- 1Department of Arctic and Marine Biology, Faculty of Biosciences, Fisheries and Economics, UiT The Arctic University of Norway, Tromsø, Norway
- 2Akvaplan-niva, Fram Centre for Climate and the Environment, Tromsø, Norway
- 3Department of Arctic Biology, The University Centre in Svalbard, Longyearbyen, Norway
- 4Department of Functional and Evolutionary Ecology, University of Vienna, Vienna, Austria
- 5College of Fisheries and Ocean Sciences, University of Alaska Fairbanks, Fairbanks, AK, United States
Assessment of Arctic deep-sea ecosystem functioning is currently an urgent task considering that ongoing sea-ice reduction opens opportunities for resource exploitation of yet understudied deep-sea regions. We used Biological Trait Analysis to evaluate ecosystem functioning and test if common paradigms for deep-sea fauna apply to benthic epifauna of the deep-sea Arctic Chukchi Borderland (CBL). We also investigated the influence of environmental factors on the functional structure of the epifauna. The analysis was performed for 106 taxa collected with a beam trawl and a Remotely Operated Vehicle from 486 to 2610 m depth. The most common trait modalities were small-medium size, mobile, benthic direct and lecithotrophic larval development, and predatory feeding, which mostly supports the current view of epifauna in the global deep sea. Functional composition of epifauna differed between two depth strata (486–1059 m and 1882–2610 m), with depth and sediment carbon content explaining most of the functional variability. Proportional abundances of the modalities free-living, swimming, suspension feeders, opportunists/scavengers, internal fertilization and globulose were higher at deep stations. Functional redundancy (FR) was also higher there compared to the mid-depth stations, suggesting adaptation of fauna to the more homogeneous deep environment by fewer and shared traits. Mid-depth stations represented higher functional variability in terms of both trait modality composition and functional diversity, indicating more variable resource use in the more heterogeneous habitat. Food input correlated positively with the proportional abundance of the modalities tube-dwelling, sessile and deposit feeding. Areas with drop stones were associated with higher proportional abundance of the modalities attached, upright, and predators. Comparatively low FR may render the heterogeneous mid-depth area of the CBL vulnerable to disturbance through the risk of loss of functions. Across the study area, high occurrence of taxa with low dispersal ability among adult and larval life stages may prevent rapid adaptation to changes, reduce ability to recolonize and escape perturbation.
Introduction
The deep ocean floor covers around 65% of the surface of the earth, yet it is the least explored ocean habitat. While we have begun to identify trends in deep-sea biodiversity patterns such as mid-depth peaks and latitudinal declines in diversity, and a generally higher level of rarity and endemism compared to shelf communities (Levin et al., 2001; Stuart et al., 2003; Renaud et al., 2006; Rex and Etter, 2010; Bluhm et al., 2011), still little is known about functional characteristics of deep-sea ecosystems. This is a serious gap, because deep-sea regions experience increasing anthropogenic influences (e.g., deep-sea fisheries, oil and gas exploration, mining, marine debris), and are at the same time subjected to the influences of climate change, in particular in polar regions (Smith et al., 2008; Ramirez-Llodra et al., 2011; Levin and Le Bris, 2015; Tekman et al., 2017). These impacts may bring changes in environmental properties, biodiversity and functioning of deep-sea ecosystems (Danovaro et al., 2008; Levin and Le Bris, 2015; Sweetman et al., 2017). Understanding of the current state of deep-sea ecosystem functioning and assessing its potential vulnerability to human impact and climatic changes is, thus, essential.
In contrast to shallower marine ecosystems, the deep sea is generally thought to be a more stable environment with constantly low bottom water temperature (typically, 0.01–4°C, but down to sub-zero values in the Arctic), high pressure, and low current velocity and resuspension (e.g., Gage and Tyler, 1991; Thistle, 2003; Tyler, 2003; Sweetman et al., 2017). More than 75% of the sea floor is covered by visually homogeneous abyssal plains, interrupted by geological structures such as ridges, canyons, hydrothermal vents, and cold seeps that add substantial heterogeneity to the habitat, biota and processes (Ramirez-Llodra et al., 2010; Levin and Le Bris, 2015). Deep-sea benthic communities are driven to a large extent by the amount of energy provided to the system from surface production (Iken et al., 2001, 2005). Only ∼0.5–2% of the surface production typically reaches the deep-sea floor and by that time is dominated by heavily reworked detrital organic material (Fischer et al., 2000; Ramirez-Llodra et al., 2010). Food limitation is particularly extreme in the central Arctic basins where the high latitude and seasonal or permanent sea ice control light penetration into the upper water column, and stratification limits the availability of nutrients during the short productive seasons (Leu et al., 2015; Randelhoff and Guthrie, 2016). Consequently, primary production in the oligotrophic Arctic basins is low (typically 1–15 g C m–2 year–1) with low levels of vertical flux of generally ≤1 g C m–2 year–1 below 120 m depth, and often less than half of that reaching the deep-sea floor (Wiedmann et al., 2020). These levels are considerably lower than other deep-sea areas, where pelagic primary production is highly variable, but often exceeds 20–50 g C m–2 year–1 (Karl et al., 1996; Levin and Gooday, 2003; Emerson, 2014).
Environmental conditions shape the biological characteristics of deep-sea benthic communities. Early studies suggested that benthic “associations governed by constantly limited food availability are composed of small individuals on the average” (size-structure hypothesis, Thiel, 1975). Indeed, recent studies confirmed that organisms of comparatively smaller size dominate at greater depth (Rex et al., 2006; Wei et al., 2010), though organisms of all sizes can inhabit the benthic environment in the deep sea (e.g., Billett et al., 2001; Ruhl and Smith, 2004; Bluhm et al., 2011; Rybakova et al., 2019). Low food availability and quality on the deep-sea seafloor, and low ambient current velocity are typically reflected in high proportions of deposit-feeding fauna (Iken et al., 2001, 2005; Bergmann et al., 2009). While suspension feeders, predators and scavengers are also represented in the deep sea (e.g., Premke et al., 2006; Cartes et al., 2008; Bergmann et al., 2009; Zhulay et al., 2019), suspension feeders tend to be less frequent due to the generally low currents and thus low amount of suspended material (Thistle, 2003), and low frequency of predators likely is due to low densities of prey (e.g., Thistle, 2003; Bluhm et al., 2011). In addition, scarce food may result in a dominance of mobile taxa that are more efficient in finding food (Iken et al., 2001; Thistle, 2003; Boetius et al., 2013) than sessile taxa that can only be supported in regions with enough particle flux and stronger currents (Degen, 2015). Both pioneering and recent studies suggested that deep-sea benthic fauna present a rich assortment of reproductive modes and life-history traits, including direct development, brooding, lecithotrophic, and planktotrophic larvae (Mosely, 1880; Thorson, 1950; Pearse and Lockhart, 2004; Arellano and Young, 2009; Bennett et al., 2012; Berecoechea et al., 2017; Martinez and Penchaszadeh, 2017; Lauretta et al., 2020; Rivadeneira et al., 2020).
In summary, based on the current literature, the general view of the typical deep-sea fauna is one of taxa of small size, non-sessile, often deposit feeding and developing either directly or indirectly. These and other biological characteristics of species, also referred to as traits (Bremner et al., 2005), can be used to assess ecosystem functioning, that is the maintenance and regulation of ecosystem processes (Naeem et al., 1999), including organism-environment interaction (Bremner et al., 2006; Degen et al., 2018). Seafloor fauna are heavily involved in ecosystem processes such as consumption and transfer of organic matter to higher trophic levels, organic matter decomposition, nutrient renewal, productivity and habitat provision (e.g., Danovaro et al., 2008; Loreau, 2008; Thurber et al., 2014). These processes depend, directly or indirectly, on morphological, behavioral and life history traits that species exhibit in a community (Usseglio-Polatera et al., 2000; Bremner et al., 2003; Oug et al., 2012). Thus, an assessment of these traits can provide a deeper insight into functional structure and variation than is possible with a taxonomic description of a community alone (Bremner et al., 2003; Petchey and Gaston, 2006; van der Linden et al., 2012; Pomerleau et al., 2015). Biological trait analysis (BTA) (cf. Bremner et al., 2003) can assess functional characteristics of a given community as well as ecosystem vulnerability through metrics such as functional diversity (FD) (i.e., diversity of trait categories called modalities), and functional redundancy (FR) (i.e., a measure of the degree to which species exhibiting the same trait modalities (Bremner et al., 2003; Petchey and Gaston, 2006; van der Linden et al., 2012). Studies investigating Arctic ecosystem function using the BTA approach have advanced our understanding of functional structure of benthic communities mostly on the shelves and for macrofauna (i.e., mostly infaunal taxa ≥0.5 or 1 mm) (Cochrane et al., 2012; Krumhansl et al., 2016; Kokarev et al., 2017; Rand et al., 2018), while few studies have so far focused on biological traits of epifaunal megafauna (i.e., invertebrates and demersal fish on top of the sediment and typically ≥ ca. 5 mm) (Sutton et al., 2020) or deep ecosystems (Degen, 2015; Liu et al., 2019). Functional patterns have not been examined for epifaunal communities anywhere in the Arctic deep sea.
The goal of this study was, therefore, to characterize Arctic deep-sea epifauna using a biological traits approach in the Chukchi Borderland (CBL) in the Amerasian Arctic deep sea, an area of complex habitats created by plateau and ridge areas at mid-depths, surrounding or bordering deeper basins (Jakobsson et al., 2008). Specifically, the objectives of the present study were to: (1) identify dominant trait modalities represented in the epifauna of the study area; (2) describe variability in functional structure of epifaunal communities between mid-depth (plateau and ridge) and deeper (basin) areas; and (3) identify environmental factors influencing the functional structure of epifaunal communities in the study area. We tested the following hypotheses: (1) current deep-sea paradigms suggesting that deep-sea benthic communities are dominated by small-sized, non-sessile deposit-feeders or scavengers, with equal representation of direct and indirect development, hold true for the epifauna in the CBL; and (2) given that environmental conditions change with depth, there is a difference in functional structure between mid-depth and deep communities. Specifically, we proposed that (a) trait-modality composition changes with depth strata; (b) the more heterogeneous mid-depth habitats provide higher diversity of niches reflected in higher FD, while the more homogeneous deep-basin stations are likely to have lower FD but higher FR; and (c) depth, food availability and food quality strongly influence distribution of trait modalities across the study area.
Materials and Methods
Study Area and Field Sampling
The community composition data underlying this study were collected in the CBL, north of Alaska (7–78°N, 158–165°W) onboard USCGC Healy in July–August 2016 (Figure 1). The CBL extends from the Chukchi shelf into the Canada Basin, covering a depth gradient from ∼300 to 3000 m. It consists of the Northwind Ridge and Chukchi Plateau where stations were grouped as “mid-depth” (486–1059 m), and of the isolated Northwind Basin where stations were grouped as “deep” (1882–2610 m) (Figure 1 and Table 1; Jakobsson et al., 2008; Mayer et al., 2010). Waters of Arctic, Atlantic, and Pacific origins interact in the CBL study area with Pacific-origin water comprising the Polar Mixed Layer and upper halocline (McLaughlin et al., 2004; Steele et al., 2004; Woodgate, 2013). The lower halocline is of Atlantic origin, arriving from Fram Strait and the Barents Sea (Woodgate and Aagaard, 2005). Underneath it and characterizing the “mid-depth” stations is the Atlantic water layer (McLaughlin et al., 2004; Woodgate et al., 2007; Bluhm et al., 2015), while “deep” stations are in the Arctic Ocean deep-water layer originating from the Greenland Sea and spreading across the Eurasian Basin to the Canada Basin (Bluhm et al., 2015).
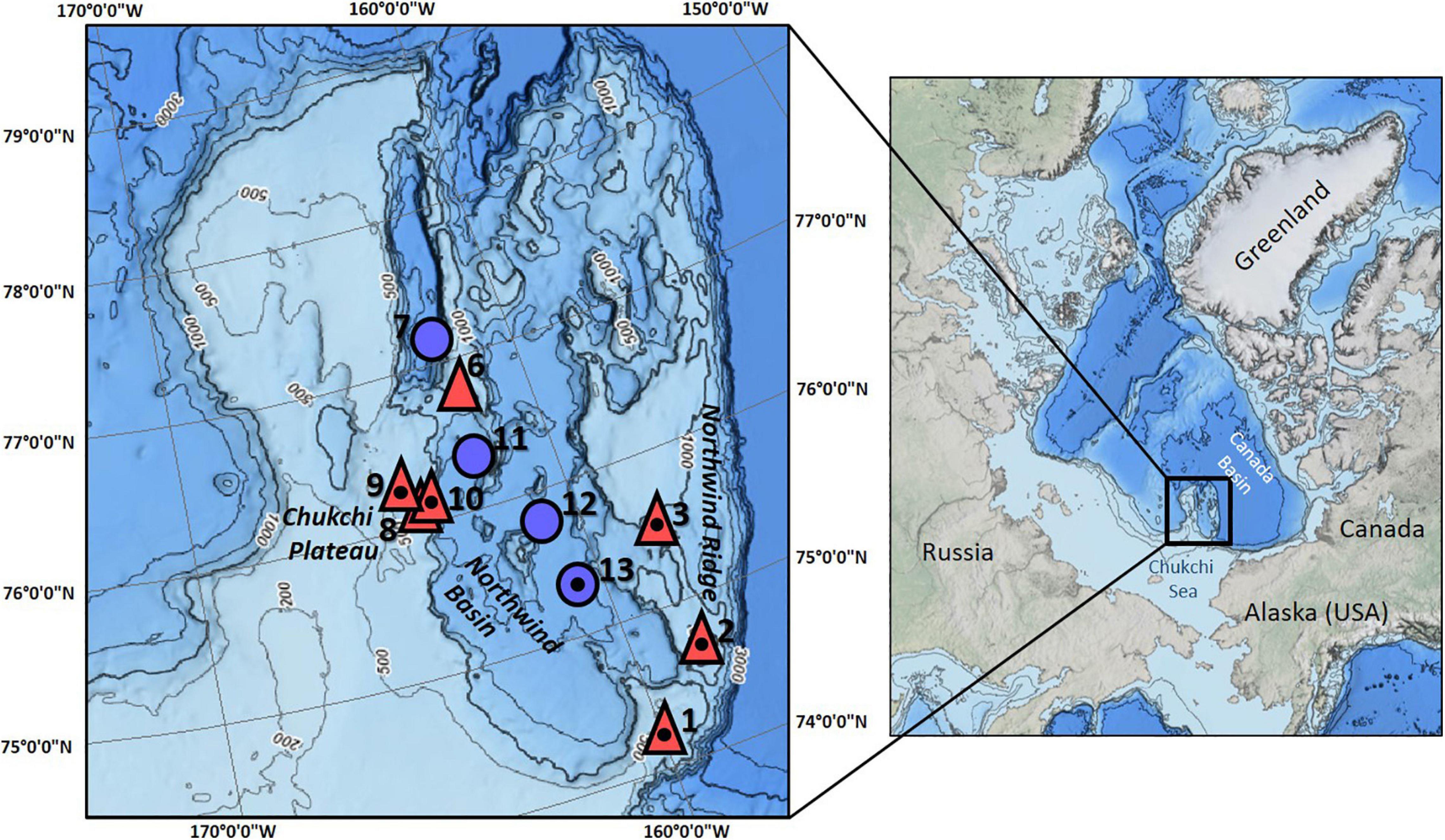
Figure 1. Study area and stations sampled in the Arctic Chukchi Borderland. Red triangles are mid-depth stations, blue circles are deep stations; numbers in bold print are station numbers; small numbers along isobaths indicate water depth. All stations were sampled by ROV, except station 3. Stations that were additionally sampled by trawl are indicated by a dot inside of the triangle/circle.
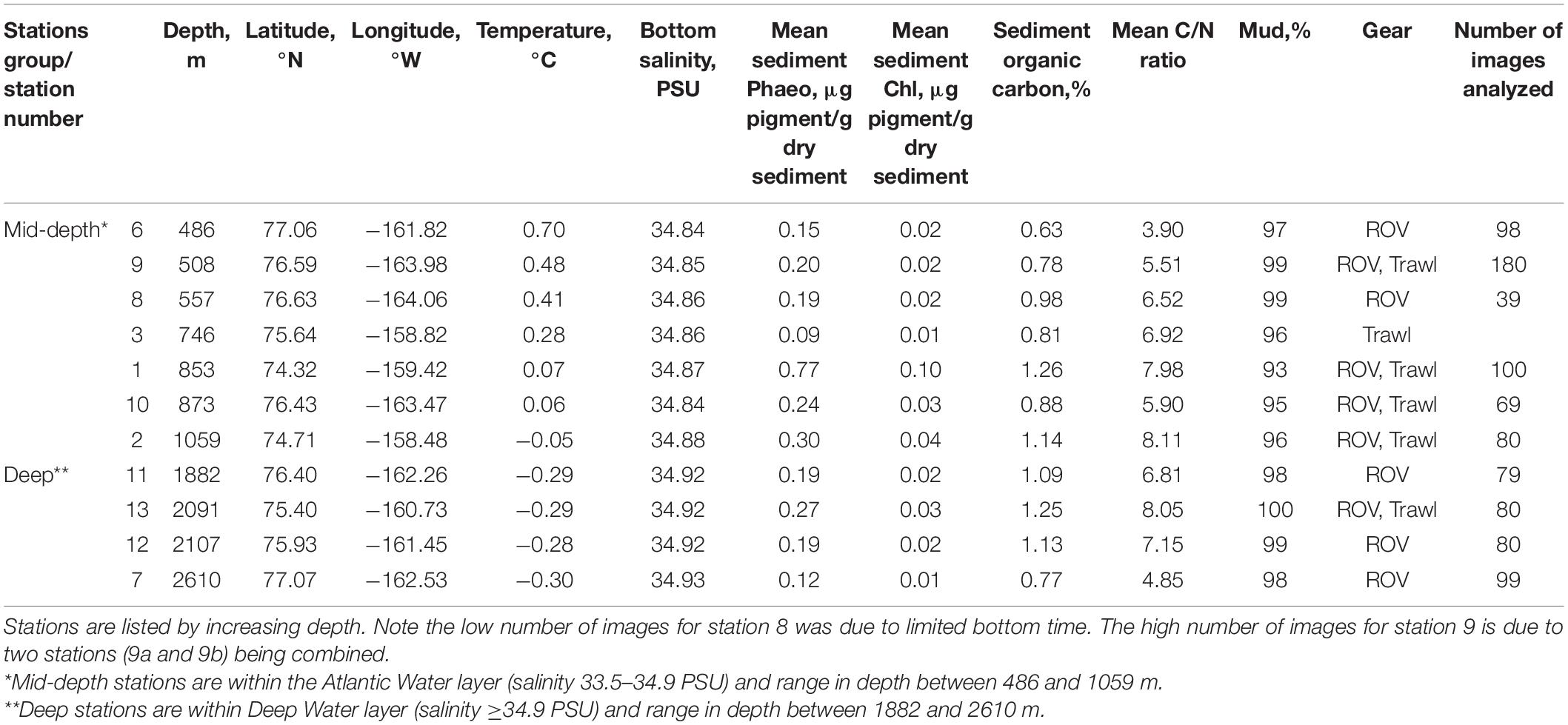
Table 1. Station information for ROV images and beam trawl samples collected in the Arctic Chukchi Borderland.
Epifauna (including invertebrates and demersal fishes) was sampled with the ROV Global Explorer (Oceaneering International), which performed a photographic survey of the seafloor at ten stations (Table 1), as described in Zhulay et al. (2019). 24-megapixel still images were collected with a downward-looking DSSI DPC-8800 digital camera along transects every 5–8 s. Four digital laser pointers, one located at each corner of a fixed distance of a 10-cm square, served as a size reference for the imaged area and size of organisms at four stations (stations 1, 6, 7, and 8), after which they stopped functioning. In addition, epifauna was sampled with a single trawl sample at six stations (stations 1, 2, 3, 9, 10, and 13, Table 1) using a 3.05 m modified plumb staff beam trawl (Abookire and Rose, 2005) equipped with a 7 mm mesh net with 4 mm in the cod end. Ca. 30 min hauls at ∼1.5 knots speed over ground were taken with bottom time estimated from a time depth recorder (Star Oddi) affixed to the net. Organisms were sorted, identified to the lowest possible taxonomic level, and counted. Select taxonomic vouchers were further identified by expert taxonomists (see section “Acknowledgments”) and taxon names were verified using WoRMS (http://www.marinespecies.org/, on September 10, 2020). The proportional abundance of each taxon was then calculated for each trawl station.
At each station, near-bottom water temperature and salinity were measured with a SBE9/11 + CTD at ∼20 m above the bottom. Sediment surface samples (0–1 cm) from box core samples were taken and frozen at −20°C for later determination of grain size composition, carbon and nitrogen content, and concentration of sediment chlorophyll a and phaeopigments (Zhulay et al., 2019). Sediment grain size was analyzed from samples pre-treated with HCl and H2O2, to remove calcium carbonate and organic material, on a Beckman Coulter Particle Size Analyzer LS 13320 at the Geology Laboratory of UiT The Arctic University of Norway in Tromsø. Sediment organic carbon and nitrogen (%) were determined on a Costech ESC 4010 elemental analyzer at the stable isotope facility at the University of Alaska Fairbanks (UAF). The C/N ratio, an indicator of food quality with higher values indicating lower food quality (e.g., Dorgelo and Leonards, 2001; Iken et al., 2010), was then calculated for each station. Concentrations of sediment chlorophyll a and phaeopigments (μg pigment/g dry sediment) were measured on a Turner Designs TD-700 fluorometer after pigment extraction with 5 ml of 100% acetone for 24 h in the dark at −20°C at UAF. The fluorescence of the sample was read before and after acidification with HCl (final concentration of HCl was 0.003 N) for determination of phaeopigments (Arar and Collins, 1997; Jeffrey and Welschmeyer, 1997).
Image Analysis
A subset of the useable ROV images of the sea floor were manually analyzed from each station (39–180 per station, 940 images in total) (Table 1, Zhulay et al., 2019). Image processing and analyses were performed with the ImageJ1 (Rasband, 2009). Taxa were identified to the lowest possible level based on a combination of morphological features visible on the ROV imagery, the voucher collection from trawls, and additional identifications by taxonomic experts (see acknowledgments). Taxa that could not be identify were excluded from this analysis due to difficulties related to assigning trait modalities to these organisms. All taxa present on the images were counted per image and proportional abundance of taxa per station was calculated. Rocks larger than two cm were counted and the average number of rocks per picture was included in the statistical analyses as an environmental factor.
Biological Traits
Nine commonly used traits represented by a total of 39 modalities were chosen for the present analysis following established definitions by Bremner et al. (2006), Costello et al. (2015), Degen et al. (2018), and Sutton et al. (2020) (Table 2). The traits reflected morphology (adult size, body form), behavior (living habitat, mobility, adult movement, feeding habit, substrate affinity) and life-cycle characteristics (larval development and reproduction) (Table 2; reviewed by Martini et al., 2020a). For the purpose of this study, modalities of larval development trait were based on the published concept for Arctic traits analysis (Degen and Faulwetter, 2019) that assumes that planktotrophs disperse farther than lecithotrophs although many exceptions are known to occur in the deep sea (Young, 2003). Every trait was coded for every taxon identified based on: (1) observations made from trawl-collected material during the cruise and/or from ROV images (i.e., traits directly measured in situ, also referred as realized traits, c.f. Martini et al., 2020a) for size, body form, adult movement, living habit, and substrate affinity or (2) information inferred from published literature (referenced in Supplementary Table 1), online traits databases (e.g., polytraits, Faulwetter et al., 2014; the Arctic Traits Database, Degen and Faulwetter, 2019) and relevant web pages (e.g., FishBase2, Sea Life Base3) (i.e., traits acquired from other sources, also referred as potential traits, c.f. Martini et al., 2020a) for the rest of the traits. The size of organisms was measured on board from specimens collected at each station in the trawl samples or from the ROV images with the ImageJ software (Rasband, 2009). Size measurements from ROV images were possible at the four stations where the digital laser pointers were functioning and where the positioning of a given organism was suitable for those measurements. The average adult size of a given species across all stations was used for the analysis. As information about biology and behavior of many epifaunal taxa in the Arctic deep sea remains limited or is at times non-existent at the species level, coding of these taxa was conducted based on information available for closely related species in the same genus or family (following, e.g., Faulwetter et al., 2015; Rand et al., 2018; Sutton et al., 2020). In a few cases, modalities common at even higher taxonomic rank (such as direct development in Peracarida) were applied. For coding a “fuzzy coding” procedure (Chevenet et al., 1994) was used, resulting in a “traits by taxon” matrix (Supplementary Table 2). The “fuzzy coding” procedure allows taxa to be coded with multiple modalities to different degrees using a 0–3 code, with 0 indicating no affinity, 1 and 2 indicating partial affinity, and 3 indicating the highest affinity for a given modality. This approach was proposed to account for variation encountered within a species (Chevenet et al., 1994) and when incorporating information from species in the same genus or family (Charvet et al., 2000). While including higher taxonomic levels may introduce uncertainty to the results, their suitability has been documented for biological traits analysis as well as taxonomic community analysis, especially in multivariate analyses that showed that the functional structure of communities could be conserved (Bournaud et al., 1996; Bowman and Bailey, 1997; Dolédec et al., 1998). To give the same weight to each taxon and trait, the fuzzy codes (0–3) were converted to proportions for each trait modality totaling to 1 (e.g., Bolam et al., 2017).
A total of 106 invertebrate and fish taxa were used for BTA, of which 53 taxa occurred in the ROV images and 77 taxa in trawls with 26 taxa common to both sampling gears. In addition to the “traits by taxon” matrix, “taxa by stations” (i.e., presence/absence of taxa or proportional abundances of taxa at each station) and “traits by stations” (i.e., trait composition at each station, obtained by multiplying the “taxa by stations” and “traits by stations” matrices and indicated by presence/absence or proportional abundance weighted scores) matrices were generated (following Beauchard et al., 2017; Degen et al., 2018). Three “taxa by stations” (Supplementary Tables 3–5) and three “traits by stations” (Supplementary Tables 6–8) matrices were compiled for subsequent analysis: presence/absence of taxa based on ROV and trawl samples combined across all stations, and one each with proportional abundances acquired from either ROV or trawl samples. Proportional abundance was chosen over absolute abundance due to the above-mentioned failure of the laser pointers that made it impossible to calculate absolute abundances for all ROV stations. Proportional abundance was also used for trawl samples for consistency.
Data Analysis
To test our first hypothesis, namely that epifaunal organisms in the CBL are predominantly small-sized, non-sessile deposit-feeders or scavengers with equal representation of direct and indirect development, we included the four traits: size, larval development, adult movement and feeding habit. The “traits by stations” matrix was used to test this hypothesis; it was based on presence/absence data of taxa collected with both the ROV and the trawl (Supplementary Table 6).
To test the second hypothesis, namely that a difference in functional structure exists between mid-depth and deep communities, all nine traits were used. To investigate differences in the overall functional composition of epifauna and visualize potential differences between these two depth strata, we applied a fuzzy correspondence analysis (FCA, Chevenet et al., 1994). FCA is an extension of a regular correspondence analysis that is suitable for fuzzy coded traits data of discrete variables (Chevenet et al., 1994). FCA is based on the “traits by stations” matrix (Supplementary Tables 7, 8) and identifies and visualizes traits and their modalities contributing most to the difference in the functional structure among stations (Bremner et al., 2006), and provides correlation ratios of each trait along the fuzzy principal axes, representing the amount of variance of a certain trait modality explained by a given axis (Chevenet et al., 1994). Correlation ratios greater than 10% were considered as the traits contributing most to variation among the stations following Conti et al. (2014). A Kruskal–Wallis test was used to test for significant differences in proportional abundance of trait modalities between mid-depth and deep stations using the “traits by stations” matrix from ROV data (Supplementary Table 7). Only one trawl sample was available from deep stations preventing statistical comparisons.
We then visualized which of the available environmental variables explained most of the variation in the functional structure of the epifaunal communities using a canonical correspondence analysis (CCA) performed on the “traits by stations” matrices for ROV (Supplementary Table 7) and trawl (Supplementary Table 8) samples. For environmental data, we included water depth, bottom water salinity and temperature, grain size composition, number of rocks in ROV images, concentration of benthic pigments in sediment (phaeopigments and Chl a), carbon content in sediment, and C/N ratio. A forward selection procedure was used to identify environmental variables explaining most of the variability in the trait-by-station data. These variables were then used in the model, whereas other factors were overlaid on the plots as passive factors. The significance of the models and environmental variables were tested with Monte Carlo permutation tests (Oksanen et al., 2013).
As part of hypothesis two, we tested for differences in functional diversity and redundancy between depth strata for both ROV and trawl-based data. FD was estimated using Rao’s quadratic entropy (Rao’s Q), which is a measure of trait dissimilarity (Rao, 1982; Botta-Dukát, 2005). Rao’s Q ranges from 0 to 1, where 0 means low FD (i.e., communities are the same in their biological trait profiles) and 1 means high FD (i.e., communities are unique in their biological trait profiles) (Van der Linden et al., 2016). FD was calculated based on the “traits by taxon” (Supplementary Table 2) and “taxa by stations” (Supplementary Tables 4, 5) matrices.
FR is the relationship between FD and species diversity (Ricotta et al., 2016), and was calculated as the ratio of FD to the taxonomically based Simpson index (D, calculated using equation (1)).
where n is the total number of organisms of a particular species, and N is the total number of organisms of all species. In order to obtain a regularly increasing index, the formula was converted to: 1–(FD/D) (Van der Linden et al., 2016). FR defines to which degree different species represent the same ecosystem functions (Petchey and Gaston, 2006; de Bello et al., 2007) and ranges from 0, where all species have different trait-categories, to 1, meaning all species display the same trait-categories (de Bello et al., 2007). A Kruskal–Wallis test was used to check for significant differences in FD and FR between mid-depth and deep ROV stations.
All statistical analyses were performed using the software R (R Core Team, 2017) with the package ade4 (Dray and Dufour, 2007) for the FCA and calculation of FD index, and the package vegan (Oksanen et al., 2013) for the CCA analyses. A schematic representation of the hypotheses tested and methods used to test the hypotheses, along with figure and table numbers representing results of the tests, is given in the Supplementary Figure 1.
Results
Trait Modality Composition
The body size modality “small-medium” was the most frequent in the epifauna across the study area, while size “large” was rarest (Figure 2A). The most frequent larval development was “direct” followed by “lecithotrophic,” while the occurrence of the modality “planktotrophic” was much lower (Figure 2B). Non-sessile adult movement modalities combined were more frequent than sessile forms. Individually, “crawlers” were dominant, though “sessile” was second most frequent and since this study focused on epifauna, “burrowers” were expectedly least frequent in the data set (Figure 2C). Feeding habit “predators” was most frequent, followed by “suspension feeder.” The least frequent modalities of feeding habit were “parasite/commensal” and “subsurface deposit feeder” (Figure 2D).
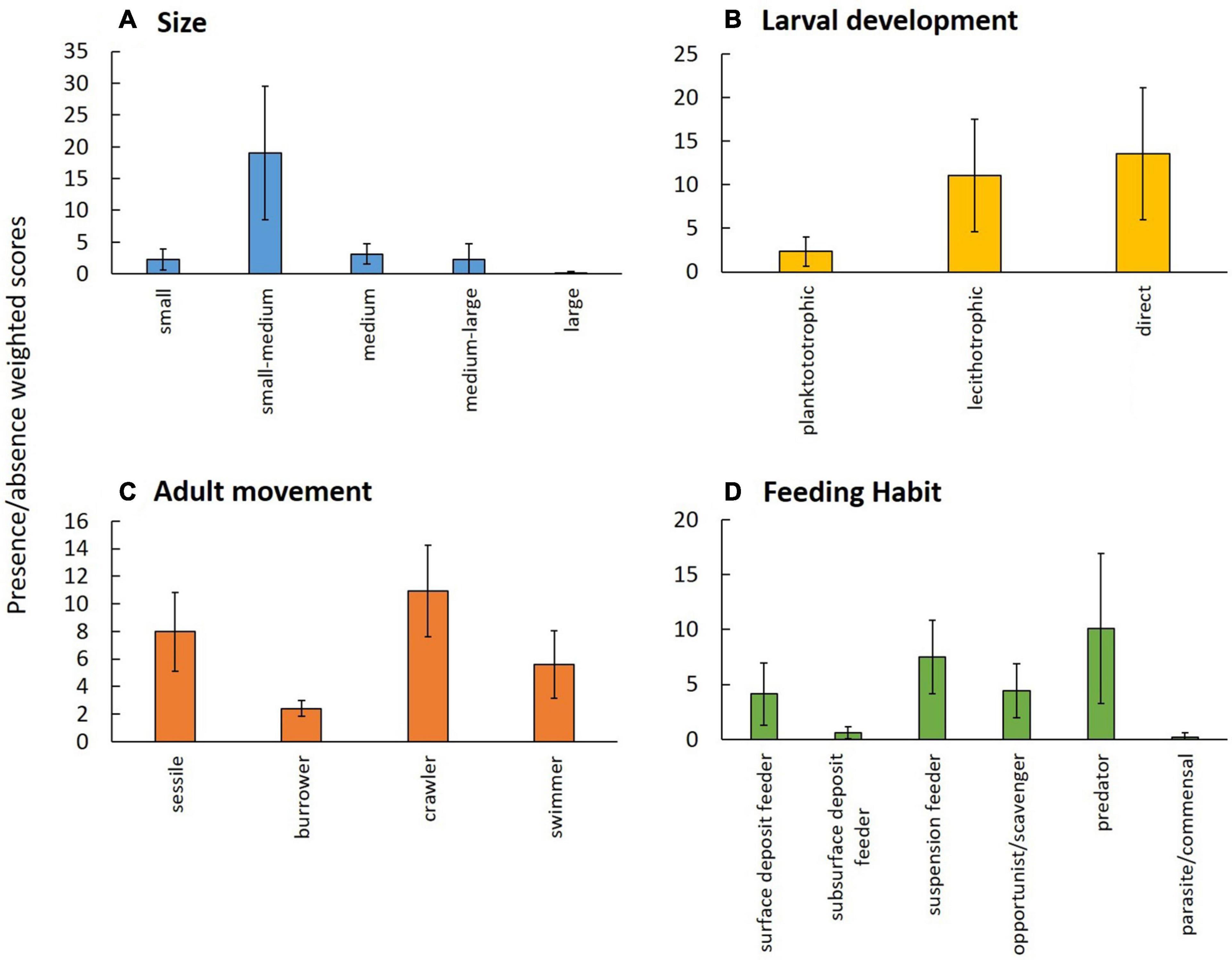
Figure 2. Occurrence of trait modalities based on presence/absence of taxa for combined ROV and trawl data of epibenthos from the Chukchi Borderland and averaged across all stations ± SD; (A) body size, (B) larval development, (C) adult movement, and (D) feeding habit; note that y-axes have different scales. Trait modalities are defined in Table 2.
Functional Structure of Epifaunal Communities
The FCA showed substantial variation in functional composition across all stations. The first two axes of the FCA accounted for 81.9% of the variability in distribution of trait modalities, with 51.3% for the first and 30.6% for the second axis (Figure 3A). Most of the variation along the first axis was explained by body form (BF) (31%), reproduction (R) (20%), living habit (LH) (14%), feeding habit (FH) (18%), and substrate affinity (SA) (19%) (Table 3).
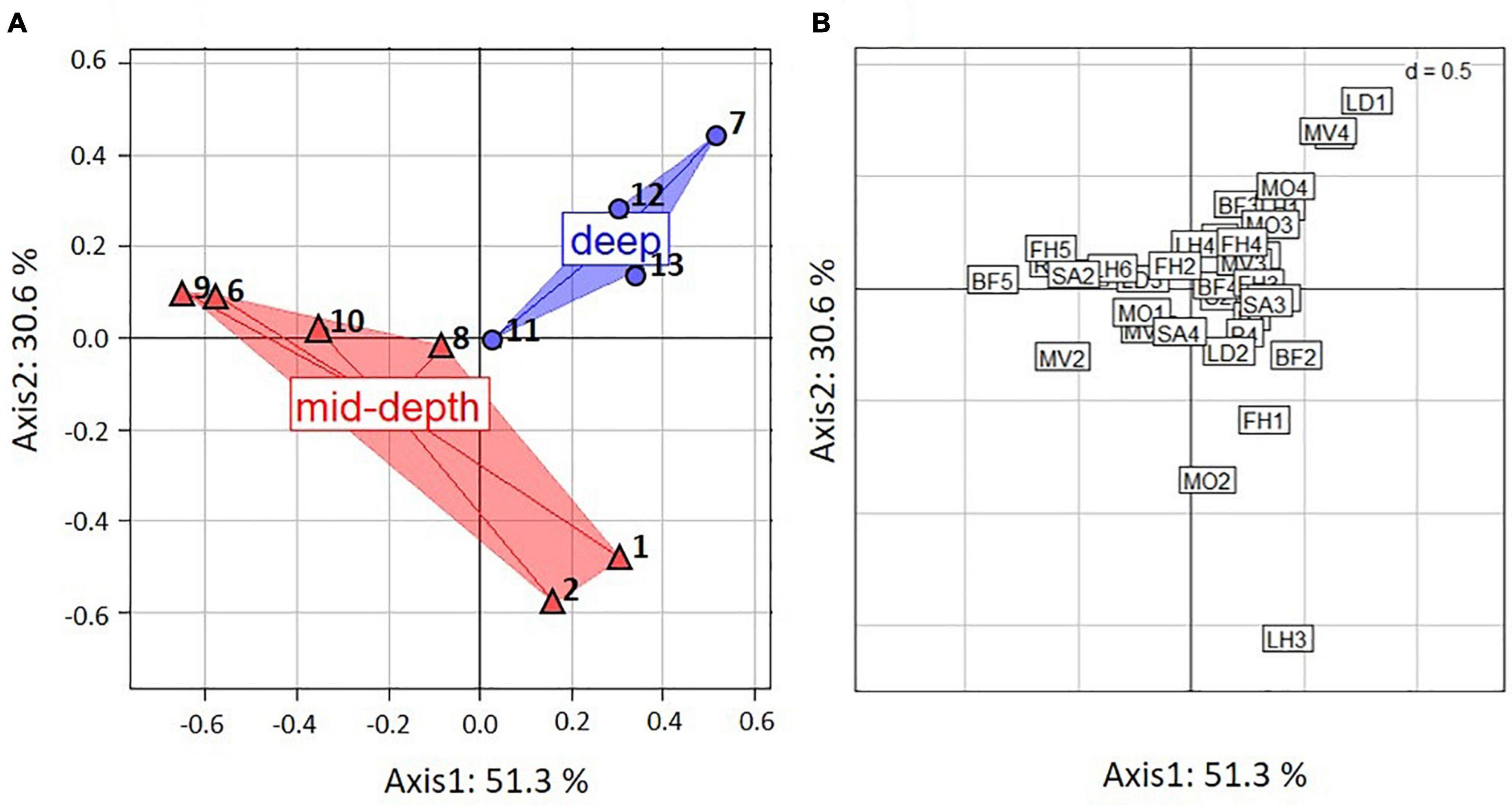
Figure 3. Results of fuzzy correspondence analysis (FCA) on the biological traits of epifauna from the Chukchi Borderland weighted by taxa proportional abundances from ROV samples, (A) ordination by stations, where numbers are station numbers, (B) ordination by traits, where trait modality codes are abbreviated by two letter-one number combinations and explained in Table 2. Red – mid-depth stations. Blue – deep stations.
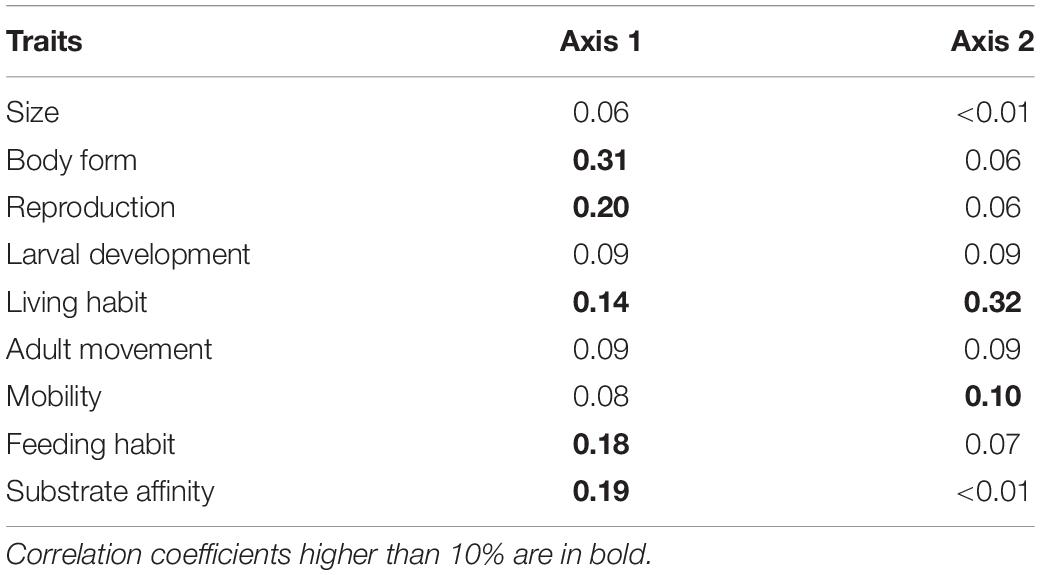
Table 3. Correlation ratios of each biological trait on the first two axes of the fuzzy correspondence analysis for ROV samples of epibenthos in the Chukchi Borderland.
Trait modality composition generally differed between mid-depth and deep stations (Figure 3A). Deep stations 7, 12, and 13 were located on the upper right hand side of the FCA plot and corresponded to higher proportional abundance of modalities “swimming” (MV4), “sexual-internal fertilization” (R3) and “planktotrophic larval development” (LD1) (Figures 3A,B). Mid-depth stations 6, 9, and 10, located on the lower left hand side of the FCA plot, were characterized by higher proportional abundance of “upright body form” (BF5), affinity for hard substrate (SA2) and “predators” (FH5) (Figures 3A,B). Variation along the second axis was driven mostly by living habit (LH) (32%) and mobility (MO) (10%) (Table 3). These traits separated mid-depth stations 1 and 2 from the rest of the stations (Figure 3A), and the two stations were characterized by higher proportional abundance of modalities “low mobility” (MO2), “sessile” (MV1), “deposit feeding” (FH1), and “tube-dwelling” (LH3) (Figure 3B). Deep station 11 and mid-depth station 8 were not differentiated from the remaining stations based on the traits used. In general, results for the trawl data supported those from the ROV (Supplementary Figure 2 and Supplementary Table 9). The difference was that higher proportional abundance of modalities “sessile” (MV1) and “small size” (S1) was observed at the single deep trawl station, while mid-depth trawl stations were characterized by high proportional abundance of modalities “free-living” (LH1), “crawlers” (MV3), “dorsoventrally compressed” (BF3), and “medium size” (S3) (Supplementary Figure 2).
Results of the Kruskal–Wallis test indicated significant differences between mid-depth and deep stations for body form (BF), feeding habit (FH), reproduction (R), and lifestyle and mobility traits [adult movement (AM), living habit (LH), and mobility (MO)]. Deep stations were characterized by significantly higher proportional abundance of the body form “globulose” (BF1), feeding habits “suspension feeder” (FH3) and “opportunist/scavenger” (FH4), reproduction “sexual-internal fertilization” (R3), and higher proportional abundance of “free-living” (LH1), “highly mobile” (MO4), and “swimmer” (MV4) modalities (Kruskal–Wallis test; Table 4 and Figure 4). Mid-depth stations had significantly higher proportional abundance of the body form “upright” (BF5), living habit “tube-dwelling” (LH3), and mobility “slow movement” (MO2) (Kruskal–Wallis test; Table 4 and Figure 4). The Kruskal–Wallis test was not run on trawl data because only one deep station was sampled by trawl, but boxplots for the trawl data indicated generally similar patterns to those from the ROV data. Differences included higher proportional abundance of modalities “attached” and “sessile” at the single deep trawl station than at deep ROV stations, and higher proportional abundance of modalities “free-living” and “crawling” at the mid-depth trawl stations (Supplementary Figure 3).
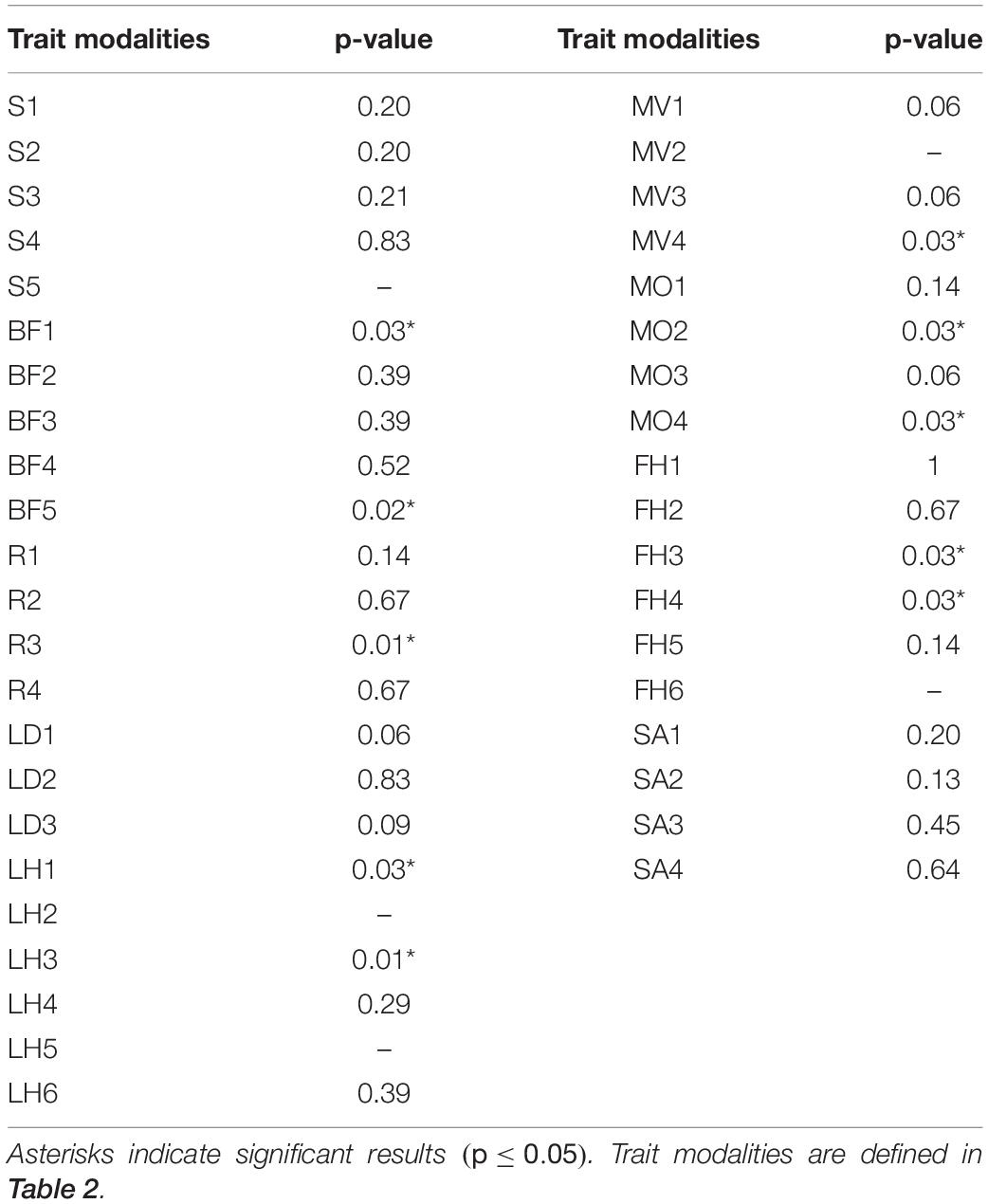
Table 4. Results of Kruskal–Wallis test comparing proportional abundance of modalities at deep and mid-depth stations for ROV data of epibenthos in the Arctic Chukchi Borderland.
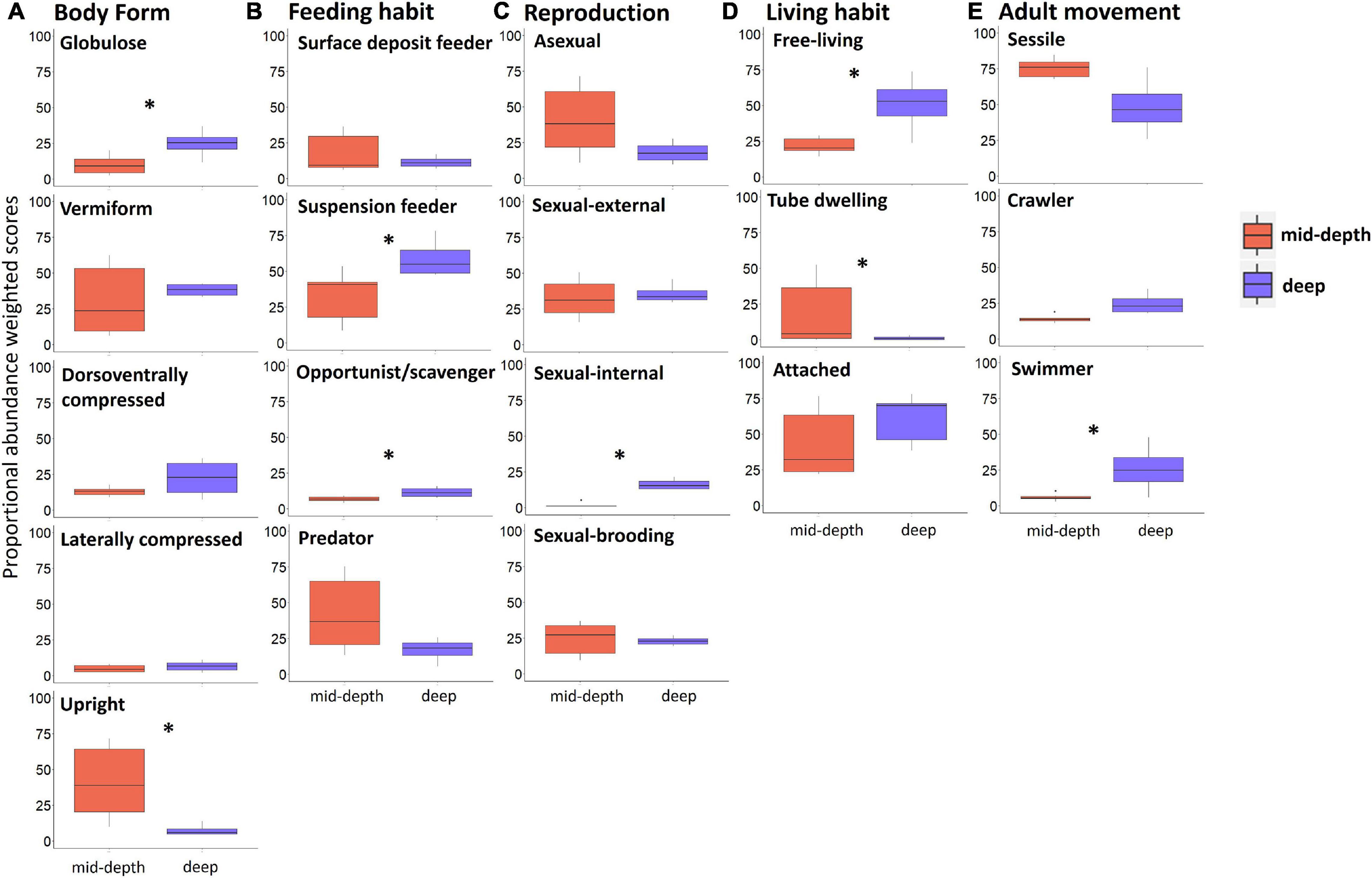
Figure 4. Boxplots comparing modalities of different traits (weighted by proportional abundance) for mid-depth (in red) and deep stations (in blue) based on ROV samples of epifauna from the Chukchi Borderland; (A) body form, (B) feeding habit, (C) reproduction, (D) living habit, and (E) adult movement. Note traits with significant difference between depth strata and traits contributing most to variability among stations based on the Fuzzy Correspondence Analysis results are shown; modalities of these traits with low proportional abundance are not shown. Asterisks indicate significant difference between mid-depth and deep stations (p < 0.05, Kruskal–Wallis test). Trait modalities are defined in Table 2.
Significantly lower FD and higher FR were found for deep ROV stations compared with mid-depth stations (p = 0.03 for both, Figure 5). Though the lack of station replication prevented statistical analysis for the trawl samples, the trends in FD and FR were similar to the ROV results, though the difference in FD and FR between the two depth strata was less distinct (Supplementary Figure 4).
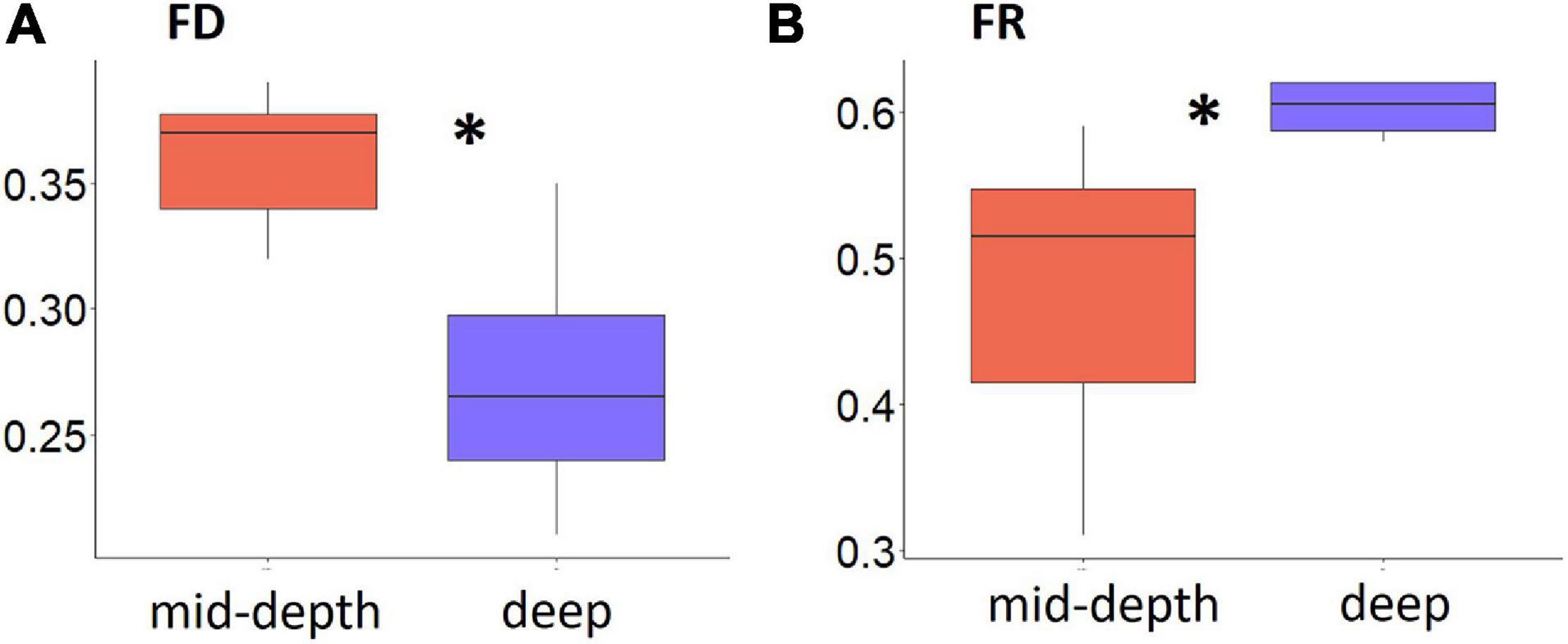
Figure 5. Boxplot of functional diversity indices for epifauna from the Chukchi Borderland, (A) Functional Diversity (FD) (represented by Rao’s Q) and (B) Functional Redundancy (FR) for mid-depth and deep ROV samples. Asterisks indicate significant differences between mid-depth (red) and deep (blue) stations (p < 0.05, Kruskal–Wallis test). Note the scales of the y-axes are different.
Environmental Factors Influencing Functional Structure of Epifaunal Communities
Results of the CCA for the ROV samples showed that depth and sediment organic carbon content were the most important factors explaining variability in the distribution of trait modalities (Table 5). These environmental factors explained 64% of the total variation. Depth was positively associated with “dorsoventrally” compressed (BF3) and “globulose” (BF1), “internal fertilization” (R3), “free-living” (LH1), “swimming” (MV4), and “suspension-feeding” (FH3), modalities (Figure 6). High carbon content was positively associated with the modalities “vermiform” (BF2), “sexual-external fertilization” (R2), “sexual-brooding” (R4), “tube-dwelling” (LH3), and “surface deposit-feeding” (FH1) (Figure 6).
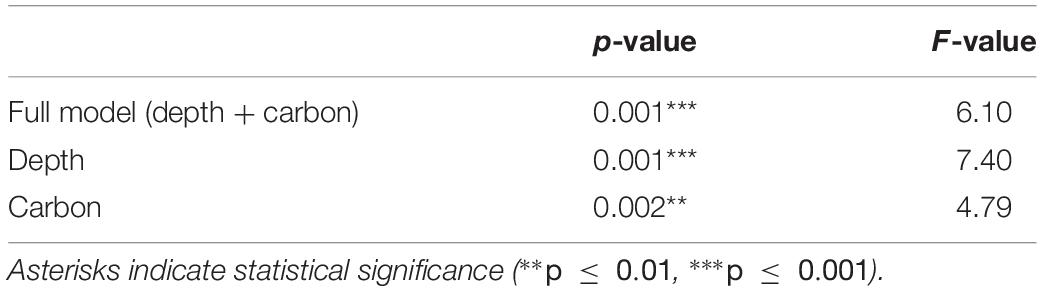
Table 5. Results of canonical correspondence analysis using Monte-Carlo permutation test, performed on a traits by stations matrix for ROV samples of epibenthos in the Arctic Chukchi Borderland.
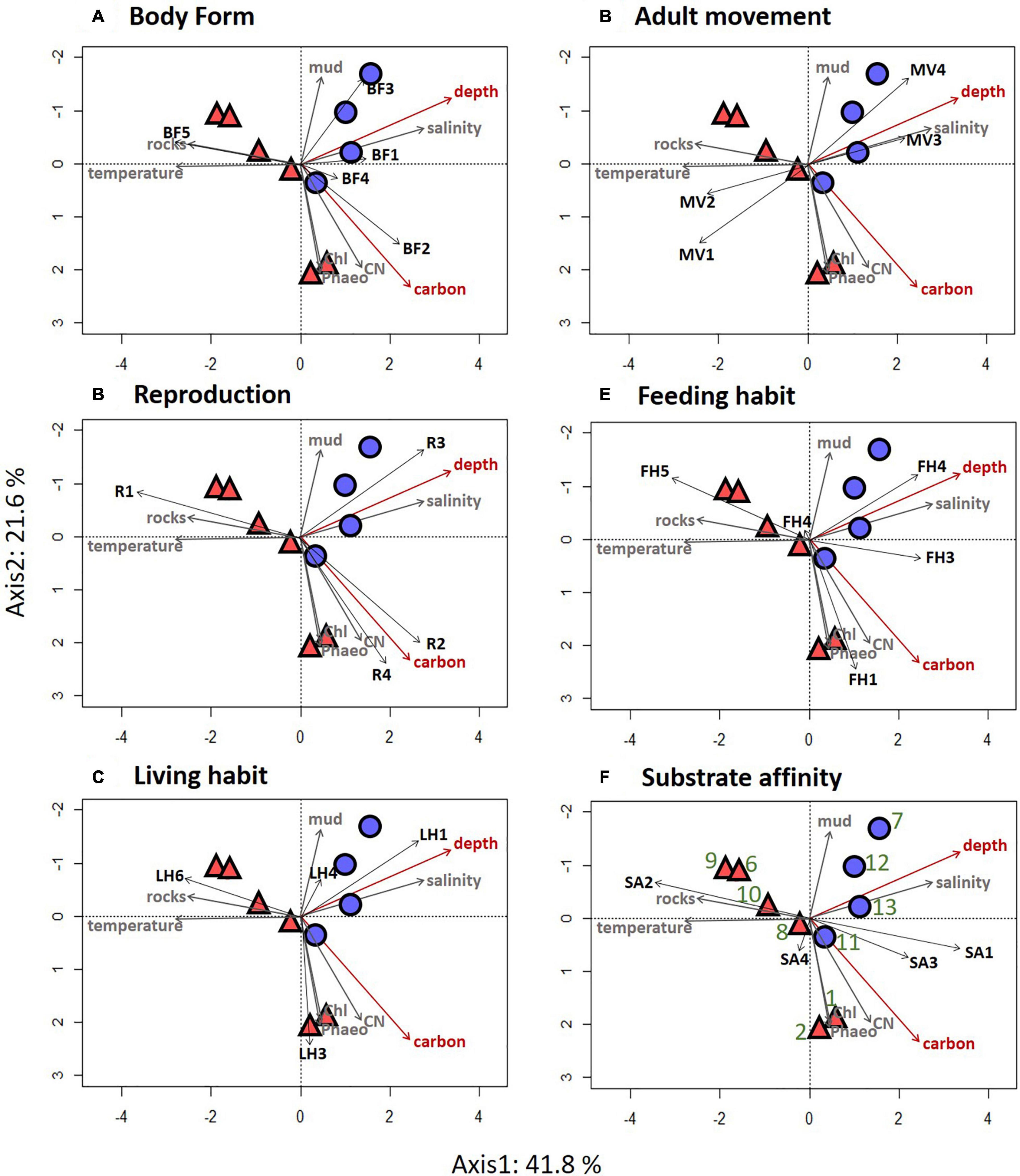
Figure 6. Results of the canonical correspondence analysis (CCA) performed on the traits by stations matrix for ROV data of the epifauna from the Chukchi Borderland. Traits identified as most important in the Fuzzy Correspondence Analysis are presented; (A) body form, (B) reproduction, (C) living habit, (D) adult movement, (E) feeding habit, and (F) substrate affinity. Passive environmental factors are overlain on the figure panels in gray color; environmental factors in red are those that were used to constrain the CCA. Mid-depth stations are present in red triangles and deep stations are present in blue circles. Station numbers are identified in green on the last CCA plot. Trait modality codes are abbreviated by two letter-one number combinations and explained in Table 2.
Results of the CCA for trawl samples also indicated depth as the most important factor influencing the functional composition of epifaunal communities, with temperature also being a significant factor (Supplementary Table 10). Both factors were used to constrain the CCA, resulting in 92.9% of the total variation explained. In contrast to the ROV data, depth was positively associated with “sessile” (MV1) and “attached” (LH6), and substrate affinity “hard” (SA2). Temperature was positively associated with “predators” (FH5) and “opportunist/scavenger” (FH4) modalities, and substrate affinity “biological” (SA3) (Supplementary Figure 5).
Discussion
Trait Modalities Composition Across the Deep-Sea CBL
Body size of organisms affects many ecological functions including energy and nutrient cycling, and secondary production (Degen et al., 2018). One of the most common characteristics of deep-sea benthos is the small size of most species (Rex and Etter, 1998). Our study results are consistent with this paradigm and hence with our hypothesis, in that the second smallest (small-medium size, 10–50 mm) organism category had the highest occurrence, while large organisms (≥50 mm) had the lowest occurrence in epifaunal communities across the CBL. Low occurrence of the smallest size category is unsurprising given that we targeted epibenthic megafauna (typically ≥10 mm). A series of studies reporting reduced average body size with depth for deep-sea meiofauna (Soltwedel et al., 1996; Soetaert et al., 2002; Kaariainen and Bett, 2006) and macrofauna (Rex et al., 1999; Kaariainen and Bett, 2006) generally support Thiel’s size-structure hypothesis (Thiel, 1975) for these groups. This decrease in body size with depth has also been found for epifauna (Rex et al., 2006; Wei et al., 2010). Opposite to this trend, some deep-sea taxa with larger body sizes than in shallow areas have also been documented (Rex and Etter, 1998), in some cases resulting in gigantism. This phenomenon, often attributed to low temperature and high oxygen availability that causes slow growth rate and longevity (Shirayama and Horikoshi, 1989), has been found for deep-sea isopods, amphipods, pycnogonids, ostracods, and anemones (Timofeev, 2001; Danovaro et al., 2014) but in our study, only the very large pycnogonid Colossendeis proboscidea could fit this concept.
Overall, we confirm our hypothesis that the majority of epifauna found in our study was non-sessile; most were crawlers but swimmers were also found. Not unexpectedly, burrowers were less common, given the focus of the study was epifauna. As a consequence of the ability to move organisms can escape from disturbance (natural or anthropogenic), disperse or migrate (Beauchard et al., 2017; Degen and Faulwetter, 2019) and increases the chance of finding scarce and patchy food compared to sessile or less mobile organisms. Still, movement rates of epibenthic megafauna are generally lower in the deep sea compared to shelves (Thistle, 2003; Ruhl, 2007). For example, deep-sea brittle stars and holothurians move at 1–3 cm min–1 and 1–2 cm min–1, respectively, compared with 15–45 cm min–1 and 7 cm min–1, respectively, in shallow waters (summarized in Thistle, 2003). When stimulated, for example by food, however, many deep-sea animals can move faster (Premke et al., 2006; MacDonald et al., 2010; Taylor et al., 2016). For example, we observed unusual swimming behavior in the brittle star Ophiostriatus striatus, perhaps an adaptation to access patchy food falls (Boetius et al., 2013). ROV observations such as ours, hence, increase our often scarce knowledge of traits of deep-sea taxa. Besides mobile taxa, we did also find an unexpectedly high occurrence of the modality sessile in our study, especially obvious in ROV imagery. Sessile taxa in our study area, including ascidians, sponges, stalked cirripedes and crinoids, and zoanthid and nephtyid cnidarians were in part present on the numerous drop stones providing hard substrate for these organisms (Zhulay et al., 2019).
Trophic structure of the Arctic benthic deep-sea communities is poorly studied (but see Iken et al., 2005), though feeding habits influence energy flow, nutrient cycling, secondary production, organic matter decomposition, and nutrient regeneration (Bremner, 2008; Degen et al., 2018). We do know that the major food source is organic detritus originating mostly from the upper productive zone (Fabiano et al., 2001; Thistle, 2003). This organic material often undergoes strong transformation while sinking, decreasing nutritional value and particle size (Thistle, 2003). The paradigm that deposit feeding is among the best strategies to collect and process this organic detritus efficiently (Thistle, 2003) has indeed been supported for both macrofaunal and megafaunal deep-sea communities (Kröncke, 1998; Iken et al., 2005; Rex and Etter, 2010). The deposit feeders were also common yet not dominant in our study area, only partly confirming our hypothesis. Predators and suspension-feeders, however, were more common among our epifaunal taxa. This is contrary to a trend of decreasing proportions of predatory asteroid and gastropod species with depth (Carey, 1972; Rex et al., 1990). Our findings, however, are in agreement with other studies that found that predation is in fact common in oligotrophic seas or in areas with little food input (Kröncke and Türkay, 2003; Wieking and Kröncke, 2003; Vacelet, 2008), and are supported by high nitrogen isotope values in certain taxa of our study area (Iken et al., 2005). One theory states that prey can be more easily detected in the deep sea compared to shallow water environments, as “flow in the benthic boundary layer is slow and thus chemical gradients and pressure waves produced by prey should be more persistent and provide better information for prey location” (Thistle, 2003). Facultative predation is even known for specific deep-sea species of, for example, sponges (Vacelet, 2006; Godefroy et al., 2019; Martini et al., 2020b), and bivalves (Morton, 2016; Morton and Machado, 2019), suggesting feeding modes may be unusual, highly plastic and require more study. A stable isotope-based assessment for the study area is ongoing and will provide more clarification of the species’ feeding modes and trophic levels.
The high occurrence of suspension feeding taxa among the CBL epifauna was initially surprising. Higher current and particle fluxes, proving food for suspension feeders, tend to occur on elevations and slopes (Clark et al., 2010) including the Chukchi Slope Current in the CBL (Corlett and Pickart, 2017) and were suggested to provide food for suspension feeders from the nearby Chukchi shelf to the Northwind Ridge (Bluhm et al., 2005). In addition, suspension feeders on the above-mentioned drop stones can extend above the substrate and into the benthic boundary layer, where the currents are slightly faster and carry food particles (Vogel, 1996). Besides on stones, some taxa (in particular anthozoans) were elevated above the seafloor in other ways, namely either on stalks of crinoids or polychaete tubes or, in the case of most hormathiid anemones (Hormathia spp., Allantactis parasitica) on gastropod shells (usually Colus spp.).
Scavengers were also represented in the study area, although not as highly occurring as reported in some deep-sea areas including the Eurasian Arctic (Klages et al., 2001; Premke et al., 2006). It is unsurprising that parasites/commensals were the least occurring feeding type in the study area given our study focused on epifaunal megafauna of which few are parasitic. Smaller external and internal parasites are in fact occurring in Arctic megafauna, especially in demersal fish (Klimpel et al., 2006), but were invisible on the ROV images. We did encountered taxa such as ribbon worms, isopods and sea leeches, which generally contain parasitic forms on fishes and arthropods (Køie, 2000; Mantelatto et al., 2003; Ravichandran et al., 2009), but we did not observe them on a potential host. Commensalism was encountered for some hormatiid anemones attached to shells of gastropods, a widespread strategy increasing probability of contact with food particles, while providing protection to the host (Buhl-Mortensen et al., 2015). Similar commensal relationships were observed for other anthozoans and the amphipod Amathillopsis spinigera that were often found in association with sessile tubeworms and stalked crinoids. Clearly, more research is needed on parasitic and commensal biotic interactions in the deep-sea.
Little is known about larval development in the Arctic Ocean in general. Recent molecular studies, however, have documented the presence of pelagic larvae of more species than previously acknowledged for the Arctic (Ershova et al., 2019) and the deep sea (Kersten et al., 2019), and detailed studies in the deep sea have added species-specific observations (e.g., Mercier and Hamel, 2008; Martinez and Penchaszadeh, 2017; Montgomery et al., 2017). This trait is important for ecological functions such as dispersal, recolonization, recovery, tolerance to stress, and link between pelagic and benthic realms (Degen and Faulwetter, 2019). In the present study, direct development dominated as a single modality, yet given the sparse species-specific literature, generalizing this conclusion for the Arctic is premature. Nevertheless, advantages of this development type include, for example, protection from various unfavorable environmental conditions in the pelagic realm and settling on unfavorable substrate, and experiencing little planktonic predation. Most important for the deep sea, juveniles are less dependent on either limited or variable food availability (Mileikovsky, 1971). Yet indirect development, including planktotrophic and lecithotrophic larvae, was almost equally prevalent in the epifaunal taxa of our study area, which, in general, supports our hypothesis. This finding is consistent with a growing number of studies documenting the occurrence of pelagic larvae in both polar waters (Schlüter and Rachor, 2001; Fetzer and Arntz, 2008; Kuklinski et al., 2013; Brandner et al., 2017; Ershova et al., 2019) and deep-sea areas (Scheltema and Williams, 2009; Kersten et al., 2019). Among larval development types, lecithotrophs were most common in our study area. This is similar to findings in the NE Greenland, the deep-sea of the NE Atlantic, and Antarctica, where more than 70% of echinoderms were found to reproduce with pelagic larvae, the majority of which were lecithotrophs (Pearse, 1994). Development with pelagic larvae allowing dispersal over broader areas is an advantage, in particular for species with limited mobility (Fetzer and Arntz, 2008; Stübner et al., 2016), which were found in high numbers in our study area. In a work by Mercier and Hamel (2008), depth-related shifts in life history strategies and a simultaneous combination of brooding and broadcast-spawning with lecithotrophic larvae were reported in a deep-sea asteroid. This finding also stresses the need to species- and habitat-specific work to help close many knowledge gaps that currently limit final conclusions on true diversity and plasticity of life-history traits in deep-sea benthos.
In summary, our investigation of functional traits of deep-sea epifauna from the CBL area generally supported our first hypothesis that small, non-sessile organisms are the most common, with a relatively equal proportion of direct and indirect (mostly through lecithotrophic larvae) development. The hypothesized predominance of deposit feeding, however, was not found in the observed species pool, though that feeding mode was more prominent in the proportional abundance-weighted data set. That modality is common in infaunal taxa, which we did not cover here (Gage and Tyler, 1991; Iken et al., 2001; Mamouridis et al., 2011). Our analysis of trait modalities highlights instead that there is no single way to live successfully under deep-sea conditions, but rather that, similar to shallower areas, multiple strategies are in fact viable.
Functional Differences Between Epifauna at Deep and Mid-Depth Stations
Trait Modality Composition at Mid-Depth and Deep Stations
Our hypothesis that functional traits of epibenthic communities would change with increasing depth in the CBL was generally confirmed. In particular, epifauna of deep stations reflecting more homogeneous habitat (Zhulay et al., 2019) had significantly higher proportional abundance of the modalities free-living, swimming, suspension feeders, opportunists/scavengers, internal fertilization and globulose compared to the mid-depth stations, which were characterized by complex habitat structure including ridges, a plateau with pockmarks, and rocks. In addition, our data also suggest that the increasing distance from the productive Chukchi shelf corresponded with spatial patterns of functionality in addition to the depth-related patterns.
The higher proportional abundance of modalities free-living and mobile/swimming at greater depths is consistent with generally decreasing food availability with increasing depth in deep-sea areas, both globally and in the Arctic (Thistle, 2003; Wiedmann et al., 2020). In the study region, this decrease is reflected in an annual POC flux on the adjacent Chukchi Sea shelf being at least an order of magnitude higher (4–166 g C m–2 year–1; Grebmeier et al., 2006) than in the Northwind Abyssal plain (0.24–0.32 g C m–2 year–1; Watanabe et al., 2014). Indeed, the deepest and most food limited basin station (station 7) had the highest proportional abundance of the modality swimming. Conversely, lower proportional abundance of free-living and mobile/swimming (i.e., more sessile, attached) modalities at mid-depth stations coincided with higher food availability at lower depths in general. This pattern was, however, not robust as trawl samples in fact showed a higher proportional abundance of the modality mobile at the mid-depth stations compared to the ROV data, where the modality crawling was most abundant. Mobile fauna is often caught with trawls (Brandt et al., 2016), while trawls can be less reliable in assessing density of some sessile fauna compared to ROV approach (Chimienti et al., 2018). The combination of both tools, thus, allowed us to get more comprehensive insights into the functional structure of benthic communities.
Suspension feeding was surprisingly more abundant at deeper stations in our study, where numerous persisting lebensspuren confirmed low bottom current velocity (Zhulay et al., 2019). The question arises as to what and how these organisms eat. In fact, suspension feeders are able to feed on a wide range of food items, ranging in size (from bacteria to zooplankton) and quality (Gili et al., 2001). Bacterial abundance and biomass do not decline with depth in the global ocean, thus becoming relatively more important in deeper layers (Rex et al., 2006; Wei et al., 2010) and, potentially, serving as food for benthic organisms in our study area. In addition, deep-water zooplankton communities in the Arctic Deep Water may provide a food source, though their abundances are low (less than 1 ind m–3) (Kosobokova and Hirche, 2000; Kosobokova et al., 2011). Adaptations that allow suspension feeders to maximize food capture even at slow current velocity might also play a role including generating feeding currents and associations with microbial communities (Gili et al., 2001; Siegl et al., 2008; Weisz et al., 2008). Finally, little maintenance energy was documented for Antarctic deep-water sponges (Gatti, 2002). Besides suspension-feeding, proportional abundance of opportunists/scavengers was also significantly higher at greater depths. These feeding strategies become increasingly more useful with depth as scavengers have an ability to detect sparse carrion across large distances (Premke et al., 2006), while opportunists can take advantage of almost whatever they come across in the food-poor environment (Drazen and Sutton, 2017). In turn, predation and deposit feeding were more common at the mid-depth stations, with deposit feeding being the dominant modality in the trawl samples. This might point to higher availability of deposited organic matter or prey at these mid-depth stations.
Proportional abundance of the modality internal fertilization was significantly higher in the deep compared to mid-depth stations. This pattern is consistent with previous studies where internal fertilization was common (Young, 2003). In an environment where chances of finding a mate are low, internal fertilization may have a higher success rate than external fertilization once a mate has indeed been found.
In addition to the depth pattern, substantial variability found in trait modality patterns was likely related to variable distance to the productive shelf rather than to depth alone. Evidence for this effect is for example the high proportional abundance of modalities tube-dwelling, sessile and deposit feeding at mid-depths stations at Northwind Ridge (stations 1, 2), which were associated with higher food input, likely from productive waters from the Chukchi shelf, as indicated by sediment pigment values and carbon content. In the same mid-depth range, higher proportional abundance of the modality predators farther north in the study area (stations 6, 9, and 10) was associated with a high amount of drop stones, where attached and upright predators took advantage of the presence of stones and elevated themselves to increase capture of prey. Additionally, mobile predators were occasionally observed in the vicinity to the stones, likely attracted by the enhanced amount of prey attached to the stones (Zhulay et al., 2019).
Functional Metrics and Ecosystem Vulnerability at Mid-Depth and Deep Stations
Both FD and FR indices showed changes with depth, where deep stations had lower FD and higher FR compared to the mid-depth stations, supporting our hypothesis. The depth-related FD trend was in agreement with results from the Arctic Nansen Basin (Degen, 2015), but contrary to a study from the Bering Sea (Liu et al., 2019), although the direct comparison of values obtained in different studies is no appropriate due to different authors using different traits or different numbers of traits in their calculations. Since FD indicates “the range of things organisms do in an ecosystem” (Petchey and Gaston, 2006; van der Linden et al., 2012), higher FD at the mid-depth stations indicates that these communities support more diverse ecological functions than those at greater depths. It seems likely that this pattern is linked to the more heterogeneous habitat structure at mid-depth stations providing more functional niche space for epifaunal organisms compared to the more homogeneous deeper abyssal plain. In contrast, higher FR at the deeper stations is, in turn, likely related to the homogeneity of the abyssal environment to which epifauna appear to have adapted by fewer and shared trait modalities. Low FR at mid-depth stations may render these areas less resilient to ongoing and future change and potential human use as functions may be lost when species loss occurs (Loreau, 2008; Van der Linden et al., 2016), a conclusion consistent with studies on Arctic benthic macrofauna (Kokarev et al., 2017; Liu et al., 2019; Sutton et al., 2020). In addition, modalities such as sessile, attached, and upright body form at these stations point to higher vulnerability of mid-depth epifauna to predation, disturbances or decreases in food availability (Degen and Faulwetter, 2019). The higher FR at deeper stations in addition to high proportional abundance of modalities mobile/swimming might indicate lower vulnerability to disturbances, higher flexibility to perturbation, and higher ability for dispersal after disturbance (Degen and Faulwetter, 2019). It is important to note, however, this conclusion is potentially biased by low faunal densities and low sampling effort. It is, therefore, premature to conclude that deeper communities in the CBL are resilient.
In support of our second hypothesis, data indicated an overall difference in functional structure of epifauna between mid-depth and deep stations in terms of trait composition, FD, and FR. In addition, depth, carbon content in sediments (reflecting food availability), and bottom temperature (reflecting difference in water masses) were the main predictors of the functional structure of epifaunal communities, which generally supported our second hypothesis.
Perspective and Outlook
Currently, we have limited ecological information on structure and function of deep-sea ecosystems in general, and in the Arctic Ocean in particular. Despite this lack, exploitation of Arctic deep-sea resources is now discussed widely in light of sea-ice cover decline. It is important to gain sufficient knowledge prior to any potential exploitation to assess and understand potential risk of human impacts and develop sustainable management strategies for possible resource use. The results generated in this study are, thus, very timely and can directly serve current assessments of biological and ecosystem resources and functions in the Central Arctic Ocean (CAO) by Scientific Experts on Fish Stocks of the Central Arctic Ocean (FisCAO) (fish stock assessment, Dupuis et al., 2019), the Working Group for Integrated Assessment of the Central Arctic Ocean (WGICA) (fisheries, ICES, 2020), and the International Union for Conservation of Nature (IUCN) (deep-sea mining plans and threats) (Cuyvers et al., 2018). Within FisCAO, the CBL is categorized as having fishable depth. In many places across the world’s oceans the documented negative effects from seabed fishing include reduced biodiversity, body size and biomass and particularly strong effects on fragile, upright and epifaunal taxa (Wassenberg et al., 2002; Blanchard et al., 2004; Jørgensen et al., 2019; Tiano et al., 2020), and shifts toward opportunistic species (Blanchard et al., 2004). Recovery from such and other impacts can be slow (Bergman et al., 2015), especially in high latitudes where recolonization is depressed by low temperature, long live cycles and high longevity (Al-Habahbeh et al., 2020). Indeed, the presence of upright body forms in the mostly sessile species visible in our imagery, in particular at the northern mid-depth stations, indicated a vulnerability of the system to trawling, as was also suggested for example for the Barents Sea shelf (Jørgensen et al., 2019). In addition, oil and gas reserves have also been quantified in this area (Bird et al., 2008). It has been made clear that the onset of such multiple pressures in deep sea habitats results in an urgent need for biodiversity and trait-based characterization of deep-sea fauna (Costa et al., 2020), a need we directly address in the present study. Our trait-based evaluation of CBL epifauna points to potentially high sensitivity of benthic community function to disturbances especially at mid-depths as indicated by high FD and low FR. In addition, rapid climate change may have a greater impact on sessile taxa reproducing with larvae of low dispersal ability than mobile species or species with high larval dispersal (Young et al., 1997). Thus, traits analysis can offer insight into resilience and recovery capacity of taxa after disturbance.
Our study forms the first step toward filling research gaps of Arctic deep-sea system functioning and vulnerability, though study limitations include the poorly known biology of many of the taxa encountered, as well as spatially limited sampling in a heterogeneous area. We strongly recommend further study that: (a) enhances spatial and temporal coverage; (b) uses traits generated from the actual species in question, as higher taxonomic levels contain different species, which may have distinctive trait modalities (Cochrane et al., 2012; van der Linden et al., 2012); (c) includes more traits, in particular those that might be helpful to indicate potential effects of direct human impact such as trawling or climate-change related impacts such as warming and acidification on organisms (e.g., fragility, temperature tolerance, life span, skeleton); and (d) generates trait information from the area of interest as, due to plasticity of organisms, modalities can change in response to local environmental settings (Bremner, 2005).
Data Availability Statement
The datasets generated and analyzed for this study can be found in the DataverseNO https://doi.org/10.18710/OGOAWN.
Author Contributions
KI and BB conceived the study idea. KI obtained the funding for fieldwork. KI and IZ conducted the fieldwork. IZ conducted the image analysis with input by BB, PR, and KI. IZ and RD compiled biological trait information with the input by BB and KI, and conducted the statistical analysis. IZ and BB conducted most writing with all authors participating in data interpretation and article preparation. All authors have approved the final article.
Funding
This work was funded through NOAA’s Office of Ocean Exploration (grant NA15OAR0110207 to KI), and we are particularly grateful to program manager Jeremy Potter for his support. IZ and BB acknowledge support by UiT The Arctic University of Norway including a mobility grant to IZ. Akvaplan-niva provided funding for PR and the Austrian Science Fund (FWF; T 801-B29) supported RD.
Conflict of Interest
The authors declare that the research was conducted in the absence of any commercial or financial relationships that could be construed as a potential conflict of interest.
Acknowledgments
We thank the crew, captain, and chief scientist of the USCGC HEALY for their support during the HEALY1601 cruise. We also thank L. Sloan, L. Sutton, A. Walker, A. Gastaldi, and K. Walker for assistance with epifaunal and fish sample collection and processing on-board, and L. Sutton for help in collecting trait information during the cruise and later in the process. J. Caba, J. Tripp, J. Aldridge, and T. Mikagawa were instrumental for the ROV sample collections. We are very grateful for the help of taxonomists in identification of epifaunal vouchers: S.D. Grebelny [Actiniaria, Zoological Institute Russian Academy of Sciences (ZIN RAS) in St. Petersburg, Russia], G. Hendler (Ophiuroidea, Natural History Museum of Los Angeles County, United States), S. Hourdez (Polynoidae, Station Biologique de Roscoff, France), K. Barwick (Aplacophora, Taxonomic Consultant, CA, United States), P. Valentich-Scott (Bivalvia, Santa Barbara Museum, United States), N. Foster (Gastropoda, NRF Taxonomic Services, United States), L. Cole (Ascidiacea, National Museum of Natural History, Washington, DC, United States), C. Mah (Asteroidea, Smithsonian Institution, Washington, DC, United States), O. Tendal (Porifera, Danish Museum of Natural History, Denmark), K. Coyle (Amphipoda, University of Alaska Fairbanks, United States), and M. Hoberg (Polychaeta, University of Alaska Fairbanks, United States). Thanks for help with identification of animals from images go to: J. Berge (Amphipoda, UiT The Arctic University of Norway), M. Bergmann (various organisms, Alfred Wegener Institute, Germany), B. Norcross (fish, University of Alaska Fairbanks, United States), B. Holladay (fish, University of Alaska Fairbanks, United States), and O. Karamushko (fish, Murmansk Marine Biological Institute, Russia). Thanks to S. Hardy and B. Jones (University of Alaska Fairbanks, United States) for benthic pigment data. We also thank the editor and two reviewers whose comments were important in improving this manuscript.
Supplementary Material
The Supplementary Material for this article can be found online at: https://www.frontiersin.org/articles/10.3389/fmars.2021.609956/full#supplementary-material
Supplementary Figure 1 | Schematic representation of the hypotheses, methods used to test the hypotheses and number of figures/tables representing results. Trait modality codes are abbreviated by two letter-one number combinations and explained in Table 2.
Supplementary Figure 2 | Results of the fuzzy correspondence analysis on the biological traits of epifauna from the Chukchi Borderland weighted by taxa proportional abundances from trawl samples, (A) ordination by stations, where numbers are station numbers, (B) ordination by traits, where trait modality codes are abbreviated by two letter-one number combinations and explained in Table 2. Red – mid-depth stations. Blue – deep stations.
Supplementary Figure 3 | Boxplots comparing modalities of different traits (weighted by proportional abundance) for mid-depth (in red) and the single deep station (grey line) based on trawl samples of epifauna from the Chukchi Borderland; (A) body form, (B) feeding habit, (C) reproduction, (D) living habit, and (E) adult movement. Note traits contributing most to variability among stations based on the Fuzzy Correspondence Analysis results are shown; modalities of these traits with low proportional abundance are not shown. Asterisks indicate significant difference between mid-depth and deep stations (p < 0.05, Kruskal–Wallis test). Trait modalities are defined in Table 2.
Supplementary Figure 4 | Boxplot of functional diversity indices for epifauna from the Chukchi Borderland, (A) Functional Diversity (FD) (represented by Rao’s Q) and (B) Functional Redundancy (FR) for mid-depth (red) and deep trawl samples of epifauna from the Chukchi Borderland. Note the scales of the y-axes are different.
Supplementary Figure 5 | Results of canonical correspondence analysis performed on the traits by stations matrix for trawl data of the epifauna from the Chukchi Borderland. Traits identified as most important in the FCA analysis are presented; (A) body form, (B) reproduction, (C) living habit, (D) adult movement, (E) feeding habit, and (F) substrate affinity. Passive environmental factors are overlain on the figure panels in gray color; environmental factors in red are those that were used to constrain the CCA. Mid-depth stations are present in red triangles and deep station is present in blue circles. Station numbers are identified in green on the last CCA plot. Trait modality codes are abbreviated by two letter-one number combinations and explained in Table 2.
Supplementary Table 1 | Literature and other sources used to collect trait information for epifauna sampled in the Arctic deep Chukchi Borderland (also available at Arctic Trait Database: https://www.univie.ac.at/arctictraits index.php).
Supplementary Table 2 | Traits by taxon matrix for epifauna sampled in the Arctic deep Chukchi Borderland using fuzzy coding.
Supplementary Table 3 | Presence and absence of epifaunal taxa at each station sampled in the Arctic deep-sea Chukchi Borderland with a remotely operated vehicle and a beam trawl.
Supplementary Table 4 | Proportional abundance of epifaunal taxa at each station sampled in the Arctic deep-sea Chukchi Borderland with a remotely operated vehicle.
Supplementary Table 5 | Proportional abundance of epifaunal taxa at each station sampled in the Arctic deep-sea Chukchi Borderland with a beam trawl.
Supplementary Table 6 | Traits by stations matrix based on presence/absence of epifaunal taxa sampled in the Arctic deep-sea Chukchi Borderland with a remotely operated vehicle and a beam trawl.
Supplementary Table 7 | Traits by stations matrix based on proportional abundance of epifaunal taxa sampled in the Arctic deep-sea Chukchi Borderland with a remotely operated vehicle.
Supplementary Table 8 | Traits by stations matrix based on proportional abundance of epifaunal taxa sampled in the Arctic deep-sea Chukchi Borderland with a beam trawl.
Supplementary Table 9 | Correlation ratios of each biological trait of epibenthos in the Arctic Chukchi Borderland with the first two axes of the fuzzy correspondence analysis for trawl samples (n = 6). Correlation ratios higher than 10% are in bold.
Supplementary Table 10 | Results of canonical correspondence analysis using Monte–Carlo permutation test, performed on a trait by station matrix for trawl samples of epibenthos in the Arctic Chukchi Borderland. Asterisks indicate significant results (p≤0.05).
Abbreviations
CBL, Chukchi Borderland; FD, functional diversity; FR, functional redundancy.
Footnotes
References
Abookire, A. A., and Rose, C. S. (2005). Modifications to a plumb staff beam trawl for sampling uneven, complex habitats. Fisheries Res. 71, 247–254. doi: 10.1016/j.fishres.2004.06.006
Al-Habahbeh, A. K., Kortsch, S., Bluhm, B. A., Beuchel, F., Gulliksen, B., Ballantine, C., et al. (2020). Arctic coastal benthos long-term responses to perturbations under climate warming. Philosop. Transac. R. Soc. 378:20190355.
Arar, E. J., and Collins, G. B. (1997). Method 445.0: In vitro determination of chlorophyll a and pheophytin a in marine and freshwater algae by fluorescence. Cincinnati: United States Environmental Protection Agency, Office of Research and Development, National Exposure Research Laboratory.
Arellano, S. M., and Young, C. M. (2009). Spawning, development, and the duration of larval life in a deep-sea cold-seep mussel. Biol. Bull. 216, 149–162.
Beauchard, O., Veríssimo, H., Queirós, A., and Herman, P. (2017). The use of multiple biological traits in marine community ecology and its potential in ecological indicator development. Ecol. Indicat. 76, 81–96. doi: 10.1016/j.ecolind.2017.01.011
Bennett, K. C., Young, C. M., and Emlet, R. B. (2012). Larval development and metamorphosis of the deep-sea cidaroid urchin Cidaris blakei. Biol. Bull. 222, 105–117.
Berecoechea, J. J., Brogger, M. I., and Penchaszadeh, P. E. (2017). New evidence of brooding in the deep-sea brittle star Astrotoma agassizii Lyman, 1876 from a South Western Atlantic Canyon. Deep Sea Res. Part I: Oceanogr. Res. Papers 127, 105–110.
Bergman, M. J., Ubels, S. M., Duineveld, G. C., and Meesters, E. W. (2015). Effects of a 5-year trawling ban on the local benthic community in a wind farm in the Dutch coastal zone. ICES J. Mar. Sci. 72, 962–972.
Bergmann, M., Dannheim, J., Bauerfeind, E., and Klages, M. (2009). Trophic relationships along a bathymetric gradient at the deep-sea observatory HAUSGARTEN. Deep Sea Res. Part I: Oceanogr. Res. Papers 56, 408–424. doi: 10.1016/j.dsr.2008.10.004
Billett, D., Bett, B., Rice, A., Thurston, M., Galéron, J., Sibuet, M., et al. (2001). Long-term change in the megabenthos of the Porcupine Abyssal Plain (NE Atlantic). Prog. Oceanogr. 50, 325–348.
Bird, K. J., Charpentier, R. R., Gautier, D. L., Houseknecht, D. W., Klett, T. R., Pitman, J. K., et al. (2008). Circum-Arctic resource appraisal: Estimates of undiscovered oil and gas north of the Arctic Circle. Washington, DC: U.S. Department of the Interior, U.S. Geological Survey. Available at: http://pubs.usgs.gov/fs/2008/3049/fs2008-3049.pdf
Blanchard, F., LeLoc’h, F., Hily, C., and Boucher, J. (2004). Fishing effects on diversity, size and community structure of the benthic invertebrate and fish megafauna on the Bay of Biscay coast of France. Mar. Ecol. Prog. Series 280, 249–260.
Bluhm, B. A., Ambrose, W. G., Bergmann, M., Clough, L. M., Gebruk, A. V., Hasemann, C., et al. (2011). Diversity of the arctic deep-sea benthos. Mar. Biodiv. 41, 87–107.
Bluhm, B., Kosobokova, K., and Carmack, E. (2015). A tale of two basins: An integrated physical and biological perspective of the deep Arctic Ocean. Prog. Oceanogr. 139, 89–121. doi: 10.1016/j.pocean.2015.07.011
Bluhm, B., MacDonald, I. R., Debenham, C., and Iken, K. (2005). Macro-and megabenthic communities in the high Arctic Canada Basin: initial findings. Polar Biol. 28, 218–231. doi: 10.1007/s00300-004-0675-4
Boetius, A., Albrecht, S., Bakker, K., Bienhold, C., Felden, J., Fernández-Méndez, M., et al. (2013). Export of algal biomass from the melting Arctic sea ice. Science 339, 1430–1432. doi: 10.1126/science.1231346
Bolam, S., Garcia, C., Eggleton, J., Kenny, A., Buhl-Mortensen, L., Gonzalez-Mirelis, G., et al. (2017). Differences in biological traits composition of benthic assemblages between unimpacted habitats. Mar. Env. Res. 126, 1–13. doi: 10.1016/j.marenvres.2017.01.004
Botta-Dukát, Z. (2005). Rao’s quadratic entropy as a measure of functional diversity based on multiple traits. J. Veget. Sci. 16, 533–540.
Bournaud, M., Cellot, B., Richoux, P., and Berrahou, A. (1996). Macroinvertebrate community structure and environmental characteristics along a large river: congruity of patterns for identification to species or family. J. North Am. Bentholog. Soc. 15, 232–253.
Bowman, M. F., and Bailey, R. C. (1997). Does taxonomic resolution affect the multivariate description of the structure of freshwater benthic macroinvertebrate communities? Can. J. Fisheries Aquat. Sci. 54, 1802–1807.
Brandner, M. M., Stübner, E., Reed, A. J., Gabrielsen, T. M., and Thatje, S. (2017). Seasonality of bivalve larvae within a high Arctic fjord. Polar Biol. 40, 263–276.
Brandt, A., Gutt, J., Hildebrandt, M., Pawlowski, J., Schwendner, J., Soltwedel, T., et al. (2016). Cutting the umbilical: new technological perspectives in benthic deep-sea research. J. Mar. Sci. Eng. 4:36. doi: 10.3390/jmse4020036
Bremner, J. (2005). Assessing ecological functioning in marine benthic communities. Newcastle upon Tyne NE1 7RU: Newcastle University.
Bremner, J. (2008). Species’ traits and ecological functioning in marine conservation and management. J. Exp. Mar. Biol. Ecol. 366, 37–47. doi: 10.1016/j.jembe.2008.07.007
Bremner, J., Frid, C., and Rogers, S. (2005). Biological traits of the North Sea benthos: does fishing affect benthic ecosystem function? Biology 2005:82651230.
Bremner, J., Rogers, S., and Frid, C. (2003). Assessing functional diversity in marine benthic ecosystems: a comparison of approaches. Mar. Ecol. Prog. Ser. 254, 11–25.
Bremner, J., Rogers, S., and Frid, C. (2006). Methods for describing ecological functioning of marine benthic assemblages using biological traits analysis (BTA). Ecol. Indicators 6, 609–622. doi: 10.1016/j.ecolind.2005.08.026
Buhl-Mortensen, L., Hodnesdal, H., and Thorsnes, T. (2015). The Norwegian Sea Floor: New Knowledge from MAREANO for Ecosystem-based Management.(Trondheim, TRH: Skipenes kommunikasjon AS), 192.
Carey, A. G. Jr. (1972). Food sources of sublittoral, bathyal and abyssal asteroids in the northeast Pacific Ocean. Ophelia 10, 35–47.
Cartes, J. E., Papiol, V., and Guijarro, B. (2008). The feeding and diet of the deep-sea shrimp Aristeus antennatus off the Balearic Islands (Western Mediterranean): Influence of environmental factors and relationship with the biological cycle. Prog. Oceanogr. 79, 37–54.
Charvet, S., Statzner, B., Usseglio−Polatera, P., and Dumont, B. (2000). Traits of benthic macroinvertebrates in semi−natural French streams: an initial application to biomonitoring in Europe. Freshwater Biol. 43, 277–296.
Chevenet, F., Doleadec, S., and Chessel, D. (1994). A fuzzy coding approach for the analysis of long−term ecological data. Freshwater Biol. 31, 295–309. doi: 10.1111/j.1365-2427.1994.tb01742.x
Chimienti, G., Angeletti, L., Rizzo, L., Tursi, A., and Mastrototaro, F. (2018). ROV vs trawling approaches in the study of benthic communities: the case of Pennatula rubra (Cnidaria: Pennatulacea). J. Mar. Biol. Assoc. U K 98, 1859–1869. doi: 10.1017/S0025315418000851
Clark, M. R., Rowden, A. A., Schlacher, T., Williams, A., Consalvey, M., Stocks, K. I., et al. (2010). The ecology of seamounts: structure, function, and human impacts. Ann. Rev. Mar. Sci. 2, 253–278. doi: 10.1146/annurev-marine-120308-081109
Cochrane, S., Pearson, T., Greenacre, M., Costelloe, J., Ellingsen, I., Dahle, S., et al. (2012). Benthic fauna and functional traits along a Polar Front transect in the Barents Sea–Advancing tools for ecosystem-scale assessments. J. Mar. Syst. 94, 204–217. doi: 10.1016/j.jmarsys.2011.12.001
Conti, L., Schmidt-Kloiber, A., Grenouillet, G., and Graf, W. (2014). A trait-based approach to assess the vulnerability of European aquatic insects to climate change. Hydrobiologia 721, 297–315. doi: 10.1007/s10750-013-1690-7
Corlett, W. B., and Pickart, R. S. (2017). The Chukchi slope current. Prog. Oceanogr. 153, 50–65. doi: 10.1016/j.pocean.2017.04.005
Costa, C., Fanelli, E., Marini, S., Danovaro, R., and Aguzzi, J. (2020). Global Deep-Sea Biodiversity Research Trends Highlighted by Science Mapping Approach. Front. Mar. Sci. 7:384. doi: 10.3389/fmars.2020.00384
Costello, M. J., Claus, S., Dekeyzer, S., Vandepitte, L., Tuama, E. O., Lear, D., et al. (2015). Biological and ecological traits of marine species. PeerJ. 3:e1201.
Cuyvers, L., Berry, W., Gjerde, K., Thiele, T., and Wilhem, C. (2018). Deep seabed mining: a rising environmental challenge. Switzerland: IUCN and Gallifrey Foundation, doi: 10.2305/IUCN.CH.2018.16.en
Danovaro, R., Gambi, C., Dell’Anno, A., Corinaldesi, C., Fraschetti, S., Vanreusel, A., et al. (2008). Exponential decline of deep-sea ecosystem functioning linked to benthic biodiversity loss. Curr. Biol. 18, 1–8. doi: 10.1016/j.cub.2007.11.056
Danovaro, R., Snelgrove, P. V., and Tyler, P. (2014). Challenging the paradigms of deep-sea ecology. Trends Ecol. Evol. 29, 465–475. doi: 10.1016/j.tree.2014.06.002
de Bello, F. D., Lepš, J., Lavorel, S., and Moretti, M. (2007). Importance of species abundance for assessment of trait composition: an example based on pollinator communities. Comm. Ecol. 8, 163–170. doi: 10.1556/ComEc.8.2007.2.3
Degen, R. (2015). The Future Arctic Biosphere: Environmental Drivers of Change in Arctic Benthic Biota. Germany: Universität Bremen.
Degen, R., and Faulwetter, S. (2019). The Arctic Traits Database–a repository of Arctic benthic invertebrate traits. Earth Syst. Sci. Data 11, 301–322. doi: 10.5194/essd-11-301-2019
Degen, R., Aune, M., Bluhm, B. A., Cassidy, C., Kêdra, M., Kraan, C., et al. (2018). Trait-based approaches in rapidly changing ecosystems: A roadmap to the future polar oceans. Ecol. Indicat. 91, 722–736. doi: 10.1016/j.ecolind.2018.04.050
Dolédec, S., Statzner, B., and Frainay, V. (1998). Accurate description of functional community structure: identifying stream invertebrates to species-level. Bull. North Am. Bentholog. Soc. 15, 154–155.
Dorgelo, J., and Leonards, P. E. (2001). Relationship between C/N ratio of food types and growth rate in the snail Potamopyrgus jenkinsi (EA Smith). J. North Am. Bentholog. Soc. 20, 60–67.
Dray, S., and Dufour, A.-B. (2007). The ade4 package: implementing the duality diagram for ecologists. J. Statist. Soft. 22, 1–20.
Drazen, J. C., and Sutton, T. T. (2017). Dining in the deep: the feeding ecology of deep-sea fishes. Annu. Rev. Mar. Sci. 9, 337–366. doi: 10.1146/annurev-marine-010816-060543
Dupuis, A., Majewski, A., Keatley, B., Hedges, K., Gold, M., Wang, L., et al. (2019). “Final Report of the Fifth Meeting of Scientific Experts on Fish Stocks in the Central Arctic Ocean (FiSCAO)”. Available at: https://epic.awi.de/id/eprint/52359/
Emerson, S. (2014). Annual net community production and the biological carbon flux in the ocean. Glob. Biogeochem. Cycles 28, 14–28. doi: 10.1002/2013GB004680
Ershova, E., Descoteaux, R., Wangensteen, O., Iken, K., Hopcroft, R., Smoot, C., et al. (2019). Diversity and distribution of meroplanktonic larvae in the Pacific Arctic and connectivity with adult benthic invertebrate communities. Front. Mar. Sci. 6:490. doi: 10.3389/fmars.2019.00490
Fabiano, M., Pusceddu, A., Dell’Anno, A., Armeni, M., Vanucci, S., Lampitt, R., et al. (2001). Fluxes of phytopigments and labile organic matter to the deep ocean in the NE Atlantic Ocean. Prog. Oceanogr. 50, 89–104.
Faulwetter, M. S., Markantonatou, M. V., Pavloudi, M. C., Papageorgiou, N., Keklikoglou, M. K., Chatzinikolaou, E., et al. (2014). Polytraits: A database on biological traits of marine polychaetes. Biodiv. Data J. 2:e1024.
Faulwetter, S., Papageorgiou, N., Koulouri, P., Fanini, L., Chatzinikolaou, E., Markantonatou, V., et al. (2015). Resistance of polychaete species and trait patterns to simulated species loss in coastal lagoons. J. Sea Res. 98, 73–82.
Fetzer, I., and Arntz, W. E. (2008). Reproductive strategies of benthic invertebrates in the Kara Sea (Russian Arctic): adaptation of reproduction modes to cold water. Mar. Ecol. Prog. Series 356, 189–202. doi: 10.3354/meps07271
Fischer, G., Ratmeyer, V., and Wefer, G. (2000). Organic carbon fluxes in the Atlantic and the Southern Ocean: relationship to primary production compiled from satellite radiometer data. Deep Sea Res. Part II: Topic. Stud. Oceanogr. 47, 1961–1997.
Gage, J. D., and Tyler, P. A. (1991). Deep-sea biology: a natural history of organisms at the deep-sea floor. Cambridge, MA: Cambridge University Press.
Gatti, S. (2002). The rôle of sponges in high-Antarctic carbon and silicon cycling-a modelling approach= Die Rolle der Schwämme im hochantarktischen Kohlenstoff-und Silikatkreislauf-ein Modellierungsansatz. Berichte zur Polar-und Meeresforschung 434:124.
Gili, J.-M., Coma, R., Orejas, C., López-González, P. J., and Zabala, M. (2001). Are Antarctic suspension-feeding communities different from those elsewhere in the world? Polar Biol. 24, 473–485. doi: 10.1007/s003000100257
Godefroy, N., Le Goff, E., Martinand-Mari, C., Belkhir, K., Vacelet, J., and Baghdiguian, S. (2019). Sponge digestive system diversity and evolution: filter feeding to carnivory. Cell Tissue Res. 2019, 1–11.
Grebmeier, J. M., Cooper, L. W., Feder, H. M., and Sirenko, B. I. (2006). Ecosystem dynamics of the Pacific-influenced northern Bering and Chukchi Seas in the Amerasian Arctic. Prog. Oceanogr. 71, 331–361. doi: 10.1016/j.pocean.2006.10.001
ICES (2020). ICES/PICES/PAME Working Group on Integrated Ecosystem Assessment (IEA) for the Central Arctic Ocean (WGICA). Chennai: ICES.
Iken, K., Bluhm, B., and Dunton, K. (2010). Benthic food-web structure under differing water mass properties in the southern Chukchi Sea. Deep Sea Res. Part II: Topic. Stud. Oceanogr. 57, 71–85.
Iken, K., Bluhm, B., and Gradinger, R. (2005). Food web structure in the high Arctic Canada Basin: evidence from δ 13 C and δ 15 N analysis. Polar Biol. 28, 238–249. doi: 10.1007/s00300-004-0669-2
Iken, K., Brey, T., Wand, U., Voigt, J., and Junghans, P. (2001). Food web structure of the benthic community at the Porcupine Abyssal Plain (NE Atlantic): a stable isotope analysis. Prog. Oceanogr. 50, 383–405. doi: 10.1016/S0079-6611(01)00062-3
Jakobsson, M., Polyak, L., Edwards, M., Kleman, J., and Coakley, B. (2008). Glacial geomorphology of the central Arctic Ocean: the Chukchi Borderland and the Lomonosov Ridge. Earth Surface Proc. Landforms 33, 526–545. doi: 10.1002/esp.1667
Jeffrey, S., and Welschmeyer, N. (1997). Appendix F: Spectrophotometric and fluorometric equations in common use in oceanography. Phytoplankton Pigments in Oceanography. Monographs on Oceanographic Methodology. Paris: UNESCO Publishing, 597–615.
Jørgensen, L. L., Primicerio, R., Ingvaldsen, R. B., Fossheim, M., Strelkova, N., Thangstad, T. H., et al. (2019). Impact of multiple stressors on sea bed fauna in a warming Arctic. Mar. Ecol. Prog. Series 608, 1–12. doi: 10.3354/meps12803
Kaariainen, J. I., and Bett, B. J. (2006). Evidence for benthic body size miniaturization in the deep sea. J. Mar. Biol. Assoc. U K 86, 1339–1345. doi: 10.1017/S0025315406014366
Karl, D., Christian, J., Dore, J., Hebel, D., Letelier, R., Tupas, L., et al. (1996). Seasonal and interannual variability in primary production and particle flux at Station ALOHA. Deep Sea Res. Part II: Topic. Stud. Oceanogr. 43, 539–568.
Kersten, O., Vetter, E. W., Jungbluth, M. J., Smith, C. R., and Goetze, E. (2019). Larval assemblages over the abyssal plain in the Pacific are highly diverse and spatially patchy. PeerJ. 7:e7691. doi: 10.7717/peerj.7691
Klages, M., Vopel, K., Bluhm, H., Brey, T., Soltwedel, T., and Arntz, W. E. (2001). Deep-sea food falls: first observation of a natural event in the Arctic Ocean. Polar Biol. 24, 292–295. doi: 10.1007/s003000000199
Klimpel, S., Palm, H. W., Busch, M. W., Kellermanns, E., and Rückert, S. (2006). Fish parasites in the Arctic deep-sea: Poor diversity in pelagic fish species vs. heavy parasite load in a demersal fish. Deep Sea Res. Part I: Oceanogr. Res. Papers 53, 1167–1181. doi: 10.1016/j.dsr.2006.05.009
Køie, M. (2000). Metazoan parasites of teleost fishes from Atlantic waters off the Faroe Islands. Ophelia 52, 25–44. doi: 10.1080/00785236.1999.10409417
Kokarev, V., Vedenin, A., Basin, A., and Azovsky, A. (2017). Taxonomic and functional patterns of macrobenthic communities on a high-Arctic shelf: a case study from the Laptev Sea. J. Sea Res. 129, 61–69. doi: 10.1016/j.seares.2017.08.011
Kosobokova, K. N., Hopcroft, R. R., and Hirche, H.-J. (2011). Patterns of zooplankton diversity through the depths of the Arctic’s central basins. Mar. Biodiver. 41, 29–50. doi: 10.1007/s12526-010-0057-9
Kosobokova, K., and Hirche, H.-J. (2000). Zooplankton distribution across the Lomonosov Ridge, Arctic Ocean: species inventory, biomass and vertical structure. Deep Sea Res. Part I: Oceanogr. Res. Papers 47, 2029–2060. doi: 10.1016/S0967-0637(00)00015-7
Kröncke, I. (1998). Macrofauna communities in the Amundsen Basin, at the Morris Jesup Rise and at the Yermak Plateau (Eurasian Arctic Ocean). Polar Biol. 19, 383–392.
Kröncke, I., and Türkay, M. (2003). Structural and functional aspects of the benthic communities in the deep Angola Basin. Mar. Ecol. Prog. Series 260, 43–53. doi: 10.3354/meps260043
Krumhansl, K., Jamieson, R., and Krkosek, W. (2016). Using species traits to assess human impacts on near shore benthic ecosystems in the Canadian Arctic. Ecol. Indicat. 60, 495–502. doi: 10.1021/es503330n
Kuklinski, P., Berge, J., McFadden, L., Dmoch, K., Zajaczkowski, M., Nygård, H., et al. (2013). Seasonality of occurrence and recruitment of Arctic marine benthic invertebrate larvae in relation to environmental variables. Polar Biol. 36, 549–560. doi: 10.1007/s00300-012-1283-3
Lauretta, D., Vidos, C., Martinez, M. I., and Penchaszadeh, P. E. (2020). Brooding in the deep-sea sea anemone Actinostola crassicornis (Hertwig, 1882)(Cnidaria: Anthozoa: Actiniaria) from the southwestern Atlantic Ocean. Polar Biol. 43, 1353–1361.
Leu, E., Mundy, C., Assmy, P., Campbell, K., Gabrielsen, T., Gosselin, M., et al. (2015). Arctic spring awakening–Steering principles behind the phenology of vernal ice algal blooms. Prog. Oceanogr. 139, 151–170. doi: 10.1016/j.pocean.2015.07.012
Levin, L. A., and Gooday, A. J. (2003). The deep Atlantic ocean. Ecosyst. World 2003, 111–178. doi: 10.1016/b978-0-12-409548-9.09071-0
Levin, L. A., and Le Bris, N. (2015). The deep ocean under climate change. Science 350, 766–768. doi: 10.1126/science.aad0126
Levin, L. A., Etter, R. J., Rex, M. A., Gooday, A. J., Smith, C. R., Pineda, J., et al. (2001). Environmental influences on regional deep-sea species diversity. Ann. Rev. Ecol. Syst. 32, 51–93. doi: 10.1146/annurev.ecolsys.32.081501.114002
Liu, K., Lin, H., He, X., Huang, Y., Li, Z., Lin, J., et al. (2019). Functional trait composition and diversity patterns of marine macrobenthos across the Arctic Bering Sea. Ecol. Indic. 102, 673–685. doi: 10.1016/j.ecolind.2019.03.029
Loreau, M. (2008). Biodiversity and ecosystem functioning: the mystery of the deep sea. Curr. Biol. 18, R126–R128. doi: 10.1016/j.cub.2007.11.060
MacDonald, I. R., Bluhm, B. A., Iken, K., Gagaev, S., and Strong, S. (2010). Benthic macrofauna and megafauna assemblages in the Arctic deep-sea Canada Basin. Deep Sea Res. Part II: Topic. Stud. Oceanogr. 57, 136–152. doi: 10.1016/j.dsr2.2009.08.012
Mamouridis, V., Cartes, J. E., Parra, S., Fanelli, E., and Salinas, J. S. (2011). A temporal analysis on the dynamics of deep-sea macrofauna: Influence of environmental variability off Catalonia coasts (western Mediterranean). Deep Sea Res. Part I: Oceanogr. Res. Papers 58, 323–337.
Mantelatto, F. L., O’Brien, J. J., and Biagi, R. (2003). Parasites and symbionts of crabs from Ubatuba Bay, Sao Paulo state, Brazil. Compar. Parasitol. 70, 211–214. doi: 10.1654/4066
Martinez, M. I., and Penchaszadeh, P. E. (2017). A new species of brooding Psolidae (Echinodermata: Holothuroidea) from deep-sea off Argentina, Southwestern Atlantic Ocean. Deep Sea Res. Part II: Topic. Stud. Oceanogr. 146, 13–17.
Martini, S., Larras, F., Boyé, A., Faure, E., Aberle, N., Archambault, P., et al. (2020a). Functional trait−based approaches as a common framework for aquatic ecologists. Limnol. Oceanogr. 9999, 1–30. doi: 10.1002/lno.11655
Martini, S., Schultz, D. T., Lundsten, L., and Haddock, S. H. (2020b). Bioluminescence in an Undescribed Species of Carnivorous Sponge (Cladorhizidae) From the Deep Sea. Front. Mar. Sci. 7:1041.
Mayer, L. A., Armstrong, A., Calder, B., and Gardner, J. (2010). Sea floor mapping in the Arctic: support for a potential US extended continental shelf. Int. Hydrogr. Rev. 3, 14–23. doi: 10.1163/ej.9789004177567.i-594.23
McLaughlin, F., Carmack, E., Macdonald, R., Melling, H., Swift, J., Wheeler, P., et al. (2004). The joint roles of Pacific and Atlantic-origin waters in the Canada Basin, 1997–1998. Deep Sea Res. Part I: Oceanogr. Res. Papers 51, 107–128. doi: 10.1016/j.dsr.2003.09.010
Mercier, A., and Hamel, J.-F. (2008). Depth-related shift in life history strategies of a brooding and broadcasting deep-sea asteroid. Mar. Biol. 156, 205–223.
Mileikovsky, S. (1971). Types of larval development in marine bottom invertebrates, their distribution and ecological significance: a re-evaluation. Mar. Biol. 10, 193–213. doi: 10.1007/bf00352809
Montgomery, E. M., Hamel, J.-F., and Mercier, A. (2017). The deep-sea neogastropod Buccinum scalariforme: Reproduction, development and growth. Deep Sea Res. Part I: Oceanogr. Res. Papers 119, 24–33.
Morton, B. (2016). The biology and functional morphology of the predatory septibranch Cardiomya costellata (Deshayes, 1833)(Bivalvia: Anomalodesmata: Cuspidariidae) from the Açores: survival at the edge. J. Mar. Biol. Assoc. U K 96, 1347–1361.
Morton, B., and Machado, F. M. (2019). Predatory marine bivalves: A review. Adv. Mar. Biol. 84, 1–98.
Naeem, S., Chapin Iii, F., Costanza, R., Ehrlich, P. R., Golley, F. B., Hooper, D. U., et al. (1999). Biodiversity and ecosystem functioning: maintaining natural life support processes. Issues Ecol. 4:001611. doi: 10.1525/california/9780520268357.003.0016
Oksanen, J., Blanchet, F. G., Kindt, R., Legendre, P., Minchin, P. R., O’hara, R., et al. (2013). Package ‘vegan’. Community ecology package, version 2(9).
Oug, E., Fleddum, A., Rygg, B., and Olsgard, F. (2012). Biological traits analyses in the study of pollution gradients and ecological functioning of marine soft bottom species assemblages in a fjord ecosystem. J. Exp. Mar. Biol. Ecol. 432, 94–105. doi: 10.1016/j.jembe.2012.07.019
Pearse, J. S. (1994). Cold-water echinoderms break Thorson’s rule. Reproduction, larval biology and recruitment in the deep-sea benthos, eds. C. M. Young and K. J. Eckelbarger, New York, NY: Columbia University Press, 27–43.
Pearse, J. S., and Lockhart, S. J. (2004). Reproduction in cold water: paradigm changes in the 20th century and a role for cidaroid sea urchins. Deep Sea Res. Part II: Topic. Stud. Oceanogr. 51, 1533–1549. doi: 10.1016/j.dsr2.2004.06.023
Petchey, O. L., and Gaston, K. J. (2006). Functional diversity: back to basics and looking forward. Ecol. Lett. 9, 741–758. doi: 10.1111/j.1461-0248.2006.00924.x
Pomerleau, C., Sastri, A. R., and Beisner, B. E. (2015). Evaluation of functional trait diversity for marine zooplankton communities in the Northeast subarctic Pacific Ocean. J. Plankt. Res. 37, 712–726. doi: 10.1093/plankt/fbv045
Premke, K., Klages, M., and Arntz, W. E. (2006). Aggregations of Arctic deep-sea scavengers at large food falls: temporal distribution, consumption rates and population structure. Mar. Ecol. Prog. Series 325, 121–135. doi: 10.3354/meps325121
R Core Team (2017). R: A language and environment for statistical computing (Version 3.4. 4)[Computer software]. Vienna: R Foundation for Statistical Computing.
Ramirez-Llodra, E., Brandt, A., Danovaro, R., De Mol, B., Escobar, E., German, C., et al. (2010). Deep, diverse and definitely different: unique attributes of the world’s largest ecosystem. Biogeosciences 7, 2851–2899. doi: 10.5194/bg-7-2851-2010
Ramirez-Llodra, E., Tyler, P. A., Baker, M. C., Bergstad, O. A., Clark, M. R., Escobar, E., et al. (2011). Man and the last great wilderness: human impact on the deep sea. PLoS One 6:0022588. doi: 10.1371/journal.pone.0022588
Rand, K., Logerwell, E., Bluhm, B., Chenelot, H., Danielson, S., Iken, K., et al. (2018). Using biological traits and environmental variables to characterize two Arctic epibenthic invertebrate communities in and adjacent to Barrow Canyon. Deep Sea Res. Part II: Topic. Stud. Oceanogr. 152, 154–169. doi: 10.1016/j.dsr2.2017.07.015
Randelhoff, A., and Guthrie, J. D. (2016). Regional patterns in current and future export production in the central Arctic Ocean quantified from nitrate fluxes. Geophys. Res. Lett. 43, 8600–8608. doi: 10.1002/2016gl070252
Rao, C. R. (1982). Diversity and dissimilarity coefficients: a unified approach. Theor. Populat. Biol. 21, 24–43.
Rasband, W. (2009). ImageJ; US National Institutes of Health. Bethesda, MD: World Wide Web, 1997–2006.
Ravichandran, S., Rameshkumar, G., and Kumaravel, K. (2009). Variation in the morphological features of isopod fish parasites. World J. Fish Mar. Sci. 1, 137–140. doi: 10.1007/s12639-010-0014-3
Renaud, P. E., Ambrose, W. G. Jr., Vanreusel, A., and Clough, L. M. (2006). Nematode and macrofaunal diversity in central Arctic Ocean benthos. J. Exp. Mar. Biol. Ecol. 330, 297–306. doi: 10.1016/j.jembe.2005.12.035
Rex, M. A., and Etter, R. J. (1998). Bathymetric patterns of body size: implications for deep-sea biodiversity. Deep Sea Res. Part II: Topic. Stud. Oceanogr. 45, 103–127. doi: 10.1016/s0967-0645(97)00082-9
Rex, M. A., and Etter, R. J. (2010). Deep-sea biodiversity: pattern and scale. Cambridge, MA: Harvard University Press.
Rex, M. A., Etter, R. J., and Nimeskern, P. W. Jr. (1990). Density estimates for deep-sea gastropod assemblages. Deep Sea Res. Part A. Oceanogr. Res. Papers 37, 555–569.
Rex, M. A., Etter, R. J., Clain, A. J., and Hill, M. S. (1999). Bathymetric patterns of body size in deep−sea gastropods. Evolution 53, 1298–1301. doi: 10.1111/j.1558-5646.1999.tb04543.x
Rex, M. A., Etter, R. J., Morris, J. S., Crouse, J., McClain, C. R., Johnson, N. A., et al. (2006). Global bathymetric patterns of standing stock and body size in the deep-sea benthos. Mar. Ecol. Prog. Series 317, 1–8. doi: 10.3354/meps317001
Ricotta, C., de Bello, F., Moretti, M., Caccianiga, M., Cerabolini, B. E., and Pavoine, S. (2016). Measuring the functional redundancy of biological communities: a quantitative guide. Methods Ecol. Evol. 7, 1386–1395. doi: 10.1111/2041-210x.12604
Rivadeneira, P. R., Martinez, M. I., Penchaszadeh, P. E., and Brogger, M. I. (2020). Reproduction and description of a new genus and species of deep-sea asteriid sea star (Echinodermata; Asteroidea) from the southwestern Atlantic. Deep Sea Res. Part I: Oceanogr. Res. Papers 163:103348.
Ruhl, H. A. (2007). Abundance and size distribution dynamics of abyssal epibenthic megafauna in the northeast Pacific. Ecology 88, 1250–1262. doi: 10.1890/06-0890
Ruhl, H. A., and Smith, K. L. (2004). Shifts in deep-sea community structure linked to climate and food supply. Science 305, 513–515. doi: 10.1126/science.1099759
Rybakova, E., Kremenetskaia, A., Vedenin, A., Boetius, A., and Gebruk, A. (2019). Deep-sea megabenthos communities of the Eurasian Central Arctic are influenced by ice-cover and sea-ice algal falls. PloS One 14:7. doi: 10.1101/515015
Scheltema, R. S., and Williams, I. P. (2009). Reproduction among protobranch bivalves of the family Nuculidae from sublittoral, bathyal, and abyssal depths off the New England coast of North America. Deep Sea Res. Part II: Topic. Stud. Oceanogr. 56, 1835–1846.
Schlüter, M., and Rachor, E. (2001). Meroplankton distribution in the central Barents Sea in relation to local oceanographic patterns. Polar Biol. 24, 582–592. doi: 10.1007/s003000100255
Shirayama, Y., and Horikoshi, M. (1989). Comparison of the benthic size structure between sublittoral, upper−slope and deep−sea areas of the Western Pacific. Int. Revue der gesamten Hydrobiol. Hydrogr. 74, 1–13. doi: 10.1002/iroh.19890740102
Siegl, A., Bayer, K., Kozytska, S., Hentschel, U., and Schmitt, S. (2008). Sponges and Microbes-New frontiers in an ancient symbiosis. Vie et Milieu 58, 165–174.
Smith, C. R., Levin, L. A., Koslow, A., Tyler, P. A., and Glover, A. G. (2008). The near future of the deep seafloor ecosystems. Cambrige, MA: Cambrige University Press.
Soetaert, K., Muthumbi, A., and Heip, C. (2002). Size and shape of ocean margin nematodes: morphological diversity and depth-related patterns. Mar. Ecol. Prog. Ser. 242, 179–193. doi: 10.3354/meps242179
Soltwedel, T., Pfannkuche, O., and Thiel, H. (1996). The size structure of deep-sea meiobenthos in the north-eastern Atlantic: nematode size spectra in relation to environmental variables. J. Mar. Biol. Assoc. U K 76, 327–344. doi: 10.1017/s0025315400030587
Steele, M., Morison, J., Ermold, W., Rigor, I., Ortmeyer, M., and Shimada, K. (2004). Circulation of summer Pacific halocline water in the Arctic Ocean. J. Geophys. Res. Oceans 109:C02027. doi: 10.1029/2003jc002009
Stuart, C. T., Rex, M., and Etter, R. J. (2003). Large-scale spatial and temporal patterns of deep-sea benthic species diversity. Ecosyst. World 2003, 295–312. doi: 10.1017/cbo9780511752360.006
Stübner, E., Søreide, J., Reigstad, M., Marquardt, M., and Blachowiak-Samolyk, K. (2016). Year-round meroplankton dynamics in high-Arctic Svalbard. J. Plankton Res. 38, 522–536. doi: 10.1093/plankt/fbv124
Sutton, L., Iken, K., Bluhm, B., and Mueter, F. (2020). Comparison of functional diversity of two Alaskan Arctic shelf epibenthic communities. Mar. Ecosyst. Prog. Series 651, 1–21. doi: 10.3354/meps13478
Sweetman, A. K., Thurber, A. R., Smith, C. R., Levin, L. A., Mora, C., Wei, C.-L., et al. (2017). Major impacts of climate change on deep-sea benthic ecosystems. Elem. Sci. Anth. 5:4. doi: 10.1525/elementa.203
Taylor, J., Krumpen, T., Soltwedel, T., Gutt, J., and Bergmann, M. (2016). Regional-and local-scale variations in benthic megafaunal composition at the Arctic deep-sea observatory HAUSGARTEN. Deep Sea Res. Part I: Oceanogr. Res. Papers 108, 58–72. doi: 10.1016/j.dsr.2015.12.009
Tekman, M. B., Krumpen, T., and Bergmann, M. (2017). Marine litter on deep Arctic seafloor continues to increase and spreads to the North at the HAUSGARTEN observatory. Deep Sea Res. Part I: Oceanogr. Res. Papers 120, 88–99. doi: 10.1016/j.dsr.2016.12.011
Thiel, H. (1975). The size structure of the deep-sea benthos. Int. Revue der Gesamten Hydrobiol. 60, 575–606.
Thistle, D. (2003). The deep-sea floor: an overview ecosystems of the deep ocean. Ecosyst. World 2003:28.
Thorson, G. (1950). Reproductive and larval ecology of marine bottom invertebrates. Biol. Rev. 25, 1–45.
Thurber, A. R., Sweetman, A. K., Narayanaswamy, B. E., Jones, D. O., Ingels, J., and Hansman, R. (2014). Ecosystem function and services provided by the deep sea. Biogeosciences 11, 3941–3963. doi: 10.5194/bg-11-3941-2014
Tiano, J. C., van der Reijden, K. J., O’Flynn, S., Beauchard, O., van der Ree, S., van der Wees, J., et al. (2020). Experimental bottom trawling finds resilience in large-bodied infauna but vulnerability for epifauna and juveniles in the Frisian Front. Mar. Env. Res. 2020:104964.
Timofeev, S. (2001). Bergmann’s principle and deep-water gigantism in marine crustaceans. Biol. Bull. Russ. Acad. Sci. 28, 646–650.
Usseglio-Polatera, P., Bournaud, M., Richoux, P., and Tachet, H. (2000). Biological and ecological traits of benthic freshwater macroinvertebrates: relationships and definition of groups with similar traits. Freshwater Biol. 43, 175–205. doi: 10.1046/j.1365-2427.2000.00535.x
Vacelet, J. (2006). New carnivorous sponges (Porifera, Poecilosclerida) collected from manned submersibles in the deep Pacific. Zool. J. Linnean Soc. 148, 553–584. doi: 10.1111/j.1096-3642.2006.00234.x
Vacelet, J. (2008). A new genus of carnivorous sponges (Porifera: Poecilosclerida, Cladorhizidae) from the deep NE Pacific, and remarks on the genus Neocladia. Zootaxa 1752, 57–65.
Van der Linden, P., Marchini, A., Dolbeth, M., Patrício, J., Veríssimo, H., and Marques, J. (2016). The performance of trait-based indices in an estuarine environment. Ecol. Indicat. 61, 378–389. doi: 10.1016/j.ecolind.2015.09.039
van der Linden, P., Patrício, J., Marchini, A., Cid, N., Neto, J. M., and Marques, J. C. (2012). A biological trait approach to assess the functional composition of subtidal benthic communities in an estuarine ecosystem. Ecol. Indicat. 20, 121–133. doi: 10.1016/j.ecolind.2012.02.004
Vogel, S. (1996). Life in moving fluids: the physical biology of flow. Princeton, NJ: Princeton University Press.
Wassenberg, T., Dews, G., and Cook, S. (2002). The impact of fish trawls on megabenthos (sponges) on the north-west shelf of Australia. Fisheries Res. 58, 141–151.
Watanabe, E., Onodera, J., Harada, N., Honda, M. C., Kimoto, K., Kikuchi, T., et al. (2014). Enhanced role of eddies in the Arctic marine biological pump. Nat. Commun. 5:3950. doi: 10.1038/ncomms4950
Wei, C.-L., Rowe, G. T., Escobar-Briones, E., Boetius, A., Soltwedel, T., Caley, M. J., et al. (2010). Global patterns and predictions of seafloor biomass using random forests. PLoS One 5:e15323. doi: 10.1371/journal.pone.0015323
Weisz, J. B., Lindquist, N., and Martens, C. S. (2008). Do associated microbial abundances impact marine demosponge pumping rates and tissue densities? Oecologia 155, 367–376. doi: 10.1007/s00442-007-0910-0
Wiedmann, I., Ershova, E., Bluhm, B. A., Nöthig, E.-M., Gradinger, R. R., Kosobokova, K., et al. (2020). What Feeds the Benthos in the Arctic Basins? Assembling a Carbon Budget for the Deep Arctic Ocean. Front. Mar. Sci. 7:224. doi: 10.3389/fmars.2020.00224
Wieking, G., and Kröncke, I. (2003). Macrofauna communities of the Dogger Bank (central North Sea) in the late 1990s: spatial distribution, species composition and trophic structure. Helgoland Mar. Res. 57, 34–46. doi: 10.1007/s10152-002-0130-2
Woodgate, R. (2013). Arctic Ocean circulation: Going around at the top of the world. Nat. Educ. Knowledge 4:8.
Woodgate, R. A., Aagaard, K., Swift, J. H., Smethie, W. M., and Falkner, K. K. (2007). Atlantic water circulation over the Mendeleev Ridge and Chukchi Borderland from thermohaline intrusions and water mass properties. J. Geophys. Res. Oceans 112:C02005. doi: 10.1029/2005JC003416
Woodgate, R. A., and Aagaard, K. (2005). Revising the Bering Strait freshwater flux into the Arctic Ocean. Geophys. Res. Lett. 32:L02602. doi: 10.1029/2004GL021747
Young, C. M. (2003). Reproduction, development and life-history traits. Ecosyst. World 2003, 381–426.
Young, C. M., Sewell, M. A., Tyler, P. A., and Metaxas, A. (1997). Biogeographic and bathymetric ranges of Atlantic deep-sea echinoderms and ascidians: the role of larval dispersal. Biodiv. Conserv. 6, 1507–1522. doi: 10.1023/A:1018314403123
Keywords: Arctic deep sea, benthic epifauna, biological trait analysis, functional composition, ROV
Citation: Zhulay I, Bluhm BA, Renaud PE, Degen R and Iken K (2021) Functional Pattern of Benthic Epifauna in the Chukchi Borderland, Arctic Deep Sea. Front. Mar. Sci. 8:609956. doi: 10.3389/fmars.2021.609956
Received: 24 September 2020; Accepted: 16 April 2021;
Published: 26 May 2021.
Edited by:
Ana Hilário, University of Aveiro, PortugalReviewed by:
Montserrat Demestre, Institute of Marine Sciences, CSIC, SpainSylvie Marylène Gaudron, Sorbonne Université, France
Copyright © 2021 Zhulay, Bluhm, Renaud, Degen and Iken. This is an open-access article distributed under the terms of the Creative Commons Attribution License (CC BY). The use, distribution or reproduction in other forums is permitted, provided the original author(s) and the copyright owner(s) are credited and that the original publication in this journal is cited, in accordance with accepted academic practice. No use, distribution or reproduction is permitted which does not comply with these terms.
*Correspondence: Irina Zhulay, aXJpbmEuemh1bGF5QHVpdC5ubw==