to trawling and in the northeast Atlantic and Mediterranean Sea is associated with N. norvegicus fisheries (Rogers and Gianni, 2010; Martinelli et al., 2013). It appears clear that specific measures to manage deep-sea fisheries targeting valuable crustaceans are necessary in SMU3, especially in the light of the importance that this area has for VME and biodiversity (Watson and Morato, 2013).
SMU4 covers areas of deeper waters between 400 and 800 m (Figure 7A) and is characterized by the highly prized crustaceans Giant red shrimp (A. foliacea) and the Golden shrimp (P. martia) and many non-commercial species including the Blackmouth Catshark (Galeus melastomus; Table 3). Persistent nurseries of A. foliacea have been previously described in SMU4 (Garofalo et al., 2011a; Colloca et al., 2015). Furthermore, this SMU has been identified as one of the biodiversity hot-spots for elasmobranchs in the Mediterranean Sea (Lauria et al., 2015). Due to their high vulnerability to fisheries, the presence of elasmobranchs and long living benthopelagic fish (such as the Silver Roughy Hoplostethus mediterraneus; Vitale et al., 2014) potentially indicates a lower fishing impact in SMU4 compared to other parts of our study area. These results agree with Gristina et al. (2006), who suggested that areas subjected to lower fishing intensity have a fish community where sensitive species such as elasmobranchs are well represented. We suggest that SMU4 might be an area where the elasmobranch assemblage is recovering, probably because of reported changes in fishing pattern in the region. Although fishing effort in this SMU is lower compared to the other SMUs (Figure 7B), it appears necessary to have management approaches that account for the recovery of elasmobranchs and giant red shrimp fishery.
Conclusion and Further Developments
The idea to manage trawling in mixed fisheries by adopting a multispecies approach that considers the assemblage, and not the single species, as the basic unit is not new (Massutí and Renones, 2005; Garces et al., 2006; Busalacchi et al., 2010). However, most previous work has focused mainly on the identification of structure and distribution of assemblages, without attempting to assess the impact of fisheries and propose some management measures. Here we propose an approach in line with spatially-based management in a region of the Mediterranean Sea highly exploited by mixed-species bottom trawling, combining information on assemblage characteristics with an estimate of trawling effort affecting them. This aimed to identify and suggest SMUs for assessment and management of the multispecies fisheries, while considering biodiversity as a main parameter related to impact of fisheries. In addition, SMUs can be viewed in the light of long-term strategies for the Blue Economy, especially because they can benefit both the local economy and marine conservation.
This study shows that in the Strait of Sicily there are marked differences in terms of target species for bottom-towed fisheries and biodiversity at a local scale, evidencing the necessity to adopt management plans that account for this, especially because selectivity of bottom trawlers is poor. The differences in diversity observed among the SMUs suggest that all of them have experienced different conditions over the years, probably as response of modification of fisher behavior across the whole region. According to the EU Marine Strategy Directive, it is necessary to achieve Good Environmental Status (GES) of the EU’s marine waters by 2020 and to protect the resource base upon which marine-related economic and social activities depend. However, having a deep local knowledge of the area can be useful to minimize the discrepancies between conservation and fishery yield goals. The identification of SMUs defined as geographic areas with a persistent biological composition and subjected to a known fishing pressure, represents a first step to incorporating an ecosystem approach into practical fishery management framework. The obvious next step of our research will focus on the analysis of the productivity of assemblages in terms of commercial species and the size-spectrum composition for each SMU over time in order to provide robust indications for different management strategies. From a general point of view, the loss of biodiversity is related to a reduced capacity of the oceans to provide food, maintain a high environmental quality and be resilient to perturbations (Worm et al., 2006). However, in some specific cases intense fishing activity can result in a simplification of the community on fishing grounds that reduces the abundance of competitors and predators (e.g., SMU2), which can directly skew productivity of fished grounds toward species of greater commercial value (Brander, 2010). The experience of the shrimp fishers in different areas of the Mediterranean Sea suggests that the reduction of predatory species and biodiversity in fishing grounds generally increases shrimp catch (Milisenda et al., 2017). Our results highlight the need to apply a fishery management approach that accounts for both the fishers’ economical profit but also includes the protection of biodiversity. For example, a way to achieve this goal could be leaving some grounds unfished for periods of time in order to rebuild marine biodiversity, potentially allowing spatial management of fishing activities with a strong impact on habitats, such as trawling, in which grounds allocated to fisheries alternate with grounds assigned to conservation. The positive effect of protection on marine species and biodiversity from fishing activities within a given area has been demonstrated to increase the economic benefits of stakeholders (Sala et al., 2019), although it is difficult to understand what the effect of this protection on adjacent areas might be where fisheries are allowed (Hilborn, 2014). However, the positive effects of spill-over from Marine Managed Areas to adjacent ones, which is one of the cornerstones of the spatial management of fishing resources, has been already showed in the north coast of Sicily, where a significant increase in the abundance of target species occurred after the application of a trawling ban in 1990 (Pipitone et al., 2000, 2014; Potoschi et al., 2006). Recently Russo et al. (2019) suggested that the application of spatial management plans based on different sets of spatial/temporal fishing closures led to a recovery of exploited fish stocks, with a decrease in profit for the fleet which was much lower than the one expected under a simple reduction of fishing effort. The management approach suggested in this study is in line with the ideas at the basis of European Marine Spatial Planning Directive. It aims to optimize, on adequate spatial scales, the ratio between productivity of fisheries resources in the fishing grounds and the overall good ecological status of communities and the environment in a large area containing mixed fishing grounds.
Data Availability Statement
The datasets generated for this study are available on request to the corresponding author.
Author Contributions
VL, MG, FF, MA, and GG conceived, designed, and wrote the manuscript. VL and GG analyzed the data. MG, FF, and MA contributed to the analysis.
Funding
This study was carried out within the project MADAMES “What is where? Mapping the habitat use of demersal assemblages to aid conservation of marine biodiversity in the central Mediterranean Sea” project code 2015-PDR-0204 funded by the Fondazione con il Sud (Rome). Fisheries data were collected within the DCR and DCF supported by the Italian Ministry of Agricultural, Food and Forestry Policies and the European Union.
Conflict of Interest
The authors declare that the research was conducted in the absence of any commercial or financial relationships that could be construed as a potential conflict of interest.
Acknowledgments
We thank Tommaso Russo for his support on VMS data and all the technical staff of CNR-IRBIM SS of Mazara del Vallo (Italy) involved in data collection and processing.
Supplementary Material
The Supplementary Material for this article can be found online at: https://www.frontiersin.org/articles/10.3389/fmars.2020.00233/full#supplementary-material
Footnotes
References
Agostini, V. N., and Bakun, A. (2002). Ocean triads in the Mediterranean Sea: physical mechanisms potentially structuring reproductive habitat suitability (example application to European anchovy, Engraulis encrasicolus). Fish. Oceanogr. 11, 128–142.
Anderson, M., Gorley, R. N., and Clarke, K. R. (2008). PERMANOVA for PRIMER: Guide to Software and Statistical Methods. Plymouth: PRIMER-E.
Arkema, K. K., Abramson, S. C., Dewsbury, B. M., Arkema, K. K., Abramson, S. C., and Dewsbury, B. M. (2006). Marine ecosystem-based management: from? characterization to implementation. Front. Ecol. Environ. 4, 525–532. doi: 10.1890/1540-9295(2006)4[525:memfct]2.0.co;2
Bastardie, F., Nielsen, J. R., Eigaard, O. R., Fock, H. O., Jonsson, P., and Bartolino, V. (2014). Competition for marine space: modelling the Baltic Sea fisheries and effort displacement under spatial restrictions. ICES J. Mar. Sci. 72, 824–840. doi: 10.1093/icesjms/fsu215
Bax, N. J., Cleary, J., Donnelly, B., Dunn, D. C., Dunstan, P. K., Fuller, N., et al. (2016). Results of efforts by the Convention on Biological Diversity to describe ecologically or biologically significant marine areas. Conserv. Biol. 30, 571–581. doi: 10.1111/cobi.12649
Bertrand, J., de Sola, L., Papaconstantinou, C., Relini, G., and Souplet, A. (2002). The general specifications of the MEDITS surveys. Sci. Mar. 66, 9–17.
Brander, K. (2010). Reconciling biodiversity conservation and marine capture fisheries production. Curr. Opin. Environ. Suistanabil. 2, 416–421. doi: 10.1016/j.cosust.2010.09.003
Bray, J. R., and Curtis, J. (1957). An ordination of the upland forest communities of Southern Wisconsin. Ecol. Monogr. 7, 325–347.
Busalacchi, B., Rinelli, P., De Domenico, F., Profeta, A., Perdichizzi, F., and Bottari, T. (2010). Analysis of demersal fish assemblages off the Southern Tyrrhenian Sea (central Mediterranean). Hydrobiologia 654, 111–124. doi: 10.1007/s10750-010-0374-9
Chao, A., Ellison, A. M., Colwell, R. K., Gotelli, N. J., Sander, E. L., Hsieh, T. C., et al. (2013). Rarefaction and extrapolation with Hill numbers: a framework for sampling and estimation in species diversity studies. Ecol. Monogr. 84, 45–67. doi: 10.1890/13-0133.1
Clarke, K. R., Somerfield, P. J., Airoldi, L., and Warwick, R. M. (2006). Exploring interactions by second-stage community analyses. J. Exp. Mar. Bio. Ecol. 338, 179–192. doi: 10.1016/j.jembe.2006.06.019
Clarke, K. R., and Warwick, R. M. (2001). Change in Marine Communities: An Approach to Statistical Analysis and Interpretation. Plymouth: PRIMER-E Ltd.
Coll, M., Piroddi, C., Albouy, C., Lasram, F. B. R., Cheung, W., Christensen, V., et al. (2012). The Mediterranean Sea under siege: spatial overlap between marine biodiversity, cumulative threats and marine reserves. Glob. Ecol. Biogeogr. 21, 465–480. doi: 10.1111/j.1466-8238.2011.00697.x
Coll, M., Piroddi, C., Steenbeek, J., Kaschner, K., Ben, F., Lasram, R., et al. (2010). The biodiversity of the mediterranean sea: estimates, patterns, and threats. PLoS One 5:e11842. doi: 10.1371/journal.pone.0011842
Colloca, F., Cardinale, M., Belluscio, A., and Ardizzone, G. (2003). Pattern of distribution and diversity of demersal assemblages in the central Mediterranean sea. Estuar. Coast. Shelf Sci. 56, 469–480. doi: 10.1016/S0272-7714(02)00196-8
Colloca, F., Garofalo, G., Bitetto, I., Facchini, M. T., Grati, F., Martiradonna, A., et al. (2015). The seascape of demersal fish nursery areas in the north mediterranean sea, a first step towards the implementation of spatial planning for Trawl fisheries. PLoS One 10:e0119590. doi: 10.1371/journal.pone.0119590
Cowan, J. H., Jake, J., Waltersray, C. R. J., Ray, H., Essington, T. E., and John, W. Jr., et al. (2012). Challenges for implementing an ecosystem approach to fisheries management challenges for implementing an ecosystem approach to fisheries management. Mar. Coast. Fish. 4, 496–510. doi: 10.1080/19425120.2012.690825
Danovaro, R., Company, J. B., Corinaldesi, C., D’Onghia, G., Galil, B., Gambi, C., et al. (2010). Deep-sea biodiversity in the Mediterranean Sea: the known, the unknown, and the unknowable. PLoS One 5:e0011832. doi: 10.1371/journal.pone.0011832
Di Lorenzo, M., Sinerchia, M., and Colloca, F. (2018). The North sector of the strait of sicily: a priority area for conservation in the Mediterranean Sea. Hydrobiologia 821, 235–253. doi: 10.1007/s10750-017-3389-7
Ehrich, S., Stelzenmüller, V., and Adlerstein, S. (2009). Linking spatial pattern of bottom fish assemblages with water masses in the North Sea. Fish. Oceanogr. 18, 36–50. doi: 10.1111/j.1365-2419.2008.00495.x
European Commission (2013). Regulation (EU) No 1380/2013 of the European Parliament and of the Council of 11 December 2013 on the Common Fisheries Policy. Brussels: European Commission.
European Commission (2014). Directive 2014/89/EU of the European Parliament and of the Council of 23 July 2014 establishing a framework for maritime spatial planning. OJL 257, 135–145.
Farriols, M. T., Ordines, F., Carbonara, P., Casciaro, L., Isajlovic, I., Jadaud, A., et al. (2019). Spatio-temporal trends in diversity of demersal fish assemblages in the Mediterranean. Sci. Mar. 2019, 189–206. doi: 10.3989/scimar.04977.13A
Fiorentino, F., Ben Hadj Hamida, O., Ben Meriem, S., Gaamour, A., Gristina, M., Jarboui, O., et al. (2013). Synthesis of Information on Some Demersal Crustaceans Relevant for Fisheries Target Species in the South-Central Mediterranean Sea (GCP/RER/010/ITA/MSM-TD-32). Technical Documents, No. 32. Rome: MedSudMed, 120.
Fogarty, M. J. (2014). The art of ecosystem-based fishery management. Can. J. Fish. Aquat. Sci. 71, 479–490. doi: 10.1139/cjfas-2013-0203
Fortibuoni, T., Bahri, T., Camilleri, M., Garofalo, G., Gristina, M., and Fiorentino, F. (2010). Nursery and spawning areas of deep-water rose shrimp, Parapenaeus longirostris (Decapoda: Penaeidae), in the strait of sicily (Central Mediterranean Sea). J. Crustac. Biol. 30, 167–174. doi: 10.1651/09-3167.1
Gaertner, J. C., Bertrand, J. A., and Souplet, A. (2002). STATIS-CoA: a methodological solution to assess the spatio- temporal organization of species assemblages. Application to the demersal assemblages of the French Mediterranean Sea. Sci. Mar. 66, 221–232.
Garces, L. R., Stobutzki, I., Alias, M., Campos, W., Koongchai, N., Lachica-Alino, L., et al. (2006). Spatial structure of demersal fish assemblages in South and Southeast Asia and implications for fisheries management. Fish. Res. 78, 143–157. doi: 10.1016/j.fishres.2006.02.005
Garcia, S. M., and de Leiva Moreno, I. (2003). “Global overview of marine fisheries,” in Responsible Fisheries in the Marine Ecosystem, eds G. Sinclair and M. Valdimarsson (Wallingford: CABI Publishing), 1–24.
García-Rodríguez, M., Abelló, P., Fernández, A., and Esteban, A. (2011). Demersal assemblages on the soft bottoms off the catalan-levante coast of the Spanish Mediterranean. J. Mar. Biol. 2011, 1–16. doi: 10.1155/2011/976396
Garofalo, G., Fiorentino, F., Bono, G., Gancitano, S., and Norrito, G. (2004). “Localisation of spawning and nursery areas of Red Mullet (Mullus barba-tus, Linnaeus) in the Italian side of the strait of sicily (centralMediterranean),” in In GIS/Spatial Analyses Infishery and Aquaticsciences, Vol. 2, eds P. Nishida, J. Kaiola, and C. E. Hollingworth (Saitama: Fishery-Aquatic GIS Research Group), 101–110.
Garofalo, G., Fiorentino, F., Gristina, M., Cusumano, S., and Sinacori, G. (2007). Stability of spatial pattern of fish species diversity in the Strait of Sicily (central Mediterranean). Hydrobiologia 580, 117–124. doi: 10.1007/s10750-006-0460-1
Garofalo, G., Fortibuoni, T., Gristina, M., Sinopoli, M., and Fiorentino, F. (2011a). Persistence and co-occurrence of demersal nurseries in the Strait of Sicily (central Mediterranean): implications for fishery management. J. Sea Res. 66, 29–38. doi: 10.1016/j.seares.2011.04.008
Garofalo, G., Gristina, M., Fortibuoni, T., Giusto, G. B., Sinacori, G., and Fiorentino, F. (2011b). Recruitment areas of demersal species in the Straits of Sicily (Central Mediterranean). Fish. Sea Resour. 24, 1979–1988.
GCFM (2007). Resolution 31/2007/2: On the Establishment of Geographical Sub-Areas in the GFCM Area. Available online at: http://www.fao.org/tempref/FI/DOCUMENT/gfcm/web/GFCM_Recommendations2007.pdf (accessed February 4, 2020).
Goovaerts, P. (1997). Geostatistics for Natural Resources Evaluation. New York, NY: Oxford University Press.
Gristina, M., Bahri, T., Fiorentino, F., and Garofalo, G. (2006). Comparison of demersal fish assemblages in three areas of the Strait of Sicily under different trawling pressure. Fish. Res. 81, 60–71. doi: 10.1016/j.fishres.2006.05.010
Gristina, M., Fiorentino, F., Gancitano, V., Massi, D., Mirto, S., and Garofalo, G. (2013). The role of juveniles in structuring demersal assemblages in trawled fishing grounds. Estuar. Coast. Shelf Sci. 133, 78–87. doi: 10.1016/j.ecss.2013.08.014
Hilborn, R. (2011). Future directions in ecosystem based fisheries management: a personal perspective. Fish. Res. 108, 235–239. doi: 10.1016/j.fishres.2010.12.030
Hilborn, R. (2014). Introduction to marine managed areas. Adv. Mar. Biol. 69, 1–13. doi: 10.1016/b978-0-12-800214-8.00001-3
Hinz, H. (2017). “Impact of bottom fishing on animal forests: science, conservation, and fisheries management,” in Marine Animal Forests, eds S. Rossi, L. Bramanti, A. Gori, and C. Orejas (Cham: Springer), 1041–1059. doi: 10.1007/978-3-319-21012-4_37
Jennings, S., Greenstrem, S. P. R., and Reynolds, J. D. (1999). Consequence, Structural changes in an exploited fish community: contrasting of differential fishing effects on species with life histories. J. Anim. Ecol. 68, 617–627. doi: 10.1046/j.1365-2656.1999.00312.x
Jennings, S., and Kaiser, M. J. (1998). The effects of fishing on marine ecosystems. Adv. Mar. Biol. 34, 201–314. doi: 10.1016/S0065-2881(08)60212-6
Jost, L. (2007). Partitioning diversity into independent alpha e beta components. Ecology 88, 2427–2439. doi: 10.1890/06-1736.1
Khoukh, M., and Maynou, F. (2018). Spatial management of the European hake Merluccius merluccius fishery in the Catalan Mediterranean: simulation of management alternatives with the InVEST model. Sci. Mar. 82, 175–188. doi: 10.3989/scimar.04748.18A
Kraft, N. J. B., Adler, P. B., Godoy, O., James, E. C., Fuller, S., Levine, J., et al. (2015). Community assembly, coexistence and the environmental filtering metaphor. Funct. Ecol. 29, 592–599. doi: 10.1098/rsbl.2018.0460
Labropoulou, M., and Papaconstantinou, C. (2008). Community structure and diversity of demersal fish assemblages: the role of fishery. Sci. Mar. 68, 215–226. doi: 10.3989/scimar.2004.68s1215
Lauria, V., Garofalo, G., Fiorentino, F., Massi, D., Milisenda, G., Piraino, S., et al. (2017). Species distribution models of two critically endangered deep-sea octocorals reveal fishing impacts on vulnerable marine ecosystems in central Mediterranean Sea. Sci. Rep. 7, 1–14. doi: 10.1038/s41598-017-08386-z
Lauria, V., Gristina, M., Attrill, M. J., Fiorentino, F., and Garofalo, G. (2015). Predictive habitat suitability models to aid conservation of elasmobranch diversity in the central Mediterranean Sea. Sci. Rep. 5:13245. doi: 10.1038/srep13245
Lauria, V., Vaz, S., Martin, C., Mackinson, S., and Carpentier, A. (2011). What influences European plaice (Pleuronectes platessa) distribution in the eastern English Channel? Using habitat modelling and GIS to predict habitat utilization. ICES J. Mar. Sci. 68, 1500–1510. doi: 10.1093/icesjms/fsr081
Legendre, P., and Anderson, M. J. (1999). Distance-based redundancy analysis: testing multispecies responses in multifactorial ecological experiments. Ecol. Monogr. 69, 1–24. doi: 10.1890/0012-9615(1999)069[0001:dbratm]2.0.co;2
Levi, D., Andreoli, G., and Giusto, G. B. (1993). An analysis based on trawl-survey data of the state of the “Italian” stock of Mullus barbatus in the Sicilian Channel, including management advice. Fish. Res. 17, 333–341. doi: 10.1016/0165-7836(93)90133-r
Levi, D., and Andreoli, M. G. (1989). Valutazione approssimata delle risorse demersali nei mari italiani. Oebalia 15, 653–674.
Levi, D., Andreoli, M. G., and Giusto, R. M. (1995). First assessment of the rose shrimp, Parapenaeus longirostris (Lucas 1846), in the central Mediterranean. Fish. Res. 21, 375–393. doi: 10.1016/0165-7836(94)00298-b
Liquete, C., Piroddi, C., Drakou, E. G., Gurney, L., Katsanevakis, S., Charef, A., et al. (2013). Current status and future prospects for the assessment of marine and coastal ecosystem services: a systematic review. PLoS One 8:e0067737. doi: 10.1371/journal.pone.0067737
Maiorano, P., Sabatella, R. F., and Marzocchi, B. M. (2019). Annuario sullo stato delle risorse e sulle strutture produttive dei mari Italiani. Biol. Mar. Mediterranea 22(1 Suppl.):358.
Marbà, N., Jordà, G., Agustí, S., Girard, C., and Duarte, C. M. (2015). Footprints of climate change on Mediterranean Sea biota. Front. Mar. Sci. 2:56. doi: 10.3389/fmars.2015.00056
Martinelli, M., Morello, E. B., Isajlovic, I., Belardinelli, A., Santojanni, A., and Atkinson, R. (2013). Towed underwater television towards the quantification of Norway lobster, squat lobsters and sea pens in the Adriatic Sea. Acta Adriat. 54, 3–12.
Massutí, E., and Renones, O. (2005). Demersal resource assemblages in the trawl fishing grounds off the Balearic Islands (western Mediterranean). Sci. Mar. 69, 167–181. doi: 10.3989/scimar.2005.69n1167
McGilliard, C. R., Punt, A. E., Methot, R. D. Jr., and Hilborn, R. (2014). Accounting for marine reserves using spatial stock assessments. Can. J. Fish. Aquat. Sci. 72, 262–280. doi: 10.1139/cjfas-2013-0364
Milisenda, G., Vitale, S., Massi, D., Enea, M., Gancitano, V., Giusto, G. B., et al. (2017). Discard composition associated with the deep water rose shrimp fisheries (Parapenaeus longirostris, Lucas 1846) in the south-central Mediterranean Sea. Mediterr. Mar. Sci. 18:53. doi: 10.12681/mms.1787
Pinello, D., Gee, J., Accadia, P., Sabatella, E. C., Vitale, S., Polymeros, K., et al. (2018). Efficiency of shallow- and deep-water trawling in the Mediterranean and its implications for discard reduction. Sci. Mar. 69, 97–106.
Pipitone, C., Badalamenti, F., D’anna, G., and Patti, B. (2000). Fish biomass increase after a four-year trawl ban in the Gulf of Castellammare (NW Sicily, Mediterranean Sea). Fish. Res. 48, 23–30. doi: 10.1016/s0165-7836(00)00114-4
Pipitone, C., Badalamenti, F., Fernández Vega, T., and D’anna, G. (2014). Spatial management of fisheries in the mediterranean sea: problematic issues and a few success stories. Adv. Mar. Biol. 69, 371–402. doi: 10.1016/b978-0-12-800214-8.00010-4
Potoschi, A., Battaglia, P., Rinelli, P., Perdichizzi, F., Manganaro, A., and Greco, S. (2006). Variazione dei rendimenti con rete a strascico in un’area di parziale protezione nel Golfo di Patti (Sicilia Settentrionale) in 20 anni di monitoraggio. Biol. Mar. Medit. 13, 149–157.
Ragonese, S., and Bianchini, M. L. (1996). Growth, mortality and yield-per-recruit of the deep-water shrimp Aristeus antennatus (Crustacea-Aristeidae) of the Strait of Sicily (Mediterranean Sea). Fish. Res. 26, 125–137. doi: 10.1016/0165-7836(95)00394-0
Rijnsdorp, K. J., van Leeuwen, P. I., Heesen, H. J. L., and Daan, N. (1996). Changes in abundance of demersal fish species in the North Sea between 1906-1909 and 1990-1995. ICES J. Mar. Sci. 53, 1054–1062. doi: 10.1006/jmsc.1996.0132
Rogers, A. D., and Gianni, M. (2010). The Implementation of UNGA Resolutions. 61/105 and 64/72 in the Management of Deep-Sea Fisheries on the HIGH SEAS. London: DIANE Publishing.
Russo, T., D’Andrea, L., Franceschini, S., Accadia, P., Cucco, A., Garofalo, G., et al. (2019). Simulating the effects of alternative management measures of trawl fisheries in the central mediterranean sea: application of a multi-species bio-economic modeling approach. Front. Mar. Sci. 6:542. doi: 10.3389/fmars.2019.00542
Russo, T., Parisi, A., and Cataudella, S. (2013). Spatial indicators of fishing pressure: preliminary analyses and possible developments. Ecol. Indic. 26, 141–153. doi: 10.1016/j.ecolind.2012.11.002
Russo, T., Parisi, A., Garofalo, G., Gristina, M., Cataudella, S., and Fiorentino, F. (2014). SMART: a spatially explicit bio-economic model for assessing and managing demersal fisheries, with an application to italian trawlers in the strait of sicily. PLoS One 9:e86222. doi: 10.1371/journal.pone.0086222
Sala, A., Notti, E., Bonanomi, S., Pulcinella, J., and Colombelli, A. (2019). Trawling in the Mediterranean: an exploration of empirical relations connecting fishing gears, otterboards and propulsive characteristics of fishing vessels. Front. Mar. Sci. 6:534. doi: 10.3389/fmars.2019.00534
Sbrocco, E., and Barber, P. (2013). MARSPEC: ocean climate layers for marine spatial ecology. Ecology 94:979. doi: 10.1890/12-1358.1
Sousa, P., Azevedo, M., and Gomes, M. C. (2005). Demersal assemblages off Portugal: mapping, seasonal, and temporal patterns. Fish. Res. 75, 120–137. doi: 10.1016/j.fishres.2005.03.012
Stecken, M., and Failler, P. (2016). Ecosystem approach to fisheries and marine ecosystem modelling: review of current approaches. J. Fish. Livest Prod. 4:199. doi: 10.4172/2332-2608.1000199
Stefansdottir, L., Solmundsson, J., Marteinsdottir, G., Kristinsson, K., and Jonasson, J. (2010). Groundfish species diversity and assemblage structure in Icelandic waters during recent years of warming. Fish. Oceanogr. 19, 42–62. doi: 10.1111/j.1365-2419.2009.00527.x
Tserpes, G., Politou, C.-Y., Peristeraki, P., Kallianiotis, A., and Papaconstantinou, C. (2008). Identification of hake distribution pattern and nursery grounds in the Hellenic seas by means of generalized additive models. Hydrobiologia 612, 125–133. doi: 10.1007/s10750-008-9486-x
Vaz, S., Carpentier, A., and Coppin, F. (2007). Eastern English Channel fish assemblages: measuring the structuring effect of habitats on distinct sub-communities. ICES J. Mar. Sci 64, 271–287. doi: 10.1093/icesjms/fsl031
Vitale, S., Ragonese, S., Cannizzaro, L., Fiorentino, F., and Mazzola, S. (2014). Evidence of trawling impact on Hoplostethus mediterraneus in the central-eastern Mediterranean Sea. J. Mar. Biol. Assoc. 94, 631–640. doi: 10.1017/s0025315413001884
Warfe, D. M., Pettit, N. E., Magierowski, R. H., Pusey, B. J., Davies, P. M., and Douglas, M. M. (2013). Hydrological connectivity structures concordant plant and animal assemblages according to niche rather than dispersal processes. Freshw. Biol. 58, 292–305. doi: 10.1111/fwb.12058
Watson, R., and Morato, T. (2013). Fishing down the deep: accounting for within-species changes in depth of fishing. Fish. Res. 140, 63–65. doi: 10.1016/j.fishres.2012.12.004
Worm, B., Barbier, E. B., Beaumont, N., Duffy, J. E., Folke, C., Halpern, B. S., et al. (2006). Impacts of biodiversity loss on ocean ecosystem services. Science 314, 787–790. doi: 10.1126/science.1132294
Keywords: biodiversity conservation, demersal assemblages, mixed fisheries, trawling, ecosystem-based approach
Citation: Lauria V, Gristina M, Fiorentino F, Attrill MJ and Garofalo G (2020) Spatial Management Units as an Ecosystem-Based Approach for Managing Bottom-Towed Fisheries in the Central Mediterranean Sea. Front. Mar. Sci. 7:233. doi: 10.3389/fmars.2020.00233
Received: 20 January 2020; Accepted: 25 March 2020;
Published: 17 April 2020.
Edited by:
Tomaso Fortibuoni, Higher Institute for Environmental Protection and Research (ISPRA), ItalyReviewed by:
Matteo Zucchetta, Ca’ Foscari University of Venice, ItalyRuth H. Thurstan, University of Exeter, United Kingdom
Copyright © 2020 Lauria, Gristina, Fiorentino, Attrill and Garofalo. This is an open-access article distributed under the terms of the Creative Commons Attribution License (CC BY). The use, distribution or reproduction in other forums is permitted, provided the original author(s) and the copyright owner(s) are credited and that the original publication in this journal is cited, in accordance with accepted academic practice. No use, distribution or reproduction is permitted which does not comply with these terms.
*Correspondence: Valentina Lauria, dmFsZW50aW5hLmxhdXJpYUBjbnIuaXQ=