- 1Instituto Mediterráneo de Estudios Avanzados (UIB-CSIC), Esporles, Spain
- 2Departament de Física, Universitat de les Illes Balears, Palma, Spain
- 3French Geological Survey (BRGM), Orléans, France
- 4Global Climate Forum, Berlin, Germany
- 5Ministry of Environment, Malé, Maldives
The Maldives, with one of the lowest average land elevations above present-day mean sea level, is among the world regions that will be the most impacted by mean sea-level rise and marine extreme events induced by climate change. Yet, the lack of regional and local information on marine drivers is a major drawback that coastal decision-makers face to anticipate the impacts of climate change along the Maldivian coastlines. In this study we focus on wind-waves, the main driver of extremes causing coastal flooding in the region. We dynamically downscale large-scale fields from global wave models, providing a valuable source of climate information along the coastlines with spatial resolution down to 500 m. This dataset serves to characterise the wave climate around the Maldives, with applications in regional development and land reclamation, and is also an essential input for local flood hazard modelling. We illustrate this with a case study of HA Hoarafushi, an atoll island where local topo-bathymetry is available. This island is exposed to the highest incoming waves in the archipelago and recently saw development of an airport island on its reef via land reclamation. Regional waves are propagated toward the shoreline using a phase-resolving model and coastal inundation is simulated under different mean sea-level rise conditions of up to 1 m above present-day mean sea level. The results are represented as risk maps with different hazard levels gathering inundation depth and speed, providing a clear evidence of the impacts of the sea level rise combined with extreme wave events.
1. Introduction
Increased coastal flooding damages are among the potentially most hazardous and costliest aspects of global warming (Hinkel et al., 2014), impacting populations, ecosystems and assets. Coastal flood exposure is currently increasing at rates higher than inland due to population growth, urbanisation and the coastward migration of people (Merkens et al., 2018), and also due to coastal extreme water levels being raised by mean sea-level rise (Marcos and Woodworth, 2017). The Special Report on the Ocean and Cryosphere in a Changing Climate (SROCC) of the Intergovernmental Panel on Climate Change (IPCC) projects that if greenhouse gas (GHG) emissions continue to rise unmitigated (i.e., RCP8.5) global-mean sea levels are likely to rise by 0.6–1.1 m by 2100, and 2.3–5.4 m by 2300 (Oppenheimer et al., 2019). Projected mean sea-level rise during the twenty-first century and beyond (Kopp et al., 2014) will inevitably increase the intensity of flood events and will thus exacerbate the exposure and vulnerability of coastal areas in the decades to come, with highest impacts expected in low-lying regions. Hinkel et al. (2014) estimated that, without adaptation, by 2100 almost 5% of the global population will be potentially flooded annually, with losses of up to 10% of the global GDP, under a 1.20 m mean sea-level rise. This will require the implementation of extensive and ubiquitous coastal adaptation solutions to avoid such large impacts (Hinkel et al., 2019). But also if emissions are reduced to meet the goal of the Paris Agreement to limit global warming “well bellow 2°C” (i.e., RCP2.6), global mean sea-level is likely to rise by 0.3–0.6 m in 2100 and 0.6–1.1 m by 2300, which will still be a tremendous challenge, in particular for very low lying regions such as atoll states.
The threats of flooding events are particularly worrisome in low-lying coastal zones, including large deltas and sinking coastal mega-cities; but the regions with the largest expected relative impacts are small island states (Nurse et al., 2014). The Maldivian archipelago is an iconic case of vulnerability to mean sea-level rise. Located in the equatorial region of the Tropical Indian Ocean, the Maldives consist of 1192 islands, dispersed across 860 km from 8° north to 1° south in latitude, of which 188 are inhabited (NBS, 2017; Wadey et al., 2017) (see Figure 1). The resident population in 2014 was 437,000 people and is estimated to reach 557,000 in 2020, with 40% of the population living in the capital, Malé, and its surrounding islands Villimalé and Hulhumalé (NBS, 2019). Average land elevations range from 0.5 m to 2.3 m above present-day mean sea level (Woodworth, 2005), with 80% falling below 1 m. Since the 1950s several land reclamation projects have been carried out to address land scarcity, for example in the southern lagoon of Malé in 1954 (Maniku, 1990). With the rapid economic development of the Maldives, land reclamation projects have also increased. The Maldivian government estimates that over 1300 hectares of reef or lagoon area have been reclaimed up until 2016 (MEE, 2017). This new land is required to be elevated between 1.5 and 1.75 m above mean sea-level. However, this static approach to island elevation ignores the differing wave exposure across the archipelago.
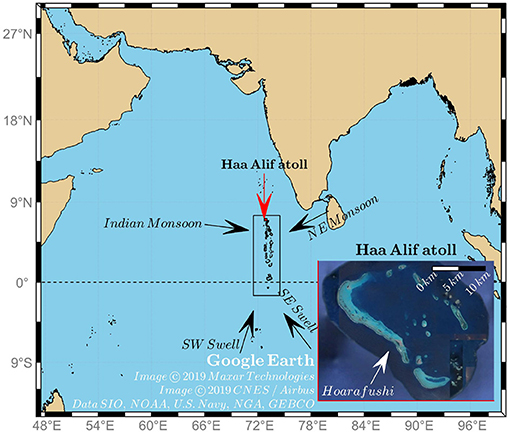
Figure 1. Map of the Indian Ocean with the Maldivian archipelago inside the black box. The four black arrows indicate the main wave direction identified. The red arrow indicates the location of the Haa Alif atoll in which is located Hoarafushi island (satellite image in the bottom-right corner extracted from Google Earth. Image © 2019 Maxar Technologies; Image © 2019 CNES/Airbus; Data SIO, NOAA, U.S. Navy, NGA, GEBCO).
A lot of land reclamation is taking place in the Maldives and a new long-term regional development strategy is currently being prepared that prioritises islands for development (Gussmann and Hinkel, 2021). While it is known that wave exposure differs across islands, this has so far not been taken into account in land reclamation and regional development. The development of adaptation plans in the framework of coastline management aimed to address flood hazards requires accurate information and a deep understanding of the driving processes. Coastal flood events are caused by extreme coastal water levels that in turn result from the combination of relative mean sea-level, tides, storm surges, wind-waves, precipitation and/or river run-off (Woodworth et al., 2019). The design of adaptation strategies therefore involves the knowledge of every individual driver and their future projections at the local scale, as well as their possible interactions (Nicholls et al., 2014). In the case of the Maldives, the tidal range is relatively small (<1 m of maximum high waters) and the storm surge contribution is negligible, as corresponds to an equatorial region (Wadey et al., 2017). Earlier studies have pointed at wind-waves as the primary mechanism causing flooding events in the Maldives, similarly to other Indian and Pacific islands (Hoeke et al., 2013). One of the first works was presented by Harangozo (2013), who investigated an event that occurred in April 1987 that flooded Mal?é, including reclaimed land below 1 m above mean sea-level and during which the hard structures designed to protect this land were destroyed. Based on altimetric wave measurements and in-situ sea-level observations, this event was attributed to prolonged swell waves originated in the Southern Indian Ocean and reaching the island during high tides. Similarly, in 2007, the Fares island, located in the southernmost atoll of the Maldives was flooded due to a series of remotely-generated swell events reaching the island (Wadey et al., 2017; Beetham and Kench, 2018) which also affected other areas of the eastern Indian Ocean (e.g., Lecacheux et al., 2012 in La Réunion Island). This event was particularly hazardous as it flooded almost the entire island and affected more than 1500 people as well as the limited water resources of the island. An extensive study was carried out in response to this event and a protective offshore breakwater was built to avoid future damages. For a comprehensive list of flooding events in the Maldives, the reader is referred to Wadey et al. (2017), where the available information of several flooding events has been collected from a number of sources.
Despite the recurrent flooding episodes associated with swells, overall, in the Maldivian archipelago a complete and accurate assessment on the wind-wave climate, including extreme waves, is hindered by the lack of observations and regionalisation of model runs. Numerical wind-wave simulations are available with a global coverage, including both re-analyses (i.e., Saha et al., 2010) and projections (i.e., Hemer and Trenham, 2016; Morim et al., 2019), although with a coarse resolution that prevents their use for many practical purposes, such as accurate local assessments. This work intends to fill this gap by providing the necessary information on waves to perform coastal studies along the Maldivian shorelines. The objectives of the present study are three-fold: first, we fully characterise the wave climate around the Maldives on the basis of global, coarse resolution numerical wave dynamical simulations for present-day, and we further evaluate the projected changes under climate change scenarios (section 3). Secondly, we downscale the extreme wave climate through propagation of the main extreme waves from the dominant directions toward the coastlines with a much higher resolution (section 4). And finally, we illustrate how this information can be translated into a flood hazard assessment in a selected location that is exposed to the largest incoming swell waves in the archipelago. To do so, we propagate wave conditions from the nearshore to the coastline under different mean sea-level rise scenarios and quantify the flooding extent with and without land reclamation (section 5). Data, methods and numerical models are described in section 2, while all the results are discussed together in section 6.
2. Data and Methods
This section describes the global wave data that is used to characterise and downscale wave information to the nearshore in the Maldives, together with the numerical models and their implementation. Local wave modelling is used as the basis of flood hazard assessment for a case study. To do so, waves are combined with a set of mean sea-level changes using a scenario-independent approach. That is, waves are propagated toward the shoreline under prescribed mean sea-level increments of 0.25, 0.5, 0.75, and 1 m with respect to present-day averaged value. Note that these values are not necessarily interpreted as climate-induced mean sea-level rise; they can also be associated to tidal oscillations or to a combination of tides and mean sea-level rise.
2.1. Global Wind-Wave Datasets
We have used the CAWCR Global wind-wave data set that is freely distributed through the CSIRO data server (Hemer et al., 2015). This set, generated with the WaveWatch III wave model (version 3.14, Tolman, 2009) in a common 1° × 1° resolution global grid, consists of a hindcast, historical runs (late twentieth century), and projections for the twenty-first century. The hindcast has been forced with surface wind fields from the NCEP CFSR (Saha et al., 2010) and covers the period from 1979 to 2009 with a temporal resolution of 1 h (this simulation is referred to as CFSR hereinafter). The historical runs and projections were generated using the output fields of 8 different CMIP5 models (ACCESS1.0, BCC-CSM1.1, CNRM-CM5, GFDL-ESM2M, HadGEM2-ES, INMCM4, MIROC5, and MRI-CGCM3), covering three different time periods with a temporal resolution of 6 h: historical runs for 1980-2005; and projected waves for mid-(2026–2045) and late-(2081–2100) twenty-first century. The projections for mid- and late-twenty-first century were run under two different emission scenarios, RCP4.5 and RCP8.5, although we will use only the latter. A detailed description of the wave climate dataset can be found in Hemer et al. (2013).
Global wave models are used to characterise the present-day and future projected changes of wave climate around the Maldivian archipelago, with emphasis on the extreme wave climate. Return levels of Hs for a set of prescribed return periods are calculated by fitting the top 1% waves to a Generalised Pareto Distribution. Given the coarse spatial resolution of the model configuration, we do not expect the small islands as the Maldives to be accurately represented by these global simulations. Given that the wave fields are modified by the presence of the islands (see for example Supplementary Video 1 from Amores and Marcos, 2019), the global fields must be downscaled in order to be usable for practical purposes. This process is described in the following.
2.2. Regional Wave Modelling
Global waves have been dynamically downscaled in the Maldives using the WaveWatchIII wave model (version 4.18, Tolman, 2014). The model was implemented on an unstructured mesh with 33160 nodes and 64456 elements over a domain ranging from 71.5 to 75.5°E in longitude and from −1.5°N to 8.5°N in latitude (black rectangle surrounding the Maldives in Figure 1). The spatial resolution of the unstructured mesh varied from 50 km along the boundaries of the domain down to 500 m in the channels between the atolls. Only the external coasts of the atoll islands were considered due to the lack of bathymetric information inside the atolls. The regional bathymetry used to build the model grid was the GEBCO bathymetry 2014 in a global 30 arc-second interval grid (https://www.gebco.net/). The wave spectrum was defined by a directional resolution of 10° and 24 frequency bands ranging non-linearly from 0.0373 to 1.1 Hz. Dynamical downscaling was preferred instead of statistical approaches because there is no local information on waves that can be used to calibrate the model.
2.3. Local Wave Modelling
Nearshore downscaled waves have been propagated toward the coastline for a case study site. The selected location corresponds to Haa Alif atoll (HA) at Hoarafushi island, located at the north of the archipelago (Figure 1). Hoarafushi has a maximum length of 2,500 m and a maximum width of 500 m (Figure 1). This site has been chosen for two main reasons: firstly, at the start of this study a land reclamation project to build a new airport next to the island was foreseen. The development of the regional airport on the newly reclaimed island on the reef of HA Hoarafushi is part of the government's regional development and decentralisation plans, which puts extra focus on the northernmost atoll Ihavandhippolhu. We therefore aimed at evaluating the exposure of this new reclaimed land to incoming waves and how its presence can alter the wave propagation over the reef and the exposure of the current island. The process of land reclamation was started on April 16th, 2019 (https://edition.mv/news/10159) and finished almost 5 months later, on September 5th, 2019 (https://edition.mv/news/12266; see Supplementary Figure 1, to see the construction process on June 15th, 2019). Secondly, information on the local bathymetry and land elevation is available and allows to simulate the wave propagation. A bathymetry around the island was generated by combining measurements on the reef flat performed by the Maldives Transport and Contracting Company, who was in charge of the design of the land reclamation project. We completed these data with reef slope measurements taken during a field trip on February 2018 (using a single beam echosounder). Our measurements included a total of 10 profiles across-slope separated around 200–500 m between them as well as several along-slope transects. The minimum depth measured in the across-slope profiles was around 3 m, that was the closest the boat could get to the reef crest, and the maximum depth recorded, that was fixed by the maximum range of the echosounder, was around 50 m. Unfortunately, there is no detailed information on the topography of the island. Instead, a constant land height of 1.5 m above present-day mean sea level has been used, according to visual inspections and in accordance with existing regulations. The coastline of the island has been represented with a constant slope, given that there are not hard structures in the oceanward side. Two topo-bathymetries have been implemented, with and without the presence of the airport. Finally, it is worth mentioning that HA Hoarafushi island is exposed to the highest incoming waves around the archipelago, as will be shown below.
The local wave propagation has used the SWASH model (Zijlema et al., 2011, code available at http://swash.sourceforge.net/) in a 2D regular grid of 6,220 m in the W-E direction and 7,320 m in the S-N direction with 10 m of spatial resolution (see the domain in Figure 8). This model is suitable for our purposes as it is capable of simulating wave setup and runup and predicting infragravity waves in the nearshore (Rijnsdorp et al., 2012), a relevant process that contributes to the amount of flooding by raising temporary the sea level near the coast. The West and South boundaries were considered active introducing the wave forcing in the domain with Jonswap spectra with a wave dispersion of 20° and a peak enhancement parameter γ of 3.3. A different combination of wave dispersion and γ was tested (5° and 10°, respectively), resulting in essentially the same results in terms of flooding. A 150 m sponge layer was placed in the eastern boundary and 1,000 m sponge layer in the northern boundary, to avoid unrealistic wave forcing from the interior of the atoll given by spurious wave reflection from the those boundaries. The lack of in-situ measurements of wave propagation and transformation along the domain made it impossible to calibrate the Manning's friction coefficient. The values for coral reefs found in the literature vary from 0.01 to 0.2. For example, Zijlema (2012) used 0.01, Prager (1991) used 0.05, Kraines et al. (1998) used 0.1, and Cialone and Smith (2007) used spatially-varying Manning's coefficient values of 0.02, 0.19, and 0.2 depending on the region of their domain. The Manning's coefficient value was finally fixed to 0.019 following Suzuki et al. (2018), who investigated the most suitable value for SWASH model applied to overtopping computation along a beach profile with defined defenses. In our case, there is not a complete beach profile, but the overtopping, which is the process of interest here, is occurring at the shoreline of a sandy beach.
With this configuration, the total simulated time for each combination of parameters was 70 min, with an initial integration time of 0.05 s and having outputs every 5 s. This is computationally intensive but still feasible for the range of experiments and for the two topographies (with and without the airport).
3. Characterisation of Wave Climate Around the Maldives
3.1. Present-Day Wave Climate
The outputs of the CFSR wave hindcast at 24 grid points around the Maldives are used to describe the large-scale present-day wave climate in the archipelago (Figure 2). Wave roses in Figure 2 identify, for each grid point, the direction of the dominant wave regimes with their corresponding significant wave heights (Hs) and peak periods (Tp). One prominent feature is that the largest significant wave heights are usually accompanied by peak periods longer than 10–12 s (and reaching up to 24 s), which suggests that these are remotely generated waves, i.e, swell waves. This is in agreement with the location of the Maldives in the Equatorial region, where winds are weak, and in a region exposed to swell waves from the Southern Ocean (Wadey et al., 2017; Amores and Marcos, 2019) and is further examined below.
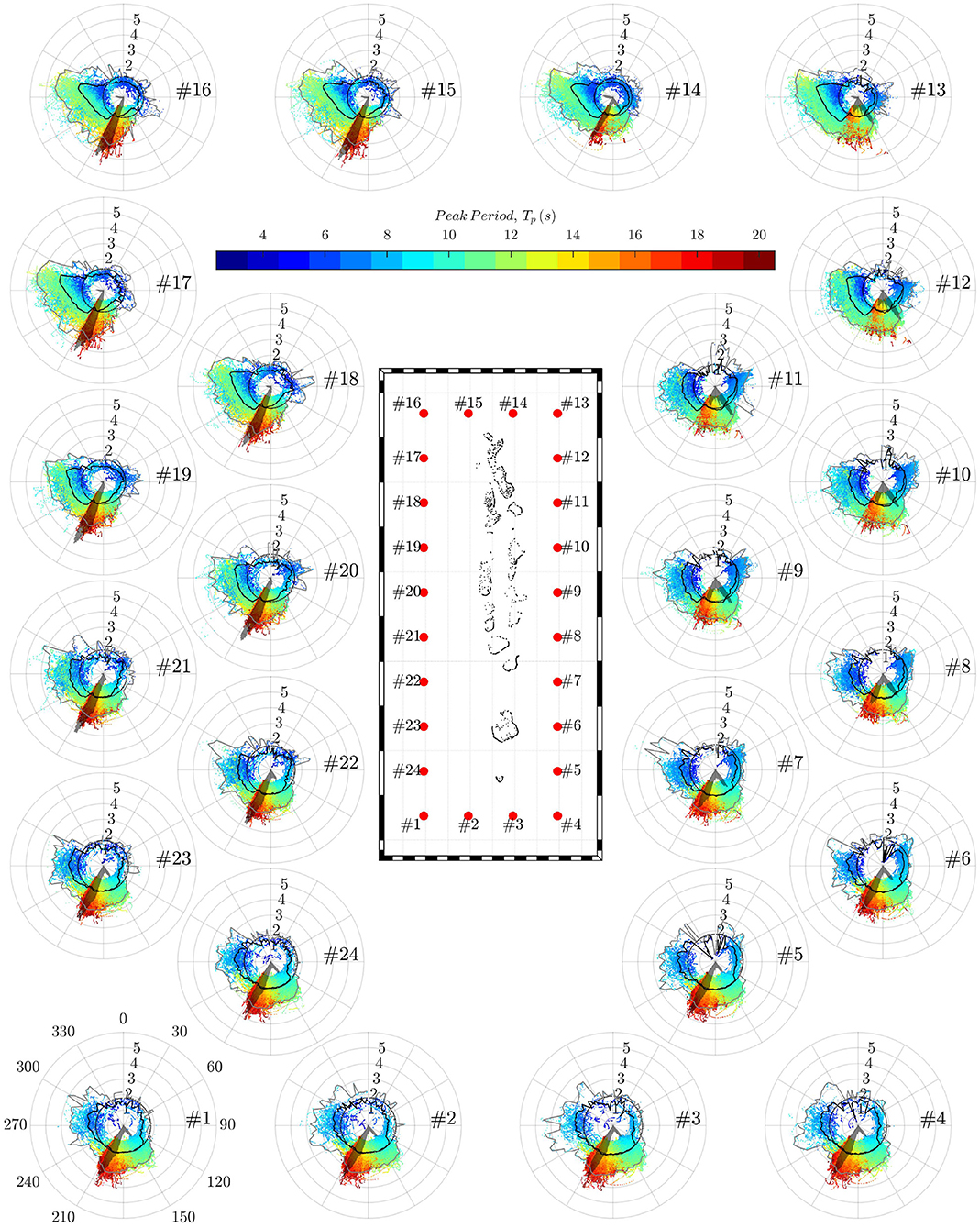
Figure 2. Distribution of the ocean wave climate around the Maldives from the CFSR Hindcast. Each wind-rose-plot corresponds to one point of the central map. The radial distance of each single point in each wind-rose indicates the wave height (m) while the azimutal value indicates the direction that the waves are coming from in nautical convention. The colour of each point shows the peak period. The continuous black (grey) line indicates the quantile 50 (99) for each direction while the dashed black like shows the quantile 50 averaging all the directions. Shadowed areas in the wave roses indicate the most frequent incoming wave directions in a 1° bins (% referred to the radial axis).
Waves from the south-west (~205°) are the most common with Hs reaching values larger than 4 m (note that the angles follow the maritime convention, as indicated by the labels in the wave rose of point #1). This finding is in line with Amores and Marcos (2019) that demonstrated that between 80 and 90% of the swell events impacting along the Maldivian coastlines are from SW and originated in a region located between south of Africa and east of South America. The second most frequent direction is the south-east (~145°). These waves reach maximum values of Hs around 3 m, thus smaller than ~205° waves, and with peak periods between 10 and 12 s. In addition to these two dominant swell wave directions, two other cases much less frequent but with non-negligible Hs are detected. In the north of the archipelago the largest waves with Hs of up to 5 m are from the west direction (~275°, see wave-rose #17 in Figure 2). And finally, waves from 60° are also found in the points of the northeastern side of the archipelago (see, for example, wave-rose #10 in Figure 2) with peak periods smaller than 10 s and Hs smaller than around 2.5 m.
The characteristics of the incoming large-scale waves are further analysed in greater detail for three grid points capturing the entire range of directions: point #17 (northwest), point #3 (south), and point #10 (northeast). Figure 3 examines the annual and seasonal distribution of incoming waves for every direction and their classification in terms of wind-seas and swells, according to the spectral partitioning provided by the global wave models. These histograms, representing the number of events per year, have been constructed with wave events separated at least 3 days to avoid over-representation of the dominant directions and with a minimum peak prominence Hs of 0.2 m to remove noise from smaller waves. The three points are representative of the four incoming wave directions identified above and all register a similar number of waves during the hindcasted period (between 45 and 50 per year, as listed in the title of the panels in Figure 3). Their distribution in directions is, however, different, and depends on their position. The most frequent wave direction, around 205°, is evident in points #17 and #3 and is equally likely throughout the entire year (see panels d, e, g, h for comparison among seasons). A composite of the wave and wind fields corresponding to these events is mapped in Supplementary Figure 2, demonstrating that these waves indeed correspond to remotely generated southwestern swells, in line with the findings in Amores and Marcos (2019). Waves from the west direction, around 275°, are the second most frequent in point #17 with a marked seasonal character, being only detected between May and October (panel d) and classified as a mixed sea+swell. These waves are generated by the Indian monsoon and only affect the northernmost area of the archipelago. The corresponding composites are shown in Supplementary Figure 3. The presence of waves generated by the Indian monsoon likely has an impact on the wave type distribution of the southwestern swell at point #17, since its percentage of sea+swell is larger between May and October; also, the wind fields of the composites corresponding to both types of waves are identical (see last rows in Supplementary Figures 2, 3).
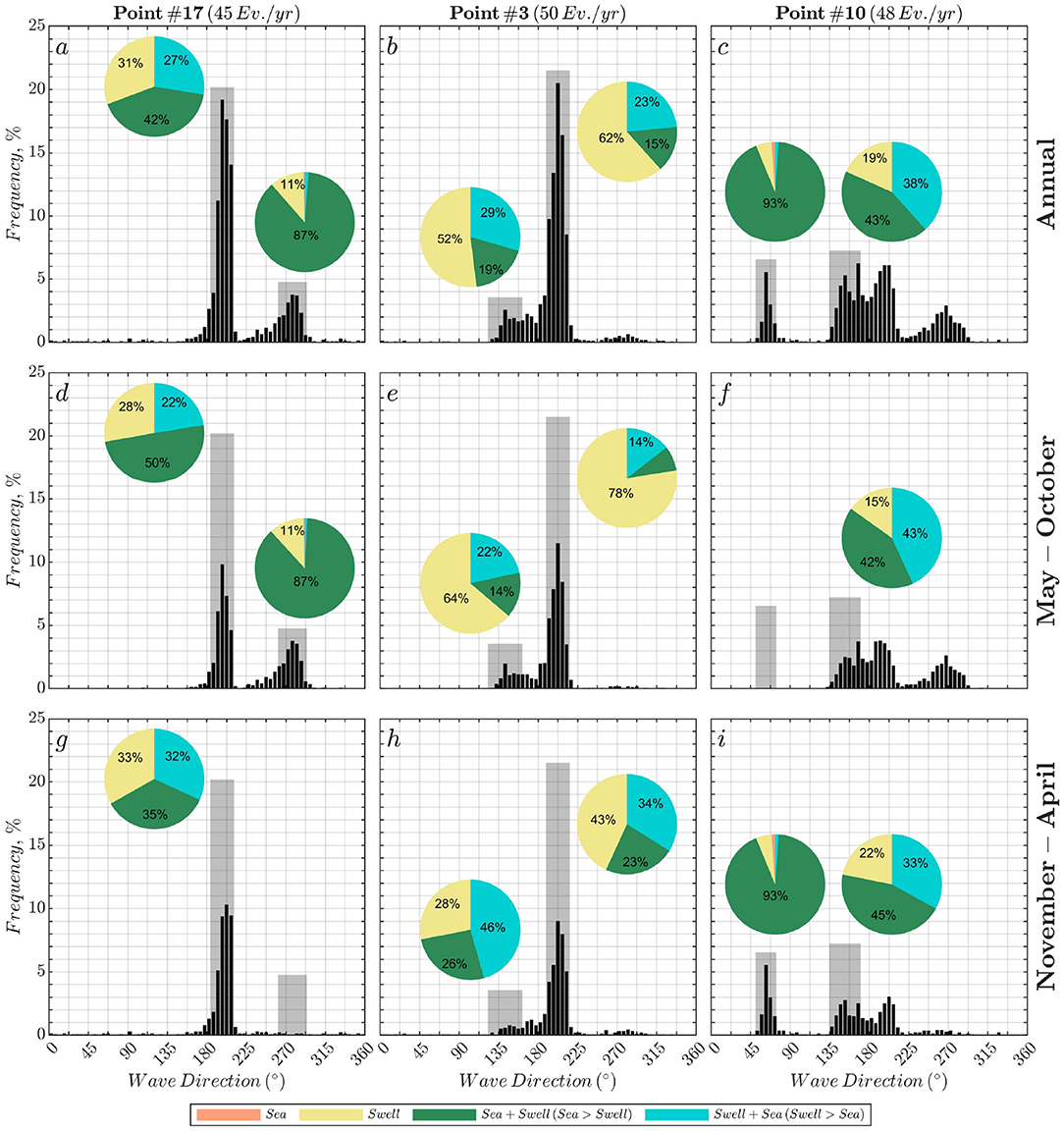
Figure 3. Histograms of wave direction (in nautical convention) registered at the three points selected as being representative (point #17 in the first column (a,d,g), #3 in the second (b,e,h), and #10 in the third) (c,f,i). The first row shows the annual histograms (a,b,c), May-October histograms are shown in the second row (d,e,f) and November-April histograms are in the last row (g,h,i). Each pie chart indicates the spectral separation by type of wave produced by WaveWatch III model [pure sea (Hs of swell = 0), pure swell (Hs of sea = 0), sea + swell dominated by sea (Hs of sea > Hs of swell) and swell + sea dominated by swell (Hs of swell > Hs of sea)] corresponding to each wave component identified (grey shadows).
The second peak in point #3, seven times less frequent that the southwestern swell and also observed in point #10, corresponds to the direction around 145°, with waves detected throughout the entire year. According to the wave and wind fields composites (Supplementary Figure 4) these are waves generated in the Southern Ocean, in a region off the southeastern coast of Australia (Amores and Marcos, 2019). Finally, the fourth incoming direction, around 55°, is clearly detected in point #10, with a strong seasonal character. These waves correspond to the northeast monsoon (Wadey et al., 2017) and are only relevant between November and April, contrasting with the Indian monsoon (panel f and i).
Return levels of Hs for every direction and for the three grid points are shown in Figure 4 and listed in Supplementary Table 1. Noteworthy are the flat tails for the southeastern swells evident in points #10 and #3. Independence among wave events is ensured with the 3-day declustering. A Generalised Pareto Distribution (GPD) has been fitted to the top 1% of the largest Hs; in the case that this subset is too small as to reliably fit the distribution, the 30 largest values (1 event per year on average) were used. The largest return levels correspond to waves generated by the Indian monsoon in point #17 (Figure 4D). This direction has a Hs of 4.75 m for a 10 year return period that is larger than all the return levels for the 500 year return periods for all cases (with the exception of the southeastern swell affecting point #3 that has 4.99 m as Hs associated with 500 year return period). On the other side, the lowest return levels correspond to the northeast monsoon affecting point #10 with a Hs equal to 2.74 m for a return period of 500 years, around 1 m lower from the closest return level (3.61 m for 500 year return period for the swell coming from southeast in point #3).
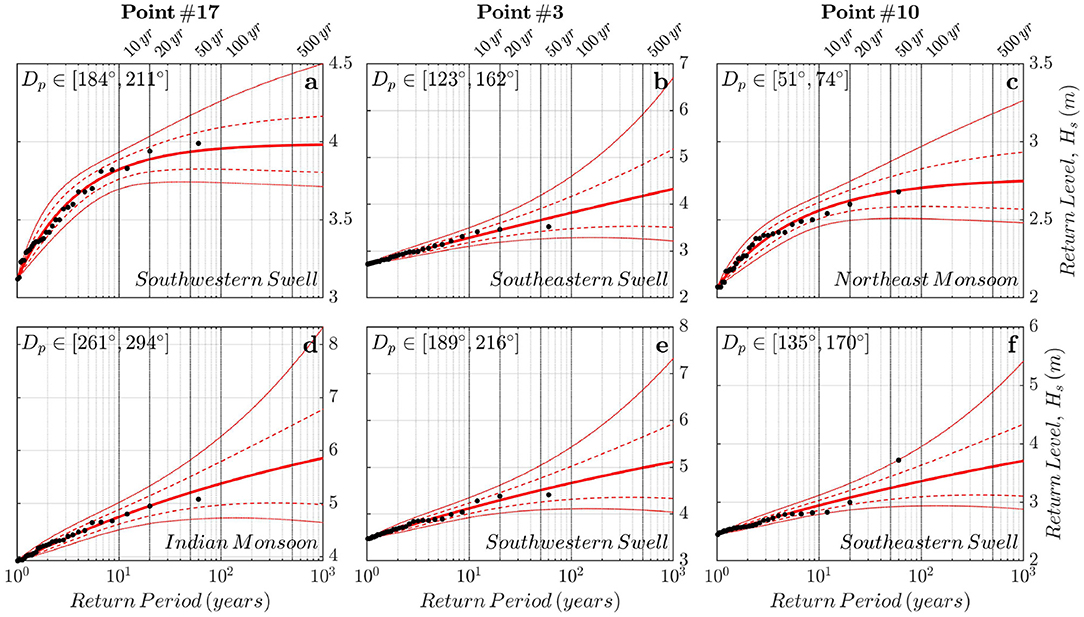
Figure 4. Return levels associated with each return period (thick continuous lines) for the three representative points (point #17 in the first column (a,d), #3 in the second (b,e), and #10 in the third) (c,f) for the wave directions identified at each point (for example, panel a corresponds to the first grey shadow in Figure 3a). The uncertainty bands correspond to ±σ (dashed lines) and the 5−95 % intervals (dotted lines) and have been computed using the delta method. The return period indicated in the top of the panels (10, 20, 50, 100, and 500 years) are the ones selected to perform the regional downscaling with WaveWatch III. Note that the y-axis are different for each panel and do not allow a direct comparison.
3.2. Wave Projections During the Twenty-First Century
The same three grid points analysed above are used as proxies to evaluate the projected changes in waves around the Maldives, using the output of historical simulations and projections during the twenty-first century. Figure 5 represents changes in the frequency of arrival of waves for each direction of propagation by the end of the twenty-first century under RCP8.5 with respect to present-day values for each point. The median of the 8 climate model projections is shown in red (blue) when the projected changes indicate an increase (decrease) in the number of wave events and the grey area represents the model spread. Global models project an increase (~3%) of the southwestern swells, consistent with the findings in Amores and Marcos (2019), who showed a greater activity in swell generation in the region of formation of these waves later in this century. For the waves generated by the Indian monsoon, models show a smaller decrease in the number of waves. Other directions do not show robust projected changes, as the model spread is larger than the median change. The same applies to projected variations in median and extreme Hs in all directions of propagation (Supplementary Figure 8).
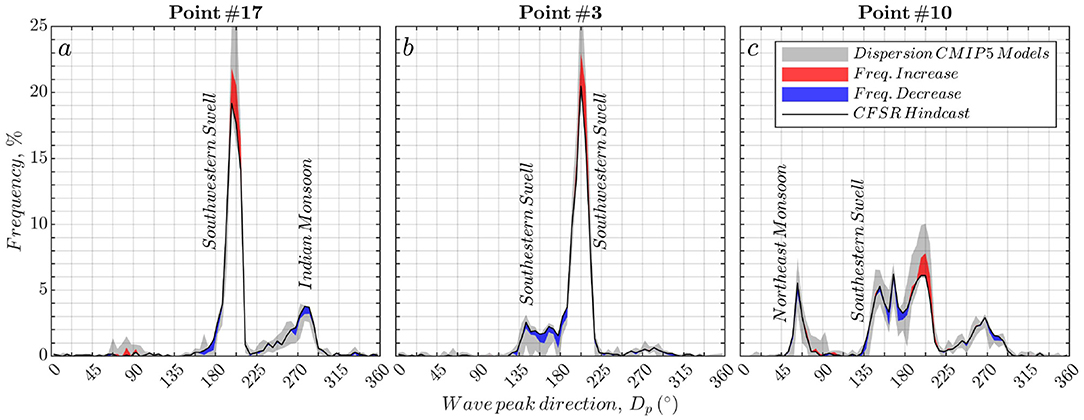
Figure 5. Projected changes in wave direction by the CMIP5 models described in section 2.1 for each one of the representative points selected (a point #17, b point #3, and c point #10). Black line represents the CFSR hindcast histogram (same as the first row in Figure 3); grey shadow indicates the spread of the CMIP5 models (RCP8.5 - Historical); red (blue) shadows show where the models agree to project a frequency increase (decrease) of a given direction.
Overall, projected changes in Hs are smaller than the multi-model spread even under RCP8.5 climate scenario. Variations are expected to be even smaller under RCP4.5. In consequence, present-day significant wave height is considered to be largely representative of future wave climate for the purposes of this work and only CFSR wave fields will be downscaled and propagated toward the shorelines. It is worth noting that changes in the frequency of each wave direction (Figure 5) can be relevant to the transport of sediments and could modify current erosion patterns.
4. Regional Wave Downscaling
Global wave information needs to be downscaled to become representative and usable in the nearshore; however, downscaling the full hourly 30-year CFSR hindcast is computationally too intensive. On the other hand, in terms of coastal impacts assessments and, in particular when coastal flooding is concerned, it is extreme values that are the most relevant metric. Therefore, our approach consists of dynamically downscaling the return levels for Hs calculated for 6 different return periods (namely, 10, 20, 50, 100, 500, and 1000 years) and the four main wave directions that were previously identified. To do so, we have used the Wave-WatchIII model configuration described in section 2.2. The Hs return level associated with a given wave direction is defined at a reference grid point and propagated along the corresponding boundary. In order to insert consistently the Hs at the rest of the grid points in the same boundary, the linear relationships between simultaneous events (±24h), arriving from the same direction and reaching the reference point and the other boundary points were computed. This procedure is illustrated in Supplementary Figures 9–12, where also the reference grid points at each boundary are marked. The linear relationships between the reference grid point and the others are used to scale Hs at each active boundary point. The boundary points where no simultaneous events with the reference point were found or, alternatively, for which there is no correlation (we set the limit value of R2 of the linear adjustment to 0.2), were assigned a linear slope of 0.01 in order to avoid introducing spurious waves. The peak period (Tp) of the incoming waves associated with each return level for Hs, have been determined using a linear relationship between all the (Hs,Tp) events extracted at each reference point for each of the four directions of the incoming waves (Supplementary Figure 13).
The resulting downscaled wave fields consist of a set of four return level curves at every coastal grid point with a spatial resolution of ~500 m. This resolution permits to model wave propagation at the scale of the archipelago. Although it is not accurate enough to perform local assessments inside an atoll, it provides, instead, the necessary boundary condition for the forcing. The full data set is provided at the Zenodo repository under this doi: 10.5281/zenodo.3886273. Figure 6 shows the results for the 100-year return level of the four directions over the entire domain, sorted by decreasing Hs. Note that the spatial patterns of different return levels will be the same for each wave direction and only the magnitude changes. Due to the limited resolution of the GEBCO topobathymetry (~1km), an accurate representation of the islands and the inner part of the atolls is not feasible. Thus, the atolls have been considered as whole entities. This assumption implies that the side of the islands that faces toward the atoll's interior is not solved by our regional downscaling. Nevertheless, it is not relevant at this scale because this side of the islands is not directly impacted by waves. We consider that, given the limited depth on the atoll rims (roughly 1 m), this assumption is reasonable, especially because a more accurate assessment would require tide-current local modelling to capture lagoon/ocean interactions.
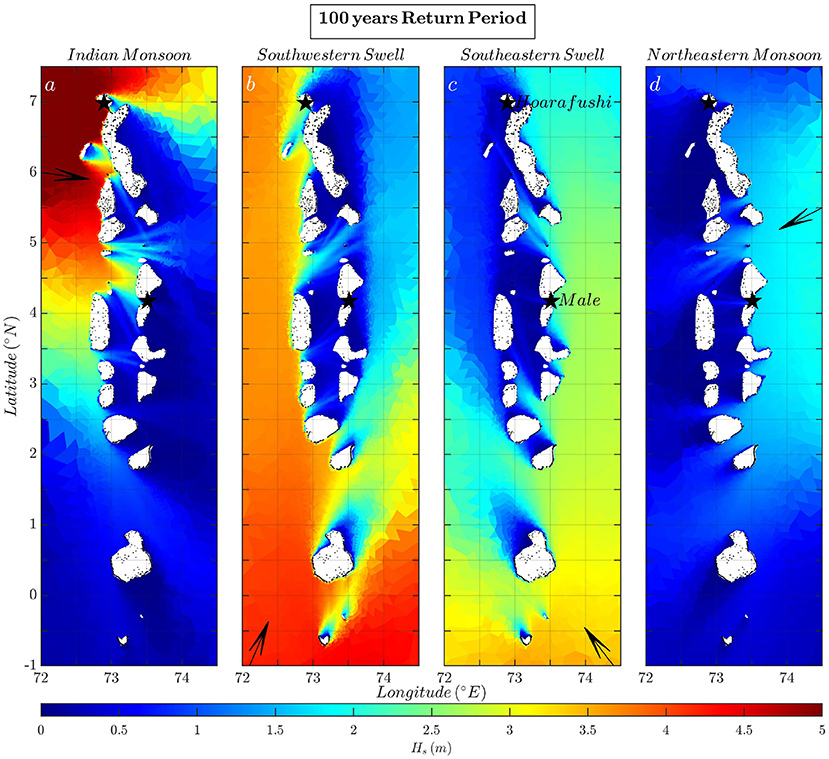
Figure 6. Regional downscaling of the 100 year return period wave event performed with WaveWatch III model (see section 2.2 for the details) for each one of the wave components identified [(a) Indian Monsoon, (b) Southwestern Swell, (c) Southeastern swell, and (d) Northeastern Mosoon]. Black arrow in each panel indicated the wave direction. Black stars indicate the position of Malé, the main city of the Maldives, and Hoarafushi, the island where the local modelling was done.
Figure 6 shows that the largest waves in the Maldives (Hs>5m) are generated by the Indian monsoon (panel a) in the northwestern part of the archipelago, with values of Hs exceeding 2 m in the area northwards from 3°N (note that the same colour scale is used for the four maps). One remarkable feature is that these waves, although attenuated, reach the western side of the Kaafu atoll, the most populated atoll in the Maldives and where the capital city Malé is located. Because of the absence of shadow effects, the western coast of the Kaafu atoll, is the inner region of the Maldives exposed to waves with larger Hs, reaching values between 2.5 and 3.0 m. The southwestern swell (panel b), the second direction with largest Hs after the Indian monsoon, is the component that spreads larger Hs to a broader scale. More precisely, it generates ocean waves with Hs >3 m (even than 3.5 m) to all the western sides of the atolls comprising the Maldives. The third ocean wave direction in terms of Hs is the southeastern swell (panel c), that affects all the eastern side of the Maldives with Hs ranging from 2 to 3.5 m. Finally, the northeastern monsoon (panel d) is the ocean wave component with smaller Hs (<2m). Its effects are concentrated in the central region of the eastern side of the archipelago, from 2 to 6.5°N. It does not strongly affect the northernmost part of the Maldives because this region is located under the shadow of the Indian continent to the monsoon winds (Supplementary Figure 6).
Combining the results of the four wave directions shown in Figure 6, we can identify the wave component with greater Hs at each grid point along the coastlines (Figure 7a), the value of this greater Hs (Figure 7b), as well as how many different directions each coastal point is exposed to Figure 7c; Hs≥1.5 m. In relative numbers, 33% of the coastlines are exposed to the large waves from the Indian monsoon, in 25% of them the highest waves arrive from the southwestern swell, in 28% from southeastern swell, and only 14% from the northeastern monsoon. As in the case of the Kaafu atoll mentioned above, a similar effect is found in the eastern part of the Faafu and Dhaalu atolls, also located in the interior of the Maldives. Here the dominant wave component reaching the eastern coast of these atolls is the southeastern swell that penetrates in the middle of the Maldives between Thaa Atoll and Meemu Atoll.
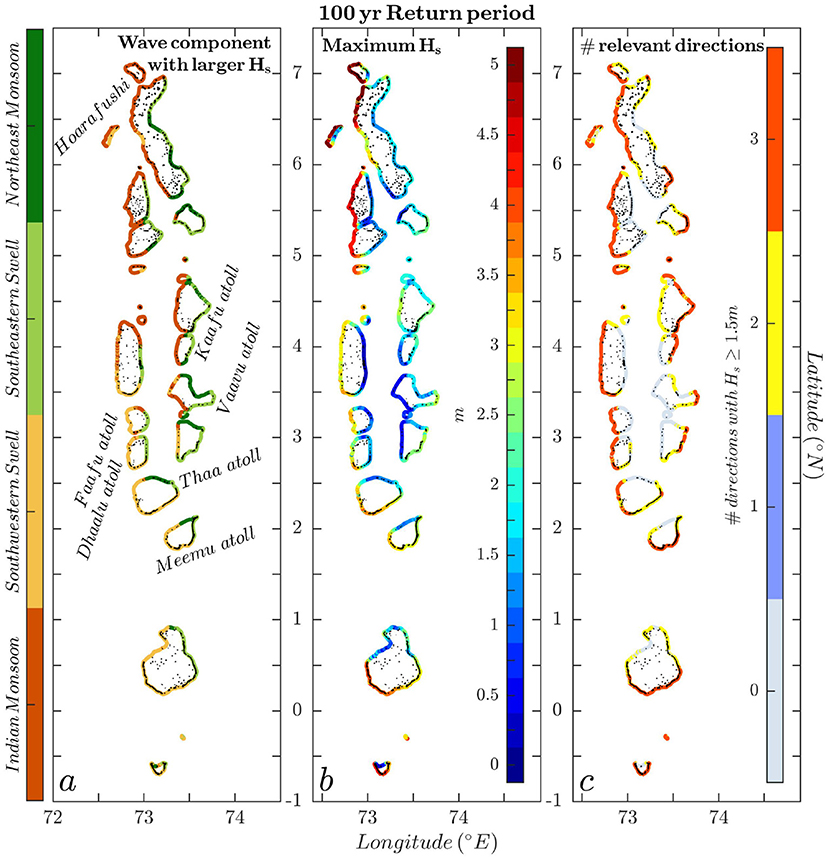
Figure 7. Products derived by combining the regional downscaling for the 100 year return period of the 4 wave components in Figure 6 along the Maldivian coastlines. (a) shows the wave component causing the largest Hs at each coastal point; (b) the maximum Hs at the coast; and (c), the number of wave directions with Hs larger than 1.5 m that hit each coastal location for the 100 year return period.
In terms of maximum Hs (Figure 7b), around 15% of the coastal points, most of them located in the interior of the archipelago, are affected by waves with 100-year return periods smaller than 1 m. The most common values are between 1 and 2 m, affecting 35% of the coastal locations. In 22% of the coasts, the 100-year return levels of Hs vary between 2 and 3 m and in 17% Hs between 3 and 4 m. The largest values, over 4 m, affect around 11% of the coastal points which are found, as expected, in regions where the Indian Monsoon dominates (Figure 7b).
Another metric for the exposure of the coasts to incoming waves is the number of swell directions reaching every coastal point. This is illustrated in Figure 7c, where we have quantified how many wave directions, from the 4 represented in Figure 6, reach each coastal point with Hs≥1.5m for the 100 year return period. The choice of the Hs threshold and the return period selected is arbitrary and used only for illustration purposes; it is not determinant for the resulting map. We conclude that, in 32% of the coastal points, the 100-year return level of Hs is always smaller than 1.5 m (grey points in Figure 7c), with these areas located mainly in the interior of the archipelago. In 29% of the coastal points waves arrive from a single direction (blue points) and in 38% from two directions (yellow points), with the latter case mainly affecting the eastern and western side of the Maldives. In only 1% of the coastal grid points waves arrive from 3 directions (red points), but these are concentrated in the easternmost side of the Vaavu atoll.
5. Local Wave Modelling and Flood Hazard in Hoarafushi Island
The outputs of the regional wave downscaling developed in the previous section are used here in a local flood hazard assessment, illustrating its direct applicability. To do so, downscaled nearshore wave information in a coastal grid point next to Hoarafushi island is propagated toward the shoreline and used to assess coastal flooding under different mean sea-level rise scenarios. The are two reasons that make this location particularly interesting for local wave modelling: first, it is affected by the two largest wave components in the archipelago, i.e., the Indian Monsoon and the southwestern swell; and second, a new island was reclaimed to host a regional airport, which raises questions of present and future climate hazards (see section 2.3). The projected airport, that will have a length of around 1.5 km and a width of 300 m in its wider section, will be located in the reef of the island that faces toward the outer side of the atoll. This means that the shoreline of the airport will be substantially closer to the reef edge than the original island (150–200 m instead of 600 m), reducing the amount of wave energy that can be absorbed by the reef. This local-case study does not pretend to give any recommendation to stakeholders on the airport island height for this specific site. To do so, detailed local information, such as a high-resolution topo-bathymetry or ocean waves in-situ data to validate the model outputs would be required. This example illustrates the applicability of the regional wave downscaling developed here to a local study if precise local information was available.
Wave propagation with SWASH was carried out in the domains in Figure 8. In total, 60 different runs were completed by combining 3 different return periods of Hs (10, 50 and 100 years), two wave directions (Indian Monsoon and the southwestern swell), and 5 different mean sea levels (0, +0.25, +0.50, +0.75, and +1m) for the island configuration with and without airport. We have followed a scenario-independent approach for mean sea-level rise, with 0m corresponding to present-day mean sea level. Mean sea-level changes with respect to the current situation may be interpreted in terms of projected mean sea-level rise (e.g., +0.50m is the median projected mean sea-level rise in 2068 under RCP8.5 and 2088 under RCP2.6, according to Kopp et al., 2014) or as a combination of mean sea-level rise and high tides (e.g., +0.50m is the mean rise in 2041 under RCP8.5 plus +0.25m of tidal amplitude). The mean sea-level changes tested may also include, besides projected mean sea-level rise and tides, other physical processes that can cause mean sea-level variations from seasonal to decadal time scales. We recall here that tides in the Maldives reach a maximum range of around 1m (0.7m median range, Wadey et al., 2017). Note that precise geodetic references relating altitudes and tidal levels are lacking in the Maldives, so these values should be considered as an order of magnitude only. Four examples of selected simulations can be found in Supplementary Videos 1, 2.
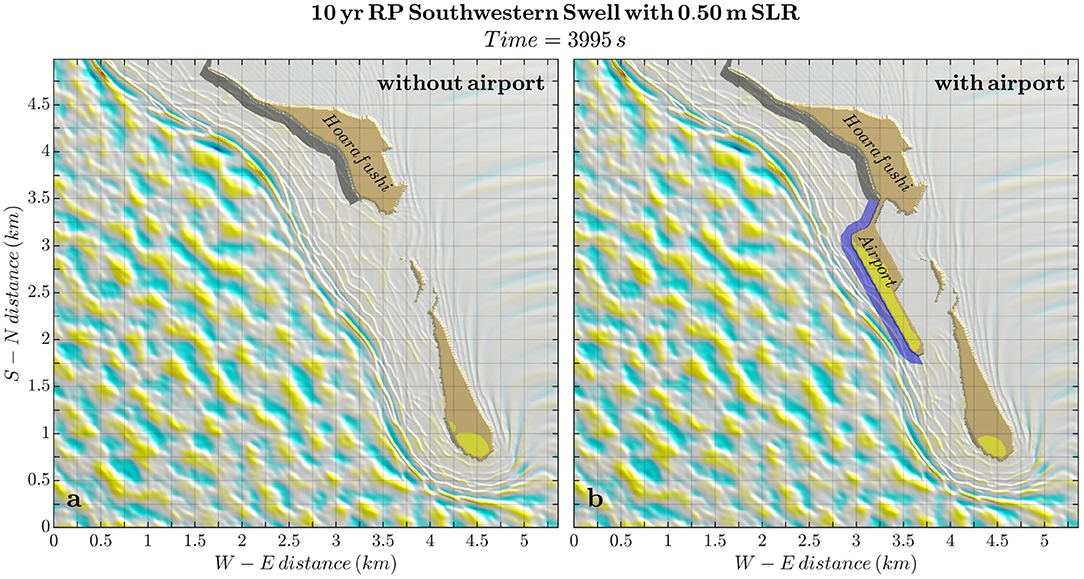
Figure 8. Example of local downscaling simulations with SWASH model (see section 2.3 for details) for the 10 year return period southwestern swell event with 0.5 m of sea level rise (this increase in sea level could mean permanent sea level or high tide with present sea level) for the case without the airport (a) and with the new airport (b). Grey (purple) stripe indicates the coastal region where the box plots from Figure 9 (Supplementary Figure 15) are computed.
It has not been possible to validate the model outputs for the present-day situation due to the lack of observations. We are providing, nevertheless, a qualitative validation by comparing the velocity field obtained with the configuration that includes the airport to a satellite photography in which the airport is under construction (Supplementary Figure 1). There is a consistency between higher current velocities in the model and the imprint of sediment transport from the new-built airport that are likely driven away by the currents.
The outputs of the first set of 30 model runs, that correspond to the spatial configuration without the airport (Figure 8a), are used to evaluate the exposure of the island in terms of the amount of flooding under different forcing conditions. The outputs along a 100-m wide coastal strip covering the western coast of the island (plotted as grey area in Figure 8a) have been gathered together. To do so, the strip is divided in 25-m long sections resulting in 25 × 100 m boxes. Simulated water level time series were extracted for each box and used to compute median and maxima water levels for each model run in each of them. Figure 9 represents the boxplots along the entire coastal strip of these median (left panel) and maxima (right panel) values under all mean sea levels and return levels considered. The horizontal black thick line in both panels marks the height of the island and the two incoming directions are separated by vertical shadowed areas for comparison. Median values of total water level, that correspond to the superposition of the mean sea level and wave setup, do not reach the threshold of land elevation, indicating that there is no overflow at any point along the coastline under all the forcing conditions considered. The results also point at the southwestern swells as the potentially most hazardous waves, as these systematically induce higher water levels than the Indian monsoon waves (shadowed areas against blanked areas). The reason lies in the longer Tp associated with the southwestern waves (~20s) in front of the monsoon waves ( ~12s). As expected, the larger wave setup for a given return period is obtained for the lowest mean sea level of 0m: wave setup reaches almost 0.4 m under present-day mean sea level conditions and reduces to 0.3 m with an increase of 1m. This is because in shallower waters the effects of wave shoaling and breaking leading to wave setup are larger. It is worth noting here that while an increased water level leads to a decreased setup, deeper water allows for larger Hs on the reef flat and an increased run-up potential which could be relevant in terms of impact to infrastructures and erosion. On the other hand, maximum values along the coastal strip have been used to measure whether there has been overtopping generated by the incoming waves. Overtopping occurs whenever these values exceed the island elevation, with their magnitude indicating the severity of the flooding. The boxplots for the maximum values (right panel in Figure 9) point to the occurrence of overtopping under several forcing configurations. For example, 100-year return level waves from southwestern swell and +0.5m mean sea level increase. Note that this may correspond to a 1 in 100-year events reaching the coast during the spring tides and under present-day mean sea level conditions. It also occurs for moderate extreme waves with a return period of 10-years in combination with +1m of mean sea level (this case is also provided in the Supplementary Video 1) and for all the return periods for the southwestern swell with +0.75m of mean sea level rise.
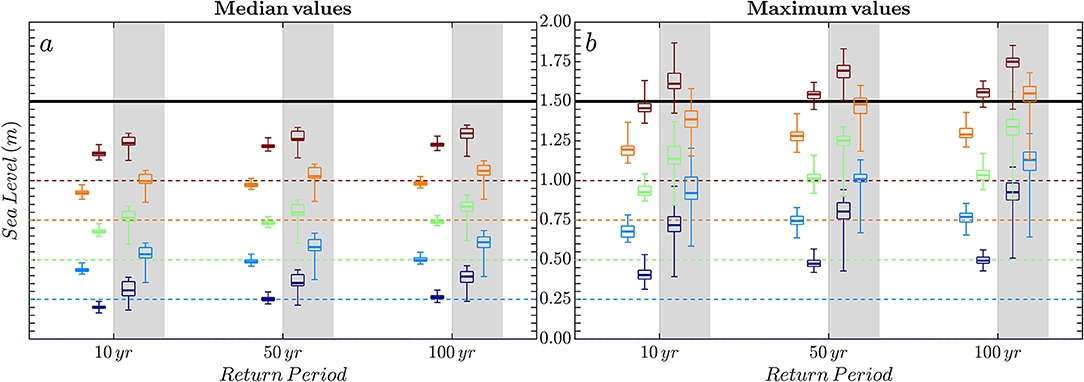
Figure 9. Box plots along Hoarafushi coast without airport computed with time series of simulated water levels: (a) median values; (b) Maximum values. Box plot colours indicate the sea level of the simulation (dark blue 0 m; light blue 0.25 m; green 0.5 m; orange 0.75 m; dark red 1 m) and are referenced from the dashed line with the same colour. Box plots on grey (white) background are for the simulations for the southwestern swell (Indian Monsoon). The thicker line of the box plots shows the median values; the lower (upper) limit of the boxes indicates the 25th (75th) quantile; and the lower and upper whiskers indicate the minimum and maximum values. The horizontal thick black line indicates the island height.
With the construction of the airport connected to Hoarafushi (Figure 8b), the median and maximum water level values computed along the coast (grey area in Figure 8b) slightly increased for all combinations of mean sea level, extreme waves and wave directions (see the equivalent figure to Figure 9 in Supplementary Figure 14). On average, the median values of water level along the coast increase around 0.05 m solely due to the presence of the airport, that partially blocks the channel between Hoarafushi and the island located southwards, leading to higher wave setup. The new reclaimed land is also exposed to incoming waves, and this exposure has been measured in a similar manner as for Hoarafushi, i.e., along a coastal strip on its western coast (blue area in Figure 8b). We remark that the airport has been built 150-200 m away from the reef edge, reducing to a large extent the protection of the wave damping induced by the reef flat. Consequently, both median and maxima water level values are significantly higher than in Hoarafushi island (Supplementary Figure 15). For example, with +1m of increase in mean sea level, even moderate extreme waves would cause overtopping (e.g., 10-year return levels or less under high tide), and under current conditions a 50-year return level southwestern swell would partially flood the airport.
The flood hazard of the new reclaimed land is summarised in Figure 10, using the set of 30 simulation runs with the airport. The flood hazard has been defined following the French standards, that define four different flooding hazard levels (low, moderate, high, and very high) that arise as combinations of inundation level and the water speed over land (see Supplementary Figure 16). The artificial island built for the airport is completely flooded with a high level of hazard for most part of the island for both wave directions and all return periods with an increase in mean sea level of ≥0.75m (with the only exception of the Indian monsoon 10-year return period). It is foreseen that the reclaimed land suffers from partial flooding under a southwestern swell extreme of 50-year return period with current conditions of mean sea level. It is worth mentioning that, given the lack of topographic data for the new airport island, flooding hazard is possibly biased high. We simulated the island as being completely flat and without any coastal defenses. This is unlikely to be the case for a critical infrastructure. However, the actual defense height remains unknown, which is why we assume compliance to land reclamation regulations i.e., 1.5 m land elevation. Coastal defenses would only delay the impact of coastal flooding, but would not avoid it.
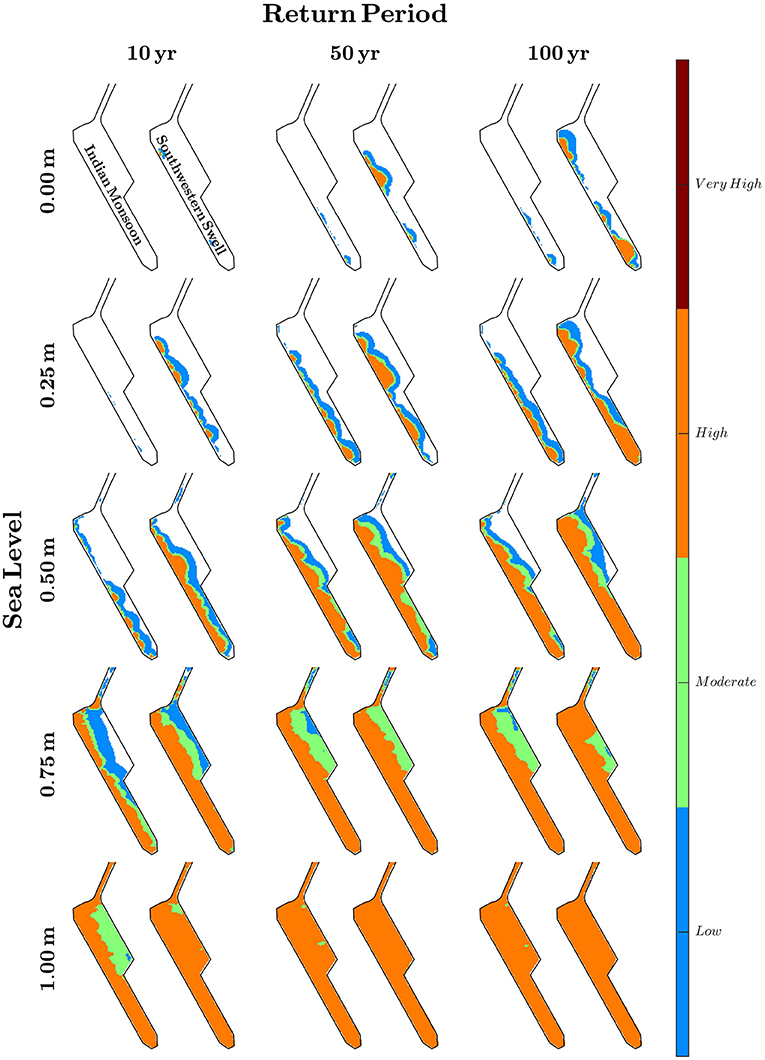
Figure 10. Level of hazard on the new airport for all the SWASH simulations. The colourscale indicates the level of hazard defined by a combination of water height on the airport and water velocity (see Supplementary Figure 16). Different sea levels are represented at each row while the columns indicate return periods of Hs (10, 50, and 100 years) as defined in the text for the regional wave climate. For each combination of sea level and return period, the result for the Indian Monsoon and Southwestern swell are shown at left and right, respectively.
6. Summary and Discussion
6.1. Global to Local Coastal Modelling
Mean sea-level rise, despite having a global origin, has severe local coastal impacts, as it raises the baseline level on top of which extreme events reach the coastlines. Yet, projections of changes in mean sea-level as well as assessments of marine extremes are often provided on a large-scale basis (e.g., Vousdoukas et al., 2017), while understanding the causes of coastal flooding and anticipating the impacts require quantitative information at the local scale. This can be feasible to implement in regions where monitoring networks, forecasting and operational systems and development programs for sustainable coastlines are well established and mature (for example, the Flood and Coastal Erosion Risk Management Programme in the UK, or the Delta Programme in the Netherlands). In many cases, however, even local assessments rely on coarse resolution, large-scale global climate information.
In this work we have focused on the Maldivian archipelago, a region where recurrent flooding episodes occur driven by remotely generated waves. These events are, furthermore, projected to become more frequent as mean sea level rises due to the low elevation of the islands. Despite their exposure to waves, to our knowledge, the only source of wave climate information in the region so far are the outputs of global wave reanalysis with a spatial resolution of the order of a degree. Our work illustrates how these global wave fields from coarse resolution climate models can be translated into usable information for regional and local studies and how it can be combined with regionalised projections of mean sea-level rise and local topo-bathymetries.
The first step consisted of a detailed analysis and characterisation of the global wave climate around the Maldives using the closest grid points from the CFSR wave reanalysis (section 2.1). This is a prior mandatory step before the design of the regionalisation. We identified four dominant incoming wave directions from remotely generated waves: the two most common, that originate in the Southern Ocean (Amores and Marcos, 2019), and swells generated by the Indian and Northwestern monsoons. In a second step, for each direction, extreme waves have been characterised in terms of Hs and Tp and a set of five return levels have been dynamically-downscaled using the spectral model WWIII (section 2.2). We have focused on extreme waves only because these are the most relevant for risk analyses; furthermore, the alternative of dynamically-downscaling a 35-year long reanalysis is unfeasible due to computational constraints (this worsens if historical runs and projections are considered). The regionalisation has resulted in a major product of the present work: a valuable data set of extreme waves along the Maldivian coasts with spatial resolutions down to 500 m in the points nearest to the coast. The data set is published at doi: 10.5281/zenodo.3886273. The output of our regionalisation provides quantitative information on extreme waves, in the form of return level curves, at the regional scale in the Maldives and for the first time. This dataset is useful for coastal engineering studies, for feeding local coastal models of flooding hazards and for planning land reclamation and other regional developments. It also serves to compute the inundation potential at every location and for every incoming swell direction, that depends on wave energy, , in line with the “response approach” discussed in Sanuy et al. (2020). Overall, it is expected to become a compelling source of scientific information that can be embedded in coastal climate services (Le Cozannet et al., 2017; Kopp et al., 2019). The users should, nevertheless, ensure that the inherent uncertainties in the method and data are considered. This means that regional waves are representative of ocean swells in the vicinity of the atolls and that, for practical purposes, a detailed topobathymetry is needed is these regional outputs are to be used as boundary forcings. Also, the four main swell directions arriving to the archipelago are considered separately, since the generation mechanisms are independent; thus, every coastal location may be exposed to a different number of incoming wave directions, and all of them should be explored in a local case study, as illustrated in section 5 above.
There is a number of limitations in our regionalised wave fields. The bathymetry used in the regional wave model (GEBCO, see section 2.2) has a spatial resolution of ~ 1 km, which is not enough to resolve the features inside the atolls. We have therefore included every atoll as a single entity in the model domain, neglecting the wave propagation in the inner region and the exchanges between the lagoon of the atoll and the ocean. We consider, nevertheless, that this assumption is reasonable because our results provide evidence that shadow effects of the atolls to incoming waves are realistically simulated from all directions. That implies that we account for the waves that reach the external coast of the atolls everywhere in the Maldives. This limitation can be overcome in areas where mesoscale (~ 100 m resolution) bathymetric data sets exist, in which case the interactions with the inner lagoon can also be accounted for. Another caveat of the regional product is that only selected return periods of Hs are provided, instead of an entire high-frequency time series at every coastal grid point. While the quantification of return levels is central to risk assessments, no information on averaged wave fields (useful for erosion studies, for example) is provided. Finally, it is worth pointing out that the regional product has not been validated against observations due to the lack of data.
6.2. Application for Coastal Flood Hazard
We have conducted a local flood hazard modelling experiment that demonstrates the applicability of the regionalised wave fields. Our case study is in the North of the archipelago, exposed to the largest incoming waves, and includes a land reclamation project. We have used the regionalised wave information to feed the wave propagation model SWASH around Hoarafushi island, where local bathymetry has been measured. We have estimated the flooding hazard under present-day conditions and also under projected future scenarios. Our analysis of the global wave climate revealed that projected changes in the large-scale wave characteristics during the twenty-first century are small in comparison to the multi-model spread even under the RCP8.5 scenario. Therefore, we rely on the downscaled regional wave reanalysis and assume that future changes in marine hazards will be driven only by mean sea-level rise. The local model does take into account the modification of the wave propagation due to higher mean sea levels, though. The set of the model experiments included the island configuration with and without the airport in order to determine how the presence of the new reclaimed land alters the flood hazard, the wave propagation and the associated currents.
Our results identified the southwestern swells as the potentially most hazardous waves in Hoarafushi, with 100-year return levels of Hs up to 4 m and associated Tp of ~20 s. This is, in addition, the most common wave direction that reaches this part of the archipelago, although not the one with largest Hs (that are associated with the Indian monsoon). Our findings indicate that a moderate incoming southwestern swell corresponding to a return period of only 10 years will cause overtopping in Hoarafushi island if it reaches the shoreline under a mean sea level 0.75 m higher than its present-day value (Figure 9). The presence of the reclaimed land slightly increases these impacts (Supplementary Figure 14). The flood hazard is much stronger in the reclaimed land, that will experience overtopping episodes with sea levels only 0.25 m above present-day mean value (Supplementary Figure 15). The reason is its location close to the reef that reduces the wave damping over the reef flat. We recall here that we have adopted a scenario-independent approach for mean sea level increases; this may be justified given that the range of mean sea level changes that we are considering (below 1 m) will be reached even under strong mitigation, as the maximum value lies within the committed global mean sea-level rise of past GHGs emissions (Nauels et al., 2019). Thus, it is not about whether these higher mean sea levels will be reached, but when it will occur. Impact studies based on scenario-independent approaches in combination with ongoing monitoring of regional mean sea-level rise can facilitate the design of adaptive solutions to climate-induced hazards.
In addition, this approach also allows to evaluate the wave-induced flood hazard under particular tidal conditions. In the example above, mean sea level 0.75 m higher than present-day values can be interpreted as a combination of climate-induced mean sea-level rise and tidal oscillations. For instance, 0.75 m can be reached with 0.5 m of climate-induced mean sea level that, according to Kopp et al. (2014), corresponds to the median projected value in 2068 under the RCP8.5 scenario, plus 0.25 m of tidal amplitude. In consequence, according to our estimates, the recently developed (in 2019) regional airport will be flooded under present-day mean sea-level conditions and 0.25 m of tidal amplitude if a moderate extreme swell event (10-year return period) reaches the area, that is, within the present decade. Note that we are not computing the likelihood of co-occurrence of extreme swells and high tides. The reasons for that are, firstly, that these two processes are uncorrelated (astronomical tides and remotely-generated swell events have independent driving mechanisms) which means that their joint probability could be computed as the product of their marginal probability distributions (Pugh and Woodworth, 2014). However, this would require a complete set of time series of the two processes at every grid point. Although there are methods to generate a set of full synthetic time series from their statistical characterisation (e.g., Solari and Losada, 2011), this is a different type of product that is beyond the scope of the present work. Secondly, our approach is more flexible since it does not constrain the interpretation of the increments in mean sea level (either climate-induced sea level rise or tides or both), hence, allowing final users to tailor our approach to their needs, based on their respective risk-taking propensity.
The modification of the island configuration with the presence of the reclaimed land significantly modifies the patterns of the currents (Supplementary Figure 1). Such changes are determinant for coastal erosion, as they control the sediment transport along the coastlines. Coastal erosion is considered a central problem in the Maldives, especially in densely populated islands (Zahir et al., 2016; Duvat and Magnan, 2019). Erosion can be prevented or enhanced by many factors, including land reclamation, dredging and building coastal defenses. Here we demonstrate that our regional wave fields are a valuable tool also for anticipating possible erosion and changing spatial patterns in particular case studies.
The major limitation of our local coastal modelling exercise is the lack of a detailed topography of Hoarafushi and its nearby reclaimed airport. While we have measured bathymetric profiles during a field trip, the information on the topography is limited to the averaged elevation of the island. Likewise, the elevation of the reclaimed land (which was not yet built when the field trip took place) has been defined according to the national regulations. In consequence, we have not included coastal defenses and we have instead considered that both the island and the new reclaimed land are flat. This implies that our estimates of overtopping and flooding could be biased high; however, the presence of coastal defenses would not completely avoid the flood hazard, they would simple delay the impacts of mean sea-level rise.
Another point worthy of discussion is the assumption of static bathymetry and null reef response to changing climatic conditions. It is clear that reefs can change over time. For example, they can accrete following sea level rise (Woodroffe and Murray-Wallace, 2012), they can degrade due to human activities (the construction of the airport is a good example) or they can die as a consequence of warmer temperatures (Bruno and Selig, 2007) (indeed, warm reefs are projected to significantly decline even with global warming only 1.5°C above pre-industrial levels (Bindoff et al., 2019) and to be virtually extinct with 2°C of warming (Hoegh-Guldberg et al., 2018). In any of these cases, changes in the reef would imply changes in the wave propagation and level of protection of the island (Sheppard et al., 2005). We disregard these potential changes in our local flood hazard modelling experiment because we analyse an artificially reclaimed island. Here, human activities generally have severe negative effects on the reef (Duvat, 2020) and the island is protected with hard measures. This is also to urban atoll islands, as Hoarafushi, that are continuously adapting to increased hazard potential by building coastal infrastructures or artificially raising the land (Duvat and Magnan, 2019; Esteban et al., 2019; Hinkel et al., 2019; Brown et al., 2020). This reduces the ability of the island to naturally increase its elevation by sediment deposition during overtopping events (Kench and Beetham, 2019). Hence, we argue that in this case human interventions are probably more important for wave propagation than changes in the reef (Duvat and Magnan, 2019). Contrastingly, in a natural island, assuming a static bathymetry and null reef response, would bias the results of model overtopping (Beetham et al., 2017; Beetham and Kench, 2018). Nevertheless, the regional downscaling that we provide serves as a boundary condition for subsequent studies of wave-induced flooding under future conditions, which then have to account for these uncertainties of future reef responses.
7. Concluding Remarks
Our study provides the framework to fill the gap between global information of marine climate drivers, including mean sea level and extremes, and local coastal flood hazard modelling. In particular, we demonstrate the feasibility of using large-scale data sets (regionalised sea-level projections and global wind-waves simulations) to inform regional planning and local decision-making. Our work focused in the Maldives, but our technique can be applied to any coastal region, being most relevant where regional and local climate information is not available. Together with the outputs, we have discussed a number of uncertainties in regional as well as local coastal modelling that are inherent to the methodology. Some of the limitations, though, stem, to a large extent, from the lack of coastal observations (i.e., local topo-bathymetries). Our study thus advocates for improved monitoring systems and data collection to reduce uncertainties and better inform final users.
We have generated a valuable regional wave data set that fulfils the purposes of characterisation of the wave climate in a sparsely observed area. This dataset, in combination with detailed local information (e.g., high-resolution topo-bathymetries), serves as a milestone for informing adaptation policy and Maldivian decision-makers facing the challenge of adapting to rising sea-levels.
Data Availability Statement
The datasets presented in this study can be found in online repositories. The names of the repository/repositories and accession number(s) can be found below: https://zenodo.org/record/3886273#.YCFTAOhKhPY.
Author Contributions
AA, MM, GL, and JH conceived the work. AA, MM, RP, and SL designed the numerical experiments. AA, MM, and JR analysed the return periods. AS and ZK retrieved the topobathimetric data. All authors contributed to the outline and writing of the manuscript.
Funding
This study was supported by the FEDER/Ministerio de Ciencia, Innovación y Universidades Agencia Estatal de Investigación through the MOCCA project (grant no. RTI2018-093941-B-C31) and by the INSeaPTION Project that is part of ERA4CS, an ERA-NET initiated by JPI Climate, and funded by Ministerio de Economía, Industria y Competitividad Agencia Estatal de Investigación (ES) (grant no. PCIN-2017-038), BMBF (DE), NOW (NL), and ANR (FR) with co-funding by the European Union (Grant 690462). This research has been also supported by the ANR project Storisk supported by the French Research Agency. AA was funded by the Conselleria d'Educació, Universitat i Recerca del Govern Balear through the Direcció General de Política Universitària i Recerca and by the Fondo Social Europeo for the period 2014–2020 (grant no. PD/011/2019). MM was supported by the Ministerio de Ciencia e Innovación and la Agencia Estatal de Investigación through grant no. IED2019-000985-I.
Conflict of Interest
The authors declare that the research was conducted in the absence of any commercial or financial relationships that could be construed as a potential conflict of interest.
Acknowledgments
We are grateful to Dr. Aurélie Maspataud for her support with bathymetric data and Dr. Fernando Méndez for advice on numerical wave modelling. We thank the Ministry of Environment, the Environmental Protection Agency, the Maldives Transport and Contracting Company and Water Solutions for their support during the field trip and data collection. AA is grateful to M. A. Blázquez for his support and comments during the preparation of the manuscript.
Supplementary Material
The Supplementary Material for this article can be found online at: https://www.frontiersin.org/articles/10.3389/fmars.2021.665672/full#supplementary-material
References
Amores, A., and Marcos, M. (2019). Ocean swells along the global coastlines and their climate projections for the 21st century. J. Clim. 33, 185–199. doi: 10.1175/JCLI-D-19-0216.1
Beetham, E., and Kench, P. S. (2018). Predicting wave overtopping thresholds on coral reef-island shorelines with future sea-level rise. Nat. Commun. 9:3997. doi: 10.1038/s41467-018-06550-1
Beetham, E., Kench, P. S., and Popinet, S. (2017). Future reef growth can mitigate physical impacts of sea-level rise on atoll islands. Earths Fut. 5, 1002–1014. doi: 10.1002/2017EF000589
Bindoff, N. L., Cheung, W. W., Kairo, J. G., Arístegui, J., Guinder, V. A., Hallberg, R., et al. (2019). “Changing ocean, marine ecosystems, and dependent communities,” in IPCC Special Report on the Ocean and Cryosphere in a Changing Climate, eds H. -O. Pörtner, D. C. Roberts, V. Masson-Delmotte, P. Zhai, M. Tignor, E. Poloczanska, K. Mintenbeck, A. Alegría, M. Nicolai, A. Okem, J. Petzold, B. Rama, N. M. Weyer, 477–587, in press.
Brown, S., Wadey, M. P., Nicholls, R. J., Shareef, A., Khaleel, Z., Hinkel, J., et al. (2020). Land raising as a solution to sea-level rise: an analysis of coastal flooding on an artificial island in the maldives. J. Flood Risk Manag. 13:e12567. doi: 10.1111/jfr3.12567
Bruno, J. F., and Selig, E. R. (2007). Regional decline of coral cover in the indo-pacific: timing, extent, and subregional comparisons. PLOS ONE 2:e711. doi: 10.1371/journal.pone.0000711
Cialone, M. A., and Smith, J. M. (2007). “Wave transformation modeling with bottom friction applied to southeast oahu reefs,” in 10th International Workshop on Wave Hindcasting and Forecasting & Coastal Hazard Assessment, (Citeseer), 1–12.
Duvat, V. K. (2020). Human-driven atoll island expansion in the maldives. Anthropocene 32:100265. doi: 10.1016/j.ancene.2020.100265
Duvat, V. K. E., and Magnan, A. K. (2019). Rapid human-driven undermining of atoll island capacity to adjust to ocean climate-related pressures. Sci. Rep. 9:15129. doi: 10.1038/s41598-019-54659-0
Esteban, M., Jamero, M. L., Nurse, L., Yamamoto, L., Takagi, H., Thao, N. D., et al. (2019). Adaptation to sea level rise on low coral islands: lessons from recent events. Ocean Coas. Manag. 168, 35–40. doi: 10.1016/j.ocecoaman.2018.10.031
Gussmann, G., and Hinkel, J. (2021). A framework for assessing the potential effectiveness of adaptation policies: coastal risks and sea-level rise in the maldives. Environ. Sci. Policy 115, 35–42. doi: 10.1016/j.envsci.2020.09.028
Harangozo, S. A. (2013). Flooding in the Maldives and Its Implications for The Global Sea Level Rise Debate (First Published: 01 January 1992), (American Geophysical Union (AGU)), 95–99.
Hemer, M., Trenham, C., Durrant, T., and Greenslade, D. (2015). Cawcr global wind-wave 21st century climate projections (v2). CSIRO Service Collect. doi: 10.4225/08/55C991CC3F0E8
Hemer, M. A., Katzfey, J., and Trenham, C. E. (2013). Global dynamical projections of surface ocean wave climate for a future high greenhouse gas emission scenario. Ocean Model. 70, 221–245. doi: 10.1016/j.ocemod.2012.09.008
Hemer, M. A., and Trenham, C. E. (2016). Evaluation of a cmip5 derived dynamical global wind wave climate model ensemble. Ocean Model. 103, 190–203. doi: 10.1016/j.ocemod.2015.10.009
Hinkel, J., Church, J. A., Gregory, J. M., Lambert, E., Le Cozannet, G., Lowe, J., et al. (2019). Meeting user needs for sea level rise information: a decision analysis perspective. Earths Fut. 7, 320–337. doi: 10.1029/2018EF001071
Hinkel, J., Lincke, D., Vafeidis, A. T., Perrette, M., Nicholls, R. J., Tol, R. S. J., et al. (2014). Coastal flood damage and adaptation costs under 21st century sea-level rise. Proc. Natl. Acad. Sci. U.S.A. 111, 3292–3297. doi: 10.1073/pnas.1222469111
Hoegh-Guldberg, O., Jacob, D., Bindi, M., Brown, S., Camilloni, I., Diedhiou, A., et al. (2018). Impacts of 1.5°c Global Warming on Natural and Human Systems. Global warming of 1.5°C. An IPCC Special Report.
Hoeke, R. K., McInnes, K. L., Kruger, J. C., McNaught, R. J., Hunter, J. R., and Smithers, S. G. (2013). Widespread inundation of pacific islands triggered by distant-source wind-waves. Glob. Planet. Change 108, 128–138. doi: 10.1016/j.gloplacha.2013.06.006
Kench, P., and Beetham, E. P. (2019). Evidence of vertical building of reef islands through overwash and implications for island futures. Coastal Sediments 916–929. doi: 10.1142/9789811204487_0080
Kopp, R. E., Gilmore, E. A., Little, C. M., Lorenzo-Trueba, J., Ramenzoni, V. C., and Sweet, W. V. (2019). Usable science for managing the risks of sea-level rise. Earths Fut. 7, 1235–1269. doi: 10.1029/2018EF001145
Kopp, R. E., Horton, R. M., Little, C. M., Mitrovica, J. X., Oppenheimer, M., Rasmussen, D. J., et al. (2014). Probabilistic 21st and 22nd century sea-level projections at a global network of tide-gauge sites. Earths Fut. 2, 383–406. doi: 10.1002/2014EF000239
Kraines, S., Yanagi, T., Isobe, M., and Komiyama, H. (1998). Wind-wave driven circulation on the coral reef at bora bay, miyako island. Coral Reefs 17, 133–143. doi: 10.1007/s003380050107
Le Cozannet, G., Nicholls, R., Hinkel, J., Sweet, W., McInnes, K., Van de Wal, R., et al. (2017). Sea level change and coastal climate services: The way forward. J. Mar. Sci. Eng. 5, 49. doi: 10.3390/jmse5040049
Lecacheux, S., Pedreros, R., Le Cozannet, G., Thiébot, J., De La Torre, Y., and Bulteau, T. (2012). A method to characterize the different extreme waves for islands exposed to various wave regimes: a case study devoted to reunion island. Natural Hazards Earth Syst. Sci. 12, 2425–2437. doi: 10.5194/nhess-12-2425-2012
Maniku, H. A. (1990). Changes in the Topography of the Maldives. Forum of Writers on Environment (Maldives).
Marcos, M., and Woodworth, P. L. (2017). Spatiotemporal changes in extreme sea levels along the coasts of the north atlantic and the gulf of mexico. J. Geophys. Res. 122, 7031–7048. doi: 10.1002/2017JC013065
MEE. (2017). State of the Environment 2016. Ministry of Environment and Energy. Available online at: https://www.environment.gov.mv/v2/wp-content/files/publications/20170202-pub-soe-2016.pdf
Merkens, J.-L., Lincke, D., Hinkel, J., Brown, S., and Vafeidis, A. T. (2018). Regionalisation of population growth projections in coastal exposure analysis. Clim. Change 151, 413–426. doi: 10.1007/s10584-018-2334-8
Morim, J. L. M., Wang, H. X., Cartwright, N., Trenham, C., Semedo, A., Young, I., et al. (2019). Robustness and uncertainties in global multivariate wind-wave climate projections. Nat. Clim. Change 9, 711–718. doi: 10.1038/s41558-019-0542-5
Nauels, A., Gütschow, J., Mengel, M., Meinshausen, M., Clark, P. U., and Schleussner, C.-F. (2019). Attributing long-term sea-level rise to paris agreement emission pledges. Proc. Natl. Acad. Sci. U.S.A. 116, 23487–23492. doi: 10.1073/pnas.1907461116
NBS (2017). “Statistical yearbook of maldives 2016,” in National Bureau of Statistics Ministry of Finance and Treasury, Republic of Maldives (Malé).
NBS (2019). “Maldives population projections 2014-2054, assumption and results analysis,” in National Bureau of Statistics Ministry of Finance and Treasury, Republic of Maldives (Malé)
Nicholls, R. J., Hanson, S. E., Lowe, J. A., Warrick, R. A., Lu, X., and Long, A. J. (2014). Sea-level scenarios for evaluating coastal impacts. WIRES Clim. Change 5, 129–150. doi: 10.1002/wcc.253
Nurse, L., McLean, R., Agard, J., Bibruglio, L., Duvat, V., Pelesikoti, N., et al. (2014). “Small islands (chapter 29),” in Climate Change 2014 : Impacts, Adaptation, and Vulnerability. Part B : Regional Aspects. Contribution of Working Group II to the Fifth Assessment Report of the Intergovernmental Panel on Climate Change, eds V. R. Barros, C. B. Field, D. J. Dokken, M. D. Mastrandrea, K. J. Mach, T. E. Bilir, M. Chatterjee, K. L. Ebi, Y. O. Estrada, R. C. Genova, B. Girma, E. S. Kissel, A. N. Levy, S. MacCracken, P. R. Mastrandrea, and L. L.White (Cambridge, UK; New York, NY: Cambridge University Press), 1613–1654. Available online at: https://www.ipcc.ch/site/assets/uploads/2018/02/WGIIAR5-Chap29_FINAL.pdf
Oppenheimer, M., Glavovic, B., Hinkel, J., van de Wal, R., Magnan, A., Abd-Elgawad, A., et al. (2019). “Sea level rise and implications for low-lying islands, coasts and communities,” in IPCC Special Report on the Ocean and Cryosphere in a Changing Climate, eds H.-O. Pörtner, D. C. Roberts, V. Masson-Delmotte, P. Zhai, M. Tignor, E. Poloczanska, K. Mintenbeck, A. Alegría, M. Nicolai, A. Okem, J. Petzold, B. Rama, N. M. Weyer. Available online at: https://www.ipcc.ch/srocc/chapter/chapter-4-sea-level-rise-and-implications-for-low-lying-islands-coasts-and-communities/
Prager, E. J. (1991). Numerical simulation of circulation in a caribbean-type backreef lagoon. Coral Reefs 10, 177–182. doi: 10.1007/BF00336771
Pugh, D., and Woodworth, P. (2014). Sea-Level Science: Understanding Tides, Surges, Tsunamis and Mean Sea-Level Changes. Cambridge: Cambridge University Press. doi: 10.1017/CBO9781139235778. Available online at: https://www.cambridge.org/core/books/sealevel-science/C5E551D95DA4E8AF116FED9F0DEB289B
Rijnsdorp, D. P., Smit, P. B., and Zijlema, M. (2012). Non-hydrostatic modelling of infragravity waves using swash. Coast. Eng. Proc. 1:currents.27. doi: 10.9753/icce.v33.currents.27
Saha, S., Moorthi, S., Pan, H.-L., Wu, X., Wang, J., Nadiga, S., et al. (2010). The ncep climate forecast system reanalysis. Bull. Am. Meteorol. Soc. 91, 1015–1058. doi: 10.1175/2010BAMS3001.1
Sanuy, M., Jiménez, J. A., Ortego, M. I., and Toimil, A. (2020). Differences in assigning probabilities to coastal inundation hazard estimators: Event versus response approaches. J. Flood Risk Manag. 13:e12557. doi: 10.1111/jfr3.12557
Sheppard, C., Dixon, D. J., Gourlay, M., Sheppard, A., and Payet, R. (2005). Coral mortality increases wave energy reaching shores protected by reef flats: examples from the seychelles. Estuarine Coast. Shelf Sci. 64, 223–234. doi: 10.1016/j.ecss.2005.02.016
Solari, S., and Losada, M. A. (2011). Non-stationary wave height climate modeling and simulation. J. Geophys. Res. 116:C09032. doi: 10.1029/2011JC007101
Suzuki, T., Altomare, C., De Roo, S., Vanneste, D., and Mostaert, F. (2018). Manning's Roughness Coefficient in Swash: Application to Overtopping Calculation. FHR Reports. Available online at: https://publicaties.vlaanderen.be/view-file/32905.
Tolman, H. (2009). User manual and system documentation of wavewatch iii version 3.14. NOAA/NWS/NCEP/MMAB Technical Note 276, (Appendices), 194.
Tolman, H. (2014). User manual and system documentation of wavewatch iii version 4.18. NOAA/NWS/NCEP/MMAB Technical Note 316.
Vousdoukas, M. I., Mentaschi, L., Voukouvalas, E., Verlaan, M., and Feyen, L. (2017). Extreme sea levels on the rise along europe's coasts. Earths Fut. 5, 304–323. doi: 10.1002/2016EF000505
Wadey, M., Brown, S., Nicholls, R. J., and Haigh, I. (2017). Coastal flooding in the maldives: an assessment of historic events and their implications. Natural Hazards 89, 131–159. doi: 10.1007/s11069-017-2957-5
Woodroffe, C. D., and Murray-Wallace, C. V. (2012). Sea-level rise and coastal change: the past as a guide to the future. Quatern. Sci. Rev. 54, 4–11. doi: 10.1016/j.quascirev.2012.05.009
Woodworth, P. L. (2005). Have there been large recent sea level changes in the maldive islands? Glob. Planetary Change 49, 1–18. doi: 10.1016/j.gloplacha.2005.04.001
Woodworth, P. L., Melet, A., Marcos, M., Ray, R. D., Wöppelmann, G., Sasaki, Y. N., et al. (2019). Forcing factors affecting sea level changes at the coast. Surveys Geophys. 40, 1351–1397. doi: 10.1007/s10712-019-09531-1
Zahir, H., Asis, M., Rasheed, A., Musthafa, Z. M., Latheef, A. T., and Mohamed, I. (2016). “Second national communication of maldives to the united nations framework convention on climate change,” in Ministry of Environment and Energy. Republic of Maldives: Malé.
Zijlema, M. (2012). “Modelling wave transformation across a fringing reef using swash,” in ICCE 2012: Proceedings of the 33rd International Conference on Coastal Engineering, Santander, Spain, 1-6 July 2012. Coastal Engineering Research Council. doi: 10.9753/icce.v33.currents.26. Available online at: https://journals.tdl.org/icce/index.php/icce/article/view/6479
Keywords: coastal flooding, wind-waves, sea-level rise, global-to-local modelling, climate services
Citation: Amores A, Marcos M, Pedreros R, Le Cozannet G, Lecacheux S, Rohmer J, Hinkel J, Gussmann G, van der Pol T, Shareef A and Khaleel Z (2021) Coastal Flooding in the Maldives Induced by Mean Sea-Level Rise and Wind-Waves: From Global to Local Coastal Modelling. Front. Mar. Sci. 8:665672. doi: 10.3389/fmars.2021.665672
Received: 08 February 2021; Accepted: 19 May 2021;
Published: 21 June 2021.
Edited by:
Juan Jose Munoz-Perez, University of Cádiz, SpainReviewed by:
Dean Bryan Gesch, United States Geological Survey (USGS), United StatesPhilip Thompson, University of Hawaii, United States
Copyright © 2021 Amores, Marcos, Pedreros, Le Cozannet, Lecacheux, Rohmer, Hinkel, Gussmann, van der Pol, Shareef and Khaleel. This is an open-access article distributed under the terms of the Creative Commons Attribution License (CC BY). The use, distribution or reproduction in other forums is permitted, provided the original author(s) and the copyright owner(s) are credited and that the original publication in this journal is cited, in accordance with accepted academic practice. No use, distribution or reproduction is permitted which does not comply with these terms.
*Correspondence: Angel Amores, YW5nZWwuYW1vcmVzQHVpYi5lcw==