- 1Animal Sciences Unit – Aquatic Environment and Quality, Flanders Research Institute for Agriculture, Fisheries and Food, Oostende, Belgium
- 2Marine Biology Section, Department of Biology, Ghent University, Ghent, Belgium
- 3Animal Sciences Unit, Flanders Research Institute for Agriculture, Fisheries and Food, Melle, Belgium
- 4Plant Sciences Unit, Flanders Research Institute for Agriculture, Fisheries and Food, Melle, Belgium
DNA metabarcoding is a promising method to increase cost and time efficiency of marine monitoring. While substantial evidence exists that bulk DNA samples adequately reflect diversity patterns of marine macrobenthos, the potential of eDNA in the ethanol preservative of benthic samples for biodiversity monitoring remains largely unexplored. We investigated species detection in bulk DNA and eDNA from the ethanol preservative in samples from four distinct macrobenthic communities in the North Sea. Bulk DNA and eDNA were extracted with different extraction kits and five COI primer sets were tested. Despite the availability of a nearly complete reference database, at most 22% of the amplicon sequence variants (ASVs) were assigned taxonomy at the phylum level. However, the unassigned ASVs represented only a small fraction of the total reads (13%). The Leray primer set outperformed the four other primer sets in the number of non-chimeric reads and species detected, and in the recovery of beta diversity patterns. Community composition differed significantly between bulk DNA and eDNA samples, but both sample types were able to differentiate the four communities. The probability of detecting a species in the eDNA from the ethanol preservative was significantly lower than for bulk DNA for macrobenthos species having small to medium body size and for species having chitine or CaCO3 in their cuticula. Detection in the bulk DNA samples was not affected by the investigated morphological traits, indicating that monitoring of macrobenthos species will be most robust when using bulk DNA as template for metabarcoding.
Introduction
The characterization of benthic diversity relies mostly on morphological taxonomy, a slow and expensive process due to manual sorting and visual identification of taxa in the samples. The development of innovative, cost-effective monitoring tools that allow a quick screening of benthic diversity in large marine areas is therefore needed (Borja et al., 2016; Elliott et al., 2018). DNA metabarcoding is a promising tool for monitoring benthic environments (Leray and Knowlton, 2015; Aylagas et al., 2016a; Elbrecht et al., 2017b; Aylagas et al., 2018) and for the early detection of non-indigenous species (Rey et al., 2020).
Instead of identifying all specimens morphologically, DNA is extracted from the total community, a short fragment of the genome is amplified through PCR, the resulting library is sequenced using high throughput sequencing and finally, the resulting sequences are processed through bioinformatic pipelines (Pawlowski et al., 2018). Each of these steps may introduce bias and errors (Alberdi et al., 2018), while the final detection of a particular species in the sample largely depends on its biomass and the primers used in the DNA metabarcoding protocol (Elbrecht et al., 2017a; Lobo et al., 2017).
Next to primer choice, the DNA source used in metabarcoding studies also affect whether or not a species is detected. DNA can be extracted from bulk specimens that have been separated from the sediment by sieving, decantation and manual sorting (Aylagas et al., 2016b) or from the ethanol preservative in which the sieved macrobenthos sample was preserved (Zizka et al., 2019b). Bulk DNA samples may further be sorted in different size fractions to enhance detection of smaller sized animals (Leray and Knowlton, 2015; Elbrecht et al., 2017a), which comes at an extra time cost to process samples.
Extracting DNA from the ethanol preservative avoids the time consuming step of having to sort specimens and has the added advantage that voucher specimens are still intact after the analyses. Importantly, preservation choice influences DNA extraction method choice, with chemical lysis of cell and tissues being the recommended method for characterizing eukaryotic diversity in the eDNA (Deiner et al., 2015). When blending specimens for bulk DNA extraction, sediment from the intestine of macrobenthos species may enter the ‘soup’ and DNA extraction kits that also allow removal of inhibiting substances associated with the sediment should be used.
Non-target species such as bacteria and fungi may be encountered more when analyzing the ethanol preservative compared to bulk samples (Gauthier et al., 2020). A comparison between bulk and ethanol samples for freshwater invertebrates showed that the two approaches yield very different community compositions (Marquina et al., 2019; Zizka et al., 2019b). Nevertheless, DNA metabarcoding of the ethanol fraction has also shown considerable overlap with morphology based analyses of freshwater communities, illustrating its potential for monitoring studies (Martins et al., 2019).
In view of the above mentioned advantages when using the ethanol preservative, understanding the different results between bulk DNA and eDNA from the ethanol preservative is essential to determine their applicability for monitoring studies in the marine environment. For instance, it has been shown that small and weakly sclerotized freshwater insects species are overrepresented in the ethanol preservative while large and strongly sclerotized species are overrepresented in the bulk DNA (Marquina et al., 2019). Consequently, morphological traits of species seem to be important to explain their detection in DNA metabarcoding studies, but this link has hitherto not been investigated for marine macrobenthos.
In this study, we sampled four distinct macrobenthic communities in the Belgian part of the North Sea and identified them using traditional morpho-taxonomy before molecular processing. We generated 104 COI reference sequences from macrobenthos species to allow taxonomic assignment of the metabarcode datasets. We evaluated whether eDNA from the ethanol preservative could be used for monitoring by comparing alpha and beta diversity patterns with those observed in bulk DNA. Since different primer sets can lead to different diversity estimates (Elbrecht and Leese, 2017; Lobo et al., 2017; Braukmann et al., 2019), we evaluated the capacity of five COI primer sets from the literature to capture the morphological species in bulk DNA and eDNA from the ethanol preservative. Subsequently, we investigated whether morphological and ecological species traits could explain the probability of detecting macrobenthos species in the bulk DNA and eDNA from the ethanol preservative. This study provides a thorough evaluation and understanding of using eDNA from the ethanol preservative for monitoring marine macrobenthos.
Materials and Methods
Sample Collection
Samples were collected in the Belgian part of the North Sea (BPNS), a relatively small area (ca. 3,600 km2) subjected to many human activities (Douvere et al., 2007). The area has been extensively monitored for almost 20 years, resulting in well described macrobenthic communities (Van Hoey et al., 2004; Degraer et al., 2008; Breine et al., 2018). In autumn 2017 we sampled four different habitats characterized by distinct macrobenthic communities (Figure 1): (1) a fine muddy habitat (ZVL) with low diversity and low abundances with mainly sessile burrowing dwelling species (the Limecola balthica community); (2) a coastal fine sand habitat (120), characterized by high diversity and high abundances with mainly sessile, tube building burrowers (the Abra alba community), (3) a medium sandy habitat (330) with low taxon richness and low abundances (the Nephtys cirrosa community) and (4) an offshore coarse sandy habitat (840), with medium diversity and low abundances (the Hesionura elongata community). The latter two habitats are characterized by relatively small, free-living and burrowing macrobenthos species. In each of the four communities, three replicate Van Veen grabs (A, B, and C) were collected on board of the RV Belgica. The sediment was sieved on board on a 1 mm sieve. All material (macrobenthos specimens + residue) that remained on the sieve was fixed in absolute ethanol, and stored at −20°C until further processing.
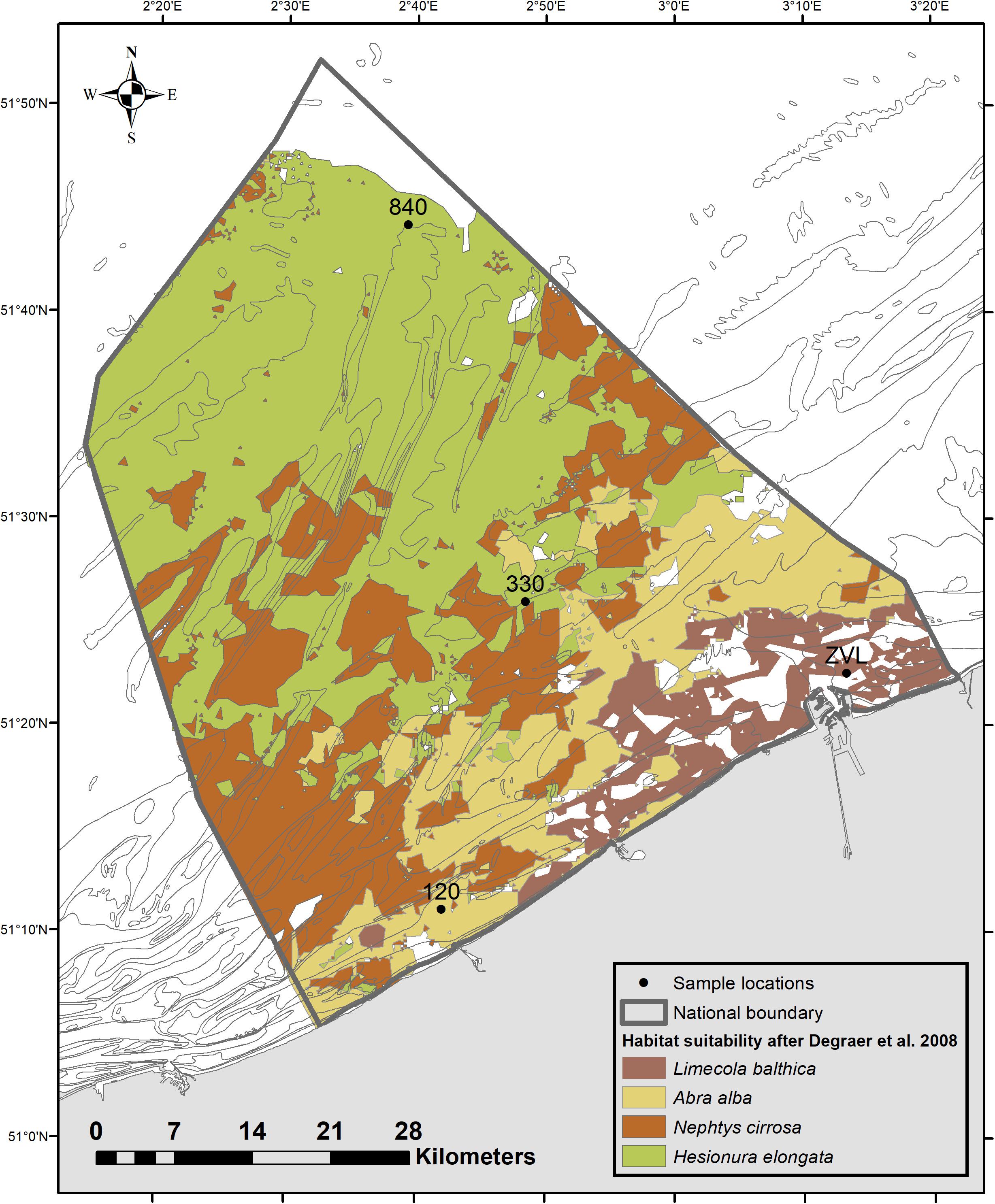
Figure 1. Overview map with sampling locations for this study in the Belgian part of the North Sea. Location 120: coastal fine sandy habitat; Location 330: medium sandy habitat; Location ZVL: fine muddy habitat; Location 840: offshore coarse sandy habitat. Colors indicate the distribution of the macrobenthic communities, white areas are undefined (after Degraer et al., 2008).
Sample Processing
After storage of ca. 8 months at −20°C, the 12 samples were shaken and mixed with a spoon to suspend DNA, after which two times 200 ml ethanol was filtered using a 100 ml microfunnel unit with a 0.45 μM GN6 Metricel membrane. Filters were stored at −80°C. Subsequently, bulk samples were processed largely following the protocol outlined by Aylagas et al. (2016b). In short, samples were six to 13 times decanted in the lab using tap water. Decanting was repeated until no animals were recovered from the samples. All animals remaining on the 1mm sieve were collected and stored in ethanol. The remaining material after decantation was sorted in a cleaned tray and left animals such as bivalves (which are heavier and as such not decanted) were picked with clean tweezers and added to the decanted material in ethanol.
From each of the four locations, one replicate was identified morphologically (ZVL-A, 120-B, 840-C, and 330-C) to the lowest taxonomic level possible prior to grinding. Specimens were identified up to species level, except for juveniles which were identified to the genus level and for specimens belonging to Nemertea, Anthozoa, and Oligochaeta, which were only identified up to phylum, class and order level, respectively, following the certified protocol for macrobenthos identification (according to ISO16665:2014 and NMBAQCS’s “Guidelines for processing marine macrobenthic samples: a Processing Requirements Protocol” Version 1.0). In total, 24 samples were used for further molecular processing (12 ethanol samples and 12 bulk samples).
DNA Extraction
For the bulk samples, specimens and ethanol were mixed into a homogeneous solution using a blender or, in case less than 100 ml sample was available, grinded with mortar and pestle. Depending on the volume of the sample, two (for samples <100 ml) or six (for samples >100 ml, this was the case for the three replicates of station 120) subsamples of 2 ml were transferred to an Eppendorf tube for DNA extraction. The remaining “soup” was stored at −20°C. The Eppendorf tubes with the 2 ml “soup” were centrifuged and the supernatant was removed. To remove the remaining ethanol, the Eppendorf tubes were incubated at 50°C with open lid until the pellet was dry. The pellet was then used to extract DNA using the DNeasy PowerSoil Kit (QIAGEN) following the manufacturer’s protocol. The lysis step was done by adding 10 μl of Proteinase K (20 mg/ml) and incubating overnight in a thermal shaker at 56°C and 1,000 rpm. The same volume of each subsample was pooled to obtain an end volume of 50 μL, which was then cleaned with the Wizard DNA Clean-Up System (Promega) according to manufacturer’s protocol. From each of the 12 bulk samples, one DNA extract of 50 μL was obtained.
For the ethanol preservative samples, DNA extractions were performed using the Promega Wizard®SV Genomic DNA Purification System. One filter was cut into six pieces and separately lysed overnight at 55°C in 275 μl lysis buffer. The lysate of two pieces was pooled and the three pooled lysates were processed separately using the manufacturer’s protocol. This resulted in three DNA extracts of 50 μl from one filter for each ethanol sample, which were pooled together. Half of the pooled DNA extract (75 μl) underwent cleanup using the Promega Wizard® DNA Clean-Up System and was eluted in 50 μl TE-buffer following the manufacturer’s protocol.
Library Preparation
We used a two step amplification protocol, which has been shown to be a robust and cost effective method for DNA metabarcoding (Zizka et al., 2019a). Each of the 24 DNA extracts (12 from bulk and 12 from ethanol) were PCR amplified in triplicates. The PCR mix was prepared in 25 μl reactions consisting of 12.5 μl 2× KAPA HiFi Hotstart ReadyMix, 0.75 μl of forward and reverse primer (10 μM), 8.5 μl nuclease free water and 2.5 μl of DNA from the bulk samples. For the eDNA samples from the ethanol preservative, the same mix was prepared but with 6 μl nuclease free water and 5 μL of eDNA. PCR cycling conditions were: initial denaturation for 3 min at 95°C, 35 cycles of denaturation for 30 s at 98°C, annealing for 30 s at 57°C and extension for 30 s at 72°C and a final extension for 1 min at 72°C. The three PCR replicates were pooled and 37.5 μl of the pooled PCR product was purified using 30 μl Clean NGS beads (GC Biotech) according to the manufacturer’s protocol and eluted in 40 μL TE-buffer.
We screened the literature to identify primers that have been successfully used in metabarcoding studies for marine species (Table 1). These primer sets were first tested in silico using EcoPCR (Ficetola et al., 2010) and the MIDORI_UNIQUE_ COI_MARINE_20180221 reference dataset (Machida et al., 2017) (maximum errors set at 3, minimum length of the amplicon set at 100, maximum length of the amplicon set at 900). Six primer sets were then tested in the wetlab, and five primer sets yielded a PCR product for our macrobenthos samples (Table 1). Four primer sets (A, B, C, and E) amplify the 3′ region and one primer set (D) targets the 5′ region of the Folmer region (Folmer et al., 1994) (Table 1). Amplicon length was 313, 481, 421, 230, and 313 bp for primer sets A, B, C, D, and E, respectively. All 24 samples were amplified with the five primer sets.
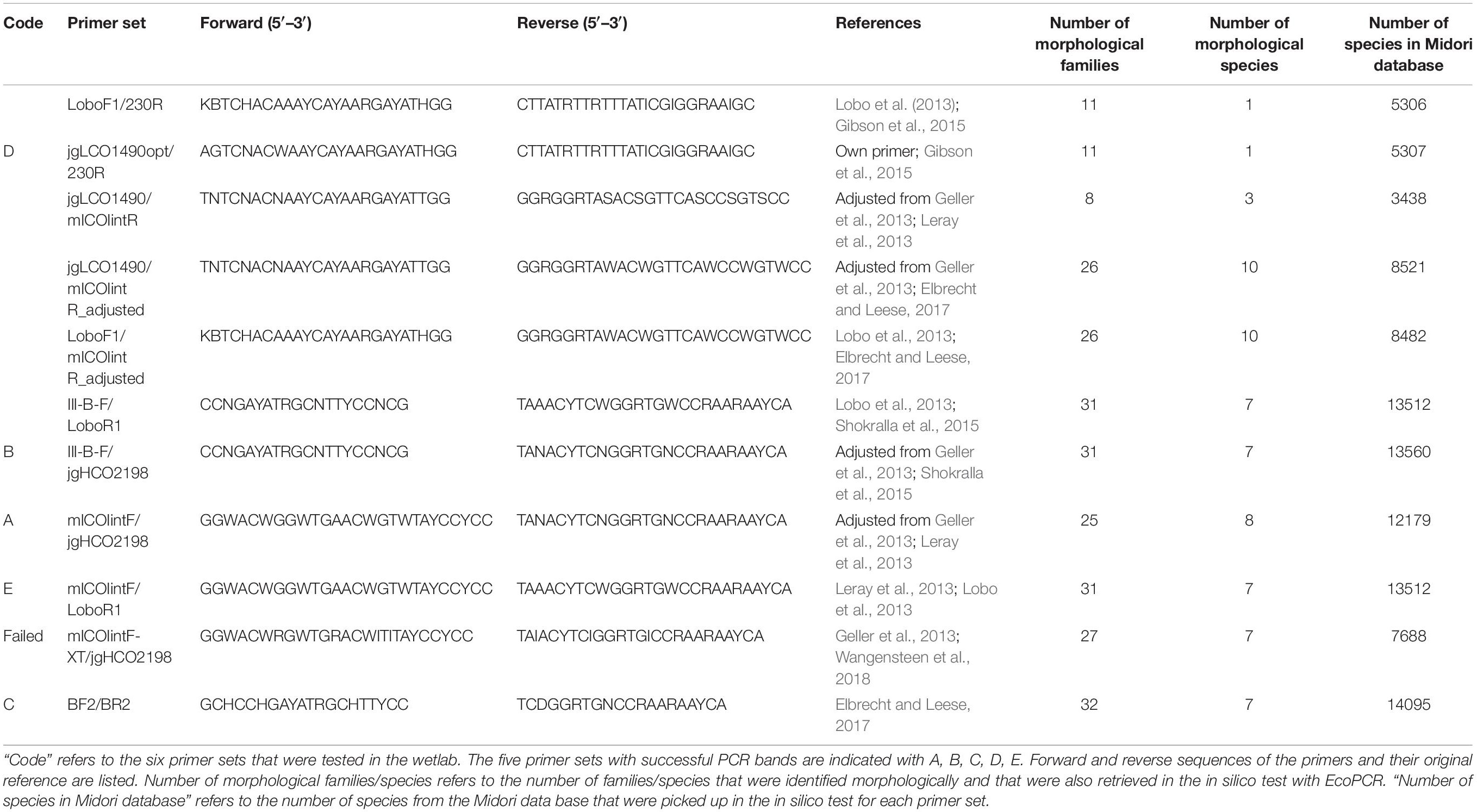
Table 1. Overview of the COI primer sets used for in silico testing against the Midori marine database.
The purified PCR product was then used as template for the index PCR using the Nextera XT Index kit v2 from Illumina. The reaction mix consisted of 5 μl nuclease free water, 12.5 μl 2× KAPA HiFi HotStart ReadyMix, 2.5 μl of each index primer (10 μM) and 2.5 μL purified PCR product. The PCR cycling conditions were: initial denaturation for 3 min at 95°C, 8 cycles of denaturation for 30 s at 95°C, annealing for 30 s at 55°C and extension for 30 s at 72°C and a final extension for 5 min at 72°C. Indexed PCR products were purified with Clean NGS beads. Successful indexing was checked by loading PCR1 and index PCR products on the Qiaxcel (Qiagen). Indexed PCR products were measured twice with the Quantus and equimolarly pooled.
The library was sequenced on two Illumina Miseq runs (300 bp, PE, sequenced by Admera Health Biopharma Services): primer sets A–D were run together, while primer set E was added to a second run containing the same macrobenthos samples for a study on the variation between biological, DNA and PCR replicates (Van Den Bulcke et al., in preparation). For both runs, 20% PhiX was added.
Bioinformatic Processing
Demultiplexed reads were provided by the sequencing company (Admera health Biopharma Services) and were checked for quality using MultiQC (Ewels et al., 2016). Forward and reverse primers were removed using Trimmomatic (Bolger et al., 2014). Reads were further processed using the Dada2 pipeline in the Dada2 v1.12.1 package (Callahan et al., 2016) in R v3.6.2 (R Core Team, 2019) to obtain amplicon sequence variants (ASVs). Standard filtering parameters were used, except for the maximum number of errors allowed in a read, which was set at 3. Reads were further trimmed to remove parts with a quality score <30 while keeping at least 5 bases overlap. For each sample and each primer set, unique reads were determined, merged and filtered for chimera’s.
Taxonomy was assigned using a custom made reference database containing in house and Bold COI sequences from macrobenthos species that have been collected during monitoring campaigns in the Belgian part of the North Sea over the last 10 years. This reference database contained 346 Sanger COI sequences from 306 species. The newly generated COI sequences have been uploaded to BOLD and are part of a larger study to build a COI reference database for macrobenthos from the whole North Sea region1. Taxonomy was assigned with the naïve Bayesian classifier (Wang et al., 2007) with the number of bootstraps set at 80 (minBoot = 80). Barplots were created in R to visualize the number of reads, ASVs and the percentage of ASVs with assigned taxonomy for the bulk and ethanol samples for each primer set.
Amplicon sequence variants that did not receive a taxonomic assignment at the phylum level using our custom reference database were extracted and taxonomic assignment was repeated as above but now using the MIDORI_UNIQUE_COI_MARINE_ 20180221 reference dataset (Machida et al., 2017) downloaded from http://genoweb.toulouse.inra.fr/frogs_databanks/assignation/to ensure that the lack of taxonomic assignment was not caused by the used reference database. Only a small fraction of the data was additionally assigned taxonomy when using the Midori dataset (see “Results” section), so all further comparisons were made using taxonomic assignments with our custom made reference database, as it has been shown that smaller training datasets tailored to the taxa and geographic region of interest yield better results for genus and species level assignments rather than using the largest possible database (Ritari et al., 2015; Macheriotou et al., 2019). For primer set A, unassigned ASVs after MIDORI were matched against the nt database of NCBI using Blastn to check whether these unassigned ASVs were from non-metazoan origin. Taxonomic assignments with qcov >50 and pident >90 were considered a reliable hit.
Comparison of Species Composition Between Morphology, Bulk DNA and eDNA Datasets
The number of unique and shared species for the morphological, bulk and ethanol datasets were evaluated using the unrarified datasets from the four samples that were identified morphologically and using the UpsetR package (Conway et al., 2017). For the morphological dataset, only specimens identified up to species level were included in the dataset (juveniles and identifications to genus, order or phylum level were removed from the dataset). For each of the five primer sets, the total number of species found for each of the four samples was determined and visualized in barplots using R.
Comparison of Beta Diversity Patterns Between Bulk DNA and eDNA Datasets of the Five Primer Sets
To compare species community composition of the bulk and ethanol samples across primer sets and locations, we rarified all samples in the five datasets at 25,000 reads. Samples with less than 25,000 reads were removed from the dataset. This threshold was chosen because the plateau phase of the rarefaction curves was reached for all primer sets while a minimum of samples had to be removed (6, 1, 2, 4, and 1 sample were removed for primer sets A, B, C, D, and E, respectively). The resulting count tables were fourth root transformed, a transformation commonly used for ecological datasets with many zeros and a few large values (Quinn and Keough, 2002). Bray–Curtis dissimilarity index was calculated between samples and used to construct a metric multidimensional scaling (MDS) plot for all primer sets combined and for each primer set separate in the R package Vegan 2.5.6 (Dixon, 2003). Next to this abundance based index, the Jaccard similarity index was calculated for each primer set separately. This index is based on presence/absence data, and determines the percentage of shared species between samples.
Each of the five primer datasets was characterized by a fully crossed design for the factors location (four levels: 120, 330, 840, and ZVL) and DNA source (two levels: eDNA from ethanol and bulk DNA). A two-way PERMANOVA was performed with 9,999 permutations to test for significant differences in macrobenthic communities between locations, between DNA source and the interaction factor location x DNA source. The homogeneity of multivariate dispersions for the main factors location and DNA source and their interaction was assessed using the betadisper and the permutest functions (9,999 permutations) from the Vegan package. Pairwise post hoc tests were conducted for the factor location when the interaction term was non-significant using the pairwise Adonis library (Martinez Arbizu, 2019). When the interaction term is significant, pairwise tests should be conducted within each level of the factor DNA source. However, in view of the low level of replication at this level, such analyses were inappropriate. The false discovery rate, i.e., the expected proportion of false discoveries amongst the rejected hypotheses, was controlled by the BH method (Benjamini and Hochberg, 1995) using 999 permutations. Statistical analyses were conducted on the Bray–Curtis and the Jaccard distance matrices for each primer set.
Morphological and Biological Traits That Impact Community Composition of Bulk DNA and eDNA From the Ethanol Preservative
To determine whether the detection of macrobenthos species in bulk DNA and eDNA datasets could be linked to morphological and biological traits of the species, four categorical traits were scored: body size (<20, 20–100, and >100 mm), larval stage (benthic or pelagic) and longevity (<1, 1–3, 3–10, and >10 years), based on the species-trait dataset of Breine et al. (2018), and sclerotization of the cuticula (chitin, CaCO3, or soft tissue), based on Motokawa (1984) and Brusca and Brusca (2002). To investigate how these four traits affect the probability of detection (presence/absence) of the species in bulk DNA and eDNA samples, we used a generalized linear mixed effects model approach with the glmer function in the lme4 package (Bates et al., 2015). The mixed logistic regression model was built with sample type (bulk DNA or eDNA) and the four traits as fixed effects, while Sample ID was considered as a random effect because eDNA from the ethanol preservative and bulk DNA came from the same sample. We used a logit-link and assumed a binomially distributed error for the presence/absence response variable. We started with simple models always including sample type and the interaction of sample type with one of the four traits. Only traits with significant terms were then used to build more complex models.
The final model was chosen based on the lowest AIC and the lowest number of parameters. This model included sample type, body size and sclerotization along with the two way interaction terms with sample type. The significance level of the Type III tests of the fixed model terms was generated using the Anova function of the car package (Fox and Weisberg, 2019). To visualize the effects of the model terms, least squares means of the fitted model were calculated using the lsmeans function of the emmeans package v 1.4.7 (Lenth, 2020). The obtained probabilities were visualized using ggplot. Post hoc pairwise significance testing for differences between body sizes within sample type and between sclerotization categories within sample type were conducted using the lsmeans function. We restricted the trait analyses to the dataset of primer set A, as this primer set detected most of the morphological species (see “Results” section).
Results
Bioinformatic Processing
The total number of sequences generated for each primer set ranged between 2,786,114 and 3,845,487 (Supplementary Table 1). One sample (ZVLA_B) received very low read numbers for all primer sets. Primer set B yielded the highest number of raw reads, but almost half of the reads were lost during the filtering step. For primer sets A and E, relatively few reads were lost during filtering, denoising and chimera removal, resulting in more than 2.5 M reads for further processing (Supplementary Table 1 and Supplementary Figure 1). Read numbers were comparable between bulk and ethanol samples for each primer set except for primer set A, where approximately three times more reads were obtained for the bulk samples (Supplementary Figure 2A). Nevertheless, a comparable number of ASVs was obtained for the ethanol and bulk samples with primer set A (average of 149 and 132, respectively, Supplementary Table 1 and Supplementary Figure 2B). For primer sets C and E, more ASVs were found in the ethanol than in the bulk samples (Supplementary Figure 2B). The total number of ASVs generated across the 24 samples substantially varied between primer sets and was lowest for primer sets A and E (2,139 and 5,230 ASVs, respectively, vs. 22,151, 14,813 and 15,211 ASVs for primer sets B, C, and D, respectively) (Supplementary Figure 3).
The percentage of ASVs that were assigned taxonomy using our custom COI reference database was low (22.6, 12.1, 11.7, 4, and 10.9% for primer sets A, B, C, D, and E, respectively; Supplementary Table 2). However, for primer set A, the 1,655 unassigned ASVs represented only 13.4% of the total number of non-chimeric reads generated for that primer set. This percentage was considerably higher for the other primer sets and ranged between 38.4 and 81.7% (Supplementary Table 2). Phylum level assignments were comparable between the ethanol and bulk samples for primer sets B, C, and D, while more ASVs from the bulk samples were assigned to phyla compared to the ethanol samples for primer sets A (bulk: 30% and ethanol: 20%) and D (bulk: 20% and ethanol: 9%) (Figure 2). At the species level, taxonomic assignment of the bulk samples was highest for primer set A (25, 9, 10, 3, and 16% for primer sets A, B, C, D, and E, respectively).
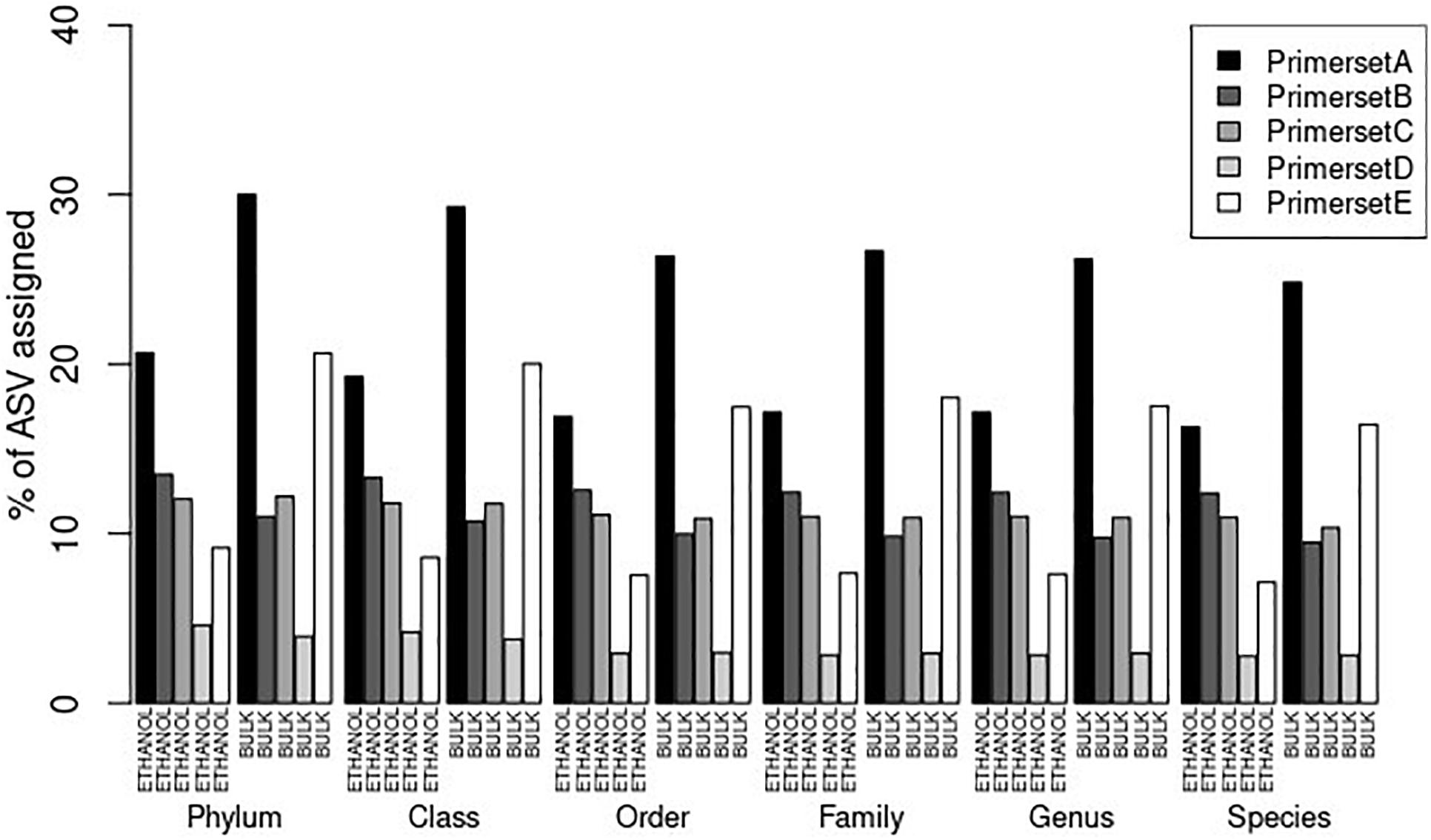
Figure 2. Percentage of ASVs found in ethanol (ETHANOL) and bulk (BULK) samples that received taxonomic assignment at the different taxonomic levels for each primer set. Unrarified datasets were used to capture the most complete taxonomic assignments for each primer set. For each dataset, the number of assigned ASVs were normalized to the total number of ASVs for that particular dataset.
When using the COI Midori dataset for taxonomic assignment of the unassigned sequences, only a small fraction were assigned at the phylum level (8.6, 0.6, 1.1, 5.7, and 5.8% for primer sets A, B, C, D, and E, respectively), and these assigned ASVs represented 5.3, 0.5, 0.8, 6.0, and 20.6% of the total non-chimeric reads for primer sets A, B, C, D, and E, respectively. For primer sets A and E, most reads were assigned to the cnidarian Obelia bidentata (1.3 and 5.1%, respectively) which was found in all ethanol samples, in all bulk samples of location 120 and 330 and in very low abundance in one bulk sample of location 840 (37 and 22 reads, respectively). A detailed list of all species detected after taxonomic assignment with the Midori dataset for each primer set is available in Supplementary Table 3.
To investigate whether the unassigned ASVs after Midori were of non-metazoan origin, a blastn search was done for primer set A against the nt database of NCBI. This resulted in only 83 of the 1471 unassigned ASVs receiving a reliable assignment (query coverage >50, % identity >90), representing 58 species. All species had low read numbers, except for L. balthica, which was detected in the bulk DNA of two replicates of the L. balthica community (ZVL) with more than 10,000 reads (Supplementary Table 4). The non-metazoan taxa were represented by three fungal, two bacterial, five Viridiplantae and 27 algal or diatom species which all together only represented 0.3% of the total non-chimeric reads (Supplementary Table 4).
Comparison of Species Composition Between Morphology, Bulk DNA and eDNA Datasets
Morphological identification of the four samples yielded a total of 57 macrobenthos species. In addition, juveniles of Ophiura, Echinoidea, Ophelia, Nephtys, Phoronis, Pontocrates, Spio, Magelona, Cirratulidae, Glycera, Lanice as well as specimens belonging to Nemertea, Oligochaeta, and Anthozoa were identified to the respective order, genus or phylum. A detailed list of all taxa and their abundance in each location is presented in Supplementary Table 5. The number of morphological species was highest in location 120, where 39 species were identified, and decreased over locations 330, 840 and ZVL (13, 10, and 3, respectively) (Supplementary Figure 4). For six morphologically identified species (Capitella minima, Caulleriella alata, Mediomastus fragilis, Pseudopolydora pulchra, Spirobranchus lamarckii, and Tanaissus lilljeborgi), no COI sequence was present in our reference COI database.
For the bulk DNA datasets, all primer sets except primer set D showed the same decreasing trend in species numbers from location 120, over 330, 840, and ZVL (Supplementary Figure 4). For the eDNA samples from the ethanol preservative, primer sets B and D identified very low species numbers, and for all primer sets the decreasing trend in species numbers was less clear compared to the bulk DNA dataset. Bulk DNA generally detected a higher number of species than the eDNA samples from the ethanol preservative (52, 37, 37, 25, and 44 species for bulk DNA vs. 45, 13, 22, 4, and 46 species for eDNA from the ethanol preservative with primer sets A, B, C, D, and E, respectively). The biggest discrepancy between primer sets was situated in the number of species detected for the most diverse sample 120B (Supplementary Figure 4).
Of the 57 species that were identified morphologically, primer set A was able to pick up the highest number of species (Figure 3): 34 and 23 of the morphological species were detected in the bulk DNA and in the eDNA from the ethanol preservative, respectively. All primer sets missed a substantial portion of the morphologically identified species: 21, 34, 33, 40, and 25 species of the 57 morphological species were only found in the morphological dataset, for primer sets A, B, C, D, and E, respectively (Figure 3). The number of morphological species detected in the bulk DNA samples (34, 23, 24, 17, and 28 for primer sets A, B, C, D, and E, respectively) was substantially higher than the number of morphological species detected in the eDNA samples from the ethanol preservative (23, 7, 9, 3, and 22 for primer sets A, B, C, D, and E, respectively). Primer sets A and E clearly outperformed the detection of the morphological species in the eDNA from the ethanol preservative.
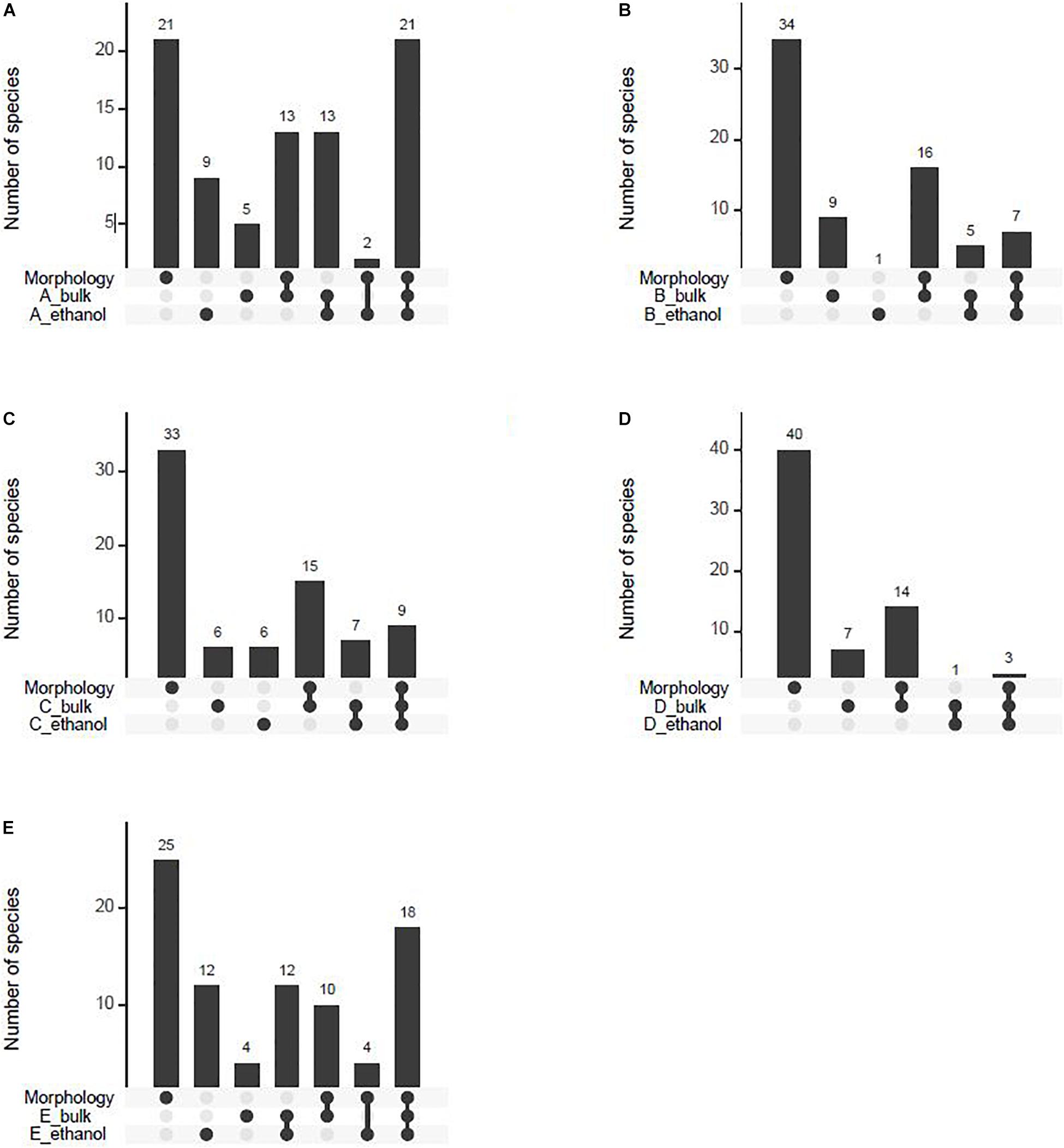
Figure 3. The number of unique and shared species between morphological, bulk DNA and eDNA from the ethanol preservative datasets for each of the five primer sets (A–E). Datasets are based on the four samples that were identified morphologically to allow a one-on-one comparison. Black dots indicate in which dataset the species represented by the black bar were found.
Comparison of Beta Diversity Patterns Between Bulk DNA and eDNA Datasets of the Five Primer Sets
The MDS plots illustrate that primer sets A and E have very similar community patterns, while primer sets B, C, and D are similar to each other but clearly distinct from the other two primer sets (Supplementary Figure 5A). All five primer sets were able to differentiate the most diverse location (120) from the three other locations (Supplementary Figure 5B), but differences in the ability to differentiate the three other locations were observed between primer sets (Figure 4). MDS plots for the five primer sets separately showed that the offshore sandy locations with intermediate diversity (330 and 840) were more similar to each other than to the fine sandy (120) and fine muddy (ZVL) coastal locations (Figure 4). For primer sets A and E, all four locations were recovered as separate clusters when using Bray Curtis dissimilarity index (Figure 4). This separation was less clear when using the Jaccard index (Supplementary Figure 6).
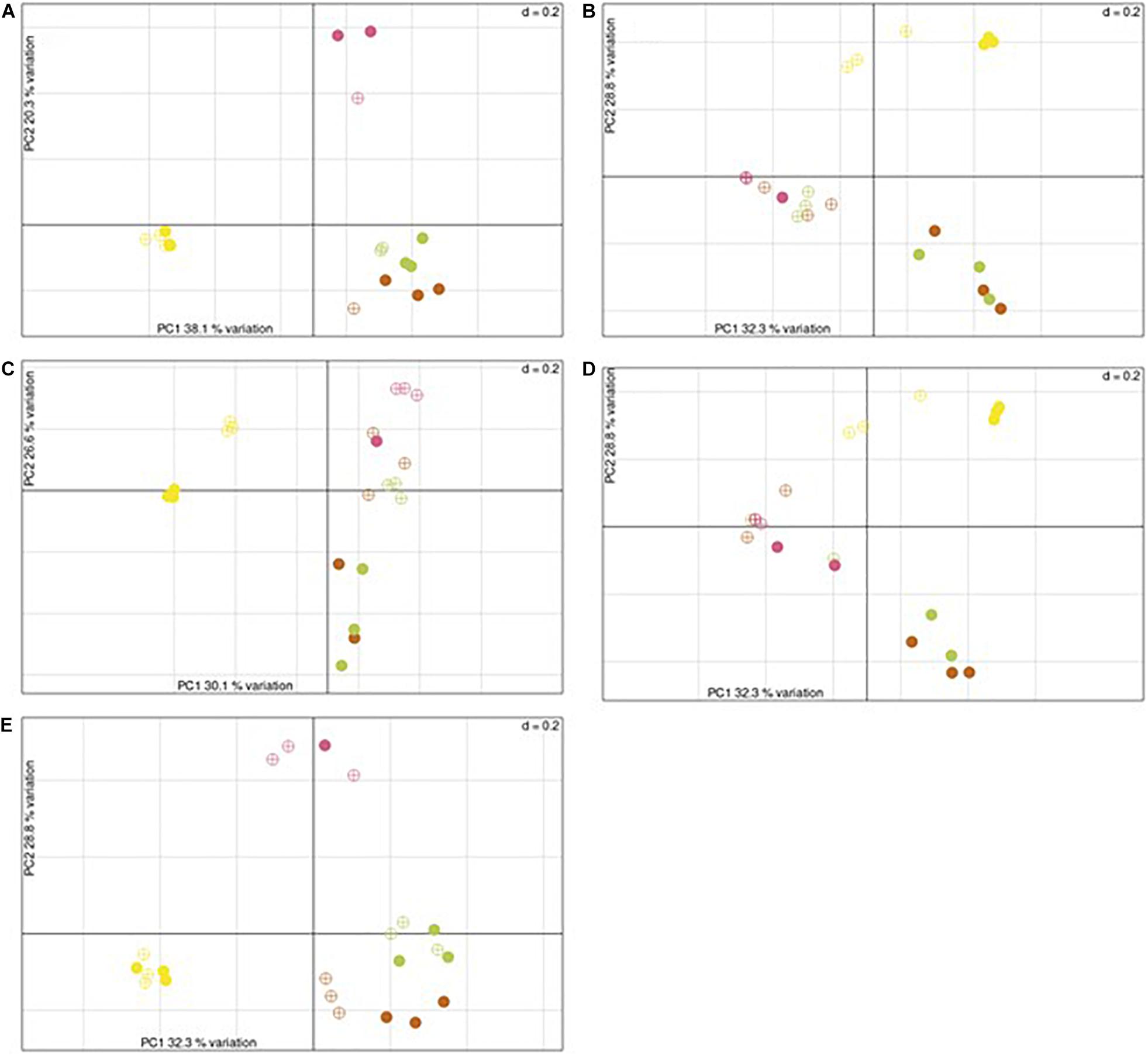
Figure 4. Multidimensional scaling plots based on Bray–Curtis dissimilarity index for each primer set (A–E). Colors indicate the four locations as proxy for the different macrobenthic communities as shown in Figure 1, full circles indicate bulk DNA and open circles eDNA samples from the ethanol preservative.
Permanova results were largely consistent across primer sets and between Bray–Curtis and Jaccard distance metrics and showed highly significant differences between locations, between bulk DNA or eDNA and for primer sets B, C, and D also the interaction effect was highly significant (Table 2). Observed differences between primer sets were the non-significant interaction term location × DNA source for primer sets A and E when the Bray–Curtis index was used and a non-significant DNA source effect and interaction term location × DNA source for primer set A when the Jaccard index was used (Table 2). Nearly all permdisp results were significant for all primer sets and for both distance metrics (Table 2), indicating that the significant Permanova results were caused by both location effects and dispersion effects. Despite the differences in dispersion of the replicates within sampling locations, the MDS plots showed a clear distinction between sampling locations which was supported by a significant main effect of location in Permanova (Table 2) as well as significant post hoc test results for all pairwise combinations of locations for primer sets A and E (Supplementary Table 6). Permanova revealed significant differences in community structure between bulk DNA and eDNA samples for all primer sets (Table 2).
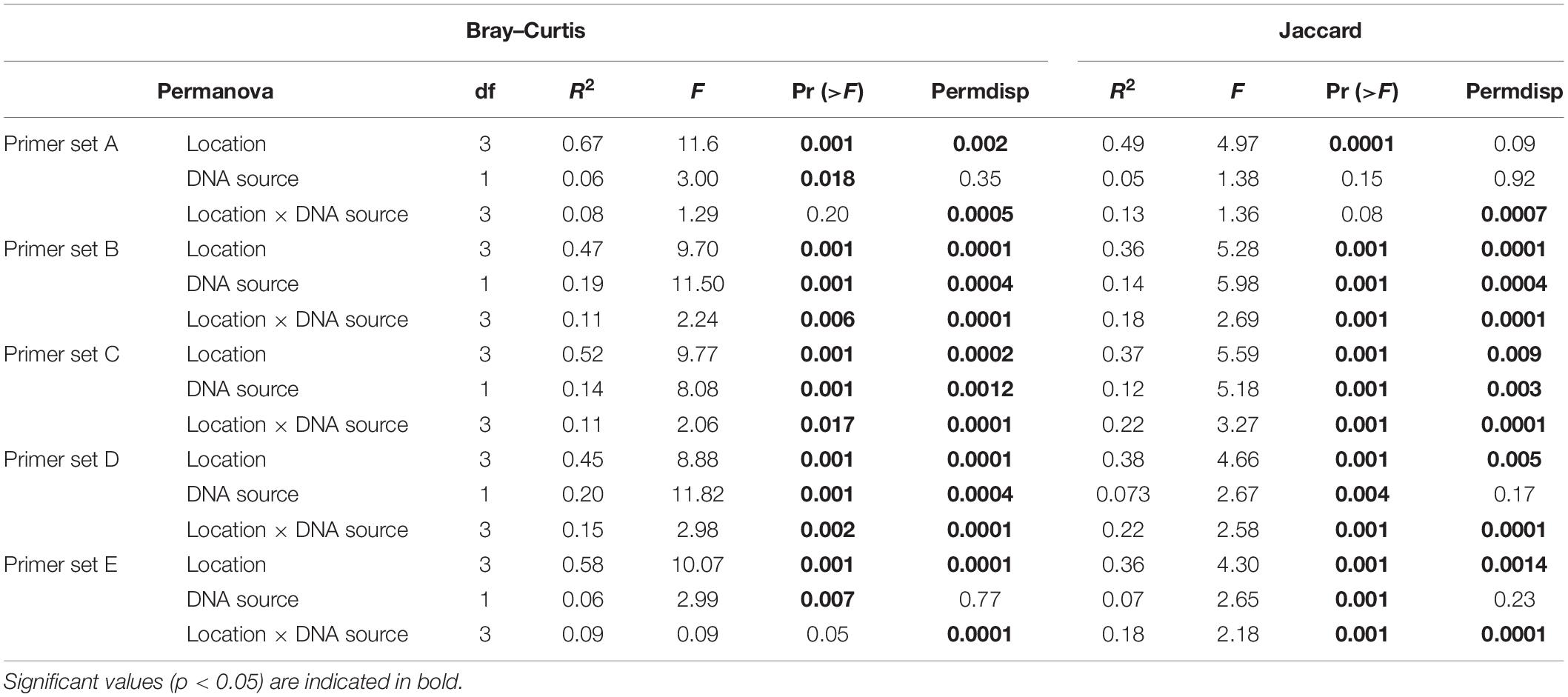
Table 2. PERMANOVA and Permdisp results based on 9,999 permutations for each of the five primer sets using Bray–Curtis distance or the Jaccard index.
Morphological and Biological Traits That Impact Community Composition of Bulk DNA and eDNA From the Ethanol Preservative
A detailed comparison between communities recovered in bulk DNA and eDNA from the ethanol preservative was conducted for primer set A. Both DNA datasets detected more species than the morphology based identification (69 species in bulk DNA, 64 in eDNA and 57 in morphology, Supplementary Figure 7). However, nine species that were only detected in the metabarcode datasets may be linked to taxa that were not identified up to species level in the morphology (Supplementary Table 7). Although community composition significantly differed between bulk DNA and eDNA of the ethanol preservative (Table 2), 49 species were also shared between the two sample types (Supplementary Figure 7).
The differences observed between the two sample types could be explained by morphological traits of the macrobenthos species. The two biological traits larval stage and life span did not result in significant terms and were not included in the final model. The final model included fixed effects of sample type, body size and sclerotization, as well as the two way interaction terms sample type × body size and sample type × sclerotization. The probability of detecting a species in bulk DNA or eDNA from the ethanol preservative was differently affected by body size and sclerotization as shown by the significant sample type x body size and sample type × sclerotization interaction effects (Table 3). The probability of detecting species in the bulk samples was overall high (Figure 5), with no significant differences between body size and sclerotization (all pairwise comparisons had p > 0.05).
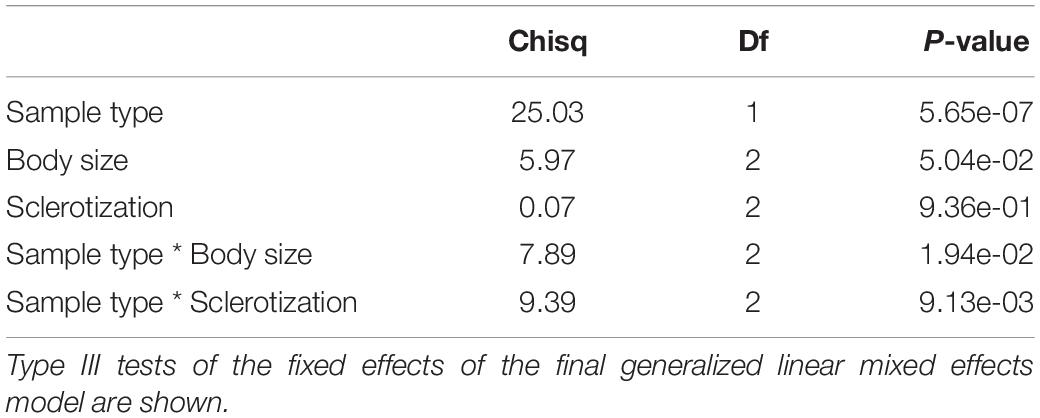
Table 3. The effect of sample type, body size, and sclerotization on the probability of detecting macrobenthos species.
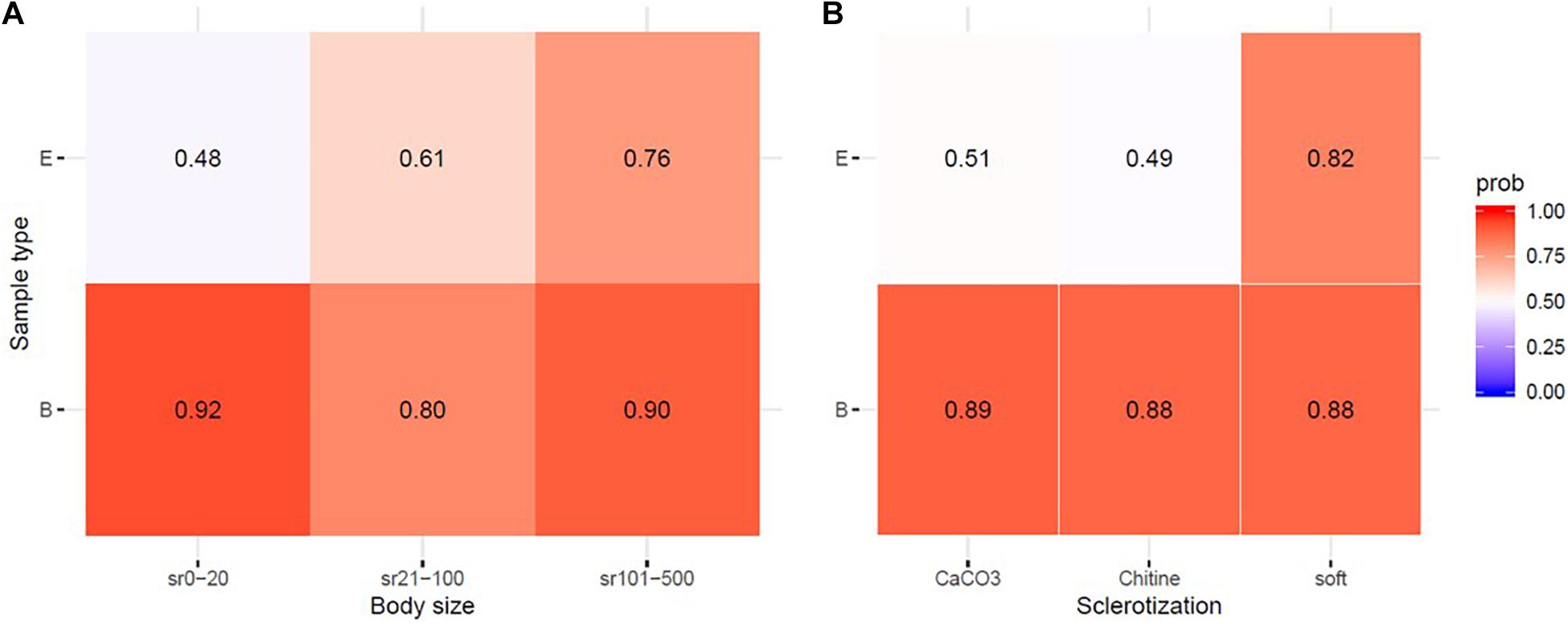
Figure 5. Effect of sample type, body size and sclerotization on the probability of detecting macrobenthos species in bulk DNA (B) or eDNA from the ethanol preservative (E) in relation to body size (A) and sclerotization (B). Probabilities are based on the generalized linear mixed effects model in Table 3.
In contrast, the probability of detecting species in the eDNA from the ethanol preservative was significantly higher for soft bodied species compared to species having chitine (p = 0.0001) or CaCO3 in their cuticula (p = 0.0001). The probability of detecting species having small and medium body sizes was significantly higher in bulk DNA samples than in the eDNA samples from the ethanol preservative (body size 0–20: p < 0.0001; body size 21–100: p = 0.003; Figure 5), while the probability of detecting species having large body sizes was not significantly different between the two sample types (p = 0.18). The presence of sclerotization had also a clear impact: the probability of detecting species having chitine or CaCO3 in their cuticula was significantly lower in the eDNA of the ethanol preservative compared to the bulk DNA samples (p = 0.0002 and p < 0.0001, respectively, Figure 5), while no significant difference was observed between the two sample types for species having soft bodies (p = 0.21).
Discussion
We found considerable differences in alpha and beta diversity patterns between the tested primer sets and between the two sample types (bulk DNA extracted with the Qiagen DNeasy PowerSoil Kit vs. eDNA extracted from the ethanol preservative with the Promega Wizard®SV Genomic DNA Purification System) and showed that, under these conditions, the detection of macrobenthos species in eDNA extracted from the ethanol preservative is linked to the morphological traits body size and sclerotization of the cuticula.
Failure to Detect Macrobenthos Species in DNA Metabarcoding Datasets Was Not Linked to the Reference Databases Used for Taxonomic Assignment
Previous COI metabarcode datasets derived from macrobenthic communities have shown that only a small fraction of OTUs received taxonomic assignment linked to macrobenthos species (Leray and Knowlton, 2015; Aylagas et al., 2018) which has been explained by the incompleteness of reference databases (Leray and Knowlton, 2015; Wangensteen et al., 2018). Our datasets also contained a low percentage of ASVs (4–22.6%) with taxonomic assignment but the availability of a nearly complete reference database showed that discrepancies between morpho-taxonomic and DNA metabarcode datasets were not the result of incomplete reference databases as such. Even when using the MIDORI reference database with almost 47,000 marine metazoan species for taxonomic assignment, only an additional 0.6–8.6% of the ASVs were assigned. Previous macrobenthos metabarcode studies reported a high portion of non-metazoan taxonomic assignments (Aylagas et al., 2018; Wangensteen et al., 2018). We tested this for primer set A, by blasting all ASVs that were unassigned after Midori (1,471 ASVs) against the nt database and found only 45 ASVs with a non-metazoan hit. It remains unclear whether the remaining unassigned ASVs represent unknown diversity, genetic noise, e.g., created by PCR and/or sequencing errors, nuclear pseudogenes, or aspecific amplification of other genomic regions.
Metabarcode studies typically detect a higher number of species compared to morphology based analyses (Elbrecht et al., 2017b; Lobo et al., 2017), which has been explained by the fact that specimens are not lost during the sorting process and also small pieces of animals – which are morphologically not identified- are included in the bulk DNA and eDNA. When looking at the four samples that have been identified morphologically, DNA metabarcoding data detected nine species that may be linked to specimens for which the morphological identification was limited to higher taxon level (Supplementary Table 7).
Metabarcode studies have also reported the detection of species that were lacking in the morphological analyses from the same samples (Aylagas et al., 2016a). This was also the case for our study, but in contrast to what we expected, fewer species were detected using DNA metabarcoding (Figure 3): for the four samples that were processed both morphologically and with DNA metabarcoding, 57 species were morphologically identified, while 52 and 45 species were identified with the bulk DNA and eDNA, respectively. This may be partly explained by the reference database and algorithm used to assign taxonomy: 51 and 76 species for the bulk and eDNA, respectively, were identified when using the MIDORI reference database, while 60 and 103 species were identified when using Blast and the NCBI database. The latter procedure was also used by Elbrecht et al. (2017b) and Lobo et al. (2017).
Importantly, DNA metabarcoding was not able to detect all morphological species within the metazoan diversity, despite the fact that our reference database included 51 out of the 57 morphospecies. When including all biological replicates, the best performing primer set A (see Supplementary Material 1 for a more detailed discussion on the performance of the five primer sets) was unable to detect 19 out of 57 morphological species. Only six of these 19 species did not have a reference sequence, indicating that other factors than the reference database influenced species detection in metabarcode datasets.
The wetlab procedure can lead to missing species because of differences in efficiency of DNA extraction between species or because primers insufficiently match with the COI gene of those species. All 19 species are relatively large animals, and for several species more than one individual was found in a particular sample. Several species have soft tissues, it is therefore unlikely that DNA extraction would be problematic for these species. Eleven species belonged to the Polychaeta, a class characterized by high species and sequence diversity in the COI gene (Carr, 2012). It is therefore likely that for these species, the primers were not a good match. This is further strengthened by the fact that for six polychaete species voucher specimens were available and good DNA was extracted, while no PCR product or bad Sanger sequences were obtained. This illustrates the great benefit of primer free methods for biodiversity assessment, although at this point, these methods are more expensive than DNA metabarcoding (Giebner et al., 2020).
Second, taxonomic assignment through the RDP classifier may be inefficient. One species, Acrocnida brachiata, was identified using the Midori reference dataset which contained 151 COI sequences of this species, including a sequence that was identical to our own reference sequence. It is known that the content and size of the training set strongly impacts taxonomic assignment with RDP Classifier (Ritari et al., 2015).
Finally, the morphological identification by our experts may have been incorrect. However, the morpho-taxonomic analyses of macrobenthos is under accreditation (accreditation certificate nr. 315-TEST, following NBN EN ISO/IEC 17025:2005) and the two experts that conducted the morphological identification had very low misidentification rates (at most 3 taxa have been misidentified in quality controlled samples over the last 9 years), suggesting that misidentification only had a minor impact on our results.
Bulk DNA Samples Detected More Macrobenthos Species Than eDNA From the Ethanol Preservative and Were Not Affected by Morphological Traits of the Species
Our results showed that the number of morphological species detected in the ethanol preservative of each sample was lower compared to the bulk DNA samples (Supplementary Figure 4), which has also been observed for insect communities (Zenker et al., 2020) and for freshwater benthic communities (Hajibabaei et al., 2019). Substantial differences between both sample types have been observed in other studies with almost no shared species between bulk DNA and eDNA (Marquina et al., 2019). Our results also showed significant differences between communities from the bulk and ethanol preservative (Table 2) but many species were detected in both sample types. The 15 species that were exclusively detected in the ethanol preservative were mainly pelagic species. In view of the low read numbers allocated to these species (at most 153 reads) these DNA molecules are most likely “contaminant” DNA molecules that were absorbed on organic debris. If the main aim is to detect as many species as possible in the environmental samples, than mixing or grinding the samples before DNA extraction greatly increases the detection of a variety of species in bulk DNA samples.
The Probability of Species Detection in eDNA From the Ethanol Preservative Is Linked to Their Morphological Traits
Our results showed that species with no sclerotization and large body size showed higher probability of being detected in the eDNA fraction, while these traits did not strongly impact the detection in bulk DNA samples (Figure 5). We used different DNA extraction kits for the two sample types, because we observed sediment – coming from the intestine of sediment dwelling organisms – in the ‘soup’ of blended organisms. For eDNA, the choice of DNA extraction impacts the detection of species when using metabarcoding, especially when different target groups are of interest (e.g., bacteria, phytoplankton, and fish). This has been explained by the type of cell wall which is more difficult to lyse for Gram-positive bacteria and plant cells (Djurhuus et al., 2017). Eukaryotic diversity assessed using the COI gene is best captured using a kit involving chemical lysis of the cells (Deiner et al., 2015). We therefore chose the extraction kits that detected the most macrobenthic species from the bulk samples and the ethanol preservative, respectively.
Despite the fact that we used different DNA extraction methods, we are confident that the detection in eDNA from the ethanol preservative can be linked to morphological features: (1) if the kit instead of morphological features would determine the DNA molecules that are detected in the eDNA, we would expect a random signal instead of the clear biological pattern observed in our data; (2) the probability of detection is significantly lower, but there is still a chance of 50 and 48% to detect species with a hard sclerotization in their cuticula or species <20 mm in the ethanol preservative. This means that the Promega Wizard®SV Genomic DNA Purification System kit does detect species with such morphological characteristics; (3) our results for the ethanol preservative corroborate findings of earlier studies that have compared community composition between the bulk DNA and eDNA from the ethanol preservative. They found clear differences, whether using different extraction kits (Gauthier et al., 2020) or the same extraction kit (Marquina et al., 2019; Zizka et al., 2019b).
The characterization of macrobenthic communities by DNA metabarcoding of the ethanol preservative instead of bulk specimens would greatly speed up sample processing time in the laboratory since the decantation and sorting step can be avoided. The use of the ethanol preservative to reliably characterize macroinvertebrate diversity and composition has been demonstrated for fresh water samples (Martins et al., 2019). Our results show that if the detection of large animals with soft body tissues is the primary focus of a monitoring study, than the ethanol preservative can be used to quickly identify environmental samples whilst preserving the specimens for future morphological research.
Toward Standardization of DNA Metabarcoding for Marine Environmental Monitoring
A European scale monitoring method has been identified as a science priority need to support benthic ecosystem assessments (Van Hoey et al., 2019). Biotic indices inferred from DNA based taxonomic assignments were comparable to indices derived from morphological identifications (Elbrecht et al., 2017b; Lobo et al., 2017; Aylagas et al., 2018). When using DNA metabarcoding for monitoring, a standardized method that compares best with traditional morphological analyses in order not to break up the existing traditional monitoring time series is preferable. Our results show that bulk samples with use of the Leray primer set would be the method of choice for benthic monitoring in the North Sea. The next step now is to showcase the repeatability and robustness of DNA metabarcoding in relation to European legislation such as the Marine Strategy Framework Directive. This may be achieved through the organization of a ring test in which the same samples are processed by different laboratories. Finally, our results showed that complete reference sequence databases will not be the holy grail to come to 100% comparability with morpho-taxonomic datasets, but at this point the reference databases are our only link with the ecological and biological information imbedded in the Linnean taxonomic system. As such, collaboration with taxonomic experts to further populate the reference database remains highly recommended (Porter and Hajibabaei, 2018) to maximize benefits from the inclusion of DNA metabarcoding in monitoring studies.
Conclusion
DNA metabarcoding is a promising and cost-efficient tool for monitoring macrobenthic communities in the marine environment. In view of the high taxonomic and phylogenetic diversity in marine communities (Mora et al., 2011; Appeltans et al., 2012) and differences in benthic communities between geographic realms, optimization of the protocol for each taxonomic group and each geographic region at hand is necessary. By aiming for the detection of the highest number of known morphological species in a given area, a link with long time series of monitoring data based on morpho-taxonomy can be made. Our results demonstrate the impact of primer choice and DNA source when using the mitochondrial COI gene to assess macrobenthos species diversity. We showed that the probability of detecting macrobenthos species in the ethanol preservative was linked to the morphological traits body size and sclerotization and species detection was most robust and most comparable with morphological results when using the Leray primer set and bulk DNA as template for DNA metabarcoding.
Data Availability Statement
The sequencing datasets and corresponding metadata generated for this study can be found as BioProject in the online NCBI repository under accession number PRJNA683616.
Author Contributions
SD designed the research, analyzed and interpreted the data, wrote the manuscript, and provided funding for the research. SM performed the labwork, submitted sequences to Bold, and wrote the manuscript. LV conducted the labwork. JV wrote the R scripts and created the Github page. JW identified the macrobenthos morphologically. HH vouchered the specimens prior to barcoding and uploaded pictures to Bold. BA helped in statistical analyses, interpreted statistical results, and critically reviewed the manuscript. AH helped in designing the study, interpreted the data, and critically reviewed the manuscript. KH provided funding for the research, interpreted the data, and critically reviewed the manuscript. AD provided funding for the research, interpreted the data, and wrote the manuscript. All authors contributed to the article and approved the submitted version.
Funding
Funding for this research was received through the Sand Fund of the Federal Public Service Economy and GEANS- Genetic tools for Ecosystem health Assessment in the North Sea region, an Interreg project supported by the North Sea Programme of the European Regional Development Fund of the European Union.
Conflict of Interest
The authors declare that the research was conducted in the absence of any commercial or financial relationships that could be construed as a potential conflict of interest.
Acknowledgments
Shiptime on RV Belgica was provided by BELSPO and RBINS–OD Nature. We thank the crew for the logistic support during sampling.
Supplementary Material
The Supplementary Material for this article can be found online at: https://www.frontiersin.org/articles/10.3389/fmars.2021.637858/full#supplementary-material
Footnotes
References
Alberdi, A., Aizpurua, O., Gilbert, M. T. P., and Bohmann, K. (2018). Scrutinizing key steps for reliable metabarcoding of environmental samples. Methods Ecol. Evol. 9, 134–147. doi: 10.1111/2041-210x.12849
Appeltans, W., Ahyong, S. T., Anderson, G., Angel, M. V., Artois, T., Bailly, N., et al. (2012). The magnitude of global marine species diversity. Curr. Biol. 22, 2189–2202.
Aylagas, E., Borja, A., Irigoien, X., and Rodriguez-Ezpeleta, N. (2016a). Benchmarking DNA metabarcoding for biodiversity-based monitoring and assessment. Front. Mar. Sci. 3:96. doi: 10.3389/fmars.2016.00096
Aylagas, E., Mendibil, I., Borja, A., and Rodriguez-Ezpeleta, N. (2016b). Marine sediment sample pre-processing for macroinvertebrates metabarcoding: mechanical enrichment and homogenization. Front. Mar. Sci. 3:203. doi: 10.3389/fmars.2016.00203
Aylagas, E., Borja, A., Muxika, I., and Rodriguez-Ezpeleta, N. (2018). Adapting metabarcoding-based benthic biomonitoring into routine marine ecological status assessment networks. Ecol. Ind. 95, 194–202. doi: 10.1016/j.ecolind.2018.07.044
Bates, D., Maechler, M., Bolker, B. M., and Walker, S. C. (2015). fitting linear mixed-effects models using lme4. J. Stat. Softw. 67, 1–48.
Benjamini, Y., and Hochberg, Y. (1995). Controlling the false discovery rate - A practical and powerful approach to multiple testing. J. R. Stat. Soc. Ser. B Methodol. 57, 289–300. doi: 10.1111/j.2517-6161.1995.tb02031.x
Bolger, A. M., Lohse, M., and Usadel, B. (2014). Trimmomatic: a flexible trimmer for Illumina sequence data. Bioinformatics 30, 2114–2120. doi: 10.1093/bioinformatics/btu170
Borja, A., Elliott, M., Snelgrove, P. V. R., Austen, M. C., Berg, T., Cochrane, S., et al. (2016). Bridging the gap between policy and science in assessing the health status of marine ecosystems. Front. Mar. Sci. 3:175. doi: 10.3389/fmars.2016.00175
Braukmann, T. W. A., Ivanova, N. V., Prosser, S. W. J., Elbrecht, V., Steinke, D., Ratnasingham, S., et al. (2019). Metabarcoding a diverse arthropod mock community. Mol. Ecol. Resour. 19, 711–727.
Breine, N. T., De Backer, A., Van Colen, C., Moens, T., Hostens, K., and Van Hoey, G. (2018). Structural and functional diversity of soft-bottom macrobenthic communities in the Southern North Sea. Estuar. Coast. Shelf Sci. 214, 173–184. doi: 10.1016/j.ecss.2018.09.012
Callahan, B. J., Mcmurdie, P. J., Rosen, M. J., Han, A. W., Johnson, A. J. A., and Holmes, S. P. (2016). DADA2: high-resolution sample inference from Illumina amplicon data. Nat. Methods 13, 581–583. doi: 10.1038/nmeth.3869
Carr, C. (2012). Polychaete diversity and distribution patterns in Canadian marine waters. Mar. Biodivers. 42, 93–107. doi: 10.1007/s12526-011-0095-y
Conway, J. R., Lex, A., and Gehlenborg, N. (2017). UpSetR: an R package for the visualization of intersecting sets and their properties. Bioinformatics 33, 2938–2940. doi: 10.1093/bioinformatics/btx364
Degraer, S., Verfaillie, E., Willems, W., Adriaens, E., Vincx, M., and Van Lancker, V. (2008). Habitat suitability modelling as a mapping tool for macrobenthic communities: an example from the Belgian part of the North Sea. Cont. Shelf Res. 28, 369–379. doi: 10.1016/j.csr.2007.09.001
Deiner, K., Walser, J. C., Machler, E., and Altermatt, F. (2015). Choice of capture and extraction methods affect detection of freshwater biodiversity from environmental DNA. Biol. Conserv. 183, 53–63. doi: 10.1016/j.biocon.2014.11.018
Dixon, P. (2003). VEGAN, a package of R functions for community ecology. J. Veg. Sci. 14, 927–930. doi: 10.1111/j.1654-1103.2003.tb02228.x
Djurhuus, A., Port, J., Closek, C. J., Yamahara, K. M., Romero-Maraccini, O., Walz, K. R., et al. (2017). Evaluation of filtration and DNA extraction methods for environmental DNA biodiversity assessments across multiple trophic levels. Front. Mar. Sci. 4:314. doi: 10.3389/fmars.2017.00314
Douvere, F., Maes, F., Vanhulle, A., and Schrijvers, J. (2007). The role of marine spatial planning in sea use management: the belgian case. Mar. Policy 31, 182–191. doi: 10.1016/j.marpol.2006.07.003
Elbrecht, V., and Leese, F. (2017). Validation and development of COI metabarcoding primers for freshwater macroinvertebrate bioassessment. Front. Environ. Sci. 5:11. doi: 10.3389/fenvs.2017.00011
Elbrecht, V., Peinert, B., and Leese, F. (2017a). Sorting things out: assessing effects of unequal specimen biomass on DNA metabarcoding. Ecol. Evol. 7, 6918–6926. doi: 10.1002/ece3.3192
Elbrecht, V., Vamos, E. E., Meissner, K., Aroviita, J., and Leese, F. (2017b). Assessing strengths and weaknesses of DNA metabarcoding-based macroinvertebrate identification for routine stream monitoring. Methods Ecol. Evol. 8, 1265–1275. doi: 10.1111/2041-210x.12789
Elliott, S. A. M., Guerin, L., Pesch, R., Schmitt, P., Meakins, B., Vina-Herbon, C., et al. (2018). Integrating benthic habitat indicators: working towards an ecosystem approach. Mar. Policy 90, 88–94. doi: 10.1016/j.marpol.2018.01.003
Ewels, P., Magnusson, M., Lundin, S., and Kaller, M. (2016). MultiQC: summarize analysis results for multiple tools and samples in a single report. Bioinformatics 32, 3047–3048. doi: 10.1093/bioinformatics/btw354
Ficetola, G. F., Coissac, E., Zundel, S., Riaz, T., Shehzad, W., Bessiere, J., et al. (2010). An In silico approach for the evaluation of DNA barcodes. BMC Genomics 11:434. doi: 10.1186/1471-2164-11-434
Folmer, O., Black, M., Hoeh, W., Lutz, R., and Vrijenhoek, R. (1994). DNA primers for amplification of mitochondrial cytochrome c oxidase subunit I from diverse metazoan invertebrates. Mol. Mar. Biol. Biotechnol. 3, 294–299.
Gauthier, M., Konecny-Dupre, L., Nguyen, A., Elbrecht, V., Datry, T., Douady, C., et al. (2020). Enhancing DNA metabarcoding performance and applicability with bait capture enrichment and DNA from conservative ethanol. Mol. Ecol. Resour. 20, 79–96. doi: 10.1111/1755-0998.13088
Geller, J., Meyer, C., Parker, M., and Hawk, H. (2013). Redesign of PCR primers for mitochondrial cytochrome c oxidase subunit I for marine invertebrates and application in all-taxa biotic surveys. Mol. Ecol. Resour. 13, 851–861.
Giebner, H., Langen, K., Bourlat, S. J., Kukowka, S., Mayer, C., Astrin, J. J., et al. (2020). Comparing diversity levels in environmental samples: DNA sequence capture and metabarcoding approaches using 18S and COI genes. Mol. Ecol. Resour. 20, 1333–1345. doi: 10.1111/1755-0998.13201
Gibson, J. F., Shokralla, S., Curry, C., Baird, D. J., Monk, W. A., King, I., et al. (2015). Large-scale biomonitoring of remote and threatened ecosystems via high-throughput sequencing. PLoS One 10:e0138432. doi: 10.1371/journal.pone.0138432
Hajibabaei, M., Porter, T. M., Robinson, C. V., Baird, D. J., Shokralla, S., and Wright, M. T. G. (2019). Watered-down biodiversity? A comparison of metabarcoding results from DNA extracted from matched water and bulk tissue biomonitoring samples. PLoS One 14:e0225409. doi: 10.1371/journal.pone.0225409
Lenth, R. (2020). emmeans: Estimated Marginal Means, aka Least-Squares Means. R Package Version 1.4.7.
Leray, M., and Knowlton, N. (2015). DNA barcoding and metabarcoding of standardized samples reveal patterns of marine benthic diversity. Proc. Natl. Acad. Sci. U.S.A. 112, 2076–2081. doi: 10.1073/pnas.1424997112
Leray, M., Yang, J. Y., Meyer, C. P., Mills, S. C., Agudelo, N., Ranwez, V., et al. (2013). A new versatile primer set targeting a short fragment of the mitochondrial COI region for metabarcoding metazoan diversity: application for characterizing coral reef fish gut contents. Front. Zool. 10:14. doi: 10.1186/1742-9994-10-34
Lobo, J., Costa, P. M., Teixeira, M. A. L., Ferreira, M. S. G., Costa, M. H., and Costa, F. O. (2013). Enhanced primers for amplification of DNA barcodes from a broad range of marine metazoans. BMC Ecol. 13:34. doi: 10.1186/1472-6785-13-34
Lobo, J., Shokralla, S., Costa, M. H., Hajibabaei, M., and Costa, F. O. (2017). DNA metabarcoding for high-throughput monitoring of estuarine macrobenthic communities. Sci. Rep. 7:15618.
Macheriotou, L., Guilini, K., Bezerra, T. N., Tytgat, B., Nguyen, D. T., Nguyen, T. X. P., et al. (2019). Metabarcoding free-living marine nematodes using curated 18S and CO1 reference sequence databases for species-level taxonomic assignments. Ecol. Evol. 9, 1211–1226. doi: 10.1002/ece3.4814
Machida, R. J., Leray, M., Ho, S. L., and Knowlton, N. (2017). Data Descriptor: metazoan mitochondrial gene sequence reference datasets for taxonomic assignment of environmental samples. Sci. Data 4:170027.
Marquina, D., Esparza-Salas, R., Roslin, T., and Ronquist, F. (2019). Establishing arthropod community composition using metabarcoding: surprising inconsistencies between soil samples and preservative ethanol and homogenate from Malaise trap catches. Mol. Ecol. Resour. 19, 1516–1530. doi: 10.1111/1755-0998.13071
Martinez Arbizu, P. (2019). pairwiseAdonis: Pairwise Multilevel Comparison Using Adonis.”. R Package Version 0.3 ed.
Martins, F. M. S., Galhardo, M., Filipe, A. F., Teixeira, A., Pinheiro, P., Pauperio, J., et al. (2019). Have the cake and eat it: optimizing nondestructive DNA metabarcoding of macroinvertebrate samples for freshwater biomonitoring. Mol. Ecol. Resour. 19, 863–876. doi: 10.1111/1755-0998.13012
Mora, C., Tittensor, D. P., Adl, S., Simpson, A. G. B., and Worm, B. (2011). How many species are there on earth and in the ocean? PLoS Biol. 9:e1001127. doi: 10.1371/journal.pbio.1001127
Motokawa, T. (1984). Connective-tissue catch in echinoderms. Biol. Rev. Camb Philos. Soc. 59, 255–270. doi: 10.1111/j.1469-185x.1984.tb00409.x
Pawlowski, J., Kelly-Quinn, M., Altermatt, F., Apotheloz-Perret-Gentil, L., Beja, P., Boggero, A., et al. (2018). The future of biotic indices in the ecogenomic era: integrating (e) DNA metabarcoding in biological assessment of aquatic ecosystems. Sci. Total Environ. 637, 1295–1310. doi: 10.1016/j.scitotenv.2018.05.002
Porter, T. M., and Hajibabaei, M. (2018). Automated high throughput animal CO1 metabarcode classification. Sci. Rep. 8:4226.
Quinn, G., and Keough, M. (2002). Experimental Design and Data Analysis for Biologists. New York, NY: Cambridge University Press.
R Core Team (2019). R: A Language and Environment for Statistical Computing. Vienna: R Foundation for Statistical Computing.
Rey, A., Basurko, O. C., and Rodriguez-Ezpeleta, N. (2020). Considerations for metabarcoding-based port biological baseline surveys aimed at marine nonindigenous species monitoring and risk assessments. Ecol. Evol. 10, 2452–2465. doi: 10.1002/ece3.6071
Ritari, J., Salojarvi, J., Lahti, L., and De Vos, W. M. (2015). Improved taxonomic assignment of human intestinal 16S rRNA sequences by a dedicated reference database. BMC Genomics 16:1056. doi: 10.1186/s12864-015-2265-y
Shokralla, S., Porter, T. M., Gibson, J. F., Dobosz, R., Janzen, D. H., Hallwachs, W., et al. (2015). Massively parallel multiplex DNA sequencing for specimen identification using an Illumina MiSeq platform. Sci. Rep. 5:9687. doi: 10.1038/srep09687
Van Hoey, G., Degraer, S., and Vincx, M. (2004). Macrobenthic community structure of soft-bottom sediments at the belgian continental shelf. Estuar. Coast. Shelf Sci. 59, 599–613. doi: 10.1016/j.ecss.2003.11.005
Van Hoey, G., Wischnewski, J., Craeymeersch, J., Dannheim, J., Enserink, L., Guerin, L., et al. (2019). Methodological elements for optimising the spatial monitoring design to support regional benthic ecosystem assessments. Environ. Monit. Assess. 191:423.
Wang, Q., Garrity, G. M., Tiedje, J. M., and Cole, J. R. (2007). Naive Bayesian classifier for rapid assignment of rRNA sequences into the new bacterial taxonomy. Appl. Environ. Microbiol. 73, 5261–5267. doi: 10.1128/aem.00062-07
Wangensteen, O. S., Palacin, C., Guardiola, M., and Turon, X. (2018). DNA metabarcoding of littoral hard-bottom communities: high diversity and database gaps revealed by two molecular markers. Peerj 6:e4705. doi: 10.7717/peerj.4705
Zenker, M. M., Specht, A., and Fonseca, V. G. (2020). Assessing insect biodiversity with automatic light traps in Brazil: pearls and pitfalls of metabarcoding samples in preservative ethanol. Ecol. Evol. 10, 2352–2366. doi: 10.1002/ece3.6042
Zizka, V. M. A., Elbrecht, V., Macher, J. N., and Leese, F. (2019a). Assessing the influence of sample tagging and library preparation on DNA metabarcoding. Mol. Ecol. Resour. 19, 893–899. doi: 10.1111/1755-0998.13018
Keywords: metabarcoding, macrobenthos, ethanol preservative, eDNA, bulk DNA, morphological traits, North Sea
Citation: Derycke S, Maes S, Van den Bulcke L, Vanhollebeke J, Wittoeck J, Hillewaert H, Ampe B, Haegeman A, Hostens K and De Backer A (2021) Detection of Macrobenthos Species With Metabarcoding Is Consistent in Bulk DNA but Dependent on Body Size and Sclerotization in eDNA From the Ethanol Preservative. Front. Mar. Sci. 8:637858. doi: 10.3389/fmars.2021.637858
Received: 07 December 2020; Accepted: 13 May 2021;
Published: 15 June 2021.
Edited by:
Iliana B. Baums, Pennsylvania State University (PSU), United StatesReviewed by:
Daniel Garcia-Souto, University of Vigo, SpainMarta Paterno, University of Salerno, Italy
Copyright © 2021 Derycke, Maes, Van den Bulcke, Vanhollebeke, Wittoeck, Hillewaert, Ampe, Haegeman, Hostens and De Backer. This is an open-access article distributed under the terms of the Creative Commons Attribution License (CC BY). The use, distribution or reproduction in other forums is permitted, provided the original author(s) and the copyright owner(s) are credited and that the original publication in this journal is cited, in accordance with accepted academic practice. No use, distribution or reproduction is permitted which does not comply with these terms.
*Correspondence: Sofie Derycke, c29maWUuZGVyeWNrZUBpbHZvLnZsYWFuZGVyZW4uYmU=