- 1Department of Bioscience, Aarhus University, Roskilde, Denmark
- 2Danish Shellfish Centre, National Institute of Aquatic Resources, Technical University of Denmark, Nykøbing Mors, Denmark
Mussel dredging causes resuspension of sediment particles that reduce water clarity and potentially leads to reduced eelgrass growth. In order to study the impact of resuspension from mussel dredging on light conditions in the water column, field experiments were conducted at two sites in the Limfjorden. Light loggers were placed in two circular arrays around the dredge area. Vertical profiles of current velocity were measured by an ADCP and the sediment particle size composition was obtained from sediment core samples. The field data was used to force, calibrate and validate a sediment transport model developed in the FlexSem model system. Changes in sediment concentrations during and after mussel dredging were modeled for the two sites and for seven scenarios. We found that the distance and direction of the plume in the model was in good agreement with light logger data. The plume duration was less than 1 h, and the impact range was between 260–540 m. The scenarios showed that fishing intensity and current speeds were most important for shaping the sediment plumes. Changes in suspended sediment concentrations were 0.62–1.79 mg l–1 on median average and 1.22–11.61 mg l–1 for the upper quantile of the plume, which were on the same order of magnitude as background values in the Limfjorden. The amount of fishing days during the eelgrass growth season was 6–8% in Lovns Bredning and 16–35% in Løgstør Bredning and less than 1–2% of the total area was dredged per season. Even though there are substantial changes in the light conditions from the sediment plumes, the overall spatio-temporal impact in the study area is considered low. We recommend that management plans in other areas could sustain a shellfish fishery by limiting fishing intensity and frequency near eelgrass beds. The presented approach combines observational data, sediment transport modeling and reported fishing activity. It is a step forward within sediment transport modeling and could be incorporated into environmental impact assessments. The results have recently been used as scientific background for recommendations to improve the management plans according to the Danish Mussel Policy and relevant EU Directives.
Introduction
Shellfish are important ecological engineers in coastal marine areas as they often live in aggregated structures with a diverse assemblage of associated flora and fauna. They filter the water, control phytoplankton biomass and they are food source for higher trophic levels such as crabs, starfish, birds and humans (Dame, 1996; Herman et al., 1999; Maar et al., 2009; Petersen et al., 2013). Shellfish are commercially exploited in many coastal areas using different types of gears (Dolmer and Frandsen, 2002; Kamermans and Smaal, 2002). However, the shellfish fishery can be in conflict with conservation interests by reducing the amount of food availability for shellfish eating birds, reducing the benthic filtration capacity, causing mechanical disturbance of the seabed (e.g., affecting seagrass beds, benthos communities, and sediment structure) and increasing sediment resuspension affecting the water clarity (Dolmer, 2002; Dolmer and Frandsen, 2002; Neckles et al., 2005; Frandsen et al., 2015). The EU Common Fishery Policy aims to ensure that fishing is environmentally, economically and socially sustainable. Hence, in order to manage shellfish fisheries, it is important to document the impact of fishing on the ecosystem (Kamermans and Smaal, 2002).
One of the concerns of mussel dredging is the resuspension of sediment particles leading to reduced light conditions for seagrasses (Riemann and Hoffmann, 1991; Dyekjær et al., 1995; Holmer et al., 2003). Eelgrass (Zostera marina) is the most common seagrass species in northern and western Europe and is a key indicator under the European Water Framework Directive for the biological quality element ‘Macroalgae and angiosperms’ (Carstensen et al., 2013). The primary cause of seagrass degradation and loss globally is a reduction in water clarity, both from increased turbidity and increased nutrient loading (Erftemeijer and Robin Lewis, 2006). In many cases, dredging operations have contributed to a higher turbidity and loss of seagrass vegetation (Erftemeijer and Robin Lewis, 2006). In the Danish estuary Limfjorden, water clarity and eelgrass depth distributions have not improved since the 1990s despite nutrient reductions of around 30%, probably due to increased sediment resuspension (Carstensen et al., 2013). Management measures have been implemented to protect the eelgrass beds by designating “eelgrass boxes” in Natura 2000 areas, which are avoiding direct fishing 300 m around the eelgrass beds. Eelgrass boxes encompass both, the known eelgrass habitats, and locations determined by modeling where the eelgrasses could potentially recolonize (Canal-Vergés et al., 2016). However, the indirect impact from dredging on seagrass ecosystems is far from fully understood, despite various research efforts (Erftemeijer and Robin Lewis, 2006). Hence, there is a critical need to improve the ability to make predictions of the sediment plume length, intensity, and persistence of environmental impacts associated with trawling and dredging, especially when conducted close to sensitive habitats such as eelgrass meadows (Erftemeijer and Robin Lewis, 2006; Linders et al., 2018).
The processes governing the dredge plume generation and transport are complex and depend on many factors, which are often site- and substrate-specific (Canal-Vergés et al., 2016). In the following study, we used the 3D FlexSem model system (Larsen et al., 2017, 2020) as a tool for predicting potential environmental impacts of mussel dredging activities supported by field data at two study sites in the Limfjorden. The aim of this study is to estimate the effects of mussel dredging causing resuspension and transport of sediment particles, and investigate how the sediment plume changes the light conditions in the water column in different scenarios. The topic of the study is a result of discussions in the Danish Mussel Committee that is an advisory body for management of the oyster and mussel fisheries in Danish waters. The model results will be evaluated and used to provide input to management plans addressing mussel fisheries and protection of eelgrass beds.
Materials and Methods
Study Area
The Limfjorden is a eutrophic shallow, brackish water area, with a mean depth of about 4.9 m and a surface area of 1,500 km2 (Figure 1). It is connected to the open waters of the North Sea to the west and the Kattegat to the east through narrow entrances and is characterized by small tidal amplitude (<0.5 m). The dominant saltwater inflow is with westerly winds from the North Sea and the average salinity ranges from 32 in the west to 22 in the inner parts of the Limfjorden (Maar et al., 2010). Løgstør Bredning is the central basin connecting the western and eastern parts, whereas Lovns Bredning is the innermost located basin only connected through a narrow strait to the rest of the Limfjorden (Figure 1). The mussel fishery is an important industry in both basins and is restricted to water depths >5 m in Løgstør Bredning and >2 m in Lovns Bredning as well as outside eelgrass boxes, where fishery is prohibited in order to protect known eelgrass beds. Currently, there are 21 licensed boats in the Limfjorden with an annual harvest between 15,000–22,000 ton the last 5 years (Danish Fishery Agency). Mussel dredging is carried out from boats using four mussel dredges with a width of 1.45 m, two on each side of the vessel and each with a weight of 123.4 kg (Frandsen et al., 2015).
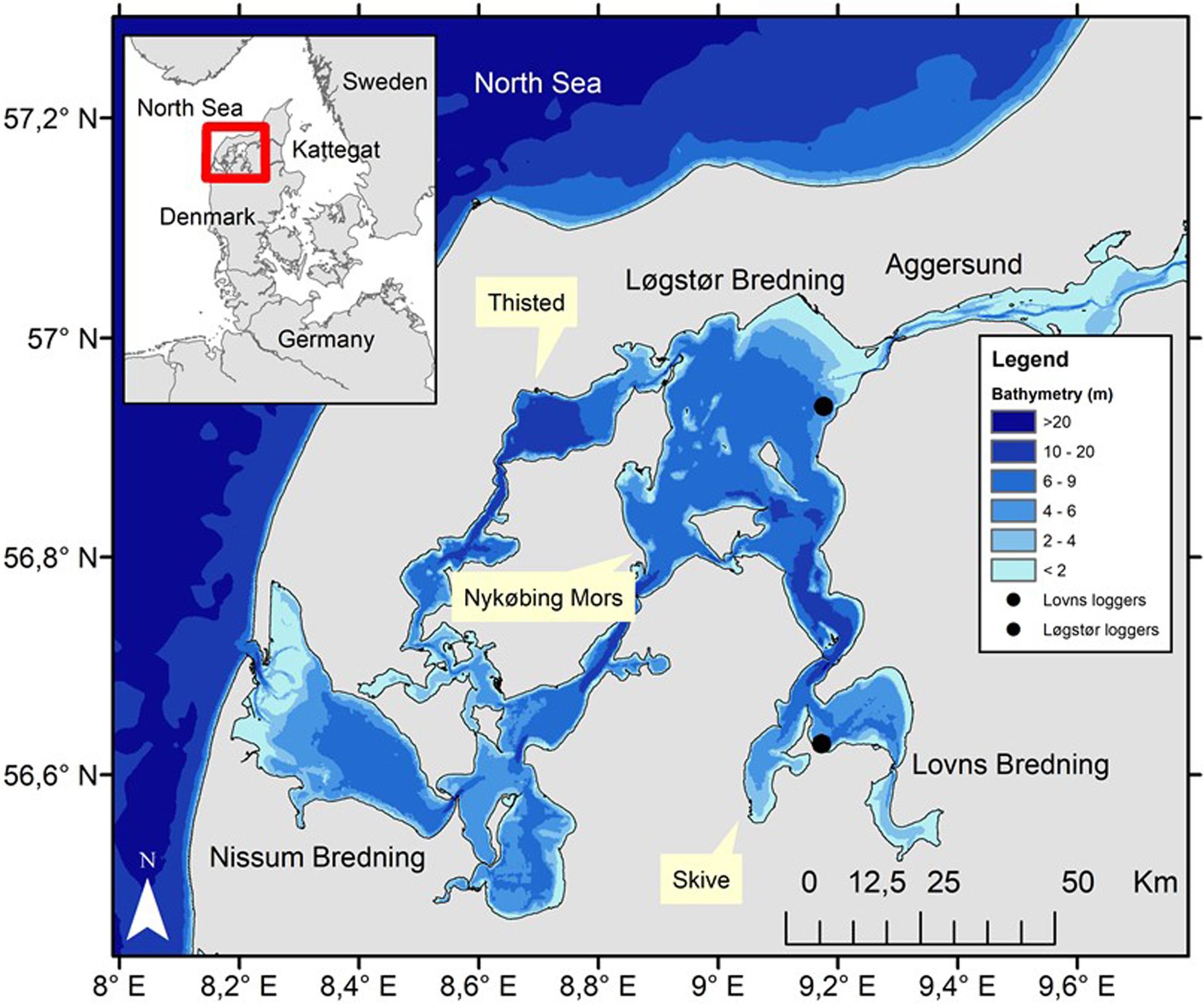
Figure 1. Map of the central and western Limfjorden showing the dredging locations (Lovns and Løgstør). Color legend indicates the water depth and yellow text boxes indicate the major cities.
Field Studies
Field studies were conducted in two different areas of the Limfjorden (Figure 1); in Lovns Bredning from 26th February to 4th March 2017 and in Løgstør Bredning from 6–10th March 2017. In each sampling area, 16 fixed-position moorings were deployed in two circular sensor arrays around the mussel dredging area (Figure 2). The inner array (stations 1–8) had a diameter of 200 m and the outer array (stations 9–16) was set up with a diameter of 600 m. Mussel dredging was performed by one fishing boat using four light dredges, two on each side of the boat (Frandsen et al., 2015). The start and end positions were mainly located inside or at the boundary of the inner mooring circle (Figure 2). The dredging process takes a couple of minutes, before the collected mussels are rinsed in the water column and taken on board. Part of the survey area in Lovns Bredning (including stations 14 and 15) was located inside a small eelgrass protection area (eelgrass box) (Figure 2A). The mussel dredging activities lasted for 1.5–2.5 h each day giving a total of 19 and 15 dredge tracks in Lovns Bredning and Løgstør Bredning, respectively, over the study period (Table 1). The highest amount of dredging events was 12 in Lovns Bredning and 9 in Løgstør Bredning occurring on the second day at both locations (Table 1). Each dredge covered a distance of approximately 115 m and 150 m and with a sailing speed of 3.2 knots and 2.6 knots in Lovns Bredning and Løgstør Bredning, respectively. The field data was used to force, calibrate and validate the sediment transport model.
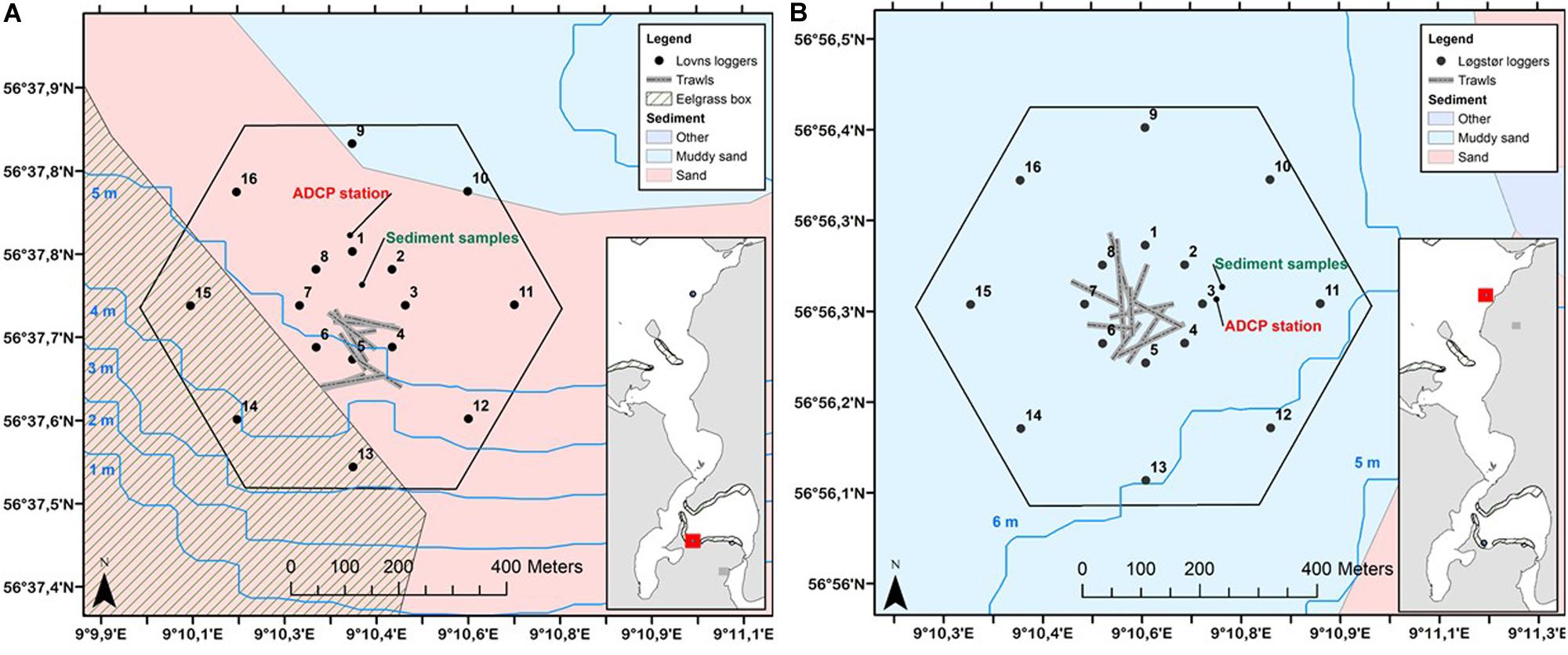
Figure 2. Mussel dredging tracks conducted in (A) Lovns Bredning and (B) Løgstør Bredning. Black circles indicate the position of loggers (numbered from 1–16), the ADCP and the sediment sampling. Trawl tracks are gray lines, sediment types are indicated as background color, water depth as blue contour lines, and the FlexSem model domain is shown as a black polygon. The hatched area indicates the location of the eelgrass box.
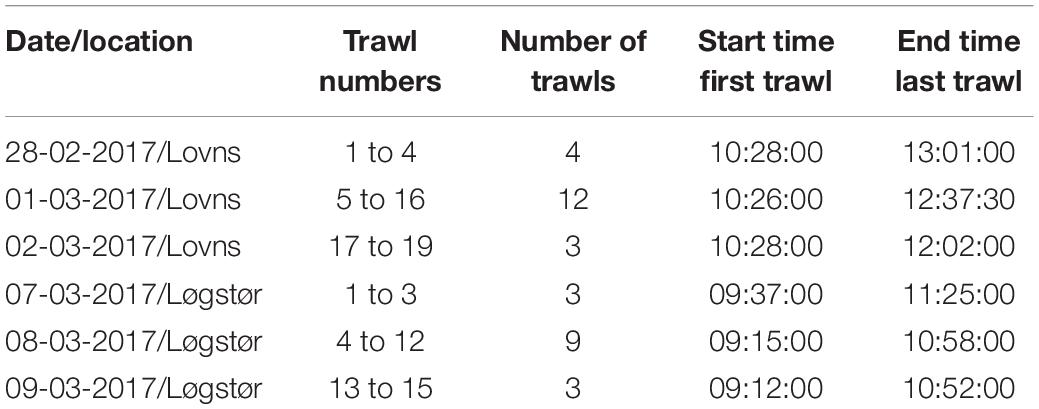
Table 1. Dredging date, trawl numbers, number of trawls per day, start time of the first trawl and end time of the last trawl in Lovns Bredning and Løgstør Bredning.
Light Measurements
Integrated temperature and light loggers (HOBO Pendant Temperature/Light 64K Data Logger) were mounted on each mooring in the two circular arrays at three different water depths (0.5, 1.5, and 3.0 m above seabed). The 48 loggers (3 depths and 16 stations) were deployed in each area over a period of 4 days and light intensity time series were collected with a sampling interval of 30 s. Five loggers in the Lovns area did not provide any data due to technical problems (two near-surface loggers and three loggers at 1 m above the seabed). However, only one bottom logger at Løgstør Bredning failed to provide data. Each time series was filtered using a 3 min moving average for removing the largest outliers, but retaining sharp changes in light intensity associated with short-lived sediment plumes caused by experimental mussel dredging. Light intensities were min-max normalized by scaling all values in the range 0–1 to compensate for large light intensity differences between moorings caused by temporal variability of solar irradiance, spatial heterogeneity in cloud cover and background light attenuation due to the presence of particles other than sedimentary material from mussel dredging. Light intensity anomalies were then calculated at each depth relative to an area-wide average of the min-max normalized data. We used a simple threshold to detect potential evidence for occurrences of dredging related turbid layers in the light logger data. Such signals were considered present, if the minimum light near-bottom intensity anomaly (low light events) at location x is smaller than the time-averaged light intensity anomaly at that location minus the highest standard deviation at any location xn and time step t:
where ΔI(x,t) is a time (t) series of light intensity anomalies at a specific location x, is the corresponding time-averaged intensity anomaly at location x and σΔI(xn,t)is the standard deviation of intensity anomalies from all mooring locations xn and time step t (n = number of locations 1–16).
ADCP Measurements
A 600 kHz ADCP (RDI Workhorse Sentinel) was deployed at each site (station 1 at Lovns Bredning mooring, station 3 at Løgstør Bredning mooring) in a bottom-mounted, upward-looking configuration. 30 seconds ensembles of 3D velocity components were collected along with corresponding records of beam correlation and error velocity. Each vertical profile had a bin size of 0.5 m and the first bin was at 1.59 m above the bottom (blanking distance: 0.88 m). The velocity data time series at each bin were filtered using a 30 min moving average. When compared to light intensity data, current measurements were less variable over time and a filter length of 30 min was chosen as a balance between retaining the dominant flow patterns and removing instrument noise. Only the first 6 bins contained error-free data (1.59–4.09 m above the bottom). Other depth bins were discarded due to the presence of strong artificial shear layers and noise mainly generated by acoustic signal reflection at the sea surface.
Sediment Samples
Sediment cores were taken by a diver in the middle of the sampling arrays in Lovns Bredning and at station 3 in Løgstør Bredning using Plexiglas cores. Three replicates were taken on each site and kept in the cold before being processed in the laboratory. The sediment cores had a surface area of 0.0021 m2 and the sediment column was sectioned into the depth layers: 0–1 and 1–2 cm. Samples from each stratum were homogenized and subsamples of 10 ml were taken for loss of weight on ignition (LOI) determination, while the rest of sediment was used for grain size analysis (surface area: 0.00115 m2). Each stratum was then sieved through test sieves of 2 mm, 1 mm, 500, 250, 125, and 63 μm. Each fraction was dried at 80°C until no difference in weight was measured (>48 h). The last fraction, <63 μm, was filtered onto 47 mm pre-dried Wattman GF/C microfiber filters (Sigma-Aldrich Denmark A/S, Copenhagen, Denmark), dried at 80°C for >48 h in order to determine dry weight. Organic matter content was determined for the 10 ml samples for each stratum as LOI at 550°C for 4 h.
Model Development
Model Approach
The FlexSem model system was used to describe the resuspension and transport of different size fractions of sediment particles with different sinking rates after a dredging event. FlexSem uses an unstructured computational mesh (Larsen et al., 2017, 2020). The computational mesh is made of 4,056 equilateral triangles with a characteristic length scale of 10 m covering a total area of 630 m × 630 m (Supplementary Figure A.1). The vertical discretization is implemented as z-coordinates, i.e., the separation between computational cells in the vertical are defined at fixed depths. For this study, 10 layers of 0.5 m thicknesses were used yielding a total water depth of 5 m. The FlexSem model system was forced by measured time-series of vertical profiles of horizontal current velocities (speed and direction) from the ADCP. It was assumed that the currents were spatially uniform within the model domain because the sea beds were smooth with slopes less than 5 per-mille. Thus, the model includes vertical velocity shear, i.e., vertical changes of the horizontal flow, but no explicit vertical velocities or diffusion. Vertical velocities from the ADCP were at least one order of magnitude smaller than the horizontal currents. A sensitivity test showed that the sediment plume only was slightly affected at vertical diffusivities >10–3 m2 s–1 (Supplementary Figure A.2), which are in the less frequent, high end of observed and simulated values (10–6–10–2 m2 s–1) from the area (Maar et al., 2007; Stevens and Petersen, 2011). The measured vertical profiles were linearly interpolated from the depth bins of the ADCP to the model depth layers.
Sediment Resuspension and Sinking
The area-specific amount of resuspended sediment (kg DW m–2) was estimated from the measured weight of particles in the sediment (kg DW m–3) in the 0–1 cm layer for Lovns Bredning and 0–2 cm for Løgstør Bredning (Table 2) multiplied by the penetration depth of the light dredge. Since there are no estimates of the depth penetration of the light dredge, we used the minimum penetration depth of 0.0015 m for the heavier Dutch dredge (Dyekjær et al., 1995). This resulted in a resuspension of 2.6 kg DW m–2 and 3.7 kg DW m–2 in Lovns Bredning and Løgstør Bredning, respectively. The differences in resuspension were due to a lower sediment density in Lovns (1,741 kg m–3) compared to Løgstør (2,480 kg m–3) (Table 2) resulting in a higher sediment release in Løgstør. The approach assumed a 100% efficient resuspension, e.g., that the dredge was in contact with the seafloor at all times. Our resuspension estimates were similar to reported values of 1.6–2.6 kg DW m–2 measured in the water column before and after dredging using the Dutch-dredge in the Limfjorden (Dyekjær et al., 1995).

Table 2. Sediment composition in Lovns Bredning and Løgstør Bredning showing particle size range (mm), sediment class, sediment concentrations (kg m–3) at 0–1 cm depth (Lovns) and 0–2 cm depth (Løgstør), and representative particle size applied in the estimated sinking rates.
The total amount of resuspended material (kg DW) was calculated multiplying the area-specific resuspension (kg DW m–2) by the length (m) of the dredge track (Figure 2) and the width of the four dredges (4 m × 1.45 m). The resuspended material was distributed on five particle size fractions (from <0.063 to 1 mm) based on field data (Table 2). The dominant particle size in Lovns Bredning was 0.125–0.50 mm (fine to medium sand). The dominant particle sizes in Løgstør Bredning were smaller, with 0.063–0.25 mm (very fine to fine sand). However, Lovns Bredning had a higher concentration of the smallest sediment fraction (<0.063 mm, silt) compared to Løgstør Bredning (Table 2).
Settling velocities (Vz, m s–1) for each sediment size fraction were calculated from the Stokes Law equation. This equation is based on the assumptions that the flow is highly viscous, the particles are impermeable and the shapes of particles are spherical (Sun et al., 2016):
where g is the gravitational acceleration (m s–2), ρzthe density of the settling particle (kg m–3),ρw the density of water (kg m–3), d the diameter of the particle (m), and μ the dynamic viscosity (kg m–1 s–1). We assumed that the particles had a density of 2,600 kg m–3, i.e., proxy for quartz (Linders et al., 2018). The calculated sinking velocities ranged from 0.002 to 0.53 m s–1 (Table 2). Preliminary model tests showed that the two larger size classes (0.5–1 mm) were settling within a few minutes, and the three smaller size classes (<0.063–0.25 mm) were the ones that contributed to generate the plume (Supplementary Figure A.3). The plume duration was estimated to be approximately 1 h for the smallest size fraction (<0.063 mm, silt).
Model Simulations and Scenarios
Simulations Using Survey Data
The resuspension of modeled sediment particles during the mussel dredging events were reproduced according to the survey data (Table 1) and followed the movement of the fishing ship for each day in Lovns and Løgstør Bredning (Figure 2). The model time-step was set to 1 s and the amount of sediment release corresponded to the light dredge (see section above). The resuspended sediment (Table 2) was released into the water column equally distributed over depth because (i) the final washing of the dredges affected the whole water column, (ii) the plume was visible from drone photos and (iii) the analysis of light attenuation anomalies demonstrated an effect to at least 3 m above bottom (Supplementary Figures B.1, B.3). The release followed the dredge track 3 to 12 times each day corresponding to the number of dredging activities (Table 1). E.g., if there were four dredging events occurring in 1 day lasting 1 min each, the particles were released in all the model cells along the dredge track within 1 min (typically 9–11 cells) for each dredging event that day. Hence, the longer the dredge track was, the more model cells would be involved in the release. After settling, there was no further resuspension. The location of the dredge tracks was based on ship movement obtained from start and end positions of the ship (Figure 2). This type of particle release following the ship movement during dredging is named ‘dynamic’ release opposed to a ‘static’ release, where particles are released from one grid cell only (see section below). Model results provided spatial data on particle concentrations of the different size fraction distributed equally in the water column over time. At the end of each simulation (1 day), the accumulated sediment concentration settled within each model element was calculated. The accumulated sediment concentration results are therefore not a representation of the suspended sediment particles at any point in time, but a time independent view of the sediment plume extent. In reality, the actual suspended sediment concentration at any point in time is likely to be lower due to the plume dispersal over time. Accordingly, plume length (m) was defined as the maximum horizontal transport of sediment estimated from the accumulated sediment concentration (Linders et al., 2018). A threshold of detectable impact on the sediment concentration was defined as >0.02 mg l–1 (>0.1 g m–2 in a 5 m deep water column) corresponding to 1% of the lower quartile of measured suspended background concentrations in the Limfjorden (Olesen, 1996). Plume intensity (mg l–1) was estimated as the median value and the 5, 25, 75, and 95% percentiles of suspended sediment concentration during 1 h after dredging.
Model Scenarios
Different model scenarios were tested in Lovns Bredning (Table 3) in order to analyze the sensitivity of the model to (i) type of particle release (static versus dynamic), (ii) amount of suspended sediment using different gear types, (iii) particle size composition, (iv) fishing intensity as number of trawls and (v) changes in current speed. Lovns Bredning was chosen due to the proximity of the eelgrass boxes to the dredging activities and the high concentration of silt. In the scenarios, particles were released from one element in the center of the mesh (i.e., static release) for each of the four dredging events on 28th February 2017 in Lovns Bredning. The mean velocity was 0.05 m s–1 during the release. The first scenario applied a minimum resuspension of 1 kg DW m–2 for the light dredge according to Frandsen et al. (2015). They estimated the resuspension from the difference in the weight of the gear before and after rinsing it immediately after the dredging event (Frandsen et al., 2015). This value was considered as a minimum estimate, because they only considered the sediment caught by the gear not including the sediment released during the dredging. The second scenario applied the release of 2.6 kg DW m–2 for Lovns Bredning and tested the effect of ‘static’ versus ‘dynamic’ release of sediment particles. In the third scenario, we used a maximum resuspension of 3.7 kg DW m–2 as estimated for Løgstør Bredning. This was considered as a worst-case scenario, due to the amount of resuspended material. In this case, it was higher than the maximum estimate by Dyekjær et al. (1995) using the heavier Dutch dredge, and it was based on a 100% efficient resuspension.
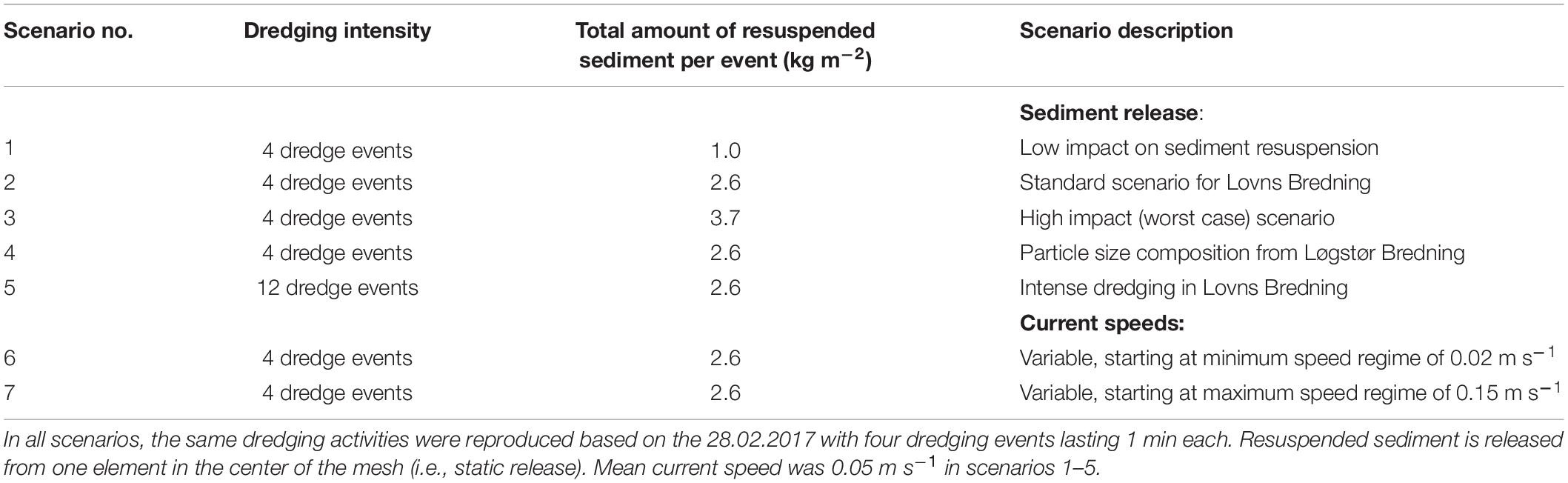
Table 3. Model scenarios description of intensity, amount of sediment release, particle size composition and current speed.
The following scenarios applied a release of 2.6 kg DW m–2 estimated for Lovns Bredning using the light dredge. Scenario 4 used the particle size composition from Løgstør Bredning (Table 2). In scenario 5, an intense dredging activity was tested with a total of 12 trawls in 1 day. In scenarios 6 and 7, the simulations started when the maximum and minimum current speeds, respectively, were observed in the data set. E.g., if the maximum current speed occurred on the 28th February, the model and the dredging activities were forced to start at that time.
Fishing Data
The data on the number of fishing days, boats and tracks, and size of the dredged area per month in 2017 and 2018 in the two studied areas was obtained from the Danish Fisheries Agency (unpublished data). The seasonal impact (%) was estimated as the number of fishing days in each area out of a total of 210 days during the eelgrass growth period from May to October (Eriander, 2017). The average track length varied between of 295 m in Lovns Bredning and 528 m in Løgstør Bredning.
Results
Field Measurements
Instantaneous currents from moored ADCP measurements are summarized in rose plots and individual time-series plots for each depth level (Figure 3). In general, currents were rather uniform over depth without significant vertical shear for most of the sampling period in each area. At the Lovns mooring, the strongest instantaneous currents were directed to the south and west during the first 2 days of sampling (Figure 3A). The highest instantaneous current speeds (0.15 m s–1) were recorded in the bottom-most layers in the early hours on the 2nd March 2017. At the end of the sampling period (after 2nd March at 12:00 pm) currents were considerably weaker (∼0.02 m s–1) and mainly directed to the south and southeast. At the Løgstør mooring, currents were mainly directed to the northeast with speeds up to 0.10 m s–1, interrupted by short periods of south-westward flow (Figure 3B). Instantaneous currents during these flow reversals showed an episodic amplification of both magnitude and vertical shear. Maximum speeds of up to 0.15 m s–1 were recorded in the near-surface layers during the second day (7th March 12:00 h–18:00 h).
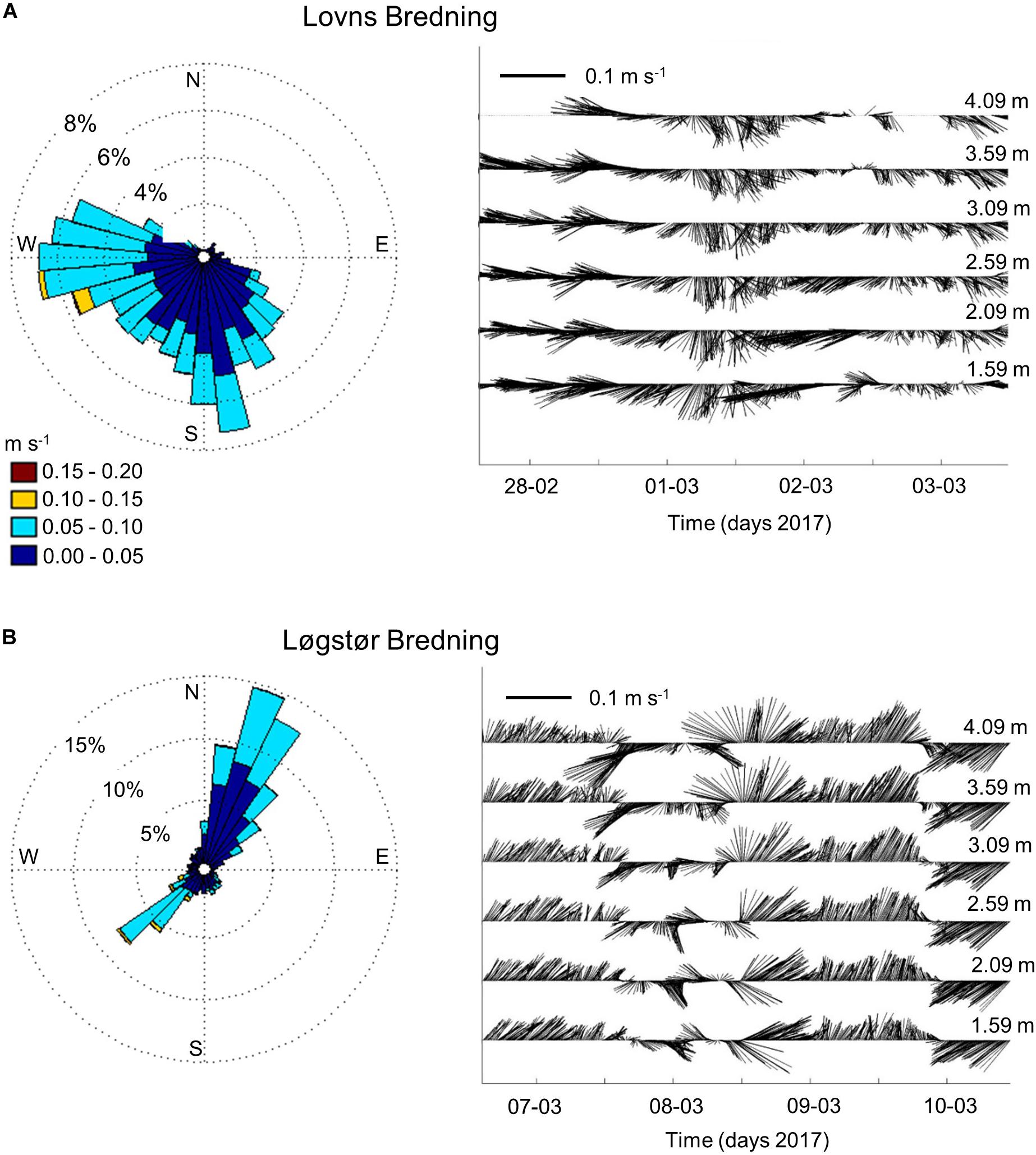
Figure 3. Rose plots of instantaneous currents at all depths (left column) and corresponding time series of instantaneous currents (every 20th data point is shown corresponding to 10 min intervals) at different depth layers (right column) measured by the ADCP at (A) Lovns Bredning and (B) Løgstør Bredning.
Light intensity anomalies at different sampling days in Lovns Bredning for the bottom-most layer (0.5 m above bottom) are presented in Figures 4A–C along with observed currents. In addition, maps including stations, where our threshold detection method predicted the presence of a dredging plume are shown in Figures 4D–F. Presence and orientation of the most characteristic negative light intensity anomalies followed the direction and magnitude of the observed near-bottom currents during all the sampling days (Figures 4D–F). On 1st March, however, some potential plume locations (stations 1, 2, 16) were predicted to be located upstream from the dredging site (Figure 4E). For most of the dredging periods, sediment plumes were predicted inside the inner sampling array (∼200 m). On 1st March, however, characteristic negative light anomalies were also identified at least 300 m to the south of the initial dredging area, during conditions of intense dredging and strong southerly flow (Figure 4E). Characteristic light intensity anomalies in the mid-layer (1.5 m above bottom) were also well aligned with subsurface currents (Supplementary Figure B.2). Results from light intensity measurements in Løgstør Bredning can also be found in the Supplementary Figures B.3–B.5.
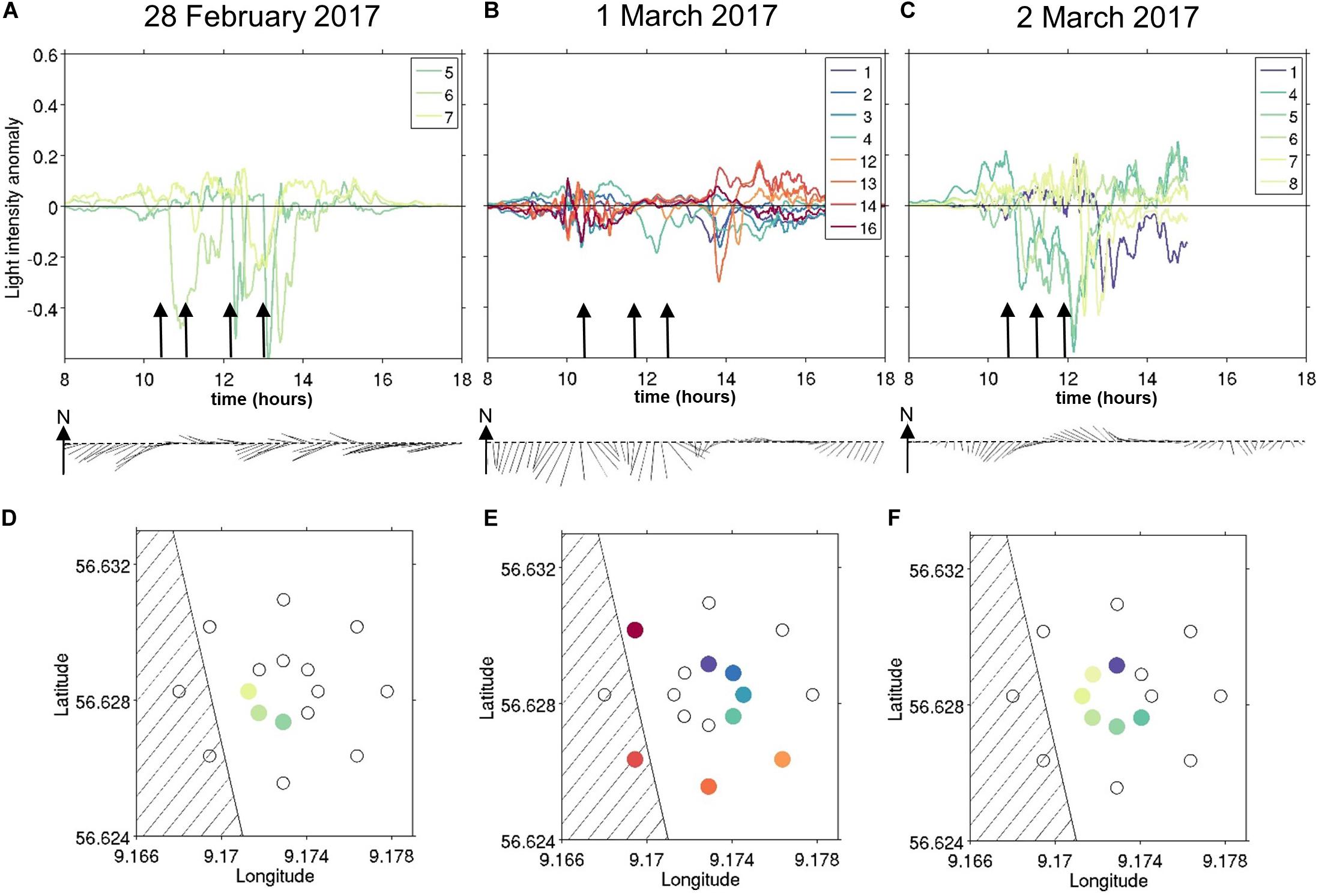
Figure 4. Bottom-most (0.5 m) light intensity anomalies from normalized light intensity logger data at all moorings in Lovns Bredning. Time-series during different sampling days are shown in subfigures (A–C) with dredging periods indicated by black arrows. Mooring stations are numbered according to Figure 2A and have different colors. The stick plots below (A–C) display the dominant flow direction in the bottom-most depth layer (see Figure 3 for more information). Stick plots represent both flow magnitude and direction and are equally scaled. In subfigures (D–F), the locations of moorings with detected light intensity anomalies are indicated using the same color code as in (A–C) for the respective days. The hatched area indicates the location of the eelgrass box.
Model Validation
Time-series of the observed near-bottom (0.5 m above the seabed) light intensity anomalies were compared with corresponding time series of modeled sediment concentrations (g m–3) at Lovns and Løgstør Bredning stations for the survey data simulations. We only considered sampling locations where presence of potential dredging plumes from light measurements was predicted by the detection threshold method in equation 1. Modeled occurrences of high sediment concentrations corresponded to negative light intensity anomalies, i.e., stronger light attenuation. Spatial sediment distributions from the model and plume presence estimated from observations showed an overall good agreement in both extent and direction for all dredging periods in Lovns Bredning (Figures 4D–F, 5A–C). The main exceptions were on the 1st March, where some observations deviated from the main current direction. At Løgstør Bredning (Figures 5D–F), we again found a good agreement with the model except for an outlier in observations at station 10 on the 8th March 2017, but this signal occurred some hours after the last dredging event (Supplementary Figures B.5B,E). There was also a good agreement in timing of the plume onset and duration between data and model results during stronger plume events (Figure 6), however, the agreement was poorer in cases of weaker plumes close to the detection threshold, as it can be seen at some locations in Lovns Bredning on the 1st March 2017 (Supplementary Figure B.6).
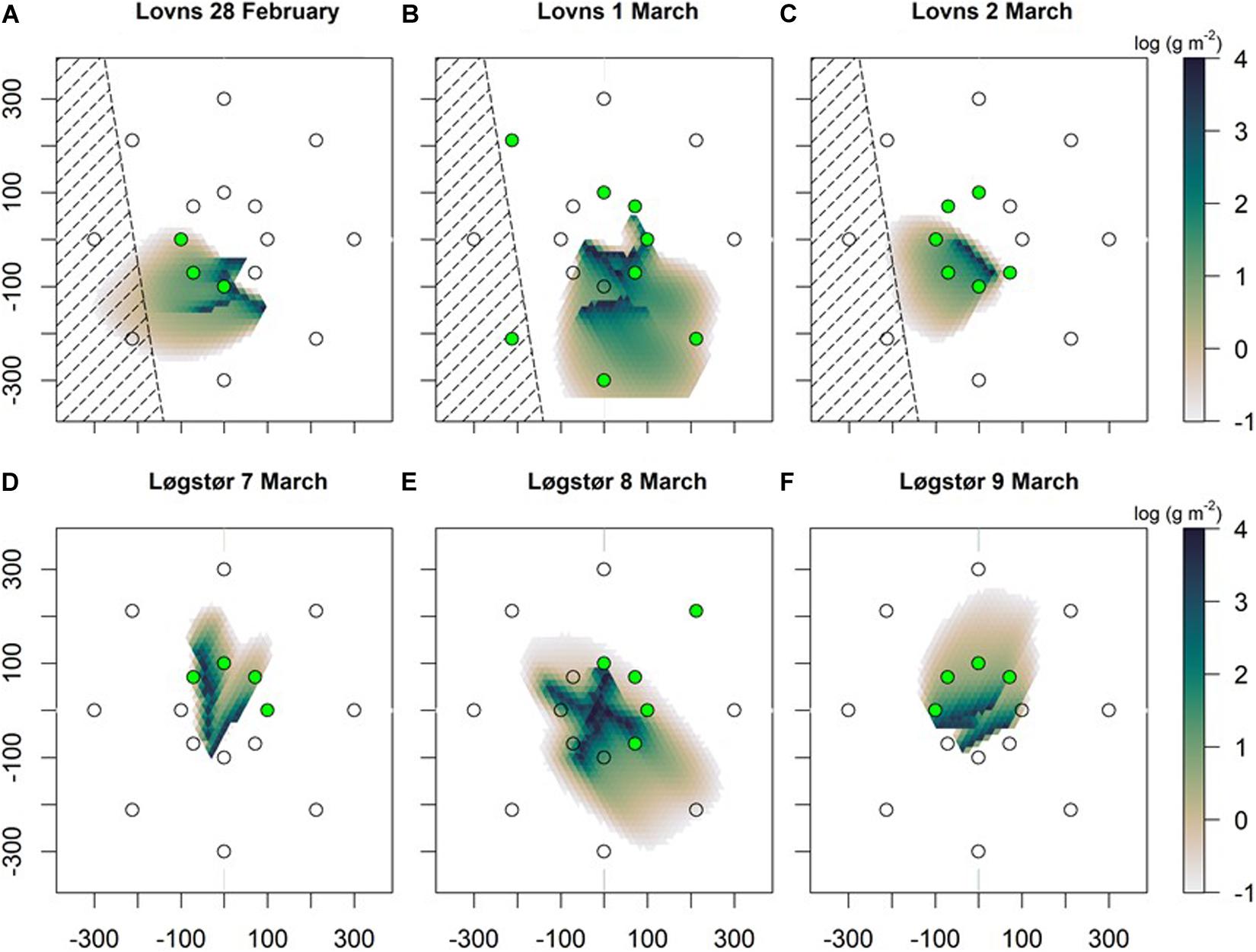
Figure 5. Accumulated sediment concentration (g m– 2 on log scale) at the end of the trawling activities in Lovns Bredning on three dates: (A) 28 February, (B) 01 March, and (C) 02 March and in Løgstør Bredning for three dates: (D) 07 March, (E) 08 March, and (F) 09 March. The hatched area indicates the location of the eelgrass box. Black circles indicate the position of loggers and filled green circles are the estimated light intensity anomalies from Figure 4.
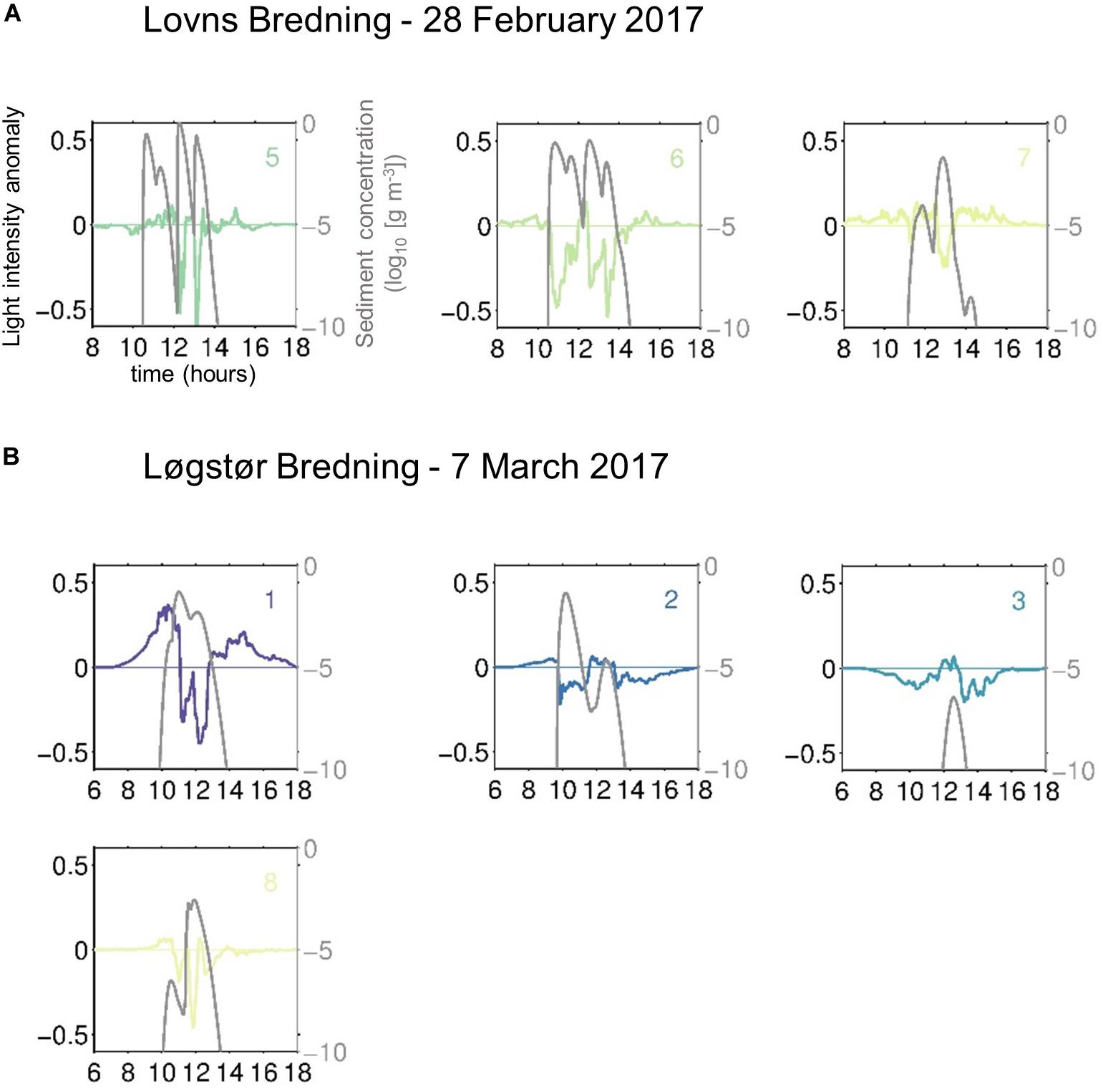
Figure 6. Observed time series of light intensity anomalies at plume presence locations (colored lines, predicted by the detection threshold analysis) versus the modeled sediment concentrations (g m– 3 on log scale, gray solid lines) at 0.5 m above seabed on the first sampling day at (A) Lovns Bredning and (B) Løgstør Bredning. Colored lines and numbers refer to predicted stations as shown in Figure 4.
Model Results of Sediment Plumes
Sediment Accumulation on the Seabed
The total amount of sediment accumulated on the seabed at the end of the dredging period was estimated for each sampling day in Lovns Bredning and Løgstør Bredning (Figure 5). The highest impact was found close to the dredge track due to the fast sedimentation of the larger particles and the signal decreased with distance from the track in the downstream current direction. On the second day, the highest impact was found during the highest dredging activity in both locations (Table 2). The smaller particles were transported further away by the prevailing currents and the impact range was 200–500 m in Lovns Bredning and Løgstør Bredning. The impacted area differed between days and locations due to differences in current patterns, dredging events and different sediment size fractions at the two locations.
In the model scenarios, the accumulated sediment on the seabed and the sediment plume length at the end of the dredging period was calculated for Lovns Bredning (Figure 7 and Table 4). The plume length was smaller and the sediment concentrations were lower in scenario 1 (330 m, Figure 7A) with minimum resuspension compared to the standard scenario 2 (360 m, Figure 7B). In scenario 3, the plume length was bigger and the sediment concentrations higher than in scenarios 1 and 2 due to the higher amount of resuspended sediment from using the Dutch dredge (>390 m, Figure 7C). In scenario 4, the lower proportion of the smallest sediment fraction (<63 μm) with lower sinking rates (Løgstør conditions) resulted in a smaller impact range (310 m) by sedimentation (Figure 7D). Scenario 5 with a higher fishing intensity showed a larger plume length (450 m) reaching further into the eelgrass box compared to the other scenarios (Figure 7E). Scenario 6 (220 m) showed that low current speeds caused a smaller plume (Figure 7F) compared to scenario 7 (>390 m) with high current speeds (Figure 7G). The plume intensity varied from 0.62 to 1.79 mg l–1 (median) between scenarios and the upper quantile showed values up to 11.61 mg l–1 in scenario 5 (Table 4). In summary, the main drivers for sediment plume impact were fishing intensity (scenario 5) and current velocity (scenarios 6–7), whereas the amount of resuspension from gear selection (scenarios 1–3) and particle size contribution (scenario 4) were less important for the study sites.
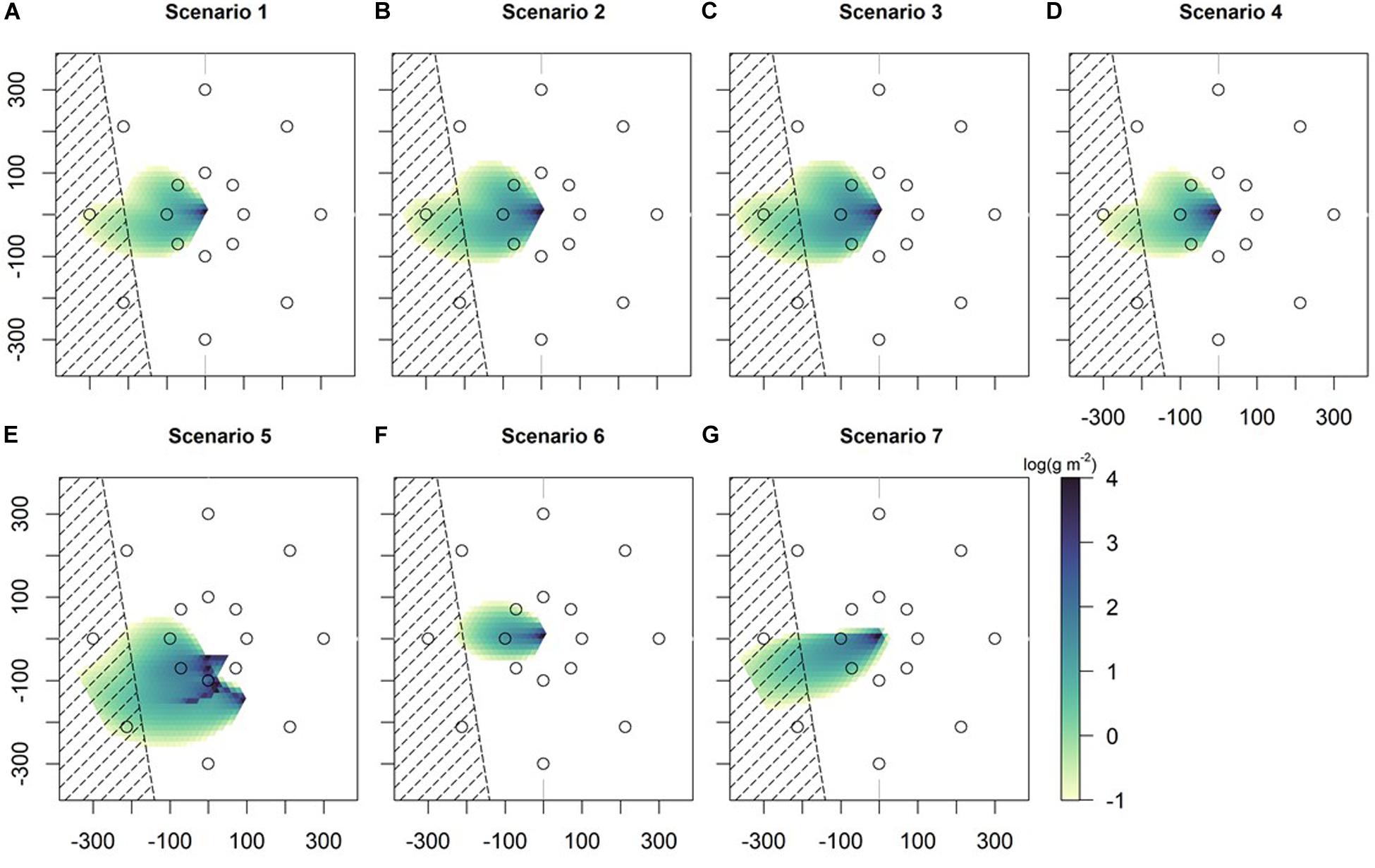
Figure 7. Accumulated sediment (g m– 2 on log scale) at the end of the trawling activities in Lovns Bredning for the different scenarios (A–G) (nos. 1–7 in Table 3). The hatched area indicates the location of the eelgrass box. Black circles indicate the position of loggers.
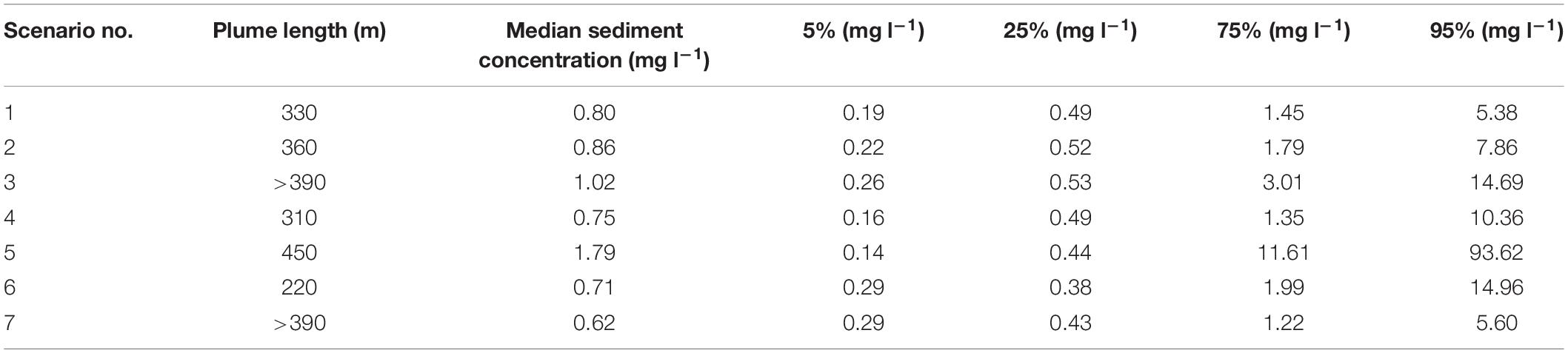
Table 4. Plume length (m) and intensity expressed as the median (50%) and the 5, 25, 75, and 95% percentiles of suspended sediment concentration in the water column 0–1 h after dredging.
Discussion
Estimated Effects of Mussel Dredging
Quantifying and modeling the transport and fate of sediments released during mussel dredging operations is essential for developing management plans in coastal shallow areas such as the Limfjorden (Sun et al., 2016). Here, we used a combination of field data and modeling to calculate the resultant sediment plumes and changes in light conditions caused by mussel dredging. During the surveys, the modeled plume size varied from 260 to 540 m depending on fishing intensity, current patterns and differences in sediment type between the two sites. The distance and direction of the plumes followed the current patterns, which showed a high day-to-day variability at the two study sites (Figures 3–5).
The generated sediment plume was most sensitive to increased fishing intensity (i.e., number of dredge tracks per day), which impacted a larger area and showed higher accumulation of sediment on the seabed (Figures 5B,E, 7E). Current patterns were similarly important, and the plume length increased from 220 m at low current speeds (0.02–0.04 m s–1), to >390 m at high current speeds (0.15 m s–1) (Table 4). A previous study on sediment plume modeling due to fish dredging estimated a horizontal transport distance of 280 m for resuspended coarse silt at current speeds of 0.04 m s–1 (Linders et al., 2018). To a lesser degree, the plume length was increased with higher amount of resuspended sediment from using different gear types (Figures 7A–C). The plume persistence was around 1 h mainly attributed to smallest particle size fractions (<0.063 mm silt) with lowest sinking velocities. The rest of the larger sand particles sank within the first 10 min after the resuspension event. A previous field study also found that non-cohesive sediment was able to settle within the first 30–60 min after eel trawling or mussel dredging (Riemann and Hoffmann, 1991). The scenario 4 using particle size composition from Løgstør Bredning confirmed a smaller plume length than for Lovns Bredning, due to the fewer silt particles (Figures 7B,D). Hence, the potential impact from mussel dredging on the eelgrass would be larger in areas dominated by silt and/or high current speeds compared to more sandy sites with faster settling and/or lower current speeds.
Light attenuation in the Limfjorden mainly depends on suspended inorganic matter and less on chlorophyll a concentrations and is closely related to wind-induced re-suspension (Dyekjær et al., 1995; Olesen, 1996). The model showed that the median concentration of the sediment plume from the dredging events (0.62–1.79 mg l–1) was slightly lower than measured background median values of 2.9 mg l–1 in the Limfjorden (Olesen, 1996). On the other hand, the 75%-percentiles values showed similar values (1.22–11.61 mg l–1) to the upper quantile of 4.7 mg l–1 of measurements (Olesen, 1996). Hence, the most intense part of the plume could have substantial effects on the light conditions as observed from the light anomaly analysis (Figure 4). Eelgrass has high light requirements and reduced light conditions that can make them more sensitive to other stressors (Kuusemäe et al., 2016). The indirect effect on eelgrass growth due to reduced light conditions from sediment plumes depends not only on the water clarity, but also on the prevailing light conditions at the bottom (i.e., if they live close to their depth limit), the season, duration, and frequency of the impact and will be discussed below.
Fishing Activities and Impact on Eelgrass Beds
Eelgrass beds are mainly located along the coastline in shallow waters, where they are protected from fishing by the eelgrass boxes, and the 2 and 5 m depth fishing limits in Lovns Bredning and Løgstør Bredning, respectively. The mussel fishery season starts in September and ends in June the following year, after which it closes for two consecutive months. The eelgrass growth season is from May to October (Eriander, 2017) with maximum biomass production during the summer months (Boström et al., 2014). Hence, there will be a short seasonal overlap from April to May to June and from September to October, but not during the summer period with maximum eelgrass growth. On average, one fishing boat would dredge an area from 2 to 50 times the same day with an average of 20 to 26 dredges within 2–7 h. The reported fishery data from 2017 to 2018 indicated an average of 57 to 100 dredging events per day. However, in two of the days, the dredging reached ∼200 events in Lovns, and in another day ∼450 events in Løgstør. These values depend on the number of boats present in the area, and on the capacity of the boats.
The recorded fishery activity in Lovns Bredning is variable from year to year. In 2017, there were 28 boat events (the same boat can fish several times) distributed on 13 days from April to October. However, in 2018, there were 64 boat-events for 17 fishing days (Danish Fisheries Agency). Hence, the daily fishing impact corresponded to 6–8% of the eelgrass growth season. Around 27 and 40% (in 2017 and 2018, respectively) of the fishing took place in less than 500 m away from the eelgrass box. This was due to the nearby high standing stock of mussel seeds in the area. The eelgrass box contains a buffer zone of 300 m around the eelgrass. Hence, if fishing is occurring close to the box, the plume may reach the eelgrass when there is high current speeds events or/and intense fishing (Table 4). Nevertheless, the impact will probably be minor at the given fishing frequency in Lovns Bredning. In Løgstør Bredning, the daily fishing activity was higher than for Lovns Bredning with 16–35% of the growth season in 2017 and 2018, respectively (126–322 boat-events, 33–75 days), whereas only <1% of the fishing occurred less than 500 m from the eelgrass box. On average, <2 and 1.2% of Lovns and Løgstør areas, respectively, are impacted by direct dredging effects from April to October in 2017. Hence, although there are more fishing days in Løgstør than in Lovns, the fishery in Løgstør is spread over a larger area further away from the coastline and the eelgrass habitats. Moreover, in all Natura 2000 areas, the eelgrass boxes take into consideration all the eelgrass beds established, and the potential habitat that could be colonized by eelgrass. Overall, for the two basins, the estimated indirect effects from fishing on light conditions and eelgrass growth must be considered to be of minor importance as long as the daily fishing intensity is kept at the same low level.
Only a few eelgrass boxes in the study areas are overlapping with mussel beds of interest to the fishermen, while for most of the boxes, fishery is occurring further than 500 m of their borders. Under new management scenarios, the 300 m buffer zone around the eelgrass beds within the eelgrass boxes could be reduced, but not less than 100 m to prevent potential direct effects, such as geogenic reefs in Natura 2000 areas (Pers. Comm. Danish Fishery Agency). This would require to run new model scenarios for the areas of interest and to consider changes in background resuspension events from dredging, in order to maintain a low fishing impact during the eelgrass growing season. The present study only considers the indirect effects on light conditions, whereas direct effects on the eelgrass through smothering from sediment suspension is not considered (Brodersen et al., 2017). Despite this assumption, only fine particles would be transported to the eelgrass beds, while larger particles (comprising most of the sediment) would sink in the vicinity of the trawl marks (Linders et al., 2018). Other direct effects such as damage on eelgrass shoots, leaves and seeds where not considered in this study. The management plans are already taking this into account in the eelgrass box areas, where the eelgrass can expand to new areas (BEK nr 1258 af 27/11/2019). Mussel (over)fishing can potentially reduce the filtration capacity by the mussel population and thereby decrease water clarity (Carstensen et al., 2013), which was not considered in the present study. Another model study found that the current fishery of 8–16% of the mussel stock in the Limfjorden increased chlorophyll a concentrations with 2–4%, which was close to the methodology detection level (Petersen et al., 2020a).
Model Validation
The largest differences between modeled sediment concentrations and observed light intensity data were found during periods of weak plumes close to the plume detection threshold applied to the light intensity data. These differences are likely due to shortcomings in individual skills of both the numerical model and the plume detection method. The numerical model only considers sediment introduced by dredging activities and does not take into account background sedimentation. Average sediment plume concentrations from dredging (Table 4) ranged within the same magnitude as wind generated resuspension (Dyekjær et al., 1995; Olesen, 1996). However, the numerical model was not considering multiple resuspension events generated by wind and waves, which can explain some of the deviations between model results and light data based plume predictions. The statistical presence/absence calculated from light intensity data might occasionally fail to differentiate correctly between light attenuation caused by dredging plumes and light attenuation caused by natural sediment resuspension events. Consequently, a pronounced mismatch between modeled sediment concentrations and observed light intensity anomalies can be expected at locations, where plume signals are weak or absent.
Model Limitations
Sediment transport modeling has many challenges due to the complex nature of local hydrodynamics, sediment transport processes and lack of data, which is a considerable obstacle for improving model predictions (Merritt et al., 2003). Sediment transport modeling does not often include ambient suspended sediments although it determines the overall impact of dredging. Natural turbidity is a common event in shallow environments, where resuspension and transport of suspended material are influenced by currents, wind, wave mixing and river outflows (Jones et al., 2016). Although there might be potential benefits from including ambient sediments in the models, the issue of ambient sediment modeling needs to be carefully assessed in the future since it requires more data on sediment dynamics and poses challenges with respect to local scale hydrodynamics. In the present study, we did not include ambient sediments in the model. Instead, we used light intensity anomalies from measurements to distinguish the dredging events from the natural turbidity, which was used to validate the modeled sediment plumes. Once the sediment is resuspended by a dredging event, it becomes more easily to resuspend with a second dredge happening nearby or by wind events (Paterson et al., 2000; Linders et al., 2018). This could cause local multiple resuspension events in the fished area that was not included in the modeling. However, on basin scale, the fished area was 7% of the Limfjorden during the period 2014–2018 (Petersen et al., 2020b). Hence, only a smaller part of the area will be affected by such multiple resuspension events after trawling and it will mainly be a problem in the areas close to the eelgrass beds.
Improving the model accuracy should be considered in the context of current science and financial costs. For example, the sinking velocity is essential for the dredge plume modeling, and measuring sinking velocity of particles in dredge plume can be done using existing technologies (Sun et al., 2016). On the other hand, cohesive sediment resuspension under surface wave forcing appears to be poorly understood and not well represented in sediment transport models; hence improving this aspect would require long-term research (Durrieu de Madron et al., 2005). In the present study, we used a conservative approach for estimation of particle sinking velocities without considering flocculation, which could be improved by more accurate measurements of the sampled particles. For future studies, it would be optimal to start recording field data on sedimentation before the dredging operations start (also known as continuous approach). This will allow us to understand the dynamics in the area in the prior phases, and be able to estimate how does other effects such as wind account for resuspension, since we potentially are under-estimating suspended sediment concentrations. In addition, parameterizations used in sediment transport models are often not reported in the Environmental Impact Assessment (EIA) documentation, leaving considerable uncertainty in assessing the model performance and inter-comparison with other models. The present model is documented and validated, and can be applied to other coastal and open sites with smooth bottom gradients, because water column structure and tides will be included in the ADCP data forcing the model. Areas with strong wave mixing will not be applicable, because this would also require a wave model. Model scenarios can be used to evaluate potential effects, and make recommendations to underpin management strategies in coastal areas affected by trawling activities.
Use of Modeling to Support Environmental Policies
Dredging for mussels and oysters has some immediate and easily measurable effects on the areas of the seabed that are directly affected by the dredge. These effects have received increased attention in recent years where in the coastal zone, fishery have to comply with environmental and nature conservation goals set be the EU Water Framework Directive and EU Species and Habitats Directive, and where focus of both direct and indirect environmental impacts of fisheries are less on the target species and more on the ecosystem. The main challenges for the fisheries management and the public are to document the effect of dredging and as a part of this make sure that the stock is not over-fished. In terms of environmental impact, the direct effect of the dredging on, e.g., eelgrass or benthic infauna can be assumed or has been demonstrated (see e.g., McLaverty et al., 2020). However, other more indirect effects, e.g., on the light in the form of resuspension of sediment caused by the fishing gear, have received less attention as they are more difficult to assess. In eutrophic areas, such additional impact on light attenuation may be critical to the key ecosystem components like eelgrass and macroalgae and thus further limit their expansion.
At present, the management objectives and principles for mussel and oyster fishery are stated in the Danish Mussel Policy1 (in Danish). This policy is balanced between utilization of the shellfish resource and protection of key ecosystem components like eelgrass, macroalgae, and benthic fauna from the direct physical damage caused by the dredging as well as potential indirect effects. The key ecosystem components are monitored regularly together with annual stock estimates of the mussel and oyster populations, and are used in the management plan. However, there was little knowledge on indirect effects of mussel dredging. As a consequence, the management had applied a precautionary approach by delimiting a buffer zone of 300 m around both the existing eelgrass beds, but also in areas where eelgrass beds can potentially expand. These areas are defined and annually revised following monitoring and modeling (Canal-Vergés et al., 2016; Nielsen et al., 2020). However, the management becomes too restrictive according to the precautionary principle and hence, in fact, does not live up to the intentions of the Common Fisheries Policy to increase productivity and profitability in the industry. Models such as the one used in this study are necessary to consider the indirect effects and thus to meet the intentions of the Common Fisheries Policy (CFP) to protect the marine environment, e.g., in relation to the implementation of the Water Framework Directive (2000/60/EC). Knowledge regarding the direct and indirect effects of fishing are therefore of great importance to the management of the fisheries and thus also to the industry. The present project has provided new knowledge about the indirect effects on the marine environment of mussel and oyster dredging activities, and the developed new model tools and methods that can establish new knowledge on fishery effects at the level of entire basins. The results have been used as scientific background for recommendations to improve management according to the Danish Mussel Policy and have been already implemented for the 2020–2021 fishery season. The buffer zone in the eelgrass boxes has now been reduced to 100 m. The model has therefore contributed to the CFP by adjusting the buffer to protect the eelgrass and by increasing productivity and profitability of the mussel fishery by allowing fishery to take place in new grounds closer to the eelgrass that were previously protected. Moreover, the results have also been used into the implementation of the Water Framework Directive by assessing the resuspension from fishery as a pressure factor. In order to continue to support decision-making processes in relation to dredging, general models that can be applied to various coastal and open sites need to evolve as effective and practical tools. Time-limited projects often restrict models to a defined set of scenarios making the tool unsustainable. The current model is available for use but still requires expert knowledge for setting up new scenarios. However, interest and need for new scenarios in specific areas where potential conflicts between fishery and marine environment arise can potentially be addressed and thus would make it a sustainable tool.
Conclusion
Sediment transport models are often not calibrated or validated due to the lack of relevant field data. In the present study, measured velocity profiles were used to force the hydrodynamic model, field data on sediment types were used to calibrate the model, whereas measured light intensity anomalies were used for successful model validation. In relation to management, the fixed buffer zones around mussel dredging that protect the eelgrass from indirect effects on light conditions, e.g., in the Limfjorden, could be more flexible depending on the dominant flow conditions and the fishing behavior, but should also consider the sediment type and risk of increased background resuspension due to dredging. Further, the present fishing intensity and frequency was found to have minimal effects on light conditions for eelgrass in the Limfjorden. Management plans for other areas with co-occurring dredging activities and seagrass beds should likewise limit the daily number of allowed dredging activities, and spread them over weeks and months to obtain a more sporadic effect on light conditions. The presented approach combining observational data with the presented modeling tool is a step forward within sediment transport modeling. The results can support more evidence-based management decisions in relation to the Danish Mussel Policy and governing EU Directives and have recently been applied in the new fishing plans for the season 2020–2021 in the Limfjorden.
Data Availability Statement
The data from field measurements can be downloaded from PANGAEA https://www.pangaea.de/. The FlexSem model code can be downloaded from https://marweb.bios.au.dk/flexsem or https://doi.org/10.5281/ZENODO.4056787, and the set-up files can be provided on request to the corresponding author.
Author Contributions
AP wrote the original draft, conducted the modeling, and analyzed the results. JL made the model configuration in the FlexSem model and contributed to the data analysis and writing. CS and CM designed and conducted the field work, analyzed the results, and contributed to the writing. JP conceptualized the study, reviewed the manuscript, and provided the funding acquisition. MM designed the model study, contributed to data analysis, finalized the writing, and submitted the revised manuscript. All authors contributed to the article and approved the submitted version.
Funding
This study was supported by the European Marine Fishery Fund and the Danish Fisheries Agency (grant no. 33113-I-16-011) and the Danish Centre for Environment and Energy at Aarhus University.
Conflict of Interest
The authors declare that the research was conducted in the absence of any commercial or financial relationships that could be construed as a potential conflict of interest.
Acknowledgments
We thank Kasper Andersen, Pascal Barreau, Finn Bak, Niels Peter Nielsen, and Lars Andersen at the Danish Shellfish Centre for supporting the laboratory and field work.
Supplementary Material
The Supplementary Material for this article can be found online at: https://www.frontiersin.org/articles/10.3389/fmars.2020.576530/full#supplementary-material
Footnotes
References
Boström, C., Baden, S., Bockelmann, A.-C., Dromph, K., Fredriksen, S., Gustafsson, C., et al. (2014). Distribution, structure and function of Nordic eelgrass (Zostera marina) ecosystems: implications for coastal management and conservation. Aquat. Conserv. Mar. Freshwat. Ecosyst. 24, 410–434. doi: 10.1002/aqc.2424
Brodersen, K. E., Hammer, K. J., Schrameyer, V., Floytrup, A., Rasheed, M. A., Ralph, P. J., et al. (2017). sediment resuspension and deposition on seagrass leaves impedes internal plant aeration and promotes phytotoxic H2S intrusion. Front. Plant Sci. 8:657. doi: 10.3389/fpls.2017.00657
Canal-Vergés, P., Petersen, J. K., Rasmussen, E. K., Erichsen, A., and Flindt, M. R. (2016). Validating GIS tool to assess eelgrass potential recovery in the Limfjorden (Denmark). Ecol. Model 338, 135–148. doi: 10.1016/j.ecolmodel.2016.04.023
Carstensen, J., Krause-Jensen, D., Markager, S., Timmermann, K., and Windolf, J. (2013). Water clarity and eelgrass responses to nitrogen reductions in the eutrophic Skive Fjord, Denmark. Hydrobiologia 704, 293–309. doi: 10.1007/s10750-012-1266-y
Dame, R. F. (1996). Ecology of Marine Bivalves. An Ecosystem Approach. Boca Raton: CRC Press. doi: 10.1201/9781420049787
Dolmer, P. (2002). Mussel dredging: impact on epifauna in Limfjorden, Denmark. J. Shellfish Res. 21, 529–538.
Dolmer, P., and Frandsen, R. P. (2002). Evaluation of the Danish mussel fishery: suggestions for an ecosystem management approach. Helgol. Mar. Res. 56, 13–20. doi: 10.1007/s10152-001-0095-6
Durrieu de Madron, X., Ferré, B., Le Corre, G., Grenz, C., Conan, P., Pujo-Pay, M., et al. (2005). Trawling-induced resuspension and dispersal of muddy sediments and dissolved elements in the Gulf of Lion (NW Mediterranean). Cont. Shelf Res. 25, 2387–2409. doi: 10.1016/j.csr.2005.08.002
Dyekjær, S. M., Jensen, J. K., and Hoffmann, E. (1995). Mussel dredging and effects on the marine environment. ICES CM. 13, 1–19.
Erftemeijer, P. L. A., and Robin Lewis, R. R. (2006). Environmental impacts of dredging on seagrasses: a review. Mar. Pollut. Bull. 52, 1553–1572. doi: 10.1016/j.marpolbul.2006.09.006
Eriander, L. (2017). Light requirements for successful restoration of eelgrass (Zostera marina L.) in a high latitude environment – Acclimatization, growth and carbohydrate storage. J. Exp. Mar. Biol. Ecol. 496, 37–48. doi: 10.1016/j.jembe.2017.07.010
Frandsen, R. P., Eigaard, O. R., Poulsen, L. K., Tørring, D., Stage, B., Lisbjerg, D., et al. (2015). Reducing the impact of blue mussel (Mytilus edulis) dredging on the ecosystem in shallow water soft bottom areas. Aquat. Conserv. Mar. Freshwat. Ecosyst. 25, 162–173. doi: 10.1002/aqc.2455
Herman, P. J., Middelburg, J. J., Van de Koppel, J., and Heip, C. R. (1999). Ecology of estuarine macrobenthos. Adv. Ecol. Res. 29, 195–240. doi: 10.1016/S0065-2504(08)60194-4
Holmer, M., Ahrensberg, N., and Jørgensen, N. P. (2003). Impacts of mussel dredging on sediment phosphorus dynamics in a eutrophic Danish fjord. Chem. Ecol. 19, 343–361. doi: 10.1080/02757540310001596708
Jones, R., Bessell-Browne, P., Fisher, R., Klonowski, W., and Slivkoff, M. (2016). Assessing the impacts of sediments from dredging on corals. Mar. Pollut. Bull. 102, 9–29. doi: 10.1016/j.marpolbul.2015.10.049
Kamermans, P., and Smaal, A. C. (2002). Mussel culture and cockle fisheries in the netherlands: finfing a balance between economy and ecology. J. Shellfish Res. 21, 509–517.
Kuusemäe, K., Rasmussen, E. K., Canal-Vergés, P., and Flindt, M. R. (2016). Modelling stressors on the eelgrass recovery process in two Danish estuaries. Ecol. Model 333, 11–42. doi: 10.1016/j.ecolmodel.2016.04.008
Larsen, J., Maar, M., Brüning, T., She, J., and Mohn, C. (2017). Flexsem: A New Method for Offline Ocean Modelling. Technical report from DCE-Danish Centre for Environment and Energy, no 105, Roskilde: Aarhus University.
Larsen, J., Maar, M., Mohn, C., and Pastor, A. (2020). A versatile marine modelling tool applied to arctic, temperate and tropical waters. PLoS One 15:e0231193. doi: 10.1371/journal.pone.0231193
Linders, T., Nilsson, P., Wikström, A., and Sköld, M. (2018). Distribution and fate of trawling-induced suspension of sediments in a marine protected area. ICES J. Mar. Sci. 75, 785–795. doi: 10.1093/icesjms/fsx196
Maar, M., Bolding, K., Petersen, J. K., Hansen, J. L. S., and Timmermann, K. (2009). Local effects of blue mussels around turbine foundations in an ecosystem model of Nysted off-shore wind farm, Denmark. J. Sea Res. 62, 159–174. doi: 10.1016/j.seares.2009.01.008
Maar, M., Nielsen, T. G., Bolding, K., Burchard, H., and Visser, A. W. (2007). Grazing effects of blue mussel Mytilus edulis on the pelagic food web under different turbulence conditions. Mar. Ecol. Prog. Ser. 339, 199–213. doi: 10.3354/meps339199
Maar, M., Timmermann, K., Petersen, J. K., Gustafsson, K. E., and Storm, L. M. (2010). A model study of the regulation of blue mussels by nutrient loadings and water column stability in a shallow estuary, the Limfjorden. J. Sea Res. 64, 322–333. doi: 10.1016/j.seares.2010.04.007
McLaverty, C., Eigaard, O. R., Dinesen, G. E., Gislason, H., Kokkalis, A., Erichsen, A. C., et al. (2020). High-resolution fisheries data reveal effects of bivalve dredging on benthic communities in stressed coastal systems. Mar. Ecol. Prog. Ser. 642, 21–38. doi: 10.3354/meps13330
Merritt, W. S., Letcher, R. A., and Jakeman, A. J. (2003). A review of erosion and sediment transport models. Environ. Model Softw. 18, 761–799. doi: 10.1016/S1364-8152(03)00078-1
Neckles, H. A., Frederick, T. S., Seth, B., and Blaine, S. K. (2005). Disturbance of eelgrass Zostera marina by commercial mussel Mytilus edulis harvesting in Maine: dragging impacts and habitat recovery. Mar. Ecol. Prog. Ser. 285, 57–73. doi: 10.3354/meps285057
Nielsen, P., Nielsen, M. M., McLaverty, C., Kristensen, K., Geitner, K., Olsen, J., et al. (2020). Management of Bivalve Fisheries in Marine Protected Areas. 2nd revision.
Olesen, B. (1996). Regulation of light attenuation and eelgrass Zostera marina depth distribution in a Danish embayment. Mar. Ecol. Prog. Ser. 134, 187–194. doi: 10.3354/meps134187
Paterson, D. M., Tolhurst, T. J., Kelly, J. A., Honeywill, C., de Deckere, E. M. G. T., Huet, V., et al. (2000). Variations in sediment properties, Skeffling mudflat, Humber Estuary, UK. Cont. Shelf. Res. 20, 1373–1396. doi: 10.1016/S0278-4343(00)00028-5
Petersen, J. K., Brooks, M. E., Dinesen, G. E., Eigaard, O. R., Maar, M., Olsen, J., et al. (2020a). “Andre presfaktorer end næringsstoffer og klimaforandringer – effekter af fiskeri på de marine kvalitetselementer bundfauna og fytoplankton,” in DTU Aqua-Rapport nr. 358-2020, ed. J. K. Petersen (Danmark: Danmark Tekniske Universitet), 1–42.
Petersen, J. K., Brooks, M. E., Edelvang, K., Eigaard, O. R., Göke, C., Hansen, F. T., et al. (2020b). “Andre presfaktorer end næringsstoffer og klima-forandringer – effekter af stedspecifikke presfaktorer på det marine kvalitetselement ålegræs,” in DTU Aqua-Rapport nr. 361-2020, ed. J. K. Petersen (Denmark: Danmarks Tekniske Universitet).
Petersen, J. K., Maar, M., Ysebaert, T., and Herman, P. M. J. (2013). Near-bed gradients in particles and nutrients above a mussel bed in the Limfjorden: influence of physical mixing and mussel filtration. Mar. Ecol. Prog. Ser. 490, 137–146. doi: 10.3354/meps10444
Riemann, B., and Hoffmann, E. (1991). Ecological consequences of dredging and bottom trawling in the Limfjord, Denmark. Mar. Ecol. Prog. Ser. 69, 171–178. doi: 10.3354/meps069171
Stevens, C. L., and Petersen, J. K. (2011). Turbulent, stratified flow through a suspended shellfish canopy: implications for mussel farm design. Aquac. Environ. Interact. 2, 87–104. doi: 10.3354/aei00033
Keywords: sediment transport model, Limfjorden, mussel fishery, sediment plumes, light
Citation: Pastor A, Larsen J, Mohn C, Saurel C, Petersen JK and Maar M (2020) Sediment Transport Model Quantifies Plume Length and Light Conditions From Mussel Dredging. Front. Mar. Sci. 7:576530. doi: 10.3389/fmars.2020.576530
Received: 26 June 2020; Accepted: 02 October 2020;
Published: 23 October 2020.
Edited by:
Chiara Piroddi, Joint Research Centre, ItalyReviewed by:
Carsten Lemmen, Helmholtz Centre for Materials and Coastal Research (HZG), GermanyHagen Radtke, Leibniz Institute for Baltic Sea Research (LG), Germany
Copyright © 2020 Pastor, Larsen, Mohn, Saurel, Petersen and Maar. This is an open-access article distributed under the terms of the Creative Commons Attribution License (CC BY). The use, distribution or reproduction in other forums is permitted, provided the original author(s) and the copyright owner(s) are credited and that the original publication in this journal is cited, in accordance with accepted academic practice. No use, distribution or reproduction is permitted which does not comply with these terms.
*Correspondence: Marie Maar, bWFtQGJpb3MuYXUuZGs=