- Dipartimento di Elettronica, Informazione e Bioingegneria, Politecnico di Milano, Milan, Italy
The seagrass Posidonia oceanica is a benthic foundation species endemic to the Mediterranean Sea. It is a key component of coastal seascapes across the Mediterranean large marine ecosystem, where it plays fundamental ecological, physical, and economic roles. Despite the importance of this iconic seagrass species, a quantitative assessment of the interplay between local dynamics and basin-wide dispersal patterns is still lacking. Here we propose a Mediterranean-scale metapopulation model for P. oceanica, accounting for both demographic processes (inter-annual survival, vegetative growth, fruit production, seed establishment) and the spatial connectivity provided by current-driven dispersal of seagrass fruits. Model simulations are used to identify hotspots of seagrass population abundance, realized connectivity, and long-distance dispersal. Our results indicate that P. oceanica multi-functional hotspots, defined as species-suitable areas that rank high in all of the considered functional roles, are unevenly distributed in the four main sub-basins of the Mediterranean Sea, and along both the European and the African coastline. Our analysis also allows us to outline a remarkable geographical gap in protection: in fact, while many of the hotspots located along European coasts occur close to protected sites, the great majority of the hotspots lying on African coasts lack any form of protection. The identification of hotspots of P. oceanica metapopulation dynamics can thus help select regions that may serve as priority candidates for focusing conservation efforts.
1. Introduction
Posidonia oceanica is a flowering plant endemic to the Mediterranean Sea, where it is the most abundant seagrass and a pivotal foundation species (Ellison, 2019). P. oceanica forms large, mono-specific underwater meadows that provide favorable conditions for the assembly of the rich and diverse coastal communities of the Mediterranean basin (Gobert et al., 2006), one of the most important and iconic biodiversity hotspots worldwide (Myers et al., 2000). Despite being often underrepresented in both scientific research and public attention (Duarte et al., 2008), seagrasses play a crucial role in the functioning of aquatic ecosystems, which in turn produce substantial benefits to the human society (Nordlund et al., 2016). P. oceanica makes no exception in this regard. It does in fact contribute supporting (nutrient cycling, primary production), provisioning (raw materials, bioindicators, animal feed), regulating (water purification and oxygenation, protection from coastal erosion and sediment deposition, carbon sequestration, limitation of invasive species, and habitat provisioning for local ones—including many endemic and/or of commercial interest), and cultural (tourism, recreational fishing, knowledge contribution, intrinsic value) ecosystem services (Campagne et al., 2015).
The status of P. oceanica is declining in many coastal Mediterranean regions, with a recent report estimating a one-third decrease in areal distribution over the past 50 years (Telesca et al., 2015), adding to the global crisis of seagrass ecosystems (Waycott et al., 2009). Threats to P. oceanica include eutrophication and pollution (caused by chemical discharges from farming, aquaculture and urban waste), coastal development (shoreline hardening, coastal infrastructure development, sand mining), mechanical damage (trawling and boat anchoring), alien species, and climate change (Pergent et al., 2016). Because of its importance for coastal Mediterranean ecosystems, coupled with a decreasing population trend and a continuing regression in habitat extent and quality, P. oceanica has been a protection target under European (Habitat Directive, Barcelona, and Bern conventions) and national laws since the 1990s (Boudouresque et al., 2012).
P. oceanica is a long-lived, slow-growing seagrass characterized by high productivity (Hemminga and Duarte, 2000). Recruitment occurs via both vegetative growth and sexual reproduction, with the former mechanism being preponderant for the maintenance and expansion of existing seagrass beds as compared to the latter (Gobert et al., 2006), which is reportedly sporadic (Balestri and Cinelli, 2003). However, sexual reproduction is essential for colonization dynamics and the preservation of genetic diversity, which is often observed to be rather low (Procaccini et al., 2001). Sexual reproduction entails the production of flowers and fruits, which occurs with considerable spatiotemporal heterogeneity (Balestri and Vallerini, 2003). Fruits are positively buoyant and can be dispersed by surface marine currents, while seeds released by fruits are negatively buoyant and cannot travel far after being released (McMahon et al., 2014). Seedling establishment has also been often reported as quite episodic, although recent investigations have suggested that sexual reproduction could be more relevant for this species than previously thought (Balestri et al., 2017).
All these observations indicate that the population dynamics of P. oceanica should be seen as a dynamical balance between processes occurring at local vs. basin spatial scales. For instance, inter-annual shoot survival is eminently a local process, whereas the implications of sexual reproduction are better understood if put in a spatial perspective (Serra et al., 2010; Jahnke et al., 2017). Vegetative growth lays somewhat between these two extremes, because it may certainly contribute to meadow expansion, albeit at rates and distances that are not comparable with those achieved through sexual reproduction and subsequent propagule dispersal (McMahon et al., 2014). In other words, the dynamics of P. oceanica in the Mediterranean should be usefully framed in a metapopulation approach (Hanski, 1999). Although not quite common yet in the study of seagrass population dynamics (Bell, 2006), the metapopulation concept has already been evoked for P. oceanica (Rozenfeld et al., 2008), but mainly concerning the assessment of potential and realized connectivity patterns at various spatial scales (Jahnke et al., 2017; Mari et al., 2020). By contrast, no studies have yet attempted a full coupling of demographic and dispersal processes in the analysis of large-scale dynamics of P. oceanica in the Mediterranean Sea.
Similarly, no studies have yet been carried out that can guide the modeling of the effects of shoot density on P. oceanica vegetative growth, survival, sexual reproduction, seed germination, and/or seedling mortality at a whole-Mediterranean and multi-decadal scale. Density-dependence has been proposed as a factor regulating the vegetative growth of P. oceanica, possibly leading to the formation of three-dimensional, topographically complex seagrass structures within local meadows (Kendrick et al., 2005). Density-dependent shading is another process that is thought to limit vegetative growth and recruitment (Duarte et al., 2006). Although not specifically developed for this seagrass species, theoretical models of colony growth have often assumed density-dependent shoot mortality (Sintes et al., 2005; Ruiz-Reynés et al., 2017). For P. oceanica, little is known also about the possible effects of shoot density on seed germination and seedling mortality (Balestri and Lardicci, 2008). In seagrass species other than P. oceanica, the former process is generally not considered density-dependent (Orth et al., 2003), and the evidence on density dependence negatively affecting the latter is also quite scarce (Statton et al., 2017). In some cases, it has been proposed that positive density dependence may affect the sexual reproduction, patch colonization, and survival of some seagrass species, including P. oceanica (Almela et al., 2008; van Katwijk et al., 2016; Valdez et al., 2020). This complicated and somewhat scattered landscape of empirical evidence clearly calls for the development of a quantitative framework allowing the inclusion of simple and testable hypotheses about density dependence.
In this work, we propose a basin-wide metapopulation model for P. oceanica that accounts for both local demographic processes and the spatial connectivity provided by current-driven dispersal of seagrass fruits. The model is driven by spatially explicit information on local suitability conditions and marine circulation patterns. Alternative hypotheses concerning density-dependence are tested and compared with each other with respect to their ability to explain presence-absence P. oceanica data. The best-performing model is then used to evaluate connectivity and dispersal metrics, as well as their temporal variability. In this way, the different coastal regions inhabited by the seagrass can be assessed according to a recently proposed multi-functional scheme (Melià et al., 2016; Mari et al., 2020) that accounts for both local retention and sink/source dynamics on larger scales. Differently from—and possibly complementary to—other studies, where key conservation areas for P. oceanica were proposed based on habitat characteristics (Houngnandan et al., 2020) or spatial connectivity coupled with local suitability conditions (Mari et al., 2020), a full metapopulation approach is used here to suggest a species-specific prioritization strategy to guide large-scale conservation efforts. Specifically, the results obtained from the metapopulation model are used to identify hotspots of various ecological functions for P. oceanica at the scale of the whole Mediterranean Sea basin, as well as to pinpoint possible gaps in protection.
2. Materials and Methods
2.1. A Metapopulation Model for P. oceanica
The geographic domain of interest is divided into n marine sectors, representing the basic spatial units of the metapopulation model. Each sector is characterized by a fraction of suitable area ai (0 < ai ≤ 1, only sectors that include suitable sites for P. oceanica are retained for analysis) with an average suitability level si (0 < si ≤ 1). The total suitability of each sector can thus be evaluated as aisi. Let xi(t) be the density (shoots m−2) of P. oceanica in the suitable area of marine sector i in year t, before sexual reproduction takes place. The basin-wide metapopulation dynamics of P. oceanica are determined by the interplay of four different demographic processes, namely year-to-year shoot survival, vegetative growth, fruit production, and propagule establishment. Indeed, the last two processes represent the initial and finale phases, respectively, of the sexual reproduction process, which additionally also includes inter-sector fruit dispersal and the ensuing ecological connectivity.
To prevent an unbounded growth of local population abundances, the metapopulation model must incorporate density dependence. As discussed above, empirical evidence is to date still too scarce to be used for inferring functional relationships that may be applied consistently over the spatial (whole Mediterranean basin) and temporal (multi-decadal) scales of interest. Therefore, to describe each of the above demographic processes, we adopted a simple, saturating functional form inspired by the classic and widely used Beverton-Holt demographic model (Beverton and Holt, 1957), here modified to account also for local suitability conditions. Specifically:
• the survival Si(xi(t)) of P. oceanica shoots is defined as
where the inter-annual survival probability of seagrass shoots, σi(xi(t)), is assumed to be positively influenced by local average suitability and inversely related to local P. oceanica shoot density according to the functional form
with σmax and δσ being, respectively, the maximum survival probability (i.e., obtained with si = 1 and xi(t) → 0) and a parameter setting the intensity of density-dependent effects on shoot survival;
• vegetative growth Gi(xi(t)) is evaluated as
where the rhizome formation rate, gi(xi(t)), is also assumed to be positively influenced by local average suitability and inversely related to local P. oceanica shoot density according to
with gmax being the maximum rhizome formation rate [i.e., with si = 1 and xi(t) → 0] and δg setting the intensity of density-dependent effects on vegetative growth. Note that, for the sake of simplicity, vegetative growth is assumed not to result in seagrass bed expansion and the possible colonization of previously unoccupied substrate within the local sector, or even neighboring sectors, which is deemed to be a reasonable hypothesis if the spatial grain of the model is not too fine. This assumption is backed up by a comparative assessment of the role played by different movement processes in P. oceanica, which showed that the dispersal of buoyant fruits allows much faster and longer-range colonization dynamics than clonal growth does (McMahon et al., 2014);
• the contribution of sexual reproduction Ri(x1(t), x2(t), …, xn(t)) is quantified as
where ri(x1(t), x2(t), …, xn(t)) is the density of P. oceanica fruits arriving to sector i after having been sexually produced in any sector j (j = 1, 2, …, n, including j = i) and transported by marine currents, while fi(xi(t)) is the probability of successful fruit settlement (accounting for fruit germination and seedling survival). In particular, fruit production and transport are described as
where
is the rate of fruit production, assumed to be positively influenced by local average suitability and inversely related to local shoot density, with hmax being the maximum rate of seed production (i.e., with sj = 1 and xj(t) → 0) and δh setting the intensity of density-dependent effects on sexual reproduction. The quantity Cji(t) is the probability that a P. oceanica fruit produced in sector j during the reproductive season of year t eventually ends up in sector i (potential connectivity; Jahnke et al., 2017). As for settling,
is defined as the probability of fruit germination and inter-annual seedling survival in sector i, also assumed to be positively influenced by local average suitability and inversely related to local shoot density, with fmax and δf being the maximum settling probability (i.e., with ai = 1 and xi(t) → 0) and a parameter setting the intensity of density-dependent effects on successful fruit germination and seedling survival, respectively.
Note that, for the sake of model simplicity, shoot age is not considered in any of the above processes. Therefore, possible age-related effects on, e.g., inter-annual survival or sexual reproduction are here neglected. However, this choice does not seem to represent an oversimplification for P. oceanica. On the one hand, in fact, observational studies suggest that shoot mortality is not strongly influenced by age (e.g., González-Correa et al., 2007a); on the other, while it is typically found that shoots of all ages (from 6-month-old on) can potentially contribute to sexual reproduction, reports vary on the shape of the relationship between age and flowering rate, with peaks being observed around 6 or 14 years of age (Balestri and Cinelli, 2003; Calvo et al., 2006). Also, no positive density-dependent processes (i.e., intraspecific facilitation) have been accounted for.
Summing up the contributions of shoot survival, vegetative growth, and sexual reproduction to the local densities of P. oceanica leads to a set of n coupled difference equations, namely
which can be used to describe P. oceanica metapopulation dynamics, once the relevant characteristics of the seascape (distribution of suitable habitat, spatial connectivity) have been evaluated.
2.2. Application of the Model to the Mediterranean Sea
Habitat suitability mapping for P. oceanica in the Mediterranean Sea has been made available as an output of the MediSeH project (Giannoulaki et al., 2013). There, observations of P. oceanica presence/absence and a set of 36 predictor variables (of which nitrate and silicate concentrations, average depth, sea surface temperature and salinity were found to be the most important) were used to train a random forest algorithm (Breiman, 2001) estimating the probability of species occurrence throughout the whole Mediterranean Sea basin at a high spatial resolution (1/240°, corresponding to a regular mesh with an ~460 m-long step). At that spatial scale, about 685,000 individual sites were characterized by positive values of habitat suitability.
Basin-wide spatial connectivity for P. oceanica in the Mediterranean Sea has already been estimated within the ECOPOTENTIAL project (https://cordis.europa.eu/project/id/641762/it) through a biophysical approach (Mari et al., 2020). In that study, more than 30 billion Lagrangian particles, representing free-floating P. oceanica fruits, were tracked over a time span of ny = 30 years (1987–2016). Particles were released from suitable sites matching the reproductive season of the seagrass (typically, January throughout April) and the duration of their floating phase (at most 1 month). The transport of P. oceanica fruits was driven by surface circulation fields (daily averages at 1/16° resolution, corresponding to a regular grid with an approximate mesh size of 6.9 km) obtained from a Mediterranean-wide physical reanalysis (Lecci et al., 2017). In the present study, potential connectivity between any two marine sectors during a given season, say sectors i and j in year t, Cij(t), is defined as the fraction of particles released during year t from suitable sites within sector i that reach suitable sites within sector j at the end of the dispersing phase. Supplementary Figure 1 displays some synthetic metrics of potential connectivity (average values and temporal variability over the 1987–2016 period), namely self-retention (quantifying how many P. oceanica fruits both are released and settle in the same marine sector), indegree (indicating the tendency of a sector to function as a potential sink of P. oceanica fruits), and outdegree (indicating the tendency of a sector to function as a potential source of P. oceanica fruits). Potential connectivity scores can also be used to evaluate metrics pertaining to the average distance traveled by dispersing fruits. In this respect, Supplementary Figure 2 reports the displacement of dispersing fruits either incoming to or outgoing from each marine sector (again, average values and temporal variability over the 1987–2016 period), where the distance dij between the centroids of any two sectors i and j, has been evaluated according to the great-circle formula.
Applying the metapopulation model at the fine spatial resolution of the suitability map would likely allow a more detailed description of local-scale demographic dynamics, but would also make it hard to obtain a robust parameterization and perform efficient simulations. Therefore, as a trade-off between accuracy, on the one hand, and robustness and applicability, on the other, the coarser spatial resolution of the circulation fields has been chosen as a reference for the application of the metapopulation model. Coherently, the suitability map has been up-scaled to the spatial resolution of the circulation fields used here. In this way, n = 9, 648 suitable marine sectors were identified (Supplementary Figure 3), each of which covers an area of ~48 km2 along the Mediterranean coastline.
Most of the other biological parameters of the metapopulation model described above can be evaluated from available observational studies on the ecology of P. oceanica. Specifically, the maximum values of inter-annual shoot survival, σmax, and of the vegetative growth (rhizome formation) rate, gmax, are taken from studies on the demography and dynamics of seagrass colonies (Marbà et al., 1996, 2005; Badalamenti et al., 2006; González-Correa et al., 2007a; Marbà and Duarte, 2010). The reference values for these parameters are evaluated as the average of the maximum figures reported by each study, under the assumption that the reported values of population recruitment were reflective of vegetative growth alone, i.e., neglecting sexual reproduction—a reasonable hypothesis, considering that flowering is considered to be episodic in P. oceanica (e.g., Balestri and Cinelli, 2003; Calvo et al., 2006). The maximum rate of fruit production, hmax, is obtained from one of the few empirical studies on the reproductive success of P. oceanica (Balestri and Cinelli, 2003). The reference value for this parameter is selected as the maximum figure reported in that study. The maximum settling probability of dispersing fruits, fmax, is estimated from studies on germination (Balestri et al., 1998a; Balestri and Bertini, 2003; Fernández-Torquemada and Sánchez-Lizaso, 2013) and long-term seedling survival (Balestri et al., 1998b; Piazzi et al., 1999). The reference value for fmax is in fact obtained as the average of the products of the maximum germination and seedling survival probabilities reported in each study. A study providing figures for overall settling probability (Balestri et al., 1998a, maximum reported value 75%) is used to validate the estimate obtained from the combination of multiple reports. Parameter values and relevant references are provided in Table 1.
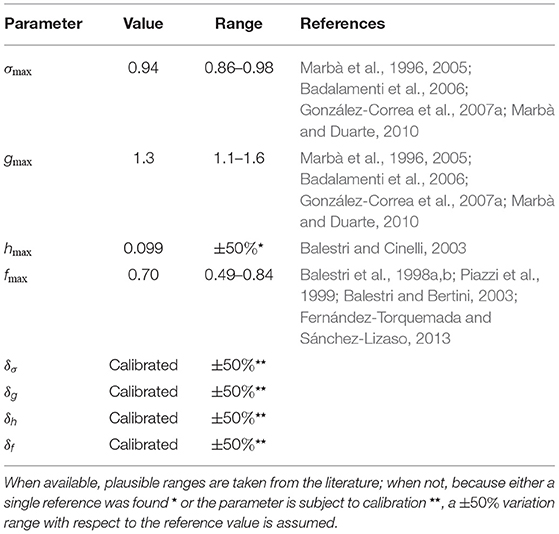
Table 1. Reference parameter values for the P. oceanica metapopulation model in the Mediterranean Sea.
2.3. Model Calibration
To understand which of the processes describing large-scale P. oceanica metapopulation dynamics may be influenced by density-dependent effects, a model selection exercise is run to compare the performances of several candidate model structures characterized by alternative hypotheses concerning density dependence. Vegetative growth has always been considered as density-dependent to avoid unbounded growth of local population densities (because, according to the literature, gmax>1, see again Table 1). Conversely, each of the other three processes (adult shoot survival, sexual reproduction, and post-dispersal settlement) is alternatively considered either density-dependent (and the corresponding δq parameter, with q∈{σ, g, f, h}, calibrated) or not (and the corresponding δq parameter set to zero). For the sake of model parsimony, we also consider simplified cases in which two or more of the parameters setting the intensity of density dependence are constrained so that they take the same numerical value(s).
For each candidate model, calibration is performed as follows: models are simulated for a period of 100 years from random initial conditions to allow the dynamics of the system to settle to a stationary state. The temporally averaged connectivity scores are used to describe propagule dispersal in this phase of the simulation, as preliminary analyses of the metapopulation model showed P. oceanica densities to be scarcely influenced by the temporal fluctuations of potential connectivity (as somewhat expected, because vegetative growth reportedly outweighs sexual reproduction in P. oceanica, as discussed above). The spatial distribution of shoot densities projected by the model at the equilibrium is compared with the binary observations of P. ocenica presence/absence collected in Telesca et al. (2015), suitably up-scaled to the spatial scale of marine sectors. Specifically, P. oceanica is assumed to be present in a sector if the majority of the available local observations reports seagrass presence and, vice-versa, absent if the majority of the observations reports absence. Simulated P. oceanica densities are used to fit a generalized linear model with a logit link (function fitglm in Matlab R2019a). The log-likelihood value (LLV) of the model given the observations is used as the objective function to maximize. The exploration of the parameter space is performed with a genetic algorithm (function ga in Matlab R2019a) to maximize each candidate model's ability to explain seagrass presence/absence patterns. For each parameter, the search region is limited to the interval 10−4–104 (shoots m−2)−1. To make sure that modeled densities are in line with field observations, parameter search is also constrained so that the 99th percentile of the simulated density distribution corresponds to 1, 000 ± 50 shoot m−2, consistent with the maximum values reported in current guidelines for P. oceanica monitoring (Pergent-Martini, 2015). The whole procedure is repeated 10 times for each candidate model starting from randomly extracted initial parameter sets to improve the exploration of the calibration region.
As the various candidate models are endowed with a different number of calibration parameters (np, from one to four), their performances are ranked through the Akaike information criterion (AIC), so as to reveal possible trade-offs between model accuracy and parsimony. Specifically, for each model we evaluate AIC as
where LLVmax is the maximum value of LLV found over the 10 independent runs of the calibration procedure. Then, Akaike differences (ΔAIC) are evaluated between the AIC scores of the various candidate models and the AIC score of the best supported model (the one with the lowest AIC score).
2.4. Performance Evaluation of the Selected Model
Once the best supported model according to AIC scoring has been selected, it may be useful to evaluate its actual explanatory power against the actual distribution of P. oceanica in the Mediterranean Sea. To that end, a receiver operating characteristic (ROC) curve can be used to evaluate the diagnostic ability of the selected model (which produces a projection of continuous local population density values) as a binary classifier of P. oceanica presence/absence data. The ROC curve is obtained by plotting the true positive rate, defined as the ratio between the number of correctly identified presences and the number of total presence data, against the false positive rate, defined as the number of observations incorrectly classified as presences divided by the number of total absence data, for different values of the discrimination threshold. In ROC analysis, a random classifier would have a value of the area below the ROC curve of 0.5, while a perfect classifier would have a value of 1. A simplistic, yet widely used metric to summarize the performances of a binary classifier is Youden's J statistics (Baker and Kramer, 2007), defined as
where sensitivity is the true positive rate and specificity is the true negative rate, defined as the number of observations correctly classified as absences divided by the number of total absence data. The discrimination threshold that yields the maximum value of Youden's J can be used to select the optimal cut-point of the classifier (Schisterman et al., 2005).
2.5. Spatiotemporal Variability and Metapopulation Dynamics
To assess the effect of the temporal variability of marine currents on the metapopulation dynamics of P. oceanica, the best-performing metapopulation model selected according to the procedure described in the previous section is simulated again starting from the steady-state solution, corresponding to a spatial distribution of seagrass shoot densities in suitable areas—this time with time-varying connectivity. Specifically, the model is run for a time span of 200 years, for each of which a connectivity matrix Cij(t) is randomly selected from the ny = 30 available. In this way, any possible multi-annual trends in potential connectivity are clearly destroyed. However, previous work (Mari et al., 2020) has shown that trends in P. oceanica connectivity can be detected in just a minority of suitable marine sectors (a fraction ≤ 5% in each potential connectivity metric considered). The first 100 years of the simulation are used as a spin-up period; afterwards, six quantities are evaluated for each marine sector over the last 100 years of the simulation, namely:
1. Total P. oceanica shoot density (shoots m−2)
2. Realized self-retention (shoots m−2 year−1)
3. Realized indegree (shoots m−2 year−1)
4. Realized outdegree (shoots m−2 year−1)
5. Realized inbound displacement (km)
6. Realized outbound displacement (km)
Quantity 1 refers to P. oceanica population density, while quantities 2–6 describe realized propagule dispersal, in terms of either connectivity intensity (2–4) or displacement distance (5–6). Quantities 2–6 account for the exchange of fruits that are produced at an origin sector and transported by currents to a destination sector where they actually germinate and survive into seagrass shoots, according to the local demographic and environmental conditions of the origin and destination sectors. Note that all these quantities need a fully dynamic and spatially explicit metapopulation framework to be evaluated. For each sector, the temporal average and variance of each quantity are recorded. The time-varying simulation with randomly extracted connectivity matrices is replicated nr times, and pooled mean and variance values are evaluated for each sector and quantity.
2.6. Identification of P. oceanica Hotspots
Ecological hotspots for P. oceanica can be identified following the methodological framework proposed by Melià et al. (2016), in which potential connectivity metrics were used to define a synthetic score recapitulating the capacity of different local populations to simultaneously act as retainers, sinks and sources. The same framework was used in Mari et al. (2020) for a Mediterranean-wide assessment of P. oceanica ecological connectivity. Here, we take advantage of the metapopulation model and expand the range of possible ecological functions played by local seagrass populations, namely by including metrics related to population density, realized connectivity, and displacement patterns. For each quantity Q defined above (with Q∈{TD, SR, ID, OD, IM, OM}) and each marine sector i, two percentile scores are computed:
• , defined as the percentage of sectors j = 1…n characterized by values of Ep[Qj] not larger than Ep[Qi], where Ep[Qi] is the pooled mean of quantity Q in sector i;
• , defined as the percentage of sectors j = 1…n characterized by values of CVp[Qj] not lower than CVp[Qi], where is the pooled coefficient of variation of quantity Q in sector i, as obtained by dividing the square root of the pooled variance by the pooled mean (note that sectors for which Ep[Qi] = 0, so that CVp[Qi] would not be defined, are attributed to the lowest percentile class).
Afterwards, for each quantity Q and sector i, the two percentiles scores are aggregated conservatively to form a synthetic percentile index defined as
Sectors where is highest can be considered as hotspots for quantity Q, because they are endowed with both large average intensity and low temporal variability of the signal associated with Q. Following Melià et al. (2016) and Mari et al. (2020), the six percentile scores can be further aggregated to identify multi-functional hotspots for P. oceanica dynamics. For instance, a multi-functional percentile index can be defined as
Note that this is, again, a conservative aggregation rule, in that sectors are evaluated according to the percentile index in which they are weakest. For this reason, sectors where is highest can be considered as multi-functional hotspots, because the seagrass populations they host are the most effective (or the least constrained) at providing simultaneously multiple ecological functions—thus arguably qualifying as possible priority candidates for protection (Orth et al., 2006).
2.7. Sensitivity Analysis
To assess the robustness of the hotspot identification procedure outlined above, two different types of sensitivity analysis are carried out, one related to geographic resolution and the other to model parameterization. In the former, robustness against the spatial grain of the model is tested, specifically concerning the evaluation of realized connectivity and displacement metrics. To that end, the hotspot identification procedure is repeated assuming that connectivity occurring between sectors located at distance from each other contributes to self-retention of the originating sector (hence, it does not count toward in/outdegree or in/outbound displacement). In the latter, robustness against parameter uncertainty is assessed, namely by repeating ns times the simulation algorithm with time-varying connectivity, each time with parameter values randomly and independently drawn from uniform distributions with supports defined within the ranges reported in Table 1. When possible (parameters σmax, gmax, and fmax), plausible ranges of variation are obtained from the studies used to estimate the reference value of each parameter; when not, plausible ranges are defined as ±50% variations of the reference (or calibrated) values.
3. Results
The lowest AIC score is attained by a model describing shoot survival, vegetative growth, and post-dispersal settlement as density-dependent processes (δσ > 0, δg > 0, δf > 0), and density-independent fruit production (δh = 0; Table 2). The model is parsimonious in that the calibrated intensities of the density feedback are the same for all selected processes (δg = δσ = δf = δ). The best-supported model can thus be rewritten as
With an area under the ROC curve of 0.926 (Figure 1), this model has a good ability to separate P. oceanica presence/absence data. As a matter of fact, at the discrimination threshold of the logit link that maximizes Youden's J statistics the model correctly identifies 90.7% of true absences and 80.3% of true presences.
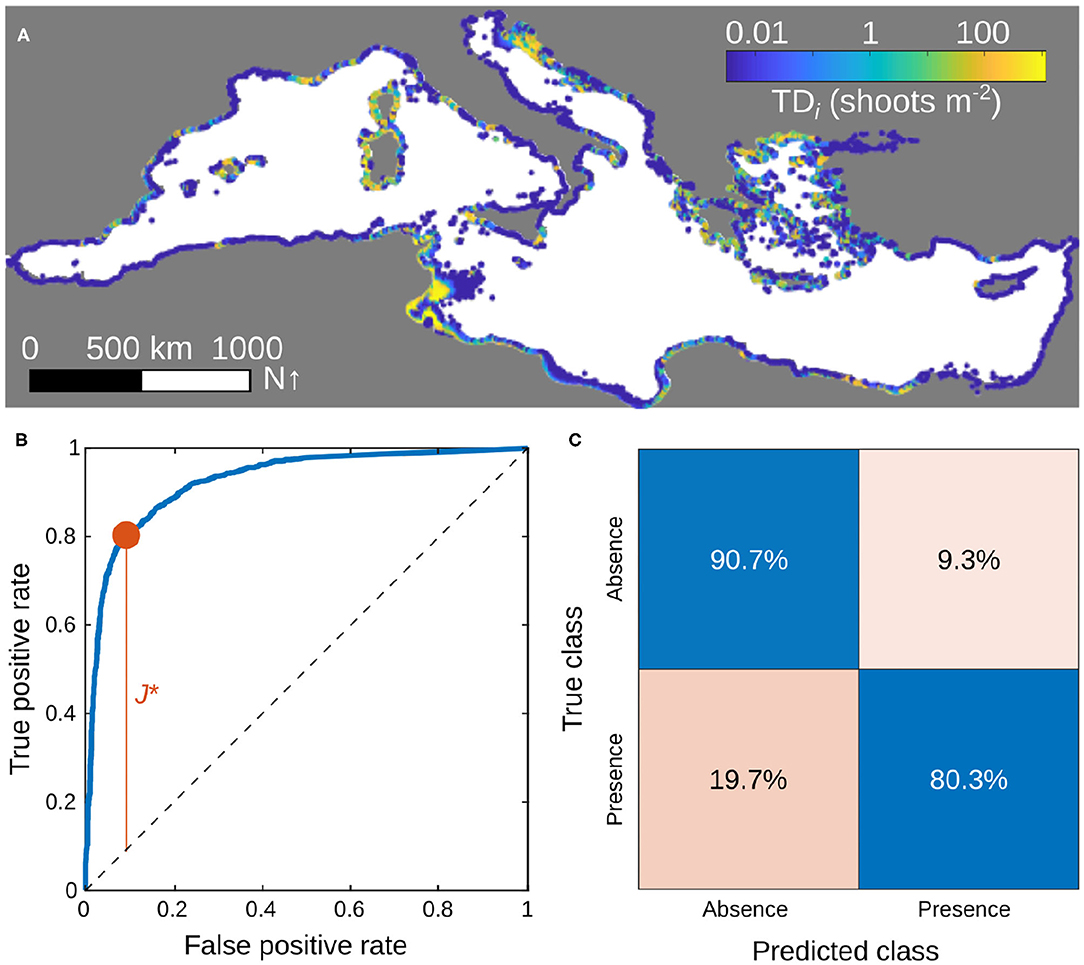
Figure 1. Calibration and classification performances of the selected metapopulation model. (A) Total density of P. oceanica shoots at equilibrium, evaluated by simulating the best-performing metapopulation model over a timespan of 100 years starting from random initial conditions and using the time-averaged connectivity scores 〈Cij〉. (B) ROC curve (in blue) of the model, evaluated as a classifier of the binary presence/absence P. oceanica data. The red dot identifies the threshold for which Youden's J-statistics is maximum. The dashed diagonal represents the line of no-discrimination, corresponding to the performance of a random binary classifier. (C) Confusion matrix evaluated for the discrimination threshold maximizing Youden's J. Calibrated parameter values: (shoots m−2)−1, δh = 0. Other parameters as in Table 1.
Other two models reach a similar level of statistical support as the first one (ΔAIC < 2). The second-ranked model according to AIC has the same density-dependence structure as the best one with two free calibration parameters instead of just one. The similarity of the calibrated parameter values provides further support for the parsimony of the top-ranked model. The third-ranked candidate is also another parsimonious model with one free parameter, but with vegetative growth and shoot survival as the only density-dependent processes. Not surprisingly, given the models' structure and parameter similarities, the shoot densities projected by the three top models appear to be very similar to each other (Supplementary Figure 4), and pairwise two-sample Kolmogorov-Smirnov testing does not reject the hypothesis that three samples of simulated total density obtained with the three top-ranked models come from the same underlying distribution at significance p ≪ 10−4. Four other models turn out to be somewhat supported (2 < ΔAIC < 4): two of them have the same structure of the two best-ranked models (with two free parameters), one also includes density-dependent sexual reproduction (although with a value of δh close to the lower bound of the search interval, which makes the process practically ineffective), and another with the same structure as the third-ranked model (but with two free parameters instead of one). All other models are considerably less supported by the data (ΔAIC > 4). Among these, the candidates not accounting for density-dependent shoot survival perform very poorly in terms of AIC (ΔAIC > 100). For all these reasons, no candidates other than the best-performing model are retained for further analysis.
With the only purpose of evaluating the role of dispersal in the overall dynamics of the metapopulation, a simulation is performed with the parameter set of the best-performing model but no connectivity, i.e., with the identity matrix of size n replacing the average connectivity scores 〈Cij 〉 (Supplementary Figure 5). This exercise shows that 63.6% of suitable marine sectors are characterized by larger total shoot density in the presence of connectivity than in the absence thereof, while 19.7% of sectors have smaller population density when dispersal is operating. Positive variations are typically small in absolute magnitude (+0.98 shoots m−2 on average), but quite large in relative terms, e.g., if compared with the model simulation shown in Figure 1 (+96.6% on average), indicating that dispersal may be instrumental to maintaining local populations (albeit at low shoot densities) in places that would not be colonized by P. oceanica meadows otherwise. Conversely, negative variations of population densities are usually larger than positive ones in absolute magnitude (−7.6 shoots m−2 on average), but remarkably smaller in relative terms (−9.3% on average).
A simulation of the selected metapopulation model run with the reference parameter set and time-varying connectivity is shown in Figure 2, specifically in terms of the pooled temporal average (A) and coefficient of variation (B) of the total density of P. oceanica shoots. The simulation converges to a condition characterized by conspicuous, geographically structured spatial heterogeneity of average shoot density. The highest values are projected to occur in Tunisia, especially in the Gulf of Gabès, and Croatia, as well as in the main islands of the Western Mediterranean basin, namely Sicily and Sardinia (Italy), Corsica (France), and the Balearic archipelago (Spain).
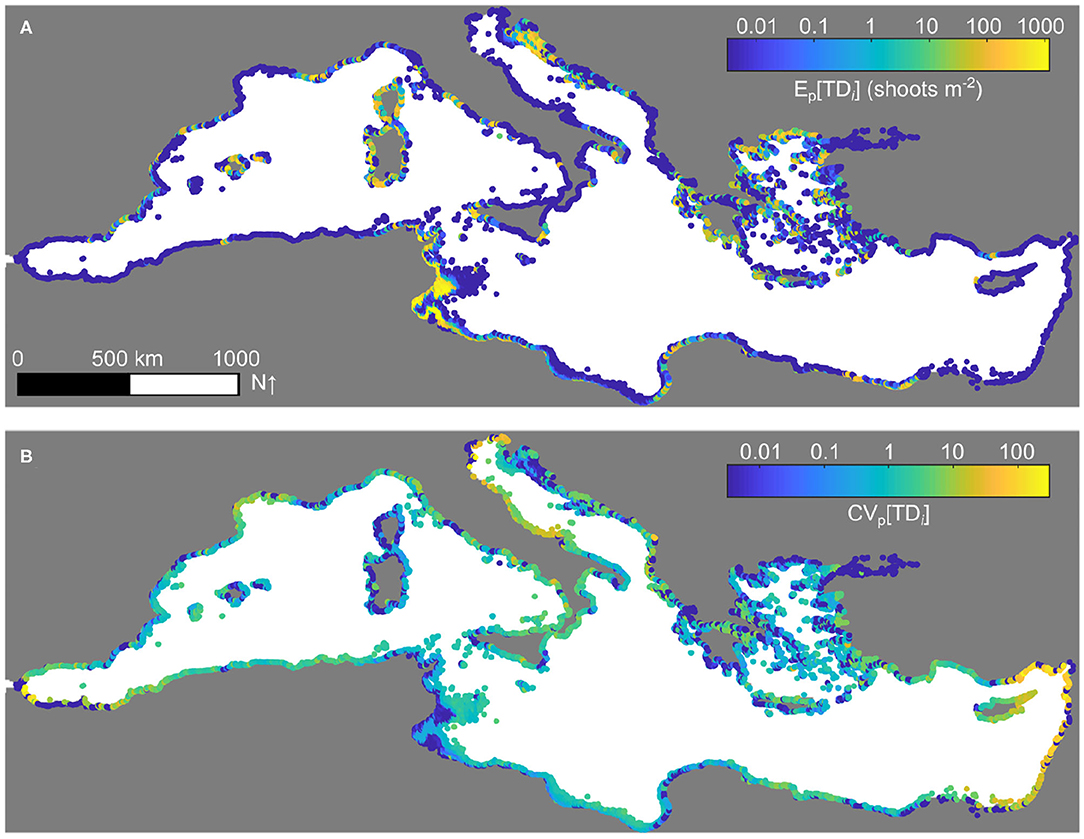
Figure 2. Reference simulation of the P. oceanica metapopulation model. The model has been run starting from random initial conditions over a time span of 100 years with the average connectivity matrix 〈Cij〉 to allow the dynamics of the system to settle to a steady-state solution (shown in Figure 1A), then for other 200 years with time-varying connectivity Cij(t) (nr = 100 replicas). The pooled, across-replica, average (A) and coefficient of variation (B) of total shoot densities, evaluated for the last 100 years of the simulation, are shown in log10 scale. Parameters as in Figure 1.
The spatial patterns of realized connectivity for P. oceanica evaluated with the top-ranked metapopulation model for the reference simulation of Figure 2 are shown in Figure 3. Because realized connectivity accounts not only for the potential means of dispersal provided by marine currents but also for the demographic dynamics of the metapopulation and their interactions with the seascape, the resulting spatial patterns turn indeed out to be quite distinct from those of potential connectivity, as it is apparent by contrasting realized (i.e., metapopulation-based) vs. potential (i.e., oceanographic) connectivity metrics (Figure 3 vs. Supplementary Figure 1). From a quantitative perspective, the contribution of average realized connectivity to the spatiotemporal dynamics of P. oceanica appears to be quite small compared to that of local population densities, reflecting the somewhat sporadic nature of sexual reproduction in P. oceanica. This observation is also backed up by the estimates of temporal variability in connectivity strength, which are large (e.g., CVp ≥ 10) in many sectors throughout the Mediterranean basin. Interestingly, the maximum values of average indegree are in line with reported observations of recruitment by seed in highly suitable locations (around 20–30 seedlings m−2; Balestri et al., 2017).
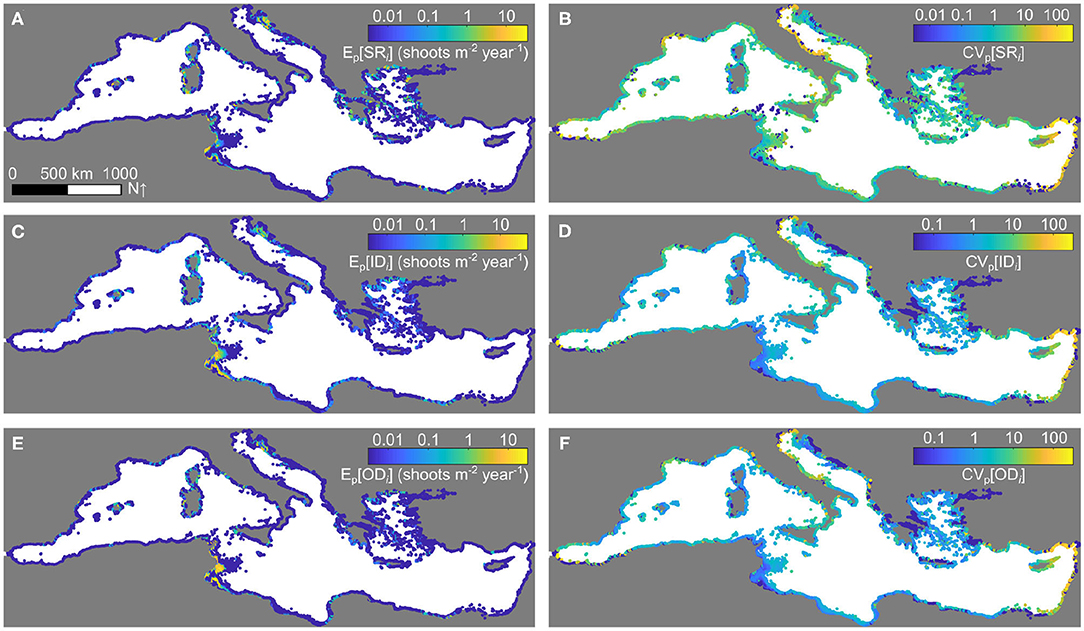
Figure 3. Realized connectivity patterns for P. oceanica evaluated from the metapopulation model. (A) Pooled average and (B) coefficient of variation of self-retention. (C) Pooled average and (D) coefficient of variation of indegree connectivity. (E) Pooled average and (F) coefficient of variation of outdegree connectivity. All quantities refer to the simulation of Figure 2 and are shown in log10 scale.
The spatial patterns of propagule displacement, i.e., the average distances traveled by P. oceanica fruits successfully dispersing to/from a given marine sector, have also been evaluated for the reference simulation of Figure 2 and are shown in Figure 4. While most connections are local, with average dispersal distances confined to a few kilometers, some sectors appear to be characterized by relatively long-distance dispersal, with average in/outbound successful propagule displacements up to a few hundred kilometers. This is the case, in particular, for the sectors located in the region of the Gulf of Gabès, at the crossroad between the western and eastern basins of the Mediterranean Sea.
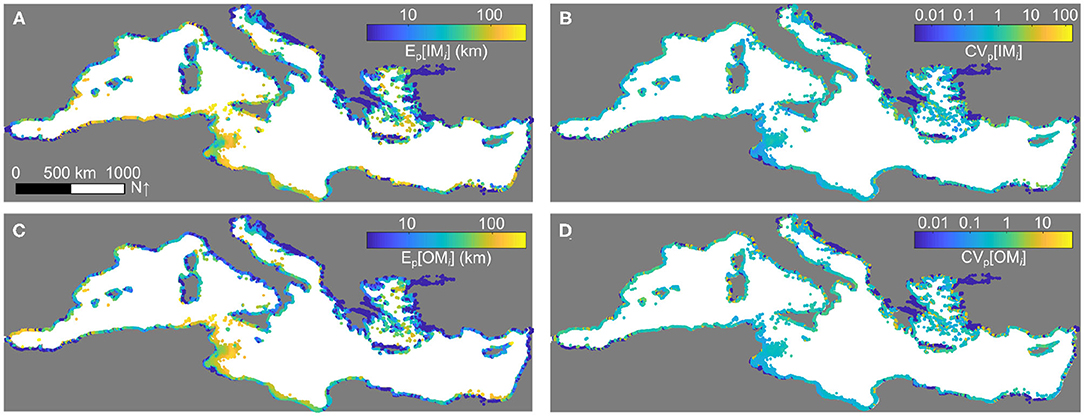
Figure 4. Realized displacement patterns for P. oceanica. (A) Pooled average and (B) coefficient of variation of inbound displacement. (C) Pooled average and (D) coefficient of variation of outbound displacement. All quantities refer to the simulation of Figure 2 and are shown in log10 scale.
The metapopulation density, connectivity, and displacement patterns projected by the reference model simulation have been used to identify P. oceanica hotspots, regions of the Mediterranean Sea that play a key role in the basin-wide metapopulation dynamics of this seagrass species. Visual inspection of the spatial distribution of the hotspots based on the percentiles (Q ∈ {TD, SR, ID, OD, IM, OM}, Figure 5) reveals distinctive spatial patterns for the hotspots identified using density (panel A), connectivity (B–D), or displacement-related measures (E–F). The diversity of the hotspots corresponding to specific ecological functions is well-reflected in the identification of multi-functional hotspots, defined according to a conservative aggregation of the performances of each sector in the various percentile scores (Figure 6A). The marine sectors identified as multi-functional hotspots (e.g., those with ) are mainly located along the coasts of Tunisia and Libya, for Africa, as well as in the Aegean Sea, in Sardinia and along the Spanish coastline—with other, more isolated occurrences in the Balearic Islands, France, and the Adriatic Sea, for Europe. Hotspot identification also helps recognize possible gaps in the protection of this foundation species. Specifically, a clear pattern emerges where European P. oceanica multi-functional hotspots are within or close to areas characterized by some form (not necessarily P. oceanica-specific) of protection, while hotspots located along the African coastline may be hundreds of kilometers away from the closest marine protected area (Figure 6B).
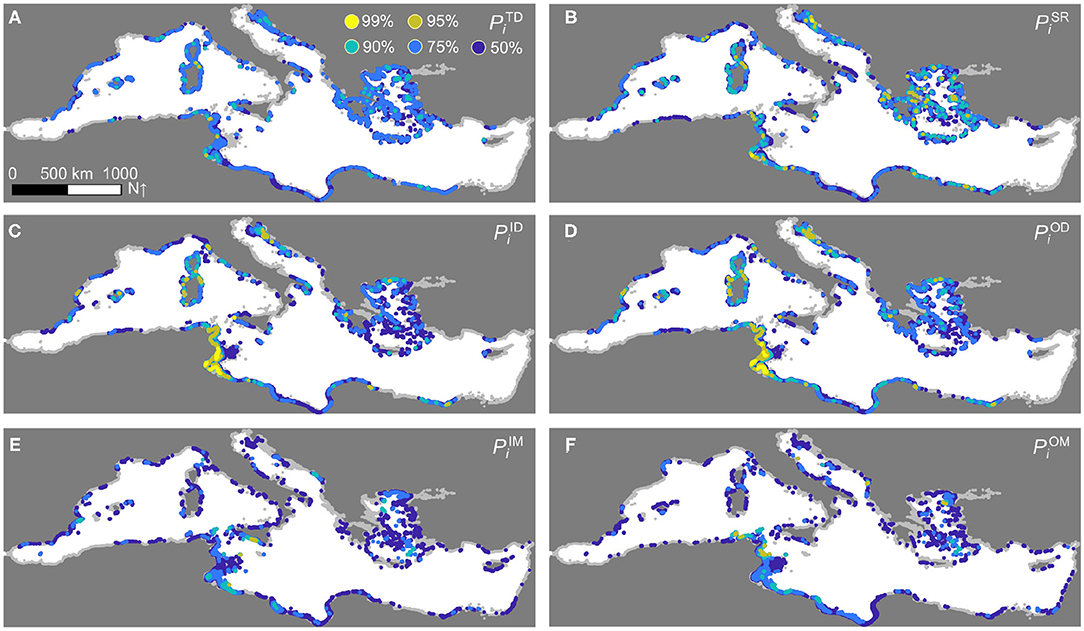
Figure 5. Hotspots of P. oceanica metapopulation dynamics. (A) Abundance hotspots. (B) Self-retention hotspots. (C) Indegree hotspots. (D) Outdegree hotspots. (E) Inbound displacement hotspots. (F) Outbound displacement hotspots. Colors identify sectors that, for a given metric Q ∈ {TD, SR, ID, OD, IM, OM}, are above the k-th percentile of the across-sector distribution (k ∈ {50, 75, 90, 95, 99}). The higher is the percentile, the hotter is the spot. Sectors for which are shown in light gray. Results refer to the simulation of Figure 2.
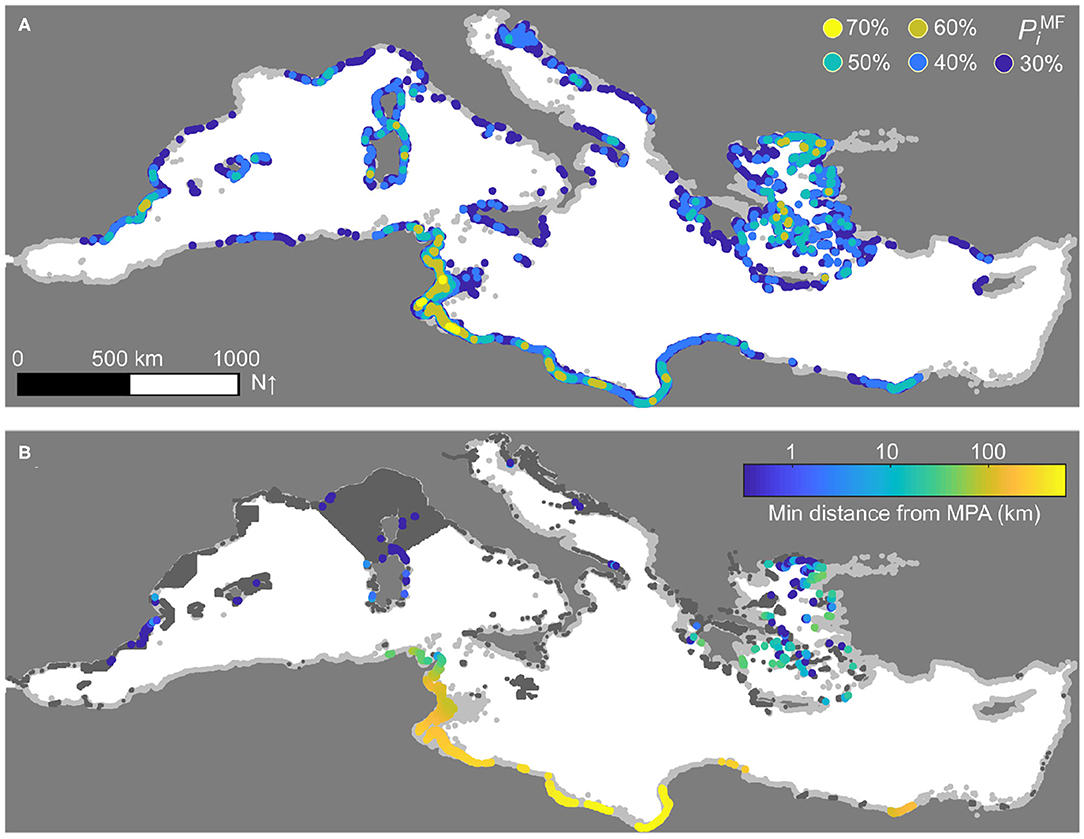
Figure 6. Multi-functional hotspots and protection gaps for P. oceanica. (A) Sectors that are above the k-th percentile in the across-sector distribution of the multi-functional percentile score (k ∈ {30, 40, 50, 60, 70}). Sectors for which are shown in light gray. (B) Distance (log10 scale) between the centroids of marine sectors with and the closest marine protected area. Protected sites have been extracted from the MPAtlas database (Marine Conservation Institute, 2019) and are shown in dark gray, while marine sectors with are in light gray. Results refer to the simulation of Figure 2.
Finally, in terms of sensitivity analysis, the hotspot identification procedure produces consistent results when the spatial grain of the metapopulation model is altered. As an example, Supplementary Figure 6 shows the computation of density, connectivity and displacement indicators from the output of the model simulation, while Supplementary Figure 7 reports the evaluation of percentile scores and their multi-functional aggregation, both with d⋆ = 50 km. The results show that, despite differences in function-specific indicators (hence, possibly, also in the relevant sector rankings) with respect to the reference case, the same key marine regions tend to emerge. A generalization of these findings to other threshold distances is shown in Supplementary Figure 8. A remarkable robustness of model projections is also found when sensitivity analysis is performed with respect to parameter uncertainty. Supplementary Figure 9 shows what happens to average seagrass densities when the model parameters are varied within biologically plausible ranges (Table 1), while Supplementary Figure 10 reports the evaluation of function-specific indicators. Based on these results, the identification of P. oceanica hotspots seems to be quite robust also to changes in the parameterization of the model, in that most of the key areas identified in Figures 5, 6A consistently emerge also under parameter uncertainty (Figure 7).
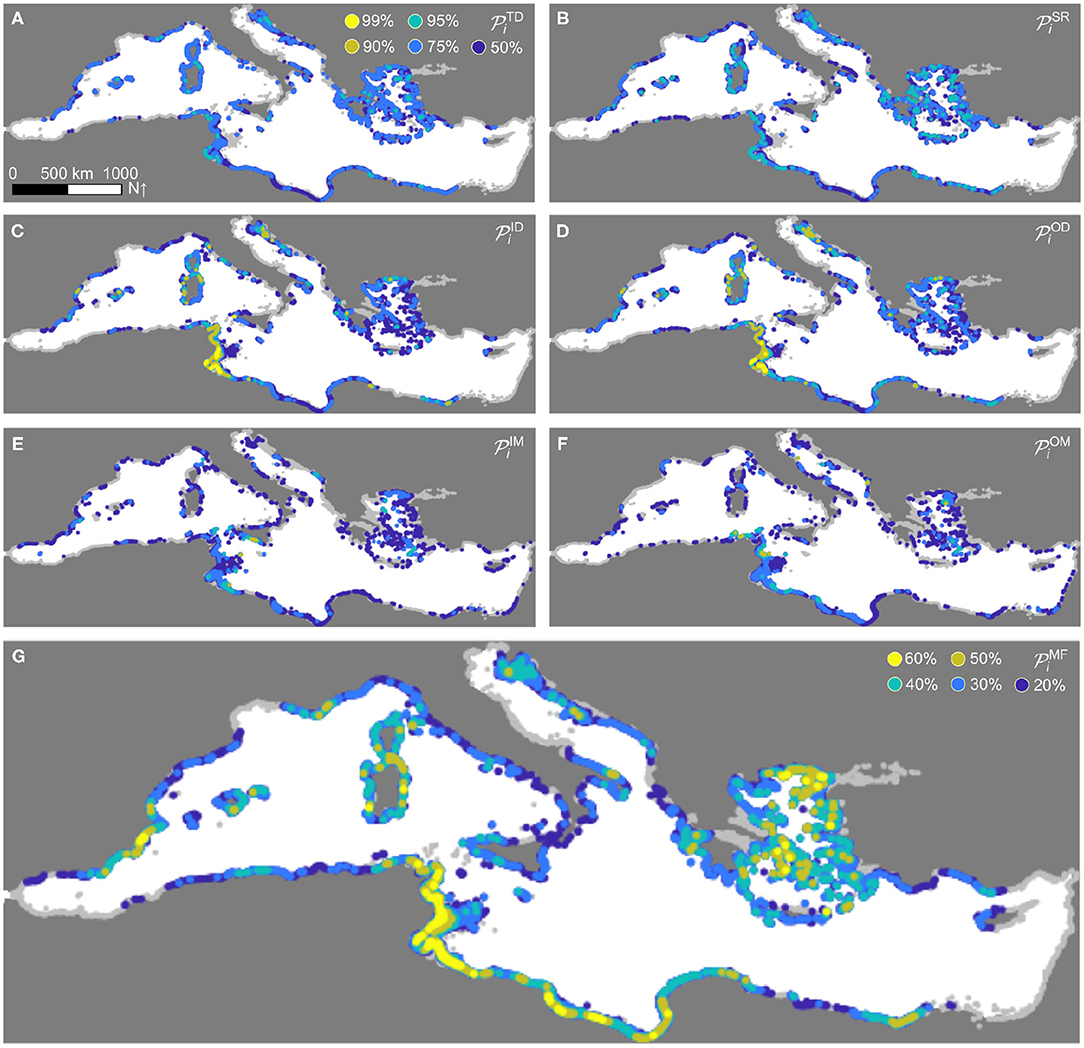
Figure 7. Sensitivity of the hotspot identification procedure to changes in the parameters of the metapopulation model. (A) Abundance hotspots. (B) Self-retention hotspots. (C) Indegree hotspots. (D) Outdegree hotspots. (E) Inbound displacement hotspots. (F) Outbound displacement hotspots. Colors identify sectors that, for a given metric Q ∈ {TD, SR, ID, OD, IM, OM}, are above the k-th percentile (k ∈ {50, 75, 90, 95, 99}) of the across-sector distribution in at least k% of the simulations performed with parameter values randomly (and uniformly) drawn from the ranges reported in Table 1 ( score). Sectors for which are shown in light gray. (G) Multi-functional hotspots, identified as sectors that are above the k-th percentile in the across-sector distribution of the multi-functional percentile score (k ∈ {20, 30, 40, 50, 60}) in at least k% of the simulations ( score). Sectors for which are shown in light gray.
4. Discussion
In this work, we have presented a quantitative analysis of the Mediterranean-wide dynamics of an iconic foundation species, the endemic seagrass P. oceanica, using a spatially explicit and dynamic metapopulation approach. The model couples local density-dependent demographic dynamics, driven by spatially explicit information on habitat suitability, with current-driven dispersal of seagrass fruits, informed by data from oceanographic reanalyses. Using a formal model-selection framework, we tested several concurrent hypotheses concerning the role possibly played by density dependence in different phenological processes. We found that a parsimonious approach describing vegetative growth, shoot survival, and post-dispersal settlement as density-dependent processes, and sexual reproduction as a density-independent process (Figure 2), may represent a well-balanced trade-off between accuracy (evaluated as the model's ability to explain binary observations of P. oceanica presence/absence throughout the Mediterranean basin) and complexity (evaluated as the number of calibration parameters). The selected model set-up has been used to evaluate simple metrics concerning realized connectivity and dispersal distance (Figures 3, 4), which formed the quantitative basis for the identification of P. oceanica functional hotspots (Figure 5). Marine sectors that can simultaneously play different ecological functions, such as retainer (high density, strong propagule retention), source (large outdegree connectivity, long outbound dispersal distance), or sink (large indegree connectivity, long inbound dispersal distance), have also been identified (Figure 6). From a modeling point of view, we believe that the approach presented in this work represents a clear improvement over previous applications describing only either the demographic traits of single P. oceanica meadows (Duarte et al., 2006) or current-driven connectivity (Mari et al., 2020).
Like all modeling studies, ours is not devoid of limitations. Firstly, the spatial resolution of the metapopulation model is relatively coarse, as our computational units correspond to marine sectors with an areal extent of about 48 km2. As customary in spatially explicit modeling applications, the choice of a reference spatial resolution represents a trade-off between accuracy, on the one hand, and robustness and applicability, on the other. Needless to say, this choice must also necessarily consider the spatial extent of the study area (in this case, the whole coastal region of the Mediterranean basin) and the temporal scale of the process of interest (in this case, multi-decadal metapopulation dynamics). A higher-resolution model would in fact allow us to more accurately represent local demographic dynamics; however, it would prove harder to parameterize (hence, arguably, less robust) and simulate at the whole-Mediterranean and multi-decadal scale that the current study focuses on. While the latter issue is purely numerical and could be tackled by deploying enough computational power, the former remains difficult to address because of the fragmented availability of high-resolution data of consistent quality across the Mediterranean basin concerning both the ecology of P. oceanica and the circulation patterns of marine currents. Nevertheless, we acknowledge that a higher-resolution model may be more appropriate for fine-scale assessments of meadow-scale P. oceanica metapopulation dynamics. In that case, though, a different metapopulation approach should be used, one in which meadows are described as individual patches, whose size and shape can change over time because of clonal growth (Arnaud-Haond et al., 2012), and that are linked by hydrodynamic connectivity through fruit dispersal and, possibly, other mechanisms that might be relevant at this scale, such as seed resuspension (McMahon et al., 2014) and the exchange of vegetative fragments (Di Carlo et al., 2005). At an even higher spatial resolution, seagrass clonal dynamics may also be described with spatially explicit, individual-based models in which each shoot (or each branch) represents an interacting agent (Kendrick et al., 2005; Sintes et al., 2005), or with integro-differential models (Ruiz-Reynés et al., 2017).
Another limitation of the present work is that of having disregarded temporal trends in both habitat suitability and current-driven connectivity. Indeed, evidence exists that P. oceanica populations have been regressing in the Mediterranean over the past decades (Telesca et al., 2015), and projections suggest that the localized effects of global climate change might exacerbate this trajectory of change in decades to come, with worst-case scenarios projecting a 75% loss by 2050 and functional extinction by the end of the century (Chefaoui et al., 2018). The warming of the marine environment is currently considered one of the most severe threats for seagrasses, including P. oceanica (Duarte et al., 2018). Thermal stress may have multiple negative effects on P. oceanica, including increased shoot mortality, limited growth, faster leaf senescence, and reduced seedling germination (Marbà and Duarte, 2010; Guerrero-Meseguer et al., 2017). However, the observation that rising temperatures may affect the phenology of P. oceanica, with non-trivial consequences for both individual plants and meadows (Peirano et al., 2011), and the high plasticity of the species (González-Correa et al., 2007b), possibly leading to higher rates of sexual reproduction (Balestri et al., 2017), increase the uncertainty of climate-related projections. Among the other factors related to global climate change, enhanced stratification (Coma et al., 2009), sea-level rise (Pergent et al., 2015), meteorological extremes (Guerrero-Meseguer et al., 2017), and water acidification (Ravaglioli et al., 2017) are all expected to play a role in determining the future suitability of the Mediterranean coasts for P. oceanica, albeit with localized and likely contrasting directions of change. Climate change is also projected to have an impact on water circulation in the Mediterranean Sea (Adloff et al., 2015), with possible implications for ecological connectivity and, ultimately, conservation (Coleman et al., 2017). In this context, the ever-expanding availability of Earth Observation data may be used in conjunction with species distribution modeling to project the future distribution patterns of P. oceanica, thus also informing large-scale conservation programs (Pasetto et al., 2018).
From a conservation perspective, our modeling results suggest that P. oceanica ecological hotspots are quite unevenly distributed along the Mediterranean coastline. We found that the region centered in the Gulf of Gabès plays an especially important role in the large-scale metapopulation dynamics of the species. Interestingly, the genetic diversity of P. oceanica is significantly higher in this region than anywhere else (Arnaud-Haond et al., 2007), suggesting the existence of a contact zone between the otherwise genetically disconnected populations of P. oceanica inhabiting the Western and Eastern basins of the Mediterranean Sea (Serra et al., 2010). As a matter of fact, high population density, strong connectivity and long-distance dispersal are all ingredients that are expected to favor population connectivity and, as a consequence, effective gene flow. Genetic diversity is one of the factors contributing to the capacity of a species to cope with climate change (Dawson et al., 2011). Therefore, preserving genetic diversity should be a priority target in the conservation of P. oceanica, given the bleak road ahead projected for Mediterranean seagrasses subject to regional climate change (Jordà et al., 2012; Chefaoui et al., 2018).
Marine protected areas seem to be an effective tool for the conservation of P. oceanica. Some field studies have in fact found no evidence of meadow decline in several protected marine sites, in stark contrast with the average trajectory of change (González-Correa et al., 2007b). In this regard, our analysis has shown that a considerable spatial gap in protection may exist for Mediterranean seagrass populations. In fact, while many of the lesser P. oceanica hotspots laying along the European coasts occur within or close to marine protected areas, virtually all of the crucial North-African ones are located hundreds of kilometers away from the closest sites of marine protection. This disparity could indeed represent an issue for the conservation of the species, which would require protection plans to be devised at a pan-Mediterranean spatial scale. Clearly, legal protection is not enough to safeguard P. oceanica populations, as field observations also suggest that deep meadows in marine protected areas may be not healthier than those in unprotected areas (Montefalcone et al., 2009). As an example, ineffective management of protected areas, especially concerning boat anchoring, can have detrimental consequences for P. oceanica meadows (e.g., La Manna et al., 2015).
The identification of a clear spatial protection gap should raise the question of how to strategically coordinate conservation efforts, possibly in a trans-boundary way (Katsanevakis et al., 2015), to ensure that resources are allocated where they are most likely to be effective (McCarthy et al., 2010). Designing protection at the scale of the whole Mediterranean Sea is clearly a major challenge, not only from an ecological point of view, but also from social, political, and economic perspectives. Twenty-one countries in three continents face the Mediterranean, with diverse human populations including many ethnic and cultural groups and, often, complicated political trajectories (Pergent et al., 2017). Proposed international plans for the expansion of Mediterranean protection are quite diverse in the underlying technical approaches, conservation goals, spatial extents, and geographic locations; however, consensus exists among different plans about some key Mediterranean regions accounting for about 10% of the areal extent of the basin (Micheli et al., 2013). Specifically, large-scale plans most often focus geographically on the Alboran Sea, the Balearic Archipelago, the Ligurian Sea, the region extending from the Gulf of Gabès to the Sicily Channel through the Tunisian Plateau, and some stretches of the Adriatic, Ionian, Aegean, and Levantine Seas, with small overlaps (mostly limited to the Ligurian Sea) with existing protected areas. Notably, most of the marine regions that we have identified as possible P. oceanica hotspots are indeed comprised within this list, suggesting that further synergies in large-scale protection planning may be possible.
In conclusion, in this work we have shown how a relatively simple metapopulation model, fed with georeferenced information from habitat distribution modeling and Earth Observations, can be used to integrate demographic and dispersal processes to analyze the spatiotemporal dynamics of an important foundation species, the endemic seagrass P. oceanica, at the scale of the whole Mediterranean Sea. Results from metapopulation modeling can then be used to identify key targets for international protection based on reproducible and quantitative procedures, thus contributing to a much-needed prioritization of conservation actions in the Mediterranean large marine ecosystem. Looking ahead, trying to anticipate how changing environmental conditions and increasing human pressure will ultimately affect P. oceanica ecological hotspots in the Mediterranean Sea is made difficult at present by the presence of multiple uncertainty sources. Our study suggests that any such predictions ought to be based on a reliable framework for the description of basin-scale and long-term metapopulation dynamics.
Data Availability Statement
The datasets presented in this study can be found in online repositories. The relevant web links are given in the text. The data and the code to perform numerical simulations of the metapopulation model are available at: https://github.com/lorenzo-mari/posidonia-metapop.
Author Contributions
LM performed the simulations and data analysis. All authors contributed to designing the experiments, interpreting the results, and drafting the manuscript, and read and approved the submitted version.
Funding
The authors acknowledge support from the European Union's Horizon 2020 research and innovation program through the ECOPOTENTIAL project, grant agreement No. 641762.
Conflict of Interest
The authors declare that the research was conducted in the absence of any commercial or financial relationships that could be construed as a potential conflict of interest.
Acknowledgments
The authors wish to thank Simonetta Fraschetti for useful discussions on the metapopulation dynamics of P. oceanica.
Supplementary Material
The Supplementary Material for this article can be found online at: https://www.frontiersin.org/articles/10.3389/fmars.2021.628976/full#supplementary-material
References
Adloff, F., Somot, S., Sevault, F., Jordà, G., Aznar, R., Déqué, M., et al. (2015). Mediterranean Sea response to climate change in an ensemble of twenty first century scenarios. Clim. Dyn. 45, 2775–2802. doi: 10.1007/s00382-015-2507-3
Almela, E. D., Marba, N., Alvarez, E., Santiago, R., Martínez, R., and Duarte, C. M. (2008). Patch dynamics of the Mediterranean seagrass Posidonia oceanica: implications for recolonisation process. Aquat. Bot. 89, 397–403. doi: 10.1016/j.aquabot.2008.04.012
Arnaud-Haond, S., Duarte, C. M., Diaz-Almela, E., Marbà, N., Sintes, T., and Serrão, E. A. (2012). Implications of extreme life span in clonal organisms: millenary clones in meadows of the threatened seagrass Posidonia oceanica. PLoS ONE 7:e30454. doi: 10.1371/journal.pone.0030454
Arnaud-Haond, S., Migliaccio, M., Diaz-Almela, E., Teixeira, S., Van De Vliet, M. S., Alberto, F., et al. (2007). Vicariance patterns in the Mediterranean Sea: east-west cleavage and low dispersal in the endemic seagrass Posidonia oceanica. J. Biogeogr. 34, 963–976. doi: 10.1111/j.1365-2699.2006.01671.x
Badalamenti, F., Di Carlo, G., D'Anna, G., Gristina, M., and Toccaceli, M. (2006). Effects of dredging activities on population dynamics of Posidonia oceanica (L.) Delile in the Mediterranean sea: the case study of Capo Feto (SW Sicily, Italy), Hydrobiologia 555, 253–261. doi: 10.1007/s10750-005-1121-5
Baker, S. G., and Kramer, B. S. (2007). Peirce, Youden, and receiver operating characteristic curves. Am. Stat. 61, 343–346. doi: 10.1198/000313007X247643
Balestri, E., and Bertini, S. (2003). Growth and development of Posidonia oceanica seedlings treated with plant growth regulators: possible implications for meadow restoration. Aquat. Bot. 76, 291–297. doi: 10.1016/S0304-3770(03)00074-3
Balestri, E., and Cinelli, F. (2003). Sexual reproductive success in Posidonia oceanica. Aquat. Bot. 75, 21–32. doi: 10.1016/S0304-3770(02)00151-1
Balestri, E., and Lardicci, C. (2008). First evidence of a massive recruitment event in Posidonia oceanica: spatial variation in first-year seedling abundance on a heterogeneous substrate. Estuar. Coast. Shelf Sci. 76, 634–641. doi: 10.1016/j.ecss.2007.07.048
Balestri, E., Piazzi, L., and Cinelli, F. (1998a). In vitro germination and seedling development of Posidonia oceanica. Aquat. Bot. 60, 83–93. doi: 10.1016/S0304-3770(97)00017-X
Balestri, E., Piazzi, L., and Cinelli, F. (1998b). Survival and growth of transplanted and natural seedlings of Posidonia oceanica (L.) Delile in a damaged coastal area. J. Exp. Mar. Biol. Ecol. 228, 209–225. doi: 10.1016/S0022-0981(98)00027-6
Balestri, E., and Vallerini, F. (2003). Interannual variability in flowering of Posidonia oceanica in the North-Western Mediterranean Sea, and relationships among shoot age and flowering. Bot. Mar. 46, 525–530. doi: 10.1515/BOT.2003.054
Balestri, E., Vallerini, F., and Lardicci, C. (2017). Recruitment and patch establishment by seed in the seagrass Posidonia oceanica: importance and conservation implications. Front. Plant Sci. 8:1067. doi: 10.3389/fpls.2017.01067
Bell, S. S. (2006). “Seagrasses and the metapopulation concept: developing a regional approach to the study of extinction, colonization, and dispersal,” in Marine Metapopulations, eds J. P. Kritzer and P. F. Sale (Burlington, MA: Elsevier Academic Press), 387–407. doi: 10.1016/B978-012088781-1/50014-5
Beverton, R. J., and Holt, S. J. (1957). On the Dynamics of Exploited Fish Populations. Dordrecht: Springer Science & Business Media.
Boudouresque, C. F., Bernard, G., Bonhomme, P., Charbonnel, E., Diviacco, G., Meinesz, A., et al. (2012). Protection and Conservation of Posidonia oceanica Meadows. Tunis: RAMOGE and RAC/SPA.
Calvo, S., Lovison, G., Pirrotta, M., Di Maida, G., Tomasello, A., and Sciandra, M. (2006). Modelling the relationship between sexual reproduction and rhizome growth in Posidonia oceanica (L.) Delile. Mar. Ecol. 27, 361–371. doi: 10.1111/j.1439-0485.2006.00132.x
Campagne, C. S., Salles, J.-M., Boissery, P., and Deter, J. (2015). The seagrass Posidonia oceanica: ecosystem services identification and economic evaluation of goods and benefits. Mar. Pollut. Bull. 97, 391–400. doi: 10.1016/j.marpolbul.2015.05.061
Chefaoui, R. M., Duarte, C. M., and Serrão, E. A. (2018). Dramatic loss of seagrass habitat under projected climate change in the Mediterranean Sea. Glob. Change Biol. 24, 4919–4928. doi: 10.1111/gcb.14401
Coleman, M. A., Cetina-Heredia, P., Roughan, M., Feng, M., van Sebille, E., and Kelaher, B. P. (2017). Anticipating changes to future connectivity within a network of marine protected areas. Glob. Change Biol. 23, 3533–3542. doi: 10.1111/gcb.13634
Coma, R., Ribes, M., Serrano, E., Jiménez, E., Salat, J., and Pascual, J. (2009). Global warming-enhanced stratification and mass mortality events in the Mediterranean. Proc. Natl. Acad. Sci. U.S.A. 106, 6176–6181. doi: 10.1073/pnas.0805801106
Dawson, T. P., Jackson, S. T., House, J. I., Prentice, I. C., and Mace, G. M. (2011). Beyond predictions: biodiversity conservation in a changing climate. Science 332, 53–58. doi: 10.1126/science.1200303
Di Carlo, G., Badalamenti, F., Jensen, A., Koch, E., and Riggio, S. (2005). Colonisation process of vegetative fragments of Posidonia oceanica (L.) Delile on rubble mounds. Mar. Biol. 147, 1261–1270. doi: 10.1007/s00227-005-0035-0
Duarte, B., Martins, I., Rosa, R., Matos, A. R., Roleda, M. Y., Reusch, T. B., et al. (2018). Climate change impacts on seagrass meadows and macroalgal forests: an integrative perspective on acclimation and adaptation potential. Front. Mar. Sci. 5:190. doi: 10.3389/fmars.2018.00190
Duarte, C. M., Dennison, W. C., Orth, R. J., and Carruthers, T. J. (2008). The charisma of coastal ecosystems: addressing the imbalance. Estuar. Coasts 31, 233–238. doi: 10.1007/s12237-008-9038-7
Duarte, C. M., Fourqurean, J. W., Krause-Jensen, D., and Olesen, B. (2006). “Dynamics of seagrass stability and change,” in Seagrasses: Biology, Ecology and Conservation, eds A. W. D. Larkum, R. J. Orth, and C. M. Duarte (Dordrecht: Springer), 271–294. doi: 10.1007/1-4020-2983-7_11
Ellison, A. M. (2019). Foundation species, non-trophic interactions, and the value of being common. iScience 13, 254–268. doi: 10.1016/j.isci.2019.02.020
Fernández-Torquemada, Y., and Sánchez-Lizaso, J. L. (2013). Effects of salinity on seed germination and early seedling growth of the Mediterranean seagrass Posidonia oceanica (L.) Delile. Estuar. Coast. Shelf Sci. 119, 64–70. doi: 10.1016/j.ecss.2012.12.013
Giannoulaki, M., Belluscio, A., Colloca, F., Fraschetti, S., Scardi, M., Smith, C., et al. (2013). Mediterranean Sensitive Habitats. DG MARE Specific Contract SI2.600741. Technical report. Available online at: https://imbriw.hcmr.gr/en/wp-content/uploads/2013/12/MEDISEH-final-report-reduced.pdf
Gobert, S., Cambridge, M. L., Velimirov, B., Pergent, G., Lepoint, G., Bouquegneau, J.-M., et al. (2006). “Biology of Posidonia,” in Seagrasses: Biology, Ecology and Conservation, eds A. W. D. Larkum, R. J. Orth, and C. M. Duarte (Dordrecht: Springer), 387–408. doi: 10.1007/1-4020-2983-7_17
González-Correa, J. M., Fernández-Torquemada, Y., and Sánchez-Lizaso, J. L. (2007a). Comparing shoot population dynamics methods on Posidonia oceanica meadows. J. Exp. Mar. Biol. Ecol. 353, 115–125. doi: 10.1016/j.jembe.2007.09.008
González-Correa, J. M., Sempere, J. T. B., Sánchez-Jerez, P., and Valle, C. (2007b). Posidonia oceanica meadows are not declining globally. Analysis of population dynamics in marine protected areas of the Mediterranean Sea. Mar. Ecol. Prog. Ser. 336, 111–119. doi: 10.3354/meps336111
Guerrero-Meseguer, L., Marín, A., and Sanz-Lázaro, C. (2017). Future heat waves due to climate change threaten the survival of Posidonia oceanica seedlings. Environ. Pollut. 230, 40–45. doi: 10.1016/j.envpol.2017.06.039
Houngnandan, F., Kéfi, S., and Deter, J. (2020). Identifying key-conservation areas for Posidonia oceanica seagrass beds. Biol. Conserv. 247:108546. doi: 10.1016/j.biocon.2020.108546
Jahnke, M., Casagrandi, R., Melià, P., Schiavina, M., Schultz, S. T., Zane, L., et al. (2017). Potential and realized connectivity of the seagrass Posidonia oceanica and their implication for conservation. Divers. Distrib. 23, 1423–1434. doi: 10.1111/ddi.12633
Jordà, G., Marbà, N., and Duarte, C. M. (2012). Mediterranean seagrass vulnerable to regional climate warming. Nat. Clim. Change 2, 821–824. doi: 10.1038/nclimate1533
Katsanevakis, S., Levin, N., Coll, M., Giakoumi, S., Shkedi, D., Mackelworth, P., et al. (2015). Marine conservation challenges in an era of economic crisis and geopolitical instability: the case of the Mediterranean Sea. Mar. Policy 51, 31–39. doi: 10.1016/j.marpol.2014.07.013
Kendrick, G. A., Marbà, N., and Duarte, C. M. (2005). Modelling formation of complex topography by the seagrass Posidonia oceanica. Estuar. Coast. Shelf Sci. 65, 717–725. doi: 10.1016/j.ecss.2005.07.007
La Manna, G., Donno, Y., Sarà, G., and Ceccherelli, G. (2015). The detrimental consequences for seagrass of ineffective marine park management related to boat anchoring. Mar. Pollut. Bull. 90, 160–166. doi: 10.1016/j.marpolbul.2014.11.001
Lecci, R., Fratianni, C., Drudi, M., and Grandi, A. (2017). Mediterranean Sea Physical Reanalysis: Product User Manual. Technical report. Available online at: https://cmems-resources.cls.fr/documents/PUM/CMEMS-MED-PUM-006–004.pdf
Marbà, N., and Duarte, C. M. (2010). Mediterranean warming triggers seagrass (Posidonia oceanica) shoot mortality. Glob. Change Biol. 16, 2366–2375. doi: 10.1111/j.1365-2486.2009.02130.x
Marbà, N., Duarte, C. M., Cebrián, J., Gallegos, M. E., Olesen, B., and Sand-Jensen, K. (1996). Growth and population dynamics of Posidonia oceanica on the Spanish Mediterranean coast: elucidating seagrass decline. Mar. Ecol. Prog. Ser. 137, 203–213. doi: 10.3354/meps137203
Marbà, N., Duarte, C. M., Díaz-Almela, E., Terrados, J., Álvarez, E., Martínez, R., et al. (2005). Direct evidence of imbalanced seagrass Posidonia oceanica shoot population dynamics in the Spanish Mediterranean. Estuaries 28, 53–62. doi: 10.1007/BF02732753
Mari, L., Melià, P., Fraschetti, S., Gatto, M., and Casagrandi, R. (2020). Recent trends of changing seagrass connectivity in the Mediterranean Sea. Divers. Distrib. 26, 169–182. doi: 10.1111/ddi.12998
Marine Conservation Institute (2019). MPAtlas. Seattle, WA. Available online at: www.mpatlas.org (accessed May 7, 2019).
McCarthy, M. A., Thompson, C. J., Hauser, C., Burgman, M. A., Possingham, H. P., Moir, M. L., et al. (2010). Resource allocation for efficient environmental management. Ecol. Lett. 13, 1280–1289. doi: 10.1111/j.1461-0248.2010.01522.x
McMahon, K., van Dijk, K., Ruiz-Montoya, L., Kendrick, G. A., Krauss, S. L., Waycott, M., et al. (2014). The movement ecology of seagrasses. Proc. R. Soc. B Biol. Sci. 281:20140878. doi: 10.1098/rspb.2014.0878
Melià, P., Schiavina, M., Rossetto, M., Gatto, M., Fraschetti, S., and Casagrandi, R. (2016). Looking for hotspots of marine metacommunity connectivity: a methodological framework. Sci. Rep. 6:23705. doi: 10.1038/srep23705
Micheli, F., Levin, N., Giakoumi, S., Katsanevakis, S., Abdulla, A., Coll, M., et al. (2013). Setting priorities for regional conservation planning in the Mediterranean Sea. PLoS ONE 8:e59038. doi: 10.1371/journal.pone.0059038
Montefalcone, M., Albertelli, G., Morri, C., Parravicini, V., and Bianchi, C. N. (2009). Legal protection is not enough: Posidonia oceanica meadows in marine protected areas are not healthier than those in unprotected areas of the northwest Mediterranean sea. Mar. Pollut. Bull. 58, 515–519. doi: 10.1016/j.marpolbul.2008.12.001
Myers, N., Mittermeier, R. A., Mittermeier, C. G., Da Fonseca, G. A., and Kent, J. (2000). Biodiversity hotspots for conservation priorities. Nature 403, 853–858. doi: 10.1038/35002501
Nordlund, L. M., Koch, E. W., Barbier, E. B., and Creed, J. C. (2016). Seagrass ecosystem services and their variability across genera and geographical regions. PLoS ONE 11:e0163091. doi: 10.1371/journal.pone.0163091
Orth, R. J., Fishman, J. R., Harwell, M. C., and Marion, S. R. (2003). Seed-density effects on germination and initial seedling establishment in eelgrass Zostera marina in the Chesapeake Bay region. Mar. Ecol. Prog. Ser. 250, 71–79. doi: 10.3354/meps250071
Orth, R. J., Harwell, M. C., and Inglis, G. J. (2006). “Ecology of seagrass seeds and seagrass dispersal processes,” in Seagrasses: Biology, Ecology and Conservation, eds A. W. D. Larkum, R. J. Orth, and C. M. Duarte (Dordrecht: Springer), 111–134. doi: 10.1007/1-4020-2983-7_5
Pasetto, D., Arenas-Castro, S., Bustamante, J., Casagrandi, R., Chrysoulakis, N., Cord, A. F., et al. (2018). Integration of satellite remote sensing data in ecosystem modelling at local scales: practices and trends. Methods Ecol. Evol. 9, 1810–1821. doi: 10.1111/2041-210X.13018
Peirano, A., Cocito, S., Banfi, V., Cupido, R., Damasso, V., Farina, G., et al. (2011). Phenology of the Mediterranean seagrass Posidonia oceanica (L.) Delile: medium and long-term cycles and climate inferences. Aquat. Bot. 94, 77–92. doi: 10.1016/j.aquabot.2010.11.007
Pergent, G., Gerakaris, V., Sghaier, Y., Zakhama-Sraier, R., Fernández Torquemada, Y., and Pergent-Martini, C. (2016). Posidonia oceanica (Errata Version Published in 2018). The IUCN red list of threatened species 2016:e.t153534a135156882. Available online at: https://www.iucnredlist.org/species/153534/135156882
Pergent, G., Gerakaris, V., Sghaier, Y., Zakhama-Sraier, R., Fernández Torquemada, Y., and Pergent-Martini, C. (2017). 2017 Mediterranean Quality Status Report. Available online at: https://www.medqsr.org/sites/default/files/inline-files/2017MedQSR_Online_0.pdf
Pergent, G., Pergent-Martini, C., Bein, A., Dedeken, M., Oberti, P., Orsini, A., et al. (2015). Dynamic of Posidonia oceanica seagrass meadows in the northwestern Mediterranean: could climate change be to blame? Comptes Rendus Biol. 338, 484–493. doi: 10.1016/j.crvi.2015.04.011
Pergent-Martini, C. (2015). Guidelines for standardization of mapping and monitoring methods of marine Magnoliophyta in the Mediterranean. Technical report, UNEP/MAP-RAC/SPA. Tunis: RAC/SPA Publ., 48 p. + Annexes.
Piazzi, L., Acunto, S., and Cinelli, F. (1999). In situ survival and development of Posidonia oceanica (L.) Delile seedlings. Aquat. Bot. 63, 103–112. doi: 10.1016/S0304-3770(98)00115-6
Procaccini, G., Orsini, L., Ruggiero, M., and Scardi, M. (2001). Spatial patterns of genetic diversity in Posidonia oceanica, an endemic Mediterranean seagrass. Mol. Ecol. 10, 1413–1421. doi: 10.1046/j.1365-294X.2001.01290.x
Ravaglioli, C., Lauritano, C., Buia, M. C., Balestri, E., Capocchi, A., Fontanini, D., et al. (2017). Nutrient loading fosters seagrass productivity under ocean acidification. Sci. Rep. 7:13732. doi: 10.1038/s41598-017-14075-8
Rozenfeld, A. F., Arnaud-Haond, S., Hernández-García, E., Eguíluz, V. M., Serr ao, E. A., and Duarte, C. M. (2008). Network analysis identifies weak and strong links in a metapopulation system. Proc. Natl. Acad. Sci. U.S.A. 105, 18824–18829. doi: 10.1073/pnas.0805571105
Ruiz-Reynés, D., Gomila, D., Sintes, T., Hernández-García, E., Marbà, N., and Duarte, C. M. (2017). Fairy circle landscapes under the sea. Sci. Adv. 3:e1603262. doi: 10.1126/sciadv.1603262
Schisterman, E. F., Perkins, N. J., Liu, A., and Bondell, H. (2005). Optimal cut-point and its corresponding Youden Index to discriminate individuals using pooled blood samples. Epidemiology 16, 73–81. doi: 10.1097/01.ede.0000147512.81966.ba
Serra, I., Innocenti, A., Di Maida, G., Calvo, S., Migliaccio, M., Zambianchi, E., et al. (2010). Genetic structure in the Mediterranean seagrass Posidonia oceanica: disentangling past vicariance events from contemporary patterns of gene flow. Mol. Ecol. 19, 557–568. doi: 10.1111/j.1365-294X.2009.04462.x
Sintes, T., Marba, N., Duarte, C. M., and Kendrick, G. A. (2005). Nonlinear processes in seagrass colonisation explained by simple clonal growth rules. Oikos 108, 165–175. doi: 10.1111/j.0030-1299.2005.13331.x
Statton, J., Montoya, L. R., Orth, R. J., Dixon, K. W., and Kendrick, G. A. (2017). Identifying critical recruitment bottlenecks limiting seedling establishment in a degraded seagrass ecosystem. Sci. Rep. 7:14786. doi: 10.1038/s41598-017-13833-y
Telesca, L., Belluscio, A., Criscoli, A., Ardizzone, G., Apostolaki, E. T., Fraschetti, S., et al. (2015). Seagrass meadows (Posidonia oceanica) distribution and trajectories of change. Sci. Rep. 5:12505. doi: 10.1038/srep12505
Valdez, S. R., Zhang, Y. S., van der Heide, T., Vanderklift, M. A., Tarquinio, F., Orth, R. J., et al. (2020). Positive ecological interactions and the success of seagrass restoration. Front. Mar. Sci. 7:91. doi: 10.3389/fmars.2020.00091
van Katwijk, M. M., Thorhaug, A., Marbà, N., Orth, R. J., Duarte, C. M., Kendrick, G. A., et al. (2016). Global analysis of seagrass restoration: the importance of large-scale planting. J. Appl. Ecol. 53, 567–578. doi: 10.1111/1365-2664.12562
Keywords: metapopulation dynamics, seagrass, realized connectivity, ecological hotspots, protection gaps, prioritization strategies
Citation: Mari L, Melià P, Gatto M and Casagrandi R (2021) Identification of Ecological Hotspots for the Seagrass Posidonia oceanica via Metapopulation Modeling. Front. Mar. Sci. 8:628976. doi: 10.3389/fmars.2021.628976
Received: 13 November 2020; Accepted: 06 April 2021;
Published: 13 May 2021.
Edited by:
Alberto Basset, University of Salento, ItalyReviewed by:
Giulia Ceccherelli, University of Sassari, ItalyJennifer Joan Verduin, Murdoch University, Australia
Copyright © 2021 Mari, Melià, Gatto and Casagrandi. This is an open-access article distributed under the terms of the Creative Commons Attribution License (CC BY). The use, distribution or reproduction in other forums is permitted, provided the original author(s) and the copyright owner(s) are credited and that the original publication in this journal is cited, in accordance with accepted academic practice. No use, distribution or reproduction is permitted which does not comply with these terms.
*Correspondence: Lorenzo Mari, bG9yZW56by5tYXJpQHBvbGltaS5pdA==