- 1Faculdade de Ciências, MARE – Marine and Environmental Sciences Centre, Universidade de Lisboa, Lisbon, Portugal
- 2Departamento de Biología Vegetal y Ecología, Facultad de Biología, Universidad de Sevilla, Seville, Spain
- 3Department of Botany, Institute for Coastal and Marine Research, Nelson Mandela University, Port Elizabeth, South Africa
- 4Department of Science and Innovation, National Research Foundation, Research Chair in Shallow Water Ecosystems, Nelson Mandela University, Port Elizabeth, South Africa
- 5Departamento de Biologia Vegetal, Faculdade de Ciências, Universidade de Lisboa, Lisbon, Portugal
- 6Departamento de Biologia Animal, Faculdade de Ciências, Universidade de Lisboa, Lisbon, Portugal
- 7Southern Seas Ecology Laboratories, School of Biological Sciences, The University of Adelaide, Adelaide, SA, Australia
Coastal areas host some of the planet’s most productive ecosystems, providing life-sustaining ecological services and several benefits to humankind, while also being some of the most threatened areas (e.g., by globalization, climate change, and biological invasion). Salt marshes are coastal habitats with a key role in food and shelter provisioning, sediment deposition, nutrient cycling and carbon storage. Spartina spp. is a genus of grass halophytes which occurs in salt marshes worldwide, and includes species with different invasive potential. We evaluated the effect of climate change in the distribution and invasion potential of five Spartina species (S. anglica, S. alterniflora, S. densiflora, S. patens, and S. maritima) at a global scale. Species distribution models (SDMs) were applied on species occurrence data and atmospheric environmental predictors (WorldClim 2.1) to project potential changes in habitat suitability and associated changes in distribution and species co-occurrence until the end of the century, across four Shared Socioeconomic Pathway scenarios (i.e., SSP1-2.6 to SSP5-8.5). Projections showed a global trend for increasing species co-occurrence, with a general range expansion potentiated by increasing pathway severity. This study suggests that Spartina species can potentially benefit from climate change, predicting poleward expansions in the Northern Hemisphere for most species, with results pointing at increased conflict and invasion potential in Northern Europe and East Asian shorelines, already under strong invasive pressure. S. anglica is projected to remain a successful invader, with more severe scenarios likely favoring greater expansions. S. alterniflora exhibits very low expansion comparatively, despite exhibiting the same northward distribution shift. SSP1-2.6 produced the smallest change to species co-occurrence, suggesting a smaller potential for invasion-related conflicts, although still registering a potential net expansion for the Genus. Despite their limitations, SDMs can help establish general trends in climate change ecology and inform policymakers and environmental agents to ensure the correct management of these habitats and, ultimately, ecosystems.
Introduction
Coastal zones present some of the planet’s most productive ecosystems, even though they occupy a relatively small percentage of total land and ocean extension (Reid, 2005; Himes-Cornell et al., 2018). These land-ocean transitional habitats harbor vegetated assemblages that provide a vast array of life-sustaining ecological services and several benefits to humankind (Barbier, 2013; Himes-Cornell et al., 2018), and include salt marshes, mangroves, and seagrass beds, which are known to play a key role in large scale biogeochemical processes such as carbon sequestration and nutrient cycling (Duarte et al., 2005, 2021; Mueller et al., 2019). Considering the proximity of these ecosystems to human-related activities, it is not surprising that coastal areas include some of the world’s most threatened ecosystems, with studies showing that nearly half of salt marshes, 35% of mangroves and 29% of seagrass beds have been lost in the past 50 years alone (Himes-Cornell et al., 2018). Salt marshes are distributed worldwide, occurring from polar to tropical regions (Mcowen et al., 2017), and establish in low-energy intertidal zones located on the fringes of the inner areas of bays and estuaries (Leonardi et al., 2018). This habitat type typically consists of assemblages between halophytic (i.e., salt-tolerant) herbs, grasses, and low shrubs, which are adapted to regular or occasional tidal immersion, as well as a great diversity of land and marine wildlife (Mcowen et al., 2017). It also plays a key role providing a wide array of ecosystem services such as habitat provision to migratory birds and vast plant and animal assemblages (Greenberg et al., 2014); sequestration of carbon and other pollutants (Duarte et al., 2010, 2021); storage of sediments and nutrients (Duarte et al., 2013, 2014); water purification, storm surge, and flood attenuation (Zedler and Kercher, 2005; Donatelli et al., 2018; Leonardi et al., 2018).
The proximity of these ecosystems to urban settlements means that salt marshes are highly vulnerable to increased pressure from anthropogenic sources (Mcowen et al., 2017; Tonti et al., 2018; Curado et al., 2020). Due to global warming, high rates of environmental degradation, and rising sea levels, together with encroachment by invasive species have increasingly being reported (Duarte et al., 2015; Himes-Cornell et al., 2018; Curado et al., 2020). Indeed, salt marshes and other coastal wetlands are among some of the ecosystem types most affected by biological invasions (Gedan et al., 2009; Vilà et al., 2011). The spread of invasive species can have broad-scale impacts in these areas, affecting community dynamics and physically changing the marsh structure, potentially reducing biological diversity, and permanently altering their ecological functions and services (Gedan et al., 2009).
Cordgrasses (of the Genus Spartina, Poaceae), are a group of halophyte species widespread in salt marshes around the world (Castillo et al., 2017; Bortolus et al., 2019). These grasses colonize intertidal mudflats and are known to be important ecosystem engineers due to their ability to stabilize sediments and contribute to salt marsh formation (van Hulzen et al., 2007; Vu et al., 2017). Indeed, this group is of great importance, since these species facilitate ecological succession, and provide marshes with the spatial structure needed to promote high biodiversity levels (Castillo et al., 2017). However, cordgrasses are also prolific invasive halophytes (Strong and Ayres, 2013; Castillo et al., 2017). Due to their physiological characteristics [e.g., root systems adapted to soil inundation and low oxygen levels (Castillo et al., 2017)], species of the Genus Spartina can easily dominate frequently flooded tidal flats (Pennings et al., 2005). Additionally, cordgrasses propagate through both sexual and clonal reproduction. While clonal growth strategy partially determines the invasive potential of this group of halophyte plants (Castillo et al., 2016), seeds, and rhizome propagules differing in size, resource requirements, and genetic identity result in different invasive potential across species (Castillo et al., 2017). Together with biomass accumulation, both aspects are responsible for invasive Spartina species overgrowing native salt marshes, leading to significant decreases in plant and fauna abundance and diversity, as well as carrying the potential to hybridize with native Spartina species (Strong and Ayres, 2013). Moreover, the presence of these invasive Spartina species is also recognized to have severe impacts on the salt marsh ecosystem services, such as its important role in the natural remediation of transitional ecosystems (Human et al., 2020).
Some areas are known to be heavily invaded by cordgrass [e.g., the Chinese coast (Strong and Ayres, 2013)], with records of these invasions going back several decades, or even centuries (Castillo et al., 2018). Some of the most studied invasive cordgrass species include the common cordgrass, Spartina anglica (Hubb); the smooth cordgrass, Spartina alterniflora (Loisel); the austral cordgrass, Spartina densiflora Brongn; and the salt meadow cordgrass, Spartina patens (Aiton) Muhl. The common cordgrass, S. anglica, is a highly aggressive non-native species (Strong and Ayres, 2013). This species emerged through allopolyploid speciation (Guenegou et al., 1988; Ainouche et al., 2004), originating from the known hybrid S. × townsendii – the F1 hybrid of Spartina maritima and S. alterniflora (Strong and Ayres, 2013) and is endemic to the British Islands. Despite having been shown to have lower growth performance and competitive ability when compared to other invasive cordgrasses (such as S. alterniflora) in some areas, S. anglica has spread from the coasts of Europe to North and South American coastlines, to China, and even to the Australian and New Zealand shore (Bortolus et al., 2019),being responsible for some of the largest continent-scale invasion events in Asia and in the Americas, with major ecological and socioeconomic impacts (Bortolus et al., 2019).
The smooth cordgrass, S. alterniflora, is present in temperate and subtropical salt marsh habitats (Bortolus et al., 2019), being native to the Atlantic and Gulf coasts of North and South America (Kirwan et al., 2009) and dominating intertidal marshes (Zheng et al., 2018). Like S. anglica, S. alterniflora is also an invasive cordgrass, both inadvertently and deliberately introduced in coastal wetlands worldwide (Zhang et al., 2017; Bortolus et al., 2019). Over the past centuries, reports of invasion of European coastlines, mainly in France and Southern England (Bertness, 1991; Baumel et al., 2003), in the Mediterranean (Hessini et al., 2009), China, and New Zealand (Feng et al., 2017; Zheng et al., 2018) have been increasing; and S. alterniflora is now very well established in most of these areas due to its high adaptability and propagation ability (Zuo et al., 2012; Zhang et al., 2017). The saltmeadow cordgrass, S. patens, is a native cordgrass to brackish salt marshes and coastal dunes of the Atlantic and Gulf coasts of North America (Bertness, 1991). This species has now a vast non-indigenous distribution in coastlines of Europe, along the western Mediterranean coast and the Atlantic coast of the Iberian Peninsula (Baumel et al., 2016; Duarte et al., 2018). Finally, the denseflower cordgrass, S. densiflora, is native to the South American continent and has spread to Europe, in particular to the region of the southwestern Iberian Peninsula (Nieva et al., 2001; Bortolus, 2006), Morocco, Mexico, and the western coast of the United States (Bortolus, 2006). The species is known to have hybridized with the small cordgrass (S. maritima) in the salt marshes of Southern Iberian Peninsula (Castillo et al., 2018) and is a case study for the potential latency of the invasion process of plant species followed by unprecedented rates of spreading (Duarte et al., 2018; Bortolus et al., 2019). An example of endemic cordgrass species suffering from invasion from other sister species is that of S. maritima. This is a known native of western and southern European shores (from the Netherlands to the south of England and Ireland), and is the only cordgrass species that is native to the salt marshes of continental Europe (Castillo et al., 2018). It also occurs along the Mediterranean Sea coastlines to the Atlantic coast of Morocco, and two separated populations in the Atlantic coasts of Namibia and South Africa (Bortolus et al., 2019). When competing with other cordgrass invasive species, S. maritima is known to have suffered hybridization at least twice: (1) with S. alterniflora, originating S. × townsendii, which later led to S. anglica; and (2) with S. densiflora; which poses an increased threat of replacement in its native habitats.
The potential for climate change together with biological invasions to affect cordgrass species distribution is of particular interest. The saltmeadow cordgrass (S. patens) is rapidly disappearing from wetlands in the coastal marshes of the United States due to current inundation patterns, and accelerated sea-level rise will likely reduce this species’ persistence (Watson et al., 2016). However, temperature rise does not seem to significantly impact S. patens or its role as a foundational species (Gedan and Bertness, 2010; Duarte et al., 2016). The hybrid formation has been shown to change in response to changes in atmospheric variables. Specifically, the establishment of reciprocal hybrids between the native S. maritima and the invasive S. densiflora in SW Iberian Peninsula was shown to change with air temperature and rainfall, with a negative effect on the native and endangered species S. maritima (Duarte et al., 2015; Gallego-Tévar et al., 2019). Reciprocal transplant trials also revealed that S. alterniflora might be stressed by future warming, leading to decreased belowground allocation and peat accumulation (Crosby et al., 2017).
An accurate description of a species’ ecological and geographical distribution is fundamental for understanding existing patterns of biodiversity, and the processes responsible for shaping them (Wisz et al., 2008). Given the differential effects from future conditions in cordgrass fitness, it is important to predict how cordgrass species might behave under climate change scenarios, in particular regarding changes to their potential distribution. By analyzing predicted distributions, it is possible to recognize where species conflicts may emerge, and identify potential areas with higher potential of invasion. This has become a very important part of conservation planning in the past decades (Elith et al., 2006), with a wide array of modeling techniques being applied in predicting species or community distribution, particularly for plant species (Bagheri et al., 2017). Species distribution models (SDMs) have seen a steep rise in their development and use (Zimmermann et al., 2010). While bounded by a set of assumptions and limitations that should be considered during interpretation (Araújo and Pearson, 2005; Araújo and Guisan, 2006; Heikkinen et al., 2006; Fitzpatrick and Hargrove, 2009), these models are widely employed and offer a good framework for predicting species distributions and their changes, regarding large species assemblages and vast geographical spaces (Elith et al., 2006). This type of models has now been used for several decades to determine suitable habitats for species or communities, namely to evaluate the invasive potential of non-indigenous species and their proliferation (Peterson and Vieglais, 2001; Araújo and Rahbek, 2006; Jiménez-Valverde et al., 2011; García-Roselló et al., 2014) and project the potential effects of climate change on the distribution of species or communities (Araújo and Rahbek, 2006; Peterson and Soberón, 2012; García-Roselló et al., 2014). Notably, SDMs that incorporate future climate change predictions are considered an effective way to address some of the questions behind climate change effects on biodiversity (Sinclair et al., 2010). Given this context, the present study aims to evaluate the potential biogeographical impact of climate change on cordgrasses worldwide. Specifically, this study implements a series of SDM ensembles to estimate how future conditions may affect habitat suitability, species richness and colonization, and extinction susceptibility for five species of the Spartina Genus [S. anglica (Hubb), S. alterniflora (Loisel), S. densiflora Brongn, S. patens (Aiton) Muhl, and S. maritima (Curtis) Fernald] at a global scale. This analysis is based on the 30 year average of projections for atmospheric variables (monthly average maximum temperature; monthly average minimum temperature; and monthly average total precipitation). The analysis encompasses both an historical timeframe (early 2000s) and different future time-spans (i.e., 2021–2040, 2041–2060, 2061–2080, and 2081–2100), considering four of the Shared Socioeconomic Pathways (SSP1-2.6, 2-4.5, 3-7.0, and 5-8.5) of the Coupled Model Intercomparison Project phase 6 (CMIP6) (Tebaldi et al., 2021).
Materials and Methods
Species Data and Curation
The occurrence data used in this study was compiled from data collected from the Global Biodiversity Information Facility (GBIF), and from literature surveyed from the Web of Knowledge in the case of specific species: S. densiflora, S. maritima, and S. patens in the Iberian Peninsula (Caçador et al., 2013); and S. alterniflora in the coastline of China (Cheng et al., 2006; Gao et al., 2007; Liu et al., 2007; Zhang et al., 2010, 2020; Wang et al., 2013, 2015; Yang et al., 2013; Jin et al., 2016; Pang et al., 2017; Maebara et al., 2020; Xia et al., 2020; Yue et al., 2021). Occurrence from GBIF was collected for S. anglica [GBIF.org (14 October 2020) GBIF Occurrence1 ]; S. maritima [GBIF.org (14 October 2020) GBIF Occurrence2 ]; S. patens [GBIF.org (14 October 2020) GBIF Occurrence3 ]; S. densiflora [GBIF.org (14 October 2020) GBIF Occurrence4 ]; and finally S. alterniflora [GBIF.org (14 October 2020) GBIF Occurrence5 ]. A specific set of filters was used for all species in each dataset to limit the data retrieved. The applied filters included “Human Observation” and “Preserved specimen” (for the Basis of Record); “Has Coordinate” set as true; “Has Geospatial issue” set as false. The endemic status (i.e., endemic versus non-indigenous species) was also retrieved for each occurrence point. The occurrence dataset, together with the plotted dataset occurrences are present in Supplementary Material (see “Spartina_Rfull.csv” and “plotted_occur.zip,” respectively). The compiled dataset was then curated using R studio. First, the original dataframe was converted into a spatial polygon object, which was then restricted by removing all points outside the extent of the coastline inland-buffer shapefile. This restriction procedure of both species’ occurrences and environmental predictors was performed since SDMs must ideally restrict model calibration to accessible areas (Peterson and Soberón, 2012). Lastly, the inclusion of elevation enables model predictions not to occur in illogical areas (e.g., deep inland areas where the elevation and abiotic conditions are similar to the coastline and suitable for the species). Afterwards, the occurrence data was checked for missing (NA) values in Longitude and Latitude, and for the existence of duplicates for each species subset. Entries that matched these criteria were removed from the analysis at this point. The final dataframe was a subset for each species, and was converted into spatial polygon format for use in the SDM analysis. The post-curation number of valid entries for the analysis per species is provided in Supplementary Table 1. Lastly, a map of the global distribution of saltmarshes and mangroves (Figure 1C of the section “Results”) was also included, in order to allow the potential pinpoint of areas of pressure and potential expansion of the genus and the species with regards to the range of these two ecosystem types. This map was plotted in ArcMap v10.7.1., using two polygon layers obtained from Ocean Data Viewer6 from (1) the Global Distribution of Saltmarshes (Mcowen et al., 2017), and (2) the World Atlas of Mangroves (Spalding et al., 2010).
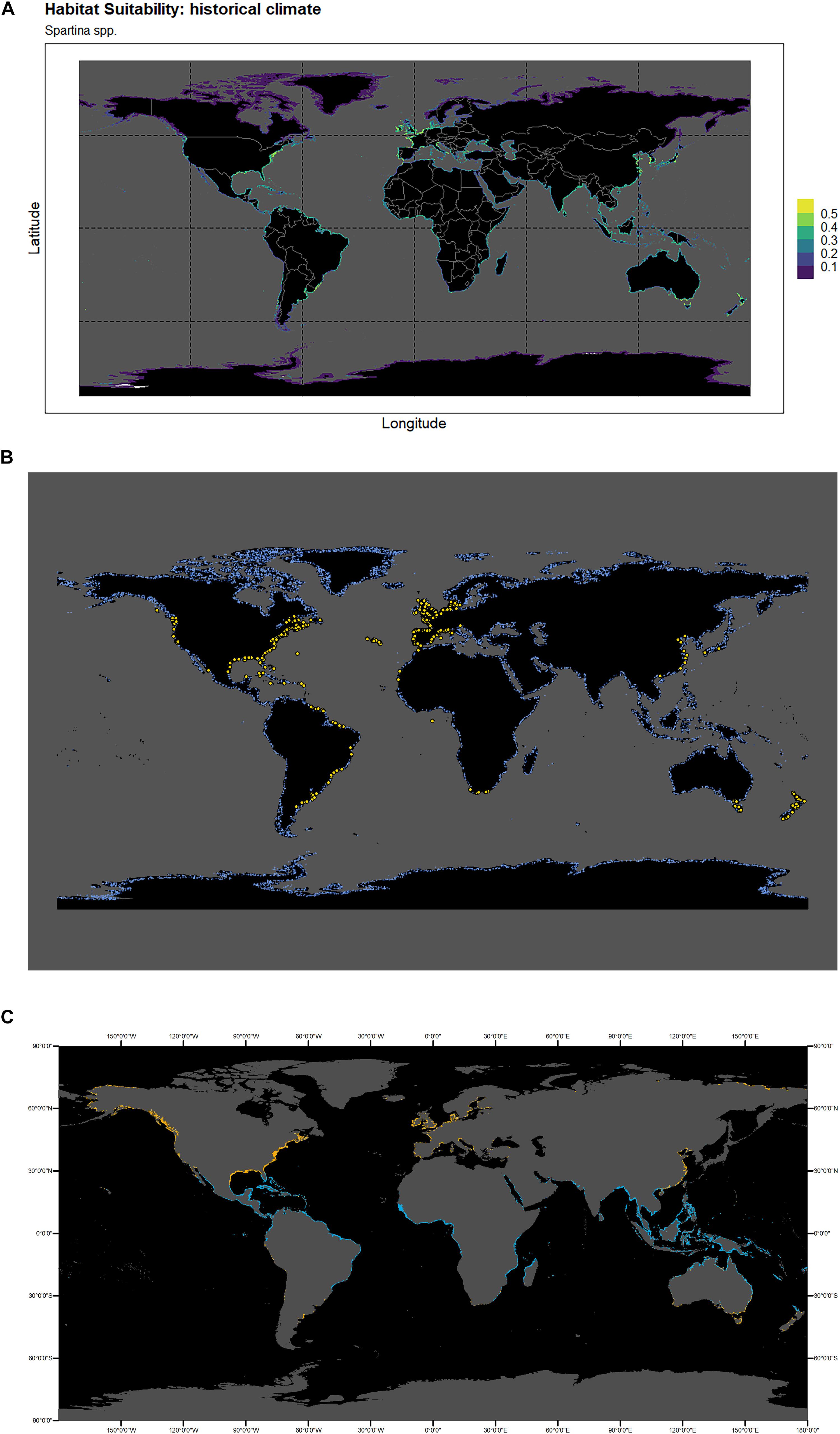
Figure 1. (A) Habitat suitability map for the historical climate conditions for the genus Spartina; and (B) Distribution of presence points of Spartina spp. in geographical space (blue), plotted from the data compiled from GBIF and the surveyed literature, and the randomly generated background pseudo-absences for the whole-genus analysis (white). (C) Worldwide distribution of saltmarshes (COR) and mangroves (COR), plotted in ArcMap v10.7.1., using two polygon layers obtained from Ocean Data Viewer (https://data.unep-wcmc.org) from (1) the Global Distribution of Saltmarshes (Mcowen et al., 2017), and (2) the World Atlas of Mangroves (Spalding et al., 2010).
Predictor Variables
For the present study, three atmospheric climate variables and one geographical variable were chosen as predictor variables. These included: (1) monthly average minimum temperature (°C); (2) monthly average maximum temperature (°C); (3) monthly total precipitation (mm); and finally, (4) elevation (m). All predictor variables were retrieved from the WorldClim database [version 2.17 (Fick and Hijmans, 2017)]. The choice of the three atmospheric climate variables was primarily based on the availability of said predictors on the WorldClim database for future periods (i.e., 2021–2040, 2041–2060, 2061–2080, and 2081–2100) as well as for historical climate data for use as a baseline (i.e., 1971–2000). WorldClim 2.1 provides downscaled data for these three variables for several Global Climate Models (GCM) of the CMIP6 (Tebaldi et al., 2021), and for the four high-priority scenarios which cover the range of possible pathways depending on socioeconomic choices. Specifically, these scenarios include: (1) SSP1-2.6 – which approximately corresponds to the previous scenario generation representative concentration pathway (RCP) 2.6, and assumes a “2°C scenario of the sustainability” SSP1 socioeconomic family; (2) SSP2-4.5 – approximately corresponding to the RCP-4.5, and referring to the “middle of the road” socioeconomic family SSP2; (3) SSP3-7.0 – which is a medium-high reference scenario within the “region-rivalry” socioeconomic family; and (4) SSP5-8.5 – which refers to a “high reference scenario” in a high fossil-fuel development world throughout the 21st century, marking the upper edge of the SSP scenarios (Meinshausen et al., 2020). For the historical period, a total of 12 GeoTiff layers were downloaded per variable from the WorldClim database, each referring to the average monthly values between 1970 and 2000. From these, the mean of the 12 layers per environmental variable was calculated to obtain the three atmospheric climate historical predictor layers. Concerning data for the future periods, downscaled monthly climate data was retrieved from the available GCMs at the time of compilation, specifically from the ones containing all the variables, time periods, and pathways selected. The resulting GeoTiff predictor layers were restricted using a 50 km inland buffer applied to a shapefile of the world coastline, downloaded from Natural Earth Data,8 with the intent of preventing SDM-predictions away from the coastal areas. This procedure was performed using ArcMap v10.7.1. Finally, the environmental predictor layers were stacked according to their period and SSP scenario to be used in the following SDM analysis. All variable layers collected from WorldClim and the shapefile used for geographical restriction were chosen at a spatial resolution of 10 min (i.e., ∼340 km2). Layer processing was performed in R studio software v1.3.959 (R Core Team, 2020), and the script is supplied in Supplementary Material (see “SDM_predictors_Rscript”).
Species Distribution Modeling
Species distribution analysis was performed using the “sdm” package (Naimi and Araújo, 2016) using the same procedures at both genus (Spartina spp.) and species level, with each species analyzed independently. First, an “sdmData” class object was created with each species, the historical climate environmental layer stack as explanatory variables, and two randomly obtained samples of the curated occurrence data for train and test data (75%). Given that the species occurrence dataframe included only presence data, an argument for background data of ten thousand (10,000) points per species using the method “gRandom” was employed, with removal of matching points, to generate pseudo-absence data (Barbet-Massin et al., 2012). This was performed since SDMs must account for absence data either with the incorporation of true data or pseudo-absence data (Peterson and Soberón, 2012); the sample size for randomly generated pseudo-absences was chosen based on Phillips and Dudík (2008). Specifically, the “gRandom” method randomly assigns absence points across the available geographical space, while the removal of matching points ensures that no pseudo-absence is placed over a known presence point. This “sdmData” object was then used for running the SDM analysis, using an ensemble of SDMs comprising generalized linear models (GLM; McCullagh and Nelder, 1989); random forest (RF; Breiman, 2001); and boosted regression tree (BRT; De’ath, 2007). In each case, four replicates with five permutations each were employed, using a train data of 70% and a test data of 30% for testing for model fit. For each model, the receiver operating characteristic curve (ROC) curves were plotted to evaluate model performance (see Supplementary Material “ROC.zip”). The mean performance (per species) using an independent test dataset is presented in Table 1. Also, for each model iteration in each analysis, the area under the curve (AUC), true skill statistic (TSS), and normalized mutual information (NMI) evaluation metrics were calculated, and each species’ predictor response curves plotted (see Supplementary Material “Model_Eval.zip,” “RespCurves.zip”). From these ensembles, the historical map of habitat suitability was plotted for each species and the genus. By applying a threshold (i.e., the mean model TSS criteria of model evaluation, “max (se + sp),” the respective maps of binomial probability of occurrence (0 or 1) were obtained. By using the “predict” function (Naimi and Araújo, 2016), the fitted models were used to generate future predictions with the future data layers (per time frame, per SSP scenario). From this process, at both the genus and species level, for each timeframe and each SSP, the habitat suitability plots (probability of occurrence) and the species presence plots (probability of presence/absence) were obtained. All resulting plots are supplied in Supplementary Material (see “SDM_outputs.zip”), together with the Rscripts for 2.2 and 2.3 (see “SDM_Rscript”).
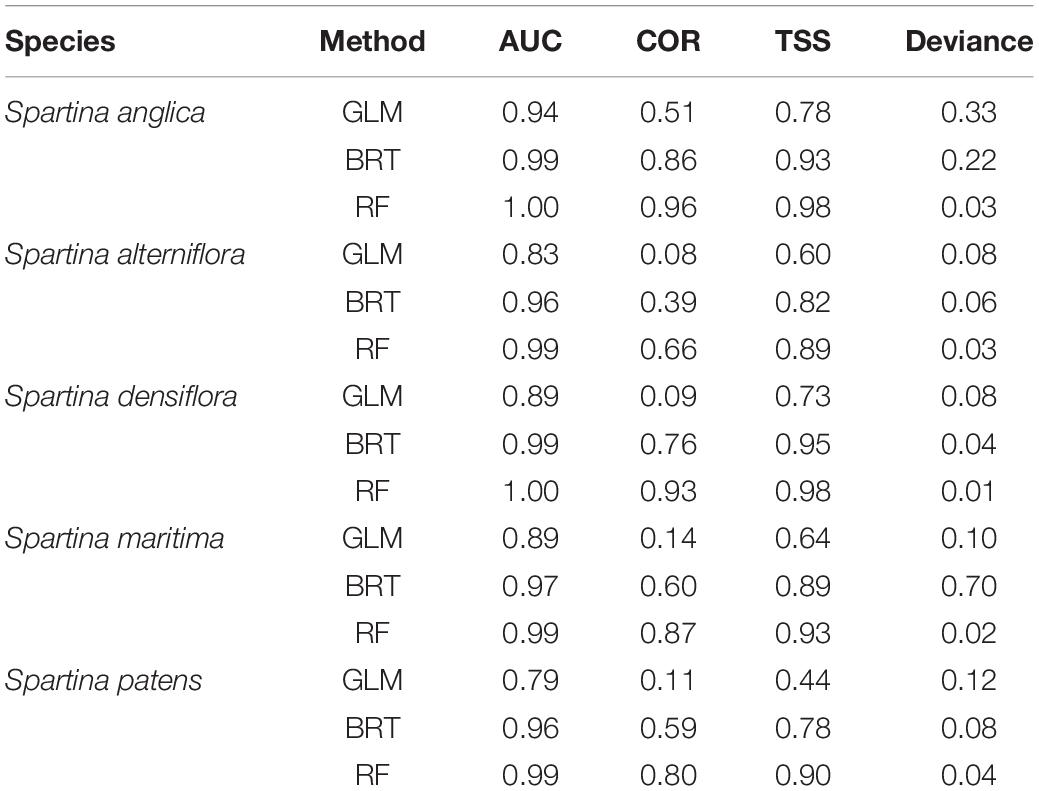
Table 1. Mean performance (per individually modeled species) of computed SDM model methods (GLM, generalized linear model; BRT, boosted regression tree; RF, random forest), using an independent test dataset.
Post-analysis
For each species and each SSP scenario, a set of post-analysis was performed on the resulting SDM outputs. Specifically, the change in habitat suitability (i.e., the difference between the predicted habitat suitability of a future period and the historical time) and the colonization/extinction potential (i.e., the same, but using the binomial presence/absence probability maps) were plotted. This provided an overview of the world areas in which conditions would improve or decay for each species and SSP scenario until the end of the century, while also following species potential colonization and extinction through all time points. From these maps, both the change in habitat suitability per SSP until 2100, and the change in presence and colonization/extinction potential for each SSP and future times were quantified. First, the habitat suitability net percentage change until 2100 was obtained by calculating the percentage of positive and negative cells from the habitat suitability change maps for each SSP between 2100 and historical times, for each species. These positive and negative percentages were then subtracted to calculate the net percentage change and, hence, the gain and loss of potential habitat suitability until the end of the century for each species. Second, to quantify both changes in the presence status of the genus/species, and changes in the potential colonization/extinction potential, cells of each raster map of probability of occurrence. Specifically, the frequency of cells with values above, equal, and below zero were counted in each map, and afterwards converted into percentages regarding the total number of raster cells in each map (see Supplementary Material archive “cell_count.zip”). The quantification of the potential colonization/extinction status allows the identification of areas where a certain species is projected to spread to or disappear from, as well as the total change in occurrence status globally, by calculating the net occurrence probability. These analyses were performed using the re-projection of the rasters into the Lambert Azimuthal equal-area projection, which preserves areas across the map’s extent, and allows for raster cells to hold equal weight, something that the standard Mercator projection used in the rest of the study does not allow.
Lastly, species overlap maps were plotted to identify areas of potential species co-occurrence and, as such, conflict and invasion/displacement potential. Specifically, for each time period and each SSP scenario, the presence/absence maps for each species were summed to obtain their respective worldwide species co-occurrence gradients. The potential change in species overlap was also plotted by subtracting each SSP scenario’s time period to the historical climate species richness map. The R scripts for the post analysis are present in Supplementary Material (see “Post_analysis_Rscript”).
Results
Historical Habitat Suitability, Distribution, and Richness
The output map of habitat suitability, the geographic distribution of compiled datapoints and randomly generated pseudo-absences, and the plotted worldwide distribution of saltmarshes and mangroves are present in Figures 1A–C. Higher suitability values were observed in temperate and sub-temperate areas, partially overlapping areas of known salt marsh distribution (Figure 1C) such as the North-eastern and South-eastern coastlines of the American continent (e.g., from the coast of Florida to Nova Scotia, and in the region north of the Amazon river’s basin and the mouth of Rio de la Plata); the English Channel and the North Sea coastlines of France and the Netherlands in Europe; South-eastern Australia coastlines in the Bass Strait and the Tasman Sea, and the Northern coastlines of New Zealand; and finally in the coastline of China and Japan in the areas of the Yellow Sea, the Sea of Japan, and South China Sea. Lower values were observed to higher latitudes typically associated with polar, subpolar, and higher latitude temperate areas, as well as in the Red Sea and Gulf of Aden, and the coastlines of Peru and the Gulf of California, and other areas typically associated to mangrove habitats.
Concerning potential species co-occurrence (Figure 2), most of the areas mentioned as having higher suitability of habitat for the entire genus correspond to areas where three or more species are predicted to co-occur historically (1971–2000). Occurrence “hotspots” (i.e., five species, in yellow) are predicted to potentially occur along the Eastern coastline of the United States; as well as in Europe, specifically in the Gulf of Biscay and the western coastline of the Iberian Peninsula; scattered areas around the Mediterranean sea; the mouth of Rio de la Plata and the Chilean coast in South America; Northern New Zealand and the Chinese coastline in the northern region of the Yellow sea. Also, four species can potentially co-occur in several areas worldwide, including the Gulf of Mexico; the Argentinian coastline South of Rio de la Plata; the Iberian Peninsula and the English and French coastlines of the Celtic Sea; the Indian coastline of the Bay of Bengal and the Gulf of Thailand and South China Sea; the Yellow Sea and the North-eastern Japanese coastline; and also in the coastlines of Papua New Guinea and South-eastern Australia. Most areas predicted to possess four or more species overlap the known distribution of salt marshes, although the model did predict the presence of high historical species richness in areas of current mangrove distribution, between approximately 12°N and 20°S such as the coast between Venezuela and French Guiana in South America; the Western and Eastern African coastlines, and Indonesia, Papua New Guinea, and Malaysia.
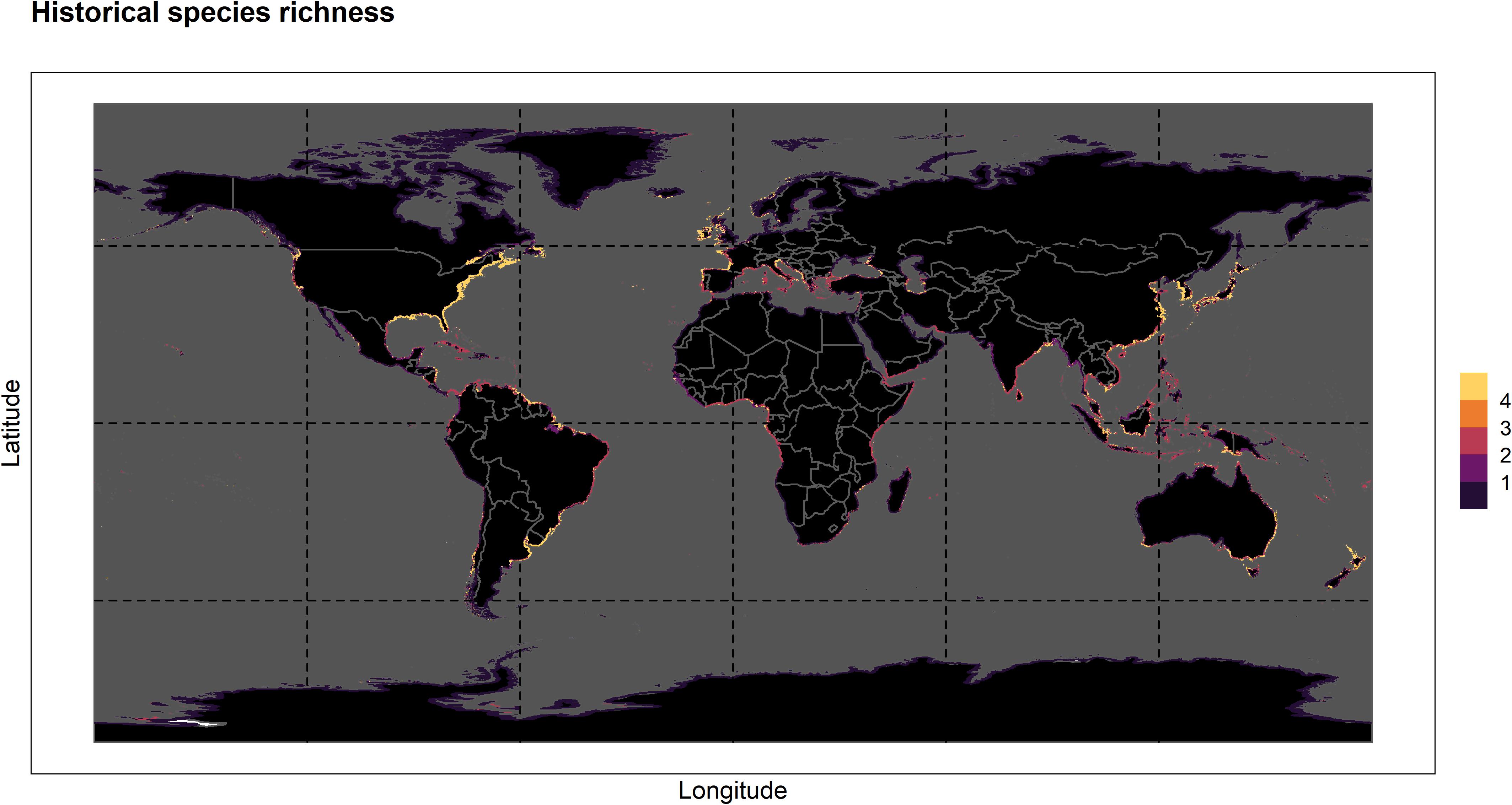
Figure 2. Potential historical (1971–2000) worldwide richness distribution of Spartina spp. species (i.e., S. alterniflora, S. anglica, S. densiflora, S. maritima, and S. patens) obtained from summing each species’ SDM-plotted historical presence and absence predictions.
Future Change in Habitat Suitability
The predicted potential change in habitat suitability until the year 2100 for the genus Spartina and the five species of interest is presented in Figure 3. Overall, there is a positive net change in suitability at the genus level, as well as for S. anglica and S. maritima; while this net change is negative for the remaining three species. Specifically, an increasing trend between shared socioeconomic pathway scenarios is observed for Spartina spp., which exhibits values ranging from the lowest net gain of 16.7% in SSP1-2.6, to increasingly higher values of 17.1% in SSP2-4.5, 23.7% in SSP3-7.0, and 24.9% in SSP5-8.5. Considering the five species per SSP scenario, for SSP1-2.6 the clear beneficiary is S. anglica, with an increase in suitability of just over 20%, followed by S. maritima (7.13%). On the negative end of the spectrum, S. densiflora loses the most net suitability (−14.7%), followed by S. alterniflora (−10.4%) and S. patens (just over 2%). In SSP-2-4.5, there seems to be an increase in positive net changes for all five species. Specifically, S. anglica increases up to approximately 21.8% in net suitability gain until 2100, and S. maritima registers a small increase to 4.3%. For the species on the negative end of the spectrum, their net values increase relative to SSP1-2.6, to −14.4% for S. densiflora, −8.3% for S. alterniflora, and finally −1.3% for S. patens. Under the SSP3-7.0 pathway scenario, the situation changes on several fronts. There is a decrease in the net suitability change for both species on the positive spectrum (to approximately 18.3% and under 3% for S. anglica and S. maritime, respectively), although both species continue to have a net gain in habitat suitability until the year 2100 in this scenario. Also, this scenario presumes a sharp decrease in suitability for the already negatively impacted S. patens (−9.0%) and again for S. alterniflora (−10.6%), while S. densiflora sees its best value at approximately −4%. Finally, in SSP5-8.5, S. anglica registers a net gain of approximately 21.5% in suitability, while S. maritima exhibits a value of 3.2%. On the negative end, S. densiflora exhibits a value of –5.3%, while S. alterniflora and S. patens show their lowest values comparatively to the other pathways (of −12.6 and −11.4%, respectively, in both cases). The Supplementary Material provides an animated version of habitat suitability over time for each species (see “HabitatGIFs.zip”).
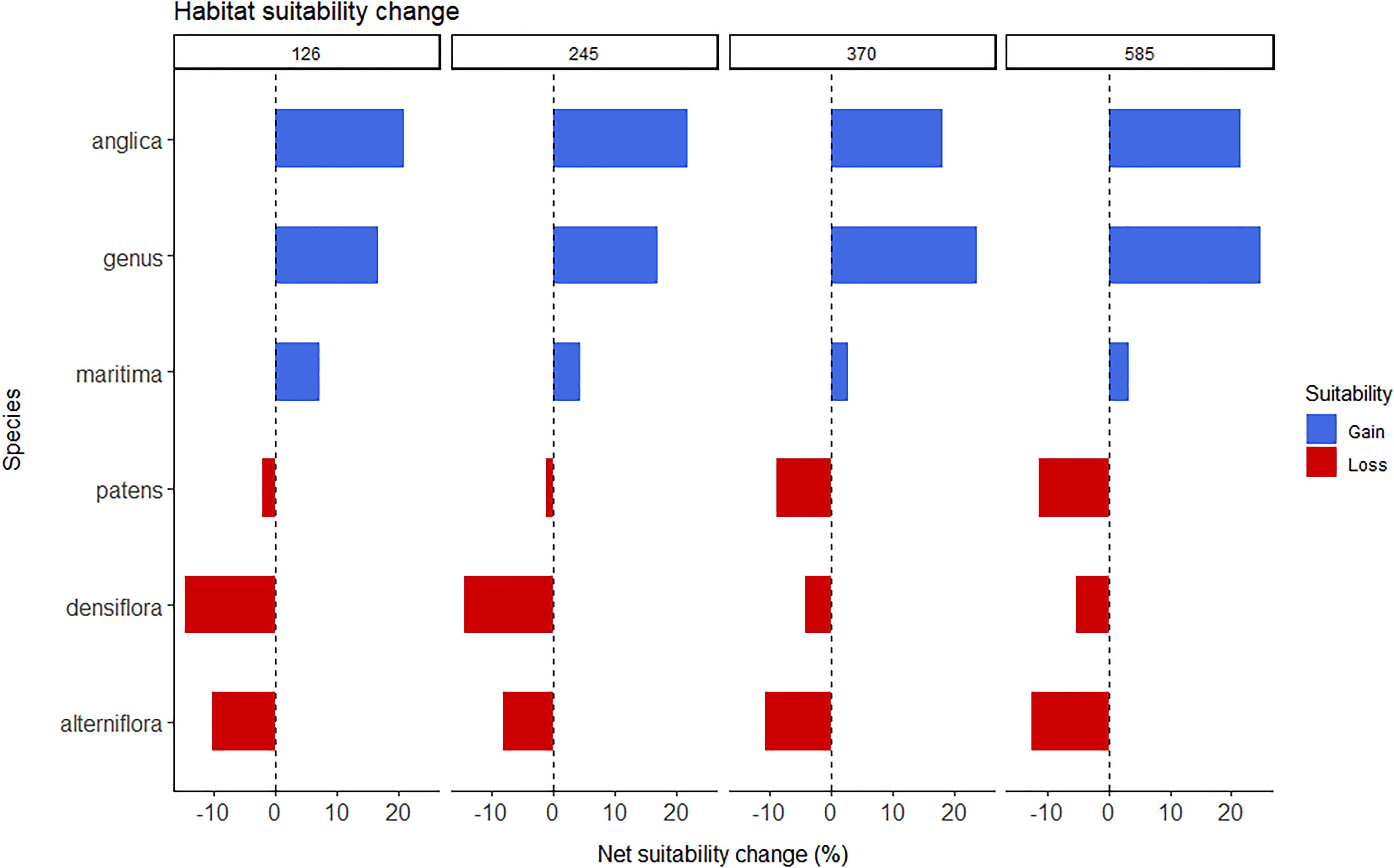
Figure 3. Net change in habitat suitability (%) until 2100, calculated from the difference between each species’ percentage gain and percentage loss in suitability between the 2081 and 2100 and the historical time periods for each CMIP6 Shared Socioeconomic pathway (i.e., SSP1-2.6, 2-4.5, 3-7.0, and 5-8.5). Presented values refer to each Spartina spp. species (i.e., S. alterniflora, S. anglica, S. densiflora, S. maritima, and S. patens) and for the whole genus.
Future Change in Occurrence Probability
Concerning predicted occurrence distribution, determined by the percentage of positive raster cells (i.e., signifying presence) in the binomial presence and absence maps, the results follow a similar pattern as before. Specifically, most species exhibit increasing values over time that are higher with increasing severity of the SSP scenario considered. For the whole genus (Figure 4A), the increase in proportion is higher for SSP3-7.0 and SSP5-8.5, with values starting at approximately 13% for all pathways in 2021–2040 and reaching approximately 17 and 18% for SSP3-7.0 and SSP5-8.5, respectively, by the end of the century. A similar increase is observed for S. maritima and S. patens (Figures 4E,F), with both species maintaining stable values until the end of the century under SSP1-2.6, and consequently increasing with SSP scenario (e.g., an increase of approximately 3.9 and 6.7% for S. maritima and S. patens, respectively, under SSP3-7.0, and of approximately 6.3 and 8.1%, respectively, under SSP5-8.5). In S. anglica (Figure 4B) the predicted occurrence distribution exhibits the largest increase by far with SSP, with increases of approximately 0.6 and 3.4% for SSP1-2.6 and SSP2-4.5, and of 7.6 and 10.7% for SSP3-7.0 and SSP-5.85, respectively. Finally, S. alterniflora and S. densiflora exhibit values that are not only very stable over time, but also very similar in what regards different SSP scenarios (Figures 4C,D). Specifically, the results show predicted increases of up to 1–2% over time for either pathway in both species. Refer to the Supplementary Material for an animated version of the occurrence plots for each species (see “PresenceGIFS.zip”).
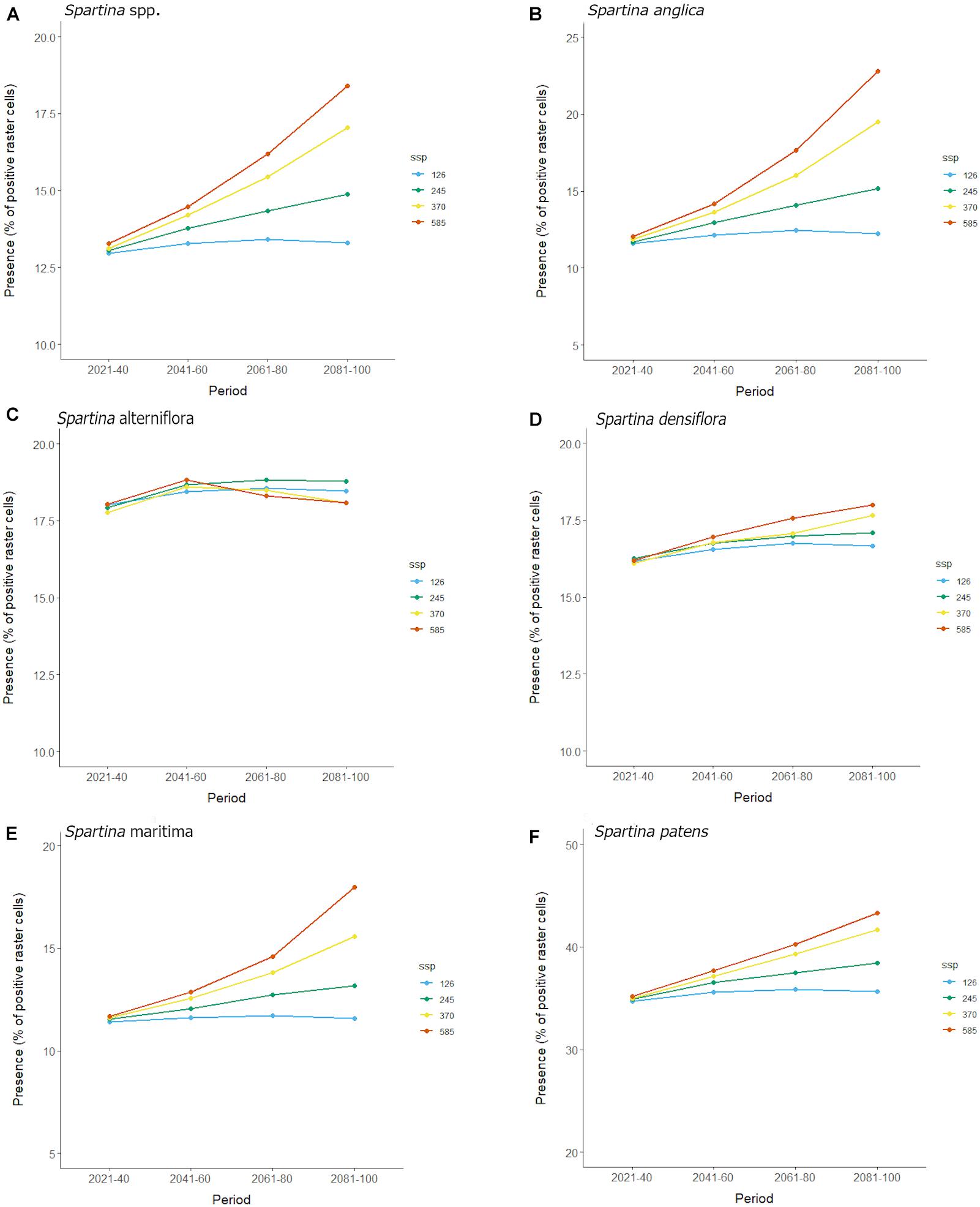
Figure 4. (A) Worldwide presence status (%) over time (until 2100) for the whole Spartina genus and (B) for species S. alterniflora, (C) for S. anglica, (D) for S. densiflora, (E) for S. maritima, and (F) for S. patens in each specific CMIP6 Shared Socioeconomic pathway (i.e., SSP1-2.6, 2-4.5, 3-7.0, and 5-8.5). Percentage of presence status was quantified as the proportion of positive (i.e., greater than 0) raster map cells.
Future Change in Colonization/Extinction Potential
Globally, increasing values of both colonization and extinction potential are observed over time at both genus and species level (Figure 5). Although all extinction potential values also increase with pathway severity, they are lower than those referring to potential colonization. Indeed, there is a clear tendency for increase over time and SSP for all species, with S. anglica and S. patens exhibiting the greatest increase in the proportion of raster cells for potential colonization, compared to potential extinction (Figures 5B,F, respectively). Although to a lesser extent, S. maritima, S. densiflora, and S. alterniflora also exhibit a similar pattern (Figures 5C–E), although for S. alterniflora and S. densiflora this increase is particularly small. For all species and the whole genus, the net value between the colonization and extinction potential is positive (Figure 6). In SSP1-2.6, the net value is extremely low for all species considered. Specifically, the lowest values occur for S. maritima and S. alterniflora (very close to 0% net value), with the remaining species registering values of approximately 1%, with S. anglica with the highest value. For SSP2-4.5, all species exhibit higher values comparatively, with S. anglica exhibiting an increase in approximately 3.4%, and S. densiflora now at the lower end with the lowest value. For SSP3-7.0 and SSP5-8.8, there is a further increase in all species’ net change values, except for S. alterniflora. In SSP3-7.0, the value almost doubles for all species compared to SSP2-4.5, except for S. alterniflora which decreases slightly. S. anglica exhibits approximately 7.6% of net gain in colonization potential, while S. patens reaches 6.7% with S. maritima not far-below, at 4%. Finally, in SSP5-8.5 all species, except for S. alterniflora, exhibit their highest values, with S. anglica reaching almost 11% net gain, and S. patens and S. maritima nearly 8.2 and 6.3%, respectively. Despite having its highest value in this pathway as well, S. densiflora falls short of a 2% net gain. Finally, S. alterniflora exhibits its lowest value, of under 0.05% net colonization, suggesting high extinction rates for this scenario.
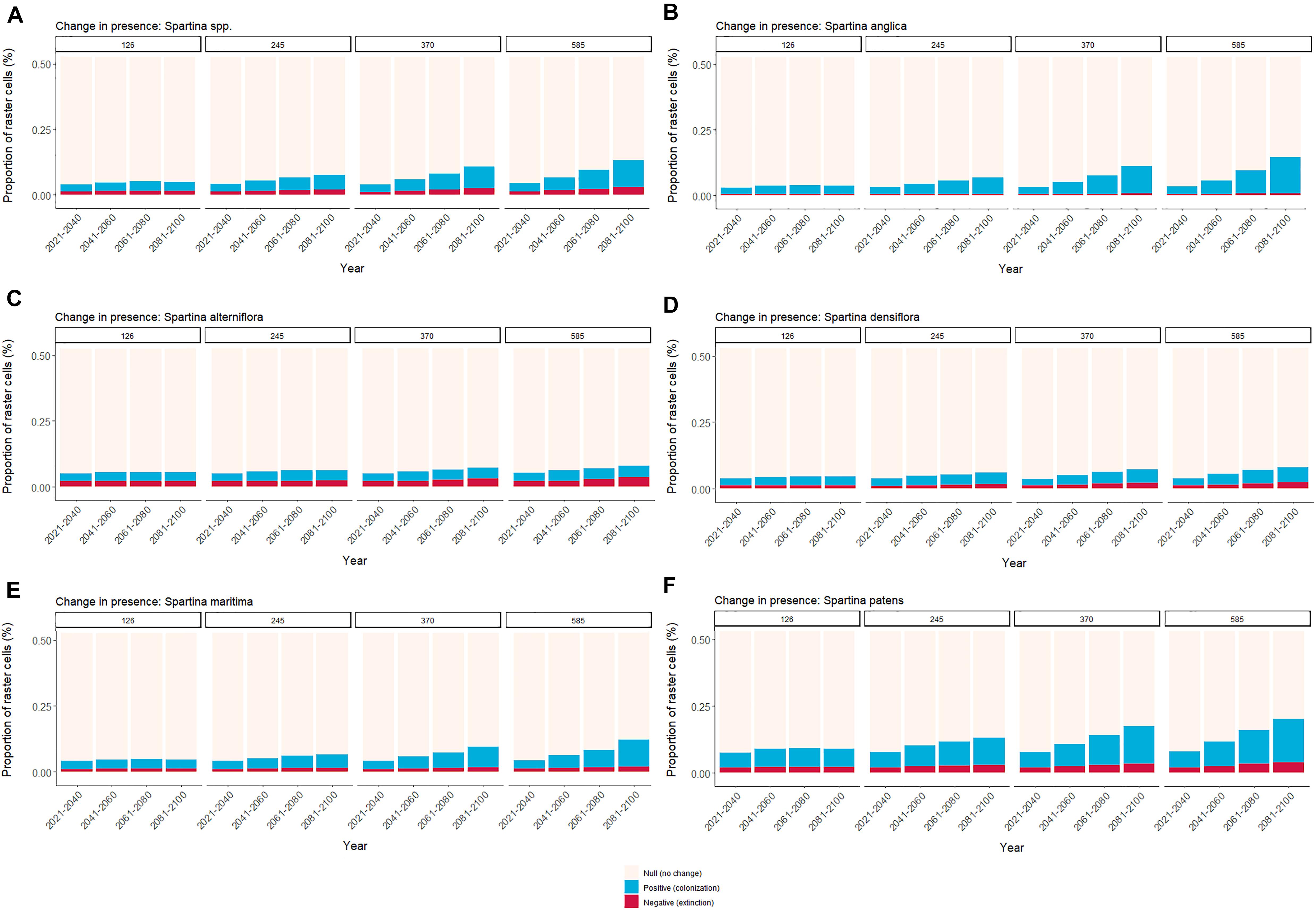
Figure 5. Relative change (colonization or extinction potential) or no change in presence over time (per period, until 2100), given in proportion of raster cells (%) with positive, negative, and null values, across specific CMIP6 Shared Socioeconomic pathways (i.e., SSP1-2.6, 2-4.5, 3-7.0, and 5-8.5), for (A) the whole genus, (B) Spartina anglica (C) Spartina alterniflora (D) Spartina densiflora (E) Spartina maritima, and (F) Spartina patens.
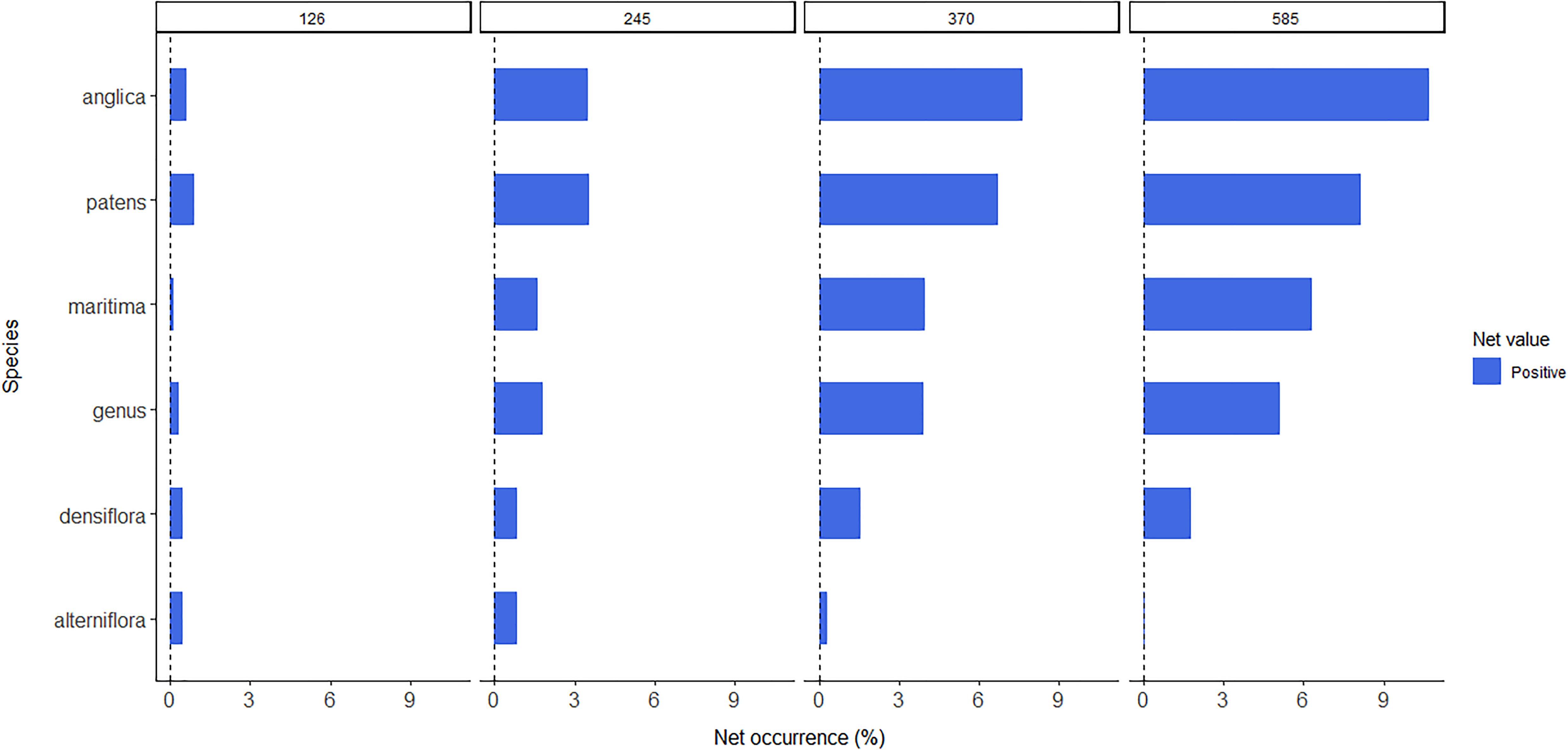
Figure 6. Net change in occurrence increment probability (%) over time (between 2100 time and the historical period) for each Spartina spp. species (i.e., S. alterniflora, S. anglica, S. densiflora, S. maritima, and S. patens) for each CMIP6 Shared Socioeconomic pathway (i.e., SSP1-2.6, 2-4.5, 3-7.0, and 5-8.5). The proportion of colonization and extinction for each species was quantified from the change in proportion of positive (gain), negative (loss), and null (no change) raster map cells between time periods. The percentage of colonization and extinction were then subtracted to obtain the net percentage change in predicted presences.
Future Change to Species Co-occurrence
Finally, the outputs of the species overlap analysis are provided in Figure 7. Overall, the maps show increasing levels of potential species overlap in certain regions, particularly in the higher latitudes of the Northern Hemisphere, which increase with the SSP in question (i.e., from SSP1-2.6 to SSP-5-8.5). Specifically, in SSP1-2.6 there appears to occur medium to long term decrease in overlap (in 2021–2040 and 2041–2060, Figure 7A) in areas such as the Eastern coast of the United States, all the way to the Atlantic coast of South America, together with losses in the Mediterranean Sea and the Iberian Peninsula and South East Asia and Oceania. In this scenario, increased species overlap is observed at a lesser, more local extent, particularly in the northern Canadian shorelines, the Baltic Sea and the North Sea in Scotland’s shores, and finally in the northern Yellow Sea.
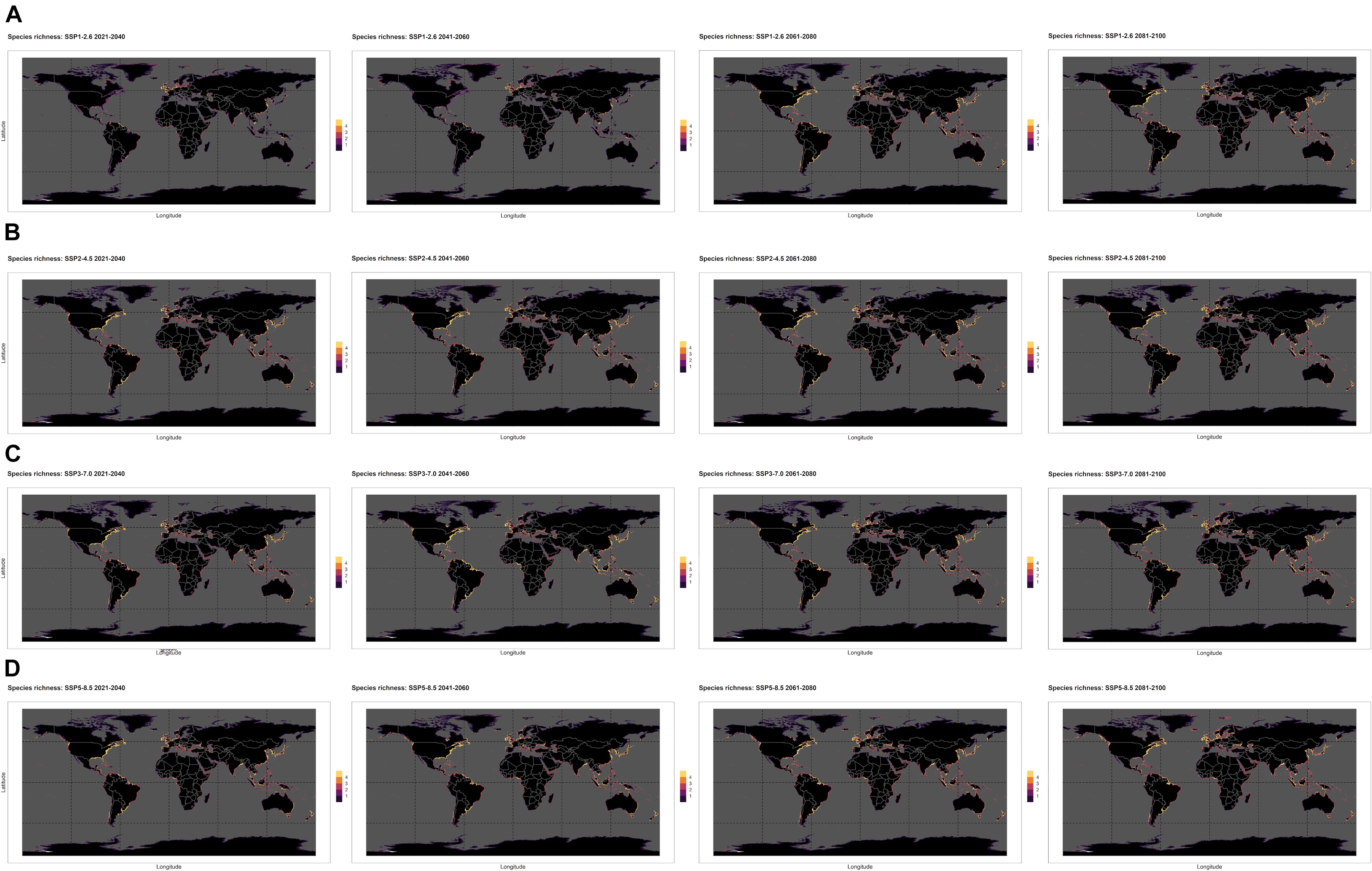
Figure 7. Worldwide distribution of species richness over time (until 2100) of Spartina spp. species (i.e., S. alterniflora, S. anglica, S. densiflora, S. maritima, and S. patens) for each CMIP6 Shared Socioeconomic pathway, SSP1-2.6, (A), 2-4.5 (B), 3-7.0 (C), and 5-8.5 (D).
Concerning the “middle of the road” SSP2-4.5 pathway (Figure 7B), decreases are observed for smaller geographical extents, primarily in the Mediterranean Sea and southern Iberian Peninsula; while overlap increases are observed particularly in the north-eastern region of the Yellow Sea, the Baltic Sea, and the north/north-eastern region of the North American shorelines. The same pattern is present, although with progressively enhanced intensity in SSP3-7.0 and SSP5-8.5 (Figures 7C,D), with enhanced reductions of overlap in the areas of the Mediterranean and southern Iberian Peninsula shorelines and southwestern Australia coastlines, as well as in areas the areas of the eastern North American coastline and north of Rio de la Plata in South America (SSP5-8.5, Figure 7D). In these medium-high and high reference pathway scenarios, the increase in species overlap is also enhanced and more widespread in higher latitude areas particularly in the Northern Hemisphere (e.g., in the areas of Northern Canada and Alaska, the Baltic and North seas, the Russian coastline of the White Sea, the Yellow and Eastern China seas), but also to some extent in the Southern Hemisphere (e.g., southern Australia and New Zealand).
Discussion
The present study presents a global analysis on the potential response of five species of the Spartina Genus in response to climate change until the end of the century. For the majority of the species, differential responses were observed regarding the pathway scenario in question. Despite that, however, there was a clear global trend of increasing species’ occurrence until the year 2100. Also, this pattern increased with increasing severity of future scenarios suggesting general range expansion with climate change. S. anglica showed the most response of all other species, and the predicted increasing habitat suitability for all scenarios explains the increase in distribution for this species worldwide, with a very high net colonization ratio compared to the other species. Habitat suitability appears to increase with latitude over time, potentially leading to the expansion of the species’ known invasive distribution in the Northern Sea and the Baltic, as well as in the Chinese eastern coastline from the Yellow Sea, where it is a known invader (An et al., 2007), potentially to the Gulf of Thailand. The present study suggests that S. anglica can potentially remain as a successful invader indifferent to the future pathway scenario of climate change, although the more severe scenarios will likely favor its expansion.
Despite being one of the more prominent cordgrass invaders (Bortolus et al., 2019), S. alterniflora showed very low levels of expansion, while exhibiting some of the worst net values for habitat suitability of all species. Notwithstanding, it followed a similar pattern in northward distribution shift as other species and remained potentially present in some of its known invasive areas. This suggests that this particular species’ invasive pressure could remain, although these results seem to indicate potential vulnerability to future conditions. S. densiflora, already invasive in the Iberian Peninsula (Bortolus, 2006), exhibits the same poleward movement worldwide, particularly in Europe, suggesting that the areas of the Baltic and North seas might witness future invasion pressure from this species. However, the relatively small sample size of datapoints available for this species in areas such as the Chinese coastline could induce subsampling bias to this specific area, potentially masking effects. Finally, S. patens exhibits the second highest net gain in occurrence distribution, with increasing pathway severity, despite showing large relative losses of habitat suitability. Already an invader in Europe (Baumel et al., 2016; Sánchez et al., 2019), known also by the name of Spartina versicolor Fabre, the present study suggests that this species could not only spread its invasive distribution in the European continent (northward as the other species), it also has the potential to expand from its native areas into South America, and eventually to Asia if taken there by human action.
From an environmental management perspective, the present study identifies the SSP1-2.6 as the most beneficial. Despite changes in habitat suitability being different for all species, the net results from colonization and extinction potential worldwide present a scenario where all species maintain their current status, with relatively small increments. Also, regarding species overlap change until the century’s end, despite localized decrease and gain in certain regions, this is the scenario with the lowest change, which indirectly suggests less potential for change in the current co-occurrence situations hence, less chance for newer regions of invasion-related conflicts. Exceptions are, however, the areas of the Baltic Sea and the Yellow Sea, which are presently invaded (by S. anglica and S. alterniflora, respectively), suggesting that these areas could become suitable for more species. On the other end of the spectrum, the scenario representing high fossil fuel-dependent development (SSP5-8.5), the potential for invasion by Spartina species increases significantly worldwide. Except for S. densiflora and S. alterniflora, this scenario leads to greater net increases in all species’ potential occurrence, despite net decrease in habitat suitability in some cases (e.g., S. patens). Also, most species’ distribution shows the potential to increase, particularly toward higher latitudes in the Northern Hemisphere. Accordingly, this scenario indicates greater increases in species richness northward (i.e., above 50°N) in all northern hemisphere coastlines, while the areas of the Mediterranean and Southern Europe experience a sharp drop in richness. The Iberian Peninsula is known to harbor native S. maritima and invasive S. alterniflora, S. patens, and S. densiflora (SanLeón et al., 1999; Castillo et al., 2017; Gallego-Tévar et al., 2019), with known occurrences of S. anglica as well. In this particular scenario, S. maritima exhibits a poleward migration of its distribution, while S. alterniflora, S. densiflora, and S. patens see their potential distribution increase in this area, although with minimum overlay. These results highlight this region as highly susceptible to substituting its native cordgrass by those species that are already invading, in those scenarios where climate change mitigation measures are less efficient. Not surprisingly, this is already occurring in other areas, such as the Adriatic Sea (Wong et al., 2018). Also, in this scenario of high fossil fuel development, the region of the Yellow sea, already home to significant invasion by the smooth cordgrass (Zheng et al., 2018; Mao et al., 2019), appears particularly susceptible to invasion, since this habitat seems suitable for all the evaluated species. A peculiar result of the present study is the predicted differential range expansion/loss between colder- and warmer adapted species. Specifically, the cold-adapted species, S. anglica, is predicted to undergo an overall range expansion when compared to the rest of the species, although all species appear subjected to the same poleward pressure. This could be due to the difference in availability of longer coastlines with novel, more suitable habitats, Northward from this species’ range, when compared to the rest of the analyzed species. An interesting contrast arises between the decrease in habitat suitability in species like S. patens, S. alterniflora, and S. densiflora; and the increase in probability of occurrence for the same species, although in relatively small percentages, which signify an increase in range expansion. This could be due to the fact that despite the overall suitability of habitats decreases over time, there is an increase in the latitudinal distribution of suitable shores (which is visible throrgh the expansion of the species northward), so species continue to exhibit increases in predicted occurrence (although quite limited), despite a net loss in habitat suitability.
However, some caution is advised in the interpretation of these SDMs, and these results must not be taken as absolute. First, the species presence records used were mostly obtained through GBIF, which being an open-source database is liable to sampling bias which can induce autocorrelation of the model data. Indeed, the GBIF database did not feature any points for S. alterniflora in the coastline of China, and despite the inclusion of data from articles retrieved from the available literature, nevertheless the chance for subsampling of important habitats is a potential risk. Second, since SDMs assume total occupation of climatically suitable areas (Araújo and Pearson, 2005), it is likely that there is some level of over-prediction affecting all species, culminating in predictions for larger areas. Also, the sample size of specie’s occurrence points used can affect the accuracy of the predictive models (Stockwell and Peterson, 2002). In this sense, although all models included at least 300 species occurrence points – which for example is greater than the minimum number required to maximize accuracy in GLM approaches (Pearce and Ferrier, 2000) – there was a significant difference in the data size for different species (e.g., over 3000 for S. anglica while other species had less than 1000), which could lead to slightly different prediction accuracies. Another issue refers to the random assignment of pseudo-absence data across geographical space, which together with potential sampling bias (i.e., the absence of occurrence points where a species exists in reality, but has not been sampled or included in the data sources) could potentially lead to misrepresentations in the projection of geographical occupancy. Lastly, other non-climatic variables would likely benefit the model predictions since they can help increase ecological fidelity (Austin and Van Niel, 2011). In the specific case of the Spartina species, the present study does not include potential species interaction through hybridization, although species were modeled separately to obtain a clear response to the atmospheric variables and their variation over time. Other variables salinity, immersion time, and soil composition could also be useful in this regard. Indeed, the heterogeneity of the world’s coasts and the differential suitability of different types of coastline for Spartina settlement (e.g., sandy beaches, rocky shorelines, and tropical mangroves) should also be addressed, since the fraction of suitable coast significantly changes with latitude (Luijendijk et al., 2018). Indeed, the models in this study predicted and projected the presence of species of the genus Spartina in areas well known to harbor mangrove habitats, as well as some areas with relatively low saltmarsh presence. The intrusion of cordgrasses into mangrove areas is not unheard of Gao et al. (2019), and if conditions are suitable, cordgrasses have the potential to colonize mudflat areas and establish the basis for new saltmarsh habitats. The present study also highlights the potential for intrusion or colonization in these new areas, potentially establishing new competitive pressures with mangrove habitats. As such, future studies should build on the present work by testing the inclusion of these and other predictors, with the potential consequence of increasing computing requirements. Despite these limitations, however, these models provide a general indication of future trends and relative habitat suitability shifts which can be useful for environmental managers (Townhill et al., 2021).
Native saltmarsh vegetation is highly valued due to the role of its constituting species as foundation species, with substantial influence on the productivity and health of biological communities and food webs. The action of aggressive invaders when deliberately or accidentally introduced to areas outside their original range of distributions has raised significant concern from ecologists and environmental managers worldwide. The present study suggests that Spartina species can potentially benefit from climate change severity, with poleward expansions in the Northern Hemisphere being predicted for most species until 2100. Increased conflict and invasion potential could occur in Northern Europe and East Asian shorelines, in areas that already suffer from increased invasive pressure. Overall, and despite their limitations, SDMs can help establish general trends in climate change ecology and inform policymakers and environmental agents to ensure the correct management of these habitats and, ultimately, ecosystems.
Data Availability Statement
The original contributions presented in the study are included in the article/Supplementary Material, further inquiries can be directed to the corresponding author.
Author Contributions
FOB, CPS, JRP, and RR performed the statistical analysis. BD, IC, and EM-N compiled and provided the occurrence data. All authors participated in the writing and revision process of the manuscript.
Funding
The present study was funded by Fundação para a Ciência e a Tecnologia (FCT) for funding the research via the project grant UIDB/04292/2020. This work was also funded by MAR 2020 program via the Project RESTAURA2020 (16-01-04-FMP-0014). BD and VFF were supported by investigation contracts (CEECIND/00511/2017 and DL57/2016/CP1479/CT0024). PR-S was supported by FCT through a postdoctoral grant (SFRH/BPD/95784/2013). FOB (SFRH/BD/147294/2019) and CPS (SFRH/BD/117890/2016) are currently supported by Ph.D. grants funded by community funds from FCT and the European Social Fund (ESF), through the Human Capital Operating Programme and Regional Operation Programme (Lisboa 2020). JRP is currently supported byproject ASCEND — PTDC/BIA-BMA/28609/2017 co-funded by FCT – Fundação para a Ciência e Tecnologia, I.P, Programa Operacional Regional de Lisboa, Portugal 2020 and the European Union within the project LISBOA-01-0145-FEDER-028609. The National Research Foundation of South Africa through the support of the DSI/NRF Research Chair in Shallow Water Ecosystems is thanked for funding (UID 84375) JBA.
Conflict of Interest
The authors declare that the research was conducted in the absence of any commercial or financial relationships that could be construed as a potential conflict of interest.
Publisher’s Note
All claims expressed in this article are solely those of the authors and do not necessarily represent those of their affiliated organizations, or those of the publisher, the editors and the reviewers. Any product that may be evaluated in this article, or claim that may be made by its manufacturer, is not guaranteed or endorsed by the publisher.
Acknowledgments
The authors would like to express their gratitude to all the funding organizations which supported this study.
Supplementary Material
The Supplementary Material for this article can be found online at: https://www.frontiersin.org/articles/10.3389/fmars.2021.696333/full#supplementary-material
Footnotes
- ^ https://doi.org/10.15468/dl.xwf3tv
- ^ https://doi.org/10.15468/dl.kkg2y5
- ^ https://doi.org/10.15468/dl.9vxpqb
- ^ https://doi.org/10.15468/dl.m2tkje
- ^ https://doi.org/10.15468/dl.7y58u4
- ^ https://data.unep-wcmc.org
- ^ https://www.worldclim.org/data/worldclim21.html
- ^ https://www.naturalearthdata.com/downloads/10m-physical-vectors/10m-coastline/
References
Ainouche, M. L., Baumel, A., Salmon, A., and Yannic, G. (2004). Hybridization, polyploidy and speciation in Spartina (Poaceae). New Phytol. 161, 165–172. doi: 10.1046/j.1469-8137.2003.00926.x
An, S. Q., Gu, B. H., Zhou, C. F., Wang, Z. S., Deng, Z. F., Zhi, Y. B., et al. (2007). Spartina invasion in China: implications for invasive species management and future research. Weed Res. 47, 183–191. doi: 10.1111/j.1365-3180.2007.00559.x
Araújo, M. B., and Guisan, A. (2006). Five (or so) challenges for species distribution modelling. J. Biogeogr. 33, 1677–1688. doi: 10.1111/j.1365-2699.2006.01584.x
Araújo, M. B., and Pearson, R. G. (2005). Equilibrium of species’ distributions with climate. Ecography 28, 693–695. doi: 10.1111/j.2005.0906-7590.04253.x
Araújo, M. B., and Rahbek, C. (2006). How does climate change affect biodiversity? Science 313, 1396–1397. doi: 10.1126/science.1131758
Austin, M. P., and Van Niel, K. P. (2011). Improving species distribution models for climate change studies: variable selection and scale. J. Biogeogr. 38, 1–8. doi: 10.1111/j.1365-2699.2010.02416.x
Bagheri, H., Ghorbani, A., Zare Chahouki, M. A., Jafari, A. A., and Sefidi, K. (2017). Halophyte species distribution modeling with MaxEnt model in the surrounding rangelands of Meighan playa. Iran. Appl. Ecol. Environ. Res. 15, 1472–1484.
Barbet-Massin, M., Jiguet, F., Albert, C. H., and Thuiller, W. (2012). Selecting pseudo-absences for species distribution models: How, Where and how many? Methods Ecol. Evol. 3, 327–338. doi: 10.1111/j.2041-210x.2011.00172.x
Barbier, E. B. (2013). Valuing ecosystem services for coastal wetland protection and restoration: progress and challenges. Resources 2, 213–230. doi: 10.3390/resources2030213
Baumel, A., Ainouche, M. L., Misset, M. T., Gourret, J.-P., and Bayer, R. J. (2003). Genetic evidence for hybridization between the native Spartina maritima and the introduced Spartina alterniflora (Poaceae) in South-West France: Spartina × neyrautii re-examined. Plant Syst. Evol. 237, 87–97. doi: 10.1007/s00606-002-0251-8
Baumel, A., Rousseau-Gueutin, M., Sapienza-Bianchi, C., Gareil, A., Duong, N., Rousseau, H., et al. (2016). Spartina versicolor Fabre: Another case of Spartina trans-Atlantic introduction? Biol. Invasions 18, 2123–2135. doi: 10.1007/s10530-016-1128-z
Bertness, M. D. (1991). Zonation of Spartina patens and Spartina alterniflora in New England Salt Marsh. Ecology 72, 138–148. doi: 10.2307/1938909
Bortolus, A. (2006). The austral cordgrass Spartina densiflora Brong.: its taxonomy, biogeography and natural history. J. Biogeogr. 33, 158–168. doi: 10.1111/j.1365-2699.2005.01380.x
Bortolus, A., Adam, P., Adams, J. B., Ainouche, M. L., Ayres, D., Bertness, M. D., et al. (2019). Supporting Spartina: interdisciplinary perspective shows Spartina as a distinct solid genus. Ecology 100:e02863.
Caçador, I., Neto, J. M., Duarte, B., Barroso, D. V., Pinto, M., and Marques, J. C. (2013). Development of an angiosperm quality assessment index (AQuA-Index) for ecological quality evaluation of Portuguese water bodies—A multi-metric approach. Ecol. Indic. 25, 141–148. doi: 10.1016/j.ecolind.2012.09.021
Castillo, J. M., Gallego-Tévar, B., Figueroa, E., Grewell, B. J., Vallet, D., and Rousseau, H. (2018). Low genetic diversity contrasts with high phenotypic variability in heptaploid Spartina densiflora populations invading the Pacific coast of North America. Ecol. Evol. 8, 4992–5007. doi: 10.1002/ece3.4063
Castillo, J. M., Grewell, B. J., Pickart, A. J., Figueroa, E., and Sytsma, M. (2016). Variation in tussock architecture of the invasive cordgrass Spartina densiflora along the Pacific Coast of North America. Biol. Invasions 18, 2159–2174. doi: 10.1007/s10530-015-0991-3
Castillo, J. M., Leira-Doce, P., and Figueroa, E. (2017). Biomass and clonal architecture of the cordgrass Spartina patens (Poaceae) as an invasive species in two contrasted coastal habitats on the Atlantic coast of the Iberian Peninsula. Plant Ecol. Evol. 150, 129–138. doi: 10.5091/plecevo.2017.1290
Cheng, X., Luo, Y., Chen, J., Lin, G., Chen, J., and Li, B. (2006). Short-term C4 plant Spartina alterniflora invasions change the soil carbon in C3 plant-dominated tidal wetlands on a growing estuarine Island. Soil Biol. Biochem. 38, 3380–3386. doi: 10.1016/j.soilbio.2006.05.016
Crosby, S. C., Angermeyer, A., Adler, J. M., Bertness, M. D., Deegan, L. A., Sibinga, N., et al. (2017). Spartina alterniflora biomass allocation and temperature: implications for salt marsh persistence with sea-level rise. Estuaries Coast. 40, 213–223. doi: 10.1007/s12237-016-0142-9
Curado, G., Gallego-Tévar, B., Figueroa, E., and Castillo, J. M. (2020). Effects of removal of alien Spartina densiflora and restoration of native S. maritima on succession and zonation in European salt marshes. Estuar. Coast. Shelf Sci. 244:105815. doi: 10.1016/j.ecss.2018.04.011
De’ath, G. (2007). Boosted trees for ecological modelling and prediction. Ecology 88, 243–251. doi: 10.1890/0012-9658(2007)88[243:btfema]2.0.co;2
Donatelli, C., Ganju, N. K., Zhang, X., Fagherazzi, S., and Leonardi, N. (2018). Salt marsh loss affects tides and the sediment budget in shallow bays. J. Geophys. Res. Earth Surf. 123, 2647–2662. doi: 10.1029/2018jf004617
Duarte, B., Baeta, A., Rousseau-Gueutin, M., Ainouche, M., Marques, J. C., and Caçador, I. (2015). A tale of two Spartinas: climatic, photobiological and isotopic insights on the fitness of non-indigenous versus native species. Estuar. Coast. Shelf Sci. 167, 178–190. doi: 10.1016/j.ecss.2015.06.015
Duarte, B., Caçador, I., Marques, J. C., and Croudace, I. (2013). Tagus Estuary salt marshes feedback to sea level rise over a 40-year period: insights from the application of geochemical indices. Ecol. Indic. 34, 268–276. doi: 10.1016/j.ecolind.2013.05.015
Duarte, B., Caetano, M., Almeida, P., Vale, C., and Caçador, I. (2010). Accumulation and biological cycling of heavy metal in the root-sediment system of four salt marsh species, from Tagus estuary (Portugal). Environ. Pollut. 158, 1661–1668. doi: 10.1016/j.envpol.2009.12.004
Duarte, B., Carreiras, J., and Caçador, I. (2021). Climate change impacts on salt marsh blue carbon, nitrogen and phosphorous stocks and ecosystem services. Appl. Sci. 11:1969. doi: 10.3390/app11041969
Duarte, B., Marques, J. C., and Caçador, I. (2016). Ecophysiological responses of native and invasive Spartina species to extreme temperature events in Mediterranean marshes. Biol. Invasions 18, 2189–2205. doi: 10.1007/s10530-015-0958-4
Duarte, B., Mateos-Naranjo, E., Redondo Goméz, S., Marques, J. C., and Caçador, I. (2018). “Cordgrass invasions in Mediterranean marshes: past, present and future,” in Histories of Bioinvasions in the Mediterranean, eds A. I. Queiroz and S. Pooley (Berlin: Springer).
Duarte, B., Valentim, J. M., Dias, J. M., Silva, H., Marques, J. C., and Caçador, I. (2014). Modelling Sea Level Rise (SLR) impacts on salt marsh detrital outwelling C and N exports from an estuarine coastal lagoon to the ocean (Ria de Aveiro, Portugal). Ecol. Modell. 289, 36–44. doi: 10.1016/j.ecolmodel.2014.06.020
Duarte, C. M., Middelburg, J. J., and Caraco, N. (2005). Major role of marine vegetation on the oceanic carbon cycle. Biogeosciences 2, 1–8. doi: 10.5194/bg-2-1-2005
Elith, J. H., Graham, C. P., Anderson, R., Dudík, M., Ferrier, S., Guisan, A. J., et al. (2006). Novel methods improve prediction of species’ distributions from occurrence data. Ecography 29, 129–151.
Feng, J., Zhou, J., Wang, L., Cui, X., Ning, C., Wu, H., et al. (2017). Effects of short-term invasion of Spartina alterniflora and the subsequent restoration of native mangroves on the soil organic carbon, nitrogen and phosphorus stock. Chemosphere 184, 774–783. doi: 10.1016/j.chemosphere.2017.06.060
Fick, S. E., and Hijmans, R. J. (2017). WorldClim 2: new 1-km spatial resolution climate surfaces for global land areas. Int. J. Climatol. 37, 4302–4315. doi: 10.1002/joc.5086
Fitzpatrick, M. C., and Hargrove, W. W. (2009). The projection of species distribution models and the problem of non-analog climate. Biodivers. Conserv. 18, 2255–2261. doi: 10.1007/s10531-009-9584-8
Gallego-Tévar, B., Infante-Izquierdo, M. D., Figueroa, E., Nieva, F. J. J., Muñoz-Rodríguez, A. F., Grewell, B. J., et al. (2019). Some Like It Hot: maternal-switching with climate change modifies formation of invasive Spartina Hybrids. Front. Plant Sci. 10:484. doi: 10.3389/fpls.2019.00484
Gao, G. F., Li, P. F., Zhong, J. X., Shen, Z. J., Chen, J., Li, Y. T., et al. (2019). Spartina alterniflora invasion alters soil bacterial communities and enhances soil N2O emissions by stimulating soil denitrification in mangrove wetland. Sci. Total Environ. 653, 231–240. doi: 10.1016/j.scitotenv.2018.10.277
Gao, J., Yang, G., and Ou, W. (2007). The influence after introduction of Spartina alterniflora on the distribution of TOC, TN and TP in the national Yancheng rare birds nature reserve, Jiangsu Province, China. Geogr. Res. 26, 799–808.
García-Roselló, E., Guisande, C., Heine, J., Pelayo-Villamil, P., Manjarrés-Hernández, A., González Vilas, L., et al. (2014). Using modestr to download, import and clean species distribution records. Methods Ecol. Evol. 5, 708–713. doi: 10.1111/2041-210x.12209
Gedan, K. B., and Bertness, M. D. (2010). How will warming affect the salt marsh foundation species Spartina patens and its ecological role? Oecologia 164, 479–487. doi: 10.1007/s00442-010-1661-x
Gedan, K. B., Silliman, B. R., and Bertness, M. D. (2009). Centuries of human-driven change in salt marsh ecosystems. Annu. Rev. Mar. Sci. 1, 117–141. doi: 10.1146/annurev.marine.010908.163930
Greenberg, R., Cardoni, A., Ens, B. J., Gan, X., Isacch, J. P., Koffijberg, K., et al. (2014). “The distribution and conservation of birds of coastal salt marshes,” in Coastal Conservation. Conservation Biology, eds B. Maslo and J. L. Lockwood (Cambridge: Cambridge University Press), 180–242. doi: 10.1017/cbo9781139137089.008
Guenegou, M. C., Citharel, J., and Levasseur, J. E. (1988). The hybrid status of Spartina anglica (Poaceae). Enzymatic analysis of the species and of the presumed parents. Can. J. Bot. 66, 1830–1833. doi: 10.1139/b88-249
Heikkinen, R. K., Luoto, M., Araújo, M. B., Virkkala, R., Thuiller, W., and Sykes, M. T. (2006). Methods and uncertainties in bioclimatic envelope modelling under climate change. Prog. Phys. Geogr. Earth Environ. 30, 751–777. doi: 10.1177/0309133306071957
Hessini, K., Martínez, J. P., Gandour, M., Albouchi, A., Soltani, A., and Abdelly, C. (2009). Effect of water stress on growth, osmotic adjustment, cell wall elasticity and water-use efficiency in Spartina alterniflora. Environ. Exp. Bot. 67, 312–319. doi: 10.1016/j.envexpbot.2009.06.010
Himes-Cornell, A., Pendleton, L., and Atiyah, P. (2018). Valuing ecosystem services from blue forests: a systematic review of the valuation of salt marshes, sea grass beds and mangrove forests. Ecosyst. Serv. 30, 36–48. doi: 10.1016/j.ecoser.2018.01.006
Human, L., Feijão, E., Carvalho, R. C., Caçador, I., Reis-Santos, P., Fonseca, V., et al. (2020). Mediterranean salt marsh sediment metal speciation and bioavailability changes induced by the spreading of non-indigenous Spartina patens. Estuar. Coast. Shelf Sci. 243:106921. doi: 10.1016/j.ecss.2020.106921
Jiménez-Valverde, A., Peterson, A. T., Soberón, J., Overton, J. M., Aragón, P., and Lobo, J. M. (2011). Use of niche models in invasive species risk assessments. Biol. Invasions 13, 2785–2797. doi: 10.1007/s10530-011-9963-4
Jin, B., Gao, D., Yang, P., Wang, W., and Zeng, C. (2016). Change of soil organic carbon with different years of Spartina alterniflora invasion in wetlands of Minjiang River estuary. J. Nat. Res. 31, 608–619.
Kirwan, M. L., Guntenspergen, G. R., and Morris, J. T. (2009). Latitudinal trends in Spartina alterniflora productivity and the response of coastal marshes to global change. Glob. Chang. Biol. 15, 1982–1989. doi: 10.1111/j.1365-2486.2008.01834.x
Leonardi, N., Carnacina, I., Donatelli, C., Ganju, N. K., Plater, A. J., Schuerch, M., et al. (2018). Dynamic interactions between coastal storms and salt marshes: a review. Geomorphology 301, 92–107. doi: 10.1016/j.geomorph.2017.11.001
Liu, J., Zhou, H., Qin, P., and Zhou, J. (2007). Effects of Spartina alterniflora salt marshes on organic carbon acquisition in intertidal zones of Jiangsu Province, China. Ecol. Eng. 30, 240–249. doi: 10.1016/j.ecoleng.2007.01.010
Luijendijk, A., Hagenaars, G., Ranasinghe, R., Baart, F., Donchyts, G., and Aarninkhof, S. (2018). The state of the world’s beaches. Sci. Rep. 8:6641.
Maebara, Y., Tamaoki, M., Iguchi, Y., Nakahama, N., Hanai, T., Nishino, A., et al. (2020). Genetic diversity of invasive Spartina alterniflora Loisel. (Poaceae) introduced unintentionally into Japan and its invasion pathway. Front. Plant Sci. 11:556039. doi: 10.3389/fpls.2020.556039
Mao, D., Liu, M., Wang, Z., Li, L., Man, W., Jia, M., et al. (2019). Rapid Invasion of Spartina alterniflora in the Coastal Zone of Mainland China: spatiotemporal Patterns and Human Prevention. Sensors 19:2308. doi: 10.3390/s19102308
McCullagh, P., and Nelder, J. A. (1989). Generalized Linear Models, ed. C. Hall (London: Routledge).
Mcowen, C. J., Weatherdon, L. V., Van Bochove, J.-W., Sullivan, E., Blyth, S., Zockler, C., et al. (2017). A global map of saltmarshes. Biodivers. Data J. 5:e11764. doi: 10.3897/bdj.5.e11764
Meinshausen, M., Nicholls, Z. R. J., Lewis, J., Gidden, M. J., Vogel, E., Freund, M., et al. (2020). The shared socio-economic pathway (SSP) greenhouse gas concentrations and their extensions to 2500. Geosci. Model Dev. 13, 3571–3605. doi: 10.5194/gmd-13-3571-2020
Mueller, P., Ladiges, N., Jack, A., Schmiedl, G., Kutzbach, L., Jensen, K., et al. (2019). Assessing the long-term carbon-sequestration potential of the semi-natural salt marshes in the European Wadden Sea. Ecosphere 10:e02556.
Naimi, B., and Araújo, M. B. (2016). Sdm: a reproducible and extensible R platform for species distribution modelling. Ecography 39, 368–375. doi: 10.1111/ecog.01881
Nieva, F. J. J., Díaz-Espejo, A., Castellanos, E. M., and Figueroa, M. E. (2001). Field Variability of Invading Populations of Spartina densiflora Brong. in Different Habitats of the Odiel Marshes (SW Spain). Estuar. Coast. Shelf Sci. 52, 515–527. doi: 10.1006/ecss.2000.0750
Pang, H. J., Lyu, S. S., Chen, X. G., Jin, A. M., Loh, P. S., Li, F., et al. (2017). Heavy metal distribution and accumulation in the Spartina alterniflora from the Andong tidal flat, Hangzhou Bay, China. Environ. Earth Sci. 76, 1–14.
Pearce, J., and Ferrier, S. (2000). Evaluating the predictive performance of habitat models developed using logistic regression. Ecol. Modell. 133, 225–245. doi: 10.1016/s0304-3800(00)00322-7
Pennings, S. C., Grant, M.-B., and Bertness, M. D. (2005). Plant zonation in low-latitude salt marshes: disentangling the roles of flooding, salinity and competition. J. Ecol. 93, 159–167. doi: 10.1111/j.1365-2745.2004.00959.x
Peterson, A. T., and Soberón, J. (2012). Species distribution modeling and ecological niche modeling: getting the concepts right. Nat. Conserv. 10, 102–107. doi: 10.4322/natcon.2012.019
Peterson, A. T., and Vieglais, D. A. (2001). Predicting species invasions using ecological niche modeling: new approaches from bioinformatics attack a pressing problem: a new approach to ecological niche modeling, based on new tools drawn from biodiversity informatics, is applied to the challenge of. Bioscience 51, 363–371. doi: 10.1641/0006-3568(2001)051[0363:psiuen]2.0.co;2
Phillips, S. J., and Dudík, M. (2008). Modeling of species distributions with Maxent: new extensions and a comprehensive evaluation. Ecography 31, 161–175. doi: 10.1111/j.0906-7590.2008.5203.x
R Core Team (2020). R: A Language and Environment for Statistical Computing. Vienna: R Foundation for Statistical Computing. Available online at: https://www.R-project.org/
Reid, W. V. (2005). Millennium Ecosystem Assessment. Available online at https://www.millenniumassessment.org/en/index.html (accessed June 20, 2020).
Sánchez, J. M., Sánchez, C., and Navarro, L. (2019). Can asexual reproduction by plant fragments help to understand the invasion of the NW Iberian coast by Spartina patens? Flora 257:151410. doi: 10.1016/j.flora.2019.05.009
SanLeón, D. G., Izco, J., and Sánchez, J. M. (1999). Spartina patens as a weed in Galician saltmarshes (NW Iberian Peninsula). Hydrobiologia 415, 213–222. doi: 10.1007/978-94-017-0922-4_30
Sinclair, S. J., White, M. D., and Newell, G. R. (2010). How useful are species distribution models for managing biodiversity under future climates? Ecol. Soc. 15:8.
Spalding, M., Kainuma, M., and Collins, L. (2010). World Atlas of Mangroves (version 3.1). A Collaborative Project of ITTO, ISME, FAO, UNEP-WCMC, UNESCO-MAB, UNU-INWEH and TNC. (London: Earthscan), 319. doi: 10.34892/w2ew-m835
Stockwell, D. R. B., and Peterson, A. T. (2002). Effects of sample size on accuracy of species distribution models. Ecol. Modell. 148, 1–13. doi: 10.1016/s0304-3800(01)00388-x
Strong, D. R., and Ayres, D. R. (2013). Ecological and evolutionary misadventures of Spartina. Annu. Rev. Ecol. Evol. Syst. 44, 389–410. doi: 10.1146/annurev-ecolsys-110512-135803
Tebaldi, C., Debeire, K., Eyring, V., Fischer, E., Fyfe, J., Friedlingstein, P., et al. (2021). Climate model projections from the Scenario Model Intercomparison Project (ScenarioMIP) of CMIP6. Earth Syst. Dyn. 12, 253–293.
Tonti, N. E., Gassmann, M. I., and Pérez, C. F. (2018). First results of energy and mass exchange in a salt marsh on southeastern South America. Agric. For. Meteorol. 263, 59–68. doi: 10.1016/j.agrformet.2018.08.001
Townhill, B. L., Couce, E., Bell, J., Reeves, S., and Yates, O. (2021). Climate change impacts on atlantic oceanic island tuna fisheries. Front. Mar. Sci. 8:634280. doi: 10.3389/fmars.2021.634280
van Hulzen, J. B., van Soelen, J., and Bouma, T. J. (2007). Morphological variation and habitat modification are strongly correlated for the autogenic ecosystem engineer Spartina anglica (Common Cordgrass). Estuaries Coast. 30, 3–11. doi: 10.1007/bf02782962
Vilà, M., Espinar, J. L., Hejda, M., Hulme, P. E., Jarošík, V., Maron, J. L., et al. (2011). Ecological impacts of invasive alien plants: a meta-analysis of their effects on species, communities and ecosystems. Ecol. Lett. 14, 702–708. doi: 10.1111/j.1461-0248.2011.01628.x
Vu, H. D., Wie‘ ski, K., and Pennings, S. C. (2017). Ecosystem engineers drive creek formation in salt marshes. Ecology 98, 162–174. doi: 10.1002/ecy.1628
Wang, D., Zhang, R., Xiong, J., Guo, H., and Zhao, B. (2015). Contribution of invasive species Spartina alterniflora to soil organic carbon pool in coastal wetland: Stable isotope approach. Chin. J. Plant Ecol. 39, 941–949. doi: 10.17521/cjpe.2015.0091
Wang, G., Yang, W., Wang, G., Liu, J., and Hang, Z. (2013). The effects of Spartina alterniflora seaward invasion on soil organic carbon fractions, sources and distribution. Acta Ecol. Sin. 33, 2474–2483.
Watson, E. B., Szura, K., Wigand, C., Raposa, K. B., Blount, K., and Cencer, M. (2016). Sea level rise, drought and the decline of Spartina patens in New England marshes. Biol. Conserv. 196, 173–181. doi: 10.1016/j.biocon.2016.02.011
Wisz, M. S., Hijmans, R. J., Li, J., Peterson, A. T., Graham, C. H., Guisan, A., et al. (2008). Effects of sample size on the performance of species distribution models. Divers. Distrib. 14, 763–773.
Wong, J. X. W., Costantini, F., Merloni, N., Savelli, L., Geelen, D., and Airoldi, L. (2018). The widespread and overlooked replacement of Spartina maritima by non-indigenous S. anglica and S. townsendii in north-western Adriatic saltmarshes. Biol. Invasions 20, 1687–1702. doi: 10.1007/s10530-017-1654-3
Xia, L., Geng, Q., and An, S. (2020). Rapid genetic divergence of an invasive species, Spartina alterniflora, in China. Front. Genet. 11:284. doi: 10.3389/fgene.2020.00284
Yang, W., Zhao, H., Chen, X., Yin, S., Cheng, X., and An, S. (2013). Consequences of short-term C4 plant Spartina alterniflora invasions for soil organic carbon dynamics in a coastal wetland of Eastern China. Ecol. Eng. 61, 50–57. doi: 10.1016/j.ecoleng.2013.09.056
Yue, S., Zhou, Y., Xu, S., Zhang, X., Liu, M., Qiao, Y., et al. (2021). Can the non-native salt marsh halophyte Spartina alterniflora threaten native seagrass (Zostera japonica) habitats? a case study in the Yellow River Delta, China Front. Plant Sci. 12:643425. doi: 10.3389/fpls.2021.643425
Zedler, J. B., and Kercher, S. (2005). Wetland resources: status, trends, ecosystem services, and restorability. Annu. Rev. Environ. Resour. 30, 39–74. doi: 10.1146/annurev.energy.30.050504.144248
Zhang, D., Hu, Y., Liu, M., Chang, Y., Yan, X., Bu, R., et al. (2017). Introduction and Spread of an Exotic Plant, Spartina alterniflora, Along Coastal Marshes of China. Wetlands 37, 1181–1193. doi: 10.1007/s13157-017-0950-0
Zhang, X., Xiao, X., Wang, X., Xu, X., Chen, B., Wang, J., et al. (2020). Quantifying expansion and removal of Spartina alterniflora on Chongming island, China, using time series Landsat images during 1995–2018. Remote Sens. Environ. 247:111916. doi: 10.1016/j.rse.2020.111916
Zhang, Y., Ding, W., Luo, J., and Donnison, A. (2010). Changes in soil organic carbon dynamics in an Eastern Chinese coastal wetland following invasion by a C4 plant Spartina alterniflora. Soil Biol. Biochem. 42, 1712–1720. doi: 10.1016/j.soilbio.2010.06.006
Zheng, S., Shao, D., and Sun, T. (2018). Productivity of invasive saltmarsh plant Spartina alterniflora along the coast of China: a meta-analysis. Ecol. Eng. 117, 104–110. doi: 10.1016/j.ecoleng.2018.03.015
Zimmermann, N. E., Edwards, T. C. Jr., Graham, C. H., Pearman, P. B., and Svenning, J.-C. (2010). New trends in species distribution modelling. Ecography 33, 985–989. doi: 10.1111/j.1600-0587.2010.06953.x
Keywords: biological invasion, coastal areas, salt marshes, cordgrass, native, species distribution model
Citation: Borges FO, Santos CP, Paula JR, Mateos-Naranjo E, Redondo-Gomez S, Adams JB, Caçador I, Fonseca VF, Reis-Santos P, Duarte B and Rosa R (2021) Invasion and Extirpation Potential of Native and Invasive Spartina Species Under Climate Change. Front. Mar. Sci. 8:696333. doi: 10.3389/fmars.2021.696333
Received: 16 April 2021; Accepted: 27 July 2021;
Published: 17 August 2021.
Edited by:
Paul Snelgrove, Memorial University of Newfoundland, CanadaReviewed by:
Peter M. J. Herman, Delft University of Technology, NetherlandsDeyan Liu, Institute of Soil Science, Chinese Academy of Sciences, China
Copyright © 2021 Borges, Santos, Paula, Mateos-Naranjo, Redondo-Gomez, Adams, Caçador, Fonseca, Reis-Santos, Duarte and Rosa. This is an open-access article distributed under the terms of the Creative Commons Attribution License (CC BY). The use, distribution or reproduction in other forums is permitted, provided the original author(s) and the copyright owner(s) are credited and that the original publication in this journal is cited, in accordance with accepted academic practice. No use, distribution or reproduction is permitted which does not comply with these terms.
*Correspondence: Francisco O. Borges, ZmRib3JnZXNAZmMudWwucHQ=