- 1Department of Microbiology and Immunology, University of Otago, Dunedin, New Zealand
- 2Department of Marine Science, University of Otago, Dunedin, New Zealand
- 3National Institute of Water and Atmospheric Research (NIWA)/University of Otago Research Centre for Oceanography, Dunedin, New Zealand
- 4Department of Functional & Evolutionary Ecology, University of Vienna, Vienna, Austria
Sinking organic particles from surface waters provide key nutrients to the deep ocean, and could serve as vectors transporting microbial diversity to the deep ocean. However, the effect of this seasonally varying connectivity with the surface on deep microbial communities remains unexplored. Here, a three-year time-series from surface and deep (500 m) waters part of the Munida Microbial Observatory Time-Series (MOTS) was used to study the seasonality of epipelagic and mesopelagic prokaryotic communities. The goal was to establish how seasonally dynamic these two communities are, and any potential linkages between them. Both surface and deep prokaryotic communities displayed seasonality with high variation in community diversity. Deep prokaryotic communities mirrored the seasonal patterns in heterotrophic production and bacterial abundance displayed by surface communities, which were related to changes in chlorophyll-a concentration. However, the magnitude of this temporal variability in deeper waters was generally smaller than in the surface. Detection of surface prokaryotes in the deep ocean seemed seasonally linked to phytoplankton blooms, but other copiotrophic or typically algal-associated surface groups were not detected in the mesopelagic suggesting only specific populations were surviving the migration down the water column. We show transfer of organisms across depths is possibly not always unidirectional, with typically deep ocean microbes being seasonally abundant in surface waters. This indicates the main mechanism linking surface and deep communities changes seasonally: sinking of organic particles during productive periods, and vertical convection during winter overturning.
Introduction
Seasonal blooms of photosynthetic primary producers (i.e., phytoplankton) drive global ocean productivity (Sigman and Hain, 2012). Phytoplankton fix carbon and supply global oceans with both organic carbon and energy allowing survival of most life in the ocean. Of this fixed carbon, approximately 50% is recycled in surface waters by heterotrophic bacteria while the rest enters the marine food web via grazers, or sinks to fuel deep ocean life (Cole et al., 1988; Ducklow et al., 1993; Mitchell and Kirchman, 2008; Herndl and Reinthaler, 2013). This provision of carbon and energy through sinking particles is termed the biological carbon pump (Eppley and Peterson, 1979). This mechanism implies that not only surface but also deep ocean microbial communities might be strongly connected to the presence and extent of surface phytoplankton blooms. Evidence of this connection has been demonstrated in the Southern Ocean by Richert et al. (2019) where both epipelagic and mesopelagic communities (not size fractioned) were linked to phytoplankton. This was also shown in the Atlantic, Indian and (North) Pacific oceans by Ruiz-González et al. (2020) where communities from larger (>3 μm) size fractions were linked to surface primary production in both mesopelagic and bathypelagic waters.
Phytoplankton blooms in surface waters are seasonal and trigger microbial community successions (Ducklow et al., 1993; Bird and Karl, 1999; Arrieta and Herndl, 2002; Teeling et al., 2012). It has been suggested that environmental factors are most important when defining variation in seasonal communities, particularly day length, with >65% of the variance in microbial communities attributed to it (Gilbert et al., 2012). In contrast, the deep ocean is considered a more stable environment; in part due to the lack of light cycles associated with surface periods of production and senescence (Giovannoni and Vergin, 2012).
This linkage of surface and deep water through the biological carbon pump predicts that transfer of carbon and energy is key, and bacterioplankton processes across the deep ocean have been shown to be potentially coupled with fluxes in sinking particles (Nagata et al., 2000; Hansell and Ducklow, 2003; Yokokawa et al., 2013; Santoro et al., 2017). Since particles are readily colonised by microorganisms, the substantial transport of carbon to the bathypelagic zone (estimated at 0.04 Pmol C yr–1) via the biological carbon pump (Honjo et al., 2014), represents a great potential for transfer of organisms. Settling of marine snow and particulate organics play a role in shaping deep microbial communities by “seeding” these ecosystems with surface organisms (Mestre et al., 2018). The synoptic study by Ruiz-González et al. (2020) demonstrated that when surface productivity is higher, there is stronger vertical connectivity in communities associated with large particles (20–200 μm). In the same sampling event Mestre et al. (2018) demonstrates that the largest sinking particles facilitate surface and bathypelagic (waters 4,000 m deep) prokaryotic community connections the best. The link from surface to deep communities is clear, whether it is directly via transport, or through the facilitation of carbon or nutrient transport. Others have suggested advection or mixing of water masses can also play a role in shaping community composition (Wilkins et al., 2013; Luna et al., 2016; Severin et al., 2016) with the potential for deep water mixing up to 800 m recorded in subantarctic waters, the same water mass as the present study (Rintoul and Trull, 2001). However, since these were all synoptic studies, it remains unclear how dynamic these deeper communities are, and the amount and identity of organisms being transferred over different seasons/years. This is particularly important bearing in mind the strong seasonal nature of surface marine ecosystems, seasonally shifting from productive to non-productive periods. The shift in seasons and subsequent change in quality and quantity of particle export to the deep ocean has been linked to changes in deep sea bacterioplankton processes (Tamburini et al., 2003). A long term study by Cram et al. (2015) based on DNA fingerprinting showed evidence of seasonal patterns in microbial communities at the surface and very bottom of the water column, but not at intermediate depths as sampled in the present study. The source of particle-attached communities reaching the bathypelagic, and their biological processes, change seasonally at station ALOHA (Poff et al., 2021), but the presence of seasonal connections from the surface to the entire community (both particle attached and free living) from deeper layers (500 m) remains unclear.
In the present study, we examine community dynamics by analysing 3 years of chlorophyll-a concentration data along with prokaryotic production, abundance and community (both free-living and particle attached) composition data (16S rRNA gene amplicons) collected from surface (2 m) and deep (500 m) waters from a single station in the Southern Ocean part of the Munida Microbial Observatory Time-Series (MOTS). We aim to observe how seasonally dynamic both surface and deep microbial communities are and detect the presence of any linkages between them. We test the hypothesis that seasonal variations in surface phytoplankton communities will result in buffered but detectable seasonal variability in deeper waters through vertical transfer of nutrients or cells. We demonstrate that both surface and deep water show seasonal dynamics, with larger variation in surface waters. We provide evidence supporting a potential link between surface and deep waters, but rather than direct transfer of primary producers (e.g., Synechococcus) which was minimal, we detect heterotrophic groups involved in successive turnover of nutrients and surface specific microbial groups associated with phytoplankton blooms. In our seasonal and inter-annual study we also reveal that the linkage between surface and deep-water communities is not straightforward just based on the sinking of particles during surface phytoplankton blooms, but that the dominant transport mechanism changes seasonally, with winter overturning playing a key role.
Materials and Methods
Study Site and Sampling
Samples were collected from one station from the Munida Microbial Observatory Time-Series (MOTS1) (Supplementary Figure 1). The Munida transect spans the waters associated with the subtropical front (STF) in the South Pacific Ocean off the South Island of New Zealand. The Munida time series transect was established in 1998 to investigate long term changes in carbonate chemistry, but since then several other works have taken place and sampling for MOTS began in 2014 (Currie and Hunter, 1999; Currie et al., 2011; Brix et al., 2013; Jones et al., 2013; Baltar et al., 2015, 2016; Morales et al., 2018; Thomson et al., 2019, 2020; Allen et al., 2020). The transect extends 65 km offshore and encompasses coastal neritic waters, modified subtropical waters of the Southland Current, the STF and subantarctic waters (SAW) (Jillett, 1969). This frontal system is shaped by the continental shelf beneath, and although there is some seasonal variation in their spatial distribution, the water masses are a constant feature (Jillett, 1969). Samples for this study were taken from the eastern end (i.e., most oceanic) station (8), that lies within SAW (45.833 S 171.500 E). These waters are characterised by high (macro) nutrient and low chlorophyll (HNLC) concentrations, due to trace metal limitations (Fe) (Behrenfeld et al., 2008).
Samples were collected bimonthly when possible on the RV Polaris II, from a total of 15 sampling cruises from January 2014 to March 2017. Surface samples were collected with an onboard continuous pump with the inlet located approximately 2 m below the surface, and deep water samples (500m) were collected (weather permitting, with the exception of April 2015 and March 2017) with a Niskin bottle attached to a conductivity temperature depth (CTD) profiler. All samples were collected into acid washed plastic bottles prior to processing. Samples (500 mL) for chlorophyll-a (Chl-a) analysis were filtered on-board on Whatman GF/F filters using low vacuum (e.g., <200 mm Hg). Following filtration, these filters were folded and placed into pre-labelled 15 mL polypropylene centrifuge tubes and then frozen. These filters were extracted in 90% acetone for 16–24 h and measured using a pre-calibrated Turner Designs Fluorometer as previously described (Parsons et al., 1984).
For prokaryotic community composition, samples were prepared as described in Baltar et al. (2016). Briefly, duplicate 0.5–1 L water samples were filtered through 47 mm 0.22 μm polycarbonate filters and stored on board at –20°C, then transferred to –80°C on shore.
Seasons were determined by meteorological season classification in the Southern Hemisphere where summer begins 1st December, autumn the 1st March, winter the 1st June and spring the 1st September.
Heterotrophic Prokaryotic Abundance and Production Quantification
Bacterial abundance was determined via epifluorescence microscopy for samples up until March 2016, after which counts were made with a flow cytometer (FCM). These two methods have been shown to be comparable when counting bacteria in aquatic samples (Monfort and Baleux, 1992). Samples for both methods were immediately fixed onboard with glutaraldehyde (0.5–2% final concentration) for 15 min and frozen in liquid N2. Epifluorescence microscopy was performed as described by Baltar et al. (2015). Briefly, 10–15 mL for each sample was stained with 5 μgmL–1 final concentration of 4′,6-diamidino-2-phenylindole (DAPI) for 5 min, then filtered through 0.22 μm, 25 mm black polycarbonate filters. Filters were placed on a glass slide with immersion oil and a cover slip, then counted on a Zeiss Universal microscope under UV excitation. Flow cytometric methods were derived from Marie et al. (1997) and Gasol and del Giorgio (2000) and performed on a FACS Canto II (Benton and Dickinson) with a blue laser (488 nm). A 0.4 mL volume of sample was stained with 1:10,000 final concentration of Sybr Green I for 15 min in the dark. Samples were run through the cytometer at low speed for 2 min each and FL1 positive events counted on a FL1 vs. SSC plot using FlowJo10 software.
Heterotrophic prokaryotic production was obtained from the incorporation rate of [3H]leucine as described by Smith and Azam (1992). For each sample, triplicate 1.2 mL were incubated with 3H-Leucine (specific activity 150 Ci mmol–1, final concentration 20 nM) for 1–2 h, immediately after collection. Each sample also had a 1.2 mL control with the addition of trichloroacetic acid (TCA) (final concentration 5%) before incubation with 3H-Leucine. Incorporation of the radio labelled leucine was stopped with the addition of TCA (final concentration 5%), before storage at –20°C. The levels of isotope incorporation were determined after 24–48 h incubation with 1 mL of scintillation cocktail on a Tri-Carb Liquid Scintillation Counters scintillation counter (Perkin-Elmer) with quenching correction.
Community Profiling
DNA was extracted from filters with a Qiagen DNeasy Powersoil kit, as per the manufacturer’s instructions, with the bead shaking step modified with substitution of a vortex adapter for 10 min with a SPEX SamplePrep 1600 MiniG for 2 × 1 min. Duplicate samples were processed and analysed separately throughout. 16S rRNA gene amplification was performed using the Earth Microbiome Project protocol and barcoded primer set (V4 region: 515F [5′-GTGTGCCAGCMGCCGCGGTAA-3′] and 806R [5′-GGACTACHVGGGTWTCTAAT-3′]) and then sequenced in an Illumina MiSeq 2 × 150 bp run for samples January 2014–April 2015 (21 samples), and an Illumina HiSeq 2 × 150 bp run for August 2015–March 2017 (34 samples) (Caporaso et al., 2012). Runs were combined and quality filtered and assigned to amplicon sequence variants (ASVs) with the dada2 package and associated pipeline (Callahan et al., 2016). Taxonomy was assigned using SILVA 132 release reference database (Quast et al., 2013). All further analysis was performed in RStudio with the phyloseq package (McMurdie and Holmes, 2013) and visualised with ggplot2 (Wickham, 2009). Samples were rarefied 10 times using the rarefy_even_depth command to randomly select 3,700 reads (this excluded a failed April 2015 surface sample with three reads) before ASV tables were merged. This level of sampling was chosen to retain the highest number of samples while still retaining maximal reads (Supplementary Figure 2). Alpha diversity measures (Shannon and richness) were calculated using the estimate_richness command. Beta diversity was assessed with an NMDS ordination generated with the ordinate function based on Bray-Curtis dissimilarity. To show the effect of seasonality for each depth group, a Bray Curtis dissimilarity matrix was calculated using the vegan package (Oksanen et al., 2019). The matrix was filtered to show only comparisons to summer samples for surface and deep separately, and then grouped by season to visualise the change in dissimilarity through seasons. To show changes in taxonomic groups between seasons, reads were grouped at order level and taxa with relative abundance greater than 5% shown. To find which taxonomic groups were contributing to observed seasonal changes, a generalised linear model was generated for each pair of seasons for surface and deep communities separately with edgeR (Robinson et al., 2009). From each model, taxonomic groups with a false discovery rate <0.1 were considered seasonal as a result of different abundances between each pair of seasons. These were combined giving a separate list of seasonal ASVs for each depth which were then visualised as above at the genus level when possible with ampvis2. To show potential linkages between surface and deep communities, ASVs considered seasonal at both surface and deep but showing a lag in their peak were selected and their relative abundance over time visualised with ampvis2. All R code available at https://github.com/JWenley/MOTS-Surface-Deep. All sequence data from this study are available in NCBI under BioProject PRJNA576923.
Statistical Analyses
A Kruskal-Wallis test was performed for Chl-a, bacterial abundance and bacterial production to test whether there was a change in these parameters over the 15 sampling time points for both surface and deep waters. A Kruskal-Wallis test was also used to confirm if there was a difference in the mean ranks between each season for both surface and deep prokaryotic communities from the Bray Curtis dissimilarity matrix. Kruskal-Wallis tests were used to assess alpha diversity, while beta diversity was assessed with analysis of similarity (ANOSIM) and the Adonis function from the vegan package (Permutational multivariate analysis of variance) to test similarity firstly between depths, and then between seasons in each depth group separately.
Results and Discussion
Surface Phytoplankton Blooms Drive Seasonality in the Deep Ocean
A critical finding in this study is the first confirmation of a seasonally dynamic mesopelagic (500 m) microbial community in subantarctic waters. Seasonality within both surface and deep waters was evident across multiple microbial parameters during the sampling period (Figure 1). A Kruskal-Wallis test (KW) confirmed significant differences across the sampling time points for chlorophyll-a (Chl-a) as a proxy for phytoplankton biomass (surface: Chi square = 27, p = 0.01, df = 13) (see Supplementary Table 1), bacterial abundance (surface: Chi square = 27, p = 0.01, df = 13, deep: Chi square = 23, p = 0.03, df = 12) and bacterial production (surface: Chi square = 25, p = 0.01, df = 12, deep: Chi square = 21, p = 0.03, df = 11) with change over time indicative of seasonality. More than a 2-fold change in surface Chl-a was detected from peak bloom in early autumn to winter (0.47 mg/m3 in March 2015 to 0.16 mg/m3 in August 2015) (Supplementary Table 1), with concentrations consistent with previously recorded Chl-a levels in subantarctic surface waters (Boyd et al., 2004; Jones et al., 2013). This was also associated with elevated heterotrophic bacterial production, which increased up to 16-fold in surface waters during bloom periods (268.5 pmol Leu/l/h in March 2015 and 16.7 pmol Leu/l/h in March 2017). In contrast, Chl-a concentrations in deep waters were below detection level (0.02 mg/m3) indicating both an expected lack of primary producer blooms in the deep and little transfer of Chl-a from surface to deep. Similar cycles of activity were found for both bacterial abundance and production across surface and deep waters, although they appeared dampened in deep water. Interestingly, a disruption in the seasonal cycle was observed which coincided with an El-Niño event that occurred from July 2015 to April 2016 (shaded region Figure 1). This event may have influenced seasonal patterns as highlighted in Chl-a and bacterial production levels which typically peak during summer/early autumn but were absent for 2016. Bacterioplankton production and abundance generally decrease with depth (Nagata et al., 2000) and production has recently been shown to be negatively correlated with species richness 2 weeks after a surface phytoplankton bloom (Baumas et al., 2021). Ruiz-González et al. (2020) explored changes in bacterial abundance and production across different stations in relation to surface properties, and found linkages between prokaryotic production from bathypelagic layers and surface microplankton (20–200 μm) groups and surface dissolved organic matter quality. Richert et al. (2019) found clear changes in surface bacterial abundance and richness related to surface chlorophyll, while trends for mesopelagic were not significant.
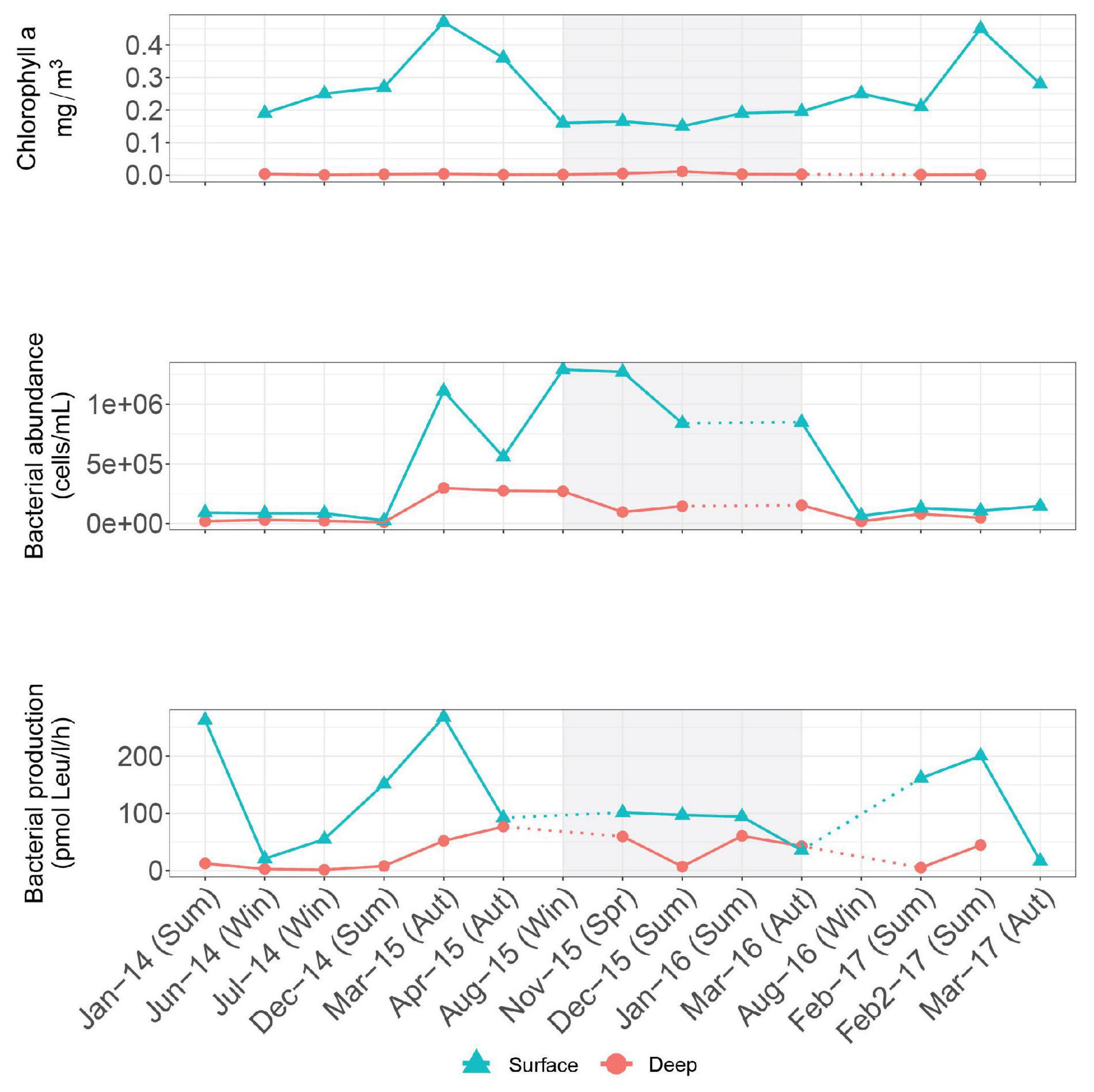
Figure 1. A 3-year time-series of subantarctic surface and deep (500 m) water chlorophyll-a, prokaryotic abundance and heterotrophic production samples taken from the Munida Microbial Observatory Time-Series (MOTS). Shaded area shows period of El Niño, dashed lines indicate missing data and meteorological seasons are indicated for each sampling date: Spr, spring; Sum, summer; Aut, autumn; Win, winter.
Prokaryotic community alpha diversity was greatest and more evenly distributed in winter (average richness = 177) for surface samples and declined to a low point during spring (average richness = 122) (Figure 2A). Deep communities were the most diverse and evenly distributed in summer (average richness = 258), with almost double the observed richness of surface communities (Figure 2A). This is likely due to surface samples being dominated by only a few copiotrophic taxa during periods of blooms. This observation has been made in the Southern Ocean by Richert et al. (2019) where an increase in surface Chl-a correlated with a decrease in surface bacterioplankton community diversity and an increase in mesopelagic community diversity, but this may be location dependent as a different observation was made in the Baltic Sea with the highest community diversity in surface waters at the very beginning of blooms before peak Chl-a was reached (Camarena-Gómez et al., 2021). Although these changes in the present study were not statistically different, trends were still visible when collating the variation over 3 years into seasons.
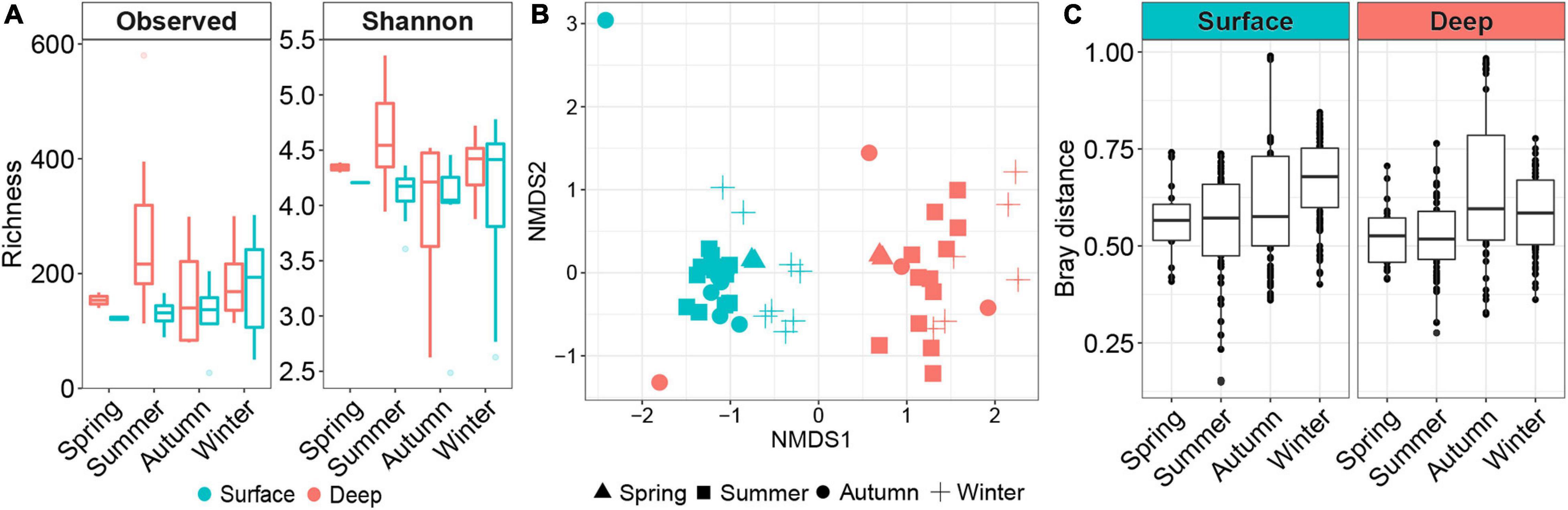
Figure 2. Seasonal variation in surface (blue) and deep (500 m) (red) water prokaryotic community (A) alpha diversity, both observed and Shannon index, (B) beta diversity visualised with non-metric multidimensional scaling (NMDS) based on Bray-Curtis dissimilarities with seasons depicted with different shapes (stress = 0.108), and (C) comparison of prokaryotic community composition seasonal dissimilarity where all samples are compared to summer for surface and deep separately. Samples were collected from the Munida Microbial Observatory Time-Series (MOTS) over a 3-year period and grouped into meteorological seasons.
Changes in prokaryotic communities were distinguished most notably by depth (ANOSIM by depth: R = 0.82, p = 0.001), with some seasonal separation evident (ANOSIM season by depth: R = 0.62, p = 0.001) (Adonis season by depth: F = 5.8, p = 0.001), particularly for winter samples which were more clearly separated for both depths (Figure 2B). Many other studies have also observed community changes with depth across global oceans (Cram et al., 2015; Luna et al., 2016; Mestre et al., 2018; Richert et al., 2019; Baumas et al., 2021). This was consistent with an increase in community dissimilarity as communities transitioned from spring to winter in surface samples (KW surface: Chi-squared = 41.897, df = 3, p < 0.001) (Figure 2C). A similar pattern was observed in deep communities, but community dissimilarity peaked in autumn (KW deep: Chi-squared = 26.452, df = 3, p < 0.001) (Figure 1C). Despite this seasonality, large variation was observed within each season suggesting that although seasonal changes are cyclical, the communities at each season can be variable over multiple years. Needham and Fuhrman (2016) showed that following a surface bloom, the subsequent surface communities are more highly correlated to each other, rather than to other environmental parameters. This suggests that whichever taxa spikes in abundance during/following bloom periods will then determine the succession of the community, resulting in the high variability we see between seasons (Figure 2C).
Seasonal changes were associated with transitions in dominant taxa, with shifts in a few key groups at the taxonomic order level for both surface and deep waters (Figure 3). Bacteroidetes (Flavobacteriales) dominated surface communities in summer and autumn. Proteobacteria, in particular the ubiquitous SAR11, were consistently abundant in surface and deep waters regardless of season. Camarena-Gómez et al. (2021) also observed the constant presence of SAR11 in surface waters, while previous studies have reported their presence from surface to mesopelagic depths (Morris et al., 2005; Cram et al., 2015). The Cyanobacteria Synechococcales was present throughout all seasons at the surface with peak relative abundance in winter. This group did not show seasonal variability in the deep and were consistently found in low (<1%) relative abundance suggesting that direct transfer is limited. Previous studies have detected photosynthetic material in the bathypelagic zone, however, associated with or attached to sinking particles rather than free living (Agusti et al., 2015; Poff et al., 2021). In contrast, the Thaumarcheaota (Nitrosopumilales) were always abundant in the deep but were also significantly abundant in surface waters only during winter, quickly disappearing during spring (<1% relative abundance) and absent in summer and autumn (Figure 3). Thaumarcheaota have been previously shown to prefer a free-living lifestyle in bathypelagic waters (Salazar et al., 2015) with the highest abundance among endemic bathypelagic taxa in smaller/free living size fractions (Ruiz-González et al., 2020).
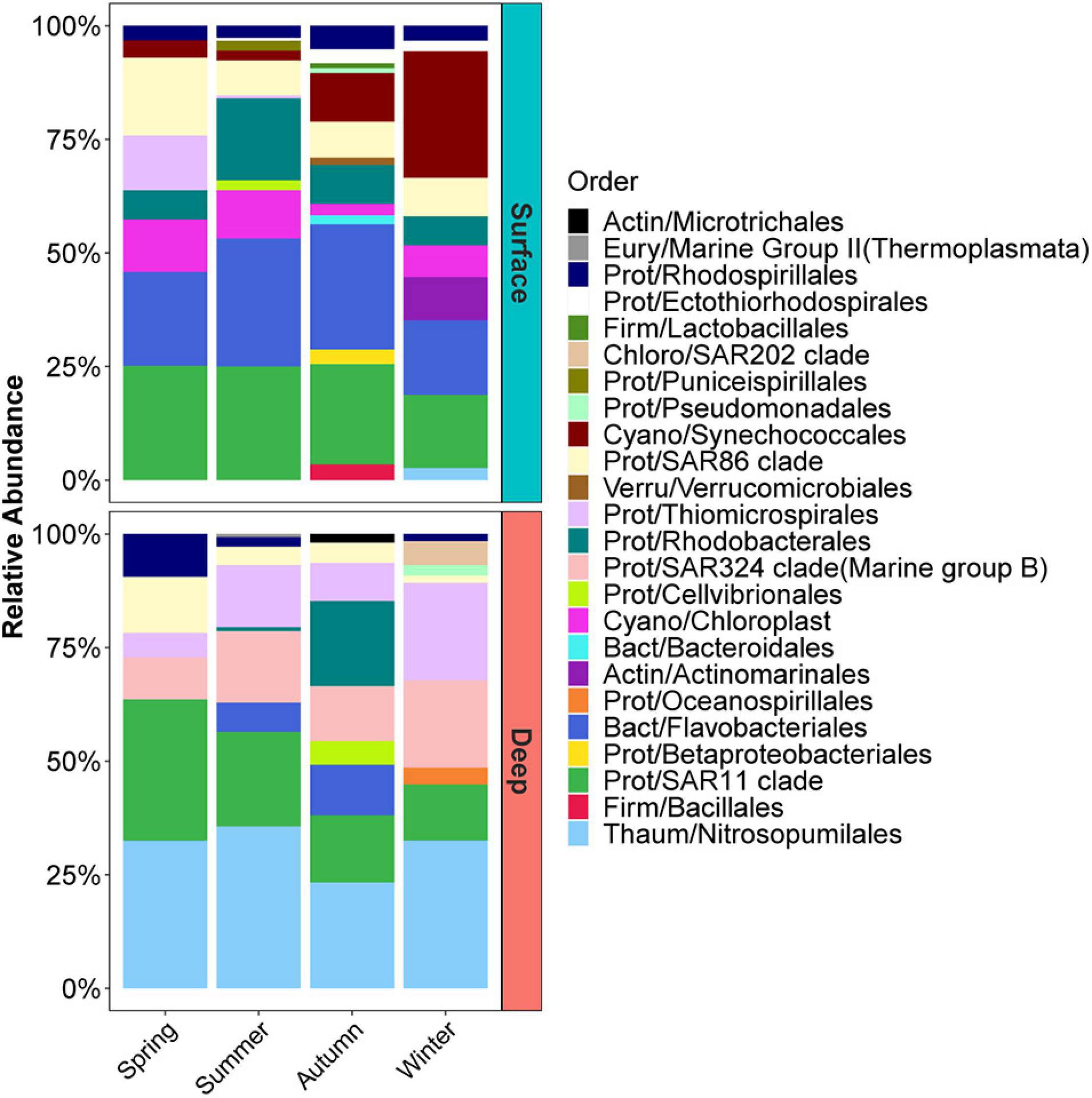
Figure 3. Seasonal changes in subantarctic surface and deep (500 m) water prokaryotic community composition of taxa with >5% relative abundance grouped at order level. Corresponding Phylum groups are indicated as follows: Actin, Actinobacteria; Bact, Bacteroidetes; Chloro, Chloroflexi; Cyano, Cyanobacteria; Eury, Euryarchaeota; Firm, Firmicutes; Prot, Proteobacteria; Thaum, Thaumarchaeota; Verru, Verrucomicrobia. Samples were collected over 3 years from the Munida Microbial Observatory Time-Series (MOTS) and grouped into meteorological seasons.
Distinct communities were identified for surface and deep, but conserved patterns were recognised. In winter when surface production ceases, the presence of endemic deep taxa at the surface, their associated genes (Könneke et al., 2014) and a more evenly distributed community could suggest that vertical convection mixes the communities, transporting surface oligotrophs down, and deep free-living archaea up. This is also supported by the decrease in temperature stratification in winter months where surface temperatures are nearer that of the deep water, potentially enabling deep water mixing to occur (Supplementary Table 1 and Supplementary Figure 3; Brix et al., 2013).
In the only other available study comparing seasonal community composition of surface and mesopelagic prokaryotes (although based on the fingerprinting technique ARISA–Automated Ribosomal Intergenic Spacer Analysis) a seasonal pattern in community structure was shown at the bottom of a coastal hypoxic ocean basin (890°m) at the San Pedro Ocean Time series, but no seasonality was detected at 500°m (Cram et al., 2015). Those results were attributed to sinking particles, despite using a methodology that excluded particle associated communities (by pre-filtering). The lack of detectable seasonality at 500°m could be due to a potential uncoupling between surface and intermediate depths as discussed by Ruiz-González et al. (2020), who also observed a weaker connection between surface conditions and mesopelagic communities. Others have posited that observed annual changes in prokaryotic communities can be attributed to convective overturn rather than sinking particles (Morris et al., 2005).
Key Taxa Associated With Community Seasonality
We screened a total of 2,371 ASVs to determine what taxa may be driving community changes between each season. Of these, only 28 were statistically identified as seasonal from the surface and 34 from the deep (Figure 4). As depth appears to be the main driver of community differences (Figure 2B) and we suspect seasonal differences in the mechanism linking surface and deep communities, surface and deep samples were treated separately for this analysis. The top most relative abundant seasonal surface ASV belonged to the SUP05 cluster (Gammaproteobacteria) followed by Synechococcus (Cyanobacteria). Some typically endemic deep taxa, mostly associated with the Archaea, were also seasonally abundant in the surface including Nitrosopumilus and Nitrosopelagicus (Thaumarchaeota) with the greatest relative abundance during winter (Figure 5). Seasonal deep taxa were dominated by SUP05 cluster (Gammaproteobacteria), Amylibacter (Alphaproteobacteria), and Halomonas (Gammaproteobacteria). Interestingly, many of the deep seasonal taxa were groups found in previous studies typically associated with phytoplankton blooms (many with algicidal potential): Amylibacter, NS4 marine group (Flavobacteriaceae), Ulvibacter, and SAR92 (Gammaproteobacteria), all of which were present in surface samples in the present study (Teeling et al., 2012; Buchan et al., 2014; Hahnke et al., 2015). Their presence, along with Synechococcus, in the deep ocean is assumed as a result of drifting down from the surface as aggregates of organic matter form. Consistently, most of these taxa had higher relative abundance in autumn, immediately after or on the tail end of surface bloom periods (Figure 4).
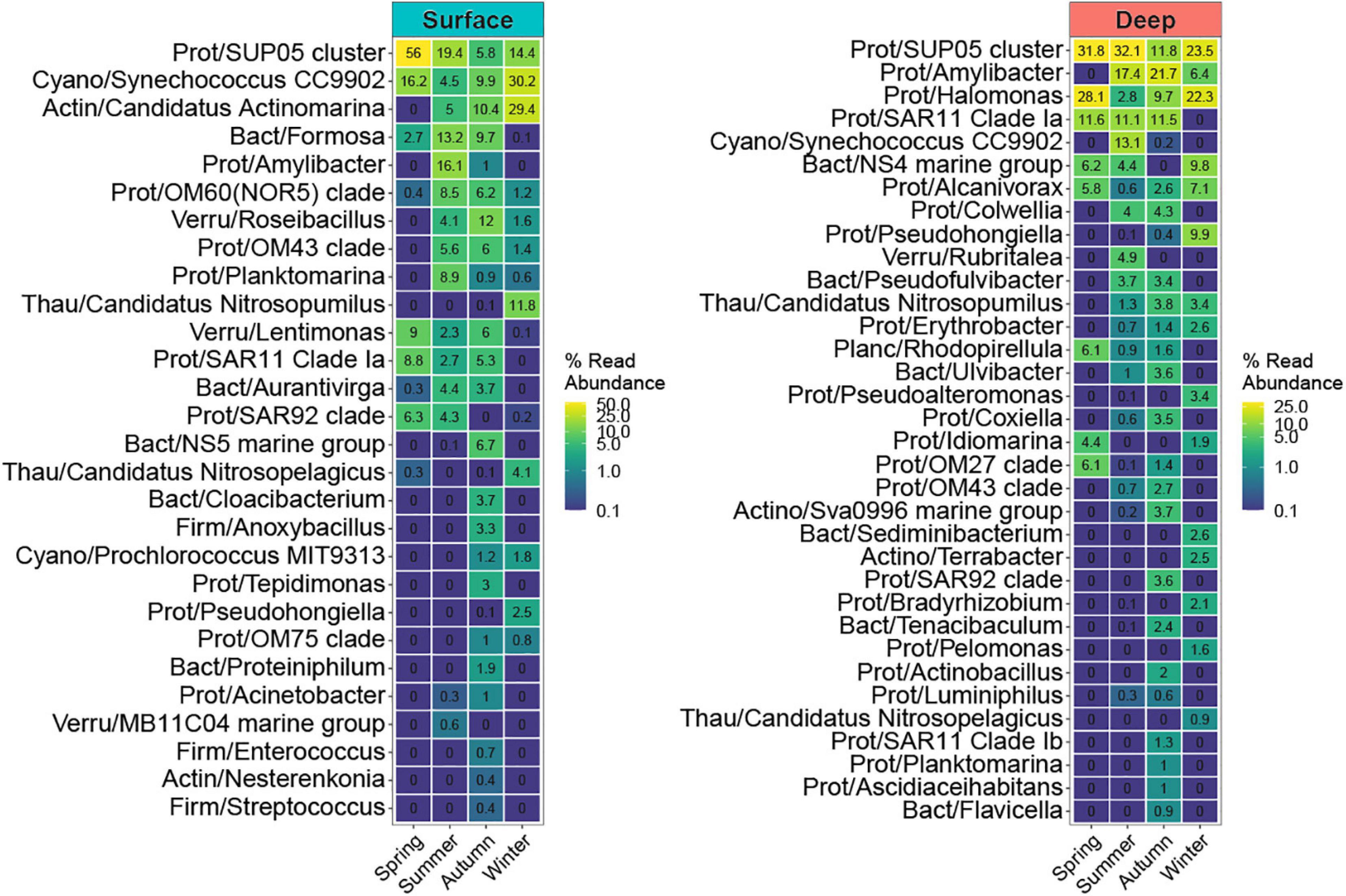
Figure 4. Prokaryotic community members associated with surface and deep (500 m) water seasonality. Seasonally changing taxa were determined from a generalised linear model generated from every combination pairing of meteorological seasons for surface and deep communities separately, collected over 3 years from the Munida Microbial Observatory Time-Series (MOTS). A total of 28 seasonal ASVs were found for the surface and 34 for deep waters, identified here at genus level where possible.
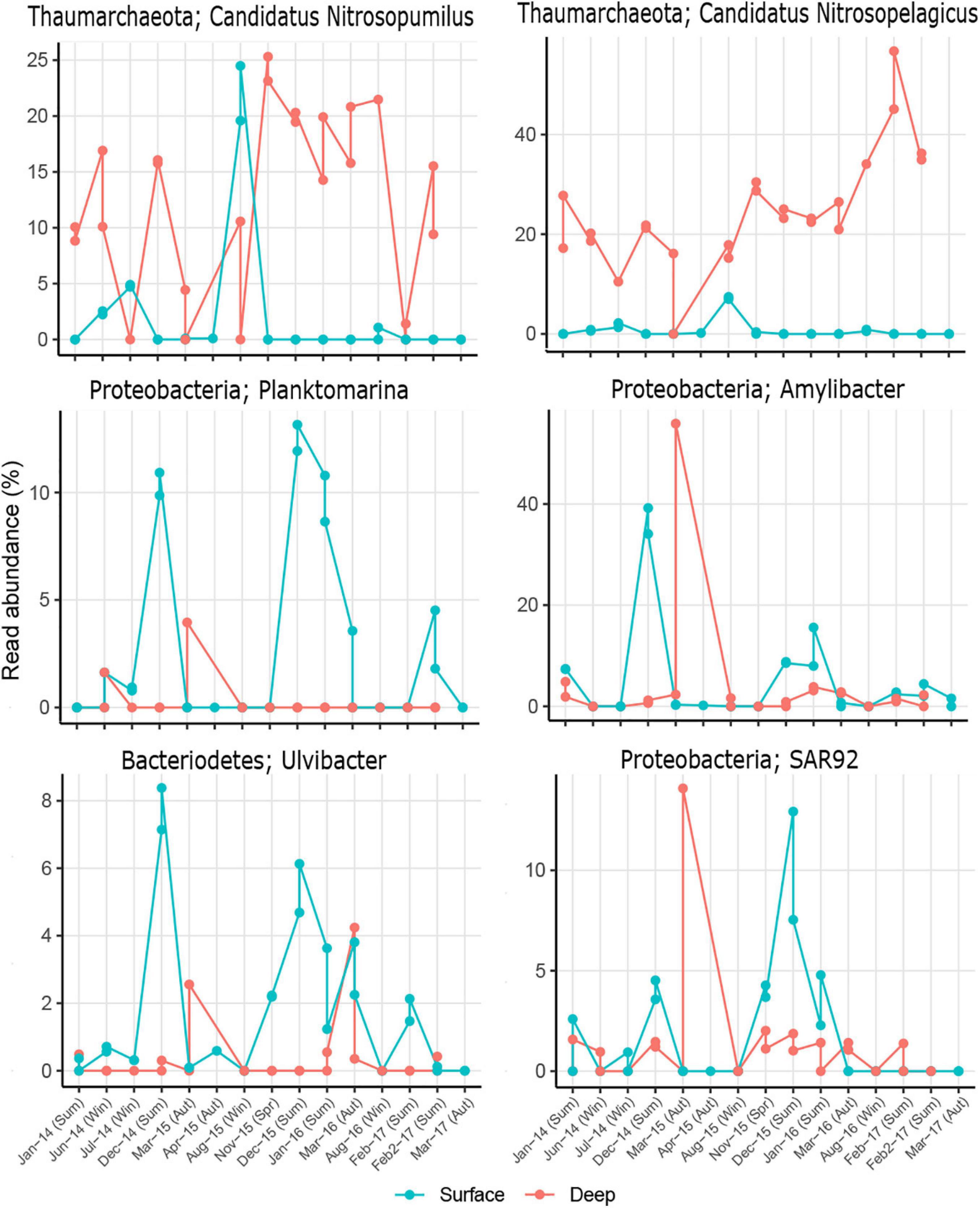
Figure 5. Seasonally changing taxa determined from a generalised linear model showing potential linkages between surface and deep (500 m) waters either by deep water mixing during winter (Candidatus Nitrosopumilus, Candidatus Nitrosopelagicus) or by sinking from the surface as indicated by a lag in peak abundance in deep waters (Planktomarina, Amylibacter, Ulvibacter, and SAR92). Samples were collected over 3 years from the Munida Microbial Observatory Time-Series (MOTS) with sampling dates and their corresponding meteorological season indicated as Spr, spring; Sum, summer; Aut, autumn, and Win, winter.
We investigated in more detail potential linkages between surface and deep communities by identifying taxa showing seasonality across both surface and deep but showing a lag in their peak abundance (Figure 5). The occurrence of this pattern was not consistent year to year, highlighting the variability of the prokaryotic community and the ability to only capture broad scale patterns with our sampling regime. Of the 34 ASVs showing seasonality in the deep, only four were also identified in the surface that fit our criteria: Planktomarina, Amylibacter, Ulvibacter, and SAR92. These taxa have been previously associated with blooms and phytoplankton degradation, so presence in the deep ocean following a peak in surface relative abundance could result from the remnants of surface bloom material drifting down (Giebel et al., 2011; Hahnke et al., 2015). As demonstrated by Mestre et al. (2018), the largest/fastest particles are better at facilitating these connections to the deep ocean, and the original phytoplankton source of these particles is also a determinant of deep bacterioplankton communities (Ruiz-González et al., 2020).
Bidirectional Exchange of Prokaryotic Diversity to the Deep Ocean
Several other studies link sinking particles to changes in deep microbial communities and their associated processes (Nagata et al., 2000; Hansell and Ducklow, 2003; Yokokawa et al., 2013; Santoro et al., 2017), while mixing/advection is also a previously proposed mechanism in different areas (Wilkins et al., 2013; Luna et al., 2016; Severin et al., 2016). We propose that at our study site, both phenomena occur and change seasonally with the sinking of organic particles following surface phytoplankton blooms, indicated by the presence of typically surface and photosynthesising taxa in the deep (Figure 4), and vertical convection during winter indicated by the presence of endemic deep taxa at the surface and a less stratified water column (Figures 4, 5, Supplementary Table 1, and Supplementary Figure 3). It has been previously shown that larger particles sink faster (Smayda, 1970) and that they contain more similar prokaryotic communities throughout the water column, suggesting they are better at providing linkages to the deep ocean (Mestre et al., 2018). Although Steinberg et al. (2001) did not find a strong relationship between particle flux and primary production over a 10 year time series at the Bermuda Atlantic Time-series Study (BATS), they did see a strong correlation between mixed layer depth and particle flux, suggesting that either sinking particles or vertical mixing could influence prokaryotic community transport between surface and deep waters. Vertical mixing could be an explanation for the presence of endemic deep taxa in surface waters during winter (Figures 3, 5), and the lower diversity of seasonal taxa at depth in winter (Figure 3) as vertical mixing may potentially homogenise entire surface and deep communities rather than only selecting for taxa on sinking particles. We would, however, expect winter communities from both surface and deep to be more closely clustered, rather than the general closer proximity of surface winter samples to all deep samples observed here (Figure 1). As the surface and deep communities are mixed there could be other selective pressures at play that only allow some deep taxa to thrive in surface waters, perhaps similar to the observation by Wilkins et al. (2013) that advection shapes microbial communities by increased potential for colonisation rather than transport of entire communities. In winter, subantarctic waters have been shown to mix down to a maximum depth of 800 m, so this could still be a possible mechanism (Rintoul and Trull, 2001).
Conclusion
Collectively, our study reveals the occurrence of seasonally changing prokaryotic communities, not only in surface waters as expected but also in deep/mesopelagic waters, along with the organisms driving seasonal changes. Additionally we show that the mechanism responsible for the linkage between surface and deep prokaryotic communities is not only through a direct sinking of organic particles that would transport surface bacteria into the deep (Mestre et al., 2018) but that vertical convection can also facilitate transport of microbes between both depth layers. Moreover, we show that the mechanism responsible for the surface-deep prokaryotic community connection changes seasonally, so that during bloom-post bloom periods (spring-summer) the sinking of particles would be a key process transporting microbes, but during winter months, overturning via vertical convection would play a more critical role. Further investigation into the mechanisms of linkages between surface and deep prokaryotic communities is required, which will help to elucidate the obscurities of the dark ocean.
Data Availability Statement
The datasets presented in this study can be found in online repositories. The names of the repository/repositories and accession number(s) can be found below: https://www.ncbi.nlm.nih.gov/, PRJNA576923.
Author Contributions
FB and SM were responsible for the project management. JW, KC, SL, and BT collected and processed samples, with KC also providing research vessel access. JW and SL analysed data. JW wrote the manuscript with assistance from SM and FB. All authors commented on the manuscript.
Funding
FB was supported by a Rutherford Discovery Fellowship (Royal Society of New Zealand).
Conflict of Interest
The authors declare that the research was conducted in the absence of any commercial or financial relationships that could be construed as a potential conflict of interest.
Acknowledgments
We would like to thank the skippers and crew of the RV Polaris II, namely Bill Dickson and Evan Kenton, for their help throughout the sampling events.
Supplementary Material
The Supplementary Material for this article can be found online at: https://www.frontiersin.org/articles/10.3389/fmars.2021.659641/full#supplementary-material
Footnotes
References
Agusti, S., González-Gordillo, J. I., Vaqué, D., Estrada, M., Cerezo, M. I., Salazar, G., et al. (2015). Ubiquitous healthy diatoms in the deep sea confirm deep carbon injection by the biological pump. Nat. Commun. 6:7608. doi: 10.1038/ncomms8608
Allen, R., Summerfield, T., Currie, K., Dillingham, P., and Hoffmann, L. (2020). Distinct processes structure bacterioplankton and protist communities across an oceanic front. Aquat. Microb. Ecol. 85, 19–34. doi: 10.3354/ame01938
Arrieta, J. M., and Herndl, G. J. (2002). Changes in bacterial β-glucosidase diversity during a coastal phytoplankton bloom. Limnol. Oceanogr. 47, 594–599. doi: 10.4319/lo.2002.47.2.0594
Baltar, F., Currie, K., Stuck, E., Roosa, S., and Morales, S. E. (2016). Oceanic fronts: transition zones for bacterioplankton community composition. Environ. Microbiol. Rep. 8, 132–138. doi: 10.1111/1758-2229.12362
Baltar, F., Stuck, E., Morales, S., and Currie, K. (2015). Bacterioplankton carbon cycling along the Subtropical Frontal Zone off New Zealand. Prog. Oceanogr. 135, 168–175. doi: 10.1016/j.pocean.2015.05.019
Baumas, C. M. J., Le Moigne, F. A. C., Garel, M., Bhairy, N., Guasco, S., Riou, V., et al. (2021). Mesopelagic microbial carbon production correlates with diversity across different marine particle fractions. ISME J. 15, 1695–1708. doi: 10.1038/s41396-020-00880-z
Behrenfeld, M. J., Halsey, K. H., and Milligan, A. J. (2008). Evolved physiological responses of phytoplankton to their integrated growth environment. Philos. Trans. R. Soc. Lond. B Biol. Sci. 363, 2687–2703. doi: 10.1098/rstb.2008.0019
Bird, D. F., and Karl, D. M. (1999). Uncoupling of bacteria and phytoplankton during the austral spring bloom in Gerlache Strait, Antarctic Peninsula. Aquat. Microb. Ecol. 19, 13–27.
Boyd, P. W., McTainsh, G., Sherlock, V., Richardson, K., Nichol, S., Ellwood, M., et al. (2004). Episodic enhancement of phytoplankton stocks in New Zealand subantarctic waters: contribution of atmospheric and oceanic iron supply. Global Biogeochem. Cycles 18:GB1029. doi: 10.1029/2002GB002020
Brix, H., Currie, K. I., and Mikaloff Fletcher, S. E. (2013). Seasonal variability of the carbon cycle in subantarctic surface water in the South West Pacific. Global Biogeochem. Cycles 27, 200–211. doi: 10.1002/gbc.20023
Buchan, A., LeCleir, G. R., Gulvik, C. A., and González, J. M. (2014). Master recyclers: features and functions of bacteria associated with phytoplankton blooms. Nat. Rev. Microbiol. 12:686. doi: 10.1038/nrmicro3326
Callahan, B. J., McMurdie, P. J., Rosen, M. J., Han, A. W., Johnson, A. J. A., and Holmes, S. P. (2016). DADA2: high-resolution sample inference from Illumina amplicon data. Nat. Methods 13, 581–583. doi: 10.1038/nmeth.3869
Camarena-Gómez, M. T., Ruiz-González, C., Piiparinen, J., Lipsewers, T., Sobrino, C., Logares, R., et al. (2021). Bacterioplankton dynamics driven by interannual and spatial variation in diatom and dinoflagellate spring bloom communities in the Baltic Sea. Limnol. Oceanogr. 66, 255–271. doi: 10.1002/lno.11601
Caporaso, J. G., Lauber, C. L., Walters, W. A., Berg-Lyons, D., Huntley, J., Fierer, N., et al. (2012). Ultra-high-throughput microbial community analysis on the Illumina HiSeq and MiSeq platforms. ISME J. 6, 1621–1624. doi: 10.1038/ismej.2012.8
Cole, J. J., Findlay, S., and Pace, M. L. (1988). Bacterial production in fresh and saltwater ecosystems: a cross-system overview. Mar. Ecol. Prog. Ser. Oldendorf 43, 1–10.
Cram, J. A., Chow, C.-E. T., Sachdeva, R., Needham, D. M., Parada, A. E., Steele, J. A., et al. (2015). Seasonal and interannual variability of the marine bacterioplankton community throughout the water column over ten years. ISME J. 9, 563–580. doi: 10.1038/ismej.2014.153
Currie, K., Reid, M., and Hunter, K. (2011). Interannual variability of carbon dioxide drawdown by subantarctic surface water near New Zealand. Biogeochemistry 104, 23–34. doi: 10.1007/s10533-009-9355-3
Currie, K. I., and Hunter, K. A. (1999). Seasonal variation of surface water CO2 partial pressure in the Southland Current, east of New Zealand. Mar. Freshw. Res. 50, 375–382. doi: 10.1071/MF98115
Ducklow, H. W., Kirchman, D. L., Quinby, H. L., Carlson, C. A., and Dam, H. G. (1993). Stocks and dynamics of bacterioplankton carbon during the spring bloom in the eastern North Atlantic Ocean. Deep Sea Res. II Top. Stud. Oceanogr. 40, 245–263. doi: 10.1016/0967-0645(93)90016-G
Eppley, R. W., and Peterson, B. J. (1979). Particulate organic matter flux and planktonic new production in the deep ocean. Nature 282, 677–680. doi: 10.1038/282677a0
Gasol, J. M., and del Giorgio, P. A. (2000). Using flow cytometry for counting natural planktonic bacteria and understanding the structure of planktonic bacterial communities. Sci. Mar. 64, 197–224.
Giebel, H.-A., Kalhoefer, D., Lemke, A., Thole, S., Gahl-Janssen, R., Simon, M., et al. (2011). Distribution of Roseobacter RCA and SAR11 lineages in the North Sea and characteristics of an abundant RCA isolate. ISME J. 5, 8–19. doi: 10.1038/ismej.2010.87
Gilbert, J. A., Steele, J. A., Caporaso, J. G., Steinbruck, L., Reeder, J., Temperton, B., et al. (2012). Defining seasonal marine microbial community dynamics. ISME J. 6, 298–308. doi: 10.1038/ismej.2011.107
Giovannoni, S. J., and Vergin, K. L. (2012). Seasonality in ocean microbial communities. Science 335, 671–676. doi: 10.1126/science.1198078
Hahnke, R. L., Bennke, C. M., Fuchs, B. M., Mann, A. J., Rhiel, E., Teeling, H., et al. (2015). Dilution cultivation of marine heterotrophic bacteria abundant after a spring phytoplankton bloom in the North Sea. Environ. Microbiol. 17, 3515–3526. doi: 10.1111/1462-2920.12479
Hansell, D. A., and Ducklow, H. W. (2003). Bacterioplankton distribution and production in the bathypelagic ocean: directly coupled to particulate organic carbon export? Limnol. Oceanogr. 48, 150–156. doi: 10.4319/lo.2003.48.1.0150
Herndl, G. J., and Reinthaler, T. (2013). Microbial control of the dark end of the biological pump. Nat. Geosci. 6, 718–724. doi: 10.1038/ngeo1921
Honjo, S., Eglinton, T. I., Taylor, C. D., Ulmer, K. M., Sievert, S. M., Bracher, A., et al. (2014). Understanding the role of the biological pump in the global carbon cycle: an imperative for ocean science. Oceanography 27, 10–16.
Jillett, J. B. (1969). Seasonal hydrology of waters off the Otago Peninsula, South-Eastern New Zealand. N. Z. J. Mar. Freshwater Res. 3, 349–375. doi: 10.1080/00288330.1969.9515303
Jones, K. N., Currie, K. I., McGraw, C. M., and Hunter, K. A. (2013). The effect of coastal processes on phytoplankton biomass and primary production within the near-shore Subtropical Frontal Zone. Estuar. Coast. Shelf Sci. 124, 44–55. doi: 10.1016/j.ecss.2013.03.003
Könneke, M., Schubert, D. M., Brown, P. C., Hügler, M., Standfest, S., Schwander, T., et al. (2014). Ammonia-oxidizing archaea use the most energy-efficient aerobic pathway for CO2 fixation. Proc. Natl. Acad. Sci. U. S. A. 111, 8239–8244. doi: 10.1073/pnas.1402028111
Luna, G. M., Chiggiato, J., Quero, G. M., Schroeder, K., Bongiorni, L., Kalenitchenko, D., et al. (2016). Dense water plumes modulate richness and productivity of deep sea microbes. Environ. Microbiol. 18, 4537–4548. doi: 10.1111/1462-2920.13510
Marie, D., Partensky, F., Jacquet, S., and Vaulot, D. (1997). Enumeration and cell cycle analysis of natural populations of marine picoplankton by flow cytometry using the nucleic acid stain SYBR Green I. Appl. Environ. Microbiol. 63, 186–193. doi: 10.1128/aem.63.1.186-193.1997
McMurdie, P. J., and Holmes, S. (2013). phyloseq: an R package for reproducible interactive analysis and graphics of microbiome census data. PLoS One 8:e61217. doi: 10.1371/journal.pone.0061217
Mestre, M., Ruiz-González, C., Logares, R., Duarte, C. M., Gasol, J. M., and Sala, M. M. (2018). Sinking particles promote vertical connectivity in the ocean microbiome. Proc. Natl. Acad. Sci. U. S. A. 115:E6799. doi: 10.1073/pnas.1802470115
Monfort, P., and Baleux, B. (1992). Comparison of flow cytometry and epifluorescence microscopy for counting bacteria in aquatic ecosystems. Cytometry 13, 188–192. doi: 10.1002/cyto.990130213
Morales, S. E., Meyer, M., Currie, K., and Baltar, F. (2018). Are oceanic fronts ecotones? Seasonal changes along the subtropical front show fronts as bacterioplankton transition zones but not diversity hotspots. Environ. Microbiol. Rep. 10, 184–189. doi: 10.1111/1758-2229.12618
Morris, R. M., Vergin, K. L., Cho, J.-C., Rappé, M. S., Carlson, C. A., and Giovannoni, S. J. (2005). Temporal and spatial response of bacterioplankton lineages to annual convective overturn at the Bermuda Atlantic Time-series Study site. Limnol. Oceanogr. 50, 1687–1696. doi: 10.4319/lo.2005.50.5.1687
Nagata, T., Fukuda, H., Fukuda, R., and Koike, I. (2000). Bacterioplankton distribution and production in deep Pacific waters: large–scale geographic variations and possible coupling with sinking particle fluxes. Limnol. Oceanogr. 45, 426–435. doi: 10.4319/lo.2000.45.2.0426
Needham, D. M., and Fuhrman, J. A. (2016). Pronounced daily succession of phytoplankton, archaea and bacteria following a spring bloom. Nat. Microbiol. 1:16005. doi: 10.1038/nmicrobiol.2016.5
Oksanen, J., Blanchet, F. G., Friendly, M., Kindt, R., Legendre, P., McGlinn, D., et al. (2019). vegan: Community Ecology Package. R package version 2.5-5. Available online at: https://CRAN.R-project.org/package=vegan.
Parsons, T. R., Maita, Y., and Lalli, C. M. (1984). A Manual of Chemical and Biological Methods for Seawater Analysis. Oxford: Pergamon Press.
Poff, K. E., Leu, A. O., Eppley, J. M., Karl, D. M., and DeLong, E. F. (2021). Microbial dynamics of elevated carbon flux in the open ocean’s abyss. Proc. Natl. Acad. Sci. U. S. A. 118:e2018269118. doi: 10.1073/pnas.2018269118
Quast, C., Pruesse, E., Yilmaz, P., Gerken, J., Schweer, T., Yarza, P., et al. (2013). The SILVA ribosomal RNA gene database project: improved data processing and web-based tools. Nucleic Acids Res. 41, D590–D596. doi: 10.1093/nar/gks1219
Richert, I., Yager, P. L., Dinasquet, J., Logares, R., Riemann, L., Wendeberg, A., et al. (2019). Summer comes to the Southern Ocean: how phytoplankton shape bacterioplankton communities far into the deep dark sea. Ecosphere 10:e02641. doi: 10.1002/ecs2.2641
Rintoul, S. R., and Trull, T. W. (2001). Seasonal evolution of the mixed layer in the Subantarctic zone south of Australia. J. Geophys. Res. Oceans 106, 31447–31462. doi: 10.1029/2000JC000329
Robinson, M. D., McCarthy, D. J., and Smyth, G. K. (2009). edgeR: a Bioconductor package for differential expression analysis of digital gene expression data. Bioinformatics 26, 139–140. doi: 10.1093/bioinformatics/btp616
Ruiz-González, C., Mestre, M., Estrada, M., Sebastián, M., Salazar, G., Agustí, S., et al. (2020). Major imprint of surface plankton on deep ocean prokaryotic structure and activity. Mol. Ecol. 29, 1820–1838. doi: 10.1111/mec.15454
Salazar, G., Cornejo-Castillo, F. M., Borrull, E., Díez-Vives, C., Lara, E., Vaqué, D., et al. (2015). Particle-association lifestyle is a phylogenetically conserved trait in bathypelagic prokaryotes. Mol. Ecol. 24, 5692–5706. doi: 10.1111/mec.13419
Santoro, A. E., Saito, M. A., Goepfert, T. J., Lamborg, C. H., Dupont, C. L., and DiTullio, G. R. (2017). Thaumarchaeal ecotype distributions across the equatorial Pacific Ocean and their potential roles in nitrification and sinking flux attenuation. Limnol. Oceanogr. 62, 1984–2003. doi: 10.1002/lno.10547
Severin, T., Sauret, C., Boutrif, M., Duhaut, T., Kessouri, F., Oriol, L., et al. (2016). Impact of an intense water column mixing (0–1500 m) on prokaryotic diversity and activities during an open-ocean convection event in the NW Mediterranean Sea. Environ. Microbiol. 18, 4378–4390. doi: 10.1111/1462-2920.13324
Sigman, D. M., and Hain, M. P. (2012). The biological productivity of the ocean. Nat. Educ. Knowl. 3:21.
Smayda, T. J. (1970). The suspension and sinking of phytoplankton in the sea. Oceanogr. Mar. Biol. Ann. Rev. 8, 353–414.
Smith, D., and Azam, F. (1992). A simple, economical method for measuring bacterial protein synthesis rates in seawater using 3H-leucine. Mar. Microb. Food Webs 6, 107–114.
Steinberg, D. K., Carlson, C. A., Bates, N. R., Johnson, R. J., Michaels, A. F., and Knap, A. H. (2001). Overview of the US JGOFS Bermuda Atlantic Time-series Study (BATS): a decade-scale look at ocean biology and biogeochemistry. Deep Sea Res. II Top. Stud. Oceanogr. 48, 1405–1447. doi: 10.1016/S0967-0645(00)00148-X
Tamburini, C., Garcin, J., and Bianchi, A. (2003). Role of deep-sea bacteria in organic matter mineralization and adaptation to hydrostatic pressure conditions in the NW Mediterranean Sea. Aquat. Microb. Ecol. 32, 209–218. doi: 10.3354/ame032209
Teeling, H., Fuchs, B. M., Becher, D., Klockow, C., Gardebrecht, A., Bennke, C. M., et al. (2012). Substrate-controlled succession of marine bacterioplankton populations induced by a phytoplankton bloom. Science 336, 608–611. doi: 10.1126/science.1218344
Thomson, B., Wenley, J., Currie, K., Hepburn, C., Herndl, G. J., and Baltar, F. (2019). Resolving the paradox: continuous cell-free alkaline phosphatase activity despite high phosphate concentrations. Mar. Chem. 214:103671. doi: 10.1016/j.marchem.2019.103671
Thomson, B., Wenley, J., Lockwood, S., Twigg, I., Currie, K., Herndl, G. J., et al. (2020). Relative importance of Phosphodiesterase vs. Phosphomonoesterase (Alkaline Phosphatase) activities for dissolved organic phosphorus hydrolysis in epi- and mesopelagic waters. Front. Earth Sci. 8:413.
Wickham, H. (2009). Ggplot2: Elegant Graphics for Data Analysis. Springer-Verlag New York. Available online at: http://ggplot2.org.
Wilkins, D., van Sebille, E., Rintoul, S. R., Lauro, F. M., and Cavicchioli, R. (2013). Advection shapes Southern Ocean microbial assemblages independent of distance and environment effects. Nat. Commun. 4:2457. doi: 10.1038/ncomms3457
Keywords: time series, community dynamics, long-term microbial observatory, marine, 16S RNA
Citation: Wenley J, Currie K, Lockwood S, Thomson B, Baltar F and Morales SE (2021) Seasonal Prokaryotic Community Linkages Between Surface and Deep Ocean Water. Front. Mar. Sci. 8:659641. doi: 10.3389/fmars.2021.659641
Received: 28 January 2021; Accepted: 31 May 2021;
Published: 21 June 2021.
Edited by:
Maria Montserrat Sala, Instituto de Ciencias del Mar, Consejo Superior de Investigaciones Científicas (CSIC), SpainReviewed by:
Clara Ruiz Gonzalez, Instituto de Ciencias del Mar, Consejo Superior de Investigaciones Científicas (CSIC), SpainMauro Celussi, National Institute of Oceanography and Experimental Geophysics (OGS), Italy
Copyright © 2021 Wenley, Currie, Lockwood, Thomson, Baltar and Morales. This is an open-access article distributed under the terms of the Creative Commons Attribution License (CC BY). The use, distribution or reproduction in other forums is permitted, provided the original author(s) and the copyright owner(s) are credited and that the original publication in this journal is cited, in accordance with accepted academic practice. No use, distribution or reproduction is permitted which does not comply with these terms.
*Correspondence: Federico Baltar, ZmVkZXJpY28uYmFsdGFyQHVuaXZpZS5hYy5hdA==; Sergio E. Morales, c2VyZ2lvLm1vcmFsZXNAb3RhZ28uYWMubno=