- 1School of Biological Sciences, The University of Western Australia, Crawley, WA, Australia
- 2School of Mathematics and Statistics, University of St Andrews, St Andrews, United Kingdom
- 3Centre for Research into Ecological and Environmental Modelling, University of St Andrews, St Andrews, United Kingdom
- 4Institute of Zoology, Zoological Society of London, London, United Kingdom
- 5School of Mathematical Sciences, Queensland University of Technology, Brisbane, QLD, Australia
- 6Australian Research Council Centre of Excellence for Mathematical and Statistical Frontiers, The University of Melbourne, Melbourne, VIC, Australia
- 7Geoscience Australia, Canberra, ACT, Australia
- 8UWA Oceans Institute, University of Australia, Crawley, WA, Australia
The conservation of marine biodiversity is firmly embedded in national and international policy frameworks. However, the difficulties associated with conducting broad-scale surveys of oceanic environments restrict the evidence base available for applied management in pelagic waters. For example, the Oceanic Shoals Australian Marine Park (AMP) was established in 2012 in a part of Australia’s continental shelf where unique topographic features are thought to support significant levels of biodiversity, yet where our understanding of ecological processes remains limited. We deployed mid-water baited remote underwater video systems (mid-water BRUVS) in the Oceanic Shoals AMP to provide the first non-extractive baseline assessment of pelagic wildlife communities in the area. We used these observations and high-resolution multibeam swaths of the seafloor to explore potential relationships between prominent geomorphological features and the (i) composition, (ii) richness, and (iii) relative abundance of pelagic communities. We documented 32 vertebrate species across three sampling areas, ranging from small baitfish to large sharks and rays, and estimated that up to nearly twice as many taxa may occur within the region as a whole. This highlights the Oceanic Shoals AMP as a reservoir of biodiversity comparable to other documented offshore oceanic hotspots. Our results also confirm the AMP as a possible distant foraging destination for IUCN red listed sea turtles, and a potential breeding and/or nursing ground for a number of charismatic cetaceans. Model outputs indicate that both species richness and abundance increase in proximity to raised geomorphic structures such as submerged banks and pinnacles, highlighting the influence of submarine topography on megafauna distribution. By providing a foundational understanding of spatial patterns in pelagic wildlife communities throughout a little studied region, our work demonstrates how a combination of non-destructive sampling techniques and predictive models can provide new opportunities to support decision-making under data shortage.
Introduction
Agencies responsible for the management of biodiversity must decide how to invest limited resources to maximize conservation gains (Meir et al., 2004). Doing so entails strategic trade-offs and value judgments about which aspects of the biosphere should be safeguarded as a priority, and which management actions are most sensible, socially acceptable, financially viable, urgently required, and/or likely to yield the greatest return on investment in light of current capacities and pressures (Bottrill et al., 2008). In order to be effective, such triaging requires adequate knowledge of wildlife biogeography and spatial biodiversity patterns. Without it, the magnitude of what is at risk from anthropogenic threats is difficult to appraise, as is our capacity to avert biodiversity loss (Caley et al., 2014).
Despite efforts to count and document all extant species on Earth (e.g., Census of Marine Life)1, global estimates of species numbers, including marine ones, have yet to converge (Caley et al., 2014). To date, only a fraction of extant organisms have been discovered (the so-called “Linnean shortfall”), and fewer still have been comprehensively studied (the “Wallacean shortfall”) (Whittaker et al., 2005). Extensive knowledge gaps therefore persist, especially in remote pelagic environments where sampling is often expensive and logistically difficult (Richardson and Poloczanska, 2008; Webb et al., 2010). For instance, the Timor Sea in the tropical Australian northwest is likely significant for marine mammals (Schipper et al., 2008; Double et al., 2014; Whiting, 1999), elasmobranchs (Lucifora et al., 2011; Guisande et al., 2013; Yon et al., 2020), teleost fishes (Edgar et al., 2014; Moore et al., 2017), seabirds (Clarke et al., 2011; Lavers et al., 2014), reptiles (Whiting et al., 2007; Eifes et al., 2013; Lukoschek et al., 2013; Letessier et al., 2015a), and several other taxa (Tittensor et al., 2010; Selig et al., 2014; Gagné et al., 2020), yet remains data-deficient and under-explored from a biological perspective (Moore et al., 2016). With a few exceptions (Lavers et al., 2014; Palmer et al., 2017; Thums et al., 2017), scant information exists on pelagic species diversity, habitat use, or abundance in the area – despite all of these attributes having been identified as Essential Ocean Variables (Miloslavich et al., 2018) and Essential Biodiversity Variables (Jetz et al., 2019) that are key to detecting spatio-temporal changes in marine biodiversity in the face increasing cumulative human impacts (Halpern et al., 2015, 2019). This dearth of information hampers ecological monitoring efforts at local and regional scales, and undermines appropriate evaluations of management performance within the recently established national network of Australian Marine Parks (AMPs)2. Novel approaches to data collection and analysis are thus critical to addressing existing knowledge gaps and determining the degree to which pelagic communities are represented within offshore AMPs around Australia and beyond (Letessier et al., 2019).
We present the first detailed description of pelagic wildlife occurring within the Oceanic Shoals AMP (ca. 127.5°E, 11.5°S), based on mid-water baited remote underwater video systems (mid-water BRUVS). BRUVS are now a prominent element of Australia’s strategy for standardized ocean monitoring (Bouchet et al., 2018; Przeslawski et al., 2019), and offer a robust, cost-effective, and non-invasive approach to sampling that overcomes the traditional shortcomings of diver-reliant surveys (Langlois et al., 2010; Murphy and Jenkins, 2010). Extensively used in studies of benthic assemblages inside and outside protected areas (Mallet and Pelletier, 2014), they have recently been engineered for mid-water applications (Santana-Garcon et al., 2014a; Bouchet and Meeuwig, 2015; Letessier et al., 2019), making them a suitable choice for surveying the neritic (10–250 m) depths of the Oceanic Shoals AMP. Our objectives were to: (1) document the pelagic wildlife inhabiting the western part of the park, (2) estimate total species richness in the area, and (3) quantify spatial variation in community composition, species richness, and relative abundance, in relation to seafloor geomorphology. These metrics are often broadly used to guide the establishment of marine parks and monitor their effects (Soykan and Lewison, 2015), and can be meaningful for managing zoning within them (Espinoza et al., 2014). Relative to other AMPs of comparable size, the Oceanic Shoals is one of the most geodiverse parks in the Australian marine estate (Heap and Harris, 2008). Its complex topography and extensive fields of submerged limestone banks/pinnacles, terraces, and shoals are believed to act as focal points for marine megafauna in an otherwise largely oligotrophic seascape, although little empirical evidence of such habitat preferences currently exists but see Thums et al. (2017). Here, we build statistical models to test this hypothesis and map predicted patterns in species richness and abundance. In doing so, we offer the first blueprint for understanding pelagic biogeography within the AMP. This information represents an important basis for future hypothesis-driven studies, and may aid the management of human activities in a remote and relatively understudied region subject to mounting pressures.
Materials and Methods
Study Region
The Oceanic Shoals AMP is a large and shallow park (71,740 km2, mean depth = 85 m, range = 10 to 250 m) that straddles the administrative boundary between the state of Western Australia and the Northern Territory, ca. 160 km northwest of the city of Darwin. Lying almost exclusively (99.7%) on the continental shelf, it is the sixth most geodiverse park in the Australian network (Harris et al., 2005), and connects two prominent and physically heterogeneous seafloor features: the Sahul Shelf in the west and the Van Diemen Rise to the east (Figure 1). These are characterized by flat-topped carbonate banks/pinnacles and terraces flanked by steep slopes and dissected by narrow, sinuous channels and valleys (van Andel and Veevers, 1967). Banks and pinnacles (hereafter “banks”) are knoll-shaped, hard-substrate structures, sometimes exceeding tens of kilometers in circumference, which rise to depths of 20–25 m (Hovland et al., 1994; O’Brien et al., 2002) and are composed of alternating layers of unconsolidated gravel and sandy sediments with high reactive organic matter (Przeslawski et al., 2011). Banks are thought to have developed from hydrocarbon seepage, and lay embedded in a matrix of uniform, featureless plains covered by silty clays and fine calcareous muds (van Andel and Veevers, 1967). Globally, the region’s terrain reflects a drowned estuarine lowland submerged ca. 18,000 years ago (in the Late Pleistocene to Holocene) following post-glacial rises in sea level (West, 1992). Strong seasonal fluvial runoff and intense tidal motions contribute to high levels of bed shear stress, particle transport and turbidity year-round (Porter-Smith et al., 2004; Nichol et al., 2013). Sporadic upwelling from the Timor Trough to the north sustains a large diversity of habitat-forming, filter-feeding heterotrophs such as corals, sponges, and gorgonians (Przeslawski et al., 2015).
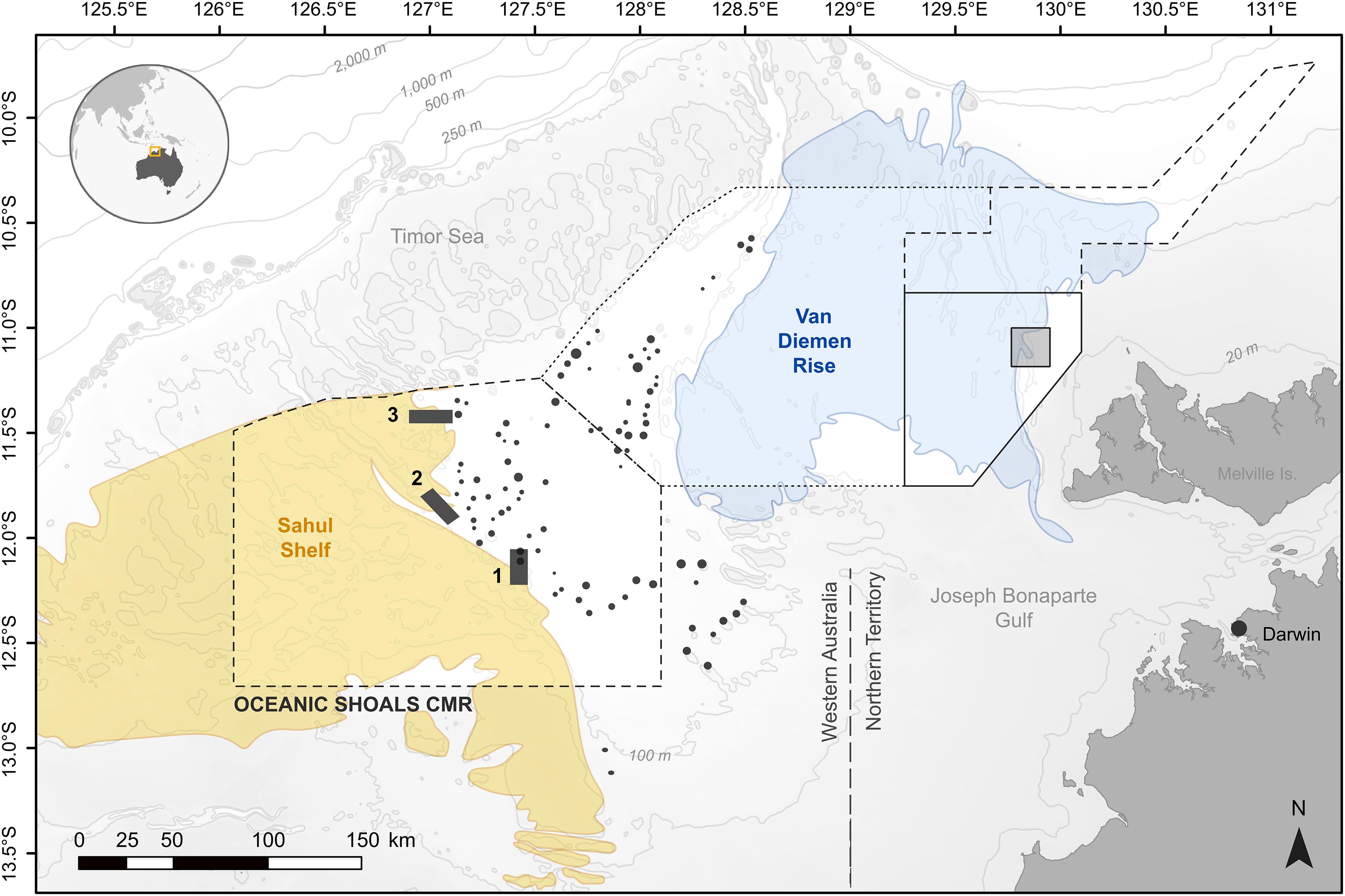
Figure 1. Map of the study region showing the survey areas (numbered 1 to 3) in relation to seabed geomorphology and the boundaries of the Oceanic Shoals Australian Marine Park (AMP). Bathymetry appears in gray, and black circles represent previously known carbonate banks. The AMP comprises four zones, namely a Multiple Use zone (IUCN VI, dashed line), a Special Purpose zone (Trawl) (IUCN VI, dotted line), a Habitat Protection zone (IUCN IV, solid line), and a National Park zone (IUCN II, shaded area). Human activities such as recreational and commercial fishing, mining, aquaculture, or shipping are permissible in the former two zones, whereas mining is prohibited in the Habitat Protection zone, and the National Park zone receives full protection.
Data Collection
Field Survey
A 21-day interdisciplinary field expedition (GA0339/SOL5650) to the Oceanic Shoals AMP was undertaken on the RV Solander in September/October 2012 by the Australian Institute of Marine Science, Geoscience Australia, the University of Western Australia, and the Museum and Art Gallery of the Northern Territory. Details of daily scientific activities were captured in a blog available at https://www.nespmarine.edu.au/rv-solander-blog. Three approximately equidistant and equal-sized areas (mean ± SD = 164.9 ± 8.3 km2) were targeted for surveying (Figure 1). Each was selected to capture a range of depths, seabed facies, and geomorphic units (Figure 2; Heap and Harris, 2008), and was sampled with a collection of geophysical, geochemical, and biological techniques, including both seabed and mid-water BRUVS (Nichol et al., 2013). Bathymetric and seabed acoustic reflectance (backscatter) data were also acquired using a Kongsberg EM3002D (300 kHz) multibeam sonar system to provide 100% coverage of the seafloor at high spatial resolution (1 m, i.e., a resolution 250× finer than the best available bathymetric maps) (Caley et al., 2015). The multibeam swath maps were interpreted in tandem with in situ sedimentological samples to generate polygon shapefiles of the region’s underlying seabed geomorphology. Geomorphological classes were as follows: bank/pinnacle, depression, mound, plain, scarp, and terrace (Figure 2). Full details of field protocols and site characteristics are given in Nichol et al. (2013).
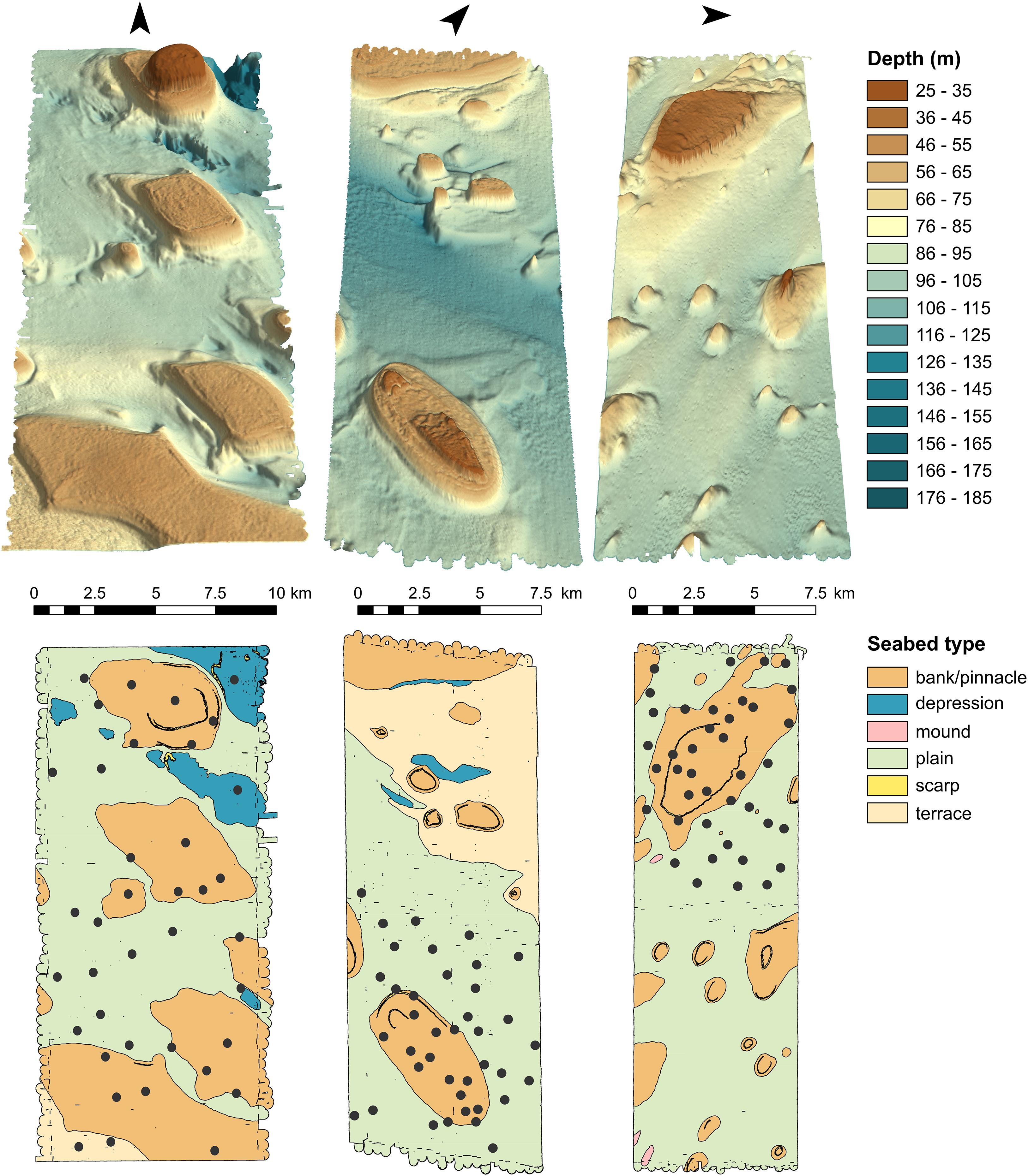
Figure 2. Bathymetry and geomorphology of the three survey areas (1–3, from left to right). Three-dimensional images of the seafloor can be seen in the upper panel. The lower panel shows the distribution of geomorphic units, interpreted from multibeam mapping. Carbonate banks cover 40.8, 19.3, and 28.2% of each area, respectively. Black circles mark the positions of mid-water BRUVS sites. Arrows indicate the direction of compass north.
Incidental visual point count surveys of air-breathing vertebrates (e.g., seabirds, cetaceans) were also carried out using compass-fitted Bushnell 7 × 50 reticle binoculars, both within and in transit between sampling areas. Whenever possible, radial distances and absolute bearings to sightings were measured, and the animals’ locations inferred from the known height of the viewing platform (top deck, 7 m above water line), time-stamped computer records of the ship’s GPS coordinates, and established trigonometry theory (Lerczak and Hobbs, 1998). Group size, group composition (if identifiable), and dominant behaviors were logged for each species (Supplementary Table S1). Due to the opportunistic nature of the counts, the resulting data were excluded from the analysis, but are reported in Supplementary Table S1.
Pelagic Videography
Mid-water BRUVS were deployed at multiple stations inside each sampling area (n1 = 38 in Area 1, including 45% on banks, n2 = 38 in Area 2, including 34% on banks, and n3 = 40 in Area 3, including 50% on banks) during daylight hours (07:00–17:00 GMT+9.30). As per Letessier et al. (2013), the instruments were suspended at a fixed depth of 10 m and moored with a plow anchor (Supplementary Figure S1). Two high-definition Hero2 GoPro cameras (set to wide field-of-view mode) mounted in a stereo-pair configuration and fitted with battery packs and 32 GB memory cards allowed the recording of wildlife activity. These are low-cost alternatives to traditional handheld video technologies (e.g., Sony HDR-CX12) that have proven effective for the ecological monitoring of natural fish communities (Letessier et al., 2015b). Deployments lasted for an average of 190 min (SD = 28 min, range = 46 to 217 min). Although soak times are conventionally shorter in baited video research, no studies had ever investigated optimal deployment durations for mid-water BRUVS at the time of the survey. Preliminary data from a pilot study conducted off Dirk Hartog Island earlier that year indicated that deployments of 120–135 min were insufficient for capturing all species (i.e., no flattening of the associated species accumulation curve) (Letessier et al., 2013). Longer soak times were also deemed necessary to allow cautious animals that first remain in the distance to more closely enter the field of view, which is critical to improve the identification of species in turbid environments such as those of the Timor Sea. To minimize pre-soak disturbance (e.g., from engine noise), mid-water BRUV units were launched while the vessel was underway. Bait arms were attached to the main frames, and made of stainless steel with a perforated PVC-coated bait canister fastened to one end (ca. 1.5 m from the cameras). Canisters were filled with pilchards (Sardinops sagax, 2.5 kg, minced using an electric meat processor), in line with conventional protocols employed throughout Australasia (Wraith et al., 2013; Bouchet et al., 2018).
Station locations were determined according to a generalized random tessellation stratified design (GRTS; Stevens and Olsen, 2004) which generates statistically robust and geographically-balanced samples that can accommodate heterogeneous inclusion probabilities (Jiménez-Valencia et al., 2014). Although its implementation is still relatively novel in Australia, GRTS has been successfully harnessed as a monitoring tool in other parts of the marine park network (Hill et al., 2014; Foster et al., 2017). The design was weighted toward shallow habitats (<50 m) to maximize data collection opportunities in the vicinity of carbonate banks whilst retaining adequate spatial coverage overall (Figure 2). As no detailed charts of regional bathymetry were available prior to the survey (Caley et al., 2015), this was done based on an interpolated nine arc-second (ca. 250 m at the equator) depth grid of the country’s exclusive economic zone3. However, high turbidity and minimal ambient light made concurrent demersal surveys (i.e., benthic BRUV deployments conducted by partners from the Australian Institute of Marine Science) logistically difficult and little effective beyond 60 m (Nichol et al., 2013). Sampling plans were therefore revised in Areas 2 and 3 to limit surveys within this depth range. Stations were separated by a minimum distance of 500 m to curtail spatial auto-correlation and reduce the potential overlap of bait plumes emanating from adjacent units (Santana-Garcon et al., 2014b).
Data Treatment
Video Processing
Mid-water BRUV videos were post-processed and annotated using the SeaGIS software EventMeasure4. All animals were identified to the lowest possible taxonomic level, and counted to yield standard assemblage metrics including species richness and MaxN, a conservative index of relative abundance that avoids double counting and corresponds to the maximum number of individuals of each species visible at any one time (Priede et al., 1994). MaxN values were then summed to generate a measure of total relative abundance for each deployment (Total MaxN) (Rees et al., 2014). Because sympatric Australian (Carcharhinus tilstoni) and common (Carcharhinus limbatus) blacktip sharks are known to hybridize and co-occur in equal frequencies (Ovenden et al., 2010; Morgan et al., 2012) but cannot be confidently distinguished other than by genetic sampling, we labeled all blacktip sharks (and potential hybrids) as C. tilstoni (Espinoza et al., 2014). Furthermore, Australian blacktip, spot-tail (Carcharhinus sorrah) and graceful (Carcharhinus amblyrhynchoides) sharks can be difficult to tell apart on video and were thus lumped together as a tilstoni/sorrah/amblyrhynchoides species complex. Narrow-barred (Scomberomorus commerson) and broad-barred (Scomberomorus semifasciatus) mackerels were grouped together for the same reasons (Supplementary Figure S2).
Trophic Levels
We also retrieved the trophic level (TL) of all species from FishBase (Froese and Pauly, 2018) using the R package rfishbase (Boettiger et al., 2012; see Supplementary Table S2). However, TLs are not documented or known for all species. Where TL values were missing, species were assigned the mean trophic level of all their congeners (or all species within the same family, as appropriate) whose known distributions encompassed the Eastern Indian Ocean (FAO Area 57) and/or the Western Central Pacific (FAO Area 71). FishBase contains two trophic position metrics (FoodTroph and DietTroph), and we relied on FoodTroph because of its higher abundance of records compared to DietTroph. Cetaceans and reptiles are not included in FishBase and were assigned TL values based on expert knowledge and a review of the published literature. We assigned killer whales (Orcinus orca) a TL of 4.5 (Pauly et al., 1998), sea snakes (Hydrophiidae spp.) a TL of 4.0 (based on Hydrophis ornatus as a best approximation of taxonomic identity), and olive ridley sea turtles (Lepidochelys olivacea) a TL of 3.1 (Peavey et al., 2017).
Geomorphometrics
A suite of nine geomorphometrics was derived from the multi-beam bathymetry grids (Supplementary Figure S3), and chosen to capture a range of meaningful terrain attributes (Bouchet et al., 2015). Among them were indices of seabed aspect, slope, and curvature, as well as several measures of terrain variability retained to encapsulate independent facets of topographic complexity, and calculated on both small (100 m) and large (1000 m) scales (Lecours et al., 2017; Supplementary Table S3). The topographic position index (TPI) was preferred over the relative difference to mean value (Lecours et al., 2017) as it could be more easily calculated at different spatial scales, given available software. Sea surface temperature, salinity, and fluorescence (a proxy for primary productivity) were also initially considered but not retained due to collinearity, inadequate resolution, insufficient spatial coverage, or a combination of the above. Each raster grid was upscaled to a 50 m resolution via bilinear interpolation before analysis. This was accomplished both to speed up computing and because the focal algorithm used to calculate seabed curvatures was mathematically constrained to a fixed 3 × 3 neighborhood around a center cell (i.e., giving a 3 m window on a 1 m resolution raster initially). This scale was deemed too fine to match the processes likely affecting the distribution of mobile pelagic species. The choice of 50 m was arbitrary, but seen as an acceptable compromise between minimizing information loss and maintaining a resolution that is appreciably greater than the best available national bathymetry dataset for the region (Caley et al., 2015).
Data Analysis
All analyses were undertaken in R v3.6.0.
Species Rarefaction
We constructed a sample-based rarefaction curve (Gotelli and Colwell, 2001) from the mid-water BRUVS data using the R package iNEXT (Hsieh et al., 2016). The curve gives the statistical expectation of the corresponding species accumulation, and was extrapolated to N = 5,000 individuals using the numerical derivations presented in Colwell et al. (2012). We assumed observations originated from a wider statistical universe (i.e., the entire theoretical assemblage), and generated robust unconditional confidence intervals that do not converge to zero at the full-sample end of the curve (Colwell et al., 2012).
PERMANOVA
We tested for differences in the structure of pelagic communities detected on, and away from, banks (coded as a fixed, two-level factor, geom) using a one-way non-parametric multivariate analysis of variance (PERMANOVA) implemented in the vegan package (Oksanen et al., 2018). A large body of research illustrates the benefits of this technique in teasing out ecological patterns from BRUVS-based experiments conducted across a variety of habitat conditions and water column positions (e.g., Claudet et al., 2010; Gladstone et al., 2012; Zintzen et al., 2012; Santana-Garcon et al., 2014a). Computations were performed on fourth root-transformed data and based on the zero-adjusted Bray Curtis dissimilarity measure, which ignores (uninformative) joint absences (Clarke et al., 2006). Variance partitioning was achieved after conditioning upon deployment length as an input covariable. Robust tests of individual terms were obtained by permutation under a reduced model (n = 9,999; type III conditional sums of squares). If any significant p-values (α = 0.05) were returned, a test for homogeneity of dispersions (PERMDISP) was done, and a similarity percentage routine (SIMPER) run to gauge the contribution of each species to discriminating assemblages as a function of geomorphology (Wakefield et al., 2013).
Spatial Modeling
We developed generalized additive mixed models (GAMMs) of both species richness (henceforth S) and Total MaxN (henceforth N) (Pedersen et al., 2019) in the mgcv R package (Wood, 2004) assuming Poisson distributions with log link functions, as is appropriate for count data. Our focus here was only on higher-order megafauna, which we defined as those species with TLs ≥ 3.5, in keeping with Tremblay-Boyer et al. (2011) and Lassalle et al. (2012). We chose GAMMs due to their success in modeling non-linear species-environment relationships (Brodie et al., 2020) and in trading off good predictive performance in unsampled conditions with reasonable ecologically intelligibility (Derville et al., 2018). Our underlying expectation was that both S and N would increase toward shallower banks (i.e., lower depth, higher backscatter), where animals supposedly aggregate. To test for this effect, we coded depth and backscatter as a tensor product term (Wood, 2006). We adopted a full-subsets approach, whereby all combinations of candidate geomorphometrics were considered up to a maximum of four uncorrelated terms per model (Pearson correlation cut-off <| 0.7|) (Lambert et al., 2014) and ranked according to their second-order Akaike’s Information Criterion (AICc) scores. We limited basis sizes to k = 4 (Best et al., 2012) in order to capture dominant ecological relationships (Mannocci et al., 2017) and we relied on restricted maximum likelihood as the criterion for model optimization as it penalizes overfitting and leads to more pronounced optima (Wood, 2011). Each model was built using thin plate splines and included an offset term for effort (i.e., the log of the duration of the video clips, in minutes), as well as a random effect term identifying each sampling area. All outputs were inspected for overdispersion, autocorrelation, and violations of residual assumptions. Finally, we created combined prediction maps from the GAMM models for each sampling area. We did this by adapting the methods of Dunstan et al. (2012) and splitting predictions of S and N into deciles, before assigning to each grid cell an additive score equal to the normalized sum of its decile ranks. This approach provides a simple approximation of the bivariate density distribution of richness and abundance values. While the decision to use decile splits was arbitrary, it was made to allow a reasonable trade-off between interpretability and the ability to capture patterns in the bivariate density (Dunstan et al., 2012). The resulting index goes from zero (low S and low N) to one (high S and high N), and can be seen as an index of pelagic “hotspots”. To mitigate extrapolation, predictions were constrained within the univariate range of each covariate (Sequeira et al., 2018a) and within an alpha-hull encompassing depth and backscatter values at sampling sites.
Uncertainty Propagation
We assessed uncertainty due to both model selection and parameter estimation using Monte Carlo techniques, as described in Wenger et al. (2013). We started by resampling the BRUVS data (non-parametric Bootstrap with replacement, n = 100 times) and building GAMMs for each replicate dataset, as per the methods above. With every run, we recorded which model minimized the AICc, allowing us to build a frequency table of the best model formulations (Supplementary Table S4). We then randomly selected a model out of this best set, with a probability equal to its frequency value. This strategy is consistent with current practices in Bayesian model averaging (Wenger et al., 2013). Next, we randomly drew values from the multivariate distribution of all fixed effects included in the chosen model. We used these to build replicate GAMMs, keeping track of all bootstrap outputs and predictions, and quantifying uncertainty as the robust coefficient of variation (rCV) across Bootstrap replicates. rCV is a modified form of the traditional CV which is less sensitive to skewness and outliers (Arachchige et al., 2019) and is calculated as rCV = 100 × normalized IQR/median, where IQR is the interquartile range (25–75 percentile) and the normalized IQR is 0.7413 × IQR (Temnerud et al., 2013).
Results
Species Richness
In a total of 368.5 h of sampling effort, we recorded 1,693 individuals from 30 identified species (corresponding to 13 taxonomic families), nearly a third of which were sharks and rays (Supplementary Table S2 and Supplementary Figure S4). The most abundant species were mackerel scads (Decapterus macarellus), unicorn leatherjackets (Aluterus monoceros), and sharks from the Carcharhinus complex, together accounting for 84% of N. Among sharks, species from the Carcharhinus complex were also the most prevalent, being observed at 44% of all sampled sites. One mobulid (the oceanic manta ray, Manta birostris), one cetacean (the killer whale, Orcinus orca) and one hydrophiid species (likely the ornate reef sea snake, Hydrophis ornatus, although identification was uncertain) were also filmed, as well as three olive ridley sea turtles (Lepidochelys olivacea), as detailed elsewhere (Letessier et al., 2015a). Both species richness and total MaxN were highest in Area 2 (S = 19, N = 589), followed by Area 3 (S = 18, N = 480) and Area 1 (S = 17, N = 570). S varied from zero to eight across sites, with a mean of 2.9 ± 1.5 SD. Abundance ranged from zero to 84 across sites, with a mean of 14.4 ± 15.8 SD. Three species of cetaceans were additionally documented in 17.5 h of opportunistic visual surveys: the bottlenose dolphin (Tursiops truncatus), the killer whale, and the false killer whale (Pseudorca crassidens) (Supplementary Figure S5). Their pods ranged in size from three to 25 individuals across species, and frequently comprised calves or juveniles seen resting, foraging and socializing (Supplementary Table S1).
Analytical rarefaction of the mid-water BRUVS samples yielded estimates of species richness per standardized sample of 40 and 50 individuals of S40 = 6.7 (95% CI = 6.3–7.1) and S50 = 7.4 (95% CI = 6.9–7.9), respectively (Figure 3), in line with previous studies conducted off Australia on the same array of taxonomic groups within a similar latitudinal range (Worm et al., 2003, 2005; Morato et al., 2010). Extrapolation suggested an asymptote of 38 species (95% CI = 28–48). A sample-based rarefaction based on species incidence data (see R code) indicates that an additional ca. 411 h of video footage would be required to document this number of species.
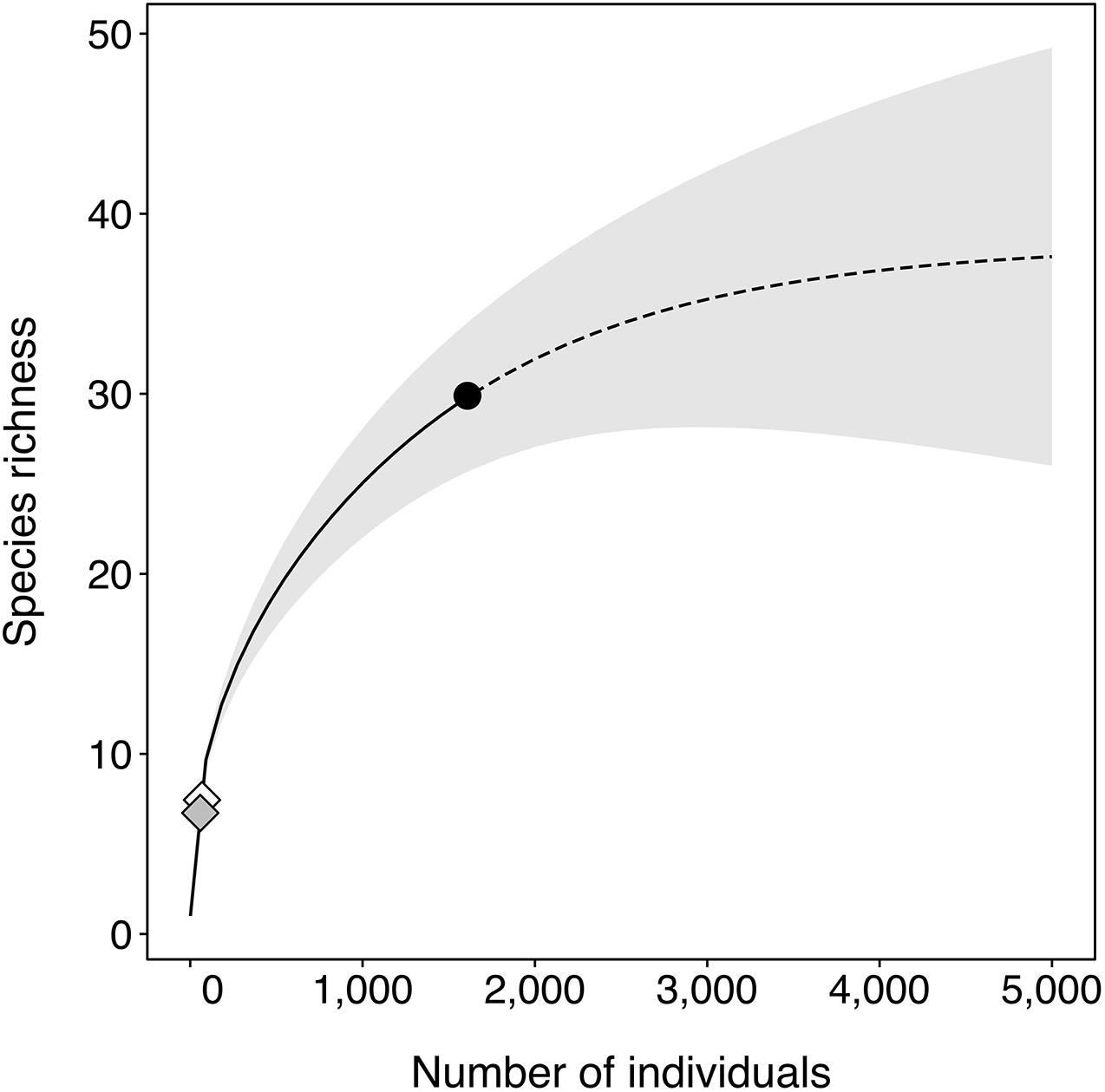
Figure 3. Individual-based rarefaction (solid line) and extrapolation (dashed line) of species richness (S) from mid-water BRUVS samples, with 95% unconditional confidence interval (shaded area). The reference value (i.e., observed species richness) is shown as a filled black circle. Diamonds mark the estimated value of S corresponding to samples of 40 (gray) and 50 (white) individuals, for comparison with previous studies (see section “Species Richness”).
Community Structure
Pelagic communities associated with banks were significantly distinct from those elsewhere (geom, p = 0.0109, Table 1). Twelve species accounted for >90% of this dissimilarity, with mackerel scads, unicorn leatherjackets, and sharks from the Carcharhinus complex again best discriminating assemblages between banks and surrounding plains (cumulative contribution >50%, Supplementary Table S5). C. tilstoni and C. sorrah are both commercially exploited, the former being a major component of the northern Australian gillnet fishery (Ovenden et al., 2010; Harry et al., 2013), and occurred in greater numbers on banks (Av. Ab = 0.60) than away from them (Av. Ab = 0.38). By contrast, more mobile and wider-ranging species like the tiger shark (Galeocerdo cuvier) and the scalloped hammerhead shark (Sphyrna lewini) were observed in deeper waters, consistent with their known movement and diving behaviors (Duncan and Holland, 2006). However, individual contributions remained low (<10%) for most species, indicating that changes in their individual abundance, rather than occurrence, were likely responsible for the observed patterns.
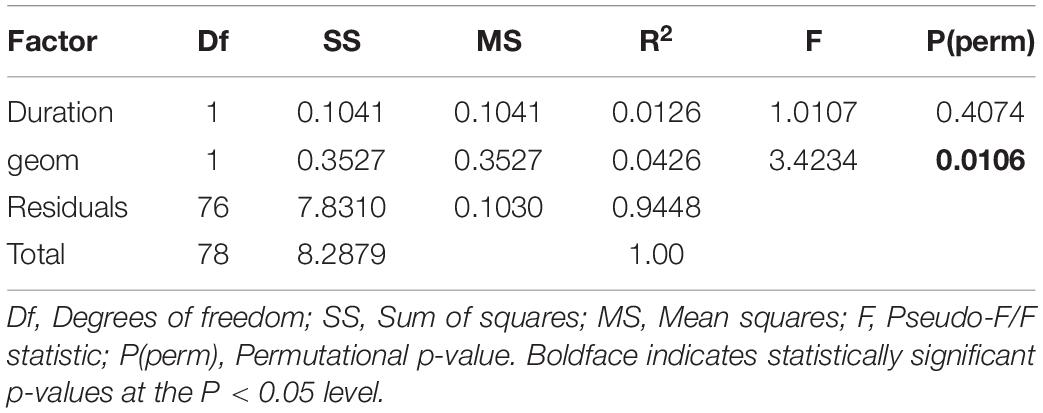
Table 1. Permutational multivariate analysis of variance (PERMANOVA) of the influence of seabed geomorphology on the structure of pelagic species assemblages in the Oceanic Shoals Australian Marine Park, based on zero-adjusted Bray-Curtis distance matrices derived from fourth-root transformed data. geom is a two-level fixed factor (on bank, off bank). Duration represents the duration of each video clip, in minutes.
Spatial Models
Generalized additive mixed models revealed a significant interaction between seabed depth and acoustic backscatter (p-values <0.05), with an increase in both S and N generally predicted in the vicinity of shallower, harder-substrate banks (Figures 4, 5). Some variation was apparent at the scale of individual banks, with higher values of S and N often fringing the banks’ margins (Supplementary Figures S6–S8). The best model for S explained 12.3% of the deviance, and only retained the tensor product term of depth × backscatter. By contrast, the best model for N explained 52.1% of the deviance, and included not only the tensor product term but also significant smooths for seabed curvature and the large-scale topographic position index, suggesting higher abundance over more convex and complex terrain (Supplementary Figure S9). Uncertainty was greater in model predictions of N, particularly along bank margins.
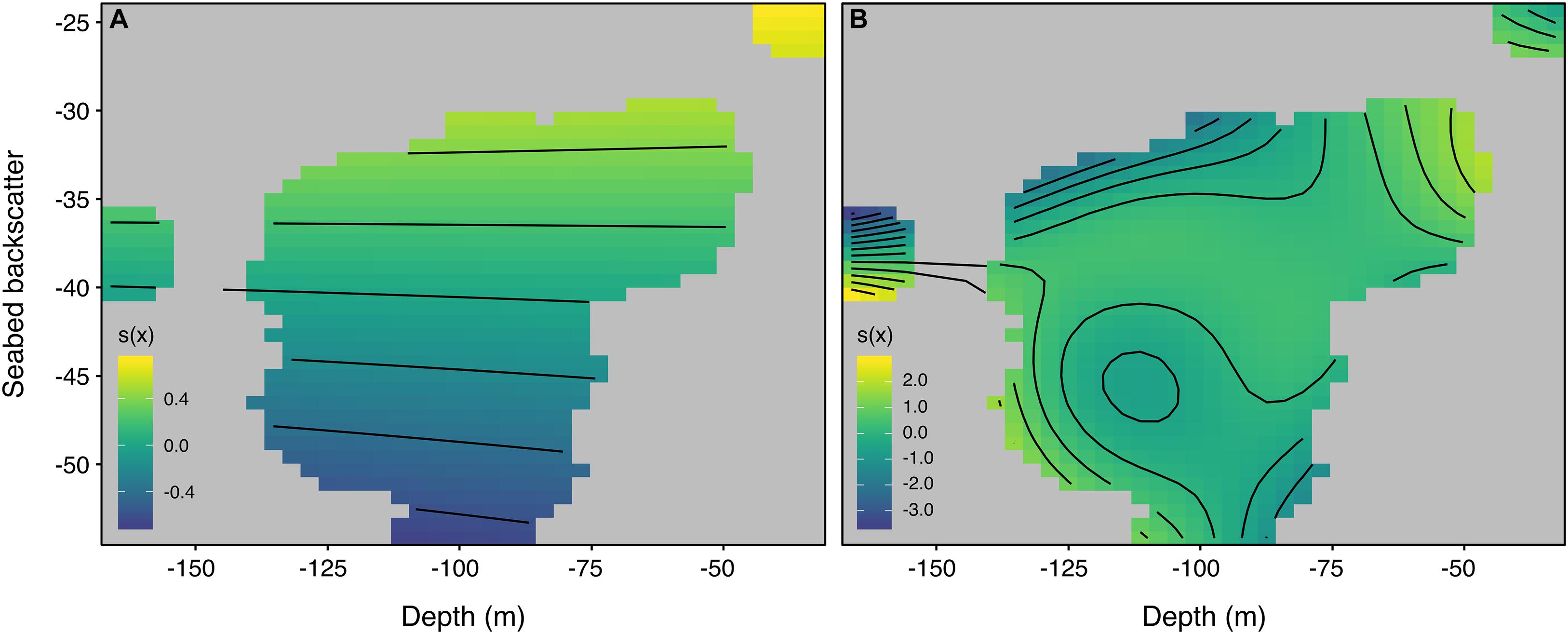
Figure 4. Tensor product smooths for the non-linear interaction between seabed depth (in meters) and acoustic backscatter (in decibels) in the models of (A) species richness, S, and (B) species relative abundance, N, minimizing the second-order Akaike’s Information Criterion (AICc). Models explained 12.3 and 52.1% of the deviance, respectively.
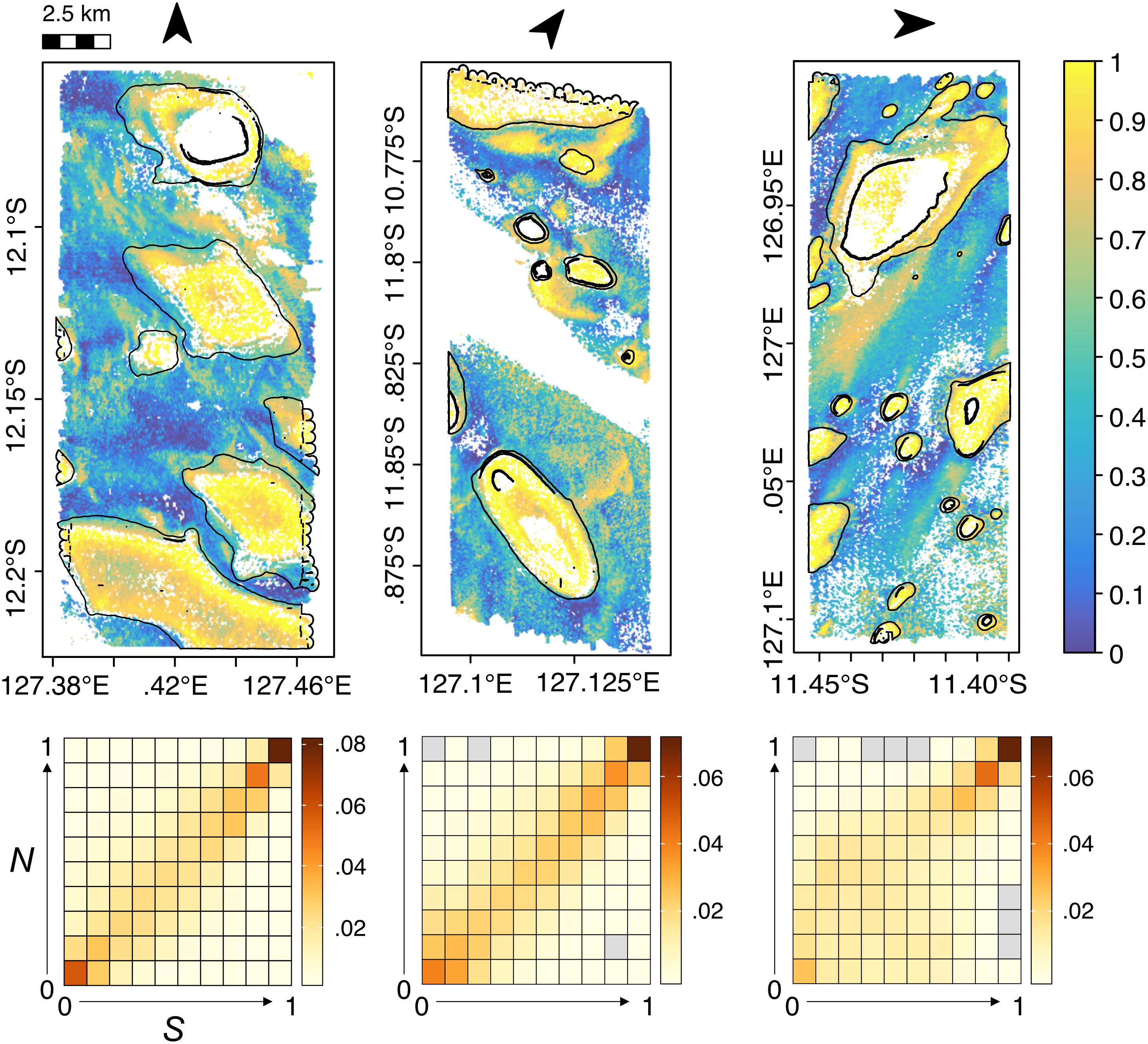
Figure 5. Maps of pelagic “hotspots” within sub-areas of the Oceanic Shoals Australian Marine Park (AMP), as predicted from the best GAMM models. Top: Median additive decile ranks (normalized to 0–1) calculated from predictions of species richness (S) and abundance (N) in each sampling site (1 to 3 from left to right). Yellow tones represent areas where both S and N are high (i.e., hotspots), whereas blue tones denote areas where both S and N are low (cold spots). Areas outside the covariate range of environmental predictors are left white (see main text for details). Bottom: Bivariate partitioning of S and N used in deriving the hotspot index. Gray cells denote combinations that were not observed in the data. The outlines of carbonate banks are superimposed as solid black lines. Arrows indicate the direction of compass north.
Discussion
Effective ocean conservation requires knowledge of the spatial ecology of marine species and the identification of essential habitats supporting their populations (Ward-Paige et al., 2012). We used non-destructive baited videography to begin the task of understanding patterns in the structure, richness, and abundance of pelagic communities across a vast, geodiverse seascape: the Oceanic Shoals AMP. We identified 32 taxa (30 on mid-water BRUVS, another two during visual point counts) spanning a broad array of life histories, phylogenies, and trophic guilds, from small schooling planktivores to large-bodied carnivores (Supplementary Table S2). Our estimate of asymptotic richness suggests that up to 48 species may be present in the area (Figure 3), consistent with other previously described open-ocean hotspots at similar latitudes (despite methodological discrepancies; Worm et al., 2003, 2005; Morato et al., 2010). Importantly, our work aligns with previous observations that pelagic hotspots are associated with prominent topographic features at local scales (Worm et al., 2003; Fontes et al., 2014; Vassallo et al., 2018). It also complements a growing body of research documenting the Timor Sea as a conspicuous and globally significant epicenter of biodiversity (Tittensor et al., 2010; Moore et al., 2017).
Marine organisms commonly form predictable aggregations at sites of abrupt topography such as submarine canyons or seamounts (Nur et al., 2011; Aïssi et al., 2012; Garrigue et al., 2015; Bouchet et al., 2017; Sutton et al., 2019). The combined importance of seabed backscatter and water depth in our models suggests that the region’s carbonate banks may play a similar role in attracting pelagic megafauna. This pattern is consistent with recent evidence that flatback (Natator depressus) and olive ridley turtles migrate to the complex seafloor of the Oceanic Shoals AMP to forage during the post-nesting season (Whiting et al., 2007; Thums et al., 2017). While the animals’ preferred prey are unknown, studies of sessile epifauna across the Van Diemen Rise and the eastern Joseph Bonaparte Gulf point to unique and dense mixed sponge and coral gardens on banks, mounds, shoals, and other raised geomorphic structures (Przeslawski et al., 2014). By promoting structural heterogeneity and providing substrate and shelter for numerous benthic fish and invertebrates, banks may concentrate prey and act as critical links in regional benthic-pelagic coupling pathways through enhanced predation opportunities. Similarly to Moore et al. (2017), our models also predicted elevated wildlife abundance around bank slopes and edges [although note that models of N performed far better than models of S, contrary to recent studies in other, perhaps more biologically complex, systems; Sequeira et al. (2018b)]. This could be explained by enhanced productivity caused by turbulent mixing and cryptic upwelling events likely taking place as tidal currents are directed around the banks, as evidenced by tidal scouring of the seabed pockmarks in their periphery (Picard et al., 2014). However, the region’s highly turbid waters will typically block exposure to ambient light beyond 60 m (Nichol et al., 2013), such that nutrient enrichment from physical forcing might be insufficient to fully sustain pelagic food webs where banks lie too deep to allow photosynthesis to occur. Additional research examining fine-scale interactions between ocean currents, complex seafloor geomorphology, and benthic/pelagic productivity is needed to disentangle the mechanisms underlying patterns of species occurrence inside this AMP (Moore et al., 2017).
Overall, carcharhinids were among the most diverse and dominant taxa, and we documented consistent patterns of co-occurrence between blacktip (C. tilstoni) and spot-tail (C. sorrah) sharks. These two species are generally site-faithful with small home ranges (Stevens et al., 2000). It is plausible, therefore, that resident populations may occupy the AMP year-round, with implications for the sustainability of the commercial fishing activities of which they are targets (Field et al., 2012). By contrast, tiger sharks (G. cuvier) display characteristically variable movements. While some animals undertake nomadic journeys that cover thousands of kilometers annually, others concentrate on discrete reefs for feeding and reproduction (Speed et al., 2010). In the absence of spatially and temporally explicit tracking data, the drivers of their presence in AMP are unclear. Likewise, how cetaceans use the park also remains unresolved, as no estimates of abundance, demographic trends or geographic dispersal presently exist for offshore odontocetes in the Timor Sea (Allen et al., 2012; but see Palmer et al., 2017). In this context, several observations of young calves and juveniles in multiple survey locations offer tentative preliminary evidence that several cetacean species may occupy the area at some point during their early life stages.
Due to its simplicity and intuitiveness, species richness has long remained a favored metric in conservation priority setting. A critical yet risky assumption underlying its use is that richness values can be readily compared, both spatially and temporally. In practice, sampling error, intensity, coverage, and detectability can all affect richness estimates to some degree (Fleishman et al., 2006). The usefulness of richness for monitoring has therefore been met with mounting controversy (Hillebrand et al., 2018) prompting calls to develop composite indicators of biodiversity across scales from genes through to ecosystems (Lyashevska and Farnsworth, 2012). However, in the face of uncertain budgets and unpredictable opportunities to invest in conservation, simple decision rules such as protecting richness hotspots may actually prove effective, especially when management actions are implemented incrementally over time (Meir et al., 2004). This is arguably the case for the Australian Marine Park network, which was proclaimed in 2012 but whose management zonation took more than 6 years to come into effect. Fleishman et al. (2006) argue that species richness retains value only if considered in consort with metrics of species endemism, threat, or abundance. With this in mind, we combined analyses of community structure with spatial models of both S and N, and reported on a list of species attributes (e.g., exploitation status, group composition when known, vulnerability; Supplementary Table S2) to facilitate improvements in our ecological understanding of the Oceanic Shoals AMP for monitoring and decision-making. This is an important initial step toward supporting park management, yet we caution that our data only represent a snapshot of species occurrence in a single season, meaning that any temporal trends cannot currently be resolved from this initial survey. The dynamics of species immigration, extinction, and taxonomic turnover are complex, and apparent stability in diversity patterns can mask potentially substantial changes in community composition (Hillebrand et al., 2018). Repeat surveys will thus be required before temporal trends in pelagic biodiversity within the AMP can be estimated with any confidence.
BRUVS are prone to a number of biases, including imperfect detection in turbid environments, and preferential recording of animals that are more active or defensive of the bait (Dunlop et al., 2014). Note, for example, that it is very difficult to determine the rate at which bait degrades and releases into the water column, making bait dispersal a key unanswered question in BRUVS research currently (Whitmarsh et al., 2017). The problem is exacerbated by the fact that oceanographic conditions (e.g., swell, current speed, etc.) may vary from site to site, and it is our experience that some of the bait canisters seemed to empty more quickly than others. There is no simple solution to this, and the implications of these shortcomings have been discussed at length elsewhere (Pelletier et al., 2011; Taylor et al., 2013; Mallet and Pelletier, 2014; Bouchet and Meeuwig, 2015). However, in deploying mid-water BRUVS inside the Oceanic Shoals AMP, we seized a critical opportunity to trial and validate baited videography in open ocean conditions away from coasts. In doing so, we addressed an essential methodological need (Santana-Garcon et al., 2014c), as few other non-invasive techniques would have yielded insights into pelagic communities at an equally appropriate level of detail. Large-scale monitoring programmes for offshore marine reserves demand rapidly deployable tools that can achieve high levels of replication (Letessier et al., 2019). Mid-water BRUVS are highly effective in this regard, and now indeed form part of a national strategy for standardized biological sampling in Australian waters (Bouchet et al., 2018; Przeslawski et al., 2019).
The place-based management of the open ocean requires the maintenance of biodiversity values in the face of competing industry interests. At one end of the spectrum, pristine and remote sites are often chosen as logical candidates for protection as they make conservation possible with minimal interruption or displacement of human activities (Singleton and Roberts, 2014), while buying insurance against future change. At the other end, pressing needs for threat reduction and population recovery prevail and focus is directed on chronically perturbed systems affected by high anthropogenic use. The pelagic waters of the Timor Sea are some of the least degraded “wilderness areas” on the planet, owing to historically low levels of resource extraction by international standards (Trebilco et al., 2011). Yet, ongoing offshore petroleum industry activity and recent evidence of widespread illegal fishing and ghost netting indicate rising risks of disturbance off northern Australia (Field et al., 2009; Wilcox et al., 2013; Edyvane and Penny, 2017). The capacity of the region to withstand these pressures is difficult to ascertain given current gaps in our understanding of species distributions and resilience. Furthermore, the submerged shoals and banks of the Oceanic Shoals AMP have been earmarked as key ecological features (KEFs) by the Australian Government but remain drastically under-represented in no-take sanctuaries (Moore et al., 2016). This limited protection is concerning as few marine industries in the north-west operate at depths greater than 200 m, making continental-shelf habitats such as the ones found within the AMP those most exposed to anthropogenic activities (Moore et al., 2016). The development of robust and cost-effective strategies for conducting regional-scale assessments of biodiversity assets that can support spatial planning throughout the Australian EEZ is therefore both timely and necessary. Integrating different observational techniques such as swath mapping and baited videography, as illustrated here, can be useful for disentangling species-habitat relationships and is thus a key step in addressing this shortfall.
Data Availability Statement
Example video footage can be viewed online at https://goo.gl/DGa9hE. R code for running the analyses can be downloaded from GitHub at https://github.com/pjbouchet/oceanicshoals. The pelagic BRUVS data are available through the Australian Ocean Data Network at http://data.imas.utas.edu.au/portal/search.
Ethics Statement
The animal study was reviewed and approved by the University of Western Australia’s Animal Ethics Committee (AE Permit RA/3/100/1090).
Author Contributions
MC, JM, PB, TL, and SN conceived the ideas and designed the methodology. PB, TL, and SN collected the data. JH provided software and technical assistance. PB conducted the analyses and wrote the manuscript. All authors contributed critically to the drafts and gave final approval for publication.
Funding
This research was undertaken for the Marine Biodiversity Hub, a collaborative partnership supported through funding from the Australian Government’s National Environmental Research Program (NERP). PB was the recipient of a scholarship for international fees during the course of this work. SN publishes with permission of the Chief Executive Officer, Geoscience Australia.
Conflict of Interest
The authors declare that the research was conducted in the absence of any commercial or financial relationships that could be construed as a potential conflict of interest.
Acknowledgments
We are grateful to all expedition members and importantly to the master and crew of the RV Solander for their expertise, dedication and hospitality during fieldwork. We thank L. Groves for logistical support as well as L. Ferreira, F. Garcia-Solis Marchant, and S. Hodge for assistance with video processing. G. Fry and K. Sanders helped with sea snake identifications.
Supplementary Material
The Supplementary Material for this article can be found online at: https://www.frontiersin.org/articles/10.3389/fmars.2020.00530/full#supplementary-material
Footnotes
- ^ http://www.coml.org/
- ^ https://parksaustralia.gov.au/marine/
- ^ http://www.ausseabed.gov.au/home
- ^ http://www.seagis.com.au
References
Aïssi, M., Fiori, C., and Alessi, J. (2012). “Mediterranean submarine canyons as stepping stones for pelagic top predators: the case of the sperm whale,” in Mediterranean Submarine Canyons: Ecology and Governance, ed. M. Würtz (Gland: IUCN), 99–104.
Allen, S. J., Cagnazzi, D. D., Hodgson, A. J., Loneragan, N. R., and Bejder, L. (2012). Tropical inshore dolphins of north-western Australia: unknown populations in a rapidly changing region. Pacific Conserv. Biol. 18, 56–63. doi: 10.1071/PC120056
Arachchige, C. N. P. G., Prendergast, L. A., and Staudte, R. G. (2019). Robust analogues to the coefficient of variation. arXiv [Preprint].
Best, B. D., Halpin, P. N., Read, A. J., Fujioka, E., Good, C. P., LaBrecque, E. A., et al. (2012). Online cetacean habitat modeling system for the US East Coast and Gulf of Mexico. Endanger. Species Res. 18, 1–15. doi: 10.3354/esr00430
Boettiger, C., Lang, D. T., and Wainwright, P. C. (2012). Rfishbase: exploring, manipulating and visualizing FishBase data from R. J. Fish Biol. 81, 2030–2039. doi: 10.1111/j.1095-8649.2012.03464.x
Bottrill, M. C., Joseph, L. N., Carwardine, J., Bode, M., Cook, C., Game, E. T., et al. (2008). Is conservation triage just smart decision making? Trends Ecol. Evol. 23, 649–654. doi: 10.1016/j.tree.2008.07.007
Bouchet, P. J., and Meeuwig, J. (2015). Drifting baited stereo-videography: a novel sampling tool for surveying pelagic wildlife in offshore marine reserves. Ecosphere 6:art137. doi: 10.1890/ES14-00380.1
Bouchet, P. J., Meeuwig, J., Huveneers, C., Langlois, T., Letessier, T., Lowry, M., et al. (2018). “Marine sampling field manual for pelagic BRUVS (Baited Remote Underwater Videos),” in Field Manuals for Marine Sampling to Monitor Australian Waters, eds R. Przeslawski and S. Foster (Canberra, ACT: National Environmental Science Programme, Australian Government), 105–132.
Bouchet, P. J., Meeuwig, J. J., Huang, Z., Letessier, T. B., Nichol, S. L., Caley, M. J., et al. (2017). Continental-scale hotspots of pelagic fish abundance inferred from commercial catch records. Glob. Ecol. Biogeogr. 26, 1098–1111. doi: 10.1111/geb.12619
Bouchet, P. J., Meeuwig, J. J., Salgado Kent, C. P., Letessier, T. B., and Jenner, C. K. (2015). Topographic determinants of mobile vertebrate predator hotspots: current knowledge and future directions. Biol. Rev. 90, 699–728. doi: 10.1111/brv.12130
Brodie, S. J., Thorson, J. T., Carroll, G., Hazen, E. L., Bograd, S., Haltuch, M. A., et al. (2020). Trade-offs in covariate selection for species distribution models: a methodological comparison. Ecography (Cop) 43, 11–24. doi: 10.1111/ecog.04707
Caley, M. J., Fisher, R., and Mengersen, K. (2014). Global species richness estimates have not converged. Trends Ecol. Evol. 29, 187–188. doi: 10.1016/j.tree.2014.02.002
Caley, M. J., Nichol, S., Przeslawski, R., Kool, J., Meeuwig, J., and Bouchet, P. (2015). Oceanic Shoals Commonwealth Marine Reserve – a guide/Fact sheet. Canberra, ACT: National Environmental Science Programme, Australian Government.
Clarke, K. R., Somerfield, P. J., and Chapman, M. G. (2006). On resemblance measures for ecological studies, including taxonomic dissimilarities and a zero-adjusted Bray–Curtis coefficient for denuded assemblages. J. Exp. Mar. Bio. Ecol. 330, 55–80. doi: 10.1016/j.jembe.2005.12.017
Clarke, R. H., Carter, M., Swann, G., and Thomson, J. (2011). The status of breeding seabirds and herons at Ashmore Reef, off the Kimberley Coast, Australia. J. R. Soc. West. Aust. 94, 365–376.
Claudet, J., Lenfant, P., and Schrimm, M. (2010). Snorkelers impact on fish communities and algae in a temperate marine protected area. Biodivers. Conserv. 19, 1649–1658. doi: 10.1007/s10531-010-9794-0
Colwell, R. K., Chao, A., Gotelli, N. J., Lin, S. Y., Mao, C. X., Chazdon, R. L., et al. (2012). Models and estimators linking individual-based and sample-based rarefaction, extrapolation and comparison of assemblages. J. Plant Ecol. 5, 3–21. doi: 10.1093/jpe/rtr044
Derville, S., Torres, L. G., Iovan, C., and Garrigue, C. (2018). Finding the right fit: comparative cetacean distribution models using multiple data sources and statistical approaches. Divers. Distrib. 24, 1657–1673. doi: 10.1111/ddi.12782
Double, M. C., Andrews-Goff, V., Jenner, K. C. S., Jenner, M. N., Laverick, S. M., Branch, T. A., et al. (2014). Migratory movements of pygmy blue whales (Balaenoptera musculus brevicauda) between Australia and Indonesia as revealed by satellite telemetry. PLoS ONE 9:e93578. doi: 10.1371/journal.pone.0093578
Duncan, K. M., and Holland, K. N. (2006). Habitat use, growth rates and dispersal patterns of juvenile scalloped hammerhead sharks Sphyrna lewini in a nursery habitat. Mar. Ecol. Prog. Ser. 312, 211–221. doi: 10.3354/meps312211
Dunlop, K. M., Marian Scott, E., Parsons, D., and Bailey, D. M. (2014). Do agonistic behaviours bias baited remote underwater video surveys of fish? Mar. Ecol. 36, 810–818. doi: 10.1111/maec.12185
Dunstan, P. K., Bax, N. J., Foster, S. D., Williams, A., and Althaus, F. (2012). Identifying hotspots for biodiversity management using rank abundance distributions. Divers. Distrib. 18, 22–32. doi: 10.1111/j.1472-4642.2011.00838.x
Edgar, G. J., Stuart-Smith, R. D., Willis, T. J., Kininmonth, S., Baker, S. C., Banks, S., et al. (2014). Global conservation outcomes depend on marine protected areas with five key features. Nature 506, 216–220. doi: 10.1038/nature13022
Edyvane, K. S., and Penny, S. S. (2017). Trends in derelict fishing nets and fishing activity in northern Australia: implications for trans-boundary fisheries management in the shared Arafura and Timor Seas. Fish. Res. 188, 23–37. doi: 10.1016/j.fishres.2016.11.021
Eifes, C. T., Livingstone, S. R., Lane, A., Lukosche, V., Sanders, K. L., Courtney, A. J., et al. (2013). Fascinating and forgotten: the conservation status of marine elapid snakes. Herpetol. Conserv. Biol. 8, 37–52.
Espinoza, M., Cappo, M., Heupel, M. R., Tobin, A. J., and Simpfendorfer, C. A. (2014). Quantifying shark distribution patterns and species-habitat associations: implications of Marine Park zoning. PLoS ONE 9:e106885. doi: 10.1371/journal.pone.0106885
Field, I. C., Buckworth, R. C., Yang, G. J., Meekan, M. G., Johnson, G., Stevens, J. D., et al. (2012). Changes in size distributions of commercially exploited sharks over 25 years in northern Australia using a Bayesian approach. Fish. Res. 12, 262–271. doi: 10.1016/j.fishres.2012.03.005
Field, I. C., Meekan, M. G., Buckworth, R. C., and Bradshaw, C. J. A. (2009). Protein mining the world’s oceans: australasia as an example of illegal expansion-and-displacement fishing. Fish Fish. 10, 323–328. doi: 10.1111/j.1467-2979.2009.00325.x
Fleishman, E., Noss, R. F., and Noon, B. R. (2006). Utility and limitations of species richness metrics for conservation planning. Ecol. Indic. 6, 543–553. doi: 10.1016/j.ecolind.2005.07.005
Fontes, J., Schmiing, M., and Afonso, P. (2014). Permanent aggregations of a pelagic predator at shallow seamounts. Mar. Biol. 161, 1349–1360. doi: 10.1007/s00227-014-2423-9
Foster, S. D., Hosack, G. R., Lawrence, E., Przeslawski, R., Hedge, P., Caley, M. J., et al. (2017). Spatially balanced designs that incorporate legacy sites. Methods Ecol. Evol. 8, 1433–1442. doi: 10.1111/2041-210X.12782
Froese, R., and Pauly, D. (2018). FishBase. Available online at: http://www.fishbase.org (accessed March 01, 2020).
Gagné, T. O., Reygondeau, G., Jenkins, C. N., Sexton, J. O., Bograd, S. J., Hazen, E. L., et al. (2020). Towards a global understanding of the drivers of marine and terrestrial biodiversity. PLoS ONE 15:e0228065. doi: 10.1371/journal.pone.0228065
Garrigue, C., Clapham, P. J., Geyer, Y., Kennedy, A. S., and Zerbini, A. N. (2015). Satellite tracking reveals novel migratory patterns and the importance of seamounts for endangered South Pacific humpback whales. R. Soc. Open Sci. 2:150489. doi: 10.1098/rsos.150489
Gladstone, W., Lindfield, S., Coleman, M., and Kelaher, B. (2012). Optimisation of baited remote underwater video sampling designs for estuarine fish assemblages. J. Exp. Mar. Bio. Ecol. 429, 28–35. doi: 10.1016/j.jembe.2012.06.013
Gotelli, N. J., and Colwell, R. K. (2001). Quantifying biodiversity: procedures and pitfalls in the measurement and comparison of species richness. Ecol. Lett. 4, 379–391. doi: 10.1046/j.1461-0248.2001.00230.x
Guisande, C., Patti, B., Vaamonde, A., Manjarrés-Hernández, A., Pelayo-Villamil, P., García-Roselló, E., et al. (2013). Factors affecting species richness of marine elasmobranchs. Biodivers. Conserv. 22, 1703–1714. doi: 10.1007/s10531-013-0507-3
Halpern, B. S., Frazier, M., Afflerbach, J., Lowndes, J. S., Micheli, F., O’Hara, C., et al. (2019). Recent pace of change in human impact on the world’s ocean. Sci. Rep. 9:11609. doi: 10.1038/s41598-019-47201-9
Halpern, B. S., Frazier, M., Potapenko, J., Casey, K. S., Koenig, K., Longo, C., et al. (2015). Spatial and temporal changes in cumulative human impacts on the world’s ocean. Nat. Commun. 6:art7615. doi: 10.1038/ncomms8615
Harris, P., Heap, A., Passlow, V., Sbaffi, L., Fellows, M., Porter-Smith, R., et al. (2005). Geomorphic features of the continental margin of Australia. Geosci. Austr. Record 200:142.
Harry, A. V., Tobin, A. J., and Simpfendorfer, C. A. (2013). Age, growth and reproductive biology of the spot-tail shark, Carcharhinus sorrah, and the Australian blacktip shark, C. tilstoni, from the Great Barrier Reef World Heritage Area, north-eastern Australia. Mar. Freshw. Res. 64, 277–293. doi: 10.1071/MF12142
Heap, A. D., and Harris, P. T. (2008). Geomorphology of the Australian margin and adjacent seafloor. Aust. J. Earth Sci. 55, 555–585. doi: 10.1080/08120090801888669
Hill, N. A., Barrett, N., Lawrence, E., Hulls, J., Dambacher, J. M., Nichol, S., et al. (2014). Quantifying fish assemblages in large, offshore marine protected areas: an Australian case study. PLoS ONE 9:e110831. doi: 10.1371/journal.pone.0110831
Hillebrand, H., Blasius, B., Borer, E. T., Chase, J. M., Downing, J. A., Eriksson, B. K., et al. (2018). Biodiversity change is uncoupled from species richness trends: consequences for conservation and monitoring. J. Appl. Ecol. 55, 169–184. doi: 10.1111/1365-2664.12959
Hovland, M., Croker, P. F., and Martin, M. (1994). Fault-associated seabed mounds (carbonate knolls?) off western Ireland and north-west Australia. Mar. Pet. Geol. 11, 232–246. doi: 10.1016/0264-8172(94)90099-X
Hsieh, T. C., Ma, K. H., and Chao, A. (2016). iNEXT: an R package for rarefaction and extrapolation of species diversity (Hill numbers). Methods Ecol. Evol. 7, 1451–1456. doi: 10.1111/2041-210X.12613
Jetz, W., McGeoch, M. A., Guralnick, R., Ferrier, S., Beck, J., Costello, M. J., et al. (2019). Essential biodiversity variables for mapping and monitoring species populations. Nat. Ecol. Evol. 3, 539–551. doi: 10.1038/s41559-019-0826-1
Jiménez-Valencia, J., Kaufmann, P., Sattamini, A., Mugnai, R., and Baptista, D. (2014). Assessing the ecological condition of streams in a southeastern Brazilian basin using a probabilistic monitoring design. Environ. Monit. Assess. 186, 4685–4695. doi: 10.1007/s10661-014-3730-9
Lambert, C., Mannocci, L., Lehodey, P., and Ridoux, V. (2014). Predicting cetacean habitats from their energetic needs and the distribution of their prey in two contrasted tropical regions. PLoS ONE 9:e105958. doi: 10.1371/journal.pone.0105958
Langlois, T. J., Harvey, E. S., Fitzpatrick, B., Meeuwig, J. J., Shedrawi, G., and Watson, D. L. (2010). Cost-efficient sampling of fish assemblages: comparison of baited video stations and diver video transects. Aquat. Biol. 9, 155–168. doi: 10.3354/ab00235
Lassalle, G., Gascuel, D., Le Loc’h, F., Lobry, J., Pierce, G. J., Ridoux, V., et al. (2012). An ecosystem approach for the assessment of fisheries impacts on marine top predators: the Bay of Biscay case study. ICES J. Mar. Sci. 69, 925–938. doi: 10.1093/icesjms/fss049
Lavers, J. L., Miller, M. G. R., Carter, M. J., Swann, G., and Clarke, R. H. (2014). Predicting the spatial distribution of a seabird community to identify priority conservation areas in the Timor Sea. Conserv. Biol. 28, 1699–1709. doi: 10.1111/cobi.12324
Lecours, V., Devillers, R., Simms, A. E., Lucieer, V. L., and Brown, C. J. (2017). Towards a framework for terrain attribute selection in environmental studies. Environ. Model. Softw. 89, 19–30. doi: 10.1016/j.envsoft.2016.11.027
Lerczak, J. A., and Hobbs, R. C. (1998). Calculating sighting distances from angular readings during shipboard, aerial, and shore-based marine mammal surveys. Mar. Mammal Sci. 14, 590–598. doi: 10.1111/j.1748-7692.1998.tb00745.x
Letessier, T. B., Bouchet, P. J., Reisser, J., and Meeuwig, J. J. (2015a). Baited videography reveals remote foraging and migration behaviour of sea turtles. Mar. Biodivers. 45, 609–610. doi: 10.1007/s12526-014-0287-3
Letessier, T. B., Juhel, J.-B., Vigliola, L., and Meeuwig, J. J. (2015b). Low-cost small action cameras in stereo generates accurate underwater measurements of fish. J. Exp. Mar. Bio. Ecol. 466, 120–126. doi: 10.1016/j.jembe.2015.02.013
Letessier, T. B., Meeuwig, J. J., Gollock, M., Groves, L., Bouchet, P. J., Chapuis, L., et al. (2013). Assessing pelagic fish populations: the application of demersal video techniques to the mid-water environment. Methods Oceanogr. 8, 41–55. doi: 10.1016/j.mio.2013.11.003
Letessier, T. B., Mouillot, D., Bouchet, P. J., Vigliola, L., Fernandes, M. C., Thompson, C., et al. (2019). Remote reefs and seamounts are the last refuges for marine predators across the Indo-Pacific. PLoS Biol. 17:e3000366. doi: 10.1371/journal.pbio.3000366
Lucifora, L. O., García, V. B., and Worm, B. (2011). Global diversity hotspots and conservation priorities for sharks. PLoS One 6:e19356. doi: 10.1371/journal.pone.0019356
Lukoschek, V., Beger, M., Ceccarelli, D., Richards, Z., and Pratchett, M. (2013). Enigmatic declines of Australia’s sea snakes from a biodiversity hotspot. Biol. Conserv. 166, 191–202. doi: 10.1016/j.biocon.2013.07.004
Lyashevska, O., and Farnsworth, K. D. (2012). How many dimensions of biodiversity do we need? Ecol. Indic. 18, 485–492. doi: 10.1016/j.ecolind.2011.12.016
Mallet, D., and Pelletier, D. (2014). Underwater video techniques for observing coastal marine biodiversity: a review of sixty years of publications (1952-2012). Fish. Res. 154, 44–62. doi: 10.1016/j.fishres.2014.01.019
Mannocci, L., Roberts, J. J., Miller, D. L., and Halpin, P. N. (2017). Extrapolating cetacean densities to quantitatively assess human impacts on populations in the high seas. Conserv. Biol. 31, 601–614. doi: 10.1111/cobi.12856
Meir, E., Andelman, S., and Possingham, H. P. (2004). Does conservation planning matter in a dynamic and uncertain world? Ecol. Lett. 7, 615–622. doi: 10.1111/j.1461-0248.2004.00624.x
Miloslavich, P., Bax, N. J., Simmons, S. E., Klein, E., Appeltans, W., Aburto-Oropeza, O., et al. (2018). Essential ocean variables for global sustained observations of biodiversity and ecosystem changes. Glob. Chang. Biol. 24, 2416–2433. doi: 10.1111/gcb.14108
Moore, C., Cappo, M., Radford, B., and Heyward, A. (2017). Submerged oceanic shoals of north Western Australia are a major reservoir of marine biodiversity. Coral Reefs 36, 719–734. doi: 10.1007/s00338-017-1564-y
Moore, C. H., Radford, B. T., Possingham, H. P., Heyward, A. J., Stewart, R. R., Watts, M. E., et al. (2016). Improving spatial prioritisation for remote marine regions: optimising biodiversity conservation and sustainable development trade-offs. Sci. Rep. 6:32029. doi: 10.1038/srep32029
Morato, T., Hoyle, S. D., Allain, V., and Nicol, S. J. (2010). Seamounts are hotspots of pelagic biodiversity in the open ocean. Proc. Natl. Acad. Sci. U.S.A. 107, 9707–9711. doi: 10.1073/pnas.0910290107
Morgan, J. A. T., Harry, A. V., Welch, D. J., Street, R., White, J., Geraghty, P. T., et al. (2012). Detection of interspecies hybridisation in Chondrichthyes: hybrids and hybrid offspring between Australian (Carcharhinus tilstoni) and common (C. limbatus) blacktip shark found in an Australian fishery. Conserv. Genet. 13, 455–463. doi: 10.1007/s10592-011-0298-6
Murphy, H. M., and Jenkins, G. P. (2010). Observational methods used in marine spatial monitoring of fishes and associated habitats: a review. Mar. Freshw. Res. 61, 236–252. doi: 10.1071/MF09068
Nichol, S. L., Howard, F. J. F., Kool, J., Stowar, M., Bouchet, P. J., Radke, L., et al. (2013). Oceanic Shoals Commonwealth Marine Reserve (Timor Sea) Biodiversity Survey: GA0339/SOL5650 – Post-Survey Report. Townsville: Australian Institute of Marine Science.
Nur, N., Jahncke, J., Herzog, M. P., Howar, J., Hyrenbach, K. D., Zamon, J. E., et al. (2011). Where the wild things are: predicting hotspots of seabird aggregations in the California Current System. Ecol. Appl. 21, 2241–2257. doi: 10.1890/10-1460.1
O’Brien, G. W., Glenn, K., Lawrence, G., Williams, A. K., Webster, M., Burns, S., et al. (2002). Influence of hydrocarbon migration and seepage on benthic communities in the Timor Sea, Australia. APPEA J. 42:225. doi: 10.1071/aj01013
Oksanen, J. F., Blanchet, G., Friendly, M., Kindt, R., Legendre, P., McGlinn, D., et al. (2018). Vegan: Community Ecology Package. R Package Version 2.5-2. Available online at: https://cran.r-project.org; https://github.com/vegandevs/vegan (accessed March, 2020).
Ovenden, J. R., Morgan, J. A. T., Kashiwagi, T., Broderick, D., and Salini, J. (2010). Towards better management of Australia’s shark fishery: genetic analyses reveal unexpected ratios of cryptic blacktip species Carcharhinus tilstoni and C. limbatus. Mar. Freshw. Res. 61, 253–262. doi: 10.1071/MF09151
Palmer, C., Baird, R. W., Webster, D. L., Edwards, A. C., Patterson, R., Withers, A., et al. (2017). A preliminary study of the movement patterns of false killer whales (Pseudorca crassidens) in coastal and pelagic waters of the Northern Territory, Australia. Mar. Freshw. Res. 68, 1726–1733. doi: 10.1071/MF16296
Pauly, D., Trites, A. W., Capuli, E., and Christensen, V. (1998). Diet composition and trophic levels of marine mammals. ICES J. Mar. Sci. 55, 467–481. doi: 10.1006/jmsc.1997.0280
Peavey, L. E., Popp, B. N., Pitman, R. L., Gaines, S. D., Arthur, K. E., Kelez, S., et al. (2017). Opportunism on the high seas: foraging ecology of olive ridley turtles in the eastern Pacific Ocean. Front. Mar. Sci. 4:348. doi: 10.3389/fmars.2017.00348
Pedersen, E. J., Miller, D. L., Simpson, G. L., and Ross, N. (2019). Hierarchical generalized additive models in ecology: an introduction with mgcv. PeerJ 7:e6876. doi: 10.7717/peerj.6876
Pelletier, D., Leleu, K., Mou-Tham, G., Guillemot, N., and Chabanet, P. (2011). Comparison of visual census and high definition video transects for monitoring coral reef fish assemblages. Fish. Res. 107, 84–93. doi: 10.1016/j.fishres.2010.10.011
Picard, K., Siwabessy, J., Radke, L., and Nichol, S. (2014). “The use of seabed scoured depressions as a proxy for near-seabed flow,” in GEOHAB (Marine Geological and Biological Habitat Mapping) 2014 Conference (Eastsound, WA: GEOHAB).
Porter-Smith, R., Harris, P. T., Andersen, O. B., Coleman, R., Greenslade, D., and Jenkins, C. J. (2004). Classification of the Australian continental shelf based on predicted sediment threshold exceedance from tidal currents and swell waves. Mar. Geol. 211, 1–20. doi: 10.1016/j.margeo.2004.05.031
Priede, I. G., Bagley, P., Smith, A., Creasey, S., and Merrett, N. (1994). Scavenging deep demersal fishes of the Porcupine Seabight, North-East Atlantic: observations by baited camera, trap and trawl. J. Mar. Biol. Assoc. U.K. 74, 481–498. doi: 10.1017/S0025315400047615
Przeslawski, R., Alvarez, B., Battershill, C., and Smith, T. (2014). Sponge biodiversity and ecology of the Van Diemen Rise and eastern Joseph Bonaparte Gulf, northern Australia. Hydrobiologia 730, 1–16. doi: 10.1007/s10750-013-1799-8
Przeslawski, R., Alvarez, B., Kool, J., Bridge, T., Caley, M. J., and Nichol, S. (2015). Implications of sponge biodiversity patterns for the management of a marine reserve in Northern Australia. PLoS ONE 10:e0141813. doi: 10.1371/journal.pone.0141813
Przeslawski, R., Daniell, J., Anderson, T., Barrie, J. V., Heap, A., Hughes, M., et al. (2011). Seabed Habitats and Hazards of the Joseph Bonaparte Gulf and Timor Sea, northern Australia. Canberra: Geoscience Australia.
Przeslawski, R., Foster, S., Monk, J., Barrett, N., Bouchet, P., Carroll, A., et al. (2019). A suite of field manuals for marine sampling to monitor Australian waters. Front. Mar. Sci. 6:177. doi: 10.3389/fmars.2019.00177
Rees, M. J., Jordan, A., Price, O. F., Coleman, M. A., and Davis, A. R. (2014). Abiotic surrogates for temperate rocky reef biodiversity: implications for marine protected areas. Divers. Distrib. 20, 284–296. doi: 10.1111/ddi.12134
Richardson, A. J., and Poloczanska, E. S. (2008). Under-resourced, under threat. Science (80-) 320, 1294–1295. doi: 10.1126/science.1156129
Santana-Garcon, J., Braccini, M., Langlois, T. J., Newman, S. J., McAuley, R. B., and Harvey, E. S. (2014a). Calibration of pelagic stereo-BRUVS and scientific longline surveys for sampling sharks. Methods Ecol. Evol. 5, 824–833. doi: 10.1111/2041-210x.12216
Santana-Garcon, J., Newman, S. J., and Harvey, E. S. (2014b). Development and validation of a mid-water baited stereo-video technique for investigating pelagic fish assemblages. J. Exp. Mar. Bio. Ecol. 452, 82–90. doi: 10.1016/j.jembe.2013.12.009
Santana-Garcon, J., Newman, S. J., Langlois, T. J., and Harvey, E. S. (2014c). Effects of a spatial closure on highly mobile fish species: an assessment using pelagic stereo-BRUVS. J. Exp. Mar. Bio. Ecol. 460, 153–161. doi: 10.1016/j.jembe.2014.07.003
Schipper, J., Chanson, J. S., Chiozza, F., Cox, N. A., Hoffmann, M., Katariya, V., et al. (2008). The status of the world’s land and marine mammals: diversity, threat, and knowledge. Science (80-) 322, 225–230. doi: 10.1126/science.1165115
Selig, E. R., Turner, W. R., Troëng, S., Wallace, B. P., Halpern, B. S., Kaschner, K., et al. (2014). Global priorities for marine biodiversity conservation. PLoS ONE 9:e82898. doi: 10.1371/journal.pone.0082898
Sequeira, A. M. M., Bouchet, P. J., Yates, K. L., Mengersen, K., and Caley, M. J. (2018a). Transferring biodiversity models for conservation: opportunities and challenges. Methods Ecol. Evol. 9, 1250–1264. doi: 10.1111/2041-210X.12998
Sequeira, A. M. M., Mellin, C., Lozano-Montes, H. M., Meeuwig, J. J., Vanderklift, M. A., Haywood, M. D. E., et al. (2018b). Challenges of transferring models of fish abundance between coral reefs. PeerJ 2018:e4566. doi: 10.7717/peerj.4566
Singleton, R. L., and Roberts, C. M. (2014). The contribution of very large marine protected areas to marine conservation: giant leaps or smoke and mirrors? Mar. Pollut. Bull. 87, 7–10. doi: 10.1016/j.marpolbul.2014.07.067
Soykan, C. U., and Lewison, R. L. (2015). Using community-level metrics to monitor the effects of marine protected areas on biodiversity. Conserv. Biol. 29, 775–783. doi: 10.1111/cobi.12445
Speed, C. W., Field, I. C., Meekan, M. G., and Bradshaw, C. J. A. (2010). Complexities of coastal shark movements and their implications for management. Mar. Ecol. Prog. Ser. 408, 275–293. doi: 10.3354/meps08581
Stevens, D. L., and Olsen, A. R. (2004). Spatially balanced sampling of natural resources. J. Am. Stat. Assoc. 99, 262–278. doi: 10.1198/016214504000000250
Stevens, J. D., West, G. J., and McLoughlin, K. J. (2000). Movements, recapture patterns, and factors affecting the return rate of carcharhinid and other sharks tagged off northern Australia. Mar. Freshw. Res. 51, 127–141. doi: 10.1071/MF98158
Sutton, A. L., Jenner, K. C. S., and Jenner, M.-N. M. (2019). Habitat associations of cetaceans and seabirds in the tropical eastern Indian Ocean. Deep Sea Res. Part II Top. Stud. Oceanogr. 166, 171–186. doi: 10.1016/J.DSR2.2018.06.002
Taylor, M. D., Baker, J., and Suthers, I. M. (2013). Tidal currents, sampling effort and baited remote underwater video (BRUV) surveys: are we drawing the right conclusions? Fish. Res. 140, 96–104. doi: 10.1016/j.fishres.2012.12.013
Temnerud, J., Düker, A., Karlsson, S., Allard, B., Bishop, K., Fölster, J., et al. (2013). Spatial patterns of some trace elements in four Swedish stream networks. Biogeosciences 10, 1407–1423. doi: 10.5194/bg-10-1407-2013
Thums, M., Waayers, D., Huang, Z., Pattiaratchi, C., Bernus, J., and Meekan, M. (2017). Environmental predictors of foraging and transit behaviour in flatback turtles Natator depressus. Endanger. Species Res. 32, 333–349. doi: 10.3354/esr00818
Tittensor, D. P., Mora, C., Jetz, W., Lotze, H. K., Ricard, D., Berghe, E., et al. (2010). Global patterns and predictors of marine biodiversity across taxa. Nature 466, 1098–1101. doi: 10.1038/nature09329
Trebilco, R., Halpern, B. S., Flemming, J. M., Field, C., Blanchard, W., and Worm, B. (2011). Mapping species richness and human impact drivers to inform global pelagic conservation prioritisation. Biol. Conserv. 144, 1758–1766. doi: 10.1016/j.biocon.2011.02.024
Tremblay-Boyer, L., Gascuel, D., Watson, R., Christensen, V., and Pauly, D. (2011). Modelling the effects of fishing on the biomass of the world’s oceans from 1950 to 2006. Mar. Ecol. Prog. Ser. 442, 169–185. doi: 10.3354/meps09375
van Andel, T. H., and Veevers, J. J. (1967). Morphology and Sediments of the Timor Sea. Melbourne: Bureau of Mineral Resources.
Vassallo, P., Paoli, C., Alessi, J., Mandich, A., Würtz, M., and Fiori, C. (2018). Seamounts as hot-spots of large pelagic aggregations. Mediterr. Mar. Sci. 19, 444–458. doi: 10.12681/mms.15546
Wakefield, C. B., Lewis, P. D., Coutts, T. B., Fairclough, D. V., and Langlois, T. J. (2013). Fish assemblages associated with natural and anthropogenically-modified habitats in a marine embayment: comparison of baited videos and opera-house traps. PLoS ONE 8:e59959. doi: 10.1371/journal.pone.0059959
Ward-Paige, C. A., Keith, D. M., Worm, B., and Lotze, H. K. (2012). Recovery potential and conservation options for elasmobranchs. J. Fish Biol. 80, 1844–1869. doi: 10.1111/j.1095-8649.2012.03246.x
Webb, T. J., vanden Berghe, E., and O’Dor, R. (2010). Biodiversity’s big wet secret: the global distribution of marine biological records reveals chronic under-exploration of the deep pelagic ocean. PLoS ONE 5:e10223. doi: 10.1371/journal.pone.0010223
Wenger, S. J., Som, N. A., Dauwalter, D. C., Isaak, D. J., Neville, H. M., Luce, C. H., et al. (2013). Probabilistic accounting of uncertainty in forecasts of species distributions under climate change. Glob. Chang. Biol. 19, 3343–3354. doi: 10.1111/gcb.12294
West, B. G. (1992). Petroleum Prospectivity of the East Malita Graben Area, Bonaparte Basin. Melbourne: Bureau of Mineral Resources.
Whiting, S., Long, J., and Coyne, M. (2007). Migration routes and foraging behaviour of Olive ridley turtles Lepidochelys olivacea in northern Australia. Endanger. Species Res. 3, 1–9. doi: 10.3354/esr003001
Whiting, S. D. (1999). Use of the remote Sahul Banks, northwestern Australia, by dugongs, including breeding females. Mar. Mammal Sci. 15, 609–615. doi: 10.1111/j.1748-7692.1999.tb00831.x
Whitmarsh, S. K., Fairweather, P. G., and Huveneers, C. (2017). What is Big BRUVver up to? Methods and uses of baited underwater video. Rev. Fish Biol. Fish. 27, 53–73. doi: 10.1007/s11160-016-9450-1
Whittaker, R. J., Araújo, M. B., Jepson, P., Ladle, R. J., Watson, J. E. M., and Willis, K. J. (2005). Conservation biogeography: assessment and prospect. Divers. Distrib. 11, 3–23. doi: 10.1111/j.1366-9516.2005.00143.x
Wilcox, C., Hardesty, B. D., Sharples, R., Griffin, D. A., Lawson, T. J., and Gunn, R. (2013). Ghostnet impacts on globally threatened turtles, a spatial risk analysis for northern Australia. Conserv. Lett. 6, 247–254. doi: 10.1111/conl.12001
Wood, S. N. (2004). Stable and efficient multiple smoothing parameter estimation for generalized additive models. J. Am. Stat. Assoc. 99, 673–686. doi: 10.1198/016214504000000980
Wood, S. N. (2006). Generalized Additive Models: An Introduction with R. London: Chapman & Hall/CRC.
Wood, S. N. (2011). Fast stable restricted maximum likelihood and marginal likelihood estimation of semiparametric generalized linear models. J. R. Stat. Soc. Ser. B Stat. Methodol. 73, 3–36. doi: 10.1111/j.1467-9868.2010.00749.x
Worm, B., Lotze, H. K., and Myers, R. A. (2003). Predator diversity hotspots in the blue ocean. Proc. Natl. Acad. Sci. U.S.A. 100, 9884–9888. doi: 10.1073/pnas.1333941100
Worm, B., Sandow, M., Oschlies, A., Lotze, H. K., and Myers, R. A. (2005). Global patterns of predator diversity in the open oceans. Science (80-) 309, 1365–1369. doi: 10.1126/science.1113399
Wraith, J., Lynch, T., Minchinton, T. E., Broad, A., and Davis, A. R. (2013). Bait type affects fish assemblages and feeding guilds observed at baited remote underwater video stations. Mar. Ecol. Prog. Ser. 477, 189–199. doi: 10.3354/meps10137
Yon, A., Meekan, M. G., Andrzejaczek, S., Martinez, S., and Speed, C. W. (2020). Shark and ray community structure in a turbid, nearshore coral reef habitat. Mar. Freshw. Res. doi: 10.1071/MF19301
Keywords: biodiversity, Northwest Australia, pelagic species, geomorphology, seabed topography, mid-water BRUVS, submerged banks and shoals
Citation: Bouchet PJ, Letessier TB, Caley MJ, Nichol SL, Hemmi JM and Meeuwig JJ (2020) Submerged Carbonate Banks Aggregate Pelagic Megafauna in Offshore Tropical Australia. Front. Mar. Sci. 7:530. doi: 10.3389/fmars.2020.00530
Received: 03 April 2020; Accepted: 10 June 2020;
Published: 30 June 2020.
Edited by:
David W. Sims, Marine Biological Association of the United Kingdom, United KingdomReviewed by:
Nigel Edward Hussey, University of Windsor, CanadaPhilip D. Doherty, University of Exeter, United Kingdom
Copyright © 2020 Bouchet, Letessier, Caley, Nichol, Hemmi and Meeuwig. This is an open-access article distributed under the terms of the Creative Commons Attribution License (CC BY). The use, distribution or reproduction in other forums is permitted, provided the original author(s) and the copyright owner(s) are credited and that the original publication in this journal is cited, in accordance with accepted academic practice. No use, distribution or reproduction is permitted which does not comply with these terms.
*Correspondence: Phil J. Bouchet, cGpib3VjaGV0QGdtYWlsLmNvbQ==