- 1Department of Biological Science, Florida State University, Tallahassee, FL, United States
- 2Channel Islands National Park, Ventura, CA, United States
- 3Marine Science Institute, University of California, Santa Barbara, Santa Barbara, CA, United States
- 4Department of Geography, Florida State University, Tallahassee, FL, United States
Fish abundance and diversity are core measurements taken by many nearshore marine monitoring projects. The most common approaches for counting fish include belt transects and timed counts by roving divers, each with its own limitations. Here we evaluate a fish counting method developed by the Channel Islands National Park’s Kelp Forest Monitoring Program (KFMP), in which roving observers make fish counts that are standardized both by the time taken (30 min) and the area sampled (2,000 m2). This method is potentially very useful because it combines an advantage of simple timed counts – the ability to rapidly sample a large area – with the potential to calculate area-specific density of fish, not just their relative abundance. However, the method has not been comprehensively evaluated and it is uncertain whether fish can effectively be counted in such a large target area within the allotted time. Fortunately, many sites surveyed with this method are also sampled with a more standard fish counting approach of belt transects, both by the KFMP and by the Partnership for Interdisciplinary Studies of Coastal Oceans. Here we compare estimates of fish density obtained through the area-standardized roving diver method and belt transect methods. In paired samples we find substantial and species-specific differences in densities estimated by each method. Considering all fish taxa together we find that roving divers are likely under-sampling the target area. Despite considerable species-level variation, the different methods produce similar estimates of average diversity and find similar regional and temporal patterns in fish abundance, demonstrating that they can successfully be used in parallel even if the datasets cannot be easily combined. These analyses can guide the interpretation of roving diver data for basic research and management decisions.
Introduction
Long-term monitoring is a critical tool for the management of marine systems. Monitoring data are often the only way to distinguish human impacts from natural variability, to detect changes in biodiversity over time, and to evaluate the performance of particular management strategies (Wolfe et al., 1987; Sukhotin and Berger, 2013; Mieszkowska et al., 2014; Reed et al., 2016). Despite their utility, such programs can also be expensive, and so it is necessary to carefully consider the relative strengths and weaknesses of alternative monitoring designs. Once established, consistency in data collection over time is necessary for interpretation of temporal trends, discouraging the redesign of existing monitoring programs and making the initial design of monitoring methods particularly critical.
The choice of methods for counting fish is particularly important for marine monitoring projects. There are three common methods for diver-based fish counts: stationary point counts, belt transects, and timed swims (Caldwell et al., 2016). In a stationary point count, the diver stays in the same spot and records the fish observed within a fixed radius for a given time (Bohnsack and Bannerot, 1986). In a belt transect, the diver swims a fixed distance or time in a straight line, counting organisms within a specified width on either side of the transect (Brock, 1954). In a timed swim, the diver swims for a fixed time while recording the fish encountered along the way (Kimmel, 1985).
Each method has its own strengths and weaknesses for estimating fish density and community structure. Transect-based methods have the advantage that they require little judgment on the part of the observer about the area being surveyed. The observer must estimate only whether fish counted fall within the area of the transect (typically 5 m or less on either side of the transect line and within a few meters of the bottom). Even so, transect-based methods have been shown to overestimate fish density either because fish swim into the transect and are incorrectly included in counts or because fish just outside the transects are counted (Davis and Anderson, 1989; Ward Paige et al., 2010). Transect-based methods are also known to be sensitive to swimming speed and transect width. In particular, shy species are better sampled by fast swimmers sampling a wide belt while cryptic and benthic species are better sampled by slow swimmers sampling a narrow belt (Pais and Cabral, 2018). Finally, they can also be challenging to implement, as a diver must lay out a measuring tape as fish are being counted (or a tape must be laid beforehand or by a second diver).
Point counts are simpler to implement than transects, but typically require the observer to judge whether fish fall within a larger radius (often 7.5 m or more) (Ayotte et al., 2011). They are also known to be sensitive to the time spent counting and can often overestimate fish densities particularly for bold fish attracted to divers (McCauley et al., 2012; Pais and Cabral, 2018). Timed swim counts are the easiest to implement and can cover large areas, as divers do not need to estimate distances or take measurements as the survey progresses. But they come with a critical drawback: because there is no accurate way of measuring the area being sampled, timed counts cannot be used to estimate fish density on an area-specific basis, thereby limiting their utility for informing fisheries management and conservation. In addition, it can be difficult to compare data from timed swims with data on fish densities collected by other methods.
In an attempt to find a compromise between the limitations of these different approaches, the Channel Islands National Park’s Kelp Forest Monitoring Program (KFMP) has been implementing a “roving diver fish count” that is standardized by both time and area. This method is essentially identical to a traditional timed count except that it takes place within a fixed area centered on a permanent 100 m long transect. Divers are assigned to count all fish encountered anywhere in the water column within 10 m of both sides of that transect over a period of 30 min, swimming a zig-zag pattern on one side of the transect for the first 15 min and then spending 15 min swimming a similar pattern on the other side (Kushner et al., 2013). This process yields a nominal sampling area of 2,000 m2. The strategy of searching such a large fixed area is unusual and made possible by the presence of long, permanently installed transects at each sampling site that can be used to orient divers. We are not aware of comparable methods being used elsewhere, although timed counts are sometimes interpreted as densities based on an assumed swim speed or the use of global positioning systems to estimate area searched (Beck et al., 2014).
Although this method is potentially very powerful because it rapidly samples a large fixed area, it has potential limitations as well. For example, the navigation involved in sampling the target area is substantially more complex than that required for swimming a straight transect of fixed length. The area (and volume) sampled within 30 min is also ambitious, with divers searching the entire water column, from the surface to the substrate, at depths ranging from 5 to 15 m. While previous studies using these data have calculated densities based on the assumption that the entire 2,000 m2 area is being sampled (Lamy et al., 2018), the effective area sampled by this method could be considerably smaller. This effect may be exacerbated when there is dense kelp or murky water limiting visibility. Thus, a key step to assess the usefulness of the data from this method is a validation of the size of the area effectively sampled. More generally, the novelty of this sampling approach means that its effectiveness and potential biases have not been thoroughly evaluated.
The Santa Barbara Channel is an exceptionally well-surveyed marine ecosystem and presents an ideal location for evaluating a novel method of ecological monitoring. The longest running program is the Park’s KFMP, which has been conducting annual surveys of subtidal rocky reef ecosystems throughout the five Park islands since 1982 (Kushner et al., 2013). The Partnership for Interdisciplinary Studies of Coastal Oceans (PISCO) has also undertaken extensive monitoring of fish, invertebrates, and algal communities since 1999 at a large number of sites that encompass the region monitored by KFMP. In some cases, the two programs have sampled at the same locations in a collaborative effort to better understand the differences between the monitoring techniques. This creates a valuable opportunity to compare the alternative sampling strategies employed by the two groups. Additionally, the KFMP also collects transect-based data in the same locations as the roving diver method, which can also be used for comparison.
Here we compare the fish survey data from the KFMP’s time-and-area-standardized roving diver fish counts with data from PISCO’s transect-based fish counts, as well as with the KFMP’s transect-based counts. We address three key questions: (1) How do estimates of fish density and community structure generated by roving diver counts compare to estimates derived from transect-based counts? and (2) Does KFMP’s roving diver data perform similarly to PISCO’s fish counts for detecting biogeographic and temporal abundance patterns in the region? The answers to these questions reveal the potential utility of time-and-area-standardized roving diver fish counts, and can help guide the analysis of monitoring data in the region.
Materials and Methods
Study Site
The fish surveys included in this study were performed at nearshore rocky reef and kelp forest sites located around five islands in the Santa Barbara Channel (San Miguel, Santa Rosa, Santa Cruz, Anacapa, and Santa Barbara; Figure 1). A strong gradient in sea surface temperature (SST) exists across the region, with lower temperatures toward the westernmost part of the channel where the California Current transports cold water from the north, and warmer temperatures toward the eastern and southern parts of the Channel where the Southern California Countercurrent runs along the mainland transporting warm water from Baja California, Mexico (Hendershott and Winant, 1996). Our coldest sampling location had an average annual temperature of 14.6°C, while our warmest averaged 16.6°C. Upwelling-favorable winds can bring cold and nutrient-rich waters to the surface all year, but are particularly prevalent during spring (Brzezinski and Washburn, 2011). Being located among this confluence of currents makes the Channel Islands’ ecosystems highly productive and diverse (Nishimoto and Washburn, 2002; Brzezinski and Washburn, 2011). In part due to this ecological richness, the Channel Islands were designated a National Park in 1980. The protection that terrestrial habitats were afforded was extended to the marine environment with the establishment of 13 marine protected areas (MPAs) in 2003 (Figure 1).
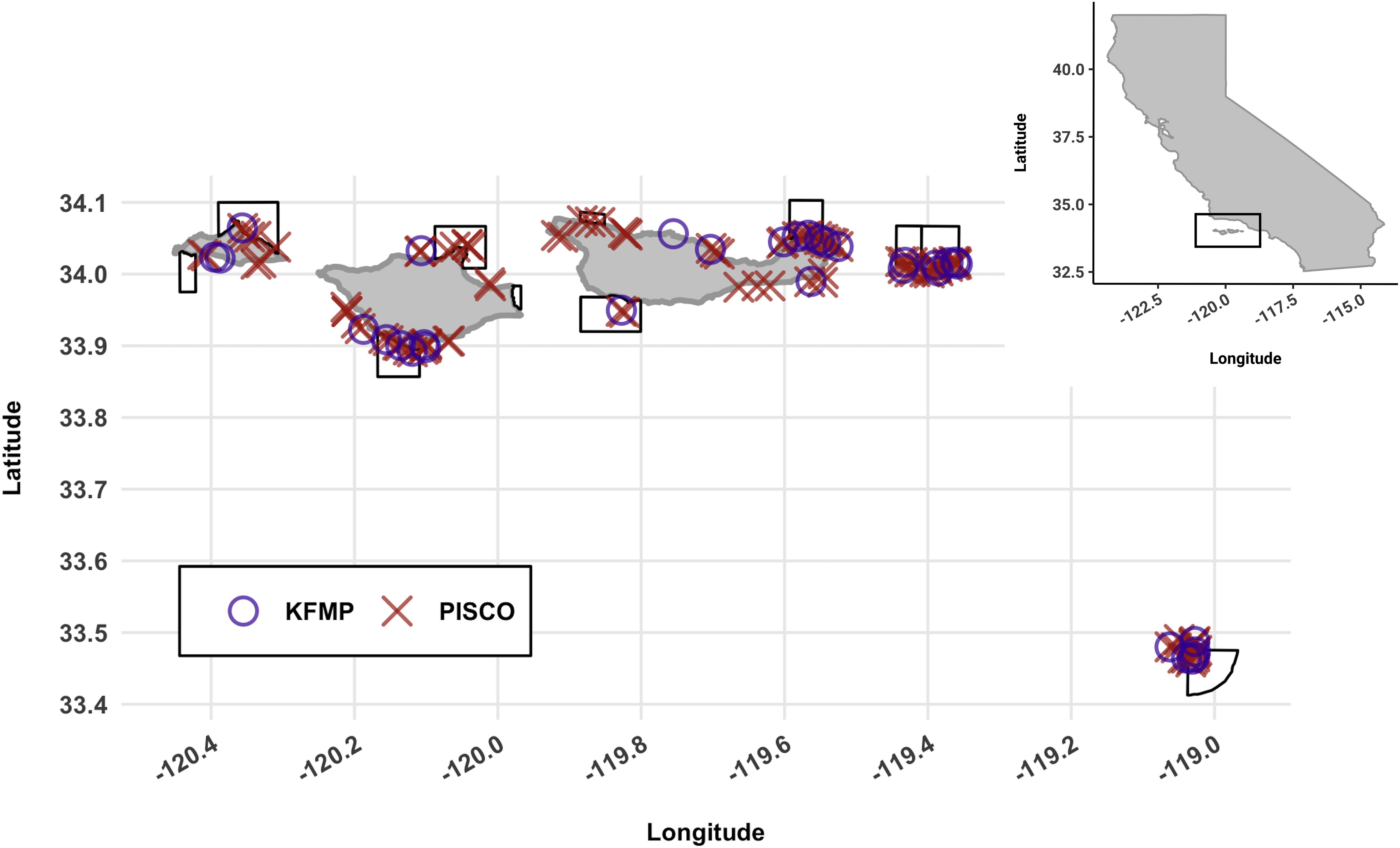
Figure 1. Map of the study area, the Channel Islands National Park, CA, United States. All sites sampled between 2000 and 2016 are shown. Circles represent KFMP sites (n = 33) and crosses represent PISCO sites (n = 100). Black bordered polygons outline marine protected areas. Location within the state of California is show in the inset plot.
Monitoring Programs
In order to collect baseline information about the kelp forest ecosystem, the Channel Islands National Park established the KFMP in 1982. Initially covering 16 sites, the monitoring network was expanded to 17 sites in 2001 and then to 33 sites in 2005. Each site is organized around a single 100 m long transect, which consists of a weighted line permanently fixed to the bottom by steel bolts. The area around each transect is sampled each summer (sometime between May and October) using various methods to collect size and abundance data of algae, invertebrates and fish (Kushner et al., 2013).
Since the inception of the program, fish have been sampled in a 2-m wide belt transect, in which a diver swims at a uniform speed of 20 m min–1 on one side of the 100 m long fixed transect and then reverses direction and swims a 2 m wide belt on the other, previously unsampled, side of the main transect, covering a total area of 400 m2 (treated here as a single sample). In this count, only a limited number of species are recorded (Table 1), and fish are only counted if observed within 3 m of the substrate.
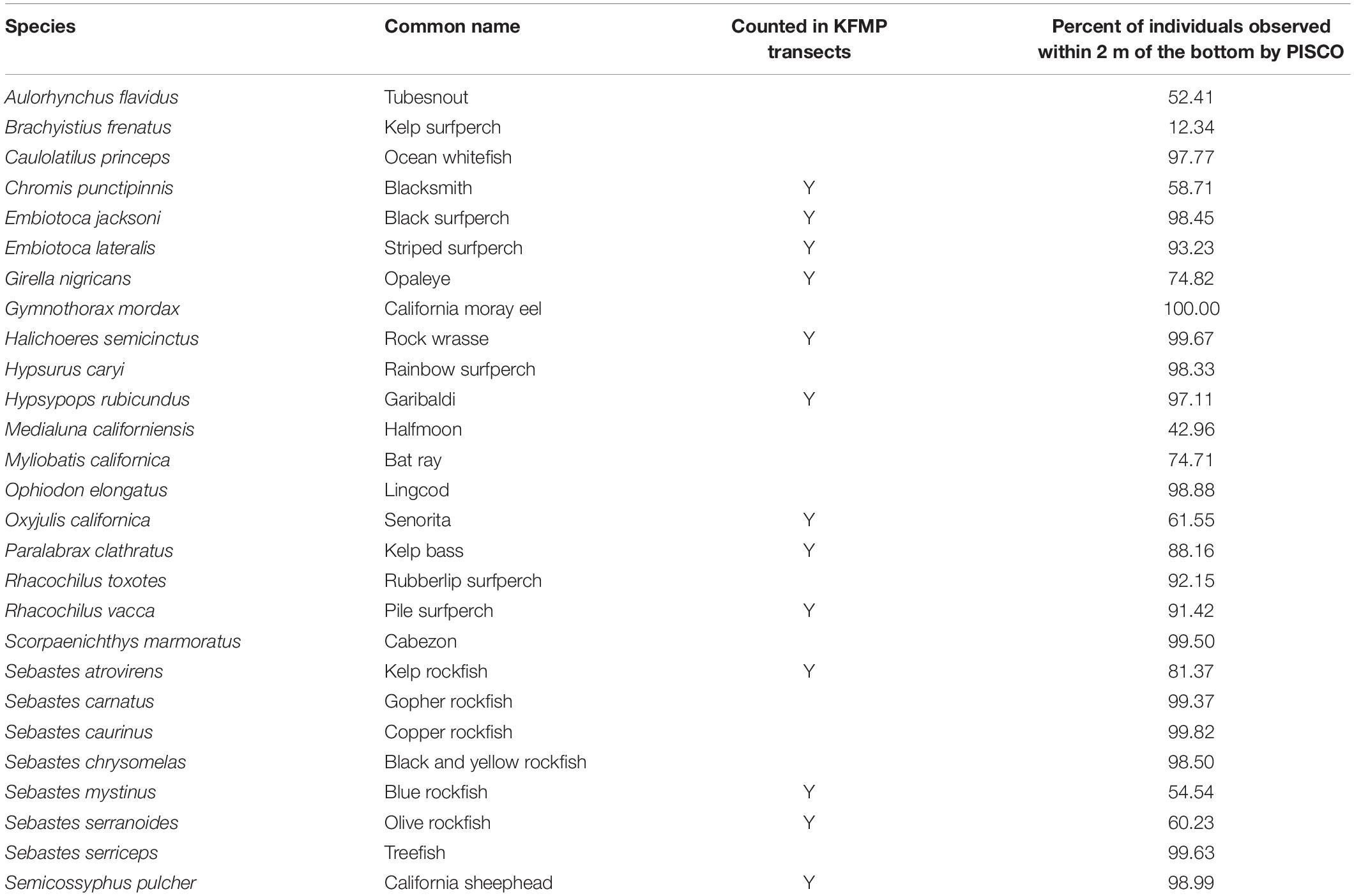
Table 1. List of 35 species included in at least 10 KFMP roving diver-PISCO paired samples. The species also counted on KFMP transects are noted.
In 1996, as a result of a comprehensive program review, the KFMP introduced a “Roving Diver Fish Count” (Davis et al., 1996). The method was conceived of as a way to rapidly sample the entire fish community at a site, recording all fish encountered (instead of the restricted list of indicator species used for the transect surveys) and covering a larger area than the transects. In the roving diver method, divers swim on one side of the 100 m fixed transect, slowly moving from one end of the transect to the other and roving back and forth within 10 m of the transect counting all fish on the substrate, midwater and canopy within this area. Each diver employs a flexible search strategy, moving up and down through the water column and adjusting their speed based on the habitats being surveyed. After 15 min the diver should have reached the end of the transect and then switches sides, spending the next 15 min covering the area within 10 m of the other side of the transect for a total area covered of 2,000 m2. Initially, the roving diver counts recorded fish abundance in broad categories (“single 1,” “few 2–10,” “common 11–100,” and “many >100”), but in 2003, roving divers began recording counts of all fish encountered. The resulting data have often been interpreted as densities by dividing counts by the target area being sampled (2,000 m2) although the area effectively sampled could be less than this target area.
In 1999, the PISCO established a monitoring program with the aim of conducting long-term and large-scale studies of United States. West coast rocky shores, kelp forests and the coastal ocean. In the Channel Islands, the PISCO program has sampled nearshore habitats in order to better understand population dynamics and connectivity of marine species and also to evaluate the effectiveness of MPAs. PISCO performs annual subtidal surveys each summer to quantify diversity, density and biomass of macroalgae, invertebrates, and fishes, using an array of standardized methods (Carr and Caselle, 2009). The number of PISCO sampling sites has changed over time but the program has excellent representation across the northern Channel Islands including extensive sampling inside and outside of the MPAs (Figure 1).
Unlike the KFMP, PISCO sites are sampled with randomly placed (rather than fixed) transects in fixed depth strata. PISCO samples fish using 2 m wide × 30 m long transects. Between 8 and 12 randomly placed transects are sampled per site, stratified between 5 and 20 m in depth and each running parallel to the shoreline. Each transect is composed of three water column depths (bottom, midwater, and kelp canopy). A diver at the bottom lays the transect line while counting fish within 2 m of the substrate, while another diver swims above, at roughly half the depth of the bottom diver, counting fish in the midwater. When kelp canopy is present, a third level is surveyed in the kelp canopy. On the shallowest transects, only bottom and canopy counts are performed to avoid overlapping counts. All species are recorded and sizes estimated to the nearest cm.
Data Processing
We focused our analysis on data collected between 2003 and 2016, identifying all cases where KFMP and PISCO sampled sites within 500 m of each other within a 60 day interval (Miller et al., 2018). We treated each such case as a pair of sampling events for the analysis, yielding 241 pairs. In cases where a sampling event had multiple potential pairs, we selected the physically closest potential pair so that each observation was used in only one pair. The distribution of distances between the resulting paired sites is plotted in Supplementary Figure S1. In both datasets, we limited our analyses to adult fish, operationally defined for most species as fish larger than 10 cm in length (Kushner et al., 2013). Reef fish recruits and young-of-year can be extremely patchy in space and time; focusing on patterns of adult abundance avoids confounding our analyses with transient recruitment dynamics.
In the roving diver counts, we calculated the density of each species by dividing the number of fish observed by the target area sampled (by default 2,000 m2). For each sampling event, we averaged these densities across three divers, selected at random from available divers if more than three observers sampled in an event. The KFMP employs roving divers with a wide range of experience, and we only used data collected by the most experienced class of diver. We do not attempt to estimate and correct for biases associated with individual observers; though such an analysis would likely reduce inter-observer variation, it is less relevant for the cross program comparisons undertaken here.
PISCO typically samples 12 transects per site, but those transects are more variable in depth and substrate covered than the KFMP sites. To account for these factors, we used only PISCO sites in habitats that were less than 50% sand (only nine sampling events did not meet this criteria), and for each pair we only used PISCO transects with bottom depths within 5 m of depth of the paired KFMP site sampled by roving divers (with KFMP depths based on the average depth of the main transect). Within these parameters, we calculated fish density for each transect, adding up all fish observed on the transect (including bottom, midwater, and canopy counts except where otherwise noted) and then dividing by the area of bottom covered (60 m2). To standardize the amount of effort for each sampling event, we chose six transects at random from those meeting the depth and substrate characteristics. We then averaged these densities across those transects conducted during a sampling event.
Some PISCO transects were deliberately placed precisely on permanent KFMP transects, yielding the closest possible match in the areas sampled. We repeated some analyses using only PISCO transects within 2 m of depth, using only PISCO sites with less than 50% sand, within 100 m distance, and sampled within 60 days of a KFMP roving diver count, yielding 126 paired events.
Comparing Sampling Methods
To compare the density of each species observed by roving divers with the density observed in paired transect-based samples, we calculated the ratio of the estimated PISCO density to the KFMP density for every pair of sampling events, with ratios log10 transformed so that a value of 1 indicates that PISCO counted 10 times as many individuals of that species, while a value of −1 means KFMP counted 10 times as many individuals of that species. Zero density values were treated as 0.00005 to avoid undefined ratios, and medians and quantiles (which were not sensitive to this offset) were calculated across all sample pairs. For these comparisons we focused on 27 taxa that appeared in more than 10 site pairings (Table 1).
In order to evaluate how effectively the roving diver methods sampled fish community structure, we compared diversity metrics derived from roving diver counts to those in PISCO’s transect-based methods. We compared species richness (i.e., the total number of species observed in each sampling event) and because richness is highly dependent on sampling effort, we also performed individual-based rarefaction to estimate the diversity that would be expected if 50 individuals had been sampled. If more than 50 fish were observed across a sampling event, this procedure subsamples those to estimate the diversity that would have been measured if only 50 fish had been seen. If fewer than 50 fish were observed, the procedure extrapolates the diversity-accumulation curve to estimate diversity if 50 fish had been seen. Due to the limitations of rarefaction, only 85 site pairs were usable. For each sampling event we calculated rarified diversity for different Hill numbers using the iNEXT package in R (Hsieh et al., 2016): 0D (richness), 1D (the effective number of species at Hill number 1, a metric closely related to the Shannon–Wiener index), and 2D (the effective number of species at Hill number 2, a metric closely related to the Gini–Simpson index). All three indices are “true” diversity metrics, expressing diversity in comparable units, with the higher order metrics taking greater account of the relative abundance of each species observed (Jost, 2006; Chao et al., 2014). All taxa observed were included in this analysis, including a few only identified to genus or family level (full taxa list in Supplementary Table S1), recognizing that these data underestimate true fish diversity because neither method effectively samples very small and cryptic species (e.g., gobies, blennies, and most sculpins).
To estimate the slope of the relationship between diversity metrics obtained from the roving diver counts to those obtained from transect-based methods, we used a Deming regression rather than a traditional GLM-based approach, using the mcr package in R (Manuilova et al., 2014). The Deming regression technique is a reliable method of fitting a Model II regression (Cornbleet and Gochman, 1979), and is commonly used for paired method comparison (Pollock et al., 1992; Linnet, 1998). This method fits a line that assumes both the X and Y variables (in this case the two fish survey methods) are equally likely to have errors associated with them. Rather than minimizing errors in the Y-dimension, it minimizes the residuals from a given best fit line as measured orthogonally from that line. Estimates of the fit of the relationship are obtained from Pearson’s correlation coefficient, which similarly is not sensitive to which variable is assigned to the X and Y axis.
One goal of this study was to evaluate the default assumption that the full 2,000 m2 area is effectively sampled by KFMP’s roving divers. To this end, we performed Deming regressions for a series of comparisons, in each case comparing the density of each species observed in a roving diver count to the density observed at a paired site sampled with a transect-based method. We calculated the slopes of these relationships to assess the discrepancies between the two methods across all species and sampling events. A slope lower than one indicates that KFMP roving diver surveys estimate higher densities than PISCO or KFMP transect-based counts. A slope higher than one indicates that PISCO or KFMP transect-based counts estimate higher densities than KFMP roving diver surveys. We also calculated a Pearson’s correlation coefficient to describe the fit of the relationship. We compared the roving diver estimates to: (1) PISCO transects, (2) PISCO transects excluding canopy counts, (3) PISCO transects excluding canopy and midwater counts, and (4) PISCO transects only including species where >90% of individuals are found within 2 m of the bottom (Supplementary Table S1). The latter three comparisons were intended to explore the possibility that roving diver counts might only effectively sample fish near the substrate. Roving divers are trained to look up and in all directions, but they often swim closer to the bottom and may find it easier to observe fish below than above them. Furthermore, we compared roving diver estimates to the KFMP’s transect-based counts (for the 13 species sampled with that method), to PISCO’s bottom transects limited to the same 13 species, and to the subset of PISCO transects matched more closely with the KFMP sites in space.
Comparing the Ability to Detect Major Patterns
We compared regional trends in fish community structure obtained with roving diver data and PISCO’s transect-based data. We evaluated spatial trends, testing the ability of each data set to estimate the relationship between SST and abundance for each species, as temperature is known to influence biogeography in the region. We also compared temporal trends of increasing and decreasing abundance in the region as identified by the two methods.
For the spatial analysis, we selected all sites from each program with mean annual SST (in the period 2003–2016) between 14.5 and 17°C. SST was derived from Pathfinder AVHRR (advanced very high 188 resolution radiometer) that was optimally interpolated at daily and 0.25° latitude/longitude resolution (Reynolds et al., 2007). Density of each species was averaged for each site over all years for both the PISCO transect and KFMP roving diver datasets. A general linear model was used to predict the mean density of each species that had been observed by both programs as a function of temperature (n = 44). A Deming regression was used to estimate the relationship between these taxa-specific slope estimates and a Pearson’s correlation coefficients was used to describe the correspondence between estimates.
For the temporal analysis, we calculated the average density per taxon for each sampling event in the PISCO transect and KFMP roving diver datasets. We then fit a linear mixed effect model for each species, modeling the density of the species as a function of the date sampled (a continuous fixed effect) and the site (a categorical random effect). This yielded estimates of abundance trends over time, estimated separately from the PISCO and KFMP roving diver datasets, for each of the 44 species observed by both programs. We used a Deming regression to estimate the relationship between these taxa-specific trend estimates and Pearson’s correlation coefficients used to describe the correspondence between estimates.
Results
Comparing Sampling Methods
We found considerable variation among species when comparing densities of fish in the roving diver counts to densities recorded by PISCO’s transect-based methods at nearby sites sampled in the same season (i.e., sampling within 500 m, 5 m of depth and 60 days; Figure 2). The observed differences were generally large but rarely exceeded an order of magnitude (only one species had a log ratio outside the range of −1 to 1). Even quite common and conspicuous species such as California sheephead (Semicossyphus pulcher) and garibaldi (Hypsypops rubicundus) showed substantial inter-program variation (Figure 2). The densities of 17 species were higher in PISCO’s transect counts, whereas 10 species occurred at higher densities in the KFMP’s roving diver counts. Discrepancies between survey methods did not seem related to a species’ body type, size, or whether the species is targeted by fishing, although qualitatively, the roving divers seem to sample more cryptic fish (e.g., cabezon, lingcod, morays, and treefish) and large rare species (e.g., seabass and bat rays). More abundant species, by contrast, may yield higher density estimates in transects than in roving counts. Taxa with a higher median density (calculated across all data from both programs) had higher estimated densities in the PISCO transects, while taxa with lower densities were split as to which program estimate higher abundances (Supplementary Figure S2).
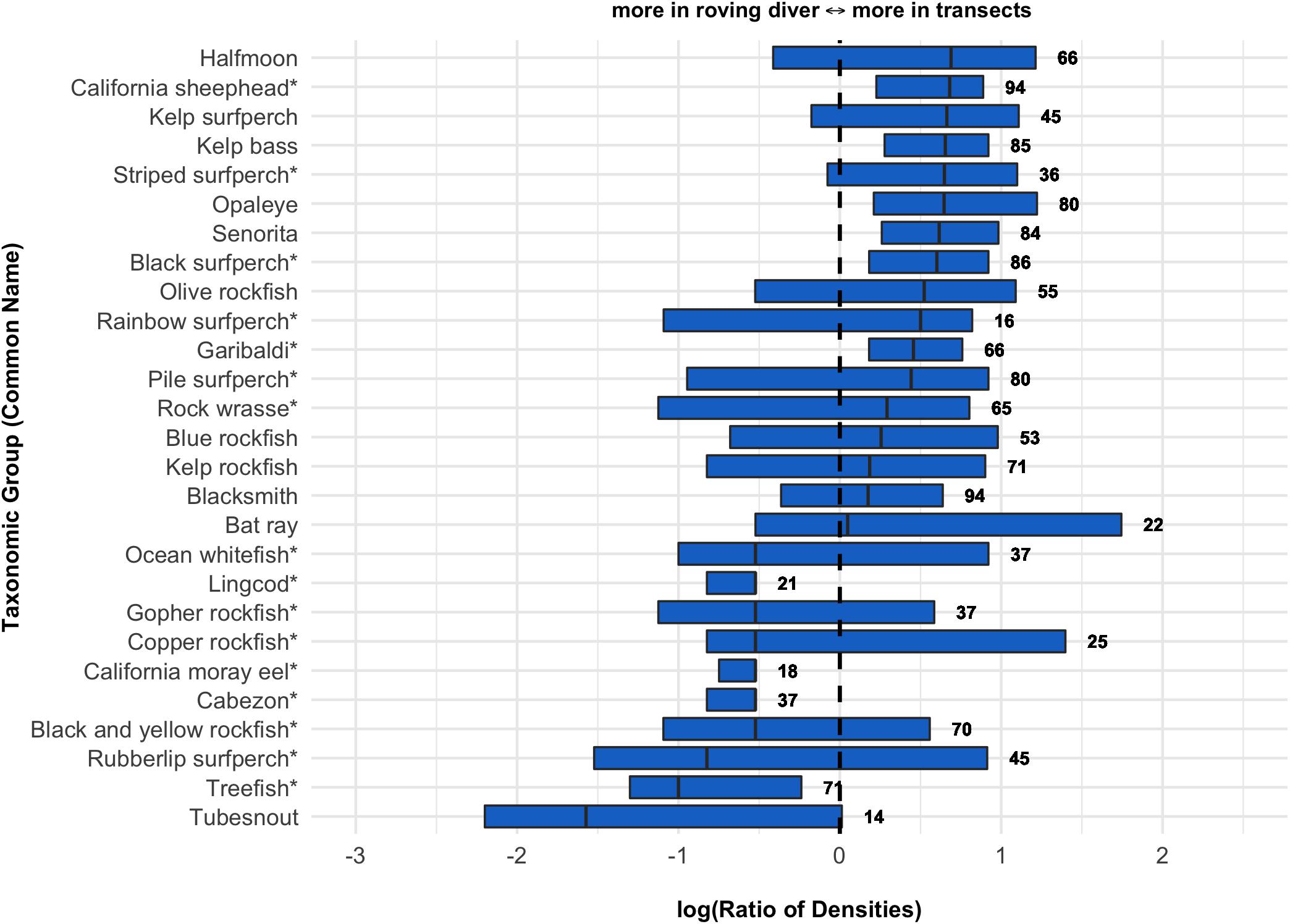
Figure 2. Boxplots showing the log(10) ratios of densities estimated from the KFMP roving diver counts compared to paired sites sampled by PISCO transects. Boxes indicate 25th, 50th, and 75th percentiles. The number of paired samples included in calculations for each taxa is indicated to the right of each bar (only taxa with n ≥ 10 are shown). A star next to the common name indicates more than 90% of individuals are found within 2 m of the bottom in PISCO sampling.
Despite substantial inter-program variation for species-level density estimates, levels of diversity estimated by the two methods were similar on average although they showed considerable scatter at the site scale. When comparing nearby sites sampled with both methods, the roving diver fish counts recorded more species on average, having higher observed richness in 87% of pairs (Figure 3A). This suggests that roving diver methods may have an advantage for documenting the presence of rare species. However, this advantage is derived from a much higher number of individuals recorded in roving diver counts on average. In the paired samples, the roving diver counts recorded an average of 1,133 individuals in a sampling event, while PISCO’s transect-based methods recorded 195 individuals. When individual-based rarefaction was employed to obtain estimates of diversity independent of the numbers of individuals observed, we found there was no average deviation between the two methods (i.e., the relationship is similar to the 1:1 line; Figure 3B), including for the higher order diversity metrics 1D (a metric closely related to Shannon diversity; Figure 3C) and 2D (a metric closely related to Simpson’s diversity; Figure 3D). Although diversity estimates were unbiased, there was low but significant agreement between the methods at the site level, with diversity metric correlations ranging from 0.45 for 0D to 0.32 for 2D and all Pearson’s correlation coefficients significant at p < 0.01.
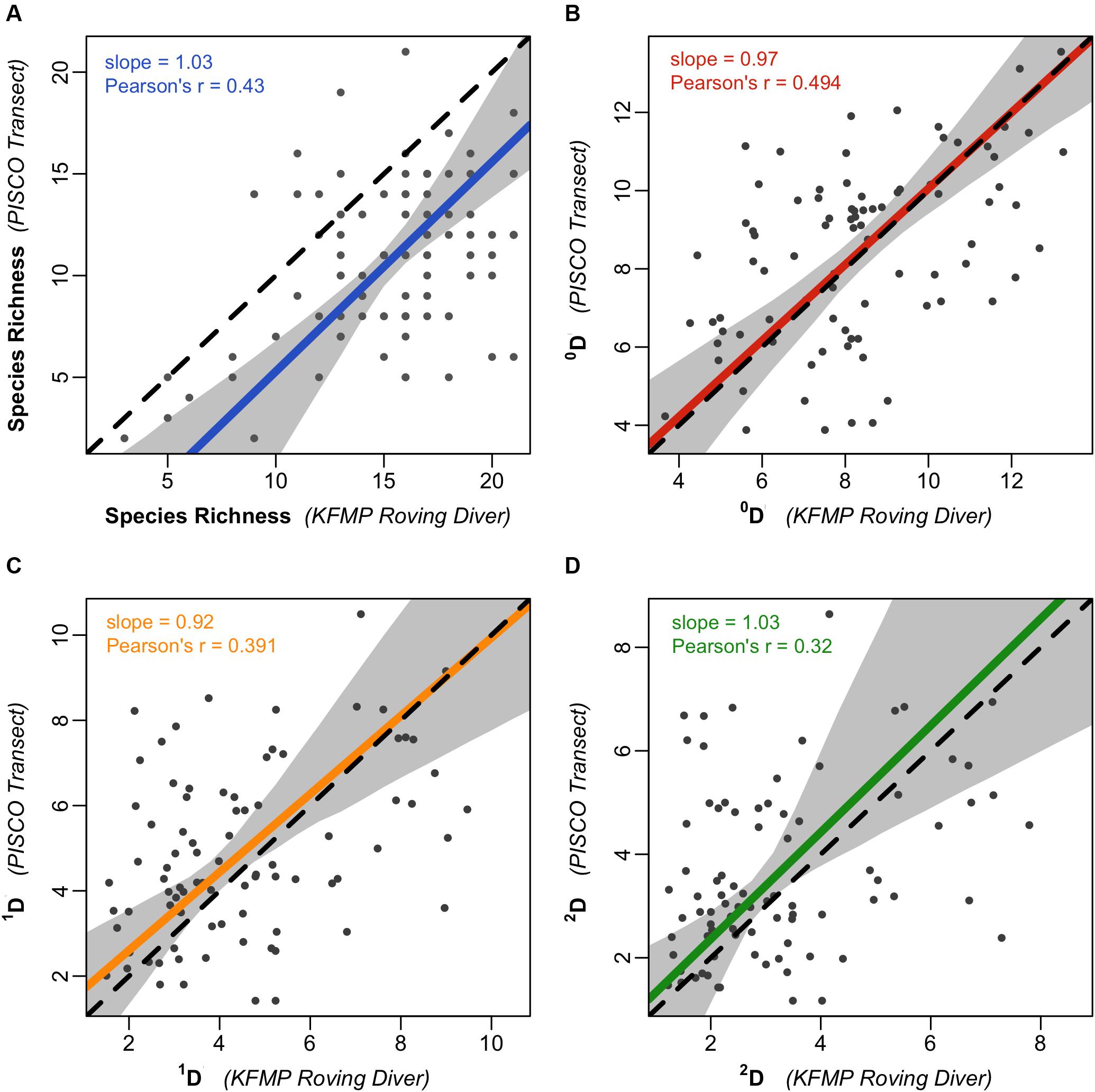
Figure 3. Diversity metrics for the two fish survey methodologies. Scatterplots representing (A) the relationship of raw species richness between paired KFMP roving diver counts and PISCO transects (n = 241 paired sampling events). Remaining plots represent similar analyses for (B) 0D, (C) 1D, and (D) 2D, each rarefied and extrapolated/interpolated to 50 individuals (n = 85 for plots B–D). In each plot the solid line represents a Deming linear regression and the dashed line represents the 1:1 line. The 0.95 confidence bounds are calculated with the bootstrap (quantile) method. All 72 taxa are included.
We found a clear tendency toward higher overall fish density estimates in PISCO’s transect-based method than in the roving diver counts (Figure 4A). The Deming regression indicates that PISCO counts 5.8 fish for every fish observed by the roving divers in the same amount of area. Although densities are higher for the transects, there is substantial scatter in the relationship, with a correlation of 0.53 for paired sampling observations (Figure 4A).
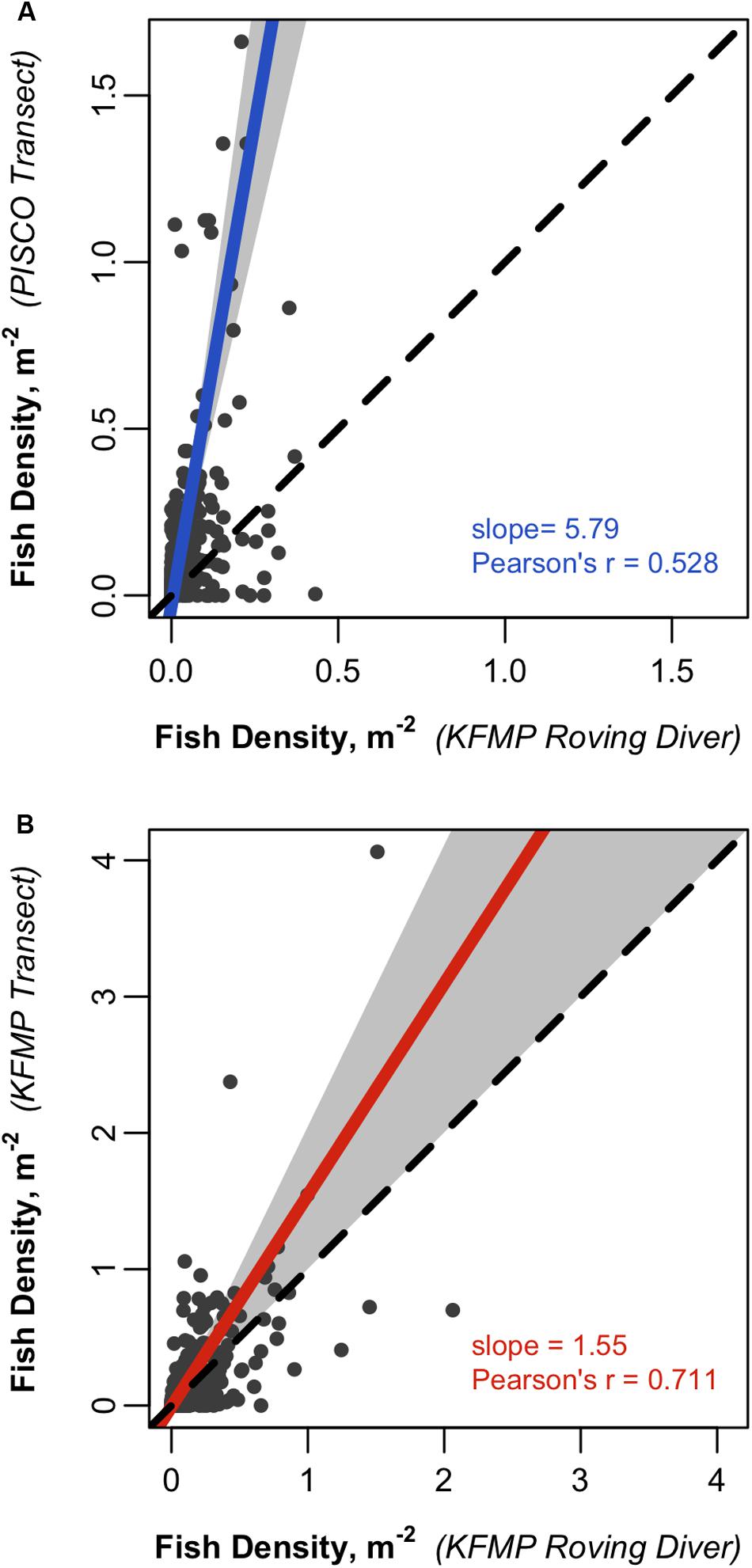
Figure 4. Scatterplots comparing fish densities estimated from paired KFMP roving diver counts and (A) PISCO or (B) KFMP transects. The solid line represents a Deming linear regression and the dashed line represents the 1:1 line. The 0.95 confidence bounds are calculated with the bootstrap (quantile) method. Only taxa seen in 10 or more site pairs included: 27 taxa for (A) and 13 taxa for (B).
The roving diver method aims to count all fish within the sampling area regardless of their position in the water column, including fish in the midwater and near the surface. However, it is likely that an observer, who is often swimming within a few meters of the bottom, may systematically underestimate fish found near the surface, particularly at times when abundant giant kelp forms vertical structure and a canopy at the surface and thus blocks sight-lines, or when water clarity is low. PISCO’s transect-based method deals with this challenge by counting fish near the bottom in one pass and midwater and canopy fish in one or two additional passes (depending on water depth and the presence of kelp canopy). We explored whether the agreement between fish densities estimated by the two programs might be higher if these canopy and midwater transects are excluded from the analysis and only fish sampled in PISCO’s benthic transects are considered. Excluding PISCO’s canopy transects had little effect on the correlation (r = 0.54; Supplementary Figure S3A), with a slope that implies PISCO transects recorded ∼5 times as many fish as the roving divers observed in the same area. If canopy and midwater transects were both excluded from the transect data, density estimates were somewhat more similar; transects counted 3.3 times as many fish as roving divers in the same area, but the correlation was somewhat lower at 0.44 (Supplementary Figure S3B). If we limit our comparison to only benthic species (species for which >90% of individuals were found in the bottom transect of PISCO surveys; Table 1), the correlation was higher at 0.51, but the slope of the relationship was very steep, suggesting transects counted 10 times as many fish as roving divers in the same area (Supplementary Figure S3C).
We also compared the roving surveys to transect-based counts collected by the KFMP on the same days at the same sites as the roving surveys, but only sampling a fixed list of 13 species. Again, we find that roving diver counts obtain consistently lower estimates of fish density than the transect-based method (Figure 4B), although the magnitude of the difference was smaller and the confidence intervals for the slope overlapped the 1:1 line. The correlation between the KFMP roving diver counts and KFMP’s transect-based method (r = 0.71) is higher than that observed between the KFMP roving diver method and the PISCO transect surveys (r = 0.53). This likely reflects the tighter pairing of these samples in space and time and not the choice of species included; comparing KFMP transects to PISCO transects for the 13 species sampled by KFMP yields lower correspondence (r = 0.31, with very wide confidence intervals on the slope; Supplementary Figure S3D).
Repeating our density comparisons using only the subset of PISCO transects paired most closely with KFMP transects in space (within 100 m in distance and 2 m in depth) had little effect on results (Supplementary Figure S4). Of particular note, the variation in density on these closely matched transects was closer to that observed in the broader KFMP to PISCO comparison (Supplementary Figure S3) than to the KFMP roving diver to KFMP transect comparison (Figure 4B), suggesting that the discrepancy represents inter-program variation or temporal effects rather than a spatial effect.
The roving diver method does not have an explicit swim speed or search width, but we can calculate combinations of swimming speed and search widths that are consistent with a given area sampled; this analysis suggests the necessary search behavior to cover each proposed area, and sheds light on the plausibility of such a search. For example, we can consider speed and search width combinations consistent with the nominal area sampled (2,000 m2 in 30 min) and compare these to the swim speeds and search widths used in transect-based fish counts (Figure 5). Observers sampling the PISCO transects swim a 2-m swath at rates ranging from 3 to 6 m min–1. A roving diver swimming twice as fast as the PISCO observers, and covering a swath twice as wide, would still sample only 1,440 m2 in 30 min, less than 3/4 of the target area. The KFMP transects are searched at a rate covering 1,200 m2 in 30 min (a 2-m swath at 20 m min–1) but this survey samples only 13 species that were chosen because they are conspicuous and easy to count. Although this analysis is useful for understanding the area that is effectively searched, it is a simplification that does not reflect the fundamental difference in the roving divers’ search strategy. By design, roving divers can change speeds and widths, lingering in habitats with many hiding spots and speeding through open areas with high visibility. However, taken together with the much higher densities of most species of fish observed by PISCO transects (Figures 2, 4), these comparisons suggest that the roving divers are not effectively sampling the full 2,000 m2 target area.
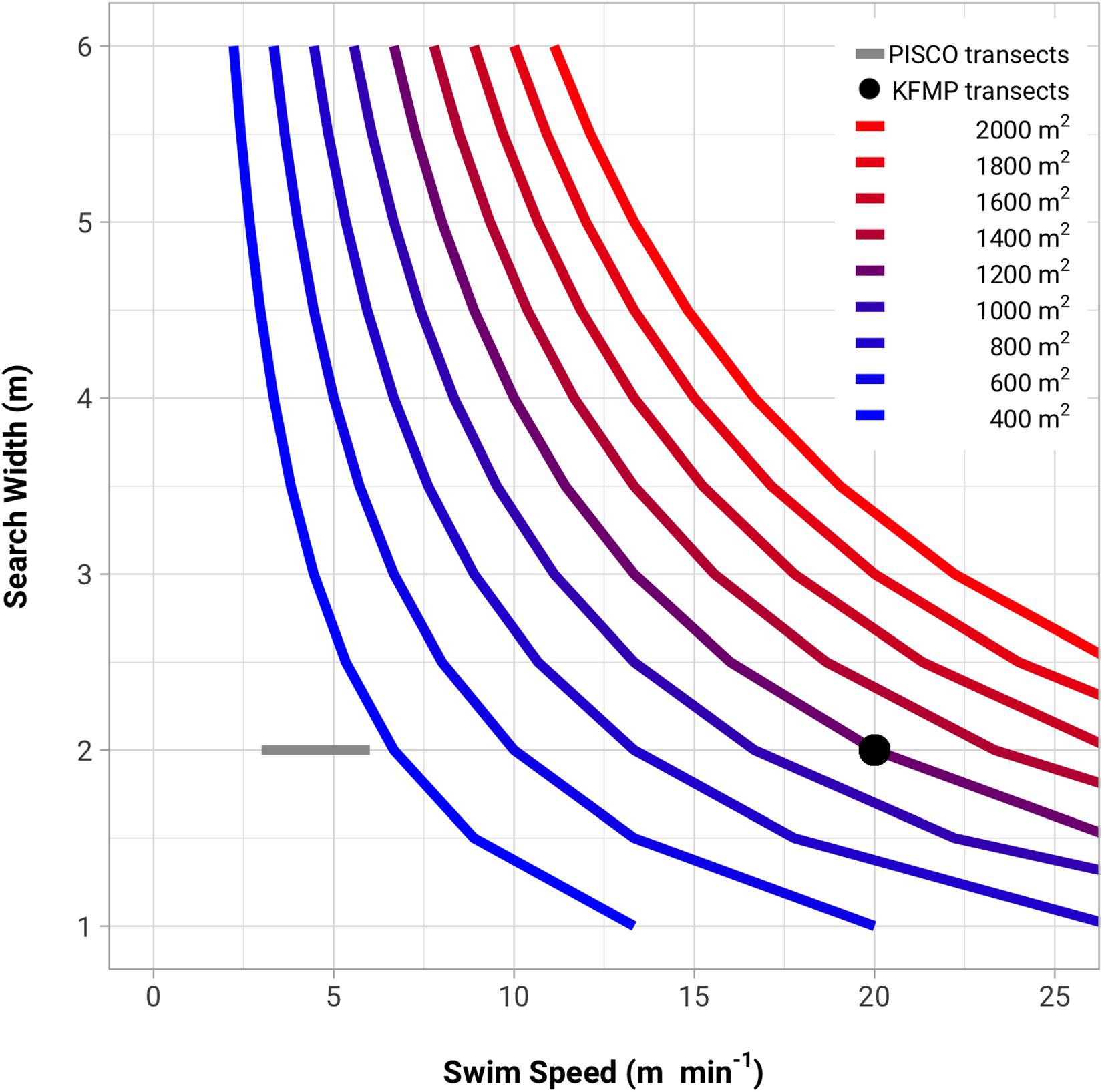
Figure 5. Swimming speeds and search widths required to meet different assumptions about the total area being sampled in KFMP’s roving diver counts. Each curve represents a different assumed sampled area. The nominal transect width and swimming speed of PISCO and KFMP transect-based sampling are also plotted for comparison.
Comparing Patterns of Community Structure
The considerable differences in species-level abundance estimates from the two methods pose challenges for any analyses attempting to incorporate data from both types of datasets. However, analyses that are run on each dataset in parallel suggest that both identify important ecological patterns in the region. For example, the Santa Barbara Channel exhibits strong biogeographic patterns that have been associated with gradients in temperature, as warm water associated species are more common in the south and east while cold water associated species are more common in the west (Hamilton et al., 2010). When data from each method are used to estimate the relationship between average SST and the average abundance of each species at a site (both averaged from 2003 to 2016), we find very close agreement in the slopes (correlation = 0.95, Figure 6 and Supplementary Table S2), indicating that the survey methods agree about which species are associated with warm and cold water. Two species have great leverage on this relationship: Chromis punctipinnis (blacksmith) which is strongly associated with warm water (positive slope), and Aulorhynchus flavidus (tubesnout) which is more abundant in colder parts of the region (negative slope). However, even if these two species are excluded, the relationship remains relatively strong (correlation = 0.7). We obtain similar results when evaluating temporal trends in the region. Trends over time varied from species to species, with a mix of increasing and decreasing abundances over time (Supplementary Table S3). When the species-specific trends were compared, the survey methods showed high correspondence, with a slope close to 1 and a correlation of 0.9 (Figure 7).
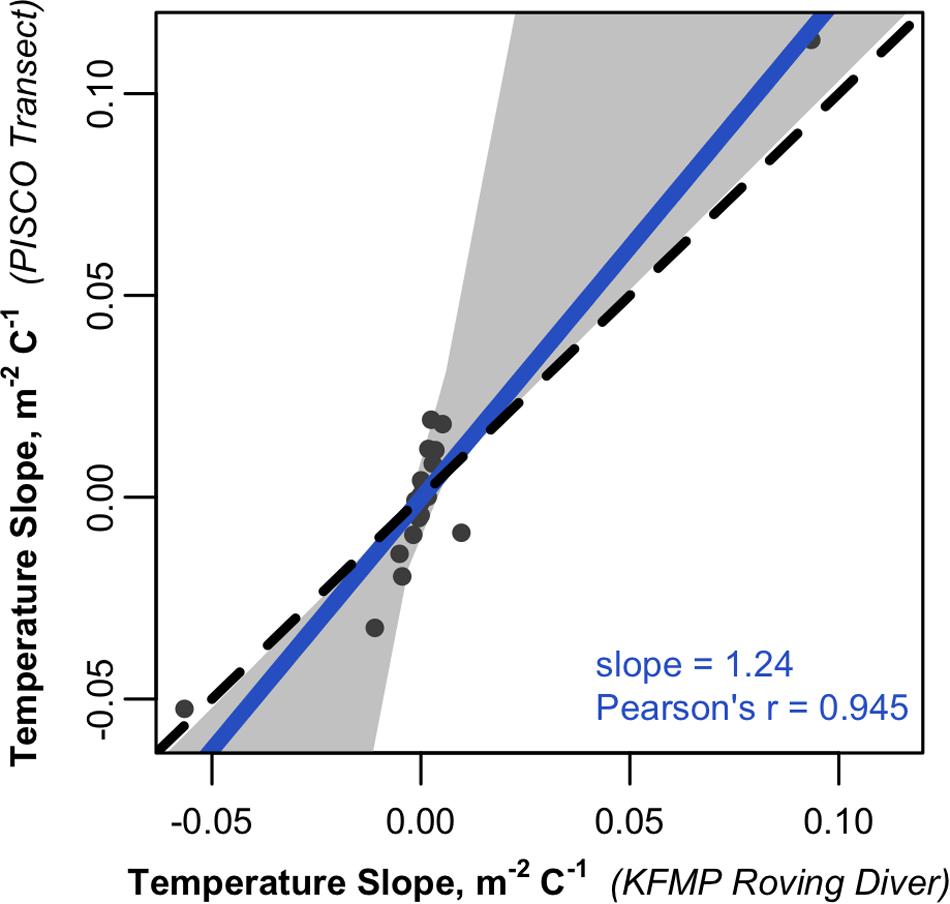
Figure 6. Scatterplot comparing the slopes of the relationship between temperature and fish densities estimated from KFMP roving diver counts to those calculated from PISCO transect data. Each point is the slope of a least squares linear regression between sea surface temperature and the density of a species observed by both programs within the region (n = 44; many dots are concentrated near the 0:0 point). The solid line represents a Deming linear regression and the dashed line represents the 1:1 line. The 0.95 confidence bounds are calculated with the bootstrap (quantile) method.
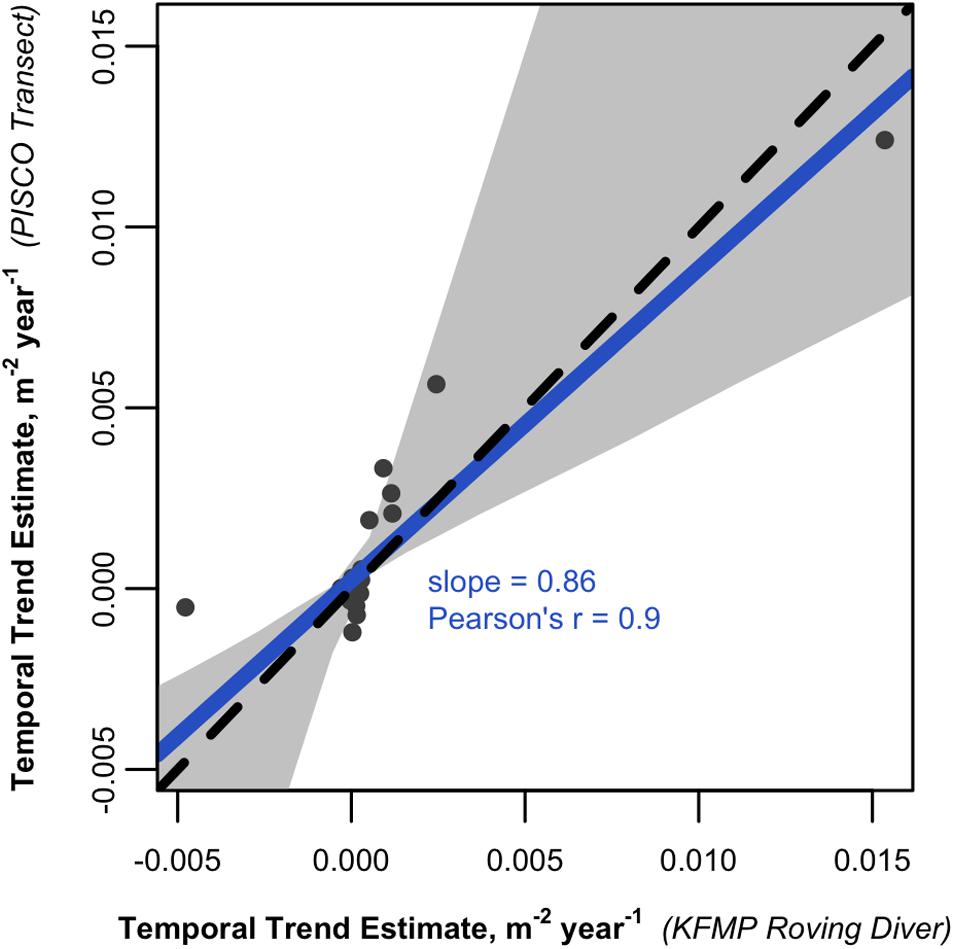
Figure 7. Scatterplot comparing the relationship between time and fish densities estimated from KFMP roving diver counts to those calculated from PISCO transect data. Each point is the coefficient for the effect of time from a linear mixed effects model between date, site and the density of a species observed by both programs within the region (n = 44; many dots are concentrated near the 0:0 point). The solid line represents a Deming linear regression and the dashed line represents the 1:1 line. The 0.95 confidence bounds are calculated with the bootstrap (quantile) method.
Discussion and Conclusion
Our analyses provide the first rigorous comparison of fish densities measured using area- and time-standardized roving diver counts to more traditional transect-based survey methodologies. While the effectiveness of roving diver counts had been evaluated previously (Schmitt et al., 2002) we are not aware of other efforts to validate the area-specific density estimates of such roving diver counts. All of our comparisons with transect-based methods suggest that the roving divers are sampling an effective area well below their nominal search zone (in this case, 2,000 m2). The slopes of these comparisons are quite varied depending on the subset of data being used (Figure 4 and Supplementary Figures S3, S4). However, all comparisons indicate that more fish per unit area are sampled with transects than with roving divers, likely because roving divers are not searching the whole target area.
Examination of the swimming speeds and search widths that are necessary to achieve different sampling areas are consistent with this explanation (Figure 5). A roving diver matching the speed and search width of PISCO’s transect-based methods would sample less than 20% of the target area in 30 min. Swim speeds and widths used for the KFMP’s fish transects are consistent with search areas of up to 1,200 m2, but still short of what would be needed to sample 2,000 m2. Moreover, such speeds would need to be maintained while enumerating and sizing all species in the fish community, throughout the entire water column, rather than the subset of 13 species sampled in KFMP transects. This is not an inherent problem with the area- and time-standardized diver counts in general, but suggests the particular methodology studied here is too ambitious in the area and volume of water in which divers are expected to count fish.
Conversely, these same results emphasize a potential advantage of the roving diver counts for documenting the presence of rare species; even conservative assumptions about area sampled suggest that a diver is covering a much larger area in a given time with the roving diver approach than with most transect-based approaches. PISCO’s transect-based approaches would cover 360 m2 of bottom in 30 min based on an assumed swim speed of 6 m min–1, but because two to three passes per transect are done at different depth strata, divers more typically spend 9 min per transect, thus covering ∼200 m2 of area in 30 min of sampling. Roving diver methods likely cover at least twice this area, meaning many more fish (and fish species) can be counted in a sampling event. For monitoring programs concerned with encountering and recording rare species, this is a substantial advantage of roving diver counts (Schmitt et al., 2002).
Several factors may explain the discrepancies we document between roving diver and transect-based counts (Figure 2). One potential source of bias can arise from non-instantaneous counts. Most fish counting methods emphasize the importance of using “mental snapshots” to avoid counting fish entering the survey area as the count progresses, but if snapshots are imperfectly implemented, and fish entering the survey area are added to an ongoing count, fish movement can lead to an overestimation of more mobile fish species (Ward Paige et al., 2010). Movement is a particular problem when fish are attracted or repulsed by the presence of the observer (Dickens et al., 2011). Biases driven by fish movement have been found in comparisons between video transects and in-person counts, as well as in simulation studies (Davis and Anderson, 1989; Ward Paige et al., 2010). This is a particularly challenging bias to account for when a species exhibits contrasting behavior in different contexts; for example, being more willing to approach divers inside of MPAs (Kulbicki, 1998; Willis et al., 2000). The low perimeter to area ratio of the large plots sampled by roving divers may mitigate these problems to some degree, as fish are less likely to move across the boundary of the sampling area while being counted. However, the large areas increase the possibility that an individual fish will be encountered multiple times within a survey, and thus recorded repeatedly.
The discrepancies documented in this study do not align perfectly with results from previous work. The most consistent difference we observed is a tendency for fish found higher in the water column to be under sampled by roving divers. This result occurs despite the fact that roving divers are nominally sampling the whole water column, while PISCO transects sample fixed depth strata; gaps between these strata mean that transects should be underestimating densities on an area-specific basis. Our finding that roving divers underestimate fish found in the midwater and canopy illustrates the difficulty of simultaneously counting fish through 10 m or more of the water column, often obscured by dense stands of giant kelp and low visibility.
There may be some tendency for abundant fish to have higher estimated densities in the transects and rare fish to be better sampled by roving divers. The most abundant fishes in the system, señorita (Oxyjulis californica) and blacksmith (Chromis punctipinnis), both have higher density estimates in the transect data than in the roving counts (these species are the leftmost two points in Supplementary Figure S2). However, these are also species commonly seen in the water column, so may be underestimated by roving divers not because of their abundance but because they are better sampled by methods with sampling strata explicitly focused on the canopy and midwater. By contrast, the roving diver counts yielded higher estimates of the abundance of several rare and cryptic species such as cabezon and moray eels, which are typically encountered sitting on the bottom or hiding in crevices. Many previous studies have argued that semi-cryptic species such as these should be better sampled by slow swimming observers working narrow transects (Willis, 2001; MacNeil et al., 2008; Bozec et al., 2011; Dickens et al., 2011), but any plausible interpretation of the area sampled by roving divers indicates they are searching a wider area than divers executing corresponding belt transects.
The freedom afforded by the roving diver count may permit observers to better find hard to observe fish, since divers can search in and around structure in a way that transect-based approaches do not allow. Roving divers often preferentially target specific fish habitats like crevices where moray eels, lingcod, and treefish hide, whereas belt transect observers must stick to the transect and move more systematically. However, this flexibility in search method comes at a cost to consistency. Transect-based approaches constrain diver behavior, ensuring that search techniques are the same for each survey, while roving diver counts offer more freedom in search strategy by design. The KFMP addresses this consistency issue by ensuring that each site gets sampled by at least three roving divers on each sampling date; although individual search strategies may vary, using multiple divers permits averaging across this variation. Issues with underwater visibility may also affect the two methods differently. Temperate reefs can be quite variable over space and time in underwater visibility, as low water clarity and giant kelp can greatly limit how far an observer can see. This variability will more strongly effect the roving diver counts, where the observer has the freedom to scan a larger area on a high visibility day.
Another potential source of variation is the level of observers’ previous survey experience. Less experienced observers tend to under-count small, mobile, or cryptic fishes, but more reliably count conspicuous or slow-moving taxa (Williams et al., 2006). Both programs studied here have well developed systems for training observers and ensuring they have extensive practice, so it is unlikely that differences in experience fully explain the variation we observed. In addition, the two groups have almost identical diversity metrics once adjusted for the number of individuals sampled (i.e., rarified species richness), suggesting one program is not systematically missing cryptic or mimic species (Figures 3B–D).
There is surprisingly low correspondence between diversity and density observations within sampling event pairs, a pattern that cannot be corrected for by adjusting assumptions about area sampled. The roving diver counts and PISCO transect counts have been chosen to cover similar depths, substrates, sampling locations and sampling dates, and yet correlations between the metrics are consistently below 0.6. Some of this may represent unavoidable variability in counting mobile species with complex behaviors; if KFMP roving divers are randomly sorted into two groups, and their counts compared, correlations between the groups are only 0.65 on average. Some scatter may also reflect a high variation at small spatial scales which has been observed previously in the system (Lamy et al., 2018), although correlations are lower than expected even in comparisons with the KFMP’s own fish transects, done on the same day and centered on precisely the same location. Variation may also derive from the sampling design in which considerable diving activity is concentrated on a small area of reef, potentially altering the fish community as sampling progresses. This further reflects the high degree of difficulty of sampling communities of mobile species (Prato et al., 2017).
Despite the low correspondence in estimated densities, the datasets agree on the direction and relative magnitude of spatial and temporal trends in the region. We observed a wide range of temperature dependence in the taxa examined, including fish with higher abundances in warmer and colder waters (Figure 6). Parallel analysis of these patterns using PISCO’s transect data and KFMP’s roving diver data gave very similar ordering of the taxa from cold to warm associated (r = 0.95) and even gave similar magnitudes ranging from Chromis punctipinnis (blacksmith) which increased in abundance by 0.1 m–1 for each °C increase in temperature in both datasets, to Aulorhynchus flavidus (tubesnout), which declined in both datasets by 0.05 m–1 for each °C increase in temperature. There was similar agreement on temporal trends (Figure 7) with a high correspondence between the programs in their estimates of which fish were increasing and decreasing over time (r = 0.86), and a slope near 1, indicating similar estimated magnitudes of change.
Taken together, our results suggest that time and area-based sampling strategies such as the roving diver fish counts are potentially useful, with particular strengths in their ability to efficiently observe rare species. They also may better sample cryptic fish associated with benthic habitats, although at costs to the ability to sample fish commonly found high in the water column. This latter limitation can partly be corrected for in data analysis by focusing on the subset of species most commonly found near the substrate, or could potentially be mitigated in future field work by depth stratifying the roving counts. We found that area-standardized roving counts may substantially under sample the nominal zone of inference, but this can be at least partly corrected for by revising assumptions about the area sampled or by reducing the area of the survey. For questions where precise measures of fish density are important, the roving diver technique may not provide adequate precision. If the roving diver fish surveys were to be redesigned (or similar surveys undertaken as part of a new project), our results suggest that the area covered should be reduced so that it could be thoroughly surveyed during the allotted time period. In such a case, the method could provide reliable density estimates while retaining the advantages for observing rare and cryptic species.
Finally, our results show that although the two datasets cannot be combined, both are useful independently, and where they show similar results, these concordant results may offer additional support for management actions. We show that some species are much more abundant in the roving diver data, while others are more abundant in transects, which suggests that combining data from these sources would produce confounded biases. However, depending on the research or management question of interest, analyzing the two datasets in parallel may provide useful insights. For example, both datasets gave similar estimates of biodiversity, similar relationships between species abundance and SST and similar trends in the abundance of species over time. Thus, both datasets have the potential to jointly inform our ecological understanding and to guide management and conservation decisions.
Data Availability Statement
The datasets analyzed in this study can be found in: RM, AR, JC, DK, D. Reed, Kevin D Lafferty, LK, and M. O’Brien. 2018. “Santa Barbara Channel Marine BON: Nearshore Kelp Forest Integrated Fish, 1981-Ongoing.” Environmental Data Initiative. https://doi.org/10.6073/pasta/0976f9969ef2789b77b659e9337c4c0c.
Author Contributions
All authors contributed to research planning and study design, and provided comments and edits on the manuscript. DK, JC, and JS collected the data. AD and LK processed and analyzed the data. AR, GH, and AD wrote the manuscript.
Funding
This research was supported by the National Parks Service (task agreement P14A01661), the National Aeronautics and Space Administration Biodiversity and Ecological Forecasting program (NASA Grant NNX-14AR62A), the Bureau of Ocean and Energy Management Ecosystem Studies program (BOEM award MC15AC00006), and NOAA in support of the Santa Barbara Channel Biodiversity Observation Network. The Partnership for Interdisciplinary Studies of Coastal Oceans is funded by the David and Lucile Packard Foundation.
Conflict of Interest
The authors declare that the research was conducted in the absence of any commercial or financial relationships that could be construed as a potential conflict of interest.
Acknowledgments
We are indebted to more than 100 divers who collected the data we analyze here as part of the National Park Service and PISCO monitoring programs. We thank Dan Okamoto, Katie Davis Koehn, Avrey Parsons-Field, and Cullen Molitor for help assembling data and for feedback on the work. We thank Channel Islands National Park and the Partnership for Interdisciplinary Studies of Coastal Oceans (PISCO) for making data available for this study. We also thank two reviewers for thoughtful comments.
Supplementary Material
The Supplementary Material for this article can be found online at: https://www.frontiersin.org/articles/10.3389/fmars.2020.00272/full#supplementary-material
References
Ayotte, P., Mccoy, K., Williams, I., Zamzow, J., Ayotte, P., Mccoy, K., et al. (2011). Coral Reef Ecosystem Division Standard Operating Procedures: Data Collection for Rapid Ecological Assessment Fish Surveys. Silver Spring: National Oceanic and Atmospheric Administration.
Beck, H. J., Feary, D. A., Figueira, W. F., and Booth, D. J. (2014). Assessing range shifts of tropical reef fishes: a comparison of belt transect and roaming underwater visual census methods. Bull. Mar. Sci. 90, 705–721. doi: 10.5343/bms.2013.1055
Bohnsack, J. A., and Bannerot, S. P. (1986). A stationary visual census technique for quantitatively assessing community structure of coral reef fishes. NOAA Tech. Rep. NMFS 41:21. doi: 10.1007/s10531-013-0611-4
Bozec, Y. M., Kulbicki, M., Laloë, F., Mou-Tham, G., and Gascuel, D. (2011). Factors affecting the detection distances of reef fish: implications for visual counts. Mar. Biol. 158, 969–981. doi: 10.1007/s00227-011-1623-9
Brock, V. E. (1954). A preliminary report on a method of estimating reef fish populations. J. Wildl. Manage. 18, 297–308.
Brzezinski, M. A., and Washburn, L. (2011). Phytoplankton primary productivity in the Santa Barbara channel: effects of wind-driven upwelling and mesoscale eddies. J. Geophys. Res. Ocean 116, 1–17. doi: 10.1029/2011JC007397
Caldwell, Z. R., Zgliczynski, B. J., Williams, G. J., and Sandin, S. A. (2016). Reef fish survey techniques: assessing the potential for standardizing methodologies. PLoS One 11:e0153066. doi: 10.1371/journal.pone.0153066
Carr, M. H., and Caselle, J. E. (2009). PISCO: Subtidal: Community Surveys: Fish Survey. Spain: PISCO.
Chao, A., Gotelli, N. J., Hsieh, T. C., Sander, E. L., Ma, K. H., Colwell, R. K., et al. (2014). Rarefaction and extrapolation with Hill numbers: a framework for sampling and estimation in species diversity studies. Ecol. Monogr. 84, 45–67. doi: 10.1890/13-0133.1
Cornbleet, P. J., and Gochman, N. (1979). Incorrect least-squares regression coefficients in method-comparison analysis. Clin. Chem. 25, 432–438. doi: 10.1093/clinchem/25.3.432
Davis, G. E., and Anderson, T. W. (1989). Population estimates of four kelp forest fishes and an evaluation of three in situ assessment techniques. Bull. Mar. Sci. 44, 1138–1151.
Davis, G. E., Richards, D. V., and Kushner, D. J. (1996). Kelp Forest Monitoring Design Review. Technical Report CHIS-96-01. Channel Islands National Park: Ventura (CA).
Dickens, L. C., Goatley, C. H. R., Tanner, J. K., and Bellwood, D. R. (2011). Quantifying relative diver effects in underwater visual censuses. PLoS One 6:e0018965. doi: 10.1371/journal.pone.0018965
Hamilton, S. L., Caselle, J. E., Malone, D. P., and Carr, M. H. (2010). Incorporating biogeography into evaluations of the Channel Islands marine reserve network. Proc. Natl. Acad. Sci. U.S.A. 107, 18272–18277. doi: 10.1073/pnas.0908091107
Hendershott, M. C., and Winant, C. D. (1996). Surface circulation in the santa barbara channel. Oceanography 9, 114–121. doi: 10.5670/oceanog.1996.14
Hsieh, T. C., Ma, K. H., and Chao, A. (2016). iNEXT: an R package for rarefaction and extrapolation of species diversity (H ill numbers). Methods Ecol. Evol. 7, 1451–1456. doi: 10.1111/2041-210x.12613
Kimmel, J. J. (1985). A new species-time method for visual assessment of fishes and its comparison with established methods. Environ. Biol. Fishes 12, 23–32. doi: 10.1007/BF00007707
Kulbicki, M. (1998). How the acquired behaviour of commercial reef fishes may influence the results obtained from visual censuses. J. Exp. Mar. Bio. Ecol. 222, 11–30. doi: 10.1016/s0022-0981(97)00133-0
Kushner, D. J., Rassweiler, A., McLaughlin, J. P., and Lafferty, K. D. (2013). A multi-decade time series of kelp forest community structure at the California Channel Islands. Ecology 94, 2655. doi: 10.1890/13-0562.1
Lamy, T., Reed, D. C., Rassweiler, A., Siegel, D. A., Kui, L., Bell, T. W., et al. (2018). Scale-specific drivers of kelp forest communities. Oecologia 186, 217–233. doi: 10.1007/s00442-017-3994-1
Linnet, K. (1998). Performance of Deming regression analysis in case of misspecified analytical error ratio in method comparison studies. Clin. Chem. 44, 1024–1031. doi: 10.1093/clinchem/44.5.1024
MacNeil, M. A., Tyler, E. H. M., Fonnesbeck, C. J., Rushton, S. P., Polunin, N. V. C., and Conroy, M. J. (2008). Accounting for detectability in reef-fish biodiversity estimates. Mar. Ecol. Prog. Ser. 367, 249–260. doi: 10.3354/meps07580
Manuilova, E., Schuetzenmeister, A., and Model, F. (2014). Mcr: Method Comparison Regression. R Packag. version 1.
McCauley, D. J., McLean, K. A., Bauer, J., Young, H. S., and Micheli, F. (2012). Evaluating the performance of methods for estimating the abundance of rapidly declining coastal shark populations. Ecol. Appl. 22, 385–392. doi: 10.1890/11-1059.1
Mieszkowska, N., Sugden, H., Firth, L. B., and Hawkins, S. J. (2014). The role of sustained observations in tracking impacts of environmental change on marine biodiversity and ecosystems. Philos. Trans. R. Soc. A Math. Phys. Eng. Sci. 372:20130339. doi: 10.1098/rsta.2013.0339
Miller, R., Rassweiler, A. R., Caselle, J. E., Kushner, D. J., Reed, D., Lafferty, K. D., et al. (2018). Santa Barbara Channel Marine BON: Nearshore Kelp Forest Integrated Fish, 1981-Ongoing. Sacramento: California Natural Resources Agency.
Nishimoto, M. M., and Washburn, L. (2002). Patterns of coastal eddy circulation and abundance of pelagic juvenile fish in the Santa Barbara Channel, California, USA. Mar. Ecol. Prog. Ser. 241, 183–199. doi: 10.3354/meps241183
Pais, M. P., and Cabral, H. N. (2018). Effect of underwater visual survey methodology on bias and precision of fish counts: a simulation approach. PeerJ 6:e5378. doi: 10.7717/peerj.5378
Pollock, M. A., Jefferson, S. G., Kane, J. W., Lomax, K., MacKinnon, G., and Winnard, C. B. (1992). Method comparison—a different approach. Ann. Clin. Biochem. 29, 556–560.
Prato, G., Thiriet, P., Franco, A., and Francour, P. (2017). Enhancing fish Underwater Visual Census to move forward assessment of fish assemblages: an application in three Mediterranean marine protected areas. PLoS One 12:e0178511. doi: 10.1371/journal.pone.0178511
Reed, D. C., Rassweiler, A. R., Miller, R. J., Page, H. M., and Holbrook, S. J. (2016). The value of a broad temporal and spatial perspective in understanding dynamics of kelp forest ecosystems. Mar. Freshw. Res. 67, 14–24. doi: 10.1071/MF14158
Reynolds, R. W., Smith, T. M., Liu, C., Chelton, D. B., Casey, K. S., and Schlax, M. G. (2007). Daily high-resolution-blended analyses for sea surface temperature. J. Clim. 20, 5473–5496. doi: 10.1175/2007JCLI1824.1
Schmitt, E., Sluka, R., and Sullivan-Sealey, K. (2002). Evaluating the use of roving diver and transect surveys to assess the coral reef fish assemblage off southeastern Hispaniola. Coral Reefs 21, 216–223. doi: 10.1007/s00338-002-0216-y
Sukhotin, A., and Berger, V. (2013). Long-term monitoring studies as a powerful tool in marine ecosystem research. Hydrobiologia 706, 1–9. doi: 10.1007/s10750-013-1456-2
Ward Paige, C., Mills Flemming, J., and Lotze, H. K. (2010). Overestimating fish counts by non-instantaneous visual censuses: consequences for population and community descriptions. PLoS One 5:e11722. doi: 10.1371/journal.pone.0011722
Williams, I. D., Walsh, W. J., Tissot, B. N., and Hallacher, L. E. (2006). Impact of observers’ experience level on counts of fishes in underwater visual surveys. Mar. Ecol. Prog. Ser. 310, 185–191. doi: 10.3354/meps310185
Willis, T. J. (2001). Visual census methods underestimate density and diversity of cryptic reef fishes. J. Fish Biol. 59, 1408–1411. doi: 10.1111/j.1095-8649.2001.tb00202.x
Willis, T. J., Millar, R. B., and Babcock, R. C. (2000). Detection of spatial variability in relative density of fishes: comparison of visual census, angling, and baited underwater video. Mar. Ecol. Prog. Ser. 198, 249–260. doi: 10.3354/meps198249
Keywords: ecology, marine biology, monitoring, sampling methods, biodiversity, Santa Barbara Channel, underwater visual census, UVC
Citation: Rassweiler A, Dubel AK, Hernan G, Kushner DJ, Caselle JE, Sprague JL, Kui L, Lamy T, Lester SE and Miller RJ (2020) Roving Divers Surveying Fish in Fixed Areas Capture Similar Patterns in Biogeography but Different Estimates of Density When Compared With Belt Transects. Front. Mar. Sci. 7:272. doi: 10.3389/fmars.2020.00272
Received: 22 August 2019; Accepted: 03 April 2020;
Published: 07 May 2020.
Edited by:
Mario Barletta, Federal University of Pernambuco, BrazilReviewed by:
Eneko Aspillaga, Instituto Mediterráneo de Estudios Avanzados, SpainFelipe Amezcua, National Autonomous University of Mexico, Mexico
Copyright © 2020 Rassweiler, Dubel, Hernan, Kushner, Caselle, Sprague, Kui, Lamy, Lester and Miller. This is an open-access article distributed under the terms of the Creative Commons Attribution License (CC BY). The use, distribution or reproduction in other forums is permitted, provided the original author(s) and the copyright owner(s) are credited and that the original publication in this journal is cited, in accordance with accepted academic practice. No use, distribution or reproduction is permitted which does not comply with these terms.
*Correspondence: A. Rassweiler, cmFzc3dlaWxlckBiaW8uZnN1LmVkdQ==