- 1Division of Marine Science and Conservation, Nicholas School of the Environment, Duke University, Beaufort, NC, United States
- 2Australian Research Council Centre of Excellence for Coral Reef Studies, James Cook University, Townsville, QLD, Australia
- 3Association of Responsible Krill Harvesting Companies (ARK), Toronto, ON, Canada
- 4Ocean Environmental Ltd., Wellington, New Zealand
- 5Antarctic Ecosystem Research Division, Southwest Fisheries Science Center, National Marine Fisheries Service, National Oceanic and Atmospheric Administration, La Jolla, CA, United States
- 6Black Bawks Data Science Ltd., Invergarry, United Kingdom
- 7Aker BioMarine, Oslo, Norway
- 8Alfred-Wegener-Institut, Helmholtz-Zentrum für Polar und Meeresforschung, Bremerhaven, Germany
- 9Helmholtz Institute for Functional Marine Biodiversity at the University Oldenburg (HIFMB), Oldenburg, Germany
- 10British Antarctic Survey, Cambridge, United Kingdom
- 11Australian Antarctic Division, Department of Agriculture, Water and the Environment, Kingston, TAS, Australia
- 12School of Environmental Studies, University of Victoria, Victoria, BC, Canada
As the global environmental crisis grows in scale and complexity, conservation professionals and policymakers are increasingly called upon to make decisions despite high levels of uncertainty, limited resources, and insufficient data. Global efforts to protect biodiversity in areas beyond national jurisdiction require substantial international cooperation and negotiation, both of which are characterized by unpredictability and high levels of uncertainty. Here we build on recent studies to adapt forecasting techniques from the fields of hazard prediction, risk assessment, and intelligence analysis to forecast the likelihood of marine protected area (MPA) designation in the Southern Ocean. We used two questionnaires, feedback, and a discussion round in a Delphi-style format expert elicitation to obtain forecasts, and collected data on specific biophysical, socioeconomic, geopolitical, and scientific factors to assess how they shape and influence these forecasts. We found that areas further north along the Western Antarctic Peninsula were considered to be less likely to be designated than areas further south, and that geopolitical factors, such as global politics or events, and socioeconomic factors, such as the presence of fisheries, were the key determinants of whether an area was predicted to be more or less likely to be designated as an MPA. Forecasting techniques can be used to inform protected area design, negotiation, and implementation in highly politicized situations where data is lacking by aiding with spatial prioritization, targeting scarce resources, and predicting the success of various spatial arrangements, interventions, or courses of action.
Introduction
In recent decades, the overexploitation of resources, habitat degradation and loss, and a rapidly changing climate have contributed to a precipitous decline in global marine biodiversity (Doney et al., 2012; Halpern et al., 2015; Kroodsma et al., 2018; IPBES, 2019). In response, the establishment of marine protected areas (MPAs) has been encouraged to combat these threats (Gaines et al., 2010; Spalding et al., 2013; Watson et al., 2014; Bell et al., 2018). For example, the Zero Draft of the Post-2020 Global Biodiversity Framework has recommended that countries use “protected areas and other effective area-based conservation measures” to protect “at least 30% of land and sea areas” by 2030 (CBD, 2020).
To aid in reaching these targets and national or regional priorities, scientists and conservation practitioners have used systematic conservation planning methods to guide engagement with stakeholders, prioritize key biodiversity areas, and allocate scarce resources (Margules and Pressey, 2000; Pressey and Bottrill, 2009; Groves and Game, 2015). However, the results of these approaches are commonly constrained because many planning exercises are characterized by high levels of uncertainty about the socio-political system and lack sufficient or reliable spatial/ecological data (Martin et al., 2012; McBride et al., 2012; Sutherland and Burgman, 2015). In situations such as these, expert elicitation can be used to inform protected area design and conservation decision-making in general (Cook et al., 2010; Martin et al., 2012; Sutherland et al., 2011; Wintle et al., 2018). Expert elicitation is the collection of expert knowledge, which has been defined as “substantive information on a particular topic that is not widely known by others” (Martin et al., 2012).
Expert elicitation has a long history of successful application in fields such as intelligence analysis, public health, engineering, and disaster preparedness (O’Hagan et al., 2006; Burgman et al., 2011b; Ungar et al., 2012) and has been increasingly crucial for environmental management and the success of many large-scale conservation assessments such as the IUCN Red List and IPCC Reports (O’Hagan et al., 2006; Mastrandrea et al., 2010; IUCN, 2012, 2016). To date, scientists have employed expert elicitation to assess the current state of the marine environment and cumulative anthropogenic impacts (Ward, 2014; Giakoumi et al., 2015), to assess the threats facing endangered species (Donlan et al., 2010; Wilcox et al., 2016), to parameterize Bayesian models (Choy et al., 2009; Kuhnert et al., 2010; Krueger et al., 2012a), and to collaboratively identify conservation research priorities and/or emerging issues (Kennicutt et al., 2014; Wildermann et al., 2018; Wintle et al., 2018).
Forecasting is one form of structured expert elicitation used to predict possible future outcomes that is increasingly relied upon by environmental scientists to inform conservation planning and natural resource management (Armstrong, 2001; Krueger et al., 2012b; Martin et al., 2012; O’Hagan, 2019). Forecasting methods can be used to obtain expert knowledge or judgments about uncertain quantities or events in probabilistic form (O’Hagan et al., 2006). Forecasting techniques have not, to our knowledge, been previously used to prioritize geographic areas for conservation, estimate the likelihood of MPA designation, or inform international environmental negotiations. Our study addresses this gap in the literature and uses forecasting techniques (expert-based predictions) to elicit single-event probabilities, i.e., the likelihood of occurrence, for MPA designation (from this point on, we use the terms “predicting” and “estimating” interchangeably). In doing so, we build on early systematic conservation planning frameworks (Margules and Pressey, 2000; Groves et al., 2002; Pressey and Bottrill, 2009) and attempt to pursue our research objectives in a way that complements parallel efforts to explore and operationalize the concepts of feasibility (Mills et al., 2013; Tulloch et al., 2014; Jones et al., 2018), social acceptability (Klein et al., 2008; Adams et al., 2011), social vulnerability (Thiault et al., 2017, 2018, 2019, 2021; Williamson et al., 2018), and uncertainty (Regan et al., 2002; Burgman, 2005; Halpern et al., 2006; Lechner et al., 2014; McCarthy et al., 2011).
Our specific elicitation focuses on predicting the likelihood of designating additional no-take MPAs [in the Southern Ocean context, no-take MPAs are referred to as General Protection Zones (GPZs)] along the Western Antarctic Peninsula and examining the relative influence of various biophysical, socioeconomic, geopolitical, and scientific factors in shaping those forecasts. We chose to investigate these factors’ comparative influence because research has routinely demonstrated that socioeconomic and political factors influence conservation outcomes and vice versa (Ban et al., 2019; Naidoo et al., 2019; Cinner et al., 2020). As a more general methodological contribution, we describe data collection methods for conservation scientists who may be considering using probabilistic forecasts to inform conservation planning efforts. Therefore, the three main objectives of this research were to:
1) Use expert judgement to forecast the likelihood that various geographic areas along the Western Antarctic Peninsula will be designated as no-take MPAs (GPZs);
2) Assess the importance of biophysical, socioeconomic, geopolitical, and scientific factors underpinning expert forecasts; and
3) Measure the relative influence or strength of these factors on the estimated likelihood of MPA designation.
By pursuing these objectives, we assess the feasibility of using forecasting techniques to inform conservation planning, decision-making, and ongoing negotiations over expanding the existing network of MPAs in the Southern Ocean (Coetzee et al., 2017; Sykora-Bodie and Morrison, 2019; Brooks et al., 2020). Although forecasting techniques are not a substitute for traditional site selection algorithms and spatial prioritization methods, they can be used to supplement them. For example, forecasting methods can be used to inform the design of MPA proposals by: (1) identifying additional priority conservation areas missed due to gaps in spatial data; (2) assessing their relative social, economic, and political acceptability to decision-makers; and (3) providing insights into how these factors shape broader perceptions of acceptability. Additionally, we seek to show how forecasting techniques can provide insights into situations characterized by high levels of uncertainty and unpredictability such as multi-national conservation negotiations (O’Hagan et al., 2006; Ungar et al., 2012).
Methods and Data Analysis
Case Study
Our geographic focus is on the Western Antarctic Peninsula in the Southern Ocean, which is managed under the auspices of the Convention on/Commission for the Conservation of Antarctic Marine Living Resources (CCAMLR) entrusted with “safeguarding the environment and protecting the integrity of the ecosystem of seas surrounding Antarctica” (CCAMLR, 1980). The Southern Ocean surrounding Antarctica is a highly biodiverse ecosystem and plays a key role in regulating the earth’s climate (Doney et al., 2012; Constable et al., 2014; Rintoul, 2018). A growing tourism industry, expanding fisheries, and a rapidly changing climate are increasingly threatening this system that has remained relatively intact and unimpacted by human activity as compared to other global marine ecosystems (Ballance et al., 2006; Chown et al., 2015; Halpern et al., 2015).
The CAMLR Convention entered into force on April 7th, 1982 and established a consensus-based decision-making process by which CCAMLR implements a system of precautionary, ecosystem-based management and explicitly states in Article II (1) that the primary “objective of this Convention is the conservation of Antarctic marine living resources” (CCAMLR, 1980; Fabra and Gascón, 2008; Cordonnery et al., 2015). CCAMLR has long been considered unique among international environmental agreements due to its cooperative, consensus-based negotiating process, its early emphasis on ecosystem-based management (as opposed to the single-species management models common among regional fisheries management organizations), and its precautionary approach to decision-making that was established due to the region’s remoteness and vast scale, and a commitment to the idea that a lack of data should not preclude taking action (Constable et al., 2000; Parkes, 2000; Miller and Slicer, 2014; Everson, 2015; Wenzel et al., 2016). CCAMLR is also frequently cited as a leader in high-seas conservation due to its successful efforts to reduce fishery bycatch, particularly of seabirds, the development of a Catch Documentation Scheme to combat illegal, unreported, and unregulated (IUU) fishing, the establishment of the CCAMLR Ecosystem Monitoring Program (CEMP), and a set of standards meant to systematize ecosystem monitoring throughout the Convention Area (Cullis-Suzuki and Pauly, 2010; Miller, 2011; Everson, 2015).
The Commission has also sought to designate a representative network of MPAs in the Southern Ocean to help achieve the objectives of the Convention by: (1) protecting a representative samples of ecosystems, biodiversity, and habitats at appropriate scales; (2) protecting key ecosystem processes; (3) protecting areas vulnerable to human impact; (4) protecting features critical to the function of local ecosystems; (5) establishing scientific reference areas; and (6) maintaining resilience to the effects of climate change (CCAMLR, 2011). The Commission first outlined these principles in Conservation Measure (CM) 91-04, a “General framework for the establishment of CCAMLR Marine Protected Areas,” which standardized the process by which Members would propose, negotiate, and designate new MPAs within the Southern Ocean (Fabra and Gascón, 2008; CCAMLR, 2011; Everson, 2015). To date, CCAMLR has established two MPAs within the Convention Area—the South Orkney Islands Southern Shelf MPA (SOISSMPA) in 2009 and the Ross Sea region MPA (RSRMPA) in 2016 (CCAMLR, 2009, 2016). Additional MPAs have been proposed in East Antarctica by Australia, the European Union and its Member States, New Zealand, Norway, the United States, and Uruguay, in the Weddell Sea by Australia, the European Union and its Member States, New Zealand, Norway, the United States, and Uruguay, and in the Domain 1 planning area along the Western Antarctic Peninsula (Figure 1) by Argentina and Chile (Sykora-Bodie and Morrison, 2019; Brooks et al., 2020; Delegations of Argentina and Chile, 2020).
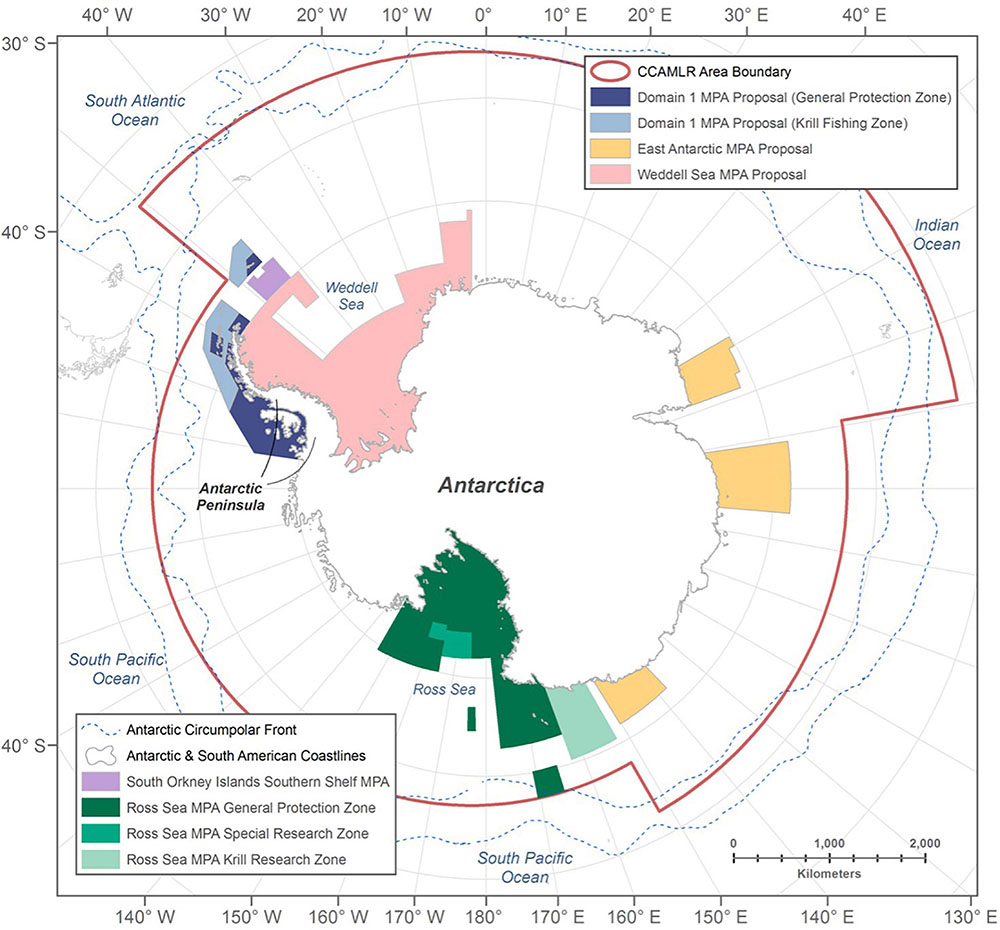
Figure 1. The area managed by the Convention on the Conservation of Antarctic Marine Living Resources (CCAMLR) – The shaded areas show existing and proposed marine protected areas, including the proposed Domain 1 MPA (shown in blue). The Ross Sea region MPA’s Krill Research Zone and the Special Research Zone permit limited fishing of krill and fish for scientific research purposes, while the General Protection Zone and entire South Orkney Islands Southern Shelf MPA prohibit commercial fishing.
The process for establishing an MPA in the Southern Ocean begins with the development of a proposal by the sponsoring nations. Once drafted, this proposal is then formally submitted to CCAMLR’s Scientific Committee (SC), which reviews the work to ensure that it is based upon the best available science. The SC then decides to either recommend further work on the proposal or determines it does represent the best available science and formally submits the proposal to the Commission for its consideration and potential adoption. Because CCAMLR operates as a consensus-based decision-making body, and Article XII states that all “matters of substance shall be taken by consensus,” adoption in effect requires the absence of any objections from signatory states (CCAMLR, 1980). Throughout this process, we see two main stages when forecasts can be complementary and informative: (1) during the proponents’ initial development of the proposal when forecasts can be employed to parameterize and refine the results of spatial optimization tools or to otherwise inform discussions about the spatial configuration of MPA proposals; and (2) during the Commission’s deliberations, when forecasts can help to prioritize objectives and provide insights into the various (sometimes implicit) social, economic, and political barriers and opportunities underpinning decision-making.
Structured Expert Elicitation Protocol
We used a Delphi-style format that relied on two elicitation rounds [investigate (1); estimate (3)] and one discussion round [Discuss (2); Figure 2] to obtain quantitative forecasts and gather data on the underlying factors that influenced experts’ estimates of the likelihood that specific geographic areas will be designated as no-take MPAs within the next eight years1 (MacMillan and Marshall, 2006; Martin et al., 2012; Hemming et al., 2017). The purpose of a Delphi-style approach is to reduce some of the common biases associated with eliciting information from individuals and groups (e.g., anchoring or overconfidence) and to provide participants with the opportunity to consider their colleagues’ estimates (and their underlying rationale) and then reconsider or revise their own forecasts (MacMillan and Marshall, 2006; O’Hagan et al., 2006; Hemming et al., 2018).
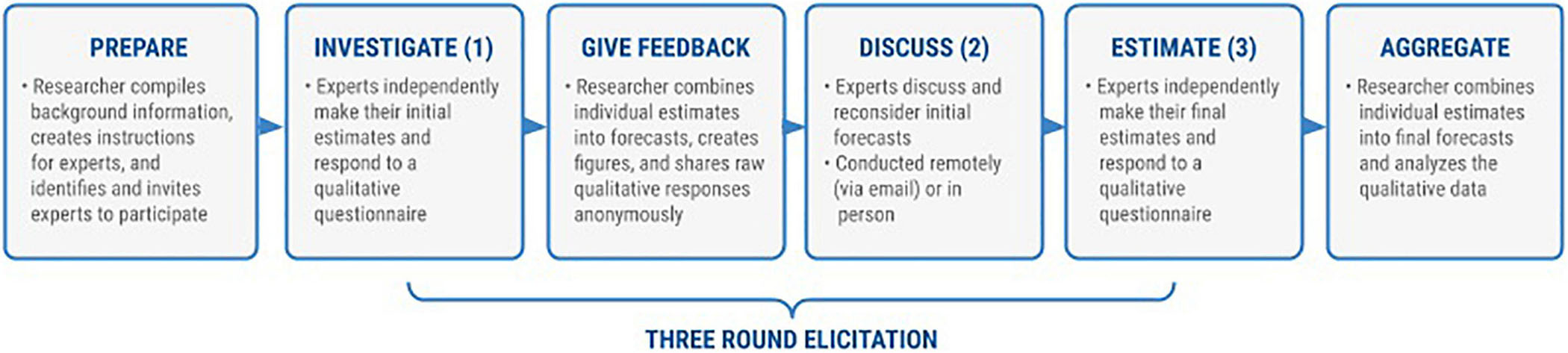
Figure 2. The elicitation format – The Delphi format elicitation consisted of a preparation stage, the three-round elicitation (numbered 1, 2, 3), and final data aggregation and analysis. Quantitative and qualitative data were collected during steps one and three.
For this elicitation, we selected experts (also referred to as “participants”) based on their membership in the Domain 1 MPA expert working group that consisted of 29 individuals from various CCAMLR member countries. Then, we solicited input from members of the Domain 1 MPA expert working group to refine our list of experts to be invited as participants in the elicitation process. To do this, we first identified and removed from our initial list those individuals who were perceived to be inactive in group discussions, meetings, and the planning/advising process in general. Second, we used snowball and triangulation techniques to identify other individuals who were not officially part of the Domain 1 MPA expert working group, but who were perceived by the other participants to be highly knowledgeable about, or able to influence, the process of developing the Domain 1 MPA proposal. The final list consisted of 25 individuals from 14 delegations who we invited to participate with a short project description and confidentiality statement/consent agreement (Duke University IRB #2018-0072). Participants included diplomats, independent scientists, academics, and scientists associated with their countries’ national Antarctic research programs. Although our participation rate was affected by the COVID-19 pandemic and several individuals were unable to take part in the study due to personal circumstances, our invitation to participate was accepted by ten individuals bringing perspectives from Australia, Germany, New Zealand, the United Kingdom, and the United States, and the Association of Responsible Krill harvesting companies (ARK; industry) and Oceanites (penguin conservation) delegations. Many of these individuals are quoted throughout this paper, but their names have not been included because they participated under an agreement that their comments would remain anonymous.
We selected ten geographic areas along the Western Antarctic Peninsula (Figure 3) to develop our forecasts. We did this by relying on spatial data that were collected for an expert elicitation in which participants identified areas they thought to be in need of protection or areas where experts believed there to be opportunities for designating MPAs along the Western Antarctic Peninsula (Sykora-Bodie et al., 2021). That project used ArcGIS 10.6.4 to combine 100+ expert elicited polygons and overlayed them with a hexagonal planning mesh to create hotspot maps and conduct additional spatial analyses. Based on these data, we identified spatial clusters, combined the hexagons, and smoothed the outer boundaries to create our geographic areas. This resulted in thirteen clusters, which we reduced to ten to avoid participant burnout (Fowler, 2013; National Academies, 2016). The ten sites used in this study were selected because they substantially overlap with areas included in the proposed Domain 1 MPA but differed enough that we were not directly commenting on the proposal itself, which could have interfered with ongoing negotiations. These ten sites were also selected to represent geographic diversity and a variety of human activities and natural environments, thus providing relevant examples of the type of areas that could be designated in actual negotiations over the proposed Domain 1 MPA.
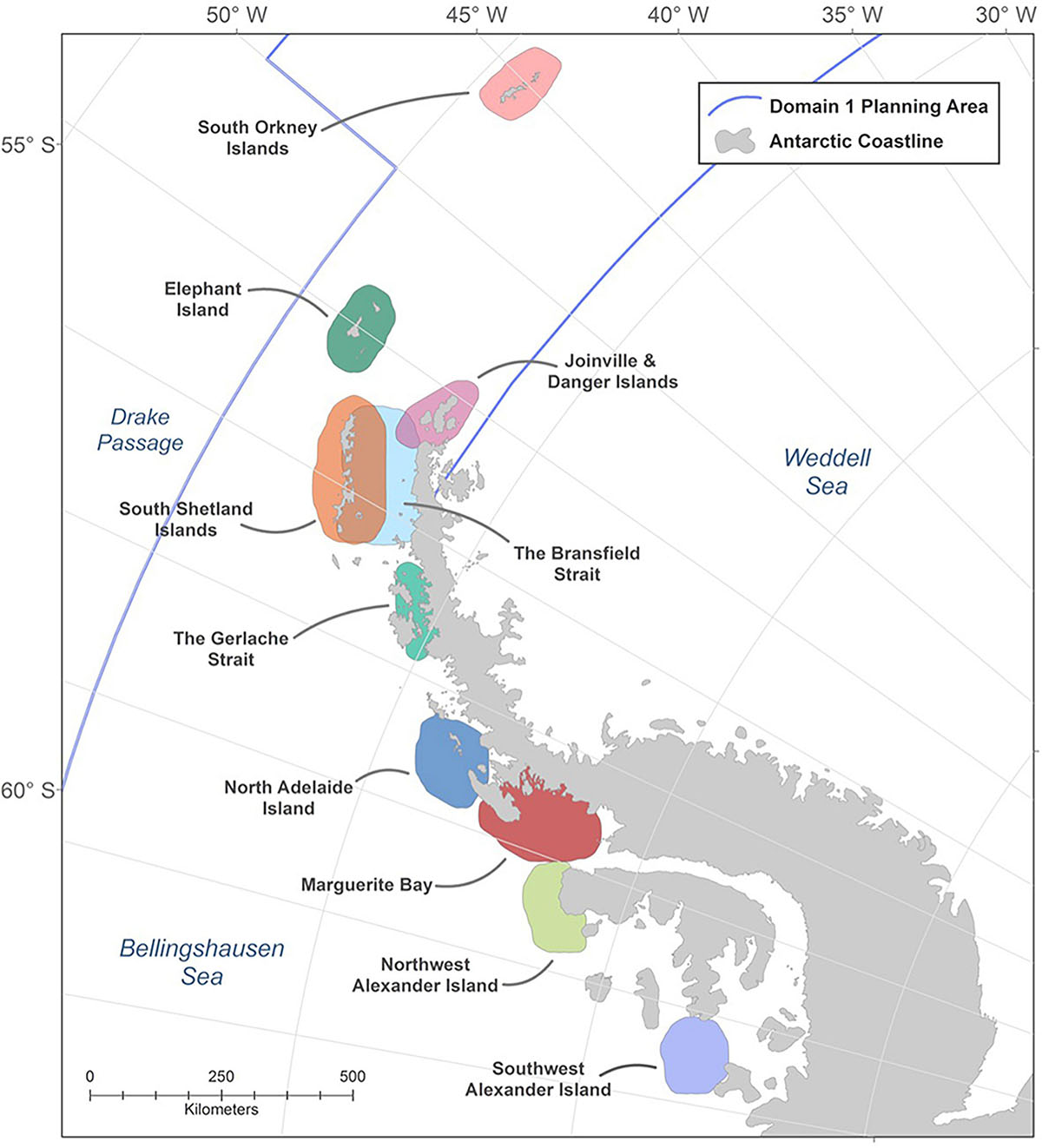
Figure 3. Geographic areas considered in the forecasting elicitation – This area along the Western Antarctic Peninsula is managed by the Commission for the Conservation of Antarctic Marine Living Resources (CCAMLR). The blue line shows the boundary of the Domain 1 planning area and the shaded polygons show the ten geographic areas that were presented to experts during the elicitation as notional MPA areas. These ten areas were based on earlier participatory mapping research (see Sykora-Bodie et al., 2021) and were selected to reflect the wide range of areas currently under consideration for designation by CCAMLR.
We used a questionnaire (Supplementary Appendix A) and the Qualtrics data collection and management platform to conduct a remote elicitation that collected quantitative forecasts, identified drivers, and gauged their relative strength (Bernard, 2011; Rolstad et al., 2011; Fowler, 2013). We structured our elicitation according to the IDEA Protocol (“Investigate,” “Discuss,” “Estimate,” “Aggregate”; Figure 2), which is designed to elicit low, high, and best probabilistic estimates between 0 and 100% (Hanea et al., 2016; Hemming et al., 2018). We selected this format because previous studies have shown that it substantially reduces overconfidence when forming probabilistic estimates (Speirs-Bridge et al., 2010).
In the first elicitation round (“Investigate”), we used our questionnaire to present experts with a list of 21 pre-identified factors (shown in Table 1A of the Results section) that could shape participants’ opinions and CCAMLR negotiations, and ultimately influence whether an MPA is designated by CCAMLR. These factors were previously identified by Sykora-Bodie and Morrison (2019), who used interviews and document analysis to identify a comprehensive list that influenced the 2016 designation of the Ross Sea Region MPA. This list of factors was further refined and used during a participatory mapping elicitation by Sykora-Bodie et al. (2021) to structure the collection of associated qualitative attribute data. In the first round, experts reviewed and confirmed that the list of pre-identified factors was comprehensive, and no additional factors were added.
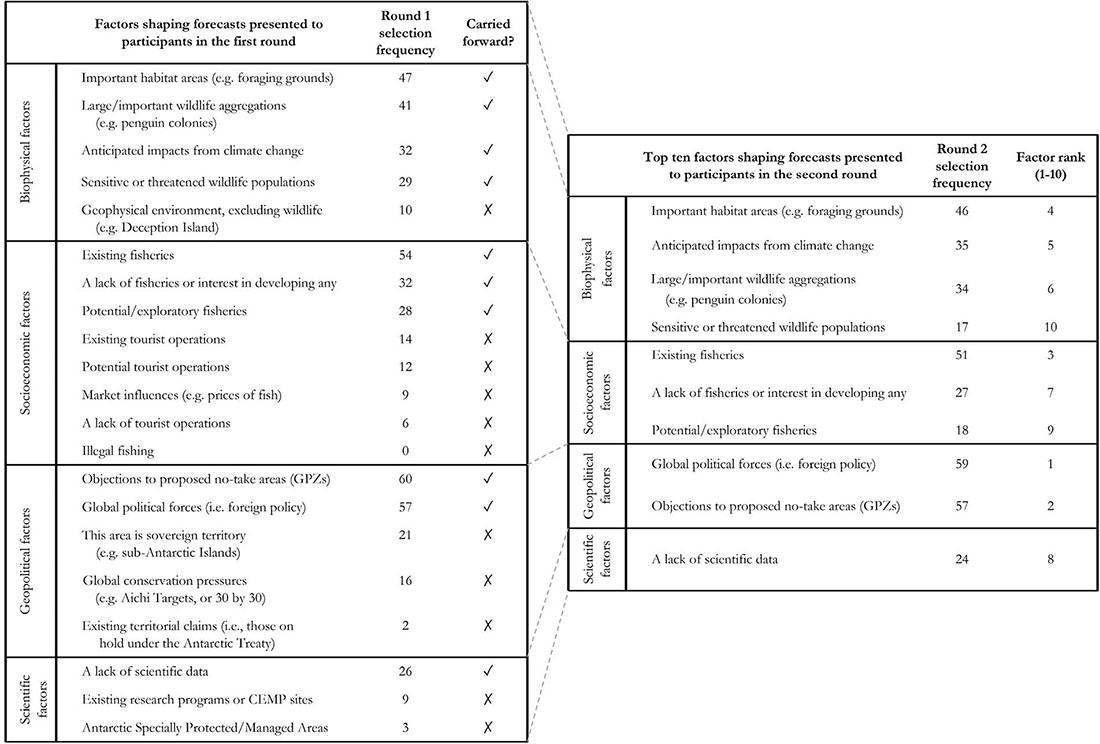
Table 1. First round selection frequency of factors influencing quantitative forecasts – The factors below were presented to experts based on prior research (Sykora-Bodie and Morrison, 2019; Sykora-Bodie et al., 2021).
In preparation for round two (“Discuss”), we summarized the first round of quantitative estimates and qualitative responses and presented them to the experts. They in turn directly corresponded with each other via email to explain the reasoning behind some of their forecasts and respond to other individuals’ explanations and comments. The text of this discussion was qualitatively analyzed to assess the importance of various factors on the forecasts (research objective 2) and to measure their relative influence (research objective 3).
For our third round, we carried forward the ten factors most commonly selected by experts and asked them to choose at least one and up to five factors that influenced their estimates (Supplementary Appendix A, Question 2). We also asked experts two open-ended questions about (1) why they changed their forecasts (if they did so) between the first and second questionnaires, and (2) which factors influenced their forecasts the most. Changes to forecasts between rounds 1 and 2 were minor, but — as expected — they generally converged closer to the group average, and participants highlighted perspectives shared by others as the primary motivations for changing their quantitative forecasts.
Data Analysis
Quantitative Probability Forecasts
To forecast the likelihood of MPA designation within the next eight years (research objective 1), we imported experts’ individualforecasts from the questionnaires into the statistical analysis program R 3.6.3 (and RStudio 1.2.5) for data organization and cleaning. We calculated three arithmetic means for experts’ low, best, and high scores, and combined them into credible interval forecasts (lowest forecast to highest forecast) for each of the ten areas under consideration along the Western Antarctic Peninsula (O’Leary et al., 2009; Speirs-Bridge et al., 2010; Hemming et al., 2017). We created boxplots for the “best” forecast scores to visualize the variation in experts’ responses and calculated the mean and standard deviation (Supplementary Appendix C). We ranked the ten most influential factors identified in round three by selection frequency to gain insight into how much influence each exerts on forecasted outcomes (Supplementary Appendix A, question 3).
Qualitative Data
To answer our second (describing the importance of influential factors) and third (to measure and/or quantify their relative strength) research objectives, we coded and analyzed the responses from the questionnaire and the round two email discussion in the qualitative data analysis software QSR NVivo 12.6.0. We used a pre-determined qualitative coding structure (Supplementary Appendix B) that was based on earlier research that identified the key factors that influence negotiated conservation outcomes at CCAMLR meetings (Sykora-Bodie and Morrison, 2019). There were 21 of these factors, which were organized into four main categories of drivers—biophysical, socioeconomic, geopolitical, and scientific—which we used as the primary organizational lens for our analysis and reporting in later sections. We built upon this research and used this coding structure to organize experts’ responses and identify key patterns and themes throughout the data (Supplementary Appendix B). After coding our data, we reviewed and synthesized each category to better understand the drivers influencing their forecasts, as well as the relative weight that each of the four categories was exerting on those forecasts.
Results
Forecasting the Likelihood of MPA Designation
Our results ranged from a mean “best” forecasted likelihood of 18% for the Bransfield Strait to 59% for Marguerite Bay (Figure 4; the dot in the middle of each forecast). These forecasts showed a latitudinal gradient with northern areas of the Western Antarctic Peninsula being perceived to have a lower likelihood of designation than southern areas. As one expert stated during the discussion round, “I think the general broad direction is pretty clear, i.e., a latitudinal gradient where the northern areas are less likely to be included in an MPA network while the southern areas are more likely.” Additionally, this latitudinal gradient was apparent when we compared the range of forecasts provided for each location. Focusing solely on the variation in the “best” forecasts (Supplementary Appendix C), we found that northern areas (e.g., the South Orkney Islands, Joinville and the Danger Islands, and the Gerlache Strait) had wider ranges of estimates on average than southern areas (e.g., North Adelaide Island, Southwest Alexander Island) suggesting that experts thought the presence of more extensive human activity makes the likelihood of designation in this region more unpredictable.
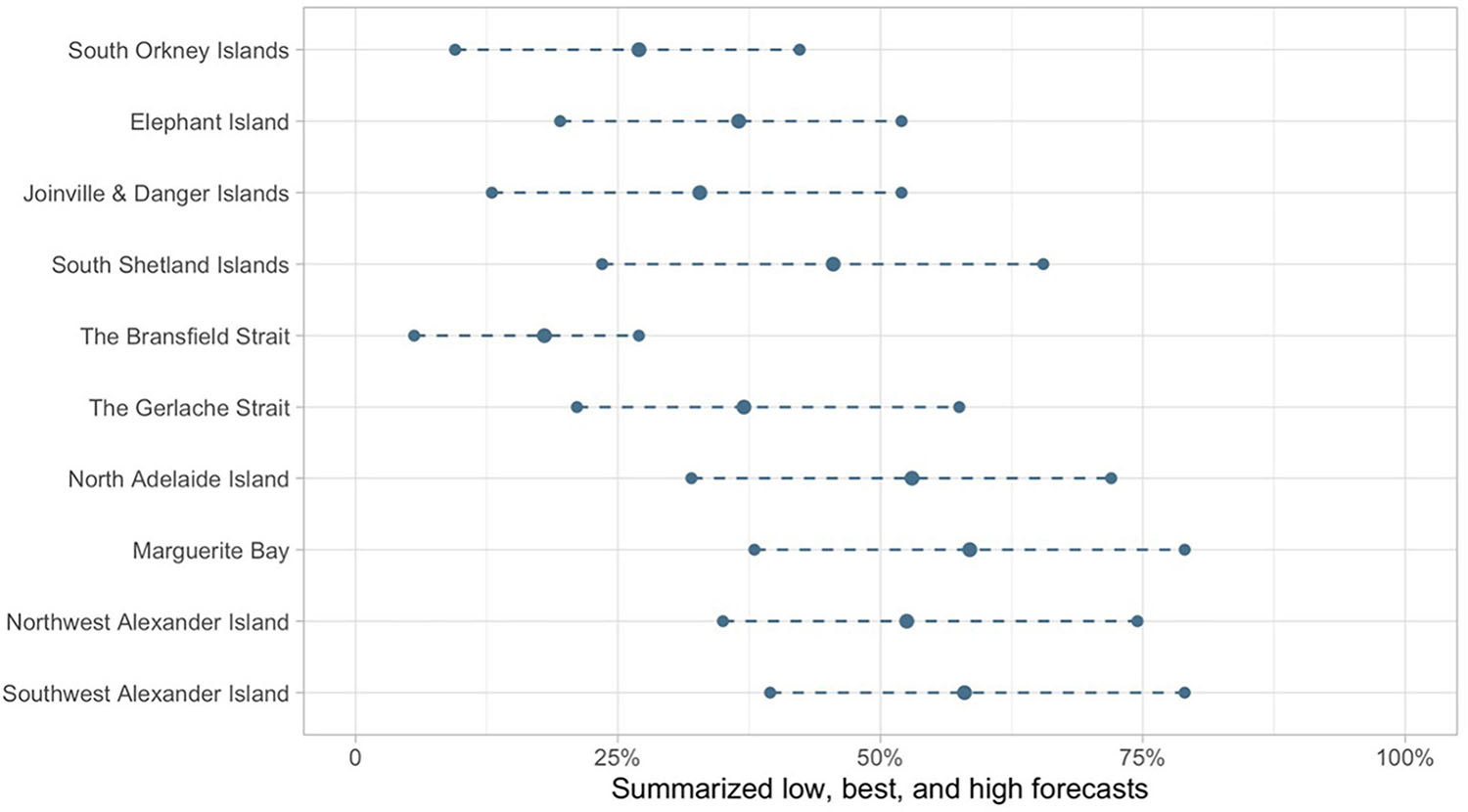
Figure 4. Final quantitative forecasts of the likelihood of MPA designation – These forecasts represent three (low, best, high) separate arithmetic mean estimates of the likelihood that each area “is designated or included within a no-take (e.g., ‘general protection zone’) MPA within the next eight years.” The lower end, dot, and upper end of each line represent the low, best, and high estimates, respectively, rather than a single estimate with two other points representing the extent of its associated confidence interval. Here, the forecasts are arranged latitudinally (north to south) on the y-axis.
When looking at individual locations, three sites were noticeable for various reasons: the Bransfield Strait, the area surrounding the Joinville/Danger Islands, and the Gerlache Strait (qualitative data; Figure 4 and Supplementary Appendix C). The Bransfield Strait stood out because it: (1) did not conform to the overall latitudinal pattern; (2) was considered much less likely to be included within an MPA designation than adjacent locations (e.g., Elephant Island and the South Shetland Islands) due to the presence and density of fishing activity; and (3) had the narrowest “best” estimate range of any location under consideration (Supplementary Appendix C) indicating high agreement between experts.
As for the Joinville/Danger Islands, this area was notable due to experts’ divergent forecasts. On the one hand, some experts provided higher forecasts, which they justified based on the region’s current inaccessibility due to sea ice, the fact that fishing in the area has significantly decreased in recent years, and important aggregations sensitive of wildlife such as Adélie penguins on Heroina Island. On the other, some experts provided lower forecasts, which they justified based on the potential for a changing climate to reduce sea ice coverage and make this area more accessible to fishing vessels.
When we looked solely at the “best” estimates for the Gerlache Strait (Supplementary Appendix C), we found a wider range of forecasts than any other location. The qualitative data indicated that existing fisheries and the complexity of setting aside such a highly trafficked area led some experts to provide lower forecasts, whereas extensive tourism, dense aggregations of whales, and the relatively small geographic size of the area caused other experts to provide higher forecasts. For example, one individual noted that “The second site [the Gerlache Strait] is key for humpback whales, a recovering krill-dependent species. Given the public perception of this species, coupled with the tourist penetration into this area, CCAMLR could achieve a second ‘easier’ win. In the case of the Gerlache, it might not be feasible to argue for year-round protection, but seasonal protection should be feasible. It could be a PR [public relations] coup for CCAMLR.”
Key Factors Influencing Experts’ Forecasts and Their Relative Weight in Determining Successful Outcomes
In the following section, we discuss the ranking of factors influencing the forecasts and the rationale given by expert respondents (research objectives two and three) and organize our reporting by using the four categories of factors identified by Sykora-Bodie and Morrison (2019)—biophysical, socioeconomic, geopolitical, and scientific. We define them as follows:
1) Biophysical—relating to the natural environment or processes, including wildlife, ecosystems, and climate change;
2) Socioeconomic—relating to human activities of a cultural, educational, commercial or economic nature;
3) Geopolitical—relating to international politics including territorial integrity/expansion, security, or conservation; and
4) Scientific—relating to scientific research or the pursuit of knowledge.
Geopolitical Factors
Of the five geopolitical factors presented in the first elicitation round, three were not carried forward into the final elicitation round (Table 1). Territorial claims received only two selections in the first round between all experts and locations, which is unsurprising given that participants may have been unlikely to suggest to researchers and others in the Delphi process that they play a role in negotiations since territorial claims are officially on hold under the terms of the Antarctic Treaty (Antarctic Treaty, 1959).
The two geopolitical factors that were carried forward [“global political forces (i.e., foreign policy related),” and politically motivated “objections to proposed no-take areas (GPZs)”] were identified as being the first and second most important factors shaping experts’ forecasts, respectively (Table 1). This is what we anticipated given the contentious nature of the debate over expanding the network of MPAs (CCAMLR, 2017, 2018) and as one individual stated, “Geopolitics and the need for a win will be the drivers that put this over the top, no science arguments will convince extractive interests to compromise.” Finally, “Objections to proposed no-take areas (GPZs)” was more frequently selected for northern areas (Figure 5, column 8) and the qualitative data showed that this was partially linked to the presence of active fisheries.
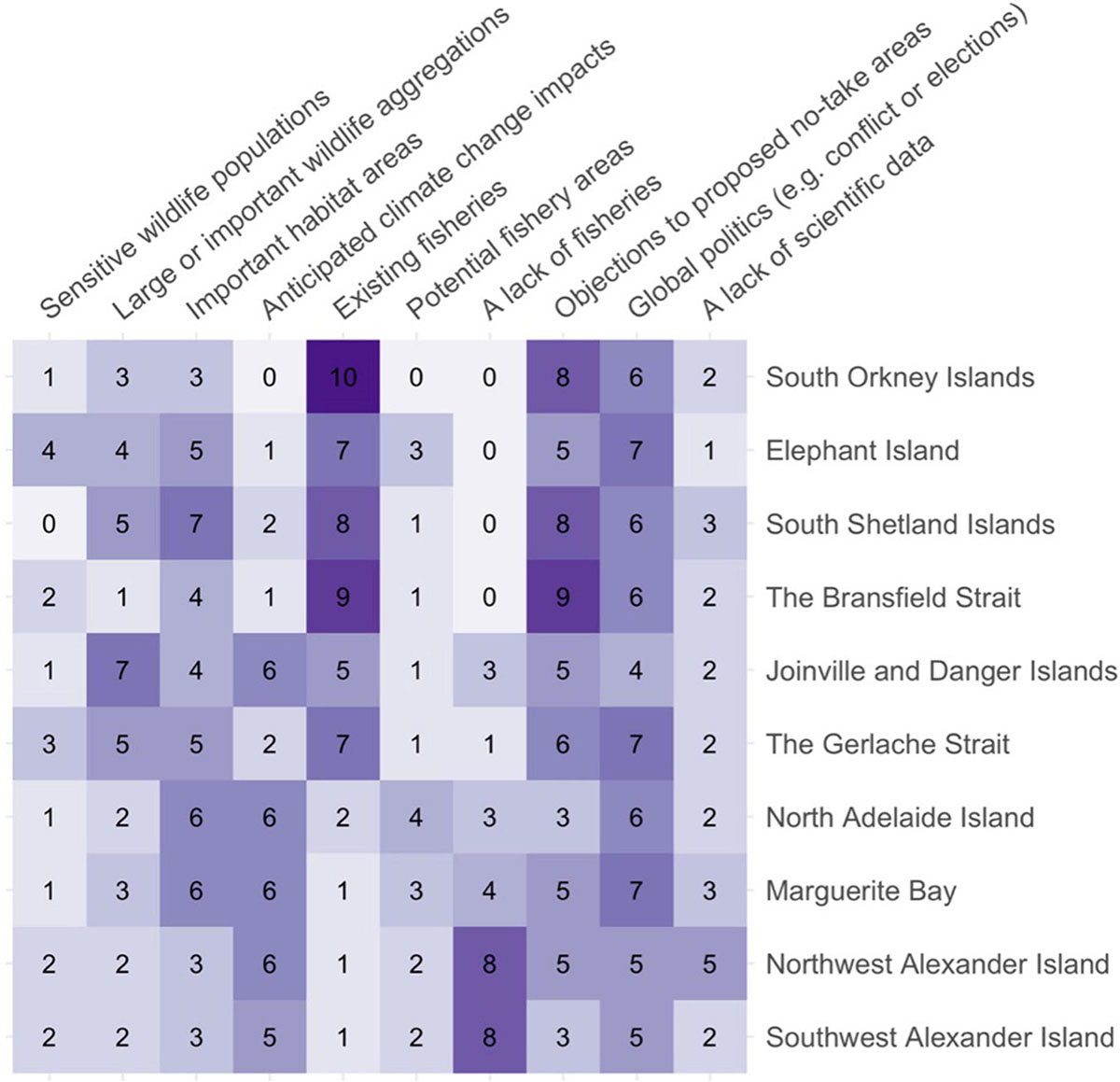
Figure 5. A heatmap of the number of times factors were selected as being influential for each area under consideration. The y-axis is arranged latitudinally, north to south.
Socioeconomic Factors
Experts selected the three socioeconomic factors linked to fishing to carry forward (Table 1) and then ranked them 3rd, 7th, and 9th, respectively (Table 1), with the two lower rankings perhaps resulting from how the factors were worded and structured as exclusive/inverse categories (“existing fisheries” ranked high while “no fisheries” ranked low). This makes sense given that fisheries are currently the sole extractive economic activity taking place in the Southern Ocean and one of the primary objections to MPA proposals has been that they will constrain or displace fisheries, which was also raised during the round two discussion. The qualitative data supported this interpretation with most experts agreeing that areas where fisheries currently operate are much less likely to be designated. Individuals repeatedly highlighted the perceived difficulty of implementing closures in heavily fished areas, as noted in the following statements: “The overlap of several areas with existing or historical krill fishing grounds pose a significant hurdle to adoption as general protection zones”; “Currently, there is no opportunity to declare as an MPA an area with active fishing”; and “All areas with existing fisheries will be extremely difficult to include in a GPZ [general protection zone].”
Areas that were historically more heavily fished also led to divergent opinions (Supplementary Appendix D). Some individuals thought areas where fishing has decreased are more likely to be designated after being abandoned by the industry. For example, certain areas around the South Shetlands, Elephant Island, and Joinville Island have seen less fishing in recent years and might be better candidates for spatial closures than the quantitative forecasts suggest. As one individual stated, “I feel that there is some hope for developing official CCAMLR spatial management in a couple of places [tip of the Peninsula (Joinville and Danger Islands) and the Gerlache]. The first of these sites is not heavily fished and has never been heavily fished, even though it clearly is a location with a lot of krill. The tip of the Peninsula is often ice covered, so inaccessible. Thus, it is an important site for Adélie penguins, but not accessible to the fishery. It should be an easy win.” Alternatively, others noted that localized overfishing, led to decreased fishing activity, which in turn has resulted in stock recovery. This stock recovery may lead to renewed fishing interest around places like the South Shetland Islands.
In combination, the existence of fisheries and political objections to establishing MPAs were the most frequently cited factors influencing experts’ forecasts (Table 1). This was supported with numerous qualitative statements. For example, one individual wrote that “Overall, the major hurdle to designation by the Commission remains their ability to resolve the trade-off between current fishing and geopolitical positions on the one hand versus an MPA’s ability to mitigate climate threats and provide protections for threatened and vulnerable populations on the other.”
Biophysical Factors
Experts frequently cited the importance of biophysical factors in shaping their quantitative forecasts with concerns about “important habitat” ranking 4th, “anticipated impacts from climate change” ranking 5th, “large aggregations of wildlife” ranking 6th, and finally concerns about “sensitive wildlife populations” ranking 10th (Table 1). “Anticipated impacts from climate change” had higher selection frequency further south, which was initially counterintuitive until qualitative data revealed that experts were selecting this factor with the understanding that southern areas of the Peninsula would be more likely to be gazetted as no-take zones given their ability to serve as refugia for species fleeing warming temperatures to the north (Figure 5, column 4). Joinville and the Danger Islands were clear exceptions to this latitudinal trend (Figure 5, column 4) because their unique local oceanographic conditions (cold water intrusion from the Weddell Sea), which led experts to suggest they may serve as cold-water refugia. As one stated, “The tip of the Peninsula is a critical environment, not just for penguins and krill, it is an area that will remain relatively cold (in the water column and on the sea bottom) because of the direct influence of the Weddell Sea. Other areas [at higher latitude WAP (Western Antarctic Peninsula)] are likely to have warmer bottom waters as offshore waters invade the shelf. Therefore, the tip could be… a refuge (or potential refugia) for more highly polar taxa.”
The qualitative responses reinforced that experts perceive specific geographic locations to be more likely to be designated as no-take areas if they harbor large aggregations of wildlife such as Adélie penguin mega-colonies in the Danger Islands (Figure 5, column 2) or seasonal aggregations of foraging whales in the Gerlache Strait. Experts also noted that a location’s contribution to the health and functioning of the broader ecosystem, for example as a krill nursery area around the South Shetland Islands (Figure 5, column 3), make it more likely to be designated in the future. As one individual stated in explaining their forecast suggesting that the Gerlache Strait is more likely than many other areas to be designated, they said that it “harbors important ecosystem processes and large concentrations of marine mammals and could serve as refugia in the event of environmental change.”
Scientific Factors
Only one scientific factor (“a lack of scientific data”) was carried forward into the second round by experts, in which they ranked it 8th overall (Table 1). Qualitative responses were mixed, with some experts saying that resolving scientific knowledge gaps is key to designating additional MPAs, while others suggested that even though gaps do exist, they do not preclude designations and are only a convenient excuse used by opponents to block consensus on proposed MPAs. One of the areas highlighted as an existing knowledge gap or area of scientific uncertainty is around predator-prey dynamics and how krill fisheries in the northern areas of the peninsula may be harming predators. To help reduce some of the uncertainty or solve some of the complexity associated with krill fishery management around specific areas like the Bransfield Strait, CCAMLR is considering implementing a new krill fishery management mechanism. However, nearly all experts agreed that doing so is incredibly complex and that it may be impossible given the current understanding of habitat-krill-predator dynamics along the Western Antarctic Peninsula. The seasonal dynamics of predator-prey interaction led several experts to suggest that seasonal closures would be more appropriate, but they cautioned that it would likely be more difficult to design and implement these types of triggers and rules than permanent spatial closures.
Discussion
Many conservation planning efforts are characterized by high levels of uncertainty and a lack of sufficient spatial/ecological data (Martin et al., 2012; McBride et al., 2012; Sutherland and Burgman, 2015). In these instances, forecasting techniques can be used to inform efforts such as MPA planning. Our research sought to fill this gap in the literature in ways that are both broadly theoretical/methodological and narrowly applied/case specific. From a theoretical/methodological standpoint, we presented data collection methods and a roadmap for conservation scientists who may be considering how to use probabilistic judgements to support various types of environmental decision-making such as spatial prioritization or allocation of management resources. From an applied standpoint, our elicitation focuses on predicting the likelihood of designating additional no-take MPAs along the Western Antarctic Peninsula and examining the relative influence of various biophysical, socioeconomic, geopolitical, and scientific factors in shaping those forecasts and serving as barriers or opportunities to further action.
Quantitative Forecasts
Because this is the first use of forecasting techniques to prioritize spaces and predict their likelihood of designation (in our case varying between 18 and 59%), we lack relevant comparative examples to help interpret their magnitude in the forecasts (should they be considered high or low?). Forecasted values are context dependent and will be interpreted differently depending on the arena. For example, a forecast that a little over 60% of marine turtles entangled in fishing lines, nets, or traps will perish may be considered high by marine turtle experts and conservation practitioners (Wilcox et al., 2016), but this example does not suggest how we should interpret our forecasts.
It is also difficult to provide context for our forecasts because proponents of the Domain 1 MPA may interpret these numbers positively and point to them as evidence that those involved in the process believe certain locations are more likely than not to be designated (North Adelaide Island, Marguerite Bay, and Northwest and Southwest Alexander Island). Similarly, though, opponents may feel positively about the fact that even these four highest forecasts are between 50% and 60%. In short, these numbers should be interpreted with care and within the constrained context of the study, and we caution against their direct use to support or oppose specific proposals. However, much of the “value” of these forecasts stems from the fact that they show a collective estimate that is stronger than anecdotal evidence. Forecasting can be used to inform negotiating strategies and future iterations of MPA proposals, and they provide insights into each area’s relative likelihood of designation. On this last point, we mean that they suggest CCAMLR members are more likely to include Marguerite Bay in a future designation than the Bransfield Strait or, alternatively, that protecting the Bransfield Strait would require significantly more negotiating effort.
Drivers of Perceived Outcomes and the Relative Strength of Various Factors
Our results highlighted the key role that geopolitical and socioeconomic factors play in shaping MPA boundaries and perceptions of the likelihood of designation, which, ultimately, further influence negotiations to designate MPAs within our study context (Supplementary Appendix D). This finding is consistent with literature on conservation planning literature (Walmsley and White, 2003; Pollnac et al., 2010; Giakoumi et al., 2011; Rossiter and Levine, 2014; Gurney et al., 2015) and Antarctica (Dodds and Hemmings, 2013; Hodgson-Johnston, 2015; Bray, 2020).
The qualitative data that we collected from experts highlighted the role of socioeconomic interests and the interplay between them and geopolitical factors. As one individual noted, and others echoed in similar comments, “It is clear that the most important factor in determining whether a CCAMLR MPA has a chance of being adopted is the [presence or absence of a] fishery.” This emphasizes the common sentiment among many experts that fisheries are one of the primary barriers impeding the further evolution of a CCAMLR ecosystem-based management regime (i.e., the establishment of a representative network of MPAs) that is consistent with the Convention’s primary objective of achieving “the conservation of Antarctic marine living resources” (CCAMLR, 1980; Miller and Slicer, 2014; Everson, 2015; Liu and Brooks, 2018).
Geographic/Spatial Patterns
Additionally, the strong latitudinal gradient indicating that southern areas are more likely to be designated than northern areas (Figures 3, 4) mimics the recent evolution of the Domain 1 MPA proposal. The preliminary 2017 Domain 1 MPA proposal contained more no-take ‘General Protection Zones’ to the north, but following objections from opponents, these were shifted further south in the 2018/2019 proposals (Delegations of Argentina and Chile, 2017, 2019) to areas that are similarly ecologically valuable but also perceived to be more politically acceptable to opponents (Brown et al., 2019; Sykora-Bodie et al., 2021). To what extent access to fisheries, geopolitical objections, and the potential benefit of southern areas as “climate refugia” (Supplementary Appendix D) have each influenced these revisions is unknown, but they collectively motivate opponents’ objections, and have therefore contributed to the proposal’s revision (CCAMLR, 2017, 2018).
Although it is possible that the evolution of the proposal influenced experts’ responses during the elicitation, we do not believe this to be the case for several reasons. First, these individuals are not drawing the boundaries for the proposal themselves, but rather providing data, serving as conduits to their national delegations, and commenting or advising on the work of the Argentinean and Chilean Domain 1 proposal planning team. Additionally, the shift of the proposal to cover more southern areas is not being driven internally by members of the planning team, but externally, by CCAMLR members wishing to protect access to existing commercial fishing grounds located further north.
Conservation Implications
Although spatially referenced ecological data remains the foundation of protected area design, this paper illustrates how forecasting techniques can complement these data, site selection algorithms, and spatial prioritization methods by accounting for and incorporating additional social, economic, and political considerations. Forecasting methods can inform protected area planning and decision making similar to how fisheries data, social-ecological vulnerability mapping, and spatially referenced social, economic, and political considerations have been used to identify areas that are more or less likely to have unacceptable socioeconomic impacts on local communities and resource users or to identify socio-political opportunities (Guerrero and Wilson, 2016; Thiault et al., 2017; Sykora-Bodie et al., 2021). In the case of the Southern Ocean, for example, these data can be used to identify areas of conservation importance that diplomats could prioritize for achieving consensus sooner, while also identifying areas that may require additional information or discussions to successfully designate. In this case, a multi-stage approach could be taken, where consideration of the more controversial areas is delayed while more focused discussion or research occurs as has happened with the proposed Weddell Sea MPA, and as was relied upon to extend the Heard Island and McDonald Islands MPA after it was originally designated (Welsford et al., 2011). Although this is not the ideal approach for selecting areas to set aside for conservation purposes, MPA designation is a political act that alters stakeholders’ access to and control over resources. As a result, we may as well accept the often overtly political nature of the process (e.g., the inclusion of the Krill Research Zone in the RSRMPA to gain Chinese support) and adapt planning processes to incorporate additional considerations that are important from a political perspective.
One concern for conservation planners is that although forecasts can inform MPA site prioritization, they have the potential to inadvertently undermine conservation efforts if not used cautiously. By identifying and highlighting areas perceived to be more socially, economically, and/or politically acceptable, forecasts may incentivize the designation of areas that provide few (if any) conservation benefits. These “residual reserves” occur when planners prioritize minimizing opportunity costs to humans and fail to separate or protect biodiversity from the human activities threatening its persistence (Devillers et al., 2014; Pressey et al., 2015). As a result, we must be clear that forecasts are not a substitute for ecological or socioeconomic data or a precautionary approach, and they should not be used as the primary method for identifying high-priority conservation areas for decision-makers to designate as MPAs. Rather, forecasting techniques are most useful when they are used to inform discussions and to supplement traditional site selection algorithms and spatial prioritization methods (Guerrero and Wilson, 2016; Thiault et al., 2017; Sykora-Bodie et al., 2021). In particular, we see two times during the broader conservation planning process that forecasting techniques can best inform decision-making: (1) during the proposal and negotiation phase to prioritize sites for inclusion (as explained above); and, not included in our study, (2) after designation, to help managers allocate resources for monitoring and enforcement, prioritize management interventions such as fire management or removing invasive species, or predict the likelihood of the successful application of these interventions.
Finally, regardless of the specific context, the conservation planning literature encourages more effective stakeholder participation and suggests the planning process itself is critical for sharing knowledge and building consensus (Pressey and Bottrill, 2009; Gleason et al., 2010; Groves and Game, 2015). In the case of the Southern Ocean, MPA proponents can (and are, in the case of the Domain 1 planning process) use a collaborative conservation planning process to strategically engage various stakeholders, build consensus, and advocate for preferred outcomes. Forecasting techniques can be similarly used as a consensus-building tool by providing a forum for stakeholder discussion.
Limitations of the Study
Consistent with guidance on expert elicitation processes, we assembled an adequate group of experts, in terms of size and diversity that represented the range of delegations and perspectives relevant to the topic. However, including the views of some additional key member countries (e.g., Argentina, Chile, China, Russia) could certainly bring additional differing viewpoints that could contribute to understanding the complex political processes being studied. Therefore, we caution that while our data is informative, and worth considering, it should not be considered exhaustive of differing viewpoints (Morgan, 2014). Similar forecasting exercises have relied on 12–15 individuals (Burgman et al., 2011a) or 13–25 individuals (Burgman et al., 2011b), and Aspinall (2010) recommends between 8 and 15 individuals and Hemming et al. (2017) 10–20 individuals (Aspinall, 2010; Hemming et al., 2017). Although we used a format designed to reduce individual and group biases, we have followed standard practice and reported the results in probabilistic terms that reflect the difficulties associated with forecasting. Developing forecasts of any event (e.g., fishery yields or species extinction risk) is difficult, but predicting human behavior is even more complex and the likelihood of various outcomes may even change in response to these forecasts—hence the field’s traditional use of Bayesian models to incorporate new data into forecasts and decision-making.
Additionally, while some research suggests that expert elicitations benefit from in-person workshops (Brown et al., 2014), other elicitations have successfully tested the efficacy of remote methods for capturing accurate assessments (McBride et al., 2012). In our case, we believe that an in-person workshop would have improved the quality of discussion during the second round by permitting more interaction and debate between the experts, but we were limited by the COVID-19 pandemic. For similar future efforts, we recommend attempting (where feasible) a remote, online discussion following the first round of forecasts. It is, however, important to remember that consensus is not the objective, and that even in a workshop, individuals must still develop their own independent first and second round estimates (Hemming et al., 2018).
Finally, structuring the ranking of factors section of the questionnaire to permit the experts to narrow the list themselves has some drawbacks. For one, this resulted in uneven lists of factors within each of the four primary categories. The result was a potential dilution of ‘votes’ for each factor that made it more difficult to clearly understand which factors played the greatest role in shaping perceptions. Were socioeconomic factors the most important, or would geopolitical factors have been selected more frequently if there had been a third option in the second round of the questionnaire? Were scientific factors truly less influential as the first round indicated? Or did the structure of the second questionnaire disadvantage them? Similarly, this approach to narrowing the categories led to somewhat overlapping categories in several instances. For example, “sensitive wildlife populations” likely overlaps with “large aggregations of wildlife”, and the inverse relationship between “existing fisheries” and “no fisheries” could be a problem. However, concerns about these very problems were the reason that we also directly asked them about the relative strength of the factors and then used the qualitative data to more closely examine what the ranking data actually represents.
Future Research
Although our elicitation consisting of ten individuals is consistent with guidance provided by the literature, we would like to test how doubling or tripling the group size to include a greater number of experts affects the precision and robustness of the forecasts. By this we mean to determine the group size at which the addition or removal of any individual forecast fails to lead to significantly different forecasts. Statistical theory suggests that larger groups would be less sensitive to the loss or addition of a single expert’s forecasts, therefore, it would be interesting to compare random subsets of forecasts to assess how many participants are required to create a more robust sample that is less sensitive to outlying forecasts. Similarly, this type of elicitation is influenced by a wide range of individual biophysical, socioeconomic, geopolitical, and scientific factors, many of which are linked to or informed by an even smaller subset of the participating experts. As a result, a larger group might make driver-based conclusions more robust by strengthening the individual factors that contribute to collective forecasts are (e.g., krill-sea ice dynamics). The challenge is that this will likely create a group that is too large for a single discussion. This might be addressed by breaking the group into sub-groups or possibly rotating participants so that they all interact with each other.
Finally, several comments during the discussion round suggested reconsidering the size of proposed no-take areas. Although larger areas are more likely to be representative and to allow species to move and adapt to the impacts of a changing climate (McLeod et al., 2009; Howard et al., 2017; Roberts et al., 2017), both of which are key objectives of CCAMLR MPAs (CCAMLR, 2011), several comments suggested that smaller areas may still provide conservation benefits while also being more politically acceptable.
Conclusion
In situations where conservation planning efforts lack sufficient spatial/ecological data or are characterized by high levels of uncertainty, we have demonstrated how expert elicitation and forecasting techniques can be an additional input into decision-making. In particular, these methods can be integrated during the early design phase by structuring data collection, during negotiations to designate an MPA, and after establishment by helping environmental decision-makers allocate resources, prioritize management interventions, or predict the likelihood of their success. Our findings show that experts can help to identify and then prioritize spaces for conservation using forecasting techniques, supports the potential application of structured expert elicitation techniques to collate, analyze and interpret judgments, which can facilitate effective knowledge sharing and consensus building through systematic and transparent processes. This is particularly true in politically charged negotiations and international environmental regimes such as CCAMLR that are highly politicized, characterized by complexity and uncertainty, and whose decisions have significant implications for global efforts to conserve biodiversity.
Data Availability Statement
The raw data supporting the conclusions of this article will be made available by the lead author upon request. However, any information that could be used to identify respondents will be removed to protect confidentiality.
Ethics Statement
The studies involving human participants were reviewed and approved by the Duke University Institutional Review Board. The participants provided their written informed consent to participate in this study.
Author Contributions
SS-B conceived of the study, secured funding for the project, managed the data collection, analyzed the data with input from NB, GM, and DG, and created the figures and led the writing of the manuscript. SS-B, JÁ-R, GM, and DG designed the questionnaire and elicitation. JA, AD, JH, GH, CJ, PS, KT, PT, and DW participated in the expert elicitation. JÁ-R, JA, AD, JH, GH, CJ, PS, KT, PT, DW, NB, GM, and DG provided edits and revisions to the final manuscript. All authors contributed to the article and approved the submitted version.
Funding
SS-B would like to thank Duke University, the Australian Government and Endeavor Research Fellowship Programme, and the French Government and the Chateaubriand STEM Research Fellowship for funding. JÁ-R acknowledges the support of the Australian Research Council.
Conflict of Interest
JA was employed by the Association of Responsible Krill Harvesting Companies, and PS by Aker BioMarine.
The remaining authors declare that the research was conducted in the absence of any commercial or financial relationships that could be construed as a potential conflict of interest.
Publisher’s Note
All claims expressed in this article are solely those of the authors and do not necessarily represent those of their affiliated organizations, or those of the publisher, the editors and the reviewers. Any product that may be evaluated in this article, or claim that may be made by its manufacturer, is not guaranteed or endorsed by the publisher.
Acknowledgments
SS-B would like to acknowledge and thank Andy Read, Victoria Hemming, Randy Kramer, John Little, Sharon Sykora, Thomas Bodie, Caleb Sykora-Bodie, and Meg Hewitt for other contributions and support. SS-B would also like to thank Christian Reiss for participating in the elicitation and the International Union for the Conservation of Nature for permitting him to attend CCAMLR meetings on its delegation. We do not have any conflicts of interest and this research was approved by the Duke University’s Institutional Research Board under protocol #2018-0072. The scientific results and conclusions, as well as any views or opinions expressed herein, are those of the authors and do not necessarily reflect those of NOAA or the U.S. Department of Commerce.
Supplementary Material
The Supplementary Material for this article can be found online at: https://www.frontiersin.org/articles/10.3389/fmars.2021.669135/full#supplementary-material
Footnotes
- ^ We chose an eight-year timeframe because the literature suggests that five years is too short (it provides insufficient time for events to progress or occur) and ten years is too long (it is difficult for participants to conceptualize/predict that far into the future). However, eight years aligns with the duration of two presidential terms, the Olympic cycle, COP meetings, etc., which is more easily conceptualized by participants for forecasting purposes.
References
Adams, V. M., Mills, M., Jupiter, S. D., and Pressey, R. L. (2011). Improving social acceptability of marine protected area networks: a method for estimating opportunity costs to multiple gear types in both fished and currently unfished areas. Biol. Conserv. 144, 350–361. doi: 10.1016/j.biocon.2010.09.012
Armstrong, J. S.(ed). (2001). Principles of Forecasting: A Handbook for Researchers and Practitioners. Norwell, MA: Kluwer Academic Publishers.
Aspinall, W. (2010). A route to more tractable expert advice. Nature 463, 294–295. doi: 10.1038/463294a
Ballance, L. T., Pitman, R. L., Hewitt, R. P., Siniff, D. B., Trivelpiece, W. Z., Clapham, P. J., et al. (2006). “The removal of large whales from the Southern Ocean: evidence for long-term ecosystem effects,” in Whales, Whaling, and Ocean Ecosystems, eds J. A. Estes, D. P. DeMaster, D. F. Doak, T. M. Williams, and R. L. Brownell Jr. (Oakland, CA: University of California Press), 215–230. doi: 10.1525/j.ctt1ppsvh.22
Ban, N. C., Gurney, G. G., Marshall, N. A., Whitney, C. K., Mills, M., Gelcich, S., et al. (2019). Well-being outcomes of marine protected areas. Nat. Sustainab. 19, 1–9. doi: 10.1038/s41893-019-0306-2
Bell, E., Kingston, N., Burgess, N., Sandwith, T., Ali, N., and MacKinnon, K. (eds) (2018). Protected Planet Report. Gland: IUCN.
Bray, D. (2020). The geopolitics of Antarctic governance: sovereignty and strategic denial in Australia’s Antarctic policy. Aust. J. Int. Aff. 70, 256–274. doi: 10.1080/10357718.2015.1135871
Brooks, C. M., Chown, S. L., Douglass, L. L., Raymond, B. P., Shaw, J. D., Sylvester, Z. T., et al. (2020). Progress towards a representative network of southern ocean protected areas. PLoS One 15:e0231361. doi: 10.1371/journal.pone.0231361
Brown, G., Donovan, S., Pullar, D., Pocewicz, A., Toohey, R., and Ballesteros-Lopez, R. (2014). An empirical evaluation of workshop versus survey PPGIS methods. Appl. Geogr. 48, 42–51. doi: 10.1016/j.apgeog.2014.01.008
Brown, G., McAlpine, C., Rhodes, J., Lunney, D., Goldingay, R., Fielding, K., et al. (2019). Integration of social spatial data to assess conservation opportunities and priorities. Biol. Conserv. 236, 452–463. doi: 10.1016/j.biocon.2019.06.002
Burgman, M. A. (2005). Risks and Decisions for Conservation and Environmental Management. Cambridge: Cambridge University Press.
Burgman, M. A., Carr, A., Godden, L., Gregory, R., McBride, M., Flander, L., et al. (2011a). Redefining expertise and improving ecological judgment. Conserv. Lett. 4, 81–87. doi: 10.1111/j.1755-263X.2011.00165.x
Burgman, M. A., McBride, M., Ashton, R., Speirs-Bridge, A., Flander, L., Wintle, B., et al. (2011b). Expert status and performance. PLoS One 6:e22998. doi: 10.1371/journal.pone.0022998
CCAMLR (1980). Convention on the Conservation of Antarctic Marine Living Resources. Canberra, ACT: CCAMLR.
Chown, S. L., Clarke, A., Fraser, C. I., Cary, S. C., Moon, K. L., and McGeoch, M. A. (2015). The changing form of Antarctic biodiversity. Nature 522, 431–438. doi: 10.1038/nature14505
Choy, S. L., O’Leary, R., and Mengersen, K. (2009). Elicitation by design in ecology: using expert opinion to inform priors for Bayesian statistical models. Ecology 90, 265–277. doi: 10.1890/07-1886.1
Cinner, J. E., Zamborain-Mason, J., Gurney, G. G., Graham, N. A. J., MacNeil, M. A., Hoey, A. S., et al. (2020). Meeting fisheries, ecosystem function, and biodiversity goals in a human-dominated world. Science 368, 307–311. doi: 10.1126/science.aax9412
Coetzee, B. W. T., Convey, P., and Chown, S. L. (2017). Expanding the protected area network in Antarctica is urgent and readily achievable. Conserv. Lett. 10, 670–680. doi: 10.1111/conl.12342
Constable, A., Melbourne-Thomas, J., Corney, S. P., Arrigo, K. R., Barbraud, C., Barnes, D. K. A., et al. (2014). Climate change and southern ocean ecosystems I: how changes in physical habitats directly affect marine biota. Glob. Change Biol. 20, 3004–3025. doi: 10.1111/gcb.12623
Constable, A. J., de la Mare, W. K., Agnew, D. J., Everson, I., and Miller, D. (2000). Managing fisheries to conserve the Antarctic marine ecosystem: practical implementation of the convention on the conservation of antarctic marine living resources (CCAMLR). ICES J. Mar. Sci. 57, 778–791. doi: 10.1006/jmsc.2000.0725
Cook, C. N., Hockings, M., and Carter, R. B. (2010). Conservation in the dark? The information used to support management decisions. Front. Ecol. Environ. 8:181–186. doi: 10.1890/090020
Cordonnery, L., Hemmings, A. D., and Kriwoken, L. (2015). Nexus and imbroglio: CCAMLR, the madrid protocol and designating antarctic marine protected areas in the southern ocean. Int. J. Mar. Coast. Law 30, 727–764. doi: 10.1163/15718085-12341380
Cullis-Suzuki, S., and Pauly, D. (2010). Failing the high seas: a global evaluation of regional fisheries management organizations. Mar. Policy 34, 1036–1042. doi: 10.1016/j.marpol.2010.03.002
Delegations of Argentina and Chile (2017). Domain 1 Marine Protected Area Preliminary Proposal PART A-1: Priority Areas for Conservation. Commission for the Conservation of Antarctic Marine Living Resources.
Delegations of Argentina and Chile (2019). Revised proposal for a conservation measure establishing a Marine Protected Area in Domain 1 (Western Antarctic Peninsula and South Scotia Arc). Hobart, Tasmania: Commission for the Conservation of Antarctic Marine Living Resources.
Delegations of Argentina and Chile (2020). Revised proposal for a conservation measure establishing a Marine Protected Area in Domain 1 (Western Antarctic Peninsula and South Scotia Arc). Hobart, Tasmania: Commission for the Conservation of Antarctic Marine Living Resources.
Devillers, R., Pressey, R. L., Grech, A., Kittinger, J. N., Edgar, G. J., Ward, T., et al. (2014). Reinventing residual reserves in the sea: are we favouring ease of establishment over the need for protection? Aquat. Conserv. 25, 480–504. doi: 10.1002/aqc.2445
Dodds, K., and Hemmings, A. D. (2013). Britain and the British Antarctic Territory in the wider geopolitics of the Antarctic and the Southern Ocean. Int. Aff. 89, 1–16.
Doney, S. C., Ruckelshaus, M., Emmett Duffy, J., Barry, J. P., Chan, F., English, C. A., et al. (2012). Climate change impacts on marine ecosystems. Annu. Rev. Mar. Sci. 4, 11–37. doi: 10.1146/annurev-marine-041911-111611
Donlan, J. C., Wingfield, D. K., Crowder, L. B., and Wilcox, C. (2010). Using expert opinion surveys to rank threats to endangered species: a case study with sea turtles. Conserv. Biol. 24, 1586–1595. doi: 10.1111/j.1523-1739.2010.01541.x
Everson, I. (2015). Designation and management of large-scale MPAs drawing on the experiences of CCAMLR. Fish Fish. 18, 145–159. doi: 10.1111/faf.12137
Fabra, A., and Gascón, V. (2008). The convention on the conservation of antarctic marine living resources (CCAMLR) and the ecosystem approach. Int. J. Mar. Coast. Law 23, 567–598. doi: 10.1163/092735208x331854
Gaines, S. D., White, C., Carr, M. H., and Palumbi, S. R. (2010). Designing marine reserve networks for both conservation and fisheries management. Proc. Natl. Acad. Sci. U.S.A. 107, 18286–18293. doi: 10.1073/pnas.0906473107
Giakoumi, S., Grantham, H. S., Kokkoris, G. D., and Possingham, H. P. (2011). Designing a network of marine reserves in the mediterranean Sea with limited socio-economic data. Biol. Conserv. 144, 753–763. doi: 10.1016/j.biocon.2010.11.006
Giakoumi, S., Halpern, B. S., Michel, L. N., Gobert, S., Sini, M., Boudouresque, C.-F., et al. (2015). Towards a framework for assessment and management of cumulative human impacts on marine food webs. Conserv. Biol. 29, 1228–1234. doi: 10.1111/cobi.12468
Gleason, M., McCreary, S., Miller-Henson, M., Ugoretz, J., Fox, E., Merrifield, M., et al. (2010). Science-based and stakeholder-driven marine protected area network planning: a successful case study from north central California. Ocean Coast. Manag. 53, 52–68. doi: 10.1016/j.ocecoaman.2009.12.001
Groves, C. R., and Game, E. T. (2015). Conservation Planning: Informed Decisions for a Healthier Planet. Greenwood Village, CO: Roberts and Company Publishers.
Groves, C. R., Jensen, D. B., Valutis, L. L., Redford, K. H., Shaffer, M. L., Scott, J. M., et al. (2002). Planning for biodiversity conservation: putting conservation science into practice. Bioscience 52, 499–512. doi: 10.1641/0006-3568(2002)052[0499:pfbcpc]2.0.co;2
Guerrero, A. M., and Wilson, K. A. (2016). Using a social-ecological framework to inform the implementation of conservation plans. Conserv. Biol. 31, 290–301. doi: 10.1111/cobi.12832
Gurney, G. G., Pressey, R. L., Ban, N. C., Álvarez-Romero, J. G., Jupiter, S., and Adams, V. M. (2015). Efficient and equitable design of marine protected areas in Fiji through inclusion of stakeholder-specific objectives in conservation planning. Conserv. Biol. 29, 1378–1389. doi: 10.1111/cobi.12514
Halpern, B. S., Frazier, M., Potapenko, J., Casey, K. S., Koenig, K., Longo, C., et al. (2015). Spatial and temporal changes in cumulative human impacts on the world’s ocean. Nat. Commun. 6:7615. doi: 10.1038/ncomms8615
Halpern, B. S., Regan, H. M., Possingham, H. P., and McCarthy, M. A. (2006). Accounting for uncertainty in marine reserve design. Ecol. Lett. 9, 2–11. doi: 10.1111/j.1461-0248.2005.00827.x
Hanea, A. M., McBride, M. F., Burgman, M. A., Wintle, B. C., Fidler, F., Flander, L., et al. (2016). Investigate discuss estimate aggregate for structured expert judgement. Int. J. Forecast. 33, 267–279. doi: 10.1016/j.ijforecast.2016.02.008
Hemming, V., Burgman, M. A., Hanea, A. M., McBride, M. F., and Wintle, B. C. (2017). A practical guide to structured expert elicitation using the IDEA protocol. Methods Ecol. Evol. 9, 169–180. doi: 10.1111/2041-210X.12857
Hemming, V., Walshe, T. V., Hanea, A. M., Fidler, F., and Burgman, M. A. (2018). Eliciting improved quantitative judgements using the IDEA protocol: a case study in natural resource management. PLoS One 13:e0198468. doi: 10.1371/journal.pone.0198468
Hodgson-Johnston, I. (2015). Australian politics and Antarctic sovereignty: themes, protagonists and antagonists. Aust. J. Marit. Ocean Aff. 7, 183–202. doi: 10.1080/18366503.2015.1101811
Howard, J., McLeod, E., Thomas, S., Eastwood, E., Fox, M., Wenzel, L., et al. (2017). The potential to integrate blue carbon into MPA design and management. Aquatic Conserv: Mar. Freshw. Ecosyst. 27, 100–115. doi: 10.1002/aqc.2809
IPBES (2019). Summary for Policymakers of the Global Assessment Report on BIODiversity and Ecosystem Services of the Intergovernmental Science-Policy Platform on Biodiversity and Ecosystem Services, eds S. Díaz, J. Settele, E. S. Brondízio, H. T. Ngo, M. Guèze, J. Agard, et al. (Bonn: IPBES secretariat), 56. doi: 10.5281/zenodo.3553579.
Jones, K. R., Maina, J. M., Kark, S., McClanahan, T. R., Klein, C. J., and Beger, M. (2018). Incorporating feasibility and collaboration into large-scale planning for regional recovery of coral reef fisheries. Mar. Ecol. Prog. Ser. 604, 211–222. doi: 10.3354/meps12743
Kennicutt, M. C., Chown, S. L., Cassano, J. J., Liggett, D., Massom, R., Peck, L. S., et al. (2014). Six priorities for Antarctic science. Nature 512, 23–25. doi: 10.1038/512023a
Klein, C. J., Chan, A., Kircher, L., Cundiff, A. J., Gardner, N., Hrovat, Y., et al. (2008). Striking a balance between biodiversity conservation and socioeconomic viability in the design of marine protected areas. Conserv. Biol. 22, 691–700. doi: 10.1111/j.1523-1739.2008.00896.x
Kroodsma, D. A., Mayorga, J., Hochberg, T., Miller, N. A., Boerder, K., Ferretti, F., et al. (2018). Tracking the global footprint of fisheries. Science 359, 904–908. doi: 10.1126/science.aao5646
Krueger, T., Page, T., Hubacek, K., Smith, L., and Hiscock, K. (2012a). The role of expert opinion in environmental modelling. Environ. Model. Softw. 36, 4–18. doi: 10.1016/j.envsoft.2012.01.011
Krueger, T., Page, T., Smith, L., and Voinov, A. (2012b). A guide to expert opinion in environmental modelling and management. Environ. Model. Softw. 36, 1–3. doi: 10.1016/j.envsoft.2012.01.006
Kuhnert, P. M., Martin, T. G., and Griffiths, S. P. (2010). A guide to eliciting and using expert knowledge in Bayesian ecological models. Ecol. Lett. 13, 900–914. doi: 10.1111/j.1461-0248.2010.01477.x
Lechner, A. M., Raymond, C. M., Adams, V. M., Polyakov, M., and Gordon, A. (2014). Characterizing spatial uncertainty when integrating social data in conservation planning. Conserv. Biol. 28, 1497–1511. doi: 10.111/cobi.12409
Liu, N., and Brooks, C. M. (2018). China’s changing position towards marine protected areas in the southern ocean: implications for future Antarctic governance. Mar. Policy 94, 189–195. doi: 10.1016/j.marpol.2018.05.011
MacMillan, D. C., and Marshall, K. (2006). The delphi process–an expert-based approach to ecological modelling in data-poor environments. Anim. Conserv. 9, 11–19. doi: 10.1111/j.1469-1795.2005.00001.x
Margules, C. R., and Pressey, R. L. (2000). Systematic conservation planning. Nature 405, 243–253. doi: 10.1038/35012251
Martin, T. G., Burgman, M. A., Fidler, F., Kuhnert, P. M., Low-Choy, S., McBride, M., et al. (2012). Eliciting expert knowledge in conservation science. Conserv. Biol. 26, 29–38. doi: 10.1111/j.1523-1739.2011.01806.x
Mastrandrea, M. D., Field, C. B., Stocker, T. F., Edenhofer, O., Ebi, K. L., Frame, D. J., et al. (2010). Guidance Note for Lead Authors of the IPCC 5th Assessment Report on Consistent Treatment of Uncertainties. Jasper Ridge, CA: Intergovernmental Panel on Climate Change, 1–7.
McBride, M. F., Garnett, S. T., Szabo, J. K., Burbidge, A. H., Butchart, S. H. M., Christidis, L., et al. (2012). Structured elicitation of expert judgments for threatened species assessment: a case study on a continental scale using email. Methods Ecol. Evol. 3, 906–920. doi: 10.1111/j.2041-210X.2012.00221.x
McCarthy, M. A., Thompson, C. J., Moore, A. L., and Possingham, H. P. (2011). Designing nature reserves in the face of uncertainty. Ecol. Lett. 14, 470–475. doi: 10.1111/j.1461-0248.2011.01608.x
McLeod, E., Salm, R., Green, A., and Almany, J. (2009). Designing marine protected area networks to address the impacts of climate change. Front. Ecol. Environ. 7:362–370. doi: 10.1890/070211
Miller, D. (2011). “Sustainable management in the southern ocean: CCAMLR science,” in Science Diplomacy Antarctica, Science, and the Governance of International Spaces, eds P. A. Berkman, M. A. Lang, D. W. H. Walton, and O. R. Young (Washington, DC: Smithsonian Institution Scholarly Press).
Miller, D., and Slicer, N. M. (2014). “CCAMLR and Antarctic conservation: the leader to follow?,” in Governance of Marine Fisheries and Biodiversity Conservation, eds S. M. Garcia, J. Rice, and A. Charles (West Sussex: John Wiley & Sons, Ltd), 253–270. doi: 10.1002/9781118392607.ch18
Mills, M., Pressey, R. L., Ban, N. C., Foale, S., Aswani, S., and Knight, A. T. (2013). Understanding characteristics that define the feasibility of conservation actions in a common pool marine resource governance system. Conserv. Lett. 6, 418–429. doi: 10.1111/conl.12025
Morgan, G. M. (2014). Use (and abuse) of expert elicitation in support of decision making for public policy. Proc. Natl. Acad. Sci. U.S.A. 111, 7176–7184. doi: 10.1073/pnas.1319946111
Naidoo, R., Gerkey, D., Hole, D., Pfaff, A., Ellis, A. M., Golden, C. D., et al. (2019). Evaluating the impacts of protected areas on human well-being across the developing world. Sci. Adv. 5:eaav3006. doi: 10.1126/sciadv.aav3006
National Academies (2016). “Reducing response burden in the american community survey,” in Proceedings of a Workshop (Washington, DC: The National Academies Press). doi: 10.17226/23639
Neuwirth, E. (2014). RColorBrewer: ColorBrewer Palettes. R package version 1.1-2. Available online at: https://CRAN.R-project.org/package=RColorBrewer.
O’Hagan, A. (2019). Expert knowledge elicitation: subjective but scientific. Am. Stat. 73, 69–81. doi: 10.1080/00031305.2018.1518265
O’Hagan, A., Buck, C. E., Daneshkhah, A., Eiser, J. R., Garthwaite, P. H., Jenkinson, D. J., et al. (2006). Uncertain Judgements. Chichester: John Wiley & Sons, Ltd.
O’Leary, R. A., Choy, S. L., Murray, J. V., Kynn, M., Denham, R., Martin, T. G., et al. (2009). Comparison of three expert elicitation methods for logistic regression on predicting the presence of the threatened brush−tailed rock−wallaby Petrogale penicillata. Environmetrics 20, 379–398. doi: 10.1002/env.935
Parkes, G. (2000). Precautionary fisheries management: the CCAMLR approach. Mar. Policy 24, 83–91. doi: 10.1016/S0308-597X(99)00015-9
Pollnac, R., Christie, P., Cinner, J. E., Dalton, T., Daw, T. M., Forrester, G. E., et al. (2010). Marine reserves as linked social-ecological systems. Proc. Natl. Acad. Sci. U.S.A. 107, 18262–18265. doi: 10.1073/pnas.0908266107
Pressey, R. L., and Bottrill, M. C. (2009). Approaches to Landscape- and Seascape-Scale Conservation Planning: Convergence, Contrasts and Challenges. Cambridge: Cambridge University Press.
Pressey, R. L., Visconti, P., and Ferraro, P. J. (2015). Making parks make a difference: poor alignment of policy, planning, and management with protected-area impact, and ways forward. Philos. Trans. R. Soc. B. Biol. Sci. 370:20140280.
R Core Team (2017). R: A language and Environment for Statistical Computing. Vienna: R Foundation for Statistical Computing.
Regan, H. M., Colyvan, M., and Burgman, M. A. (2002). A taxonomy and treatment of uncertainty for ecology and conservation biology. Ecol. Appl. 12, 618–628.
Rintoul, S. R. (2018). The global influence of localized dynamics in the Southern Ocean. Nature 558, 209–218. doi: 10.1038/s41586-018-0182-3
Roberts, C. M., O’Leary, B. C., McCauley, D. J., Cury, P. M., Duarte, C. M., Lubchenco, J., et al. (2017). Marine reserves can mitigate and promote adaptation to climate change. Proc. Natl. Acad. Sci. U.S.A. 114, 6167–6175. doi: 10.1073/pnas.1701262114
Rolstad, S., Adler, J., and Rydén, A. (2011). Response burden and questionnaire length: is shorter better? A review and meta-analysis. Value Health 14, 1101–1108. doi: 10.1016/j.jval.2011.06.003
Rossiter, J. S., and Levine, A. S. (2014). What makes a “successful” marine protected area? The unique context of Hawaii’s fish replenishment areas. Mar. Policy 44, 196–203. doi: 10.1016/j.marpol.2013.08.022
Spalding, M. D., Meliane, I., Milam, A., Fitzgerald, C., and Hale, L. Z. (2013). Protecting marine spaces:global targets and changing approaches. Ocean Yearbook 27, 213–248.
Speirs-Bridge, A., Fidler, F., McBride, M., Flander, L., Cumming, G., and Burgman, M. A. (2010). Reducing overconfidence in the interval judgments of experts. Risk Anal. 30, 512–523. doi: 10.1111/j.1539-6924.2009.01337.x
Sutherland, W. J., Fleishman, E., Mascia, M. B., Pretty, J., and Rudd, M. A. (2011). Methods for collaboratively identifying research priorities and emerging issues in science and policy. Methods Ecol. Evol. 2, 238–247. doi: 10.1111/j.2041-210X.2010.00083.x
Sykora-Bodie, S. T., Álvarez-Romero, J. G., Adams, V. M., Gurney, G. G., Cleary, J., Pressey, R. L., et al. (2021). Methods for identifying spatially referenced conservation needs and opportunities. Biol. Conserv. 21, 1–15. doi: 10.1016/j.biocon.2021.109138
Sykora-Bodie, S. T., and Morrison, T. H. (2019). Drivers of consensus−based decision−making in international environmental regimes: lessons from the Southern Ocean. Aquatic Conserv. Mar. Freshw. Ecosyst. 8, 311–325. doi: 10.1002/aqc.3200
Thiault, L., Gelcich, S., Marshall, N., Marshall, P., Chlous, F., and Claudet, J. (2019). Operationalizing vulnerability for social-ecological integration in conservation and natural resource management. Conserv. Lett. 13:e12677. doi: 10.1111/conl.12677
Thiault, L., Jupiter, S. D., Johnson, J. E., Cinner, J. E., Jarvis, R. M., Heron, S. F., et al. (2021). Harnessing the potential vulnerability of assessments for managing social-ecological systems. Ecol. Soc. 26:1. doi: 10.575/ES-12167-260201
Thiault, L., Marshall, P., Gelcich, S., Collin, A., Chlous, F., and Claudet, J. (2017). Mapping social-ecological vulnerability to inform local decision making. Conserv. Biol. 32, 447–456. doi: 10.1111/cobi.12989
Thiault, L., Marshall, P., Gelcich, S., Collin, A., Chlous, F., and Claudet, J. (2018). Mapping social-ecological vulnerability to inform local decision making. Conserv. Biol. 32, 447–456.
Tulloch, A. I. T., Tulloch, V. J. D., Evans, M. C., and Mills, M. (2014). The value of using feasibility models in systematic conservation planning to predict landholder management uptake. Conserv. Biol. 28, 1462–1473. doi: 10.1111/cobi.12403
Ungar, L., Mellors, B., Satopää, V., Baron, J., Tetlock, P., Ramos, J., et al. (2012). The Good Judgment Project: A Large Scale Test of Different Methods of Combining Expert Predictions AAAI Fall Symposium-Technical report, Association for the Advancement of Artificial Intelligence, 37–42.
Walmsley, S. F., and White, A. T. (2003). Influence of social, management and enforcement factors on the long-term ecological effects of marine sanctuaries. Environ. Conserv. 30, 388–407. doi: 10.1017/S0376892903000407
Ward, T. J. (2014). The condition of Australia’s marine environment is good but in decline-an integrated evidence-based national assessment by expert elicitation. Ocean Coast. Manag. 100, 86–100. doi: 10.1016/j.ocecoaman.2014.07.012
Watson, J. E. M., Dudley, N., Segan, D. B., and Hockings, M. (2014). The performance and potential of protected areas. Nature 515, 67–73. doi: 10.1038/nature13947
Welsford, D. C., Constable, A. J., and Nowara, G. B. (2011). The heard island and McDonald islands marine reserve and conservation zone–a model for southern ocean marine reserves? Cybium 35, 297–304. doi: 10.26028/cybium/2011-35SP-034
Wenzel, L., Gilbert, N., Goldsworthy, L., Tesar, C., McConnell, M., and Okter, M. (2016). Polar opposites? Marine conservation tools and experiences in the changing Arctic and Antarctic. Aquatic Conserv. Mar. Freshw. Ecosyst. 26, 61–84. doi: 10.1002/aqc.2649
Wickham, H., Averick, M., Bryan, J., Chang, W., McGowan, L., François, R., et al. (2019). Welcome to the tidyverse. J. Open Source Softw. 4:1686. doi: 10.21105/joss.01686
Wickham, H., and Bryan, J. (2019). readxl: Read Excel Files. R package version 1.3.1. Available online at: https://CRAN.R-project.org/package=readxl
Wickham, H., François, R., Henry, L., and Müller, K. (2020). dplyr: A Grammar of Data Manipulation. R package version 0.8.5. Available online at: https://CRAN.R-project.org/package=dplyr
Wilcox, C., Mallos, N. J., Leonard, G. H., Rodriguez, A., and Hardesty, B. D. (2016). Using expert elicitation to estimate the impacts of plastic pollution on marine wildlife. Mar. Policy 65, 107–114. doi: 10.1016/j.marpol.2015.10.014
Wildermann, N. E., Gredzens, C., Avens, L., Barrios-Garrido, H. A., Bell, I., Blumenthal, J., et al. (2018). Informing research priorities for immature sea turtles through expert elicitation. Endanger. Species Res. 37, 55–76. doi: 10.3354/esr00916
Williamson, M. A., Schwartz, M. W., and Lubell, M. N. (2018). Spatially explicit analytical models for social–ecological systems. Bioscience 68, 885–895. doi: 10.1093/biosci/biy094
Keywords: Antarctica, CCAMLR, conservation planning, expert elicitation, forecasting, marine conservation, marine protected areas, Southern Ocean
Citation: Sykora-Bodie ST, Álvarez-Romero JG, Arata JA, Dunn A, Hinke JT, Humphries G, Jones C, Skogrand P, Teschke K, Trathan PN, Welsford D, Ban NC, Murray G and Gill DA (2021) Using Forecasting Methods to Incorporate Social, Economic, and Political Considerations Into Marine Protected Area Planning. Front. Mar. Sci. 8:669135. doi: 10.3389/fmars.2021.669135
Received: 18 February 2021; Accepted: 21 June 2021;
Published: 13 August 2021.
Edited by:
Holly J. Niner, University of Plymouth, United KingdomReviewed by:
Marissa F. McBride, Harvard University, United StatesDanielle Smith, University of Tasmania, Australia
Copyright © 2021 Sykora-Bodie, Álvarez-Romero, Arata, Dunn, Hinke, Humphries, Jones, Skogrand, Teschke, Trathan, Welsford, Ban, Murray and Gill. This is an open-access article distributed under the terms of the Creative Commons Attribution License (CC BY). The use, distribution or reproduction in other forums is permitted, provided the original author(s) and the copyright owner(s) are credited and that the original publication in this journal is cited, in accordance with accepted academic practice. No use, distribution or reproduction is permitted which does not comply with these terms.
*Correspondence: Seth T. Sykora-Bodie, c2V0aHN5a29yYWJvZGllQGdtYWlsLmNvbQ==