- 1Graduate School of Agriculture, University of Miyazaki, Miyazaki, Japan
- 2Shikoku Marine Life Laboratory, Otsuki, Japan
- 3Geological Survey of Japan, National Institute of Advanced Industrial Science and Technology (AIST), Tsukuba, Japan
- 4Department of Life Science and Technology, School of Life Sciences and Technology, Tokyo Institute of Technology, Ookayama, Japan
- 5Interdisciplinary Graduate School of Agriculture and Engineering, University of Miyazaki, Miyazaki, Japan
- 6Faculty of Geo-Environmental Science, Rissho University, Kumagaya, Japan
- 7Laboratories of Biochemistry, Faculty of Science, Kochi University, Kochi, Japan
- 8Okinawa Churashima Research Center, Okinawa Churashima Foundation, Motobu, Japan
- 9Parasitology, Faculty of Medicine, University of Miyazaki, Miyazaki, Japan
- 10Department of Marine Biology and Environmental Sciences, Faculty of Agriculture, University of Miyazaki, Miyazaki, Japan
Estimating the spatial extent of gamete and larval dispersal of deep-sea coral species, is challenging yet important for their conservation. Spatial autocorrelation analysis is useful for estimating the spatial range of dispersal of corals; however, it has not been performed for deep-sea coral species using genome-wide single nucleotide polymorphisms (SNPs). In this study, we examined the spatial genetic structure of a deep-sea coral species—the Japanese red coral, Corallium japonicum, sampled off the coast of Kochi, which lies to the southwest of the Shikoku Island in Japan; the Kochi region suffers from over-harvesting because of its high commercial value. We also examined the power of detecting significant spatial genetic structure by changing the number of loci and the proportion of missing data using both de novo analysis and mapping analysis. Similar results were obtained for both de novo and mapping analysis, although a higher number of loci were obtained by the mapping method. In addition, “many SNPs with a lot of missing data” was generally more useful than “a small number of SNPs with a small amount of missing data” to detect significant fine-scale spatial genetic structure. Our data suggested that more than 700 neutral SNPs were needed to detect significant fine-scale spatial genetic structure. The maximum first distance class that can detect significant spatial genetic structure within Kochi for the C. japonicum population was less than 11 km, suggesting that the over-harvesting of C. japonicum within a diameter of approximately 11 km in the Kochi area should be avoided, because this can cause the local extinction of this species.
Introduction
Deep-sea corals play an important role in increasing the biodiversity of the deep-sea by providing complex habitat structures for other species (Roberts et al., 2006). Some of them are also one of the most valuable marine resources with high economic value, as despite their limited supply, they are used as raw material for jewelry, especially in China and Taiwan (Tsounis et al., 2010). In Japan, three deep-sea coral species (Corallium japonicum, Pleurocorallium elatius, and Pleurocorallium konojoj) are harvested and used to produce jewelry as precious corals in Kochi, Kagoshima, Okinawa, and Ogasawara Islands (CITES, 2007, 2009). Precious corals have low fecundity rate (Nonaka et al., 2015), slow growth rate (Luan et al., 2013), and are highly threatened by poaching and overexploitation (Iwasaki, 2019). The Ministry of the Environment, Japan, has listed the three coral species as critically endangered species in the Red List (Ministry of the Environment Government of Japan, 2017).
The diametric and linear growth rate of the Japanese red coral C. japonicum is only 0.2 mm and 2–6 mm per year, respectively (Luan et al., 2013), with a maximum growth of up to 30 cm (Iwasaki et al., 2009), implying that it has a high longevity. Corallium japonicum is a gonochoristic broadcast spawning species (Kishinouye, 1904); thus, the size of a population and connectivity among different populations can be defined by the extent of larval dispersal. Defining population boundaries is important for conservation of a species, although it is particularly problematic in marine species (Berry et al., 2012), especially long-lived sessile species (Ayre and Hughes, 2000). Moreover, the pelagic larval dispersal duration (PLD) for most deep-sea coral species, including C. japonicum, is unknown (Waller, 2005), which further challenges the estimation of the extent of spatial larval dispersal.
Spatial genetic structure (SGS) based on a sufficient number of loci can examine the extent of genetic interactions between continuously distributed populations and can serve as an indicator for estimating the spatial extent of a population, i.e., a minimum and critical unit for conservation of a species (Epperson, 2005; Schwartz and McKelvey, 2009). In the Mediterranean Sea, SGS analyses of the red coral Corallium rubrum that was found at depths shallower than 50 m have suggested the presence of a marked genetic structuring within a few meters (Costantini et al., 2007); another analysis revealed an estimated patch size of less than 40 cm for this species (Ledoux et al., 2010; Costantini et al., 2018), indicating that self-recruitment appears to be crucial for the maintenance of the populations of red coral species (Ledoux et al., 2010). To the best of our knowledge, however, there is no previous study on SGS analysis for deep-sea marine species due to the difficulty in obtaining global positioning system (GPS) information for marine species, especially deep-sea species, and the unavailability of sufficient and suitable markers for SGS.
Multiplexed inter simple sequence repeat (ISSR) genotyping by sequencing (MIG-seq) can detect a moderate number of single nucleotide polymorphisms (SNPs) from putatively neutral loci adjacent to microsatellite regions using polymerase chain reaction (PCR) and high-throughput technology. This method is relatively easy, cost-effective, and can be performed even without a reference genome. Because MIG-seq is a PCR-based method, low concentration and/or low-quality DNA that is often extracted from deep-sea coral species (Eguchi et al., 2020) can be used. Furthermore, previous studies on octocoral species have demonstrated that MIG-seq analysis is useful for uncovering the genetic structures and lineages that were undetectable using a small number of traditional genetic markers, including microsatellite loci and mitochondrial DNA (Richard et al., 2018; Takata et al., 2019).
There is always a trade-off between the number of SNPs obtained and the number of missing data per locus when using high-throughput analysis, such as restriction site-associated DNA sequence (RAD-seq) (Miller et al., 2007). Therefore, maintaining a balance between the number of SNPs and the ratios of missing data per locus is important for conducting accurate genetic analysis, although much information is not available for SGS analysis (Shafer et al., 2017); thus, the robustness of using a reference genome for detecting SGS using MIG-seq analysis has never been empirically examined.
In this study, we (1) examined the robustness of detecting SGS by changing the number of loci and the proportion of missing data using both in de novo analysis and mapping methods, and (2) revealed the extent of C. japonicum (Japanese red coral) population (in terms of larval dispersal) in one of the most important fishing grounds, Kochi.
Materials and Methods
Coral Sampling and DNA Extraction for MIG-Seq Analysis
We exhaustively sampled 88 individual colonies of C. japonicum using a traditional coral net, and GPS information for each coral was recorded based on the ship locations at which fishermen started drawing the nets off the coast of Kochi in Japan from 2011 to 2014 (Figure 1). The horizontal distance at which each coral was sampled was approximately 18 km × 18 km; the maximum distance between the colonies was 18.6 km. The depth at which each coral was sampled was similar (100–140 m), and, thus, we only considered horizontal distance among the samples. Almost 1 cm of coral fragment from each sample was collected and preserved in 99.5% ethanol until genomic DNA extraction.
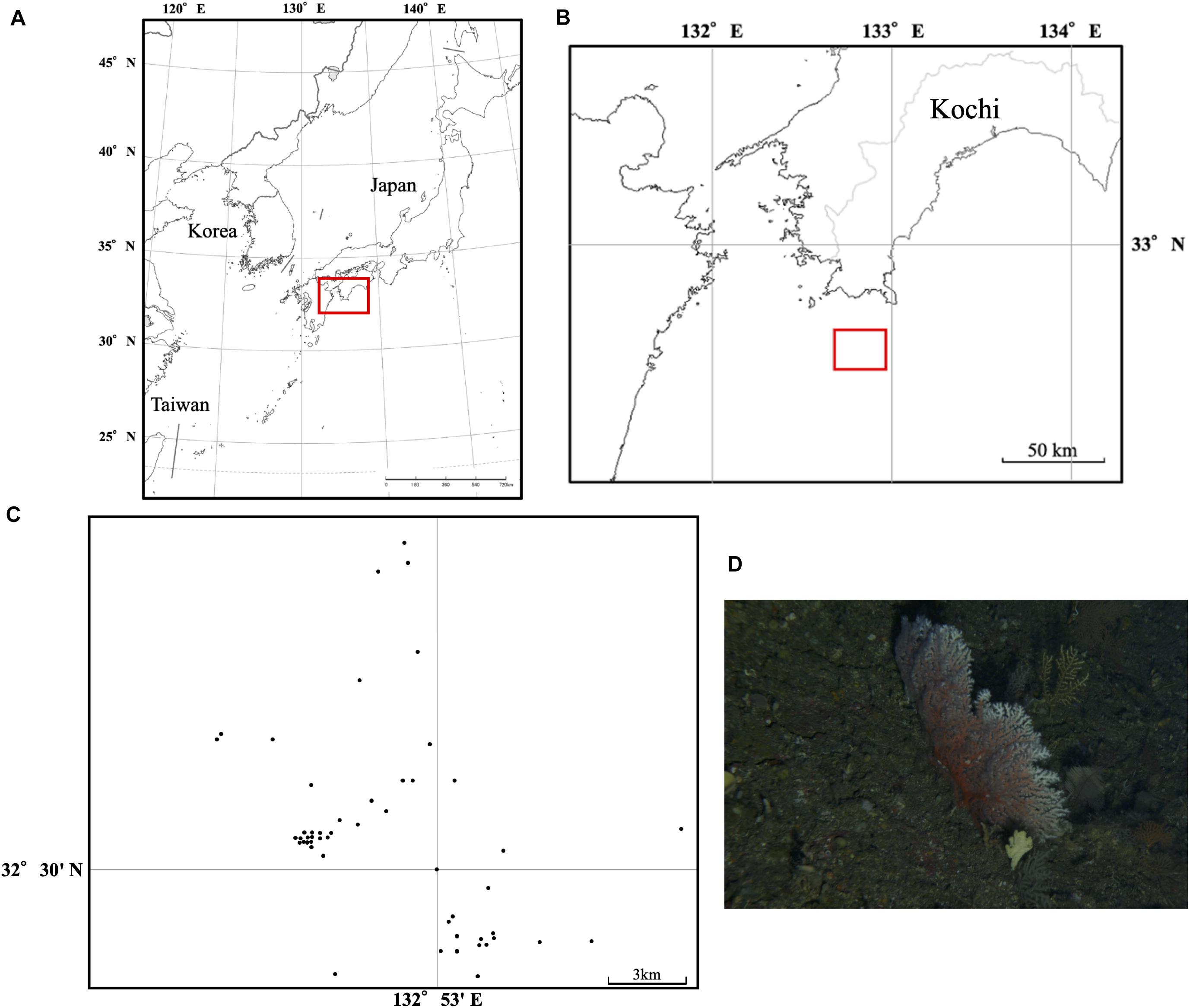
Figure 1. Sampling location off Kochi (A,B) and distribution of 88 individual colonies of C. japonicum collected in this study area (C). A picture of the Japanese red coral C. japonicum (D).
The genomic DNA was extracted using a modified heat alkaline method (Nakabayashi et al., 2019; Takata et al., 2019). We used the MIG-seq method (Suyama and Matsuki, 2015) to obtain genome-wide SNP data. MIG-seq amplifies putatively neutral, anonymous genome-wide ISSR regions (Gupta et al., 1994; Zietkiewicz et al., 1994) using a simple 2-step PCR procedure. We used eight pairs of multiplex ISSR primers (MIG-seq primer set 1, Suyama and Matsuki, 2015) with the Multiplex PCR Assay Kit Ver. 2 (TaKaRa Bio Inc., Otsu, Shiga, Japan) for the first PCR; we used a total reaction volume of 7 μL in a T100TM Thermal Cyclerr (Bio-Rad) with the following conditions: initial denaturation at 94°C for 1 min, followed by 27 cycles of 94°C for 30 s, 38°C for 1 min, 72°C for 1 min, and final extension at 72°C for 10 min. The samples were indexed in the second PCR, which was performed with PrimeSTAR GXL DNA polymerase (TaKaRa Bio Inc., Otsu, Shiga, Japan) using a total of 12 μL reaction volume in a thermal cycler under the following conditions: 21 cycles of 98°C for 10 s, 54°C for 15 s, and 68°C for 1 min. The PCR products were mixed and pooled and run on an agarose gel; the gel extraction of the PCR products that were 350–800 bp in length was performed. The gel-extracted DNA samples were sequenced using MiSeq (sequencing control software v2.0.12, Illumina) with the MiSeq Reagent v3 150 cycle kit (Illumina). Image analysis and base calling were performed using the real-time analysis software v1.17.21 (Illumina).
Field Study Permissions
The samples were collected under the Kochi Prefecture sampling permit (Sa 401, Sa 412, and Sa 423) and Okinawa Prefecture sampling permit (Oki 21-131).
Data Analysis
To eliminate low-quality reads and primer sequences from the raw data, we used the FASTX-toolkit version 0.0.14 (fastaq_quality_filter)1 with a fastq-quality-filter setting of –q 30 –p 40. We removed adapter sequences for the Illumina MiSeq run from both the 5′ end (GTCAGATCGGAAGAGCACACGTCTGAACTCCAGTCAC) and the 3′ end (CAGAGATCGGAAGAGCGTCGTGTAGGG AAAGAC) using Cutadapt version 1.13 (Martin, 2011), and we excluded short reads of less than 80 bp.
For de novo analysis, the quality-filtered sequence data were demultiplexed and filtered using Stacks v1.46 (Catchen et al., 2011; Catchen et al., 2013). We used Stacks v. 1.4 (Catchen et al., 2013) to stack the reads and extract the SNPs. First, we used Ustacks with the following settings: “minimum depth of coverage required to create a stack (m)” = 3, “maximum distance allowed between stacks (M)” = 1, and “maximum distance allowed to align secondary reads to primary stacks (N)” = 1; deleveraging (d) and removal (r) algorithms were also enabled. Second, we used Cstacks with the following settings: “number of mismatches allowed between sample loci when building the catalog (n)” = 4, followed by the Sstacks. We created different SNP sets using the population software implemented in Stacks v. 1.4 by restricting data analysis: the minimum percentage of individuals required to process a locus across all data (r) was set at 0.5–1.0 (0.1 increments), and all SNPs per locus were used. For all of these analyses, we used the following parameters: “the minimum minor allele frequency required to process a nucleotide site at a locus (min_maf)” = 0.01, and “the maximum observed heterozygosity required to process a nucleotide site at a locus (max_obs_het)” = 0.99.
For mapping analysis, we used the Burrows-Wheeler Aligner (BWA) program, specifically, the BWA-MEM algorithm (Li and Durbin, 2009). The SAM files were converted into BAM output files, which were subsequently sorted and indexed; the quality and mapping percentages per scaffold in these files were then checked. The SAM files were then used to perform SNP calling in the software Gstacks implemented in Stacks v. 2.2 (Catchen et al., 2013; Rochette and Catchen, 2017).
For the reference genome sequencing of C. japonicum, we used one specimen of target species collected off Shiraho, Okinawa, Japan, in 2011, using remotely operated vehicle. We extracted DNA from this specimen by using the DNeasy Blood and Tissue Kit (Qiagen, Hilden, Germany) following the manufacturer’s instructions. Using the Nextera XT DNA Sample Prep Kit (Illumina), we sequenced the genome of C. japonicum and obtained paired-end (2 × 300 bp) reads. The adapter sequences in the reads were filtered using Cutadapt version 1.9.1 software (Martin, 2011); the reads with poor-quality bases (Q < 20) and those with lengths < 40 bp were excluded. We assembled these reads using SPAdes version 3.9.0 (Bankevich et al., 2012) with the following parameters: -k 21, 33, 55, 77, 99, and 127. Then, we retained contig sequences with coverage between 1 × to 24 × and removed sequences artificially produced by genome assembly software using Purge Haplotigs with the setting: -l 1 -m 3 -h 24 (Roach et al., 2018).
We used Gstacks option (-rm, -pcr, -duplicates) in Stacks v. 2.2 to remove PCR duplicates by randomly discarding all but one pair of each set of reads. We used the population software to prepare different datasets for subsequent analyses. We changed the ratio of missing data (r; proportion of shared SNPs among samples) ranging from 0.5 to 1 (0.1 increments). For all of these analyses, we also used the following parameters: “the minimum minor allele frequency required to process a nucleotide site at a locus (min_maf)” = 0.01, and “the maximum observed heterozygosity required to process a nucleotide site at a locus (max_obs_het)” = 0.99. BayeScan v 2.1 (Narum and Hess, 2011) was used to detect possible SNPs under natural selection with a default setting.
Indicators of genetic diversity, nucleotide diversity (π) and heterozygosity (HE), and inbreeding coefficient (FIS) were calculated using the population software and data from de novo and mapping were compared based on Mann-Whitney U test. Hardy–Weinberg equilibrium (HWE) was examined for both the de novo (2,280 SNPs with r = 0.5) and mapping (892 SNPs with r = 0.9) datasets using GeneAlEx ver. 6.5 (Peakall and Smouse, 2012).
Spatial Autocorrelation Analysis
To detect SGS, we used GenAlEx ver. 6.5 (Peakall and Smouse, 2012), which calculates a pairwise genetic and pairwise geographical distance matrix to generate an autocorrelation coefficient (indicated as rs; the correlation value between the genetic and geographic distance in this manuscript; see Figure 4) for a given distance class. Each distance class is bound by an upper and lower value (e.g., 0–1 km). All pairwise comparisons within a geographic range given the distance class are used to test the null hypothesis that the genotype pairs are randomly distributed within the geographic range. Spatial autocorrelation analysis superimposed the 95% confidence interval for the null hypothesis of a random SGS on the correlogram. Under restricted gene flow, and in the absence of selection, we will observe positive significance (the rs value is higher than the 95% confidence interval for the null hypothesis of random SGS) at short distance classes, indicating that the geographically close individuals tend to have closer genotypes. Subsequently, the autocorrelation coefficient will decline through zero (here we define this point as x-intercept, see Figure 4) and become negative. This x-intercept provides an estimate of the extent of the positive genetic structure only if a positive significant SGS was found.
Currently, two complimentary analyses of significant SGS are often used (Peakall et al., 2003; Underwood et al., 2007): the first one uses x-intercepts (conservative permutation test using relatively short distance class). The intercept reflects the population size that is useful for the conservation of a species and for accurately assessing genetic diversity (Diniz-Filho and Telles, 2002). The second one relies on more powerful permutation tests and the use of the maximum first distance class that can detect significant SGS. This is because a single correlogram can be strongly influenced by the interactions between the extent of genetic structure and distance class sizes, together with the number of samples existing within that geographic range (Peakall et al., 2003; Underwood et al., 2007). In this study, we calculated both the x-intercepts and the maximum first distance class, but the maximum significant first distance class was regarded as the maximum range of gamete and larval dispersal.
The number of permutation tests was set to 9,999 for each analysis. We tested 15 different distance classes from 1 to 15 km using different ratios of missing data per locus for de novo (r = 0.5–0.9; 1.0 failed) and mapping (r = 0.5–1.0) analyses. To estimate the minimum number of SNPs that can detect significant SGS, we used r values (at intervals of 0.01) 0.7–0.8 and 0.9–1.0 for de novo and mapping analyses, respectively. We used the PGD spider ver 2.0.8.3 (Lischer and Excoffier, 2011) to convert SNP data file formats.
Results
Detection of SNPs
A total of 23,991,966 raw reads with an average of 272,636 reads per sample (SD = 92,029.33, SE = 147.81) were obtained for the 88 sampled corals, and after filtering out the low-quality reads, 23,828,764 reads remained. We obtained 16,679,162 reads with an average of 189,536 reads per individual (SD = 61,902.56, SE = 122.51) after two-step filtering. We obtained de novo reference genome assemblies using paired-end short reads of C. japonicum and the amount of sequence data used for assembly, total assembly size, number of sequences, percentage of gaps, N50 value, longest and minimum sequence lengths are available in Supplementary Table 1. The mean value of GC contents of the de novo genome sequences were 38.3%, which is almost similar to that for other anthozoan genomes (Shinzato et al., 2011). Single sharp peaks on the histograms of the GC contents also support the low contamination level of this assembly (Supplementary Figure 1). However, caution should be warranted for the usage of the C. japonicum genome assembly, as no low-coverage sequences were not removed by Purge Haplotigs, DNA sequences from bacterial contaminants and other organisms might be also included in the C. japonicum genome assembly (Supplementary Figure 2).
We identified 0–2,280 SNPs by de novo analysis and 132–5,884 SNPs by mapping analysis (Figure 2). We detected 3–15 times more SNPs by mapping analysis than by de novo analysis when comparing with the same missing data ratio (r). BayeScan indicated that all the SNPs were neutral (q > 0.05). FIS (variant positions) values were significantly higher (P > 0.05) in de novo analysis than in mapping analysis (Figure 3). The number of SNPs that deviated from HWE was 1,813 out of 2,280 SNPs (79.5%) for de novo analysis and 223 out of 892 SNPs (25.0%) for mapping analysis.
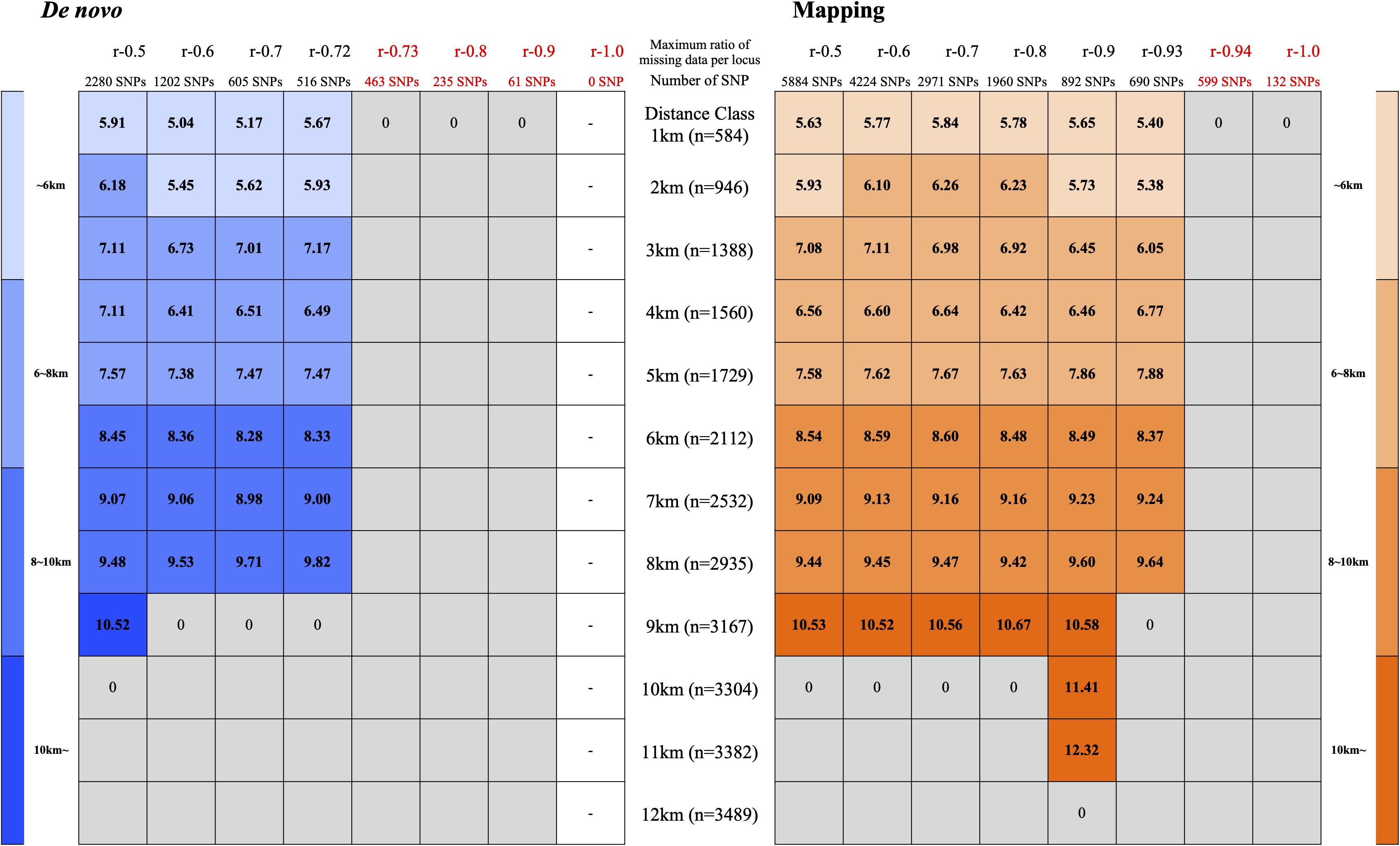
Figure 2. Outcomes of spatial genetic autocorrelation analysis with the different distance classes (1–15 km) using different ratios of missing data per locus (r = 0.5–1.0; r–1.0 for de novo was failed). Note that the x-intercepts were converted to zero when the correlation was not significantly different from zero and shown in gray. The color counter reflects the size of the x-intercept (lighter colors indicate smaller x-intercept values).
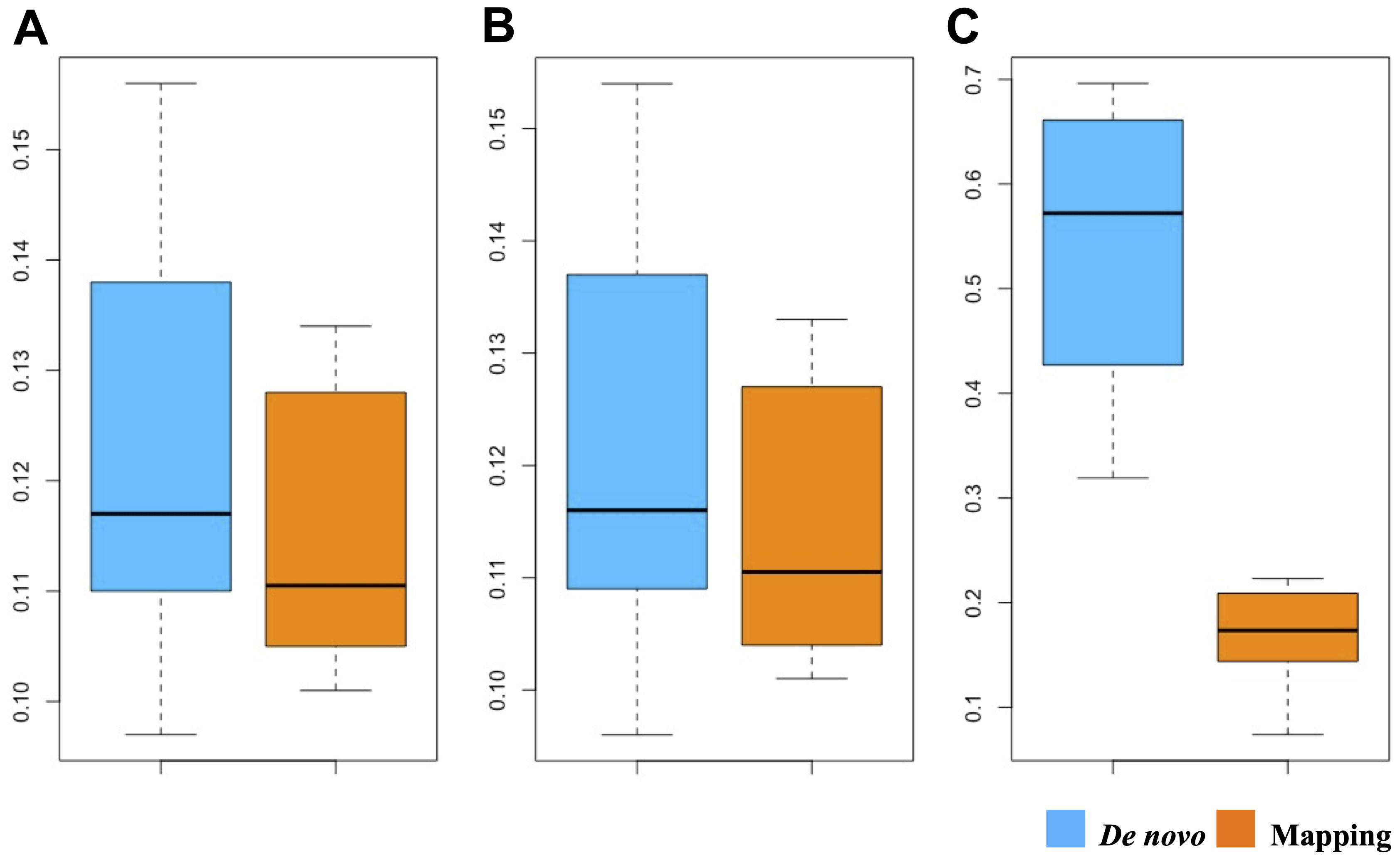
Figure 3. Boxplot showing the relationship between π (A), expected heterozygosity (B) and FIS (C) using SNPs obtained by de novo and mapping.
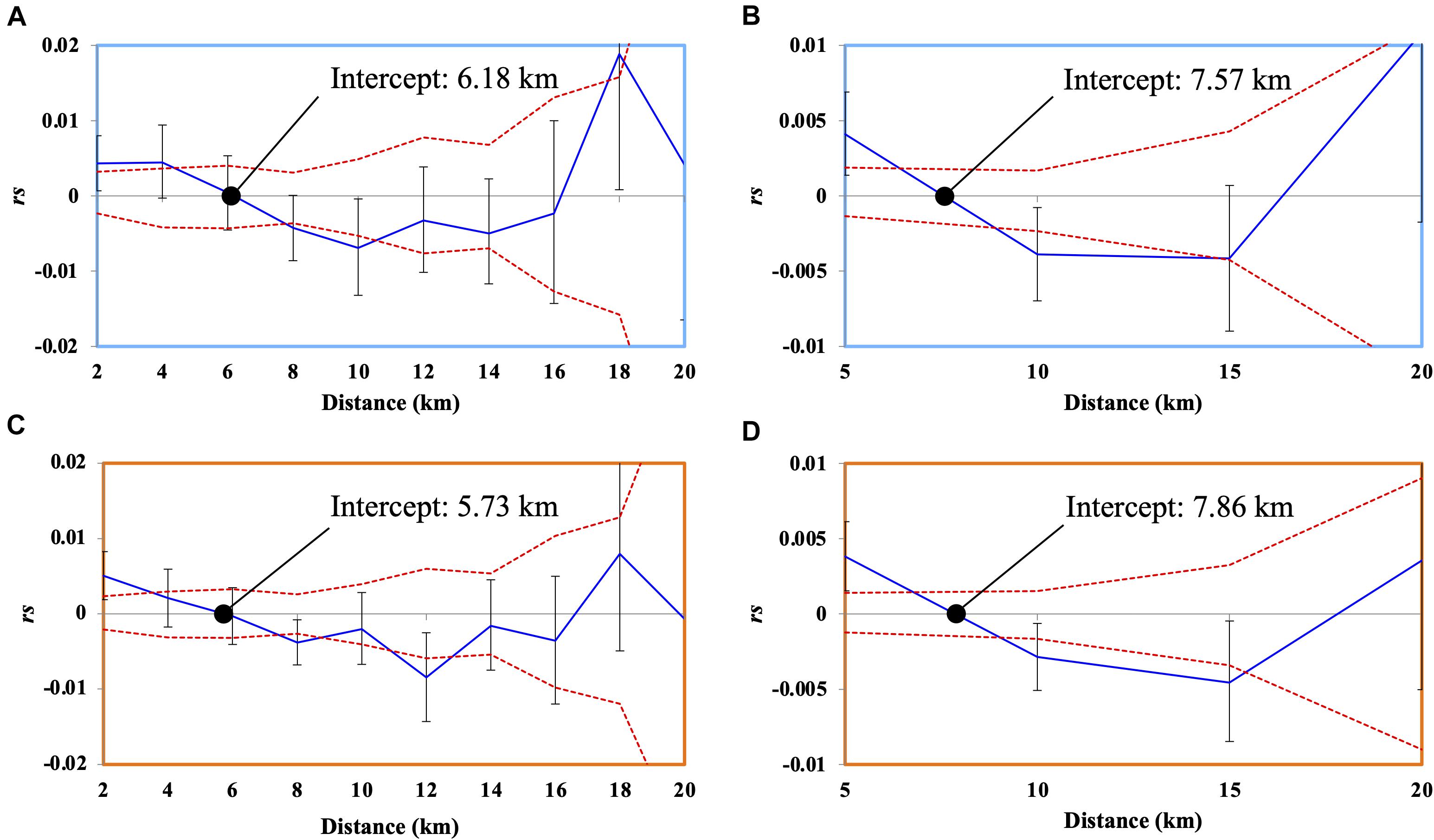
Figure 4. Correlograms showing the spatial autocorrelation rs (here “rs” is used instead of “r” that is generally used for correlation coefficient of spatial autocorrelation analysis to avoid confusion with the ratio of missing data per locus “r”) across as a function of distance, 95% confidence interval about the null hypothesis of a random distribution of C. japonicum, and 95% confidence error bars about rs as determined by bootstrapping. Autocorrelation for distance class size of (A) 2 km using de novo (2,280 SNPs), (B) 5 km using de novo (2,280 SNPs), (C) 2 km using mapping method (892 SNPs), and (D) 5 km using mapping method (892 SNPs).
Spatial Autocorrelation Analysis
Spatial autocorrelation analysis was performed with 15 different classes of distance (1–15 km) using different ratios of missing data and numbers of SNPs based on both de novo analysis and mapping analysis.
Overall, when using a sufficient number of loci (>700 SNPs, Figure 2 and Supplementary Table 2), both de novo analysis and mapping analysis showed similar results (Figure 2 and Supplementary Table 2); the maximum distances up to which the significant genetic structure detected were 11 km for the mapping analysis and 9 km for de novo analysis. However, the mapping analysis detected a wider range of significantly positive structures for the x-intercepts (summarized in Figure 2 and Supplementary Table 2); the range of x-intercepts was from 5.04 km (r = 0.6) to 10.52 km (r = 0.5) for the de novo analysis and from 5.63 km (r = 0.5) to 12.32 km (r = 0.9) for the mapping analysis (Figure 2 and Supplementary Table 2).
The correlograms based on de novo analysis (r = 0.5, 2,280 SNPs) and mapping analysis (r = 0.9, 892 SNPs) indicated similar results (Figure 4). When using the 2 km distance class, the correlation values were positive and significant up to 4 km and significantly negative from 8 km with an x-intercept of 6.18 km (de novo analysis, Figure 4A) and positive and significant up to 2 km and significantly negative from 8 km with an x-intercept of 5.73 km (mapping analysis, Figure 4C). When using the 5 km distance class, the correlation values were positive and significant up to 5 km, and negative and significant from 10 to 15 km, with an x-intercept of 7.57 km (de novo analysis, Figure 4B) and 7.86 km (mapping analysis, Figure 4D). The correlograms (including most correlograms in our data) show oscillations of high and low autocorrelation for negative and significant rs values (Figure 4), indicating chaotic spatial patterns of genetic relatedness of C. japonicum at these scales. Such positive autocorrelation in a short distance class indicates limited larval dispersal within the sampling site (Peakall et al., 2003).
The Number of SNPs Required for Detecting SGS
To examine the minimum number of SNPs required to detect significant SGS, analysis was performed at r = 0.7–0.8 for de novo analysis and r = 0.9–1.0 for mapping analysis with an interval of 0.01. De novo analysis could detect significant SGS when using > 516 SNPs (r = 0.72), while the mapping data could detect significant SGS when using > 690 SNPs (r = 0.93), indicating that more than 700 neutral SNPs obtained by MIG-seq are sufficient to detect significant SGS in our C. japonicum dataset (Figure 2 and Supplementary Table 3).
Discussion
Estimating the spatial extent of gamete and larval dispersal is important for defining the size of a population and assessing the conservation management unit of marine organisms, although it has been challenging, especially for deep-sea organisms whose spawning period and PLD are unknown. In this study, we showed that both de novo analysis and mapping analysis are useful for detecting significant SGS when using a sufficient number of loci (> 700 SNPs), although mapping analysis has more advantages in obtaining a larger number of SNPs with a lower ratio of missing data. Furthermore, we demonstrated that the size of C. japonicum population that withstands frequent gamete and larval dispersal is within an area of less than 11 km off the Kochi area.
Robustness of Detecting SGS Using de novo Analysis and Mapping Analysis in MIG-Seq Analysis
Many population genetic inferences based on high-throughput sequence data are influenced by bioinformatic pipelines on population genetic parameters, but this field has received little attention (Shafer et al., 2017). Thus, a comparative analysis of the robustness of different parameter settings for de novo analysis and mapping analysis to detect SGS would help fill the knowledge gap. This study suggests that significant SGS and comparative results with the mapping method can be obtained even without reference genome sequence data when a sufficient number of loci is used (>700 SNPs).
All genetic diversity statistics, FIS, were higher in de novo analysis than in mapping analysis. A larger number of SNPs significantly deviated from HWE in de novo analysis than in mapping analysis. Mapping analysis using reference genome sequences has more advantages in distinguishing repetitive and/or duplicated regions from real SNPs than de novo analysis. It is likely that some of the SNPs obtained by de novo analysis included some repetitive and/or duplicated regions that resulted in higher genetic diversity and inbreeding coefficient. Similar to the present study, Shafer et al. (2017) also calculated summary statistics to confirm the validity of the results obtained by de novo and mapping analyses based on the RAD-seq data. They reported that mapping on a close reference genome detected more SNPs and reduced FIS. In the present study, we found that the mapping analysis could detect a wider spatial extent of positive significant structure (up to 11 km) when using a moderate number of SNPs with a lower proportion of missing data (r = 0.9). Arnold et al. (2013) showed that the summary statistics can deviate from true values and biases subsequent to population genetic analysis. Therefore, it is also possible that a higher proportion of missing data in de novo analysis failed to detect significant SGS. As previously recommended by Shafer et al. (2017), we also conclude that employing reference-based approaches wherever possible is preferable.
Balance Between the Number of Loci and the Ratio of Missing Data for SGS Analysis
In high-throughput sequencing of reduced representation libraries, it is difficult to choose between “a large number of SNPs with a lot of missing data” and “a small number of SNPs with a small amount of missing data.” Although a few studies have examined the impact of missing data obtained by RAD-seq on population genetics (Arnold et al., 2013; Buerkle and Gompert, 2013) and phylogenetic analysis (Roure et al., 2013; Huang and Knowles, 2016), to the best of our knowledge, the present study is the first to empirically examine the balance between the number of SNPs and the ratio of missing data for SGS analysis using MIG-seq data.
Significant SGS was detected when using > 516 SNPs (r = 0.72) for de novo analysis and 690 SNPs (r = 0.93) for mapping analysis, indicating that approximately > 700 SNPs are necessary to detect significant SGS using MIG-seq data. Our results imply that choosing “a large number of SNPs with a lot of missing data” is more efficient than choosing “a small number of SNPs with a small amount of missing data” to detect significant SGS. Spatial autocorrelation analysis examines genetic relatedness among different individuals. Examining the relative genetic distance among such genetically close kinships may require a relatively large number of SNPs, even with relatively high missing data. In phylogenetic analysis, even though missing data have adverse effects, such as decreasing resolving power and the detection of multiple substitutions (Roure et al., 2013), empirical studies have suggested that a large number of SNPs despite a large amount of missing data has a higher resolution in providing phylogenetic inferences (Rubin et al., 2012; Wagner et al., 2013).
Although a sufficient number of loci is important for detecting significant SGS, a smaller ratio of missing data in mapping analysis (r = 0.9) can detect significant SGS in a wider geographical range, implying that missing data may also slightly influence the robustness of detecting significant SGS.
Gamete and Larval Dispersal of C. japonicum Estimated by SGS Analysis
Spatial autocorrelation analysis is a theoretically well-established method to estimate the extent of dispersal of target species (e.g., Epperson and Li, 1996). Spatial autocorrelation analysis of C. japonicum based on MIG-seq loci revealed a pattern of significant positive local genetic structure, suggesting that proximate C. japonica colonies are genetically more similar than the distant colonies. Generally, the first x-intercept of the correlogram provides an estimate of the extent of positive genetic structure that is interconnected by dispersal (Peakall et al., 2003); it is also proposed to be used for deciding the management unit for conservation and to establish sampling strategies (Diniz-Filho and Telles, 2002). However, the x-intercept is largely dependent on the interaction among pre-defined distance-class size, unknown genetic structure, and the associated number of samples per distance class (Peakall et al., 2003). As suggested by Peakall et al. (2003), we examined the maximum first distance class by calculating SGS using different distance classes. We interpreted the distance class at which the estimation of r was no longer significant enough to serve as the maximum extent of the C. japonicum population that was formed by gamete and larval dispersal in the Kochi area. In the present study, we detected 11 km of significant maximum SGS with (mapping method r = 0.9, 892 SNPs) indicating that the spatial extent of gamete and larval dispersal occurred within this scale.
The SGS (<11 km) estimated for C. japonicum was larger than that of another Coralliidae coral Corallium rubrum (< 40 cm) that was revealed using microsatellite loci in a previous study (Ledoux et al., 2010; Costantini et al., 2018). Differences in habitat environments could be attributed to this difference; C. japonicum lives in a relatively open continental shelf deep-sea, while the C. rubrum sampled in Costantini et al. (2007, 2018) and Ledoux et al. (2010) lived in a shallow, semi-closed cave. Further SGS studies using C. rubrum specimens obtained from deep water (e.g., deeper than 100 m, Costantini et al., 2010, 2013) would be useful to test this hypothesis. Alternatively, it is possible that broadcast spawner C. japonicum (Kishinouye, 1904) has more gamete and larval dispersal potential than the brooder C. rubrum (Lacaze-Duthiers, 1864). Generally, broadcast spawners have greater dispersal potential than brooding coral species (e.g., Nishikawa et al., 2003). Similarly, a brooder scleractinian coral in shallow water, Seriatopora hystrix (Fan et al., 2002), has significant SGS within 100 m (Underwood et al., 2007), which is also a smaller scale of dispersal than that of C. japonicum. In contrast, another brooding coral, but with a relatively long larval dispersal period (Pocillopora damicornis, 100 days) (Harii et al., 2002), has larger SGS (60 km, Thomas et al., 2014), indicating that the reproductive strategy alone cannot predict the spatial genetic extent.
Our data suggest the extent of a population of C. japonicum off Kochi is less than 11 km and intensive coral sampling within 11 km in diameter would result in failure of local reproduction and subsequently cause local extinction. Although different locations may have different environmental conditions and local coral densities, a population size of 11 km in the main coral fishing ground (relatively high coral density in Kochi (Cannas et al., 2019)) implies that the extent of a population in other areas with a lower population density may be smaller than 11 km.
Conclusion
In this study, we demonstrated that both de novo and mapping analyses using MIG-seq are effective in detecting significant SGS for C. japonicum populations when a sufficient number of loci (>700 SNPs) are used. Mapping analysis is preferred, as it is more robust in detecting SGS by using accurate SNPs data with lesser missing data. The results suggest that avoiding intensive coral fishing within 11 km is important for the conservation of C. japonicum in the Kochi area. Collectively, the findings of this study provide important implications for the development of conservation strategies for C. japonicum. Further spatial genetic analysis of deep-sea species using genome-wide SNPs would be useful to understand undetectable dispersal that is important for marine conservation.
Data Availability Statement
The datasets presented in this study can be found in online repositories. The names of the repository/repositories and accession number(s) can be found below: DDBJ (accession: DRA011567 and DRA010782), Figshare (https://doi.org/10.6084/m9.figshare.13678735.v2).
Author Contributions
NY conceived the study. FI, NI, KU, and TS collected the samples. FI, NI, and MN conducted the morphological analysis. KT and TK conducted the molecular experiments. AI and NI sequenced the draft genome. KT, AI, HY, HT, TK, and NY analyzed the data. KT and NY drafted the manuscript. All authors have checked and edited the final manuscript.
Funding
This study was supported by a Grant-in-Aid for Young Scientists (A) (17H04996), a Grant-in-Aid for Research Fellows (19J21342), Grant-in-Aid for Scientific Research (C) (17K07274), Grant-in-Aid for Scientific Research (A) (20254934) and the Sumitomo Foundation Fiscal 2018 Grant for Basic Science Research Projects.
Conflict of Interest
The authors declare that the research was conducted in the absence of any commercial or financial relationships that could be construed as a potential conflict of interest.
Acknowledgments
We are grateful to Mr. Masao Nakano, Yoshihiko Niiya, and Katsuhiro Fujita for their assistance with the collection of samples in Kochi. We thank Akemi Yoshida and Eriko Nagahiro for their help in the data analysis.
Supplementary Material
The Supplementary Material for this article can be found online at: https://www.frontiersin.org/articles/10.3389/fmars.2021.667481/full#supplementary-material
Supplementary Figure 1 | Mean value of the GC contents of Corallium japonicum collected in Okinawa using a Nextera XT DNA Sample Prep Kit (Illumina).
Supplementary Figure 2 | A graph showing coverage read-depth of Corallium japonicum.
Supplementary Table 1 | Summary of sequence assembly of Corallium japonicum.
Supplementary Table 2 | Outcomes of spatial genetic autocorrelation calculated with the different first distance classes (1–15 km) using different ratios of missing data per locus (r=0.5–1.0). The correlation rs (here, “rs” is used instead of “r,” which is generally used to represent the correlation coefficient of spatial autocorrelation analysis to avoid confusion with the ratio of missing data per locus “r”), is shown for the first distance class in each analysis along with the number of pairwise comparisons “n” for the calculation of “rs,” and the upper (U) and lower (L) bounds for the 95% confidence interval for the null hypothesis of no spatial structure (rs = 0), and the upper (Ur) and lower bounds (Lr) for r, as determined by bootstrap resampling, the probability P of a one-tailed test for positive autocorrelation, and the estimated x-intercept. Note that the x-intercepts were converted to zero when the rs was not significantly different from zero.
Supplementary Table 3 | Outcomes of spatial genetic autocorrelation calculated with the different first distance sizes of 1–15 km using r-values at intervals of 0.01 between 0.7–0.8 and 0.9–1.0 for the de novo and mapping analysis, respectively.
Supplementary Table 4 | Genpop format file for de novo analysis (r−0.5).
Supplementary Table 5 | Genpop format file for mapping analysis (r−0.9).
Supplementary Table 6 | Geographic Distance of each sample (km).
Footnotes
References
Arnold, B., Corbett-Detig, R. B., Hartl, D., and Bomblies, K. (2013). RADseq underestimates diversity and introduces genealogical biases due to nonrandom haplotype sampling. Mol. Ecol. 22, 3179–3190. doi: 10.1111/mec.12276
Ayre, D. J., and Hughes, T. P. (2000). Genotypic diversity and gene flow in brooding and spawning corals along the Great Barrier Reef, Australia. Evolution 54, 1590–1605. doi: 10.1111/j.0014-3820.2000.tb00704.x
Bankevich, A., Nurk, S., Antipov, D., Gurevich, A. A., Dvorkin, M., Kulikov, A. S., et al. (2012). SPAdes: a New Genome Assembly Algorithm and Its Applications to Single-Cell Sequencing. J. Comput. Biol. 19:5. doi: 10.1089/cmb.2012.0021
Berry, O., England, P., Marriott, R. J., Burridge, C. P., and Newman, S. J. (2012). Understanding age-specific dispersal in fishes through hydrodynamic modeling, genetic simulations and microsatellite DNA analysis. Mol. Ecol. 21, 2145–2159. doi: 10.1111/j.1365-294X.2012.05520.x
Buerkle, A. C., and Gompert, Z. (2013). Population genomics based on low coverage sequencing: how low should we go? Mol. Ecol. 22, 3028–3035. doi: 10.1111/mec.12105
Cannas, R., Follesa, M. C., Cau, A., Cau, A., and Friedman, K. (2019). Global Report on the Biology, Fishery and Trade of Precious Corals. FAO Fisheries and Aquaculture Circulation no, 118. Rome, FAO.
Catchen, J. M., Amores, A., Hohenlohe, P., Cresko, W., and Postlethwait, J. H. (2011). Stacks: building and genotyping loci de novo from short-read sequences. G3 1, 171–182. doi: 10.1534/g3.111.000240/-/DC1
Catchen, J., Hohenlohe, P. A., Bassham, S., Amores, A., and Cresko, W. A. (2013). Stacks: an analysis tool set for population genomics. Mol. Ecol. 22, 3124–3140. doi: 10.1111/mec.12354
CITES. (2007). Inclusion of all Species in the Genus Corallium in Appendix ii of Cites. This Taxon Comprises 26 Closely Related Species. Consideration of Proposals for Amendment of Appendices i and ii. CITES cop14 inf. 36. Netherlands. Available online at: https://www.cites.org/sites/default/files/common/cop/14/inf/E14i-36.pdf (accessed January 19, 2021).
CITES. (2009). Consideration of Proposals for Amendment of Appendices i and ii. CITES cop15 prop. 21, 1. Available Online at: https://www.cites.org/sites/default/files/eng/cop/15/prop/E-15-Prop-21.pdf (accessed January 19, 2021).,Google Scholar
Costantini, F., Carlesi, L., and Abbiati, M. (2013). Quantifying spatial genetic structuring in mesophotic populations of the precious coral Corallium rubrum. PLoS One 8:e61546. doi: 10.1371/journal.pone.0061546
Costantini, F., Fauvelot, C., and Abbiati, M. (2007). Fine-scale genetic structuring in Corallium rubrum: evidence of inbreeding and limited effective larval dispersal. Mar. Ecol. Prog. Ser. 340, 109–119. doi: 10.3354/meps340109
Costantini, F., Rugiu, L., Cerrano, C., and Abbiati, M. (2018). Living upside down: patterns of red coral settlement in a cave. PeerJ 6:e4649. doi: 10.7717/peerj.4649
Costantini, F., Taviani, M., Remia, A., Pintus, E., Schembri, P. J., and Abbiati, M. (2010). Deep-water Corallium rubrum (L., 1758) from the Mediterranean Sea: preliminary genetic characterization. Mar. Ecol. 31, 261–269. doi: 10.1111/j.1439-0485.2009.00333.x
Diniz-Filho, J. A. F., and Telles, M. P. C. (2002). Spatial autocorrelation analysis and the identification of operational units for the conservation in continuous populations. Conserv. Biol. 16, 924–935. doi: 10.1046/j.1523-1739.2002.00295.x
Eguchi, K., Oguri, E., Sasaki, T., Matsuo, A., Nguyen, D. D., Jaitrong, W., et al. (2020). Revisiting museum collection in the genomic era: potential of MIG-seq for retrieving phylogenetic information from aged minute dry specimens of ants (Hymenoptera: formicidae) and other small organisms. Myrmecol. News 30, 151–159. doi: 10.25849/myrmecol.news_030:151
Epperson, B. K. (2005). Estimating dispersal from short distance spatial autocorrelation. Heredity 95, 7–15. doi: 10.1038/sj.hdy.6800680
Epperson, B. K., and Li, T. (1996). Measurement of genetic structure within populations using Moran’s spatial autocorrelation statistics. Proc. Natl. Acad. Sci. U. S. A. 93, 10528–10532. doi: 10.1073/pnas.93.19.10528
Fan, T. Y., Li, J. J., Ie, S. X., and Fang, L. S. (2002). Lunar periodicity of larval release by pocilloporid corals in Southern Taiwan. Zool. Stud. 41, 288–294.
Gupta, M., Chyi, Y. S., Romero-Severson, J., and Owen, J. L. (1994). Amplification of DNA markers from evolutionarily diverse genomes using single primers of simple-sequence repeats. Theoret. Appl. Genet. 89, 998–1006. doi: 10.1007/BF00224530
Harii, S., Kayanne, H., Takigawa, H., Hayashibara, T., and Yamamoto, M. (2002). Larval survivorship, competency periods and settlement of two brooding corals, Heliopora coerulea and Pocillopora damicornis. Mar. Biol. 141, 39–46.
Huang, H., and Knowles, L. L. (2016). Unforeseen consequences of excluding missing data from next-generation sequences: simulation study of RAD sequences. Syst. Biol. 56, 400–411. doi: 10.1093/sysbio/syu046
Iwasaki, N. (2019). “Asian regional report on the biology, fishery and trade of precious and semi-precious corals” in Global Report On The Biology, Fishery And Trade Of Precious Corals, eds R. Cannas, M. C. Follesa, A. Cau, A. Cau, and K. Friedman (Rome: FAO Fisheries and Aquaculture Circulation), 191–254.
Iwasaki, N., Hasegawa, H., Suzuki, T., and Yamasa, M. (2009). “Biology of Japanese Corallium and Paracorallium” in Proceedings of the 1st International Workshop on Corallium Science, Management, and Trade, eds A.W. Bruckner and G.G. Roberts (Silver Spring, MD: NOAA Technical Memorandum NMFS-OPR-43 and CRCP-8), 68–70.
Ledoux, J. B., Garrabou, J., Bianchimani, O., Drap, P., Feral, J. P., and Aurelle, D. (2010). Fine-scale genetic structure and inferences on population biology in the threatened Mediterranean red coral, Corallium rubrum. Mol. Ecol. 19, 4204–4216. doi: 10.1111/j.1365-294X.2010.04814.x
Li, H., and Durbin, R. (2009). Fast and accurate short read alignment with Burrows–Wheeler transform. Bioinformatics 25, 1754–1760. doi: 10.1093/bioinformatics/btp324
Lischer, H. E., and Excoffier, L. (2011). PGDSpider: an automated data conversion tool for connecting population genetics and genomics programs. Bioinformatics 28, 298–299. doi: 10.1093/bioinformatics/btr642
Luan, N. T., Rahman, M. A., Maki, T., Iwasaki, N., and Hasegawa, H. (2013). Growth characteristics and growth rate estimation of Japanese precious corals. J. Exp. Mar. Biol. Ecol. 441, 117–125. doi: 10.1016/j.jembe.2013.01.012
Martin, M. (2011). Cutadapt removes adapter sequences from high-throughput sequencing reads. EMBnet J. 17, 10–12. doi: 10.14806/ej.17.1.200
Miller, M. R., Dunham, J. P., Amores, A., Cresko, W.A., and Johnson, E. A. (2007). Rapid and cost-effective polymorphism identification and genotyping using restriction site associated DNA (RAD) markers. Genome Res. 17, 240–248. doi: 10.1101/gr.5681207
Ministry of the Environment Government of Japan. (2017). Marine Species Red List In Japanese. Available Online at: http://www.env.go.jp/press/files/jp/106407.pdf (accessed January 19, 2021).
Nakabayashi, A., Yamakita, T., Nakamura, T., Aizawa, H., Kitano, Y. F., Iguchi, A., et al. (2019). The potential role of temperate Japanese regions as refugia for the coral Acropora hyacinthus in the face of climate change. Sci. Rep. 9:1892. doi: 10.1038/s41598-018-38333-5
Narum, S. R., and Hess, J. E. (2011). Comparison of FST outlier test for SNP loci under selection. Mol. Ecol. Resour. 11, 184–194. doi: 10.1111/j.1755-0998.2011.02987.x
Nishikawa, A., Katoh, M., and Sakai, K. (2003). Larval settlement rates and gene flow of broadcast-spawning (Acropora tenuis) and planula-brooding (Stylophora pistillata) corals. Mar. Ecol. Prog. Ser. 256, 87–97. doi: 10.3354/meps256087
Nonaka, M., Nakamura, M., and Muzik, K. (2015). Sexual reproduction in precious corals (Coralliidae) collected in the Ryukyu Archipelago. Pac. Sci. 69, 15–46. doi: 10.2984/69.1.2
Peakall, R., and Smouse, P. E. (2012). GenAlEx 6.5: genetic analysis in Excel. Population genetic software for teaching and research- an update. Bioinformatics 28, 2537–2539. doi: 10.1093/bioinformatics/bts460
Peakall, R., Ruibal, M., and Linenmayer, D. (2003). Spatial autocorrelation analysis offers new insights into gene flow in the Australian bush rat, Rattus fuscipes. Evolution 57, 1182–1195. doi: 10.1111/j.0014-3820.2003.tb00327.x
Richard, Z. T., Yasuda, N., Kikuchi, T., Foster, T., Mitsuyuki, C., Stat, M., et al. (2018). Integrated evidence reveals a new species in the ancient blue coral genus Heliopora (Octocorallia). Sci. Rep. 8, 15875. doi: 10.1038/s41598-018-32969-z
Roach, M. J., Schmidt, S. A., and Borneman, A. R. (2018). Purge Haplotigs: allelic contig reassignment for third-gen diploid genome assemblies. BMC Bioinf. 19:460. doi: 10.1186/s12859-018-2485-7
Roberts, J. M., Wheeler, A. J., and Freiwald, A. (2006). Reefs of the deep: the biology and geology of cold-water coral ecosystems. Science 312, 543–547. doi: 10.1126/science.1119861
Rochette, N. C., and Catchen, J. M. (2017). Deriving genotypes from RAD-seq short-read using Stacks. Nat. Protoc. 12, 2640–2659. doi: 10.1038/nprot.2017.123
Roure, B., Baurain, D., and Philippe, H. (2013). Impact of missing data on phylogenies inferred from empirical phylogenomic data sets. Mol. Biol. Evol. 30, 197–214. doi: 10.1093/molbev/mss208
Rubin, B. E. R., Ree, R. H., and Moreau, C. S. (2012). Inferring phylogenies from RAD sequence data. PLoS One 7:e33394. doi: 10.1371/journal.pone.0033394
Schwartz, M. K., and McKelvey, K. S. (2009). Why sampling scheme matters: the effect of sampling scheme on landscape genetic results. Conserv. Genet. 10, 441–452. doi: 10.1007/s10592-008-9622-1
Shafer, A. B. A., Peart, C. R., Tusso, S., Maayan, I., Brelsford, A., Wheat, C.W., et al. (2017). Bioinformatic processing of RAD-seq data dramatically impacts downstream population genetic inference. Methods Ecol. Evol. 8, 907–917. doi: 10.1111/2041-210X.12700
Shinzato, C., Shoguchi, E., Kawashima, T., Hamada, M., Hisata, K., and Tanaka, M. (2011). Using the Acropora digitifera genome to understand coral responses to environmental change. Nature 476, 320–323. doi: 10.1038/nature10249
Suyama, Y., and Matsuki, Y. (2015). MIG-seq: an effective PCR-based method for genome-wide single-nucleotide polymorphism genotyping using the next-generation sequencing platform. Sci. Rep. 5:16963. doi: 10.1038/srep16963
Takata, K., Taninaka, H., Nonaka, M., Iwase, F., Kikuchi, T., Suyama, Y., et al. (2019). Multiplexed ISSR genotyping by sequencing distinguishes two precious coral species (Anthozoa: Octocorallia: Coralliidae) that share a mitochondrial haplotype. PeerJ 7:e7769. doi: 10.7717/peerj.7769
Thomas, L., Kendrick, G. A., Stat, M., Travaille, K. L., Shedrawi, G., and Kennington, W. J. (2014). Population genetic structure of the Pocillopora damicornis morphospecies along Ningaloo Reef, Western Australia. Mar. Ecol. Prog. Ser. 513, 111–119 doi: 10.3354/meps10893
Tsounis, G., Rossi, S., Grigg, R., Santangelo, G., Bramanti, L., and Gili, J. M. (2010). “The exploitation and conservation of precious corals,” in Oceanography and Marine Biology: An Annual Review, eds R. N. Gibson, R. J. A. Atkinson, and J. D. M. Gordon (Boca Raton, FL: CRC Press), 161–212.
Underwood, J. N., Smith, L. D., van Oppen, M. J. H., and Gilmour, J. P. (2007). Multiple scales of genetic connectivity in a brooding coral on isolated reefs following catastrophic bleaching. Mol. Ecol. 16, 771–784. doi: 10.1111/j.1365-294X.2006.03187.x
Wagner, C. E., Keller, I., Wittwer, S., Selz, O. M., Mwaiko, S., Greuter, L., et al. (2013). Genome-wide RAD sequence data provide unprecedented resolution of species boundaries and relationships in the Lake Victoria cichlid adaptive radiation. Mol. Ecol. 22, 787–798. doi: 10.1111/mec.12023
Waller, R. G. (2005). “Deep-water Scleractinia (Cnidaria: Anthozoa): current knowledge of reproductive processes” In Cold-Water Corals And Ecosystems, eds A. Freiwald and J. M. Roberts (Springer-Verlag, Berlin Heidelberg), 691–700.
Keywords: precious coral, spatial genetic structure, spatial autocorrelation, conservation, larval dispersal, deep-sea coral
Citation: Takata K, Iwase F, Iguchi A, Yuasa H, Taninaka H, Iwasaki N, Uda K, Suzuki T, Nonaka M, Kikuchi T and Yasuda N (2021) Genome-Wide SNP Data Revealed Notable Spatial Genetic Structure in the Deep-Sea Precious Coral Corallium japonicum. Front. Mar. Sci. 8:667481. doi: 10.3389/fmars.2021.667481
Received: 13 February 2021; Accepted: 19 May 2021;
Published: 24 June 2021.
Edited by:
Tamotsu Oomori, University of the Ryukyus, JapanReviewed by:
Chuya Shinzato, The University of Tokyo, JapanFederica Costantini, University of Bologna, Italy
Copyright © 2021 Takata, Iwase, Iguchi, Yuasa, Taninaka, Iwasaki, Uda, Suzuki, Nonaka, Kikuchi and Yasuda. This is an open-access article distributed under the terms of the Creative Commons Attribution License (CC BY). The use, distribution or reproduction in other forums is permitted, provided the original author(s) and the copyright owner(s) are credited and that the original publication in this journal is cited, in accordance with accepted academic practice. No use, distribution or reproduction is permitted which does not comply with these terms.
*Correspondence: Nina Yasuda, bmluYTI3QGNjLm1peWF6YWtpLXUuYWMuanA=