Integrated Population Genomic Analysis and Numerical Simulation to Estimate Larval Dispersal of Acanthaster cf. solaris Between Ogasawara and Other Japanese Regions
- 1Graduate School of Agriculture, University of Miyazaki, Miyazaki, Japan
- 2Graduate School of Engineering and Science, University of the Ryukyus, Nishihara, Japan
- 3School of Environment and Society, Tokyo Institute of Technology, Tokyo, Japan
- 4Department of Life Science and Technology, School of Life Sciences and Technology, Tokyo Institute of Technology, Tokyo, Japan
- 5Institute of Boninology, Ogasawara, Japan
- 6Interdisciplinary Graduate School of Agriculture and Engineering, University of Miyazaki, Miyazaki, Japan
- 7Faculty of Medicine, University of Miyazaki, Miyazaki, Japan
- 8Japan Agency for Marine-Earth Science and Technology, Yokosuka, Japan
- 9Department of Genomics and Evolutionary Biology, National Institute of Genetics, Shizuoka, Japan
- 10Faculty of Agriculture, University of Miyazaki, Miyazaki, Japan
The estimation of larval dispersal on an ecological timescale is significant for conservation of marine species. In 2018, a semi-population outbreak of crown-of-thorns sea star, Acanthaster cf. solaris, was observed on a relatively isolated oceanic island, Ogasawara. The aim of this study was to assess whether this population outbreak was caused by large-scale larval recruitment (termed secondary outbreak) from the Kuroshio region. We estimated larval dispersal of the coral predator A. cf. solaris between the Kuroshio and Ogasawara regions using both population genomic analysis and simulation of oceanographic dispersal. Population genomic analysis revealed overall genetically homogenized patterns among Ogasawara and other Japanese populations, suggesting that the origin of the populations in the two regions is the same. In contrast, a simulation of 26-year oceanographic dispersal indicated that larvae are mostly self-seeded in Ogasawara populations and have difficulty reaching Ogasawara from the Kuroshio region within one generation. However, a connectivity matrix produced by the larval dispersal simulation assuming a Markov chain indicated gradual larval dispersal migration from the Kuroshio region to Ogasawara in a stepping-stone manner over multiple years. These results suggest that the 2018 outbreak was likely the result of self-seeding, including possible inbreeding (as evidenced by clonemate analysis), as large-scale larval dispersal from the Kurishio population to the Ogasawara population within one generation is unlikely. Instead, the population in Ogasawara is basically sustained by self-seedings, and the outbreak in 2018 was also most likely caused by successful self-seedings including possible inbreeding, as evidenced by clonemate analysis. This study also highlighted the importance of using both genomic and oceanographic methods to estimate larval dispersal, which provides significant insight into larval dispersal that occurs on ecological and evolutionary timescales.
Introduction
Many benthic marine invertebrates in coral reef ecosystems exhibit planktonic larval dispersal during their early life histories. Such larval dispersal connects different populations and forms a meta-population structure (Shanks, 2009). Thus, for effective conservation and management of benthic marine invertebrates, assessment of larval dispersal is important (Almany et al., 2009; Botsford et al., 2009). Because it is challenging to directly track the movement of numerous larvae that are smaller than 1 mm in the field, several different indirect methods, including population genetic analysis (e.g., Arndt and Smith, 1998; Yasuda et al., 2009), oceanographic simulation (e.g., Miyake et al., 2009; Storlazzi et al., 2017), towed nets (e.g., Uthicke et al., 2015; Yasuda et al., 2015a; Suzuki et al., 2016), and drifting buoys (e.g., Fukuda and Hanamura, 1996), have been used to estimate larval dispersal. Although each method has advantages and disadvantages, the first two methods can be applied to relatively large spatial scales and thus have been used more frequently than the other methods. On the one hand, the data obtained by population genetic analysis reflect the biological processes of larval dispersal and recruitment. However, these results not only include larval dispersal occurring on an ecological timescale but also reflect historical gene flow and genetic breaks caused by past climate change and plate tectonics (Benzie, 1999a; Crandall et al., 2014). Oceanographic simulation, on the other hand, can capture snapshots of larval dispersal, although even a sophisticated biophysical model cannot accurately simulate larval behavior, recruitment, and survival in the ocean (White et al., 2019). It is therefore important to integrate different methods to estimate larval dispersal (Marko and Hart, 2017), although few studies have attempted to do so (but see Alberto et al., 2011; Schunter et al., 2011; Nakabayashi et al., 2019; Taninaka et al., 2019).
In this study, we examined larval dispersal of a species of crown-of-thorns sea star, Acanthaster cf. solaris, which is a notorious predator of reef-building corals in the Pacific. Recent chronic outbreaks of crown-of-thorns sea stars have destroyed coral reef ecosystems in the Indian and Pacific oceans (Baird et al., 2013). For example, more than 90% of coral reefs over 38 km2 in area were lost due to the feeding damage of crown-of-thorns sea star in Guam (Chesher, 1969). On Australia’s Great Barrier Reef (GBR), more than half of the coral reefs have been reduced by 50% in size in the last 27 years and 42% of the decline of corals was due to population outbreaks of crown-of-thorns starfish (De’ath et al., 2012). The crown-of-thorns starfish is a species complex consisting of four genetically different lineages (Haszprunar et al., 2017); Pacific crown-of-thorns sea star (which is considered to be Acanthaster cf. solaris), and three other Indian ocean crown-of-thorns sea stars (Acanthaster planci in the north Indian Ocean and two more Acanthaster spp. in Red Sea and south Indian Ocean). Population outbreaks of crown-of-thorns sea star in the Indian and Pacific Oceans are largely categorized into two types. One is called “primary outbreak,” which is defined as an independent, sudden increase of crown-of-thorns sea star population at a location. The other is called “secondary outbreak,” which is defined as an outbreak caused by the spread of larvae released by another outbreak in neighboring regions. While the causes of primary outbreaks are still controversial, the mechanisms of secondary outbreaks are more obvious. With high fecundity and a larval stage that lasts a few to several weeks (Lucas, 1973; Yamaguchi, 1977), once a population outbreak takes place at a population, the large number of larvae produced and dispersed from the population can cause successive population outbreaks at neighboring sites, especially in the western Pacific regions that are impacted by strong warm currents (reviewed in Birkeland and Lucas, 1990; Pratchett et al., 2014; Yasuda, 2018). Given that early monitoring and control are key for management of A. cf. solaris (Birkeland and Lucas, 1990), assessment of the spatial range of secondary outbreaks of A. cf. solaris is necessary for predicting successive outbreaks and thus important for coral reef conservation.
Many population genetic and phylogeographic studies of A. cf. solaris have been conducted using various molecular markers (e.g., Benzie, 1992, 1999a; Yasuda et al., 2009, 2015b; Timmers et al., 2011; Vogler et al., 2013). These studies have indicated strong gene flow over a long distance along western boundary currents such as the Kuroshio Current region (genetic homogeneity from across the Ryukyu Islands to the temperate Pacific coast of mainland Japan) and the Eastern Australian Current region (the GBR, Nash et al., 1988; Benzie, 1999b; Vogler et al., 2013; Harrison et al., 2017), while relatively limited gene flow and genetic differentiation were observed among distant Pacific islands (Yasuda et al., 2009; Timmers et al., 2012; Vogler et al., 2013). Overall, the studies found strong gene flow and genetic homogeneity in regions with strong currents. Genetic data, however, strongly reflect historical gene flow that occurred during past climate change, and the spatial extent of secondary outbreaks could be overestimated (Benzie, 1999b; Yasuda et al., 2009, Yasuda et al., 2015b). Larval dispersal simulation studies in the GBR have indicated southward larval dispersal along the Eastern Australian Current, which is consistent with historically observed patterns of secondary outbreaks of A. cf. solaris (Dight et al., 1990a,b; James and Scandol, 1992; Scandol and James, 1992). Net surveys also have shown evidence of large-scale larval dispersal of A. cf. solaris along the GBR (Uthicke et al., 2015). While intensive studies of connectivity for A. cf. solaris have been conducted at the Indo-Pacific scale and in some localized regions where secondary outbreaks were suspected (e.g., the Kuroshio region and the GBR), connectivity between such regions and some relatively isolated oceanic island regions has yet to be examined.
In 2018, a population outbreak of A. cf. solaris on Ogasawara Island was reported (Biodiversity Center, Natural Environment Bureau, Ministry of the Environment, 2021), which was the second population outbreak on Ogasawara since 1979 (Kurata, 1984). Ogasawara Island is an oceanic island situated ca. 1,000 km south of the Tokyo urban center, and it is out of the mainstream of the Kuroshio Current. It is therefore still unknown whether this population outbreak is caused by mass larval recruitment produced by the outbreaks in the Kuroshio region.
The aim of this study was to estimate larval dispersal between the Kuroshio region and Ogasawara to determine whether the population outbreak in Ogasawara was successively caused by outbreaks in the Kuroshio region. To meet this aim, we used two different methods to estimate larval dispersal; population genomic analysis called multiplexed ISSR genotyping by sequencing (MIG-seq) and oceanographic larval dispersal simulation based on the Global HYbrid Coordinate Ocean Model (HYCOM).
Materials and Methods
Sampling and DNA Extraction
A total of 187 starfish samples were collected from six Kuroshio regions (Tatsukushi, Miyazaki, Sakurajima, Onna Village, Miyako, and Sekisei Lagoon) and Ogasawara via scuba diving (Table 1 and Figure 1). The tube feet were preserved in 99.5% ethanol prior to DNA extraction. Genomic DNA extraction was carried out using a modified heat alkaline method (Meeker et al., 2007; Nakabayashi et al., 2019).
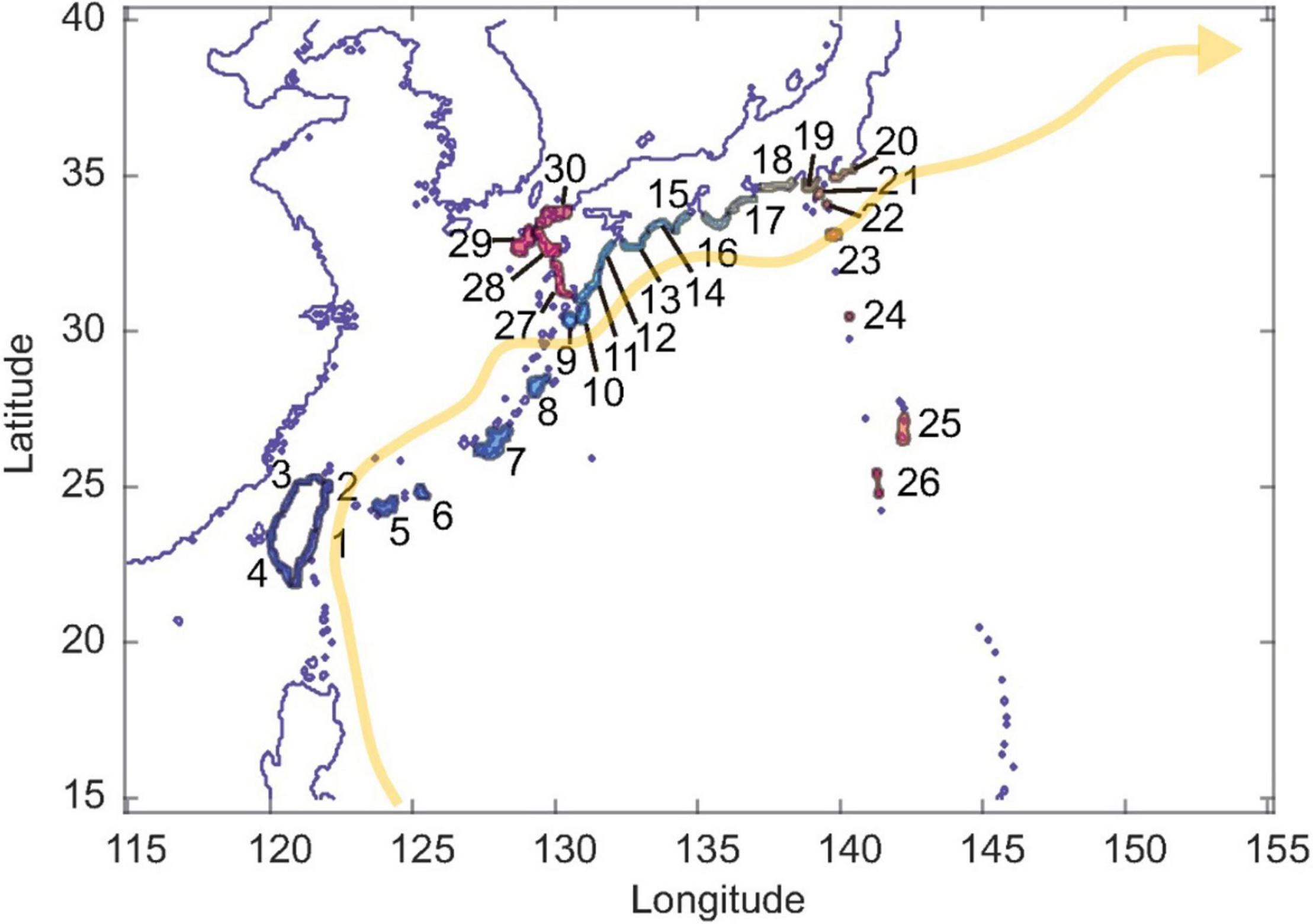
Figure 1. The site numbers for numerical simulation including sampling sites for genomic analysis [5 = Sekisei Lagoon (SKS), 6 = Miyako (MYK), 7 = Onna Village (ONN), 11 = Miyazaki (MYZ), 13 = Tatsukushi (TTK), 25 = Ogasawara (OGS), and 27 = Sakurajima (SKR)]. Yellow arrow indicate Kuroshio current.
Whole-Genome Sequencing and Assembly of a Reference Genome
We obtained a new high-quality whole-genome sequence for mapping. Whole-genome sequencing of the sample collected from Miyazaki (BioSample ID, SAMD00056692) was performed using Sequel, which is a single-molecule real-time sequencer from Pacific Biosciences (PacBio; Menlo Park, CA, United States), according to the manufacturer’s protocol. Previously published whole-genome short-read sequencing data (Wada et al., 2020) were downloaded from the BioProject PRJDB4009 in the ENA/Genbank/DDBJ database. Briefly, we obtained two paired-end (PE) libraries (insert sizes: 300 and 500 bp) and six mate-pair (MP) libraries (insert sizes: 3k, 5k, 8k, 10k, 12k, and 15k).
For the in silico procedures in this section, default parameters were used except where otherwise noted. Illumina PE and MP reads were filtered and trimmed using Platanus_trim v1.0.7.1 We assembled trimmed PE and MP reads using Platanus v1.2.5 (Kajitani et al., 2014) with the commands “assemble,” “scaffold,” and “gap_close,” resulting in the scaffolds.
In addition to the short-read assembly, PacBio long-read assembly was conducted using Canu v1.7 (Koren et al., 2017). We polished the Canu contigs with the PacBio long reads using Pbalign v0.3.12 and Arrow in the GenomicConsensus package v2.2.2.3 We additionally polished the contigs with Illumina (Illumina, San Diego, CA, United States) short reads using Bowtie2 v2.3.5.1 (Langmead and Salzberg, 2012) and Pilon v.1.22 (Walker et al., 2014). The short read-based polishing was iterated until the number of corrected sites did not increase (number of iterations: 18).
Gaps in the short read-based scaffolds (Platanus result) were filled with polished long read-based contigs using an in-house program. The procedure is as follows (Supplementary Figure 1):
(1) Fixed-length regions flanking the gaps in scaffolds were extracted.
(2) The flanking sequences were aligned (queried) to the long read-based contigs using Minimap2 v2.17 (Li, 2018) with the options of “-c -k 19.”
(3) The alignment results were filtered out if sequence identity < 90%, query coverage < 25%, or multiple best-scoring hits were identified.
(4) Each gap was filled with a region between the alignments in the contig if the following conditions were satisfied:
(i) The flanking region pair was aligned to the same contig.
(ii) The distance between alignments < 50 kbp.
(iii) The strands of the alignments were consistent.
(iv) There was no “N” in the corresponding regions in the contig.
(5) Steps (1) to (4) were iterated eight times. Here, the specific length of the flanking regions was applied for each iteration (500, 1k, 5k, 10k, 20k, 40k, 80k, and 160k).
(6) Step (5) was iterated twice.
Finally, to eliminate contamination, we performed homology searches of the assembly against the NCBI genome database (human, bacteria, and virus), the mitochondrial genome of A. planci (accession no. NC007788), and the chromosome sequence of the COTS symbiont (COTS27, accession no. AP019861) using BLASTN v2.7.1 (Altschul et al., 1990). The assembled sequences (scaffolds or contigs) were eliminated as contamination if sequence identity ≥ 90% and query coverage ≥ 50% (Supplementary Table 1). We assessed the completeness of the draft genome using BUSCO v.4.0.6 with the Metazoa Odb10 dataset (Seppey et al., 2019). K-mer-based (k = 17) estimations of the sizes of the genome and duplicated regions were also conducted using Jellyfish v.2.2.10 (Marçais and Kingsford, 2011) and GenomeScope v.2.0 (Ranallo-Benavidez et al., 2020).
Multiplexed ISSR Genotyping by Sequencing Analysis
MIG-seq is a population genomic method that can easily detect a moderate number of neutral single nucleotide polymorphisms (SNPs) (Suyama and Matsuki, 2015). Previous studies using MIG-seq on marine species successfully discovered species boundaries and genetic structures that were undetectable using traditional genetic markers such as mitochondrial DNA and nuclear ribosomal internal transcribed spacer 2 (Nakabayashi et al., 2019; Takata et al., 2019).
We used eight pairs of multiplex ISSR primers (MIG-seq primer set 1; Suyama and Matsuki, 2015) with the Multiplex PCR Assay Kit Ver. 2 (TaKaRa Bio Inc., Shiga, Japan) for the first polymerase chain reaction (PCR). We slightly modified the protocol by Suyama and Matsuki (2015) and used a total reaction volume of 7 μL in a T100™ Thermal Cycler (Bio-Rad, Hercules, CA, United States) under the following conditions: initial denaturation at 94°C for 1 min; followed by 25 cycles of 94°C for 30 s, 38°C for 1 min, and 72°C for 1 min; and final extension at 72°C for 10 min. The samples were indexed in the second PCR, which was performed with PrimeSTAR GXL DNA polymerase (TaKaRa Bio Inc.) using a total reaction volume of 12 μL in a thermal cycler under the following conditions: 21 cycles of 98°C for 10 s, 54°C for 15 s, and 68°C for 1 min. PCR products were run on an agarose gel, and 350–800 bp PCR products were extracted from the gel. The gel-extracted DNA was pooled and sequenced using MiSeq Control Software v2.0.12 (Illumina) with the MiSeq Reagent v3 150 cycle kit (Illumina). Image analysis and base calling were performed using Real-Time Analysis Software v1.17.21 (Illumina).
To eliminate low-quality reads and primer sequences from the raw data, we used the FASTX-Toolkit v 0.0.14 (Gordon and Hannon, 2012)4 with a fastq-quality-filter setting of –q 30 –p 40. We removed adapter sequences for the Illumina MiSeq run from both the 5′ end (GTCA GATCGGAAGAGCACACGTCTGAACTCCAGTCAC) and the 3′ end (CAGAGATCGGAAGAGCGTCGTGTAGGGAAAGAC) using Cutadapt v1.13 (Martin, 2011), and then we excluded short reads less than 80 bp. We used Stacks v2.2 (Catchen et al., 2013; Rochette and Catchen, 2017) to stack the reads and extract the SNPs. We used the newly sequenced A. cf. solaris genome as a reference, as described below. We used the Gstacks option (-rm, -pcr, -duplicates) in Stacks v2.2 to remove PCR duplicates by randomly discarding all but one pair of each set of reads. We used population software in Stacks to prepare the dataset. We used the minimum percentage of individuals required to process a locus across all data (r) equal to 095. After excluding individuals missing more than 10% of SNP data, we used a single SNP option to avoid strong linkage between loci for Structure analysis. We used all SNPs for the remaining genetic analyses.
Population Genetics
BayeScan v2.1 (Narum and Hess, 2011) was used to detect possible SNPs under natural selection with a default setting. Genetic diversity (expected and observed heterozygosity, HE and HO, respectively), Hardy–Weinberg Equilibrium, global FST including and excluding Ogasawara samples, and pairwise FST values across different populations were calculated using GenAlEx v6.502 (Peakall and Smouse, 2012) with 9,999 permutations.
GenoDive v. 3.0 (Meirmans, 2020) was used to estimate possible clones within each population. Because the maximum pairwise genetic distance across all the samples ignoring missing data was 62 and there was a clear disjunction between a few possible clonemates and normal distribution histogram of non-clonemates that start from pairwise genetic distance of 13 (Supplementary Figure 2), we heuristically set a limit of possible clonemate as genetic distance of six to identify clones. Probability of Identify (Table 1) using all loci for each population was also estimated using GenAlEx 6.5 (Peakall and Smouse, 2012).
To test whether the Ogasawara population is genetically different from other populations, a hierarchical analysis of molecular variance was conducted with prior grouping (Ogasawara vs. other populations) using Arlequin v3.5 (Excoffier and Lischer, 2010).
Bayesian clustering analysis was conducted using Structure software v2.3.4 (Pritchard et al., 2000) with 500,000 burn-in followed by 500,000 steps of Markov chain Monte Carlo simulations and 10 iterations. We used admixture- and allele frequency-correlated models that were expected to best explain the data set. The LOCPRIOR model (Hubisz et al., 2009) was used with Ogasawara vs. other populations and without prior regional grouping, and the number of putative clusters (K) was set from 1 to 7. The online software Structure Harvester (Earl and von Holdt, 2012) was used to summarize the likelihood value. Structure plots were summarized using the online software CLUMPAK (Kopelman et al., 2015). To further visualize the genetic relationships among different populations, principal coordinate analysis was conducted using GenAlEx v6.502 based on pairwise genetic distances among populations. PGDspider v2.0.8.3 (Lischer and Excoffier, 2012) was used to convert the data formats.
Simulation of Larval Dispersal
We conducted a Lagrangian larval dispersal simulation to elucidate the oceanographic connectivity between the regions. For this simulation, we used Connectivity Modeling System v2.0 (Paris et al., 2013) with the ocean analysis/reanalysis products produced by the Global Ocean Forecasting System 3.0 of Global HYCOM (Chassignet et al., 2007), which are 1/12° resolution (∼9 km) daily products archived from 1993 to 2018.5 We extracted the surface water current and sea surface temperature (SST) datasets from the Global HYCOM products ranged from 115°E–155°E longitude and 15°N–40°N latitude. Thirty source and sink sites were chosen to create source sink polygons that fit purpose of this study (see Figure 1). The particles regarded as COTS larvae were numerically released from ocean areas inside the polygons and tracked. Once a particle passed more than 14 days after release entered a polygon, the particle was removed and judged as successful settlement, and the connection between the source polygon and sink polygon was counted. If a particle passed 35 days without entering a polygon, it was removed and scored as failure to recruit. The timing of particle release (start time of COTS mass spawning) and duration of spawning at each site were determined by the following rules using a history of SST at the site provided by Global HYCOM analysis/reanalysis products based on Yasuda et al. (2010): (1) If SST at the site rose above 28°C, the particles were released until SST dropped below 26°C or 30 days passed; (2) If SST at the site did not reach 28°C, the particles were released while SST was higher than 26°C. A total of 100 particles per day were released from each source polygon under the above conditions. Finally, we obtained a connectivity matrix that was created from larval dispersal simulations over 26 years (1993–2018).
Because the connectivity based on simulated larval dispersal is a one-generation direct linkage, indicating that stepping-stone migration had not been considered, we conducted back-tracing to the origin through a discrete-time Markov chain using the connectivity matrix. To this end, we first set the following form of the connectivity matrix N(m × m):
The matrix elements were normalized by the total number of sink particles as follows:
Where
The normalized connectivity matrix can be regarded as the transition matrix of the Markov chain. The n-th power of the transition matrix Pn represents the n time-step back-trace to the origin source.
Results
Sequencing and Single Nucleotide Polymorphism Metrics
In total, 11,461,866 raw reads ranging from 9,190 to 281,474 reads per sample were obtained for 187 A. cf. solaris samples. After filtering out the low-quality reads, 6,125,996 reads ranging from 5,996 to 107,468 reads per sample remained. On average, 189,536 reads per individual remained after two-step filtering. We excluded seven samples missing more than 10% data and used a total of 182 samples for analysis. Using all SNP options, we obtained a total of 464 SNPs, which were used for all subsequent analyses except for Structure analysis. For Structure analysis, 115 SNPs using a single SNP option were used. BayeScan indicated that all loci were neutral (q-values > 0.05).
Genetic Diversity and Genetic Differentiation
Genetic diversity (HO and HE) of the studied populations, including that from Ogasawara, were comparable and ranged from 0.039 to 0.044 and 0.039–0.045 for HO and HE, respectively (Table 1). In total five pairs of possible clonemates were found in Tatsukushi (TTK, one pair out of 12), Onna Village (ONN; one pair out of 41), and Ogasawara (OGS; three pairs out of 30) populations (Table 1). Global FST values were significant (P < 0.001) but small both for groups including (FST = 0.005) and excluding the Ogasawara population (FST = 0.006). Likewise, analysis of molecular variance with prior grouping (Ogasawara vs. other populations) indicated that FCT was low and not significant (0.00167, P = 0.219). Pairwise FST values across the studied populations were also small, and only two pairs [Sekisei Lagoon (SKS) and Tatsukushi (TTK), Miyazaki (MYZ), and Ogasawara (OGS)] became significant after sequential Bonferroni correction (α < 0.05) (Table 2).
Mean log likelihood values [mean lnP(D)] were highest at K = 1 without prior grouping and comparable at K from 1 to 7 with prior grouping, with the lowest standard deviation value of mean lnP(D) found at K = 1 (Supplementary Table 2). Structure bar plots with and without prior geographic grouping suggested genetic homogeneity across the populations (Figure 2A and Supplementary Figure 4). Principal coordinate analysis indicated that, along the x-axis, SKS, MYK, and Sakurajima (SKR) were divided from the others, although it was not related to geographic location or the year of sampling (Figure 2B).
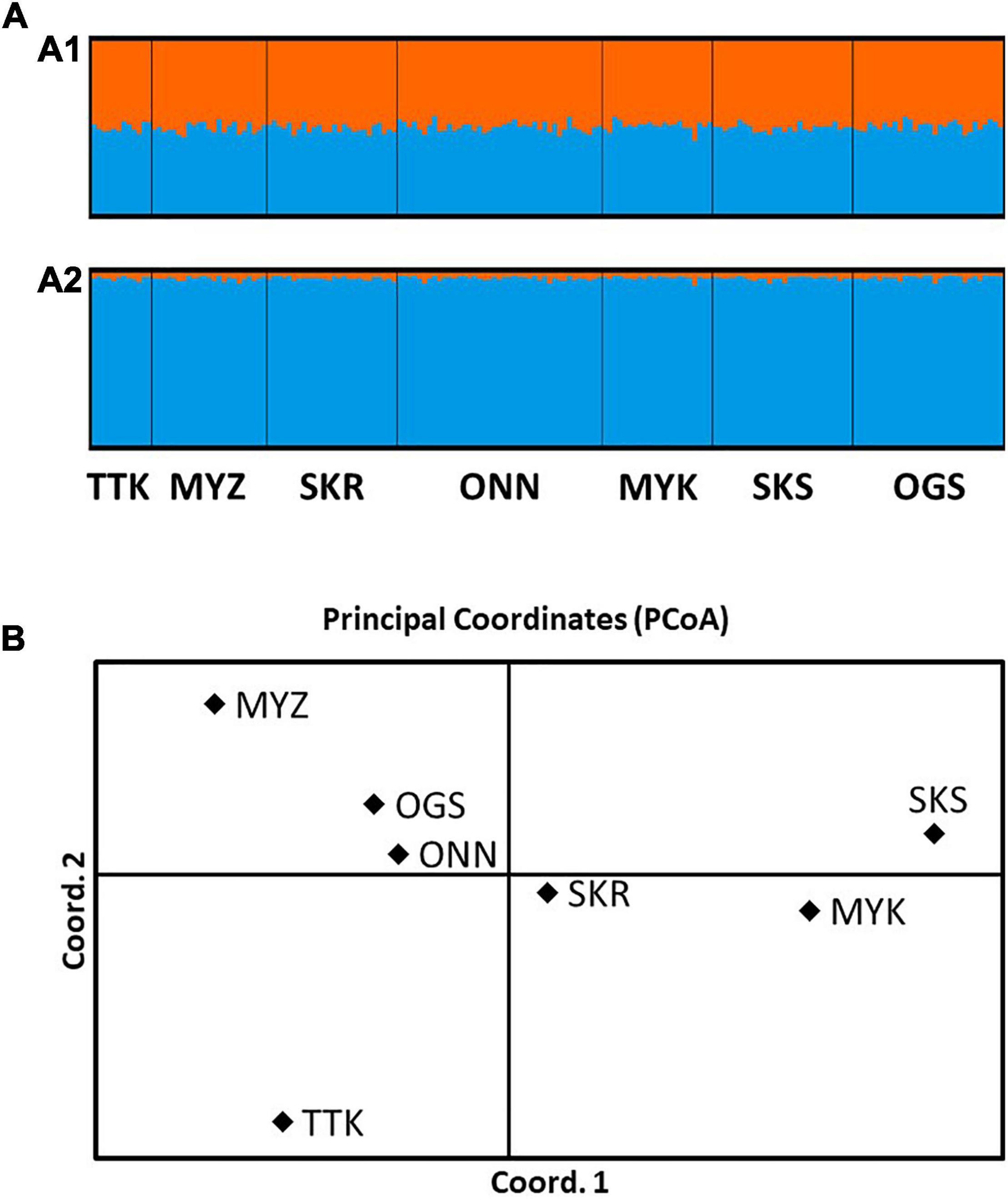
Figure 2. (A) Bar plot from Structure analysis at K = 2. (A1) Without prior geographic grouping. (A2) With prior geographic grouping (Ogasawara vs. other regions), indicating the population structure estimated by Structure v2.3.4 (Pritchard et al., 2000). The x-axis indicates individuals, and the y-axis indicates the proportion of hypothetical clusters, which are shown in different colors. (B) Results of principal coordinate analysis based on a covariance matrix with standardized data. The first and second axes explain 70.03 and 21.20% of the variation, respectively.
The Larval Dispersal Simulation
The results of the larval dispersal simulations are shown as a connectivity matrix (Supplementary Figure 3). The diagonal elements of the matrix indicate self-seeding. Basically, site numbers from 1 to 20 were assigned from south to north along the Kuroshio Current; site numbers from 21 to 25 were assigned from Nii-Jima and Shikine islands, which are closer to Japan’s main island, to the Ogasawara and Iwo-Jima Islands, which are further; and site numbers from 26 to 30 were assigned along the Tsushima Warm Current. The linkage of the site numbers for numerical simulation to site names for genomic analysis was as follows: 5 = Sekisei Lagoon (SKS), 6 = Miyako (MYK), 7 = Onna Village (ONN), 11 = Miyazaki (MYZ), 13 = Tatsukushi (TTK), 25 = Ogasawara (OGS), and 27 = Sakurajima (SKR). The results of this analysis confirmed that the larvae were transported from south to north by following the Kuroshio Current. However, they also confirmed that self-seeding dominated in the Ogasawara area, and it is very rare for larvae from the other sites to reach the Ogasawara area directly or for larvae from the Ogasawara area to reach the other sites directly.
Figure 3 shows the back-tracing results obtained using Markov chains. The bar of each site indicates the ratio of the number of particles released from the source site (laws of transition matrix Pn). The results indicate that the origin sites gradually converged with some of the upstream sites of the Kuroshio Current. The population in the Ogasawara area is not well mixed with that of the Kuroshio region, but the 200–500 time-steps, which may be regarded as representing over a few hundred generations, confirmed that the populations in the Kuroshio region and the Ogasawara region are almost mixed.
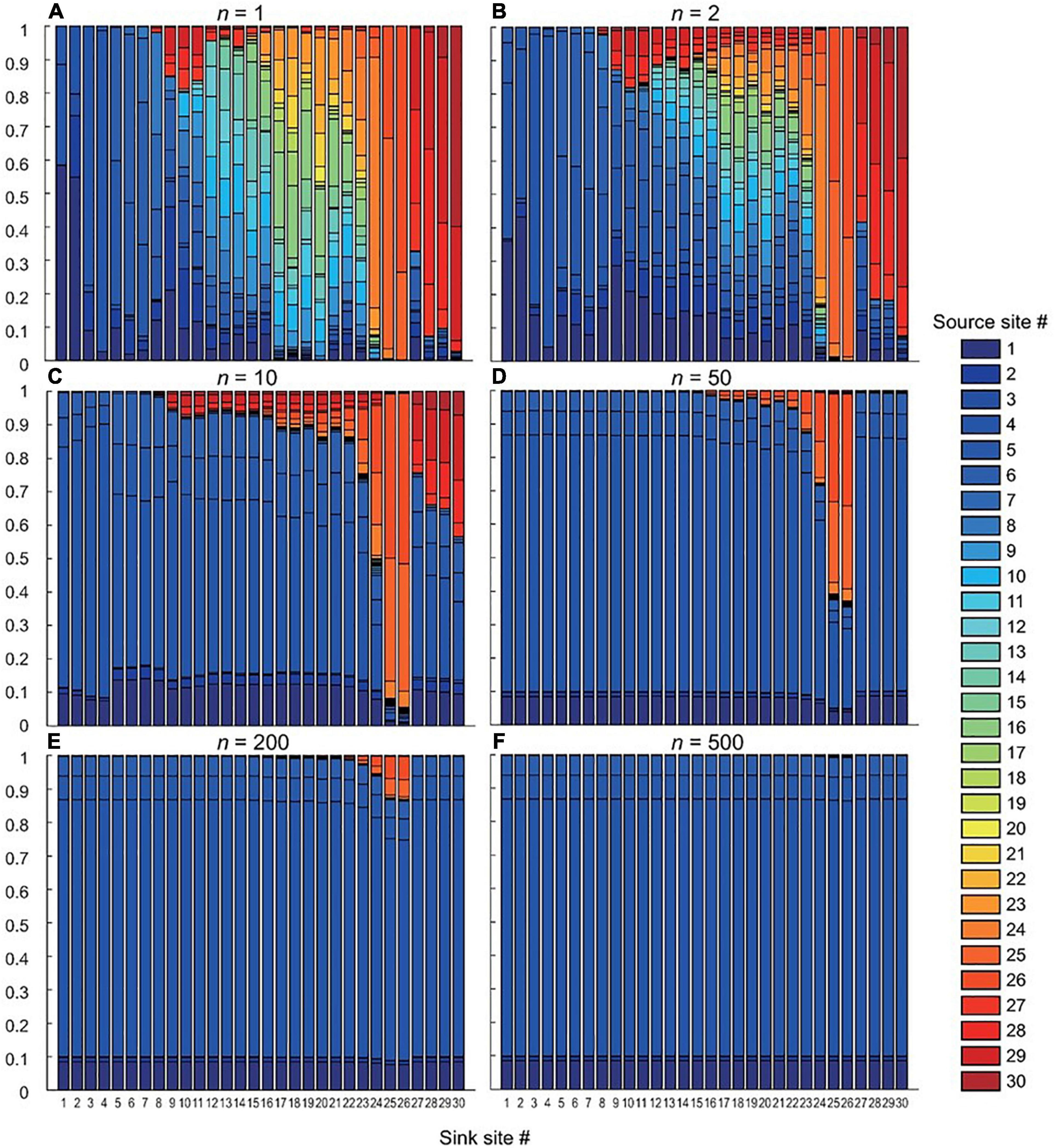
Figure 3. The ratio of the number of particles originating from each source site. (A) Simulation of 26-year larval dispersal (see sites, Figure 1 and connectivity matrix, Supplementary Figure 3). The ratio determined by Markov chains with (B) time-step n = 2, (C) n = 10, (D) n = 50, (E) n = 200, and (F) n = 500. Site numbers are assigned as follows: 5 = Sekisei Lagoon (SKS), 6 = Miyako (MYK), 7 = Onna Village (ONN), 11 = Miyazaki (MYZ), 13 = Tatsukushi (TTK), 25 = Ogasawara (OGS), and 27 = Sakurajima (SKR).
Discussion
This study examined larval dispersal of the coral predator A. cf. solaris between the Kuroshio region and Ogasawara using population genomic analysis and oceanographic simulation to test the secondary outbreak hypothesis. Contrasting results were obtained using different methods. Population genomic analysis indicated genetic homogeneity between the populations of the two regions, and oceanographic numerical simulation indicated that multiple-generation stepping-stone migration was required to disperse larvae from the Kurshio region to Ogasawara. These results indicate that the Ogasawara population of A. cf. solaris has the same genetic origin as that of the Kuroshio region, but a large amount of one-generation larval dispersal, such as a secondary outbreak from the Kuroshio region to Ogasawara, is unlikely. Rather, the data suggest that the population outbreak in Ogasawara might have been caused by successful self-seeding. This study highlights the importance of an integrated approach for estimating larval dispersal, including its timescale, for conservation purposes.
Potential of Secondary Outbreak Between Ogasawara and Kuroshio Region
In this study, all population genomic results indicated genetic similarity and no genetic structure between A. cf. solaris populations in the Ogasawara and Kuroshio regions. The analysis of molecular variance and Structure results indicate that the origins of A. cf. solaris populations in the Ogasawara and Kuroshio regions are genetically similar. Low FST/FCT values between the Ogasawara and Kuroshio regions indicate (1) a contemporary, large amount of larval dispersal, (2) accumulation of stepping-stone migrants over multiple years, or (3) recent separation of a population with no or limited ongoing gene flow. Based on the oceanographic numerical simulation analysis results and historical records of A. cf. solaris in Ogasawara, the latter two are more likely than contemporary, large amounts of larval dispersal.
The result of the 26-year oceanographic simulation suggests that the chance of direct one-generation larval dispersal between the Kuroshio region and Ogasawara is very low, although stepping-stone migration (e.g., via Hachijyo-jima in Izu islands) between the two regions over multiple generations is physically possible. The Markov chain simulation, which simulated stepping-stone migration over multiple generations, indicated that the Ogasawara population is dominated by migrants from the Kuroshio region after 200–500 generations. This implies that the accumulation of larvae from low levels of migration between the Kuroshio and Ogasawara regions over multiple years would result in genetic homogeneity. Given that there are no observed reports of population outbreaks of A. cf. solaris in stepping-stone regions (e.g., Izu islands) in the last 40 years (Yasuda, 2018), genetic homogeneity between the Ogasawara and Kuroshio regions observed by F-statistics and Structure analysis could be attributed to stepping-stone larval migration over multiple generations. Crandall et al. (2018) demonstrated observed results of the marine species along Hawaii archipelagos where FST is not significantly different from zero were not actually panmictic but having weak hierarchical structure of isolation-by-distance caused by stepping-stone migration. Coalescent simulation including A. cf. solaris samples from Izu islands would confirm this hypothesis.
Alternatively, it is possible that the observed strong gene flow resulted from recent colonization. According to a literature survey, there was no record of A. cf. solaris in the Ogasawara region before 1945, and the first record of this species in Ogasawara was in 1968 (Kurata, 1984; Yasuda, 2018). Since then, the density of A. cf. solaris in Ogasawara has been low (Yasuda, 2018). However, a lack of a record of A. cf. solaris in Ogasawara before 1945 does not necessarily mean a complete absence of this species in the region, given that almost no studies or surveys were conducted before 1945. In addition, crown-of-thorns sea stars are cryptic, and it would have been difficult to detect a few individuals that have been colonized for the first time. Recent migration with a small population size would predict low genetic diversity due to the founder effect, although we observed almost the same genetic diversity in the Ogasawara and Kuroshio regions. Therefore, high genetic diversity in Ogasawara and strong gene flow between the Ogasawara and Kuroshio regions is more likely caused by stepping-stone larval migration over multiple generations, as suggested by the oceanographic simulation.
Whichever may be the case, the oceanographic simulation suggests that contemporary, one-generation larval dispersal between the Ogasawara and Kuroshio regions is limited, and thus, direct secondary population outbreaks that require a large amount of larval dispersal within a generation from the Kuroshio region to Ogasawara are unlikely.
Kurata (1984) speculated that A. cf. solaris in Ogasawara might have colonized either from the Kuroshio region or from Mariana regions such as Guam. According to a population genetics analysis using microsatellite markers, genetic differentiation between the Kuroshio and Guam regions has been identified (Tusso et al., 2016), implying that colonization and/or secondary population outbreaks from Guam to the Ogasawara region are less likely than those from the Kuroshio region.
Only one previous study examined the genetic connectivity of coral reef organisms between the Kuroshio and Ogasawara regions. A reef-building coral species, Acropora sp., which has a shorter (average 3–4 days) larval stage than that of A. cf. solaris, showed genetic differentiation between the Ogasawara and Kuroshio regions (Nakajima et al., 2012). This implies that larval dispersal from the Kuroshio region to the Ogasawara region of coral reef organisms with short larval stages is quite limited.
Possible Causes of Population Outbreak in Ogasawara
Oceanographic simulation suggests that many larvae are self-recruited in Ogasawara compared with other A. cf. solaris populations in the Kuroshio region (Supplementary Table 3). This fact indicates that the observed population outbreak in Ogasawara in 2018 was caused by a sudden increase in regional self-seeding (namely “primary outbreak” as opposed to “secondary outbreak”). While several hypotheses have been proposed to explain the primary outbreak of A. cf solaris, the causes of primary outbreaks are still unclear (Pratchett et al., 2014). The causes of outbreak would also depend on several regional environmental conditions being met simultaneously (Pratchett et al., 2017; Okaji et al., 2019): elevated levels of larval nutrition and subsequent higher survival rates supported by favorable physical, chemical, and biological conditions such as lower predation on larvae and juveniles; and physical processes acting on larvae that increase their survival (e.g., weather conditions and current patterns) (Keesing and Halford, 1992; Okaji et al., 2019).
The population outbreak in Ogasawara in 2018 could be attributed to successful local larval recruitment in 2016, given that it takes 2 years for A. cf. solaris larvae to become adults (Birkeland and Lucas, 1990). Oceanographic simulation also suggests that the number of self-recruited larvae in Ogasawara was larger (397) in 2016, which exceeded the average (252) over 26 years (Supplementary Table 4). The large number of self-recruited larvae might be partly due to the smaller number of typhoons during the larval period (July to October, estimated by the spawning period associated with water temperature; Yasuda et al., 2010); in 2016, three typhoons passed through the Ogasawara region, which is below the 70-year average (4.47) (data from Japan Meteorological Agency, Supplementary Table 5). A previous field survey discovered dense clouds of A. cf. planci larvae before one typhoon, but they were scattered and disappeared from the coral reef area after the typhoon (Suzuki et al., 2016), implying that typhoons prevent mass settlement of larvae. However, in some years with a higher number of self-recruits and fewer typhoons, no population outbreak was observed in Ogasawara.
Recently, self-fertilization and/or clonal propagation hypothesis has been proposed by Guerra et al. (2020) to explain the cause of primary outbreak as evidenced by the existence of hermaphrodites and the finding of possible larval cloning of A. cf. solaris (Allen et al., 2019). In the present study, we found three pairs of individuals in Ogasawara population were possible clonal or genetically very close individual (Table 1 and Supplementary Figure 2). It is also notable that we also found one pair of possible clonemates in Tatsukushi population where previous study found hermaphrodites (Guerra et al., 2020). Although we need more precise analysis to identify true clonemates, it is possible that inbreeding including self-fertilization or larval cloning also partly facilitated the initiation of population outbreak in the oceanographically isolated Ogasawara populations.
Other factors such as increased success of cross-fertilization (Rogers et al., 2017), lower salinity, and higher phytoplankton biomass during the larval stage (Lucas, 1973), and reduced predation during the post-settlement stage (McCallum, 1987; Keesing and Halford, 1992) also may be associated with the demographic fluctuation of A. cf. solaris in the Ogasawara area. An Allee threshold of three starfish ha–1 (below which reproductive capacity is greatly reduced regardless of the aggregation level) is proposed and aggregation during spawning period is key in causing population outbreaks (Rogers et al., 2017). Laboratory experiments indicated that lower salinity and increased phytoplankton biomass that are often caused by territorial run-off enhances the survival rate of A. cf. solaris (Lucas, 1973; Birkeland, 1982; Fabricius et al., 2010). Keesing and Halford (1992) also suggested that juveniles of A. cf. solaris would be strongly influenced by predation because of their slow growth and movement, underlining the importance of posttreatment processes for population outbreak. All these factors may influence the population dynamics of A. cf. solaris in Ogasawara.
Multiple Approaches to Estimate Larval Dispersal in Marine Organisms
This study estimated larval dispersal of A. cf. solaris between Kuroshio and Ogasawara using two different approaches: population genomic analysis and larval dispersal simulation. Gene flow estimation by the population genomic approach often reflects both contemporary and evolutionary processes, rendering the interpretation of the amount of contemporary dispersal difficult because of the long evolutionary timescale. Genetic homogeneity can be observed due to a large amount of contemporary larval dispersal (secondary population outbreak) or stepping-stone migrants over multiple generations or recent divergence with no contemporary dispersal. Our study is unique in that it included an oceanographic model with Markov chain simulation. Such a simulation was useful for testing these alternative hypotheses. A simple oceanographic simulation averaged over 26 years first rejected the secondary population outbreak hypothesis by indicating limited physical larval transport from the Kuroshio to the Ogasawara region within a generation. This result supports the idea that stepping-stone migrants over multiple generations is responsible for the genetic homogeneity observed in the FST and Structure analysis between the Kuroshio and Ogasawara populations.
This may give the impression that oceanographic simulation alone is enough for testing the secondary population hypothesis; however, it is generally imperfect for estimating larval dispersal. Our model regarded larvae as neutral passive particles without any of the biological characteristics of larvae that may influence actual larval dispersal, such as vertical movement, natural selection, food availability, and mortality. In addition, the model only simulates the dispersal process, which does not include post-settlement survival. In some marine species, genetic connectivity is quite limited despite having long pelagic larval duration possibly caused by several biological features of larvae and juveniles (e.g., Barber et al., 2000, 2002). Thus, a comparison with genetic connectivity that reflects all the biological characteristics of the species is also important. While several previous studies have reported good agreement between oceanographic models and genetic analysis and have provided robust and straightforward interpretations of larval dispersal occurring on an ecological timescale (e.g., Galindo et al., 2006; White et al., 2010; Sunday et al., 2014; Taninaka et al., 2019), only a few have discussed the usefulness of oceanographic modeling for identifying alternative hypotheses or provided additional insights into the causes of observed population genetic structure (Galindo et al., 2010; Crandall et al., 2014). By applying Markov chain simulation in the numerical simulation, we could also include the effect of migration over multiple generations, which is easier to compare with genetic results than a single generation simulation result in terms of timescale. This method underlines the usefulness and importance of using both genetic and oceanographic methods to estimate larval dispersal and provides significant insight into larval dispersal that occurs on ecological and evolutionary timescales.
Conservation Implications
This study indicated that population outbreaks of A. cf. solaris in the Ogasawara Islands are independent of successive outbreaks in the Kuroshio region and that outbreaks are more likely caused by local self-seedings. Considering it takes at least 2 years for A. cf. solaris to reach adulthood (Birkeland and Lucas, 1990), caution and early monitoring should be taken 2 years following the observation of local ocean current system retention and other preferable conditions for the survival during early life stage of A. cf. solaris. For example, a large amount of terrestrial run-off will increase the survival rate of A. cf. solaris by increasing food available for larvae. In addition, high levels of larvae aggregation were observed only before a typhoon (Suzuki et al., 2016), and thus, a fewer number of typhoons during spawning periods may increase the chance of high levels of larval aggregation and settlement. Local monitoring of juvenile starfish 2 years after such preferable conditions would be useful for early detection and predicting population outbreaks and to avoid being too late for coral reef management (Okaji et al., 2019).
Data Availability Statement
The datasets presented in this study can be found in online repositories. The names of the repository/repositories and accession number(s) can be found below: DDBJ (accession: DRA011826).
Author Contributions
NY conceived the study. MH, NY, RK, HY, and TY drafted the manuscript. RK, HY, TI, and AT sequenced, assembled, and reconstructed the reference genome. NY, YA, and TS collected the Acanthaster samples. MH, HT, HY, TK, and NY conducted the MIG-seq analysis. TN and TY conducted oceanographic simulations. All authors edited the draft and approved it for publication.
Funding
Funding for this study was provided by the Environmental Research and Technology Development Fund (4RF–1501 and 4–1304) of the Ministry of the Environment, Japan; a Grant-in-Aid for Young Scientists (A) (17H04996), JSPS KAKENHI 221S0002, 16H06279 (PAGS) Grant-in-Aid for Research Fellows (19J21342 and 18J23317) and Bilateral Joint Research (JPJSBP120209929) from the Japan Society for the Promotion of Science (JSPS).
Conflict of Interest
The authors declare that the research was conducted in the absence of any commercial or financial relationships that could be construed as a potential conflict of interest.
Publisher’s Note
All claims expressed in this article are solely those of the authors and do not necessarily represent those of their affiliated organizations, or those of the publisher, the editors and the reviewers. Any product that may be evaluated in this article, or claim that may be made by its manufacturer, is not guaranteed or endorsed by the publisher.
Acknowledgments
We express our gratitude to Eriko Nagahiro for her help in the molecular experiment.
Supplementary Material
The Supplementary Material for this article can be found online at: https://www.frontiersin.org/articles/10.3389/fmars.2021.688139/full#supplementary-material
Footnotes
- ^ http://platanus.bio.titech.ac.jp/pltanus_trim
- ^ https://www.pacb.com/support/software-downloads/
- ^ https://github.com/PacificBiosciences/GenomicConsensus
- ^ http://hannonlab.cshl.edu/fastx_toolkit/index.html
- ^ https://www.hycom.org/
References
Alberto, F., Raimondi, P. T., Reed, D. C., Watson, J. R., Siegel, D. A., Mitarai, S., et al. (2011). Isolation by oceanographic distance explains genetic structure for Macrocystis pyrifera in the Santa Barbara channel. Mol. Ecol. 20, 2543–2554. doi: 10.1111/j.1365-294X.2011.05117.x
Allen, J. D., Richardson, E. L., Deaker, D., Agüera, A., and Byrne, M. (2019). Larval cloning in the crown-of-thorns sea star, a keystone coral predator. Mar. Ecol. Prog. Ser. 609, 271–276. doi: 10.3354/meps12843
Almany, G. R., Connolly, S. R., Heath, D. D., Hogan, J. D., Jones, G. P., McCook, L. J., et al. (2009). Connectivity, biodiversity conservation and the design of marine reserve networks for coral reefs. Coral Reefs 28, 339–351. doi: 10.1007/s00338-009-0484-x
Altschul, S. F., Gish, W., Miller, W., Myers, E. W., and Lipman, D. J. (1990). Basic local alignment search tool. J. Mol. Biol. 215, 403–410. doi: 10.1016/S0022-2836(05)80360-2
Arndt, A., and Smith, M. J. (1998). Genetic diversity and population structure in two species of sea cucumber: differing patterns according to mode of development. Mol. Ecol. 7, 1053–1064. doi: 10.1046/j.1365-294x.1998.00429.x
Baird, A. H., Pratchett, M. S., Hoey, A. S., Herdiana, Y., and Campbell, S. J. (2013). Acanthaster planci is a major cause of coral mortality in Indonesia. Coral Reefs 32, 803–812. doi: 10.1007/s00338-013-1025-1
Barber, P. H., Palumbi, S. R., Erdmann, M. V., and Moosa, M. K. (2000). A marine Wallace’s line? Nature 406, 692–693. doi: 10.1038/35021135
Barber, P. H., Palumbi, S. R., Erdmann, M. V., and Moosa, M. K. (2002). Sharp genetic breaks among populations of Haptosquilla pulchella (Stomatopoda) indicate limits to larval transport: patterns, causes, and consequences. Mol. Ecol. 11, 659–674. doi: 10.1046/j.1365-294x.2002.01468.x
Benzie, J. A. H. (1992). Review of the genetics, dispersal and recruitment of crown-of-thorns starfish (Acanthaster planci). Mar. Freshw. Res. 43, 597–610. doi: 10.1071/MF9920597
Benzie, J. A. H. (1999a). Genetic structure of coral reef organisms: ghosts of dispersal past. Am. Zool. 39, 131–145. doi: 10.1093/icb/39.1.131
Benzie, J. A. H. (1999b). Major genetic differences between crown-of-thorns starfish (Acanthaster planci) populations in the Indian and Pacific Oceans. Evolution 53, 1782–1795. doi: 10.1111/j.1558-5646.1999.tb04562.x
Biodiversity Center, Natural Environment Bureau, Ministry of the Environment (2021). Monitoring Site 1000 Coral Reef Survey Report. Available online at: http://www.biodic.go.jp/moni1000/findings/reports/pdf/2019_coral_reef.pdf (accessed December 16, 2021).
Birkeland, C. (1982). Terrestrial runoff as a cause of outbreaks of Acanthaster planci (Echinodermata: Asteroidea). Mar. Biol. 69, 175–185. doi: 10.1007/BF00396897
Birkeland, C., and Lucas, J. (1990). Acanthaster planci: Major Management Problem of Coral Reefs. Boca Ranton, FL: CRC Press, 272.
Botsford, L. W., White, J. W., Coffroth, M. A., Paris, C. B., Planes, S., Shearer, T. L., et al. (2009). Connectivity and resilience of coral reef metapopulations in marine protected areas: matching empirical efforts to predictive needs. Coral Reefs 28, 327–337. doi: 10.1007/s00338-009-0466-z
Catchen, J., Hohenlohe, P. A., Bassham, S., Amores, A., and Cresko, W. A. (2013). Stacks: an analysis tool set for population genomics. Mol. Ecol. 22, 3124–3140. doi: 10.1111/mec.12354
Chassignet, E. P., Hurlburt, H. E., Smedstad, O. M., Halliwell, G. R., Hogan, P. J., Wallcraft, A. J., et al. (2007). The HYCOM (HYbrid Coordinate Ocean Model) data assimilative system. J. Mar. Syst. 65, 60–83. doi: 10.1016/j.jmarsys.2005.09.016
Chesher, R. H. (1969). Destruction of Pacific corals by the sea star Acanthaster planci. Science 165, 280–283. doi: 10.1126/science.165.3890.280
Crandall, E. D., and Toonen, R. J. ToBo Laboratory Selkoe, K. A. (2018). A coalescent sampler successfully detects biologically meaningful population structure overlooked by F-statistics. Evol. Appl. 12, 255–265. doi: 10.1111/eva.12712
Crandall, E. D., Treml, E. A., Liggins, L., Gleeson, L., Yasuda, N., Barber, P. H., et al. (2014). Return of the ghosts of dispersal past: historical spread and contemporary gene flow in the blue sea star Linckia laevigata. Bull. Mar. Sci. 90, 399–425. doi: 10.5343/bms.2013.1052
De’ath, G., Fabricius, K. E., Sweatman, H., and Puotinen, M. (2012). The 27-year decline of coral cover on the Great Barrier Reef and its causes. Proc. Natl. Acad. Sci. U.S.A. 109, 17995–17999. doi: 10.1073/pnas.1208909109
Dight, I. J., Bode, L., and James, M. K. (1990a). Modelling the larval dispersal of Acanthaster planci I. Large scale hydrodynamics, Cairns Section, Great Barrier Reef Marine Park. Coral Reefs 9, 115–123. doi: 10.1007/BF00258222
Dight, I. J., James, M. K., and Bode, L. (1990b). Modelling the larval dispersal of Acanthaster planci. II. Patterns of reef connectivity. Coral Reefs 9, 125–134. doi: 10.1007/BF00258224
Earl, D. A., and von Holdt, B. M. (2012). STRUCTURE HARVESTER: a website and program for visualizing STRUCTURE output and implementing the Evanno method. Conserv. Genet. Resour. 4, 359–361. doi: 10.1007/s12686-011-9548-7
Excoffier, L., and Lischer, H. E. L. (2010). Arlequin suite ver 3.5: a new series of programs to perform population genetics analyses under Linux and Windows. Mol. Ecol. Resour. 10, 564–567. doi: 10.1111/j.1755-0998.2010.02847.x
Fabricius, K. E., Okaji, K., and De’ath, G. (2010). Three lines of evidence to link outbreaks of the crown-of-thorns seastar Acanthaster planci to the release of larval food limitation. Coral Reefs 29, 593–605. doi: 10.1007/s00338-010-0628-z
Fukuda, M., and Hanamura, Y. (1996). Small scale distribution and transport of the Japanese anchovy, Engraulis japonica, larvae determined from a free-drifting buoy survey in Iyo-Nada, Seto Inland Sea [Japan] [1995]. J. Agric. Sci. Technol. 28, 9–20.
Galindo, H. M., Olson, D. B., and Palumbi, S. R. (2006). Seascape genetics: a coupled oceanographic-genetic model predicts population structure of Caribbean corals. Curr. Biol. 16, 1622–1626. doi: 10.1016/j.cub.2006.06.052
Galindo, H. M., Pfeiffer-Herbert, A. S., McManus, M. A., Chao, Y. I., Chai, F. E. I., and Palumbi, S. R. (2010). Seascape genetics along a steep cline: using genetic patterns to test predictions of marine larval dispersal. Mol. Ecol. 19, 3692–3707. doi: 10.1111/j.1365-294X.2010.04694.x
Gordon, A., and Hannon, G. J. (2012). FASTX-toolkit. FASTQ/A Short-Reads Pre-Processing Tools. Available online at: http://hannonlab.cshl.edu/fastx_toolkit/ (accessed August 30, 2020).
Guerra, V., Haynes, G., Byrne, M., Yasuda, N., Adachi, S., Nakamura, M., et al. (2020). Non-specific expression of fertilization genes in the crown-of-thorns Acanthaster cf. solaris: unexpected evidence of hermaphroditism in a coral reef predator. Mol. Ecol. 29, 363–379. doi: 10.1111/mec.15332
Harrison, H. B., Pratchett, M. S., Messmer, V., Saenz-Agudelo, P., and Berumen, M. L. (2017). Microsatellites reveal genetic homogeneity among outbreak populations of crown-of-thorns starfish (Acanthaster cf. solaris) on Australia’s Great Barrier Reef. Diversity 9:16. doi: 10.3390/d9010016
Haszprunar, G., Vogler, C., and Wörheide, G. (2017). Persistent gaps of knowledge for naming and distinguishing multiple species of crown-of-thorns seastar in the Acanthaster planci species complex. Diversity 9:22. doi: 10.3390/d9020022
Hubisz, M. J., Falush, D., Stephens, M., and Pritchard, J. K. (2009). Inferring weak population structure with the assistance of sample group information. Mol. Ecol. Resour. 9, 1322–1332. doi: 10.1111/j.1755-0998.2009.02591.x
James, M. K., and Scandol, J. P. (1992). Larval dispersal simulations: correlation with the crown-of-thorns starfish outbreaks database. Mar. Freshw. Res. 43, 569–581. doi: 10.1071/MF9920569
Kajitani, R., Toshimoto, K., Noguchi, H., Toyoda, A., Ogura, Y., Okuno, M., et al. (2014). Efficient de novo assembly of highly heterozygous genomes from whole-genome shotgun short reads. Genome Res. 24, 1384–1395. doi: 10.1101/gr.170720.113
Keesing, J. K., and Halford, A. R. (1992). Importance of postsettlement processes for the population dynamics of Acanthaster planci (L.). Mar. Freshw. Res. 43, 635–651. doi: 10.1071/MF9920635
Kopelman, N. M., Mayzel, J., Jakobsson, M., Rosenberg, N. A., and Mayrose, I. (2015). CLUMPAK: a program for identifying clustering modes and packaging population structure inferences across K. Mol. Ecol. Resour. 15, 1179–1191. doi: 10.1111/1755-0998.12387
Koren, S., Walenz, B. P., Berlin, K., Miller, J. R., Bergman, N. H., and Phillippy, A. M. (2017). Canu: scalable and accurate long-read assembly via adaptive k-mer weighting and repeat separation. Genome Res. 27, 722–736. doi: 10.1101/gr.215087.116
Kurata, Y. (1984). Crown-of-thorns starfish of Ogasawara Island [in Japanese]. Mar. Park Inf. 61, 7–9.
Langmead, B., and Salzberg, S. L. (2012). Fast gapped-read alignment with Bowtie 2. Nat. Methods 9, 357–359. doi: 10.1038/nmeth.1923
Li, H. (2018). Minimap2: pairwise alignment for nucleotide sequences. Bioinformatics 34, 3094–3100. doi: 10.1093/bioinformatics/bty191
Lischer, H. E. L., and Excoffier, L. (2012). PGDSpider: an automated data conversion tool for connecting population genetics and genomics programs. Bioinformatics 28, 298–299. doi: 10.1093/bioinformatics/btr642
Lucas, J. S. (1973). Reproductive and larval biology and Acanthaster planci (L.) in Great Barrier Reef. Micronesica 9, 197–203.
Marçais, G., and Kingsford, C. (2011). A fast, lock-free approach for efficient parallel counting of occurrences of k-mers. Bioinformatics 27, 764–770. doi: 10.1093/bioinformatics/btr011
Marko, P. B., and Hart, M. W. (2018). “Genetic analysis of larval dispersal, gene flow, and connectivity,” in Evolutionary Ecology of Marine Invertebrate Larvae (Oxford: Oxford University Press), 165–166.
Martin, M. (2011). Cutadapt removes adapter sequences from high-throughput sequencing reads. EMBnet J. 17:10. doi: 10.14806/ej.17.1.200
McCallum, H. I. (1987). Predator regulation of Acanthaster planci. J. Theor. Biol. 127, 207–220. doi: 10.1016/S0022-5193(87)80131-5
Meeker, N. D., Hutchinson, S. A., Ho, L., and Trede, N. S. (2007). Method for isolation of PCR-ready genomic DNA from zebrafish tissues. Biotechniques 43, 610, 612, 614. doi: 10.2144/000112619
Meirmans, P. G. (2020). GenoDive version 3.0: easy-to-use software for the analysis of genetic data of diploids and polyploids. Mol. Ecol. Resour. 20, 1126–1131. doi: 10.1111/1755-0998.13145
Miyake, Y., Kimura, S., Kawamura, T., Horii, T., Kurogi, H., and Kitagawa, T. (2009). Simulating larval dispersal processes for abalone using a coupled particle-tracking and hydrodynamic model: implications for refugium design. Mar. Ecol. Prog. Ser. 387, 205–222. doi: 10.3354/meps08086
Nakabayashi, A., Yamakita, T., Nakamura, T., Aizawa, H., Kitano, Y. F., Iguchi, A., et al. (2019). The potential role of temperate Japanese regions as refugia for the coral Acropora hyacinthus in the face of climate change. Sci. Rep. 9:1892. doi: 10.1038/s41598-018-38333-5
Nakajima, Y., Nishikawa, A., Iguchi, A., and Sakai, K. (2012). Regional genetic differentiation among northern high-latitude island populations of a broadcast-spawning coral. Coral Reefs 31, 1125–1133. doi: 10.1007/s00338-012-0932-x
Narum, S. R., and Hess, J. E. (2011). Comparison of FST outlier tests for SNP loci under selection. Mol. Ecol. Resour. 11, (Suppl. 1) 184–194. doi: 10.1111/j.1755-0998.2011.02987.x
Nash, W. J., Goddard, M., and Lucas, J. S. (1988). Population genetic studies of the crown-of-thorns starfish, Acanthaster planci (L.), in the Great Barrier Reef region. Coral Reefs 7, 11–18. doi: 10.1007/BF00301976
Okaji, K., Ogasawara, K., Yamakita, E., Kitamura, M., Kumagai, N., Nakatomi, N., et al. (2019). Comprehensive management program of crown-of-thorns starfish outbreaks in Okinawa [in Japanese]. J. Jpn. Coral Reef Soc. 21, 91–110. doi: 10.3755/jcrs.21.91
Paris, C. B., Helgers, J., van Sebille, E., and Srinivasan, A. (2013). Connectivity modeling system: a probabilistic modeling tool for the multi-scale tracking of biotic and abiotic variability in the ocean. Environ. Model. Softw. 42, 47–54. doi: 10.1016/j.envsoft.2012.12.006
Peakall, R., and Smouse, P. E. (2012). GenAlEx 6.5: genetic analysis in excel. Population genetic software for teaching and research: an update. Bioinformatics 28, 2537–2539. doi: 10.1093/bioinformatics/bts460
Pratchett, M. S., Caballes, C. F., Rivera-Posada, J., and Sweatman, H. P. A. (2014). Limits to understanding and managing outbreaks of crown-of-thorns starfish (Acanthaster spp.). Oceanogr. Mar. Biol. 52, 133–200. doi: 10.1201/b17143-4
Pratchett, M. S., Caballes, C. F., Wilmes, J. C., Matthews, S., Mellin, C., Sweatman, H., et al. (2017). Thirty years of research on crown-of-thorns starfish (1986–2016): scientific advances and emerging opportunities. Diversity 9:41. doi: 10.3390/d9040041
Pritchard, J. K., Stephens, M., and Donnelly, P. (2000). Inference of population structure using multilocus genotype data. Genetics 155, 945–959. doi: 10.1093/genetics/155.2.945
Ranallo-Benavidez, T. R., Jaron, K. S., and Schatz, M. C. (2020). GenomeScope 2.0 and Smudgeplot for reference-free profiling of polyploid genomes. Nat. Commun. 11:1432. doi: 10.1038/s41467-020-14998-3
Rochette, N. C., and Catchen, J. M. (2017). Deriving genotypes from RAD-seq short-read data using Stacks. Nat. Protoc. 12, 2640–2659. doi: 10.1038/nprot.2017.123
Rogers, J. G. D., Pláganyi, ÉE., and Babcock, R. C. (2017). Aggregation, Allee effects and critical thresholds for the management of the crown-of-thorns starfish Acanthaster planci. Mar. Ecol. Prog. Ser. 578, 99–114. doi: 10.3354/meps12252
Scandol, J. P., and James, M. K. (1992). Hydrodynamics and larval dispersal: a population model of Acanthaster planci on the Great Barrier Reef. Mar. Freshw. Res. 43, 583–595. doi: 10.1071/MF9920583
Schunter, C., Carreras-Carbonell, J., Macpherson, E., Tintoré, J., Vidal-Vijande, E., Pascual, A., et al. (2011). Matching genetics with oceanography: directional gene flow in a Mediterranean fish species. Mol. Ecol. 20, 5167–5181. doi: 10.1111/j.1365-294X.2011.05355.x
Seppey, M., Manni, M., and Zdobnov, E. M. (2019). BUSCO: assessing genome assembly and annotation completeness. Methods Mol. Biol. 1962, 227–245. doi: 10.1007/978-1-4939-9173-0_14
Shanks, A. L. (2009). Pelagic larval duration and dispersal distance revisited. Biol. Bull. 216, 373–385. doi: 10.1086/BBLv216n3p373
Storlazzi, C. D., van Ormondt, M., Chen, Y.-L., and Elias, E. P. L. (2017). Modeling fine-scale coral larval dispersal and interisland connectivity to help designate mutually supporting coral reef marine protected areas: insights from Maui Nui, Hawaii. Front. Mar. Sci. 4:381. doi: 10.3389/fmars.2017.00381
Sunday, J. M., Popovic, I., Palen, W. J., Foreman, M. G. G., and Hart, M. W. (2014). Ocean circulation model predicts high genetic structure observed in a long-lived pelagic developer. Mol. Ecol. 23, 5036–5047. doi: 10.1111/mec.12924
Suyama, Y., and Matsuki, Y. (2015). MIG-seq: an effective PCR-based method for genome-wide single-nucleotide polymorphism genotyping using the next-generation sequencing platform. Sci. Rep. 5:16963. doi: 10.1038/srep16963
Suzuki, G., Yasuda, N., Ikehara, K., Fukuoka, K., Kameda, T., Kai, S., et al. (2016). Detection of a high-density Brachiolaria-stage larval population of crown-of-thorns sea star (Acanthaster planci) in Sekisei Lagoon (Okinawa, Japan). Diversity 8:9. doi: 10.3390/d8020009
Takata, K., Taninaka, H., Nonaka, M., Iwase, F., Kikuchi, T., Suyama, Y., et al. (2019). Multiplexed ISSR genotyping by sequencing distinguishes two precious coral species (Anthozoa: Octocorallia: Coralliidae) that share a mitochondrial haplotype. PeerJ 7:e7769. doi: 10.7717/peerj.7769
Taninaka, H., Bernardo, L. P. C., Saito, Y., Nagai, S., Ueno, M., Kitano, Y. F., et al. (2019). Limited fine-scale larval dispersal of the threatened brooding corals Heliopora spp. as evidenced by population genetics and numerical simulation. Conserv. Genet. 20, 1449–1463. doi: 10.1007/s10592-019-01228-7
Timmers, M. A., Andrews, K. R., Bird, C. E., de Maintenton, M. J., Brainard, R. E., and Toonen, R. J. (2011). Widespread dispersal of the crown-of-thorns sea star, Acanthaster planci, across the Hawaiian archipelago and Johnston Atoll. Mar. Sci. 2011:934269. doi: 10.1155/2011/934269
Timmers, M. A., Bird, C. E., Skillings, D. J., Smouse, P. E., and Toonen, R. J. (2012). There’s no place like home: crown-of-thorns outbreaks in the central Pacific are regionally derived and independent events. PLoS One 7:e31159. doi: 10.1371/journal.pone.0031159
Tusso, S., Morcinek, K., Vogler, C., Schupp, P. J., Caballes, C. F., Vargas, S., et al. (2016). Genetic structure of the crown-of-thorns seastar in the Pacific Ocean, with focus on Guam. PeerJ 4:e1970. doi: 10.7717/peerj.1970
Uthicke, S., Doyle, J., Duggan, S., Yasuda, N., and McKinnon, A. D. (2015). Outbreak of coral-eating crown-of-thorns creates continuous cloud of larvae over 320 km of the Great Barrier Reef. Sci. Rep. 5:16885. doi: 10.1038/srep16885
Vogler, C., Benzie, J. A. H., Tenggardjaja, K., Ambariyanto, Barber, P. H., and Wörheide, G. (2013). Phylogeography of the crown-of-thorns starfish: genetic structure within the Pacific species. Coral Reefs 32, 515–525. doi: 10.1007/s00338-012-1003-z
Wada, N., Yuasa, H., Kajitani, R., Gotoh, Y., Ogura, Y., Yoshimura, D., et al. (2020). A ubiquitous subcuticular bacterial symbiont of a coral predator, the crown-of-thorns starfish, in the Indo-Pacific. Microbiome 8:123. doi: 10.1186/s40168-020-00880-3
Walker, B. J., Abeel, T., Shea, T., Priest, M., Abouelliel, A., Sakthikumar, S., et al. (2014). Pilon: an integrated tool for comprehensive microbial variant detection and genome assembly improvement. PLoS One 9:e112963. doi: 10.1371/journal.pone.0112963
White, C., Selkoe, K. A., Watson, J., Siegel, D. A., Zacherl, D. C., and Toonen, R. J. (2010). Ocean currents help explain population genetic structure. Proc. Biol. Sci. 277, 1685–1694. doi: 10.1098/rspb.2009.2214
White, J. W., Carr, M. H., Caselle, J. E., Palumbi, S. R., Warner, R. R., Menge, B. A., et al. (2019). Empirical approaches to measure connectivity. Oceanography 32, 60–61. doi: 10.5670/oceanog.2019.311
Yamaguchi, M. (1977). Larval behavior and geographic distribution of coral reef asteroids in the Indo-west Pacific. Micronesica Ser. 13, 283–296.
Yasuda, N. (2018). “Distribution expansion and historical population outbreak patterns of crown-of-thorns starfish, Acanthaster planci sensu lato, in Japan from 1912 to 2015,” in Coral Reefs of the World, eds A. Iguchi and C. Hongo (Singapore: Springer), 125–148. doi: 10.1007/978-981-10-6473-9_9
Yasuda, N., Kajiwara, K., Nagai, S., Ikehara, K., and Nadaoka, K. (2015a). First report of field sampling and identification of crown-of-thorns starfish larvae. J. Coral Reef Stud. 17, 15–16. doi: 10.3755/galaxea.17.15
Yasuda, N., Taquet, C., Nagai, S., Yoshida, T., and Adjeroud, M. (2015b). Genetic connectivity of the coral-eating sea star Acanthaster planci during the severe outbreak of 2006–2009 in the Society Islands, French Polynesia. Mar. Ecol. 36, 668–678. doi: 10.1111/maec.12175
Yasuda, N., Nagai, S., Hamaguchi, M., Okaji, K., Gérard, K., and Nadaoka, K. (2009). Gene flow of Acanthaster planci (L.) in relation to ocean currents revealed by microsatellite analysis. Mol. Ecol. 18, 1574–1590. doi: 10.1111/j.1365-294X.2009.04133.x
Keywords: crown-of-thorn starfish, Kuroshio Current, larval dispersal, Ogasawara (Bonin) Islands, population genetic analyses, population genetics, secondary outbreak, oceanographic dispersal simulation
Citation: Horoiwa M, Nakamura T, Yuasa H, Kajitani R, Ameda Y, Sasaki T, Taninaka H, Kikuchi T, Yamakita T, Toyoda A, Itoh T and Yasuda N (2022) Integrated Population Genomic Analysis and Numerical Simulation to Estimate Larval Dispersal of Acanthaster cf. solaris Between Ogasawara and Other Japanese Regions. Front. Mar. Sci. 8:688139. doi: 10.3389/fmars.2021.688139
Received: 30 March 2021; Accepted: 07 December 2021;
Published: 17 January 2022.
Edited by:
Charles Alan Jacoby, St. Johns River Water Management District, United StatesReviewed by:
Maria Byrne, The University of Sydney, AustraliaGretchen Goodbody-Gringley, Central Caribbean Marine Institute, Cayman Islands
Copyright © 2022 Horoiwa, Nakamura, Yuasa, Kajitani, Ameda, Sasaki, Taninaka, Kikuchi, Yamakita, Toyoda, Itoh and Yasuda. This is an open-access article distributed under the terms of the Creative Commons Attribution License (CC BY). The use, distribution or reproduction in other forums is permitted, provided the original author(s) and the copyright owner(s) are credited and that the original publication in this journal is cited, in accordance with accepted academic practice. No use, distribution or reproduction is permitted which does not comply with these terms.
*Correspondence: Nina Yasuda, nina27@cc.miyazaki-u.ac.jp