- 1Museum Zoology Bogoriense (MZB), Zoology Division of Research Center for Biology, Indonesia Institute of Science (LIPI), Cibinong, Indonesia
- 2Interdisciplinary Graduate School of Agriculture and Engineering, University of Miyazaki, Miyazaki, Japan
- 3Faculty of Fisheries and Marine Sciences, Halu Oleo University, Kendari, Indonesia
- 4Division of Parasitology, Faculty of Medicine, University of Miyazaki, Miyazaki, Japan
- 5School of Life Sciences and Technology, Department of Life Science and Technology, Tokyo Institute of Technology, Tokyo, Japan
- 6Field Science Center, Graduate School of Agricultural Science, Tohoku University, Miyagi, Japan
- 7Faculty of Agriculture, Department of Marine Biology and Environmental Science, University of Miyazaki, Miyazaki, Japan
Spatial autocorrelation analysis is a well-established technique for detecting spatial structures and patterns in ecology. However, compared to inter-population genetic structure, much less studies examined spatial genetic structure (SGS) within a population by means of spatial autocorrelation analysis. More SGS analysis that compares the robustness of genome-wide single nucleotide polymorphisms (SNPs) and traditional population genetic markers in detecting SGS, and direct comparison between the estimated dispersal range based on SGS and the larval dispersal range of corals directly surveyed in the field would be important. In this study, we examined the SGS of a reef-building coral species, Heliopora coerulea, in two different reefs (Shiraho and Akaishi) using genome-wide SNPs derived from Multiplexed inter-simple sequence repeat (ISSR) genotyping by sequencing (MIG-seq) analysis and nine microsatellite loci for comparison. Microsatellite data failed to reveal significant spatial patterns when using the same number of samples as MIG-seq, whereas MIG-seq analysis revealed significant spatial autocorrelation patterns up to 750 m in both Shiraho and Akaishi reefs based on the maximum significant distance method. However, detailed spatial genetic analysis using fine-scale distance classes (25–200 m) found an x-intercept of 255–392 m in Shiraho and that of 258–330 m in Akaishi reef. The latter results agreed well with a previously reported direct field observation of larval dispersal, indicating that the larvae of H. coerulea settled within a 350 m range in Shiraho reef within one generation. Overall, our results empirically demonstrate that the x-intercept of the spatial correlogram agrees well with the larval dispersal distance that is most frequently found in field observations, and they would be useful for deciding effective conservation management units for maintenance and/or recovery within an ecological time scale.
Introduction
Coral reefs are highly diverse marine ecosystems, although they are now facing threats such as degradation due to climate change, ocean acidification, frequent outbreaks of corallivore, and other anthropogenic stressors (Wilkinson, 2006). Reef-building corals are the foundation species of coral reef ecosystems that require effective conservation efforts (Carpenter et al., 2008). Although the adults are sessile, reef-building corals release gametes and/or larvae that disperse from their native habitats (Fisk and Harriott, 1990). Because such gamete and larval dispersal results in genetic connectivity among population forming metapopulation structure, estimating the range of gamete and larval dispersal is essential for conservation management (Connolly and Baird, 2010).
Spatial autocorrelation analysis is a well-established technique for assessing spatial dispersal patterns (Epperson, 2005; Schwartz and McKelvey, 2009), but has been overlooked in the field of molecular ecology, especially for benthic marine invertebrates (Billingham and Ayre, 1996; McFadden and Aydin, 1996; Adjeroud and Tsuchiya, 1999; Yund and O’Neil, 2000; Calderón et al., 2007). Compared to intensively studied inter-population genetic structure, much less studies have examined the spatial genetic structure (SGS) of reef-building coral species within a population. Such individual-based SGS within a population have provided important insights into the micro-evolutionary processes and sampling strategies of coral populations (Underwood et al., 2007, 2020; Gorospe and Karl, 2013; Chan et al., 2019; Dubé et al., 2020). However, most of the studies were based on traditional microsatellite loci and to our knowledge no study has used genome-wide single nucleotide polymorphisms (SNPs) and compared the results with the dispersal range directly measured in the field. Theoretically, the detailed genotype information for each sample using genome-wide SNPs would improve the efficiency of detecting significant SGSs, as stochastic error in genotype is one of the challenges in spatial autocorrelation analysis (Slatkin and Arter, 1991).
Owing to the advent of next-generation sequencing (NGS) technology, genome-wide analysis has also become available for non-model organisms. Multiplexed inter-simple sequence repeat (ISSR) genotyping by sequencing (MIG-seq) (Suyama and Matsuki, 2015) is an easy and cost-effective method to obtain a moderate amount of genome-wide SNP data. MIG-seq amplifies putatively neutral ISSR regions, and has proven useful for delimitating species boundaries (Richards et al., 2018; Takata et al., 2019) and for population genetic analysis (Takahashi et al., 2016).
In this study, we used an exhaustive sampling strategy and conducted spatial autocorrelation analysis of two populations of a reef-building coral species, Heliopora coerulea, using both traditional microsatellite loci and MIG-seq. H. coerulea is a gorgonian coral (Anthozoa, Octocorallia) characterized by an exceptionally hard skeleton. Similar to the primary reef-building corals (Anthozoa, Hexacorallia), this species provides complex habitats for other reef species and thus contributes to sustain high biodiversity in coral reef ecosystems (Zann and Bolton, 1985). In some Indo-Pacific reefs, large populations of H. coerulea are found, and is a dominant coral species (Zann and Bolton, 1985; Takino et al., 2010; Yasuda et al., 2012; Atrigenio et al., 2017). However, the International Union for Conservation of Nature now lists this species as a threatened species because its habitats are degrading, similar to other reef-building coral species (Obura et al., 2008). In this context, identifying the spatial scale of an operational conservation unit – the spatial range of larval and gamete dispersal that occurs in ecological time scales – for H. coerulea, is important. Heliopora coerulea is a brooding species whose internal fertilization occurs inside female polyps, and almost-competent larvae are released once a year during the summer (Babcock, 1990; Harii et al., 2002; Taninaka et al., 2018). Consistent with the short dispersal duration (Harii et al., 2002), Harii and Kayanne (2003) used settlement tiles in Shiraho reef and directly demonstrated that H. coerulea larvae settle within a range of 350 m. Previous population genetic studies performed using microsatellite markers and numerical simulation analyses indicated that larval dispersal among different reef habitats is quite limited (Taninaka et al., 2019); however, one population genetic analysis study reported infrequent long-distance larval dispersal in multiple generations (Yasuda et al., 2014). Strong population genetic differentiation has been observed within a 20 m × 40 km area (Taninaka et al., 2019), indicating that H. coerulea may have a finer SGS even within a continuous large population. Individual-based SGS analysis is more useful to detect such a finer scale genetic structure than other population-based genetic methods, and thus would provide more practical larval dispersal estimates for species conservation. Thus, applying SGS analysis for large H. coerulea populations would be feasible and beneficial for the conservation of H. coerulea. SGS analysis often involves two complementary methods to estimate dispersal range (Peakall et al., 2003; Underwood et al., 2007); one is a conservative estimation that uses the x-intercept of the spatial correlogram and the other is a more progressive estimation to find the maximum first distance class that is significant. However, no study has ever compared the dispersal distances estimated by alternative SGS methods with direct larval dispersal measurements in the field.
Therefore, this study aimed (i) to examine the SGS of a reef building coral, H. coerulea, using traditional microsatellite and genome-wide SNP data for evaluating the effectiveness of using genome-wide SNPs for SGS analysis and then (ii) to compare the dispersal distances estimated by SGS with the direct larval dispersal measurement in the field reported in a previous study (Harii and Kayanne, 2003) to reveal which dispersal estimation method of SGS best matches the direct larval dispersal measurement in the field within ecological time scales.
Materials and Methods
Sample Collection and Study Sites
All samples from Shiraho reef were collected in 2007 for microsatellite analysis, and the genotyping data were re-used in this study (Yasuda et al., 2010). Samples from Akaishi reef were collected in August 2008 by snorkeling and were preserved in 99.5% ethanol. Genomic DNA samples for microsatellite and MIG-seq analysis were extracted using DNeasy Blood and Tissue kit (Qiagen) from ethanol-preserved tissue samples and were then genotyped as described by Yasuda et al. (2008, 2010). In brief, nine highly polymorphic microsatellite loci were amplified on a PCR thermal cycler, and electrophoresed on 6% Long Ranger sequencing gel (EMC Bioproducts) using SQ-5500E sequencer (Hitachi). Allele sizes were determined using FRAGLYS ver. 3 (Hitachi). First, we used 87 samples from the Shiraho population and 22 samples from the Akaishi population for both MIG-seq and microsatellite analyses (Figure 1). Additional microsatellite analysis was performed by increasing the number of samples (271 for Shiraho and 48 for Akaishi reef). In both the reefs, the maximum depth of the sampling sites during high tide was approximately 3 m and the morphologies of the colonies were either lobate growth form or thick-branching growth form that belongs to H. coerulea group in Taninaka et al. (2021).
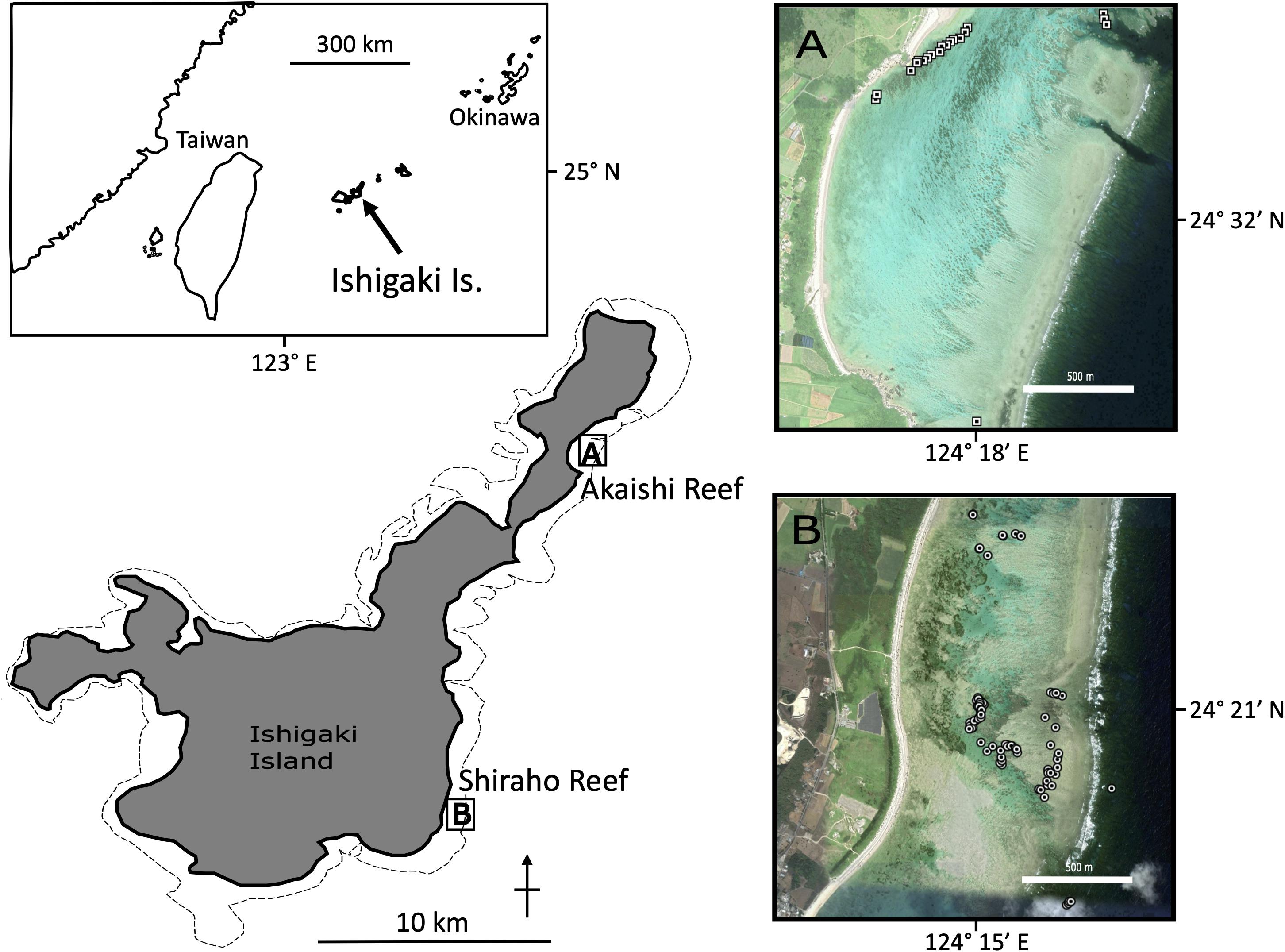
Figure 1. Map of sampling locations for H. coerulea. Each circles in (A) Shiraho reef and (B) Akaishi reef indicate the sampling points for individuals. The corresponding GPS coordinates can be found in Supplementary Materials.
Both the Shiraho and Akaishi populations are developed within fringing reefs forming micro-atoll structures (most of the colony tops reach the sea level during low tide) located on the southeast (Shiraho) and northeast (Akaishi) coast of Ishigaki Island, southwest Japan. The Shiraho population is one of the largest Heliopora populations in the Northern Hemisphere (Harii and Kayanne, 2003). Heliopora coerulea in Shiraho is densely, but unevenly distributed on the reef, forming a massive reef pavement behind the crest. Its abundance is high at the center of its distribution in the south (approximately 400 m NS in length with 200 m EW in width and the maximum distance between a pair of samples was found to be 1,288 m). However, the density decreased sharply, forming small patches with colonies spanning several meters to several decimeters in the further north and south of the reef (Figure 1). The Akaishi population is also a large, dense population that was discovered in 2008 (Takino et al., 2010). The Akaishi reef population is 400 m in length (NE–SW) and 30 m in width (NW–SE), and the maximum distance between a pair of samples was 1,948 m (Figure 1). There is a channel crossing the fringing reef crest that exchanges seawater via tidal currents approximately 400 m NE, away from the aggregated population, where we could find a few H. coerulea colonies. The Akaishi population is developed near the beach that is 60–80 m away, different from the Shiraho population, which is developed near the reef crest (Takino et al., 2010). The dense colonies of H. coerulea in each reef look continuous.
We tried the following two different sampling strategies: random sampling, covering the dense population as evenly as possible, and transect-like linear sampling with relatively regular sampling. In Shiraho reef, we randomly collected samples by covering the whole main dense populations in the south as well as patchily distributed samples in the north. In Akaishi reef, we linearly collected samples from the main population at regular intervals as much as possible, and also collected the patchily-distributed samples near the channel of the reef crest. The raw data for pair-wised geographic distances across samples and microsatellite data sets for Shiraho and Akaishi populations are available in Supplementary Data 1–3.
Single Nucleotide Polymorphisms Detection of MIG-seq
We performed MIG-seq analysis to obtain a relatively large number of SNPs, following the protocol described by Suyama and Matsuki (2015). We used eight pairs of multiplex ISSR primers (MIG-seq primer set 1 in Suyama and Matsuki, 2015). The DNA libraries from each sample with a different index were pooled and sequenced using MiSeq (Sequencing Control Software v2.0.12, Illumina) and MiSeq Reagent v3 150 cycle kit (Illumina). Image analysis and base calling were performed using the real-time analysis software v1.17.21 (Illumina). We then eliminated the low-quality reads and primer sequences from the raw data using FASTX-toolkit (version 0.0.14; fastaq_quality_filter) (Gordon and Hannon, 2012) with a fastq-quality-filter setting of -Q 33 -q 30 -p 40. We removed the adapter sequences for the Illumina MiSeq run from both the 5′ end (GTCAGATCGGAAGAGCACACGTCTGAACTCCAGTCAC) and the 3′ end (CAGAGATCGGAAGAGCGTCGTGTAGGGAA AGAC) using Cutadapt version 2.10 (Martin, 2011) with the −e 0.05 option, and we then excluded the short reads less than 80 bp. The quality-filtered sequence data were demultiplexed using Stacks v2.2 (Catchen et al., 2011, 2013). We used the GSTACKS program in Stacks v. 2.2 (Catchen et al., 2013; Rochette and Catchen, 2017) to genotype the individuals using the reference sequence of H. coerulea obtained from symbiont-free larvae (Richards et al., 2018). GSTACKS reads all input files simultaneously from the external alignment program, Burrows-WheelerAligner (BWA) version 0.0.17-r118 (Li and Durbin, 2009), and scans the genome for MIG-seq loci using a sliding window method. We used the GSTACKS option (-rm-pcr-duplicates) to remove PCR duplicates by randomly discarding all but one pair of each set of reads. We used the POPULATIONS program in Stacks v. 2.2 (Catchen et al., 2011) to exclude loci with more than 40% missing data (−r 0.6). We also changed the r values (0.9, 0.8, and 0.7) and confirmed that the results did not change for MIG-seq analysis (data not shown here). BayeScan v 2.1 (Narum and Hess, 2011) was used to detect the possible SNPs under natural selection, assuming different species or populations with a default setting for each data set. The SNPs data in the GenAlEx format are available in Supplementary Data 4.
Spatial Autocorrelation Analysis
Multilocus genotypes (MLGs) were determined using the microsatellite dataset. We assumed that the same MLGs found within each population by microsatellite analysis were possible asexually fragmented clones. GenAlEx ver. 6.5 (Peakall and Smouse, 2012) was used to calculate the probability of identity (PI) and spatial autocorrelation analysis. We used two different methods to estimate dispersal range (Peakall et al., 2003; Underwood et al., 2007). One method involved estimation of the dispersal range using the x-intercept of spatial autocorrelation correlograms, in which the correlation r is plotted for a given range of distance classes. If larval dispersal is limited and thus gene flow is restricted, the r value will be significantly positive in the short distance class and will then cross the x-axis and become negative (Peakall et al., 2003). The x-intercept indicates the point at which the random effect of genetic drift can be used to estimate the extent of the positive genetic structure. However, estimation of the x-intercept is strongly affected by the chosen distance class size and the number of samples within the distance class. In this study, because the dense sampling was within 400 m apart at both sites, we used a distance class size of up to 200 m to estimate the x-intercept value (so that if any, negative significance could be also detected using a sufficient number of sample pairs). We tested five different distance classes: 25, 50, 100, 150, and 200 m. Another method to estimate dispersal range involves finding the maximum first distant class using multiple distance class plots. Compared to the rather conservative permutation test of the x-intercept method, this method uses a more powerful permutation, but the range includes weak correlations (low r value). We used the Multiple Dclass option and a 50 m base distance class size for testing 16 distance class sizes.
The analysis superimposes the 95% confidence interval for the null hypothesis of random SGS on the correlogram. The number of permutation tests was set to 9,999 for each analysis. Although we conducted MIG-seq and microsatellite analysis using the same number of samples, we also performed extra spatial autocorrelation analyses using more samples collected only for the microsatellite data set to test whether the increased number of samples led to significant SGS detection in the microsatellite data (271 Shiraho and 48 Akaishi samples). All data are available in the Supplementary Material 1–4.
Results
Using nine microsatellite markers, we identified possible clone-mates as sample pairs that have exactly the same genotype. Based on the genotyping data using nine microsatellite markers, the PIs were 5.3 × 10–5 for Shiraho and 4.6 × 10–5 for Akaishi reef. In this study, to estimate the range of larval dispersal rather than fragmentation (asexual clonal propagation), we excluded possible clones and used a total of 87 Shiraho samples and 21 Akaishi samples for both MIG-seq and microsatellite analysis. In the microsatellite analysis samples, we used 266 Shiraho samples and 47 Akaishi samples after excluding the possible clones for subsequent analysis.
For MIG-seq analysis, 46,541,760 raw reads with an average of 426,989 reads per sample were obtained from 109 individuals. Low-quality reads were filtered, and 45,269,554 reads with an average of 415,317 reads per sample were obtained. We further excluded index, adapter, and short reads less than 80 bp, and obtained 13,245,206 reads with an average of 121,515 reads. In total, 6,535,144 reads were mapped onto the reference genome, with an average of 59,955 reads per sample. Finally, 2,012 SNPs were obtained from the MIG-seq analysis. BayeScan analysis indicated that no loci were under natural selection (q values >0.0.5); thus, we used all the loci for the subsequent analysis.
Spatial autocorrelation analysis using a moderate number of loci (2,012 SNPs) based on MIG-seq analysis indicated a significant SGS in both the Shiraho and Akaishi reefs; however, microsatellite analysis using the same number of samples as MIG-seq (87 Shiraho and 21 Akaishi samples) could not reveal a significant SGS in both reefs (Figure 2 and Table 1). By increasing the number of samples, spatial autocorrelation analysis using the microsatellite data (266 samples) in Shiraho reef indicated a significant genetic structure similar to MIG-seq analysis, whereas microsatellite analysis (47 samples, excluding one possible clone) in Akaishi reef could not reveal any significant genetic structure (Figure 2 and Table 1), indicating that MIG-seq analysis is more robust than microsatellite analysis for detecting SGS. Hereafter, we used the SGS data based on MIG-seq analysis.
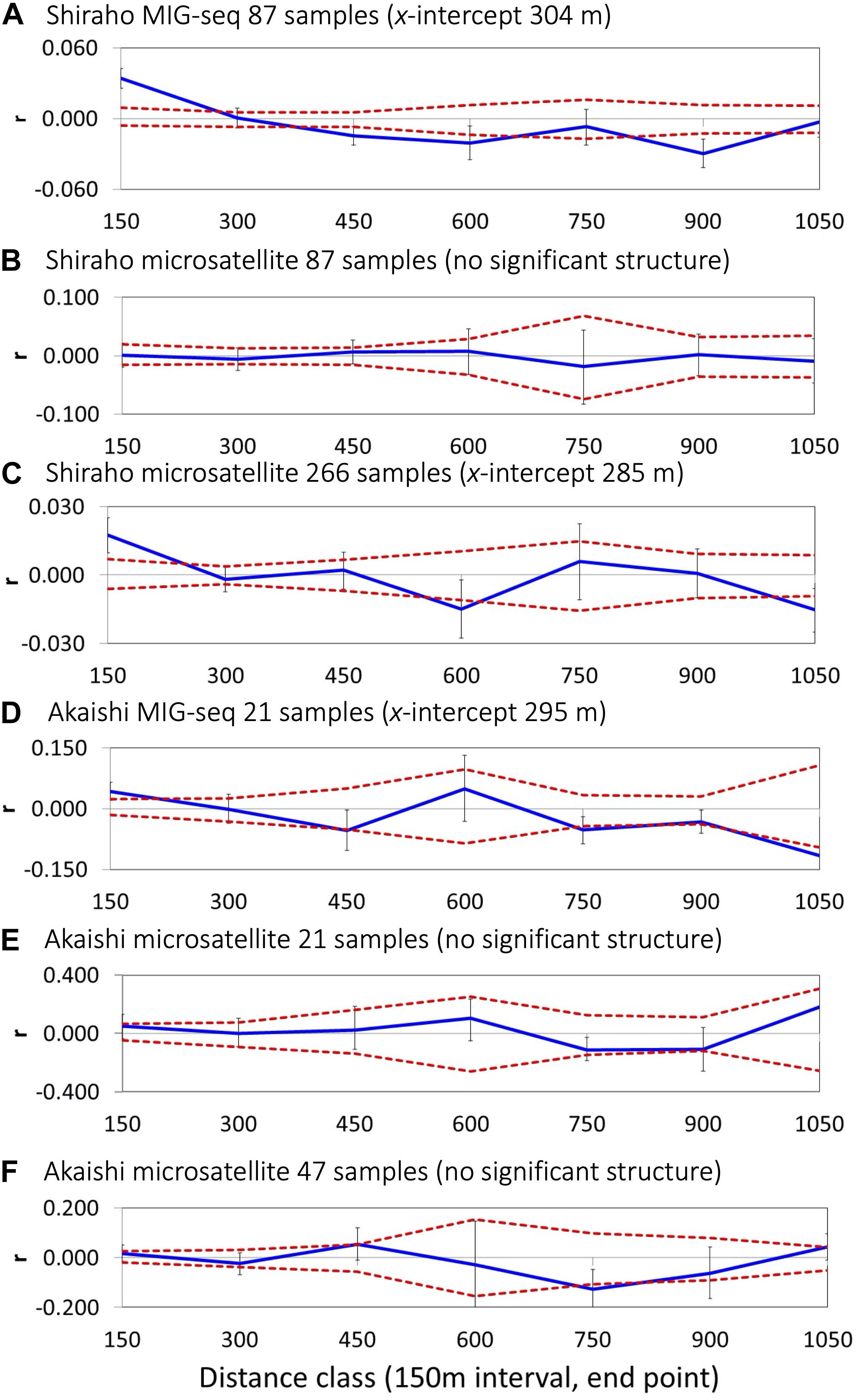
Figure 2. Correlograms showing the spatial correlation r as a function of distance, 95% CI about the null hypothesis of a random distribution of Heliopora coerulea, and 95% confidence error bars about r as determined by bootstrapping. Autocorrelation for the distance class size of 150 m for Shiraho reef using (A) MIG-seq data (n = 87), (B) nine microsatellite data (n = 87), (C) nine microsatellite data (n = 266) and that for Akaishi reef using (D) MIG-seq data (n = 21) and (E) nine microsatellite data (n = 21), (F) nine microsatellite data (n = 48).
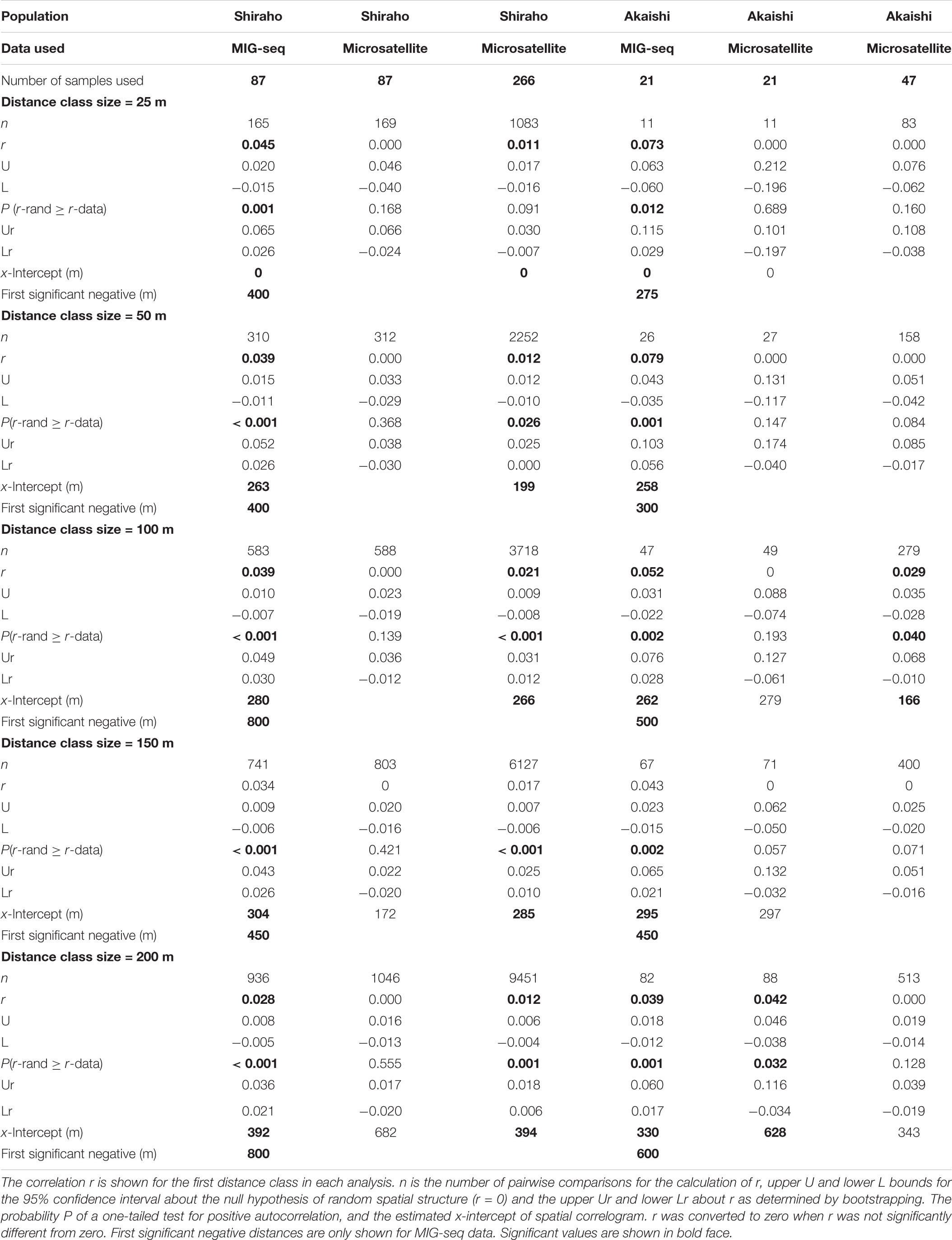
Table 1. The results of spatial autocorrelation of Heliopora coerulea and for the analysis with distance class sizes of 25, 50, 100, 150, and 200 m.
As mentioned above, the estimation of x-intercept was strongly affected by the selected distance class size and the number of samples within the distance class; using five different distance classes, the x-intercept values ranged from 255 to 392 m in Shiraho reef and from 241 to 330 m in Akaishi reef for MIG-seq analysis and from 199 to 394 m in Shiraho reef for microsatellite analysis (using 266 samples) (Table 1). The x-intercept increased as we used a larger distance class, whereas the correlation value r for the first distance class became smaller as we used a larger distance class (Table 1), indicating a stronger correlation within a smaller geographic range. On the contrary, a finer scale of isolation by distance patterns was detected using a smaller distance class; the shortest first negative significance was detected at 400 m (using 25 and 50 m of distance class) in Shiraho reef and at 275 m (using 25 m of distance class) in Akaishi reef (Table 1).
The maximum first distant class results indicated that the maximum significant distance was up to 750 m in both the Shiraho and Akaishi reefs (Figure 3).
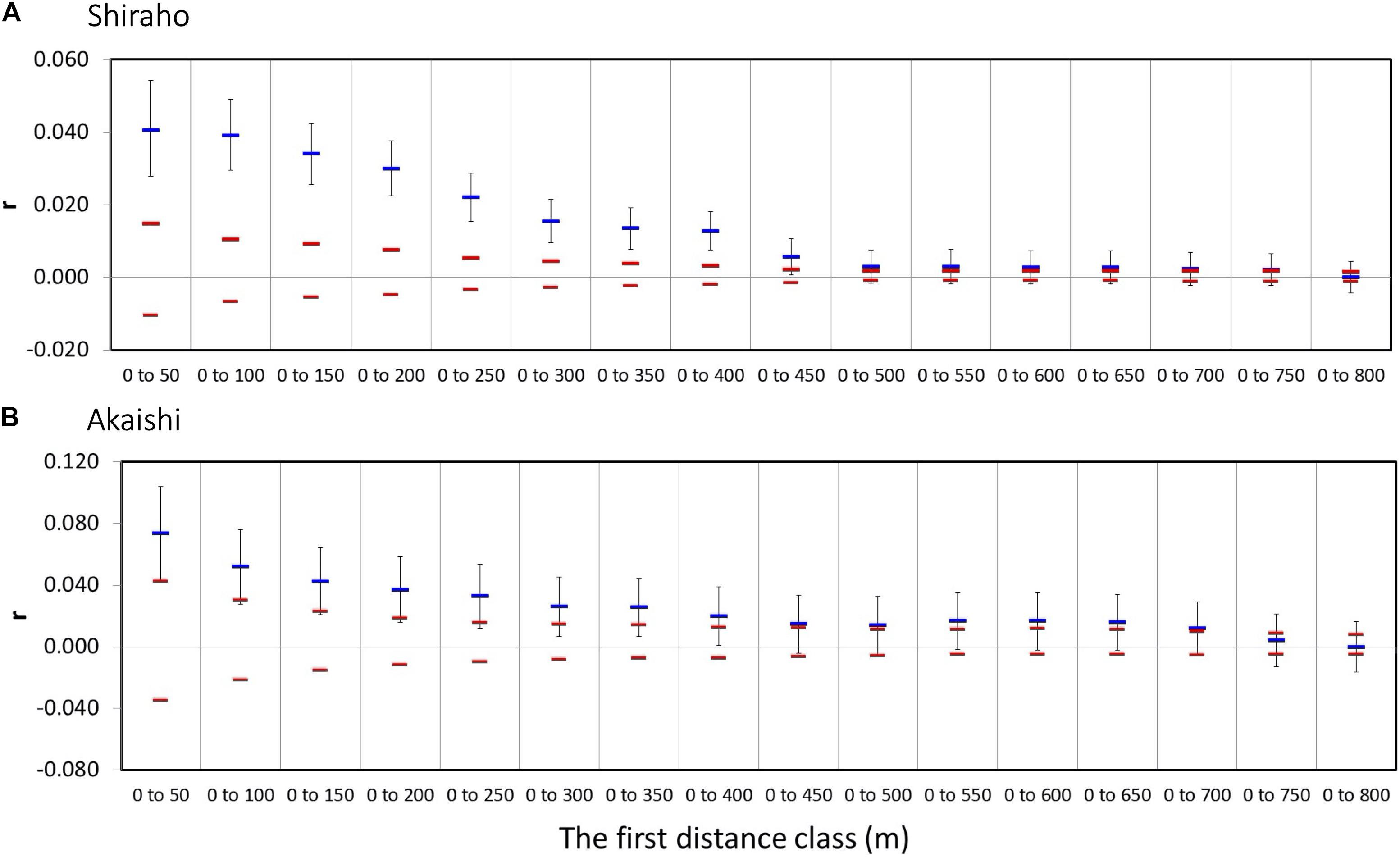
Figure 3. Multiple distance class plots of Heliopora coerulea showing the maximum significant first distance class size. The permuted 95% confidence intervals are also shown in red bars. (A) Shiraho reef and (B) Akaishi reef.
Discussion
MIG-seq Data for Spatial Autocorrelation Analysis
Using a moderate number of MIG-seq loci (2,012 SNPs and 87 Shiraho, 21 Akaishi samples), we successfully detected the significant SGS of H. coerulea populations both in Shiraho and Akaishi reef. Thus, proximate H. coerulea colonies were found to be genetically more alike than more distant colonies. Although spatial autocorrelation analysis is affected by several variances caused by population genetics, stochastic processes, and spatial processes (Slatkin and Arter, 1991), similar patterns of SGS were observed for the two different populations (Shiraho and Akaishi) with different microhabitat environments. This suggests that the positive spatial autocorrelation observed at short distance classes in MIG-seq analyses supports the small-scale isolation-by-distance patterns due to the limitation of gamete and larval dispersal in H. coerulea, as theoretically predicted for species with limited dispersal in the absence of natural selection (Smouse and Peakall, 1999).
In this study, we could directly compare the robustness of microsatellite markers and genome-wide SNP data to detect SGS in sessile shallow-water marine invertebrate species. Microsatellite data using a small number of samples failed to reveal significant structure whereas MIG-seq could successfully reveal significant SGS even when using the same number of samples, thus indicating the robustness of the moderate number of SNPs (2,012 SNPs) in MIG-seq for SGS analysis. It is possible that the resolution of detecting different genetic distances among individuals was improved by increasing the number of loci (from nine microsatellite loci to 2,012 SNPs). Notably, we could detect significant SGS similar to MIG-seq analysis when using a larger number of samples (266) in the microsatellite analysis. The increased number of samples might have compensated for the small number of loci, and therefore increased the power of SGS detection in the microsatellite analysis. This study demonstrated the robustness of MIG-seq analysis in detecting SGS, which can easily reveal a sufficient number of loci to detect SGS even with a limited number of samples.
Comparison of SGS Results and Larval Dispersal Based on Direct Field Observation
Estimating the larval dispersal of benthic marine species with pelagic larval duration that occurs on an ecological time scale (within a few generations) is often difficult to extract from genetic analysis, such as using F-statistics that include historical gene flow and assume genetic equilibrium (Benzie, 1999). The assignment test is a potential alternative method to estimate dispersal occurring within a few generations if the overall FST values are large, such as in terrestrial animals (Berry et al., 2004). Spatial autocorrelation analysis has been proven as a robust method for estimating dispersal range (e.g., Epperson and Li, 1996) and is often applied to plant species (e.g., Smouse and Peakall, 1999), but compared to plant, much less been applied to marine organisms, especially reef-building corals [although there are increasing number of recent references such as Underwood et al. (2007); Thomas et al. (2014), Chan et al. (2019); Dubé et al. (2020), and Underwood et al. (2020)]. This is partly because for marine species, the geographic positions of each individual are relatively laborious to record. In addition, this may also occur because interpretation of resultant distances using SGS (x-intercept and the maximum significant distance) is rather difficult, and is fundamentally related to the nature of larval dispersal itself. Even within the same population within a marine species, larval dispersal distance is highly variable and cannot be uniquely determined because of highly fluctuating ocean conditions as well as different dispersal potential among different individuals. In the case of H. coerulea, 74% of laboratory-cultured larvae settle within a day, but their competency lasts for up to 30 days (Harii et al., 2002), indicating that H. coerulea larvae have infrequent long-distance dispersal potential. The results of SGS in this study could also reflect this nature. The maximum significant (but with weak correlation value) distance found was 750 m for both Shiraho and Akaishi reefs, whereas the x-intercept using different distance classes ranged from 255 to 392 m in Shiraho reef and from 241 to 330 m in Akaishi reef. By testing five different distance classes (25–200 m), we found the minimum significantly negative spatial range to be 400 m (using the 25 and 50 m distance classes) in Shiraho and 275 m (using the 25 m distance class) in Akaishi reef, implying that most larvae and gametes do not disperse more than 400 m and 275 m in Shiraho and Akaishi reef, respectively. The estimated dispersal range based on the x-intercept corresponded well with the field observation that larval dispersal in Shiraho reef occurred within a range of 350 m (Harii and Kayanne, 2003), indicating that the x-intercept of the correlograms agrees well with the ecological larval dispersal range. Such larval dispersal range is essential for maintaining and/or recovering coral populations within an ecological time scale. This empirical demonstration also agreed well with the proposal by Diniz-Filho and De Campos Telles (2002) that the x-intercept of the spatial correlogram is useful for deciding the management unit for establishing conservation and sampling strategies.
It is also possible that larvae infrequently disperse up to 750 m in Shiraho and Akaishi, as small correlation values imply a maximum significant distance. A previous population genetic study also suggested an infrequent longer larval dispersal of H. coerulea (Yasuda et al., 2014). To our knowledge, this is the first study to compare larval dispersal distance measured in the field with the results of SGS analysis in a marine benthic species.
Larval and Gamete Dispersal of Heliopora coerulea
This study demonstrated that the larval and gamete dispersal of H. coerulea within well-developed fringing Shiraho and Akaishi reefs occurs at a maximum of 750 m, and the most of the dispersal occurs within 400 m. This indicates that if we are to collect samples from “one population” of H. coerulea from Shiraho and Akaishi populations, collection should be within the range of 750 m in diameter. In addition, to determine the conservation unit, a sustainable population within which a sufficient number of larvae can disperse and maintain or replenish the population within an ecological time scale would be less than 400 m in size. The maximum diameter of 750 m indicates that patchily-distributed colonies and dense colonies can be collected within 750 m as one meta-population. Less than 400 m diameter almost corresponded to the size of the dense populations of each studied reef, indicating that gene flow between the dense population and distant patchy colonies even within the same fringing reef should be conserved separately.
Spatial genetic structure analysis could not distinguish between gamete and larval dispersal. Although the gamete (sperm) dispersal range of H. coerulea is still unknown, our data suggest that gamete dispersal may not exceed larval dispersal within a fringing reef, indicating that the dispersal potential of H. coerulea sperm is also limited. This provides important insights for the conservation of H. coerulea. As H. coerulea is typically gonochoristic (only one exception was found by Taninaka and Yasuda, 2018), there would be a very low chance of fertilization if the male and female colonies are separated by more than 750 m in Shiraho and Akaishi reefs. Future studies on the spatial distribution of male and female colonies of H. coerulea in each population would be important for conservation, as such information is not available currently. The physical environment within well-developed fringing reefs, as in this study, is relatively calmer than that outside the reef crest, where corals are subject to stronger currents as described below. The larval and gamete dispersal distance of H. coerulea in a more open environment might thus be larger.
Although we obtained similar results from Shiraho and Akaishi reefs, we cannot generalize these dispersal distance results to other H. coerulea populations if each population is subject to different hydrodynamic and environmental conditions. Hydrodynamic surveys have been previously performed on Shiraho reef (e.g., Nakamori et al., 1992; Nadaoka et al., 2001) but not on Akaishi reef. Because of the shallow complex reef topologies associated with several coral microatoll and patches and emergence of well-developed reef crest during low tide, detailed measurement of the current system in Shiraho reef with acceptable accuracy is difficult (Tamura et al., 2007). Field measurement and numerical simulation in Shiraho reef during summer period showed that the current velocity and direction are highly variable depending on tidal cycle; the wave set-up effect and big channels play important role in the current system in Shiraho (Tamura et al., 2007). The study indicated that the overall weak southward tidal-driven current predominates around the dense population of H. coerulea. Harii and Kayanne (2003) found no larval disperse and settle in the northern patches in the field. They also found most H. coerulea larvae in Shiraho reef dispersed in mid to lower water column where the current velocity is weak (ranging from 1 cm s–1 during low tide and 3 cm s–1 during high tide). Given that the larvae of H. coerulea can only settle in slower flow velocity in the laboratory experiment (Harii and Kayanne, 2003), it is possible that H. coerulea larvae release only when the current velocity is weak regardless of the reef and tend to settle near their parent colonies, similar to our SGS analysis in Shiraho and Akaishi reefs.
Larval dispersal and subsequent SGS might be affected by other environmental factors. Based on the field measurement of the thermal characteristics in Shiraho reef, the distributions of different coral species well agreed with the thermal conditions among different reef micro-habitats (north and south of the moat, reef-flat, and reef-crest) during summer time (Kumagai et al., 2004). The distribution of the dense population of H. coerulea corresponded to relatively warm environment in Shiraho reef in the south (Kumagai et al., 2004). Such study implies the success of larval recruitment and survival, and subsequent SGS depends on the thermal condition of micros-habitats within a fringing reef as well as spatial competition with other coral species. It is also possible that the abundance and distribution of larval predators including butterfly fish (Villanueva and Edwards, 2010) affect dispersal and settlement within the reef.
This study also indicated that if the number of samples are sufficient, the estimated larval and gamete dispersal range found by microsatellite analysis and MIG-seq analysis are comparable and do not depend on the method used for analysis. Other reef-building shallow water brooder coral species whose dispersal range were estimated based on the microsatellite loci had a shorter SGS than that of H. coerulea; for instance, a brooding coral Pocillopora damicornis (Fan et al., 2006) with a maximum 100 days of competent larvae (Harii et al., 2002) shows only 16 m of dispersal distance based on the x-intercept of the correlogram (Tioho et al., 2001; Gorospe and Karl, 2013), whereas another brooding coral, Seriatopora hystrix (Fan et al., 2006) with at least 10 days of larval survival period in laboratory experiments (Isomura and Nishihira, 2001), shows a distance of 100 m (based on x-intercept) and up to 220 m (based on the multiple distance plots) (Underwood et al., 2007). These species are different from H. coerulea in that they are hermaphroditic brooder whose larvae have zooxanthellae (Atoda, 1947, 1951). It is unclear that why such brooding coral species that may have longer larval dispersal potential than H. coerulea have shorter dispersal range. It is possible other ecological factors such as abundance of predator of larvae and the environmental factors of the studied areas as discussed above constrain larval dispersal. Further research is needed to determine if and why H. coerulea has a relatively longer dispersal range as compared with the two brooding reef-building coral species. On the other hand, broadcast spawner coral species with longer dispersal potential, which does not release competent larvae like brooder corals and in in more open environment than fringing reef, had longer dispersal range <12 km (Takata et al., 2021) than H. coerulea as revealed by SGS analysis based on genome-wide SNPs like in this study.
Data Availability Statement
The datasets presented in this study can be found in online repositories. The names of the repository/repositories and accession number(s) can be found below: DDBJ (accession: DRA011718), FigShare (10.6084/m9.figshare.14910546).
Author Contributions
NY conceived the study and collected the samples. HT, TK, HY, and YS performed the MIG-seq analyses (including bioinformatics). DFM, HT, LS, and NY analyzed all the data. DFM and NY drafted and revised the manuscript. All authors have checked and agreed to the final manuscript.
Funding
This study was funded by the Japan Society for the Promotion of Science (grant numbers 17H04996, 19H03212, Grant-in-Aid for Research Fellows: 19J21342) and JSPS Bilateral Joint Research (JPJSBP120209929).
Conflict of Interest
The authors declare that the research was conducted in the absence of any commercial or financial relationships that could be construed as a potential conflict of interest.
Acknowledgments
Sampling was conducted under the Okinawa Prefectural Government permit (No. 18-34, 19-60).
Supplementary Material
The Supplementary Material for this article can be found online at: https://www.frontiersin.org/articles/10.3389/fmars.2021.702977/full#supplementary-material
Supplementary Data 1 | Pair-wised geographic distances of the samples.
Supplementary Data 2 | Microsatellite data of Shiraho reef.
Supplementary Data 3 | Microsatellite data of Akaishi reef.
Supplementary Data 4 | MIG-seq data in the GenAlEx format.
References
Adjeroud, M., and Tsuchiya, M. (1999). Genetic variation and clonal structure in the scleractinian coral Pocillopora damicornis in the Ryukyu Archipelago, Southern Japan. Mar. Biol. 134, 753–759. doi: 10.1007/s002270050592
Atoda, K. (1947). The larva and postlarval development of some reef-building corals. I. Pocillopora damicornis cespitosa (Dana). Sci. Rep. Tohoku Univ. 18, 24–47.
Atoda, K. (1951). The larva and postlarval development of some reef-building corals. V. Seriatopora hystrix Dana. Sci. Rep. Tohoku Univ. 19, 33–39.
Atrigenio, M., Aliño, P., and Conaco, C. (2017). Influence of the Blue coral Heliopora coerulea on scleractinian coral larval recruitment. J. Mar. Sci. 2017:6015143. doi: 10.1155/2017/6015143
Babcock, R. (1990). Reproduction and development of the blue coral Heliopora coerulea (Alcyonaria: Coenothecalia). Mar. Biol. 104, 475–481. doi: 10.1007/BF01314352
Benzie, J. A. H. (1999). Genetic structure of coral reef organisms: ghosts of dispersal past. Am. Zool. 39, 131–145. doi: 10.1093/icb/39.1.131
Berry, O., Tocher, M. D., and Sarre, S. D. (2004). Can assignment tests measure dispersal? Mol. Ecol. 13, 551–561. doi: 10.1046/j.1365-294X.2004.2081.x
Billingham, M., and Ayre, D. J. (1996). Genetic subdivision in the subtidal, clonal sea anemone Anthothoe albocincta. Mar. Biol. 125, 153–163. doi: 10.1007/BF00350769
Calderón, I., Ortega, N., Duran, S., Becerro, M., Pascual, M., and Turon, X. (2007). Finding the relevant scale: clonality and genetic structure in a marine invertebrate (Crambe crambe, Porifera). Mol. Ecol. 16, 1799–1810. doi: 10.1111/j.1365-294X.2007.03276.x
Carpenter, K. E., Abrar, M., Aeby, G., Aronson, R. B., Banks, S., Bruckner, A., et al. (2008). One-third of reef-building corals face elevated extinction risk from climate change and local impacts. Science 321, 560–563. doi: 10.1126/science.1159196
Catchen, J. M., Amores, A., Hohenlohe, P., Cresko, W., and Postlethwait, J. H. (2011). Stacks: building and genotyping loci de novo from short-read sequences. G3 1, 171–182. doi: 10.1534/g3.111.000240
Catchen, J., Hohenlohe, P. A., Bassham, S., Amores, A., and Cresko, W. A. (2013). Stacks: an analysis tool set for population genomics. Mol. Ecol. 22, 3124–3140. doi: 10.1111/mec.12354
Chan, A. N., Lewis, C. L., Neely, K. L., and Baums, I. B. (2019). Fallen pillars: the past, present, and future population dynamics of a rare, specialist coral–algal symbiosis. Front. Mar. Sci. 6:218. doi: 10.3389/fmars.2019.00218
Connolly, S. R., and Baird, A. H. (2010). Estimating dispersal potential for marine larvae: dynamic models applied to scleractinian corals. Ecology 91, 3572–3583. doi: 10.1890/10-0143.1
Diniz-Filho, J. A. F., and De Campos Telles, M. P. (2002). Spatial autocorrelation analysis and the identification of operational units for conservation in continuous populations. Conserv. Biol. 16, 924–935. doi: 10.1046/j.1523-1739.2002.00295.x
Dubé, C. E., Boissin, E., Mercière, A., and Planes, S. (2020). Parentage analyses identify local dispersal events and sibling aggregations in a natural population of Millepora hydrocorals, a free-spawning marine invertebrate. Mol. Ecol. 29, 1508–1522. doi: 10.1111/mec.15418
Epperson, B. K. (2005). Estimating dispersal from short distance spatial autocorrelation. Heredity 95, 7–15. doi: 10.1038/sj.hdy.6800680
Epperson, B. K., and Li, T. (1996). Measurement of genetic structure within populations using Moran’s spatial autocorrelation statistics. Proc. Natl. Acad. Sci. U.S.A. 93, 10528–10532. doi: 10.1073/pnas.93.19.10528
Fan, T. Y., Lin, K. H., Kuo, F. W., Soong, K., Liu, L. L., and Fang, L. S. (2006). Diel patterns of larval release by five brooding scleractinian corals. Mar. Ecol. Prog. Ser. 321, 133–142.
Fisk, D. A., and Harriott, V. J. (1990). Spatial and temporal variation in coral recruitment on the great barrier reef: implications for dispersal hypotheses. Mar. Biol. 107, 485–490. doi: 10.1007/BF01313433
Gordon, A., and Hannon, G. J. (2012). FASTX-Toolkit. FASTQ/A Short-Reads Pre-Processing Tools. Available online at: http://hannonlab.cshl.edu/fastx_toolkit/
Gorospe, K. D., and Karl, S. A. (2013). Genetic relatedness does not retain spatial pattern across multiple spatial scales: dispersal and colonization in the coral, Pocillopora damicornis. Mol. Ecol. 22, 3721–3736. doi: 10.1111/mec.12335
Harii, S., and Kayanne, H. (2003). Larval dispersal, recruitment, and adult distribution of the brooding stony octocoral Heliopora coerulea on Ishigaki Island, Southwest Japan. Coral Reefs 22, 188–196. doi: 10.1007/s00338-003-0302-9
Harii, S., Kayanne, H., Takigawa, H., Hayashibara, T., and Yamamoto, M. (2002). Larval survivorship, competency periods and settlement of two brooding corals, Heliopora coerulea and Pocillopora damicornis. Mar. Biol. 141, 39–46. doi: 10.1007/s00227-002-0812-y
Isomura, N., and Nishihira, M. (2001). Size variation of planulae and its effect on the lifetime of planulae in three pocilloporid corals. Coral Reefs 20, 309–315. doi: 10.1007/s003380100180
Kumagai, W., Tamura, H., Nadaoka, K., Harii, S., Mitsui, J., Suzuki, Y., et al. (2004). Characteristics of thermal environment and distribution of reef-building coral communities in Shiraho reef, Ishigaki Island. J. JSCE 51, 1066–1070. doi: 10.2208/proce1989.51.1066
Li, H., and Durbin, R. (2009). Fast and accurate short read alignment with Burrows-Wheeler transform. Bioinformatics 25, 1754–1760. doi: 10.1093/bioinformatics/btp324
Martin, M. (2011). Cutadapt removes adapter sequences from high-throughput sequencing reads. EMBnet J. 17, 10–12. doi: 10.14806/ej.17.1.200
McFadden, C. S., and Aydin, K. Y. (1996). Spatial autocorrelation analysis of small-scale genetic structure in a clonal soft coral with limited larval dispersal. Mar. Biol. 126, 215–224. doi: 10.1007/BF00347446
Nadaoka, K., Nihei, Y., Kumano, R., Yokobori, T., Omija, T., and Wakaki, K. (2001). A field observation on hydrodynamic and thermal environments of a fringing reef at Ishigaki Island under typhoon and normal atmospheric conditions. Coral Reefs 20, 387–398. doi: 10.1007/s00338-001-0188-3
Nakamori, T., Suzuki, A., and Iryu, Y. (1992). Water circulation and carbon flux on Shiraho coral reef of the Ryukyu Islands, Japan. Cont. Shelf Res. 12, 951–970. doi: 10.1016/0278-4343(92)90054-N
Narum, S. R., and Hess, J. E. (2011). Comparison of FST outlier tests for SNP loci under selection. Mol. Ecol. Resour. 11, (Suppl. 1), 184–194. doi: 10.1111/j.1755-0998.2011.02987.x
Obura, D., Fenner, D., Hoeksema, B., Devantier, L., and Sheppard, C. (2008). Heliopora coerulea. The IUCN Red List of Threatened Species 2008: e.T133193A3624060. Available online at: https://dx.doi.org/10.2305/IUCN.UK.2008.RLTS.T133193A3624060.en
Peakall, R., Ruibal, M., and Lindenmayer, D. B. (2003). Spatial autocorrelation analysis offers new insights into gene flow in the Australian Bush Rat, Rattus fuscipes. Evolution 57, 1182–1195. doi: 10.1554/0014-3820(2003)057[1182:SAAONI]2.0.CO;2
Peakall, R., and Smouse, P. E. (2012). GenAlEx 6.5: genetic analysis in excel. population genetic software for teaching and research–an update. Bioinformatics 28, 2537–2539. doi: 10.1093/bioinformatics/bts460
Richards, Z. T., Yasuda, N., Kikuchi, T., Foster, T., Mitsuyuki, C., Stat, M., et al. (2018). Integrated evidence reveals a new species in the ancient blue coral genus Heliopora (Octocorallia). Sci. Rep. 8:15875. doi: 10.1038/s41598-018-32969-z
Rochette, N. C., and Catchen, J. M. (2017). Deriving genotypes from RAD-seq short-read data using Stacks. Nat. Protoc. 12, 2640–2659. doi: 10.1038/nprot.2017.123
Schwartz, M. K., and McKelvey, K. S. (2009). Why sampling scheme matters: the effect of sampling scheme on landscape genetic results. Conserv. Genet. 10, 441–452. doi: 10.1007/s10592-008-9622-1
Slatkin, M., and Arter, H. E. (1991). Spatial autocorrelation methods in population genetics. Am. Nat. 138, 499–517. doi: 10.1086/285228
Smouse, P. E., and Peakall, R. (1999). Spatial autocorrelation analysis of individual multiallele and multilocus genetic structure. Heredity 82, 561–573. doi: 10.1038/sj.hdy.6885180
Suyama, Y., and Matsuki, Y. (2015). MIG-seq: an effective PCR-based method for genome-wide single-nucleotide polymorphism genotyping using the next-generation sequencing platform. Sci. Rep. 5:16963. doi: 10.1038/srep16963
Takahashi, Y., Suyama, Y., Matsuki, Y., Funayama, R., Nakayama, K., and Kawata, M. (2016). Lack of genetic variation prevents adaptation at the geographic range margin in a damselfly. Mol. Ecol. 25, 4450–4460. doi: 10.1111/mec.13782
Takata, K., Iwase, F., Iguchi, A., Yuasa, H., Taninaka, H., Iwasaki, N., et al. (2021). Genome-wide SNPs data revealed significant spatial genetic structure in the deep- sea precious coral Corallium japonicum. Front. Mar. Sci. 8:667481. doi: 10.3389/fmars.2021.667481
Takata, K., Taninaka, H., Nonaka, M., Iwase, F., Kikuchi, T., Suyuma, Y., et al. (2019). Multiplexed ISSR genotyping by sequencing distinguishes two precious coral species (Anthozoa: Octorallia: Coralliidae) that share a mitochondrial haplotype. PeerJ 7:e7769. doi: 10.7717/peerj.7769
Takino, T., Watanabe, A., Motooka, S., Nadaoka, K., Yasuda, N., and Taira, M. (2010). Discovery of a large population of Heliopora coerulea at Akaishi Reef, Ishigaki Island, Southwest Japan. Galaxea J. Coral Reef Stud. 12, 85–86.
Tamura, H., Nadaoka, K., and Paringit, E. C. (2007). Hydrodynamic characteristics of a fringing coral reef on the east coast of Ishigaki Island, southwest Japan. Coral Reefs 26, 17–34. doi: 10.1007/s00338-006-0164-z
Taninaka, H., and Yasuda, N. (2018). Occurrence of simultaneous hermaphrodite in the “gonochoric” octocoral Heliopora coerulea (Pallas, 1766). Galaxea J. Coral Reef Stud. 20, 29–30.
Taninaka, H., Bernardo, L. P. C., Saito, Y., Nagai, S., Ueno, M., Kitano, Y. F., et al. (2019). Limited fine-scale larval dispersal of the threatened brooding corals Heliopora spp. as evidenced by population genetics and numerical simulation. Conserv. Genet. 20, 1449–1463. doi: 10.1007/s10592-019-01228-7
Taninaka, H., Harii, S., Kagawa, H., Ueno, M., Kitano, F. Y., Saito, Y., et al. (2018). Estimation of the reproductive timing of two genetically different lineages of the blue coral Heliopora coerulea (Pallas, 1766) around Sekisei Lagoon. J. Jpn. Coral Reef Soc. 20, 39–51. doi: 10.3755/jcrs.20.39
Taninaka, H., Maggoioni, D., Seveso, D., Huang, D., Townsend, A., Richards, Z., et al. (2021). Phylogeography of blue corals (genus Heliopora) across the Indo-West Pacific. Front. Mar. Sci. 8:714662 doi: 10.3389/fmars.2021.714662
Thomas, L., Kendrick, G. A., Stat, M., Travaille, K. L., Shedrawi, G., and Kennington, W. J. (2014). Population genetic structure of the Pocillopora damicornis morphospecies along Ningaloo Reef, Western Australia. Mar. Ecol. Prog. Ser. 513, 111–119. doi: 10.3354/meps10893
Tioho, H., Tokeshi, M., and Nojima, S. (2001). Experimental analysis of recruitment in a scleractinian coral at high latitude. Mar. Ecol. Prog. Ser. 213, 79–86. doi: 10.3354/meps213079
Underwood, J. N., Richards, Z., Berry, O., Oades, D., Howard, A., and Gilmour, J. P. (2020). Extreme seascape drives local recruitment and genetic divergence in brooding and spawning corals in remote north-west Australia. Evol. Appl. 13, 2404–2421. doi: 10.1111/eva.13033
Underwood, J. N., Smith, L. D., Van Oppen, M. J. H., and Gilmour, J. P. (2007). Multiple scales of genetic connectivity in a brooding coral on isolated reefs following catastrophic bleaching. Mol. Ecol. 16, 771–784. doi: 10.1111/j.1365-294X.2006.03187.x
Villanueva, R. D., and Edwards, A. J. (2010). Butterflyfishes feed on externally brooded larvae of the blue coral, Heliopora coerulea. Coral reefs. 29, 105–105. doi: 10.1007/s00338-009-0553-1
Wilkinson, C. (2006). Status of coral reefs of the world: summary of threats and remedial action. Coral Reef Conserv. 13, 3–39.
Yasuda, N., Abe, M., Takino, T., Kimura, M., Lian, C., Nagai, S., et al. (2012). Large-scale mono-clonal structure in the north peripheral population of blue coral, Heliopora coerulea. Mar. Genomics. 7, 33–35. doi: 10.1016/j.margen.2012.02.001
Yasuda, N., Nagai, S., Lian, C., Hamaguchi, M., Hayashibara, T., and Nadaoka, K. (2008). Identification and characterization of microsatellite loci in the blue coral Heliopora coerulea (Alcynonaria: Coenothecalia). Conserv. Genet. 9, 1011–1013. doi: 10.1007/s10592-007-9436-6
Yasuda, N., Takino, T., Kimura, M., Lian, C., Nagai, S., and Nadaoka, K. (2010). “Genetic structuring across the reef crest in the threatened blue coral Heliopora coerulea (Helioporidae, Octacorallia) in Shiraho reef, Southwest Japan,” in Advances in Genetics Research, ed. V. K. Urbano (New York, NY: Nova Science Publishers), 315–324.
Yasuda, N., Taquet, C., Nagai, S., Fortes, M., Fan, T. Y., Phongsuwan, N., et al. (2014). Genetic structure and cryptic speciation in the threatened reef-building coral Heliopora coerulea along Kuroshio Current. Bull. Mar. Sci. 90, 233–255. doi: 10.5343/bms.2012.1105
Yund, P. O., and O’Neil, P. G. (2000). Microgeographic genetic differentiation in a colonial ascidian (Botryllus schlosseri) population. Mar. Biol. 137, 583–588. doi: 10.1007/s002270000378
Keywords: spatial autocorrelation analysis, MIG-seq, coral reefs, reef-building coral, larval dispersal
Citation: Mokodongan DF, Taninaka H, Sara L, Kikuchi T, Yuasa H, Suyama Y and Yasuda N (2021) Spatial Autocorrelation Analysis Using MIG-seq Data Indirectly Estimated the Gamete and Larval Dispersal Range of the Blue Coral, Heliopora coerulea, Within Reefs. Front. Mar. Sci. 8:702977. doi: 10.3389/fmars.2021.702977
Received: 30 April 2021; Accepted: 28 June 2021;
Published: 20 July 2021.
Edited by:
Wei Jiang, Guangxi University, ChinaReviewed by:
Rafael A. Cabral-Tena, Center for Scientific Research and Higher Education in Ensenada (CICESE), MexicoCecilia Conaco, University of the Philippines Diliman, Philippines
Copyright © 2021 Mokodongan, Taninaka, Sara, Kikuchi, Yuasa, Suyama and Yasuda. This is an open-access article distributed under the terms of the Creative Commons Attribution License (CC BY). The use, distribution or reproduction in other forums is permitted, provided the original author(s) and the copyright owner(s) are credited and that the original publication in this journal is cited, in accordance with accepted academic practice. No use, distribution or reproduction is permitted which does not comply with these terms.
*Correspondence: Nina Yasuda, nina27@cc.miyazaki-u.ac.jp
†These authors share first authorship