- 1Department of Meteorology and Atmospheric Science, The Pennsylvania State University, University Park, PA, United States
- 2Virginia Institute of Marine Science, William & Mary, Gloucester Point, VA, United States
- 3Department of Materials Science and Engineering, The Pennsylvania State University, University Park, PA, United States
- 4Department of Geography, The Pennsylvania State University, University Park, PA, United States
Public awareness of microplastics and their widespread presence throughout most bodies of water are increasingly documented. The accumulation of microplastics in the ocean, however, appears to be far less than their riverine inputs, suggesting that there is a “missing sink” of plastics in the ocean. Estuaries have long been recognized as filters for riverine material in marine biogeochemical budgets. Here we use a model of estuarine microplastic transport to test the hypothesis that the Chesapeake Bay, a large coastal-plain estuary in eastern North America, is a potentially large filter, or “sink,” of riverine microplastics. The 1-year composite simulation, which tracks an equal number of buoyant and sinking 5-mm diameter particles, shows that 94% of riverine microplastics are beached, with only 5% exported from the Bay, and 1% remaining in the water column. We evaluate the robustness of this finding by conducting additional simulations in a tributary of the Bay for different years, particle densities, particle sizes, turbulent dissipation rates, and shoreline characteristics. The resulting microplastic transport and fate were sensitive to interannual variability over a decadal (2010–2019) analysis, with greater export out of the Bay during high streamflow years. Particle size was found to be unimportant while particle density – specifically if a particle was buoyant or not – was found to significantly influence overall fate and mean duration in the water column. Positively buoyant microplastics are more mobile due to being in the seaward branch of the residual estuarine circulation while negatively buoyant microplastics are transported a lesser distance due to being in the landward branch, and therefore tend to deposit on coastlines close to their river sources, which may help guide sampling campaigns. Half of all riverine microplastics that beach do so within 7–13 days, while those that leave the bay do so within 26 days. Despite microplastic distributions being sensitive to some modeling choices (e.g., particle density and shoreline hardening), in all scenarios most of riverine plastics do not make it to the ocean, suggesting that estuaries may serve as a filter for riverine microplastics.
Introduction
Small (∼5 mm) plastic debris has been a known contaminant in the marine environment for decades (Carpenter and Smith, 1972), although it is only since the beginning of the 21st century that significant attention has been given to their pervasiveness (Eriksen et al., 2014), potential environmental damage (Cole et al., 2011), and human health risks (Campanale et al., 2020). While some measurements of microplastic (≤5 mm) abundance in the environment may be biased high due to the inclusion of non-plastic microparticles, emphasizing the need for chemical analysis in addition to visual classification (Ivar do Sul, 2021), microplastics are still prevalent throughout the global ecosystem. Microplastics can originate from many components of society, with car tires and laundry textiles often being the dominating sources (Galafassi et al., 2019). The full extent of potential hazards of micro and nanoplastics (≤1 μm) in the human body is still unknown, but known risks include inflammations, lesions, damage to cells and DNA, and neoplasia, and that is only accounting for the microplastics themselves, not the compounds known to adsorb onto microplastics (Campanale et al., 2020). Finally, small plastic debris also serves as a vector for invasive species, by serving as ecosystem footholds (Riascos et al., 2019), and for organic pollutants and trace metals, many of which are known toxins and carcinogens (Hirai et al., 2011).
The factors controlling microplastics behavior (e.g., distribution and removal) in the environment are still uncertain, with much focus on biofilms and biofouling affecting particle density (Rummel et al., 2017). To this effect, there is potentially a large missing sink of plastics in the ocean (Law and Thompson, 2014); 0.4–13 million tons of plastics leave the land each year (Jambeck et al., 2015; Lebreton et al., 2017; Schmidt et al., 2017), yet only 7000–250,000 tons of plastics are estimated to be in the surface ocean (Cózar et al., 2014; Eriksen et al., 2014). One possibility is that the sources, which are diverse (sewage, industry, shipping, fishing, etc.), are poorly characterized (Vermeiren et al., 2016) and overestimated in the literature. Another possibility is that export to the deep ocean may account for a significant percentage of missing plastics (Woodall et al., 2014; Kane et al., 2020; Kvale et al., 2020; Pabortsava and Lampitt, 2020). Additionally, it is becoming increasingly likely that plastics are being trapped in estuaries and along coastlines (beaching) before reaching the open ocean (Lebreton et al., 2019; Martin et al., 2020; Olivelli et al., 2020; Ryan et al., 2020; Chenillat et al., 2021).
Estuaries may act as a filter for riverine microplastics, which are about half the total riverine plastics load (Schmidt et al., 2017), much as they act as filters for a host of other riverine materials (Schubel and Kennedy, 1984), although likely via different mechanisms; for example, estuaries substantially reduce the export of nutrients (McGlathery et al., 2007; Asmala et al., 2017) and carbon (Najjar et al., 2018; Hutchings et al., 2020) to continental shelf waters. Perhaps most relevant to microplastics is the effectiveness of estuaries as traps for riverine sediment, which very often manifests as a turbidity maximum in the upper portions of estuaries (Burchard et al., 2018).
Here we use a model of estuarine microplastic transport to test the hypothesis that a large estuary, the Chesapeake Bay, is a potentially large filter, or “sink,” of riverine microplastics. Numerical modeling has been an essential tool for understanding microplastic distributions in the open ocean environment. However, in contrast to the plethora of microplastics modeling studies in this environment (Eriksen et al., 2014; Hardesty et al., 2017; Sterl et al., 2020), such studies are rare in estuarine and coastal waters, despite the benefit they provide by informing monitoring and sampling efforts as well as the development of budgets. Even within this small group of studies, primary differences exist in particle density, the presence of beaching, the input location of particles, and simulation length. Beaching, when included, was found to have a large impact on microplastics fate in numerous modeling studies, including those of Jervis Bay, Australia (Jalón-Rojas et al., 2019); San Francisco Bay, United States (Sutton et al., 2019); the East China, Yellow, and Bohai Seas (Zhang et al., 2020); the Mediterranean Sea (Liubartseva et al., 2018); and a small (104 km2) coastal region of eastern Australia (Critchell and Lambrechts, 2016). Modeling suggests that the estuarine circulation itself can also trap microplastics (Díez-Minguito et al., 2020), much in the way the estuarine turbidity maximum forms. Two studies identified particle density to be an important factor in distribution and fate (Jalón-Rojas et al., 2019; Sutton et al., 2019). Numerous studies are idealized in terms of the input location of particles (Critchell and Lambrechts, 2016; Iwasaki et al., 2017; Cohen et al., 2019; Genc et al., 2020), but several include sources emanating from rivers and estuaries (Liubartseva et al., 2018; Sutton et al., 2019; Zhang et al., 2020). Simulation lengths vary dramatically, from several days (Critchell and Lambrechts, 2016) to a decade (Iwasaki et al., 2017). Overall, beaching and particle density stand out as notable factors in influencing the fate of microplastics. However, notable among all of these studies is the general lack of simulations of the fate of riverine plastics in estuaries, including beaching, with the study of Sutton et al. (2019) being the main exception.
The Chesapeake Bay is one of North America’s largest estuaries. The Bay is a coastal plain (drowned river valley) temperature estuary that is partially stratified and contains numerous sub-estuaries, particularly along the western shore, in addition to a main stem. The Chesapeake Bay is fed from a watershed that is home to over 18 million people (Moore et al., 2018), with a long history of water quality degradation, including excess nutrients, high levels of chlorophyll, declines in clarity, increases in hypoxia, and reductions in critical flora and fauna, such as seagrass and oysters (Kemp et al., 2005).
In contrast to the extensive water quality database that has been generated from regular monitoring since 1985, sampling efforts for microplastics within the waters of this heavily populated region are limited (Yonkos et al., 2014; Bikker et al., 2020) and are mostly located in the mid- and upper-portions of the Bay (Figure 1). Yonkos et al. (2014) sampled four tributaries five times using a 330 μm mesh net during July to December 2011, finding microplastic concentrations at these sites to be correlated with nearby population density and the percentage of developed or urbanized land use. Bikker et al. (2020) sampled 30 sites along the mid- to upper-mainstem of the Chesapeake Bay using a 330 μm mesh net during August–September 2015, finding that fragments, films, and fibers accounted for over 75% of sampled microplastics, and that concentrations were higher near cities or where large rivers entered the estuary than in the mainstem. Coles et al. (2020) sampled numerous creeks and streams in the northeastern United States using a 333 μm mesh net, seven of which are within the Chesapeake Bay watershed. These seven sites were sampled twice between June 2017 and July 2018, once during baseflow conditions and once during stormflow conditions, and had concentrations greater by an order of magnitude or more than those of Yonkos et al. (2014), which in turn reported concentrations an order of magnitude more than those of Bikker et al. (2020). The Yonkos et al. (2014) samples were processed differently than the Bikker et al. (2020) and Coles et al. (2020) datasets, the latter two using nearly the same protocol; this may have imparted a bias. This pattern of decreasing concentration moving from creeks and streams to tributaries to the middle to upper mainstem is in keeping with the finding of Bikker et al. (2020). The few in situ measurements available allow for a model comparison, and with future microplastic sampling campaigns being planned by the broader community, these model simulations can further guide those efforts.
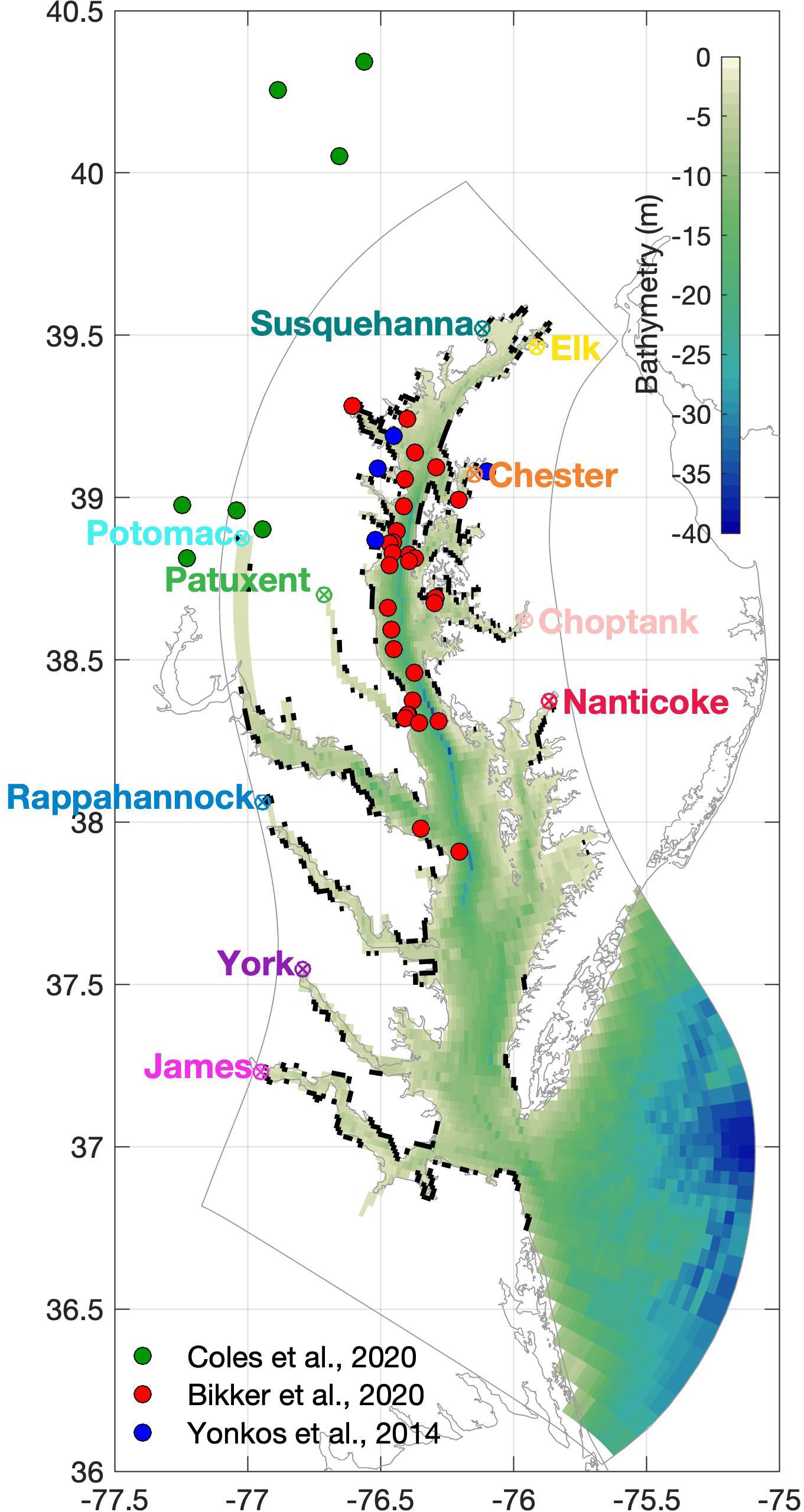
Figure 1. ChesROMS model bathymetry and the 10 locations where particles are released into the model. Locations of observations from three different studies are also shown. Black segments along coastline are considered armored in the particle tracking model.
Two recent synthesis reports on Chesapeake Bay microplastics noted the lack of information about microplastic distribution (Wardrop et al., 2016; Murphy et al., 2019), and the importance of the specific patterns of microplastic distribution to its living resources. We expect that answers to the following questions will help to guide future estuarine microplastic sampling campaigns. In addition to evaluating our hypothesis that the Chesapeake Bay traps riverine microplastics, we intend to discern distribution patterns and the factors that affect microplastic transport. Questions we seek to answer are (1) Where do microplastics reside in the Bay water column and shoreline? (2) What fraction of microplastics is beached vs. exported from the Bay? (3) How long does it take for beaching and export to occur? (4) How do microplastic distributions vary seasonally and interannually? (5) Do the answers to these questions meaningfully change with model parameters, including particle size and density?
Methodology
We simulate the transport and distribution of microplastics in the Chesapeake Bay with a particle-tracking model that uses currents derived from a hydrodynamic model. We chose a duration of 1 year for our complete simulation that traces particles from all of the major rivers emptying into the Chesapeake Bay, roughly twice duration of the Bay’s mean residence time of 180 days (Du and Shen, 2016). This simulation, which is referred to as the composite case, considers particles of a single size (5 mm) and three different buoyancies (0.90 g m–3 for positively buoyant particles, 1.345 g cm–3 for negatively buoyant particles, and lastly neutral buoyant particles) and has beaching only along non-armored shorelines. In order to assess sensitivity to year, horizontal diffusivity, particle size, particle density, and armoring, numerous additional simulations were conducted with particles sourced from only one river.
Hydrodynamic Model
The hydrodynamic model used is ChesROMS, an application of the regional ocean modeling system (ROMS) to the Chesapeake Bay (Xu et al., 2012). ROMS solves the 3-D, hydrostatic, Boussinesq, primitive equations in a structured horizontal grid with terrain-following vertical coordinates (Shchepetkin and McWilliams, 2005, 2009; Haidvogel et al., 2008). The ChesROMS configuration used here is based upon Da et al. (2018) and its previous iterations have been extensively used for circulation, biogeochemistry, and sediment transport studies within the Chesapeake Bay (Feng et al., 2015; Irby and Friedrichs, 2019; St-Laurent et al., 2020; Moriarty et al., 2021; Turner et al., 2021). The model has a curvilinear horizontal grid of 150 × 100 cells (Figure 1) and a vertical grid of 20 levels. The horizontal grid cell size ranges from 7 km on the shelf near the open boundary, to nearly 1 km in the upper Bay. Atmospheric forcing is from the European Centre for Medium-Range Weather Forecasts Reanalysis v5 [Copernicus Climate Change Service (C3S), 2017]. For our 2009–2015 simulations, daily discharge is obtained from the Chesapeake Bay Program Watershed Model (Shenk and Linker, 2013; Easton et al., 2017); for 2015–2019 simulations, values are from United States Geological Survey gage data that have been scaled to the Chesapeake Bay Program Watershed Model discharge (Bever et al., 2021). The ocean boundary conditions for temperature and salinity are World Ocean Atlas monthly climatologies with a decadal trend applied (Hinson et al., 2021). Vertical mixing is parameterized using the generic length scale (Umlauf and Burchard, 2003) implementation of the k–ω turbulence closure (Warner et al., 2005) with a modified kmin, and lateral mixing is set to zero. ChesROMS is first run for 1 year (2009) for model spin up and then for 10 years (2010–2019) so the output can inform the particle tracking model. Hourly output files are used in order to resolve tidal effects. While tides appear to be of little direct importance to microplastics in the open ocean (Sterl et al., 2020), their effects in coastal waters have been found to be considerable (Zhang et al., 2020).
Particle Tracking Model
The particle tracking model is a modified version of Ichthyop v3.3.3, which was created to study ichthyoplankton dispersal (Lett et al., 2008). Ichthyop uses the currents, temperature, and salinity from a hydrodynamic model (here ROMS) to track plankton drifters (Pires et al., 2020), turtle carcasses (Santos et al., 2018), and for microplastics as well (Frère et al., 2017; Atwood et al., 2019; Collins and Hermes, 2019). Ichthyop allows the user to repeatedly add a constant number of particles at any location. Particles are then advected by the 3-D currents and have additional buoyancy-driven vertical velocity. This vertical velocity due to buoyancy (Figure 2), as used in Ichthyop and adapted from Parada et al. (2003), shows particle size impacts only vertical speed, while particle density impacts vertical velocity. Particles in Ichthyop also have additional horizontal movement to capture unresolved turbulence through a horizontal diffusivity that is a function of turbulent dissipation rate (the rate of dissipation for turbulent kinetic energy) and cell size (Peliz et al., 2007). Finally, an option within Ichthyop is to allow for permanent beaching, which depends on proximity to the coast and the unresolved horizontal turbulence (Barrier, 2020). Hence, the main choices to be made in running Ichthyop are particle size, particle density, turbulent dissipation rate, and whether to beach or not. Ichthyop does not include or use deposition to or resuspension from bottom sediments, remobilization of beached particles (for more than one time step), vertical motion due to unresolved turbulence (vertical dispersion), direct wind forcing on particles, advection from Stokes drift, and particle transformation (biofouling, degradation, fragmentation, and aggregation); these limitations are discussed in section “Model Limitations.”
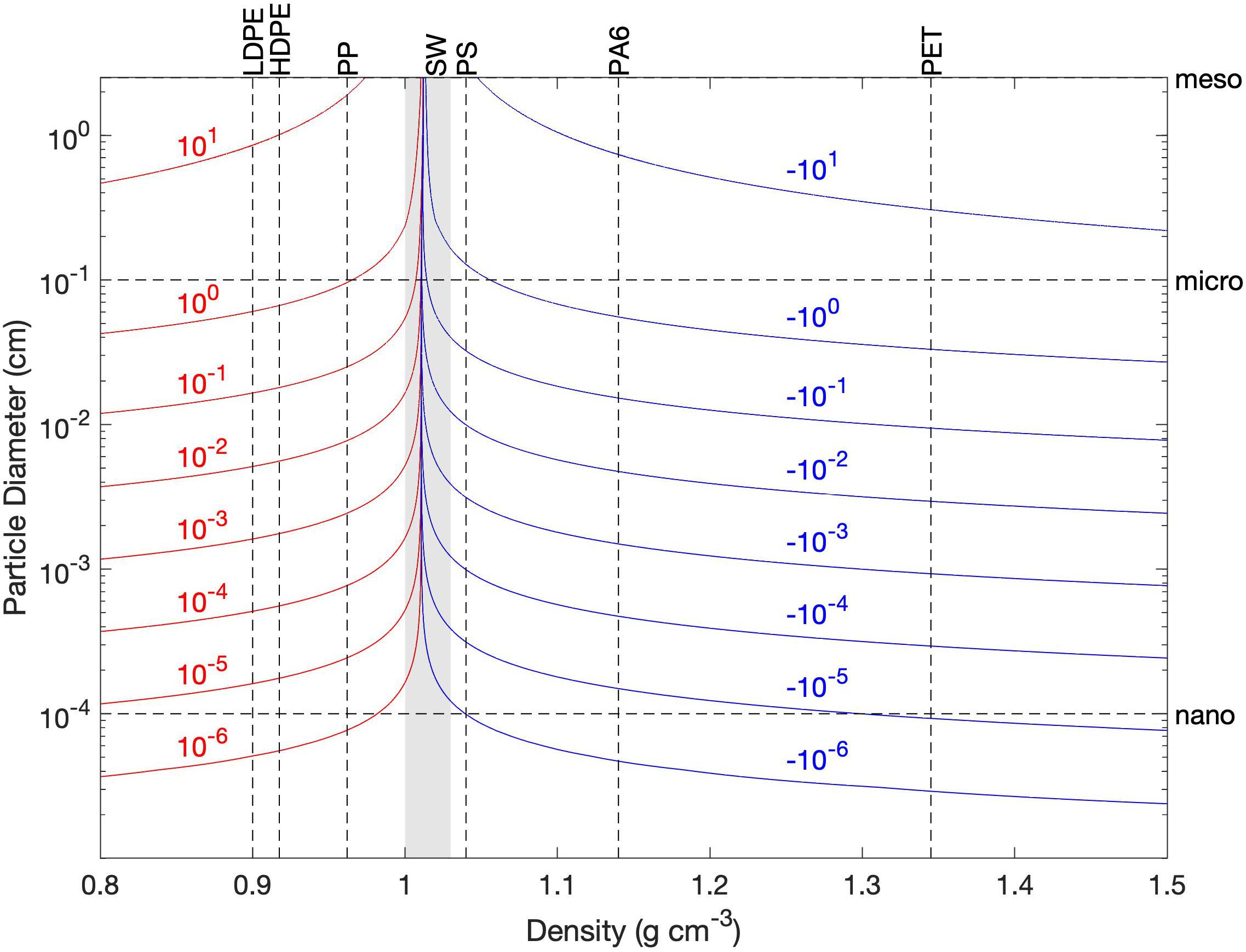
Figure 2. Vertical velocity (cm s–1) due to buoyancy as a function of spherical particle diameter and density, represented as contours (red for upward motion and blue for downward motion). Water density is calculated using 15°C and 15 PSU. Dashed lines indicate specific size and density values used in sensitivity testing; LDPE, HDPE, PP, PS, PA6, and PET refer to types of plastic encountered in the Chesapeake Bay (low-density polyethylene, high-density polyethylene, polypropylene, polystyrene, nylons, and polyethylene terephthalate, respectively). The shaded envelope about the line indicates the range of seawater (SW) density that occurs within the Chesapeake Bay based on a temperature range of 0–30°C and a salinity range of 0–30 PSU.
We made three main modifications to Ichthyop; first, we allowed the number of particles added at the 10 riverine input locations to vary daily. Note that we only add microplastics at these locations; we do not consider other sources including fishing gear, wastewater treatment plants below the head of tide, etc. We fixed the particle concentrations and assumed the number of particles added each day to vary linearly with streamflow. There is some evidence that microplastic concentrations are elevated during stormflow conditions (Moore et al., 2011; Watkins et al., 2019; Bailey et al., 2021), while concentrations during non-storm high flow conditions tend to have lower concentrations than non-storm low flow conditions, either due to dilution or reduced accumulation time relative to flow (Watkins et al., 2019; Bailey et al., 2021). However, with extremely limited concentration data for the riverine inflows, we opted for a constant concentration to facilitate understanding of transport and distribution. Each day, the number of particles added to a given river was set equal to the discharge in m3 s–1, which resulted in a concentration fixed at 1.157 × 10–5 m–3. Although this value is orders of magnitude less than stream observations report (Coles et al., 2020), using this value considerably reduces computation time and file storage requirements while still being large enough to capture the influence of each source on microplastic distribution throughout the whole Bay.
The second modification we made to Ichthyop was to allow for multiple coastline behaviors in a single simulation in order to replicate armored shorelines. By default, the beaching option in Ichthyop applies to the whole domain. We wanted to allow this behavior only where the coastline is not armored and we thus altered Ichthyop accordingly. Additionally, we created an Ichthyop-compatible file for armored shoreline location (Figure 1) using the Virginia Institute of Marine Science (VIMS) Shoreline Tidal and Marsh Shoreline Data (VIMS Comprehensive Coastal Inventory, 2018) and the ChesROMS grid. The database provides coastline segments and armored structure segments, which we then grouped by ChesROMS grid cell; as the segments may reach into more than one cell, the segments are grouped to the cell where the mean latitude and longitude of that segment is. These segments are then summed up by grid cell. Any coastline cells that did not have any segments grouped to them are then checked for segments ±1 cell in both horizontal directions and any found segments are attributed to the previously vacant cell, thereby accounting for observed shoreline data that do not perfectly coincide with the grid coastline. If the ratio between the sum of the lengths of armored shoreline structures and the sum of the lengths of shoreline is at least 50%, that cell is flagged as armored and will not allow any beaching. Application of this procedure results in 22% of the ChesROMS shoreline being armored, which agrees well with the estimate of 18% from Bilkovic et al. (2014) based solely on the VIMS database. While in reality particles may find their way onto armored shorelines or be resuspended after being deposited along unarmored shorelines, this model is our best approximation of microplastic particle behavior with the information currently available.
Ichthyop was modified in a third way by replacing the existing settling velocity equation for a prolate spheroid with that of a sphere. The settling velocity equation of Zhiyao et al. (2008) was chosen, which was found to be accurate in laboratory experiments conducted by Khatmullina and Isachenko (2017). Furthermore, we improved the estimation of kinematic viscosity from a constant value to one that depends on temperature, salinity, and dynamic viscosity (Sharqawy et al., 2010). Different shapes were not considered explicitly, though are addressed in the discussion.
Composite Simulation
For the composite simulation, choices needed to be made for the year, particle size (sphere diameter), particle density, and turbulent dissipation rate. The year 2010 was chosen for this simulation because it was typical in terms of its annual discharge (for the decade 2010–2019, 2010 was nearest to the median, and third nearest to the mean). Sphere diameter was set to 5 mm, which is within the size range of the limited observations in the Chesapeake Bay watershed (Coles et al., 2020). No observations were available for particle density within the Chesapeake Bay or its watershed, so we assumed equal amounts of floating and sinking particles, as suggested by observations in other systems (Vermeiren et al., 2016; Andrady, 2017). Specifically, we used the densities of common microplastics: polypropylene (PP, 0.90 g cm–3) and polyethylene terephthalate (PET, 1.345 g cm–3). Additionally, to help interpret the results, neutrally buoyant particles were included in the composite simulation. Over the entire composite simulation, 434,343 particles per density class were added to the Bay. Finally, the turbulent dissipation rate was set to 10–5 m2 s–3, which is within the range of 10–3 to 10–6 m2 s–3 found by Luznik and Flack (2010) in the Chesapeake Bay.
Sensitivity Testing
For our sensitivity test cases, we choose the York River to add microplastics to the Bay, which was the fifth largest contributor of fresh water to the Chesapeake Bay during 2010–2019 and, like most of the 10 rivers considered here, discharges along the Bay’s western boundary. To test sensitivity to interannual variability, we ran one decade-long (2010–2019) simulation that was then split by year for analysis. The default parameter that Ichthyop uses for turbulent dissipation rate is 10–9 m2 s–3. To include this value and the observed range from the Chesapeake Bay (Luznik and Flack, 2010), we conducted eight different simulations increasing the parameter by one order of magnitude from 10–3 to 10–10 m2 s–3 and a ninth reference simulation with 0 m2 s–3. Size and density parameters were adapted from the comprehensive analysis of common plastic types and debris metrics in Andrady (2017). Additional sizes beyond 5 mm that we tested were nano (0.001 mm), micro (1 mm), meso (25 mm), and macro (1000 mm), which are the upper bounds for each size classification from Andrady (2017). Additional densities tested beyond those of PP and PET were low-density polyethylene (LDPE; 0.9175 g cm–3), high-density polyethylene (HDPE; 0.962 g cm–3), polystyrene (PS; 1.04 g cm–3), and PA6 (nylons; 1.14 g cm–3), which are the mean values for most particle densities listed by Andrady (2017); common examples of all six plastic polymer types (e.g., rope, bottle caps, and netting for PP) can be found in Andrady (2011). Finally, to assess the sensitivity to shoreline interaction choices, we conducted one simulation with beaching everywhere and another with no beaching at all.
Results
Composite Simulation
The 1-year composite simulation (Figure 3) generates the horizontal distribution of microplastics particles in the water column and on the shoreline (see the full animated year in Supplementary Video 1). The fate of simulated particles includes three possibilities: remaining in the water column, beaching within the Bay, or being exported out of the Bay mouth to the continental shelf (Figure 4). The vast majority of all microplastics released, regardless of whether they were buoyant or not, end up being removed from the system by beaching along the Bay shoreline. Specifically, 92% of floating and 94% of sinking particles added are beached within the Bay after 1 year. Additionally, no sinking particles are exported out of the Bay and onto the continental shelf. As a result of their non-existent export from the Bay, sinking particles are typically twice as abundant as floating particles in the water column, despite their slightly greater tendency to beach (Figure 4A). Particles in the water column mainly reside near their respective source. The two largest rivers (Susquehanna and Potomac) have significant amounts of particles residing within and beaching along the mainstem of the Bay (56 and 11%, respectively), especially the eastern shore, and even up into other tributaries (e.g., the Chester). Within the tributaries, the majority of beached particles are the sinking particles that originated within that tributary; for example, 96% of floating microplastics and 100% of sinking microplastics released from the Rappahannock end up beaching along the tributary’s coasts before reaching the mainstem of the Chesapeake Bay. As shown for the whole Bay, beaching dominates the fate of particles for all 10 individual tributaries and none of the sinking particles ever make it out of the Bay. However, the Potomac and James Rivers have relatively large inventories of floating particles exiting the Bay, reflecting a combination of their high streamflow and proximity to the Bay mouth. In these rivers, there is a nearly equivalent reduction in the amount of buoyant particles beaching.
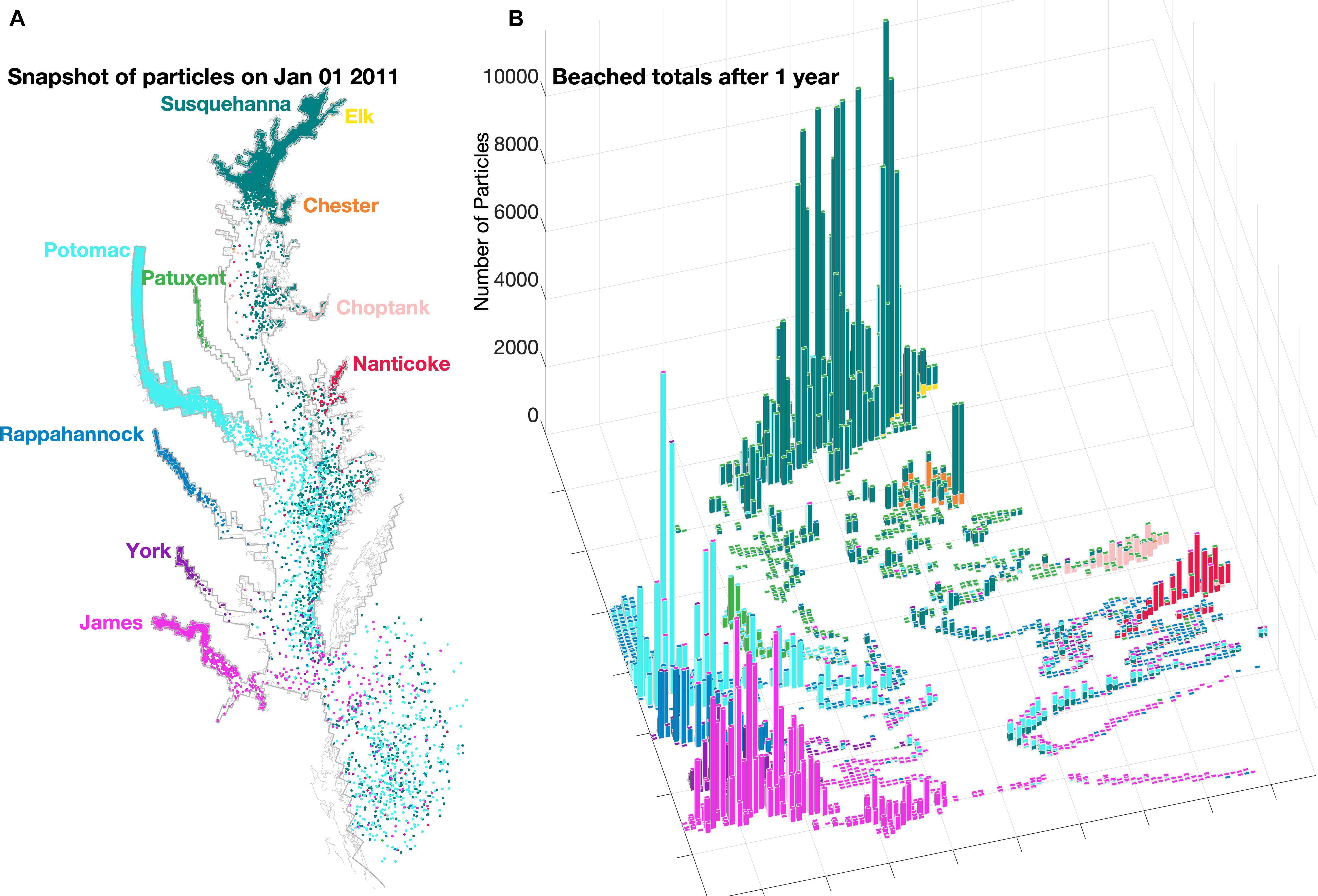
Figure 3. Simulated locations of sinking and floating particles (color-coded by river) after 1 year in (A) the water column and (B) on the shoreline (i.e., beached). An animation of the full simulation exists as Supplementary Video 1.
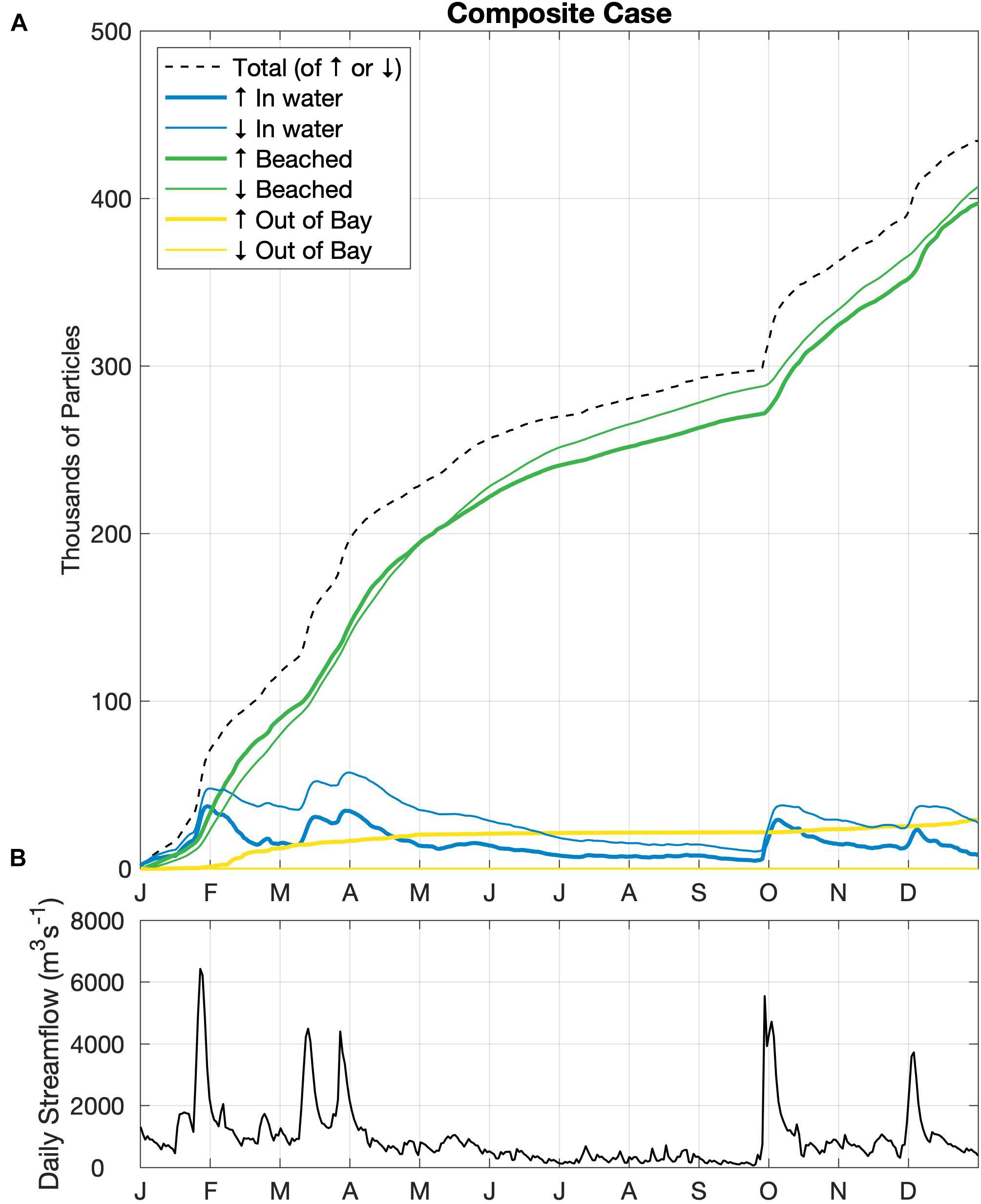
Figure 4. (A) Daily time series of the individual inventories of particles (in water inside the Bay beached inside the Bay, or out of Bay) and their total in the composite simulation for all rivers combined. Line thickness indicates whether the particles are floating (thick) or sinking (thin), and the black dashed line represents the sum of either all of the floating (↑) or sinking (↓) particles with time, as equal amounts of each were added throughout the simulation. (B) Daily time series of streamflow that enters the Chesapeake Bay in ChesROMS, obtained from Chesapeake Bay Program Watershed Model (Shenk and Linker, 2013; Easton et al., 2017); the cumulative sum of this plot is equivalent to the dashed line above, as 1 particle m–3 is added per 1 m3 s–1 of daily streamflow.
The strong seasonality seen in both floating and sinking particles in the water column, notably larger percentages from October through May compared to June through September, corresponds to streamflow. After each of the five prominent streamflow peaks throughout 2010 (Figure 4B), water column inventories increase, reflecting the increase in particle load from the rivers. These inventories then decline over the following weeks as particles are beached. The beached inventories always increase as a result of the one-way transport from water column to shoreline (as the model does not resuspend beached particles), but the rate of increase is always greatest following a streamflow peak. Most notable is the large peak in early fall, which occurred after a long period of low flow during the summer.
Individual trends between the different riverine source locations are shown in Figure 5. The impacts of the streamflow peaks for the whole Bay are apparent in all tributaries, with the peak in early fall being particularly notable, reflecting the fact that hydroclimatic variability at the intraseasonal time scale is fairly similar across the Mid-Atlantic region of the United States (Schulte et al., 2016, 2017).
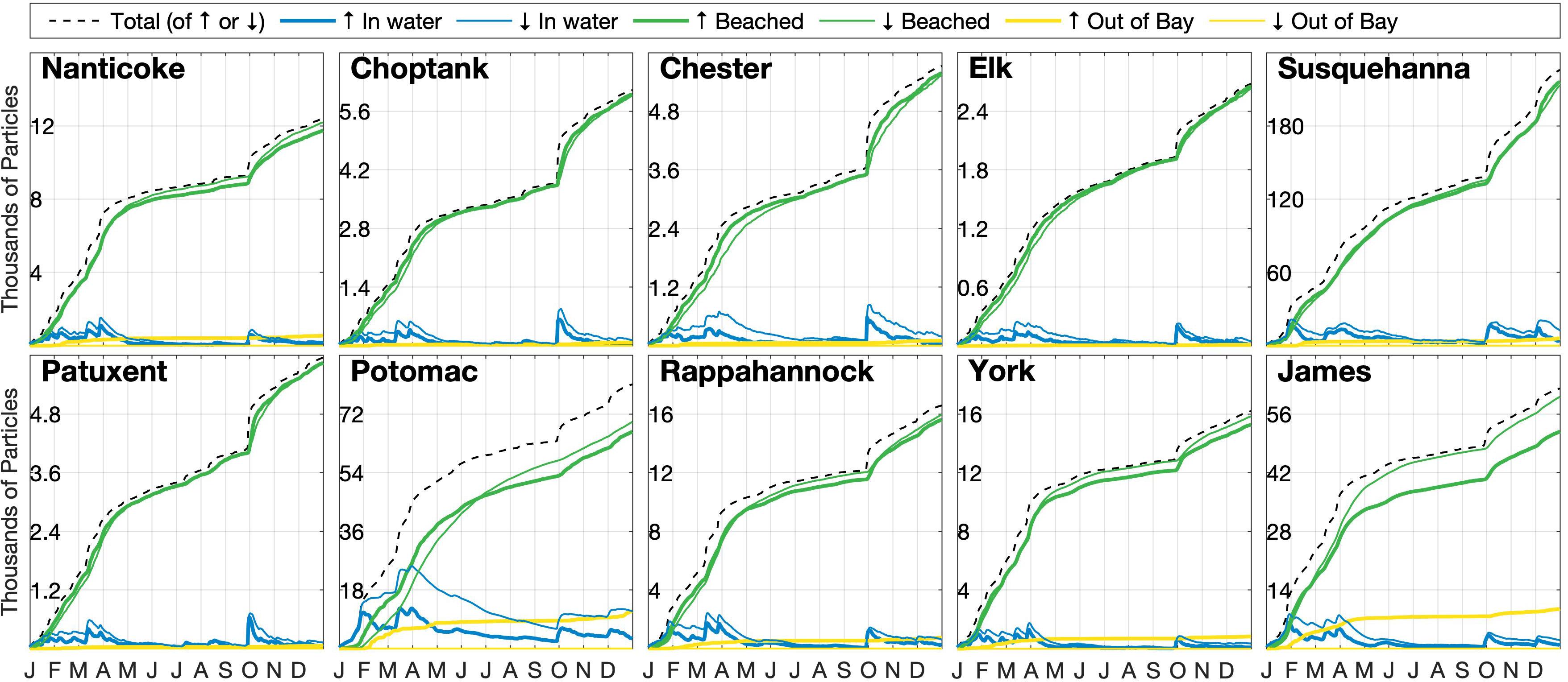
Figure 5. The composite simulation time series of the individual inventories of particles (in water inside the Bay beached inside the Bay, or out of Bay) and their total by their river source location. Line thickness indicates whether the particles are less dense than seawater (thick) or denser than seawater (thin). The black line represents the sum of either all of the floating (↑) or sinking (↓) particles with time, as equal amounts were added throughout the simulation. Note difference in vertical scales.
The length of time from when a simulated (floating or sinking) particle entered the model domain (via rivers) to when the particle was removed, either by exiting the Bay or by being deposited along the shoreline, is visualized with cumulative distribution functions (Figure 6). We can see Figure 6A that, as no sinking particles leave the Bay, the “All” curve aligns with the “floaters” curve. Half of all floaters that leave the Bay do so in 26 days. Figure 6B shows that sinking particles spend more time in the water column than floating particles do before eventually beaching, while neutrally buoyant particles nearly align with the mean timing of all floating and sinking particles. For example, half of particles beach within 7, 9, and 13 days for floaters, neutrals, and sinkers, respectively.
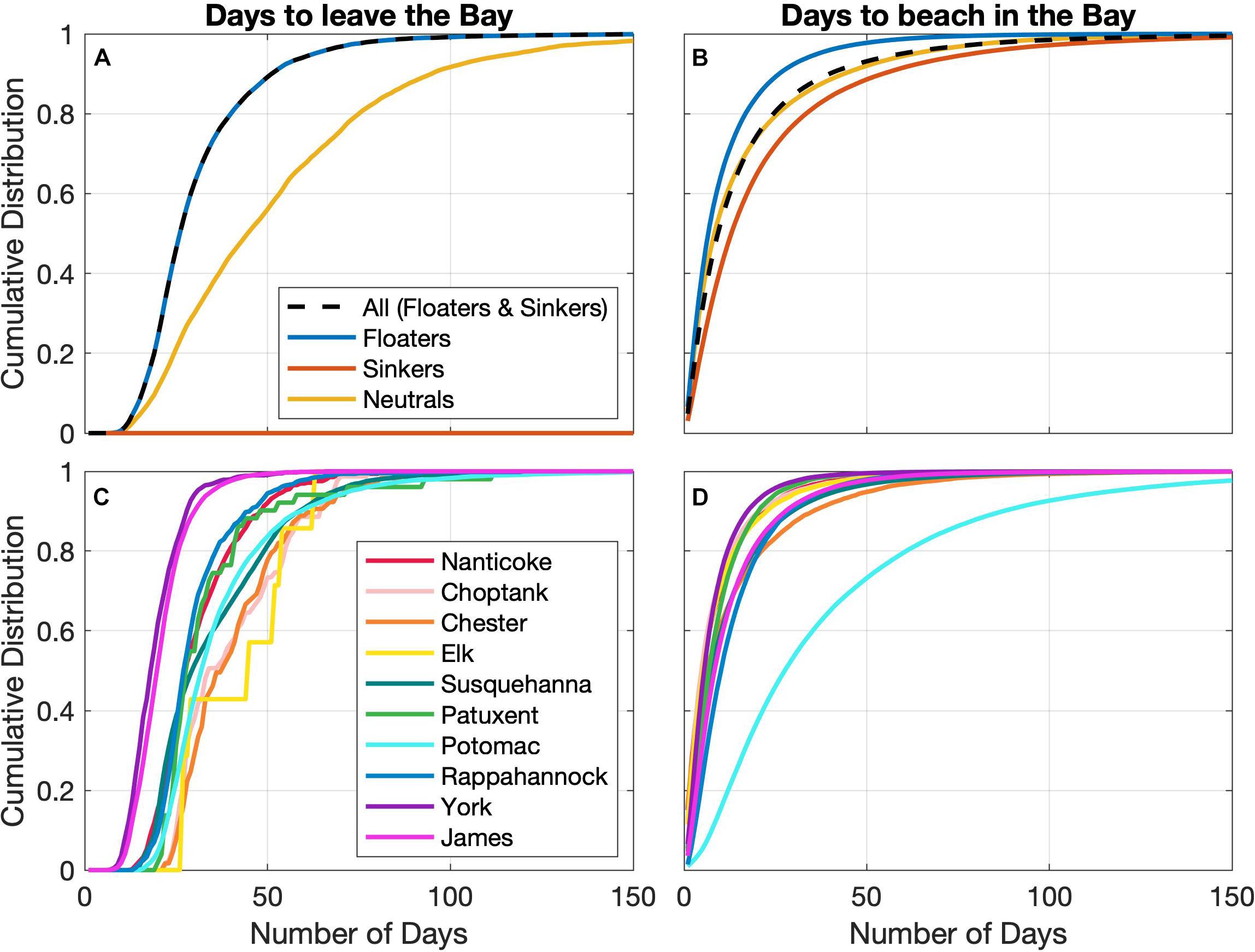
Figure 6. Cumulative distribution plots for the timings of particles to either (A,C) leave the Bay or (B,D) beach inside the Bay. (A,B) Are for all positively, negatively, and neutrally buoyant particles, as well as the mean of all floaters and sinkers. (C,D) Are the composite case mean (floaters and sinkers) for the individual source locations.
There is considerable variability among the 10 tributaries, with regards to how long it takes to exit the Bay, with half of the exiting particles leaving between 17 and 48 days (Figure 6C). The order from shortest to longest duration for half the particles to leave is similar to the order of tributary distance from the mouth of the Bay, with particles from the York and James Rivers exiting the Bay in the shortest amount of time and those from the Elk, Chester, and Choptank Rivers taking the longest. The jagged curve of the Elk River is due to the fact that so few of its particles make it out of the Bay. The residence times for the particles that are removed from the system via beaching are generally much shorter than those associated with exiting at the ocean boundary, with half the particles that beach doing so between 2 and 10 days, with one clear exception, the Potomac River, which is 27 days (Figure 6D). The distinct behavior of Potomac River particles might be attributed to the unrealistic shape of the Potomac tributary within the ChesROMS grid, which has a long straight channel that the particles must traverse before encountering more natural coastline profiles where beaching is likely (Figure 1). This artifact of the model grid may also explain the distribution of where the Potomac microplastics tend to beach; very few beach in the long straight channel, whereas many beach shortly after reaching the more realistic shoreline shape.
The vertical and horizontal distribution of simulated microplastics is dramatically influenced by particle density. Positively buoyant particles traverse the whole length of the Bay while remaining at the surface, while negatively buoyant particles are confined to the lower and upper portion of the Bay, near where the particles are injected (most come from the Susquehanna River, ∼350 km from the Bay mouth; Figure 7). The particles follow the branch of the residual circulation that they are embedded in (revealed by the salinity distribution): seaward at the surface for the positively buoyant particles and landward at the surface for the negatively buoyant particles. Neutral density particles are well-distributed throughout the water column and along the full length of the estuary. In this way the depth pattern for all particles follows that suggested by the thalweg transect shown in Figure 7A, with floaters confined to the surface, neutral particles well-distributed, and sinkers along the bottom. The sinkers’ peak at 2 m is due to 2 m being the minimum depth of the model and that most particles beach in the shallow tributaries, where the mean depth is 4 m, instead of ever reaching the greater depths of the mainstem.
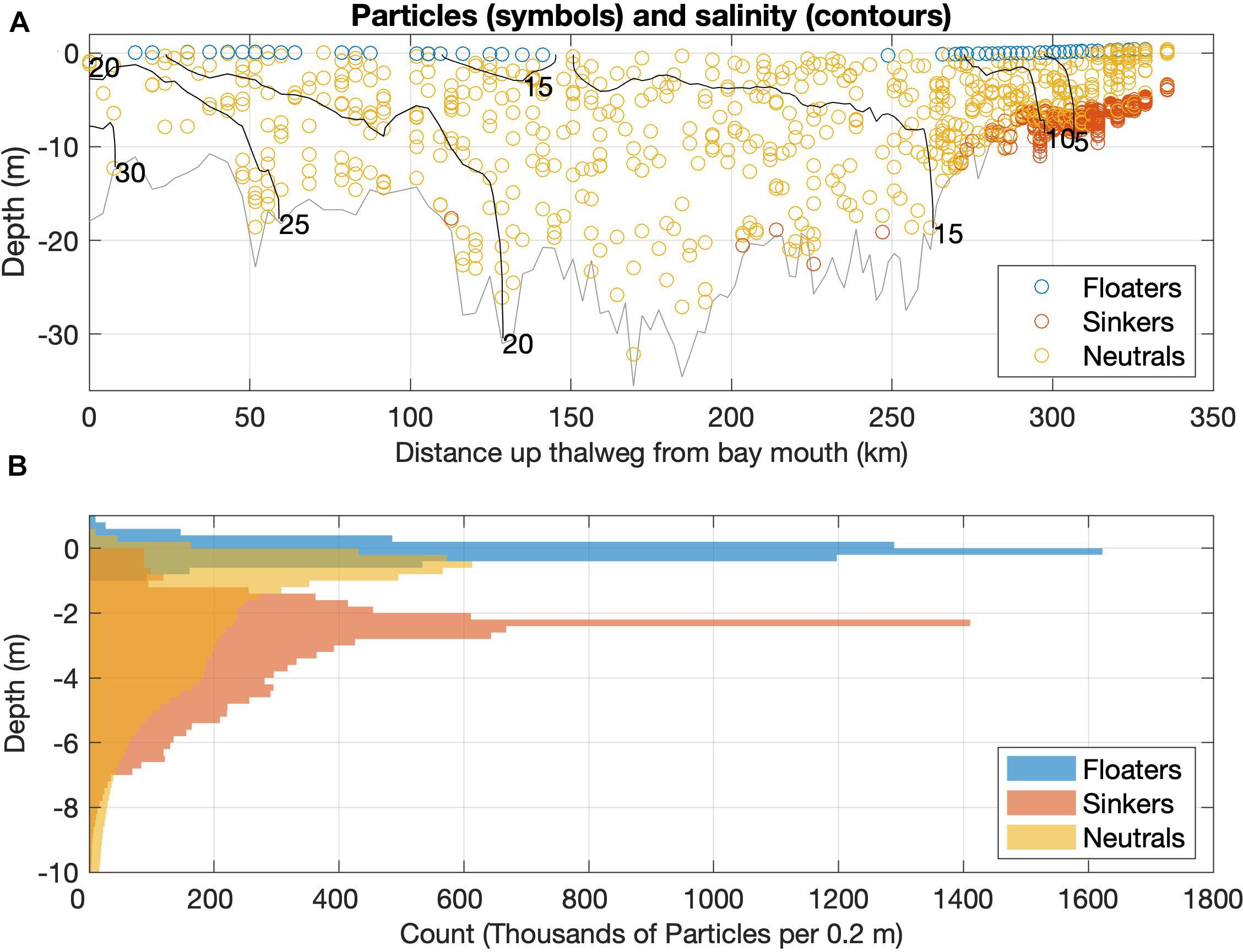
Figure 7. (A) Particle locations and salinity within a transect one grid point wide along the thalweg on the final day (366th day, January 1, 2011) of the composite simulation. (B) Particle abundance integrated over time and horizontal extent vs. depth over the course of the 1-year composite simulation for floaters, sinkers, and neutral-density particles. Each point on the plot is the cumulative sum over all days for particles within a depth interval of 0.2 m.
Sensitivity Tests
The simulated microplastic fate is highly sensitive to total freshwater discharge. This is most evident when comparing the wettest year simulated for the York River (2018), which had the second greatest fraction of particles exported out of the Bay (4.23%), to the driest year (2017) simulated, which had the second lowest fraction (2.36%); on a fractional basis, there were 79% more York River particles exported out of the Bay in 2018 than in 2017 (Figure 8). Water residence time in the Chesapeake Bay decreases with streamflow (Du and Shen, 2016), so higher-flow years should have a greater fraction of the particles that leave the Bay. Indeed, the fraction exported is moderately correlated with freshwater discharge (Pearson r2 = 0.338, p = 0.078, N = 10). In evaluating the entire decadal time series of daily values for the York River, the correlation between streamflow and particles leaving the Bay peaks at 7 days lag (12 days lag to the edge of the model domain).
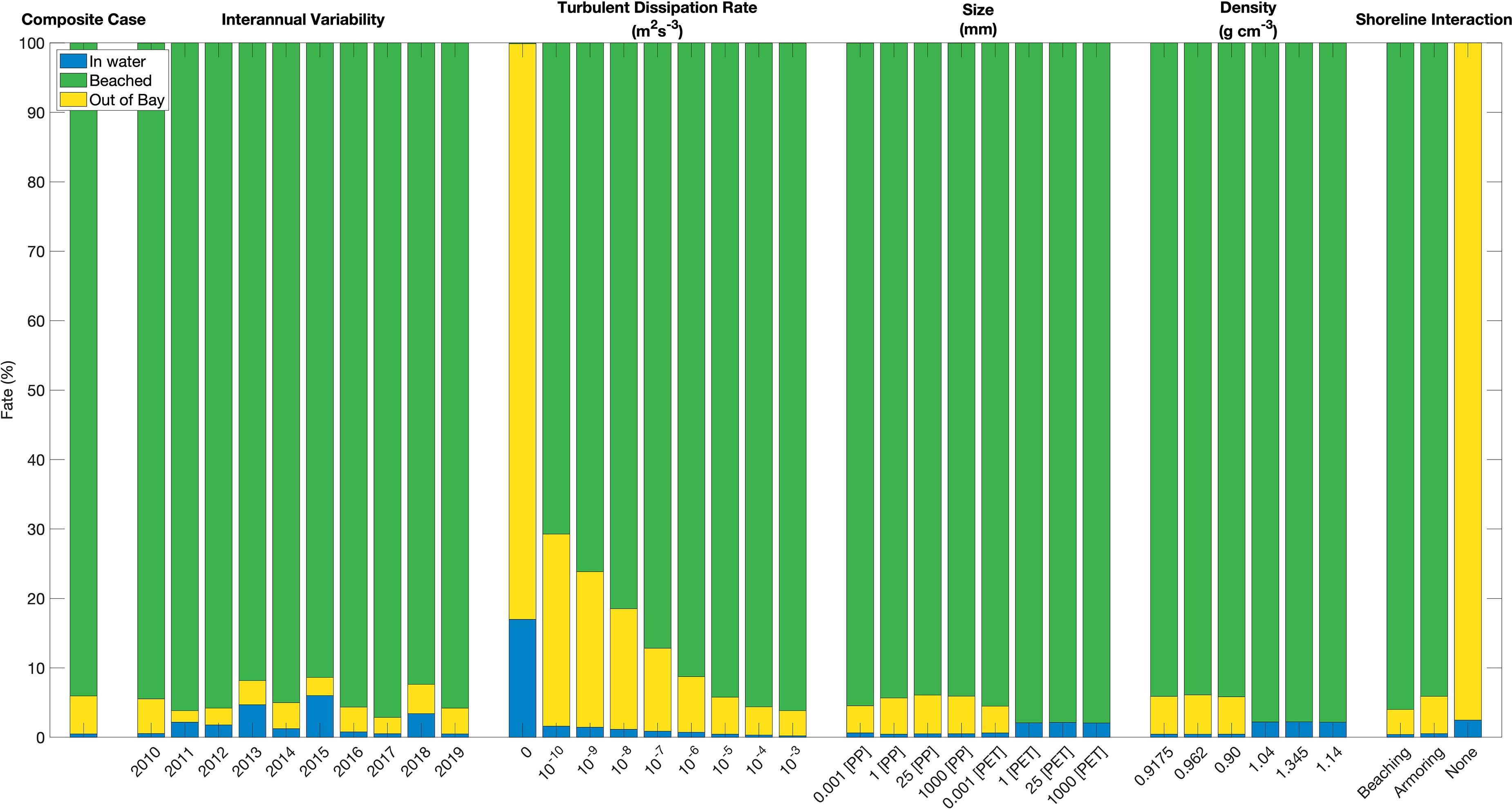
Figure 8. Sensitivity test cases for the York River, indicating final particle fate percentages. All cases are identical to the composite case for positively buoyant particles (year 2010, turbulent dissipation rate 10–5 m2 s–3, size 5 mm, density 0.90 g cm–3, and shoreline armoring) unless otherwise noted. Regarding Shoreline Interaction, Beaching and None are two options within unmodified Ichthyop that allow for particles to either beach everywhere along the entire shoreline or nowhere along the shoreline, respectively; Armoring is the modification test case that allows for particles to beach only along segments of coastline we specify within a variable in the ROMS grid file, the rest of the shoreline functioning as an armored shoreline.
The simulated microplastic fate is also sensitive to the turbulent dissipation rate, with the fraction of particles beached increasing monotonically from 70 for the lowest (non-zero) turbulent dissipation rate tested to 96% for the highest tested (Figure 8). As turbulent dissipation rate increases, lateral diffusivity increases. Due to the ability of particles to beach along the coastline and be removed from the water column, there exists a gradient perpendicular to the coastline in which particle density decreases toward the coast. Therefore, a higher diffusivity will result in a greater flux of particles toward the coastline and result in a greater percentage of particles beaching. Virtually no particles are beached with zero turbulent dissipation rate, which dramatically illustrates the importance of unresolved turbulence in the model beaching parameterization.
Particle size had little influence on the overall transport and fate of simulated plastics (Figure 8). Most of the floating particle size classes behaved similarly, and most of the sinking particle size classes behaved similarly; e.g., the fraction beached varied within a few tenths of a percent for a given particle density. The only outlier is with the nanoplastic size (1 μm), which had export out of the Bay regardless of density. Additional testing showed this tendency to persist through 10 μm size, however, a test of 100 μm showed consistency with sizes 1 mm and greater. These findings coincide with Figure 2’s vertical velocity by particle size, with positive and negative vertical velocity evident for sizes above 100 μm. For the purposes of this study, simulating microplastics at the upper bound of their size range (5 mm), this slight size sensitivity not significant, but we acknowledge the impact it would have on nanoplastic simulations, as well as smaller microplastics. In general, this lack of sensitivity is due to the timescales of sinking and rising compared to the simulated timescales for beaching or leaving the Bay; at 1 cm s–1 it would take less than an hour to travel a water column of 10 m (typical for the Chesapeake Bay) while beaching or leaving the Bay takes days to weeks (Figure 6).
In contrast to particle size, particle density had a profound influence on the microplastic fate (Figure 8). All particles with densities less than the water behaved very similarly with respect to particle fate with 5% of particles exiting the Bay, and 93% of particles beaching within the Bay (Figure 8). A similar agreement was seen amongst the three cases with densities greater than the water, with 0% of particles exiting the Bay. However, the PET and PA6 cases are more similar to one another than to the PS case, the latter having a density only slightly greater than Bay water. A particle’s distribution and residence time is sensitive to whether its density is greater or less than that of the surrounding water, but not meaningfully affected by how much greater or less than its density is to that of water.
The inclusion of shoreline armoring compared to the default unarmored condition reduces the overall percentage of beached particles and increases the number of particles that leave the Bay (Figure 9). It is notable that the reduction is not equivalent to the percentage of shoreline that has been armored, which means that even if particles are no longer able to be beached where they would originally, many are still beached along the available non-armored shorelines instead of exiting the Chesapeake Bay. If beaching is excluded entirely, the only possible fate for particles is exiting the Bay and 97% of the particles do so; additionally, particles can no longer beach close to their sources, resulting in a longer period of time spent in the Bay waters.
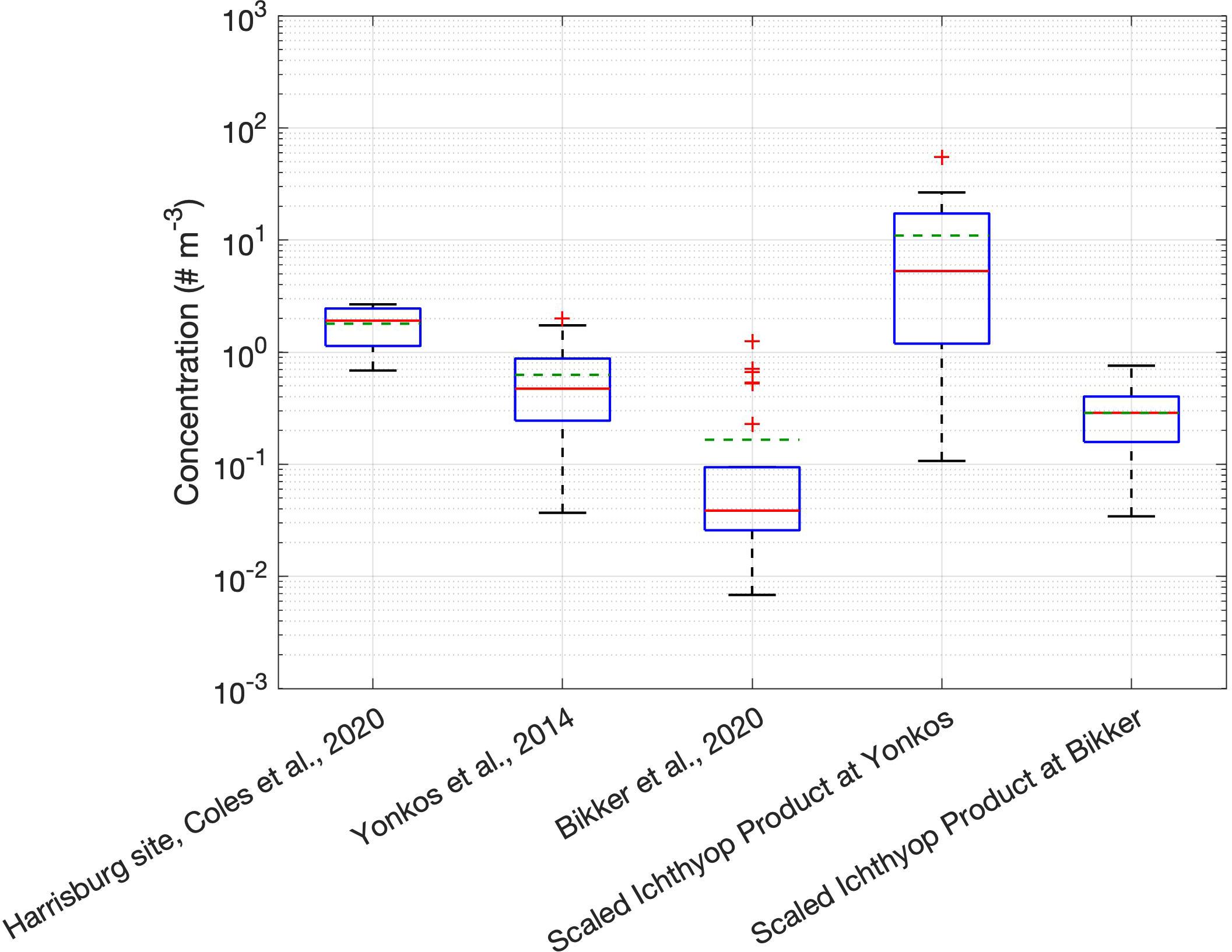
Figure 9. Box plots of concentrations from three observational studies, as well as scaled model concentrations from the same bay locations used in Yonkos et al. (2014) and Bikker et al. (2020). Green dashed lines denote the mean, while red denotes the median. Scaling was done by taking the 7-day model mean centered on the date of observation, then multiplying the model concentration for each observation by the ratio between the median of the observed concentration at the Harrisburg USGS station from the Coles et al. (2020) dataset and the concentration of particles (1.157 × 10–5 m–3) added to the particle tracking model. Note there is no Scaled Ichthyop Product for the Coles et al. (2020) dataset, as the observation sites are either outside of the model domain or within the model mask.
Discussion
We combined hydrodynamic and particle tracking models to investigate the fate of microplastic particles entering the Chesapeake Bay from its 10 primary tributaries. Returning to our motivating questions, the model results indicated that (1) microplastics mainly reside in the water column and shoreline near their riverine source, though particle abundances are also high along the eastern shore of the Bay, considering that equal particle concentrations for the rivers were applied; (2) the overwhelming majority of microplastics are beached (94%) and only a small fraction are exported from the Bay to the coastal ocean (5%); (3) beaching and export have fairly short timescales (weeks) compared to the residence time of water in the Bay (months); (4) seasonal and interannual variations of particle fate are driven by streamflow; and (5) particle density is the main factor affecting simulated particle fate, with other model parameters, such as particle size, turbulent dissipation rate (which affects horizontal dispersion and thus beaching), and shoreline armoring playing much smaller roles. The high level of beaching seen in the model results supports our hypothesis that estuaries act as a filter of riverine microplastics. To contextualize these findings, we discuss them in terms of the limitations of the model, the limited observations available in the Chesapeake Bay, the implications for estuarine sampling of microplastics, and the plastics budget of the ocean.
Model Limitations
In this section, we consider in detail model limitations related to beaching, benthic deposition, transport, riverine input, resolution, particle transformation, and particle shape. The main conclusion is that addressing these limitations will likely alter simulated microplastics distributions in the Chesapeake Bay, but not the overall finding that this estuary is trap for riverine microplastics. Our study is silent on the fate of non-riverine microplastics, such as shipping, fishing gear, and wastewater treatment plants on the estuarine shoreline.
The high level of beaching in the composite simulation (Figure 4) and the sensitivity of model results to the three shoreline interaction options (Figure 8) suggest that the lack of beached particle remobilization (Liro et al., 2020) in the model is one of the most important limitations associated with particle transport. Indeed, Jalón-Rojas et al. (2019) found their model results to be sensitive to the main remobilization parameter (there referred to as “washing-off”), which is the particle half-life on land. Inclusion of beached particle remobilization in our model would increase the export of particles out of the Bay. However, the inclusion of this remobilization still results in extensive beaching in coastal models (Liubartseva et al., 2018; Jalón-Rojas et al., 2019), so it is unclear how significant this process is in the Chesapeake Bay.
Not allowing microplastics deposition to and resuspension from the Bay bottom may be a greater transport-related limitation. Microplastics have limited photodegradation at depth and are believed to continually accrue in the sediments with partial resuspension (Andrady, 2017). Incorporation of deposition would, however, only increase support of our hypothesis of estuaries as traps for riverine microplastics. However, the inclusion of this process could also serve to inform monitoring efforts in bottom sediments, which would help constrain any efforts of a microplastics budget.
The lack of vertical dispersion in the particle tracking model is probably not an important limitation because particle buoyancy dominates vertical movement in the Bay. Supporting this contention is the insensitivity of microplastic distribution to vertical dispersion in a global model (Wichmann et al., 2019). Direct wind forcing on particles is also likely unimportant for microplastics, as suggested in the sensitivity analysis of Jalón-Rojas et al. (2019), with the exception of floating objects not simulated in this study. Stokes drift has been found to be important in some microplastics coastal modeling studies (Isobe et al., 2014; Iwasaki et al., 2017; Liubartseva et al., 2018) and in circulation modeling of the Chesapeake Bay (Dortch et al., 1992). However, the generally high quality of simulations of salinity in the Bay without Stokes drift (Da et al., 2018) suggests that it is not a first-order process and hence its inclusion likely should not alter our main findings.
We limit the model to use the same particle concentration relative to discharge for all rivers for all time, though we acknowledge that, in reality, various factors result in variable particle concentrations both across time and between rivers. The temporal constancy of particle concentration may favor particle retention in the Bay if, as suggested by some studies (Moore et al., 2011; Watkins et al., 2019; Bailey et al., 2021), riverine concentration increases with stormflow. These high-flow events result in shorter estuarine residence times and hence particles would be preferentially exported during such events, as has been seen for macroplastics in other estuaries like the Seine (Tramoy et al., 2020). Similarly, we assume all rivers have the same particle concentration, but nearby watershed population density is known to impact particle concentrations (Yonkos et al., 2014; Bikker et al., 2020). In the empirical study of Schmidt et al. (2017), six Chesapeake Bay rivers were modeled. Scaling our riverine fluxes to be consistent with those of this study while keeping the other rivers unchanged would shift particles in the water column and shoreline toward those tributaries with catchment population densities that are relatively high, such as the Patuxent and Potomac River Estuaries, at the expense of tributaries with low catchment populations densities, such as the Rappahannock and York River Estuaries. However, such scaling only slightly increases the fraction of floating particles exported from the Bay from 12.66 to 13.86% (all sinkers are still retained within the Bay), mainly because the Potomac River is highly populated and is an effective exporter (Figure 5).
Another limitation related to riverine input is the assumption of equal fractions of sinking and floating particles, which is based on a global industrial production ratio and not actual riverine data for the Chesapeake Bay watershed. Figure 7A highlights the dramatic differences between distributions of floating and sinking particles, so any change in the floating:sinking ratio would have profound impacts on distributions. The limited data available in freshwater environments suggests a greater preponderance of floating particles, though samples are likely biased in that they are usually limited to surface waters (Schwarz et al., 2019). Regardless, while sinking particles are trapped more effectively than floating ones, both types are strongly trapped, leaving our main conclusion unaltered. The model grid may be missing finer-scale detail in the small shoreline features not simulated. While we consider finer grid resolution would only facilitate greater particle beaching and less export out of the Bay, we acknowledge we cannot speak to sampling effort guidance in the subgrid-scale coastline features.
Given that model results are sensitive to particle density but not size, probably the most serious model limitation regarding particle transformation is biofouling, which is the attachment and growth of plankton and other small organisms to a plastic particle. Biofouling increases the average density of the particle and has been modeled by specifying a film thickness, density, and growth rate (Jalón-Rojas et al., 2019); polymer type also affects susceptibility to biofouling (Zhang et al., 2021). Jalón-Rojas et al. (2019) found their model results to be sensitive to biofouling parameters. Also, Besseling et al. (2017) found size to be significant for nano- and microplastic distribution, which may be due to their inclusion of biofouling (and perhaps aggregation). The inclusion of a biofouling parameterization and the subsequent increase in particle density would have led to increases in particle abundance in the water column and along the shoreline near rivers. In addition, beaching would have increased and fewer particles would have been exported out of the Bay, strengthening our main conclusion that the Bay traps riverine microplastics. Finally, aggregation, both physical and biological (e.g., via ingestion) could affect particle density, but we are unaware of parameterizations of this process in estuarine and coastal environments.
One of the key benefits of plastic in general is its durability and longevity, so to the extent that degradation does affect particle buoyancy, the timescales we have found for fate within the estuary (on the order of weeks) are short enough that known plastic polymer degradation rates (Chamas et al., 2020) and photodegradation timescales (months, Zhang et al., 2021) will not impact distribution throughout the Bay. The chemical degradation of plastics is influenced by light, temperature, oxygen, organisms, and polymer type (Zhang et al., 2021). Degradation mainly affects particle size and so it is not surprising that Jalón-Rojas et al. (2019) found a low sensitivity of their model results to this process. Fragmentation often follows degradation, which makes plastic particles more brittle; given the lack of impact of fragmentation on density and the long time scales for degradation, it seems unlikely that including fragmentation would meaningfully affect our results.
Numerous studies have shown that particle shape profoundly influences microplastic settling velocity (e.g., Khatmullina and Isachenko, 2017), which might suggest that our study, which is limited to spheres, is incomplete. However, our sensitivity studies with sphere diameter, from the nanoscale (0.001 mm) to the macroscale (1000 mm), encompass an enormous range in settling velocities. For example, at a temperature of 15°C and a salinity of 15, PET spheres settle at speeds ranging from 1 × 10–4 to 2 × 103 mm s–1 for the above diameters. Any deviations from shape from a 5 mm diameter sphere would be well within this large range of settling velocities. For example, using the parameterization of Khatmullina and Isachenko (2017), it can be shown that a very thin PET rod 5 mm in length and 0.1 mm in diameter settles at a speed roughly the same as that of a 0.3 mm diameter sphere. The same parameterization shows that a cylinder with both diameter and length of 5 mm settles at the same speed as a 40 mm diameter sphere.
Comparison With Observations
A detailed model–data skill comparison (Stow et al., 2009) is not possible given the limited observations of microplastics in the Chesapeake Bay. To compare with the few observations in the estuary that do exist, we conducted additional simulations for 2011 and 2015, which correspond to the years those observations were made. We then scaled the resulting model concentrations of the uppermost meter by the ratio of the median of the Harrisburg site observations of water surface samples from Coles et al. (2020), chosen as the Susquehanna River accounts for nearly half of the freshwater inflow to the Bay and passes through Harrisburg, to the concentration of particles in the model rivers (1.157 × 10–5 m–3); this scaling reframes the model concentrations as concentrations in line with the observations. Note that on a given day, model concentration may be zero particles m–3 due to the lower-than-observed loadings, so we use a weeklong average model concentration centered on the date of observation for comparisons (Figure 8). The model does an excellent job at capturing the factor of 10 decline from the tributaries [here meaning the Yonkos et al. (2014) dataset] median to the mainstem [Bikker et al. (2020) dataset] median. The model also does a reasonably good job at capturing the variability of the observations as given by the interquartile range, though it is higher than observed in the tributaries and lower than observed in the mainstem. The pattern (Figure 9) seen in the observations of decreasing concentration moving from the watershed [Harrisburg site from Coles et al. (2020) dataset] to the tributaries cannot be captured by the model, as the model domain does not reach up into the watershed. However, the model median in the tributaries (“Scaled Ichthyop Product at Yonkos”) is higher than the median concentration in the rivers [“Harrisburg site, Coles et al. (2020) dataset”] by about a factor of 2. This puzzling result can be explained by the concentrating effect of to the particles’ positive buoyancy, resulting in the particles rising to and clustering at the surface, as seen in Figure 7. The fact that this effect is not seen in the observations may lie in one or more of the following: (a) the different seasons and years of observation of the watershed and tributaries measurements, (b) an overestimated ratio of floaters to sinkers, or (c) the lack of deposition to the sediments. The latter two would lead to an overestimate of concentrations in the model, which, if remedied, would only strengthen the overall conclusion that the Chesapeake Bay is an effective trap of riverine microplastics. Summarizing, the comparison of the model with the limited observations available suggests some model strengths and some weaknesses, with remedies for the latter only likely to strengthen our conclusions.
Implications for Observing Strategies
The results of this study have implications for observational sampling studies not only in the Chesapeake Bay but in other estuaries as well. Our finding that particle size is much less important than particle density when it comes to dispersal is significant for shoreline sampling efforts as it means that wherever visible macroplastics exist, micro- and nanoplastics will exist as well, though the correlation may be weakened by direct wind forcing on macroplastics. Nevertheless, because it is much easier to detect macroplastics than it is microplastics in the water column and on the shoreline, field surveys that aim to detect microplastics hotspots can be guided by the visual cues of macroplastics and the databases that result from them. For example, the Marine Debris Monitoring and Assessment Project of the US National Oceanic and Atmospheric Administration has created a database through the engagement of citizens in shoreline surveys.
In our simulations, the vast majority of riverine microplastics entering the Bay tend to beach and do so within their tributaries before reaching the mainstem of the Bay; thus greater attention should be paid to tributary coastlines when planning sampling efforts, similar to the model findings Schernewski et al. (2020) of high accumulation rates along Baltic Sea shorelines near source locations. Another potential hotspot of accumulation appears to be the eastern shore of the Bay, which is supported by earlier particle tracking simulations and observations of phytoplankton, zooplankton, and Bay anchovy eggs and larvae that show accumulation is due to low flushing rates and convergence (Hood et al., 1999).
The particles that do exit the Bay are entirely comprised of buoyant particles and exit the Bay faster than the reported residence time of the Bay waters. We find it takes roughly 100 days for 95% of all surface particles that leave the Bay to do so (Figure 6). Residence time studies for the surface waters of the Bay entering from the Susquehanna are twice that duration or longer, while surface waters entering the Bay closer to the mouth are half that duration (Du and Shen, 2016). A similar relation of buoyant microplastic particles having smaller residence times than neutrally buoyant particles was found in a modeling study in the Great Lakes (Cable et al., 2017). Analogously, buoyant microplastic particles were modeled to exit the San Francisco Bay in significantly higher percentages than neutrally buoyant particles (Sutton et al., 2019). These buoyant particles moving along the surface are not within the Bay for as long as Bay residence times, and this is an important consideration for those who might use the latter value to estimate microplastic exposure duration to biota.
Other significant findings for sampling considerations include the strong seasonality of particle count within the water column (Figures 4, 5), as the same point in space will have drastically different microplastic concentrations depending on the time of year. Additionally, the density-driven water column distribution (Figure 7) has implications for sampling studies that often rely on surface trawls to collect observations. Surface sampling may preferentially select for neutral to buoyant particles, especially in the mainstem of the Bay, farthest from potential microplastic sourcing to the Bay.
Implications for the Oceanic Plastics Budget
This study answers in part the question of the missing sink for plastics in the ocean (Law and Thompson, 2014). The difference between the reported head of tide plastic concentration vs. that in the open ocean can largely be attributed to substantial beaching along estuarine shorelines. We find that over 90% of riverine microplastics entering the Chesapeake Bay do not exit the Bay; thus, the estuary serves as a filter for microplastics before the waters reach the open ocean waters. Whether the fate patterns seen in the Chesapeake Bay are unique to this estuary, or if the Chesapeake Bay is typical of all estuaries in this regard, is uncertain. However, the Chesapeake Bay does have a mean residence time of 180 days (Du and Shen, 2016), significantly higher than the ∼70 day mean for estuaries of the contiguous United States, as calculated from the Supplementary material of Hutchings et al. (2020). A lower residence time would coincide with faster export of buoyant microplastics out of the estuary, potentially meaning less microplastics are trapped inside other estuaries along the shoreline. Another factor influencing microplastic retention in estuaries may be the total beachable shoreline length; the meandering shorelines of the upper tributaries of the Bay had the greatest shoreline deposition. Estuaries with shorter coastlines with less intricacies may not trap as many particles along their coast. Vegetated shorelines have also been shown to increase macroplastic retention (Tramoy et al., 2020).
If estuaries are effective traps of riverine microplastics, as we suggest here, then global budgets for the open ocean should be modified to account for this trapping. A combination of shoreline plastics abundance data, information on the characteristics of estuaries worldwide (e.g., Dürr et al., 2011), and modeling (both process-based and empirical) would be the likely elements needed to quantify estuarine microplastic trapping on a global scale and revise ocean budgets accordingly.
Conclusion
In our simulations, the Chesapeake Bay traps over 90% of 5 mm microplastic particles released into the estuary via major rivers. These particles in the Bay are trapped via beaching, the inclusion of which significantly alters the fate of modeled microplastic particles. Only positively buoyant particles leave the Bay, and the majority do so within 26 days, although high streamflow years correlate with greater particle export out of the Bay mouth. Half of particles that beach do so within 7 days for floaters and 13 days for sinkers, meaning negatively buoyant particles spend longer in the water column before beaching. Particle size is not significant to overall distribution of particles in the Bay. Particle density, namely whether density is greater or less than seawater, is significant to overall distribution.
Our overall findings are robust to a wide range of parameter choices. The greatest model uncertainties are the beaching parameterization to include remobilization, and the lack of deposition to the sediments, both of which could be improved through a combination of process-based studies and whole-estuary budget studies. The latter would need to combine comprehensive observations of microplastic abundance at the head of tide and in the water column, shorelines, and bottom sediments of the estuary. Such an observational program could be guided by more readily conducted macroplastics surveys, the expectation of extensive beaching in the upper estuary, and temporal variability driven by streamflow.
Estuaries have long been characterized as filters of land-derived materials, such as nutrients (McGlathery et al., 2007; Asmala et al., 2017), carbon (Najjar et al., 2018; Hutchings et al., 2020), and sediments (Burchard et al., 2018). Here we suggest that this filtering capability extends to plastic debris, particularly microplastics, which are expected to increase in abundance at an exponential rate (Geyer et al., 2017). If estuaries are indeed a large sink of riverine microplastics, then it can be expected that much of the impact of marine plastic pollution can be found in estuaries, which are among the most productive waters in the world. Our study suggests that an increased research focus is needed on estuarine microplastics and that sampling should focus on shorelines in proximity to sources.
Data Availability Statement
ChesROMS-ECB uses the ROMS source code (ROMS version 3.6, SVN revision 898), which is freely accessible at the ROMS website (https://www.myroms.org) (The ROMS/TOMS Group, 2021) and is open-source licensed by the MIT/X license. Ichthyop v3.3.3 is freely accessible at the Ichthyop website (https://www.ichthyop.org) (Pverley et al., 2020) and is open-source licensed by the GNU General Public License v3.0. Modified configuration files of the Ichthyop v3.3.3 code used in this study, as well as the model output for the Configuration Case and Sensitivity Tests, are archived at The Pennsylvania State University’s institutional repository, ScholarSphere (https://doi.org/10.26207/m26x-q972).
Author Contributions
RN, MH, and DW conceptualized the project. AL conducted all model simulations, and interpreted model results with guidance by RN, MF, MH, and DW. All authors contributed to the article and approved the submitted version.
Funding
This research was funded by the College of Earth and Mineral Sciences, The Pennsylvania State University, through the Dean’s Fund for Postdoc-Facilitated Innovation through Collaboration. This manuscript is additionally the result of research funded by the National Oceanic and Atmospheric Administration’s National Centers for Coastal Ocean Science under award NA16NOS4780207 to the Virginia Institute of Marine Science.
Conflict of Interest
The authors declare that the research was conducted in the absence of any commercial or financial relationships that could be construed as a potential conflict of interest.
Publisher’s Note
All claims expressed in this article are solely those of the authors and do not necessarily represent those of their affiliated organizations, or those of the publisher, the editors and the reviewers. Any product that may be evaluated in this article, or claim that may be made by its manufacturer, is not guaranteed or endorsed by the publisher.
Acknowledgments
We appreciate the assistance of Pierre St-Laurent in the hydrodynamic model setup and Michael López in the particle tracking model setup. Discussions with Maria Herrmann and Jill Arriola helped to improve the study. We thank the three reviewers for providing helpful comments.
Supplementary Material
The Supplementary Material for this article can be found online at: https://www.frontiersin.org/articles/10.3389/fmars.2021.715924/full#supplementary-material
References
Andrady, A. L. (2011). Microplastics in the marine environment. Mar. Pollut. Bull. 62, 1596–1605. doi: 10.1016/j.marpolbul.2011.05.030
Andrady, A. L. (2017). The plastic in microplastics: a review. Mar. Pollut. Bull. 119, 12–22. doi: 10.1016/j.marpolbul.2017.01.082
Asmala, E., Carstensen, J., Conley, D. J., Slomp, C. P., Stadmark, J., and Voss, M. (2017). Efficiency of the coastal filter: nitrogen and phosphorus removal in the Baltic Sea. Limnol. Oceanogr. 62, S222–S238.
Atwood, E. C., Falcieri, F. M., Piehl, S., Bochow, M., Matthies, M., Franke, J., et al. (2019). Coastal accumulation of microplastic particles emitted from the Po River, Northern Italy: comparing remote sensing and hydrodynamic modelling with in situ sample collections. Mar. Pollut. Bull. 138, 561–574. doi: 10.1016/j.marpolbul.2018.11.045
Bailey, K., Sipps, K., Saba, G. K., Arbuckle-Keil, G., Chant, R. J., and Fahrenfeld, N. L. (2021). Quantification and composition of microplastics in the Raritan Hudson Estuary: comparison to pathways of entry and implications for fate. Chemosphere 272:129886. doi: 10.1016/j.chemosphere.2021.129886
Barrier, N. (2020). Ichthyop Documentation [Online]. Available online at: http://www.ichthyop.org/index.php/documentation/ (accessed February 1, 2021).
Besseling, E., Quik, J. T. K., Sun, M., and Koelmans, A. A. (2017). Fate of nano- and microplastic in freshwater systems: a modeling study. Environ. Pollut. 220, 540–548. doi: 10.1016/j.envpol.2016.10.001
Bever, A. J., Friedrichs, M. A. M., and St-Laurent, P. (2021). Real-time environmental forecasts of the Chesapeake Bay: model setup, improvements, and online visualization. Environ. Modell. Softw. 140:105036. doi: 10.1016/j.envsoft.2021.105036
Bikker, J., Lawson, J., Wilson, S., and Rochman, C. M. (2020). Microplastics and other anthropogenic particles in the surface waters of the Chesapeake Bay. Mar. Pollut. Bull. 156:111257. doi: 10.1016/j.marpolbul.2020.111257
Bilkovic, D. M., Greiner, J., Horan, J., and Stubbs, Q. (2014). Horan, J., D. Bilkovic, N. Gardner, J. Greiner, L. Karrh, K. Sellner, Q. Stubbs. 2014. Designing Sustainable Coastal Habitats. STAC (Chesapeake Bay Program Scientific and Technical Advisory Committee) Publ. #14-003). Edgewater, MD: STAC, 74.
Burchard, H., Schuttelaars, H. M., and Ralston, D. K. (2018). Sediment trapping in estuaries. Annu. Rev. Mar. Sci. 10, 371–395.
Cable, R. N., Beletsky, D., Beletsky, R., Wigginton, K., Locke, B. W., and Duhaime, M. B. (2017). Distribution and modeled transport of plastic pollution in the Great Lakes, the world’s largest freshwater resource. Front. Environ. Sci. 5:45. doi: 10.3389/fenvs.2017.00045
Campanale, C., Massarelli, C., Savino, I., Locaputo, V., and Uricchio, V. F. (2020). A detailed review study on potential effects of microplastics and additives of concern on human health. Int. J. Environ. Res. Public Health 17:1212. doi: 10.3390/ijerph17041212
Carpenter, E. J., and Smith, K. L. (1972). Plastics on the Sargasso Sea surface. Science 175, 1240–1241. doi: 10.1126/science.175.4027.1240
Chamas, A., Moon, H., Zheng, J., Qiu, Y., Tabassum, T., Jang, J. H., et al. (2020). Degradation rates of plastics in the environment. ACS Sustainable Chem. Engineer. 8, 3494–3511.
Chenillat, F., Huck, T., Maes, C., Grima, N., and Blanke, B. (2021). Fate of floating plastic debris released along the coasts in a global ocean model. Mar. Pollut. Bull. 165:112116. doi: 10.1016/j.marpolbul.2021.112116
Cohen, J. H., Internicola, A. M., Mason, R. A., and Kukulka, T. (2019). Observations and simulations of microplastic debris in a tide, wind, and freshwater-driven estuarine environment: the Delaware Bay. Environ. Sci. Technol. 53, 14204–14211. doi: 10.1021/acs.est.9b04814
Cole, M., Lindeque, P., Halsband, C., and Galloway, T. S. (2011). Microplastics as contaminants in the marine environment: a review. Mar. Pollut. Bull. 62, 2588–2597. doi: 10.1016/j.marpolbul.2011.09.025
Coles, J. F., Fisher, S. C., and Spanjer, A. R. (2020). Microplastics Data Collected From Streams in the Northeastern United States, 2017-2018, ed. U.S.G.S.D. Release (Reston, VA: U.S. Geological Survey data release). doi: 10.5066/P9DT5N3N
Collins, C., and Hermes, J. C. (2019). Modelling the accumulation and transport of floating marine micro-plastics around South Africa. Mar. Pollut. Bull. 139, 46–58. doi: 10.1016/j.marpolbul.2018.12.028
Copernicus Climate Change Service (C3S) (2017). “ERA5: fifth generation of ECMWF atmospheric reanalyses of the global climate,” in Copernicus Climate Change Service Climate Data Store (CDS). Available online at: https://cds.climate.copernicus.eu/cdsapp#!/home (accessed March 1, 2020).
Cózar, A., Echevarría, F., González-Gordillo, J. I, Irigoien, X., Ubeda, B., Hernández-León, S., et al. (2014). Plastic debris in the open ocean. Proc. Natl. Acad. Sci. U.S.A. 111, 10239–10244.
Critchell, K., and Lambrechts, J. (2016). Modelling accumulation of marine plastics in the coastal zone; what are the dominant physical processes? Estuarine Coast. Shelf Sci. 171, 111–122. doi: 10.1016/j.ecss.2016.01.036
Da, F., Friedrichs, M. A. M., and St-Laurent, P. (2018). Impacts of atmospheric nitrogen deposition and coastal nitrogen fluxes on oxygen concentrations in Chesapeake Bay. J. Geophys. Res.: Oceans 123, 5004–5025. doi: 10.1029/2018jc014009
Díez-Minguito, M., Bermúdez, M., Gago, J., Carretero, O., and Viñas, L. (2020). Observations and idealized modelling of microplastic transport in estuaries: the exemplary case of an upwelling system (Ría de Vigo, NW Spain). Mar. Chem. 222:103780. doi: 10.1016/j.marchem.2020.103780
Dortch, M. S., Chapman, R. S., and Abt, S. R. (1992). Application of three-dimensional Lagrangian residual transport. J. Hydraulic Eng. 118, 831–848. doi: 10.1061/(asce)0733-9429(1992)118:6(831)
Du, J., and Shen, J. (2016). Water residence time in Chesapeake Bay for 1980–2012. J. Mar. Syst. 164, 101–111. doi: 10.1016/j.jmarsys.2016.08.011
Dürr, H. H., Laruelle, G. G., van Kempen, C. M., Slomp, C. P., Meybeck, M., and Middelkoop, H. (2011). Worldwide typology of nearshore coastal systems: defining the estuarine filter of river inputs to the oceans. Estuaries Coasts 34, 441–458. doi: 10.1007/s12237-011-9381-y
Easton, Z., Scavia, D., Alexander, R., Band, L., Boomer, K., Kleinman, P., et al. (2017). Scientific and Technical Advisory Committee Review of the Phase 6 Chesapeake Bay Watershed Model. Edgewater, MD: STAC.
Eriksen, M., Lebreton, L. C. M., Carson, H. S., Thiel, M., Moore, C. J., Borerro, J. C., et al. (2014). Plastic pollution in the world’s oceans: more than 5 trillion plastic pieces weighing over 250,000 tons afloat at sea. PLoS One 9:e111913. doi: 10.1371/journal.pone.0111913
Feng, Y., Friedrichs, M. A. M., Wilkin, J., Tian, H., Yang, Q., Hofmann, E. E., et al. (2015). Chesapeake Bay nitrogen fluxes derived from a land-estuarine ocean biogeochemical modeling system: model description, evaluation, and nitrogen budgets. J. Geophys. Res. Biogeosci. 120, 1666–1695. doi: 10.1002/2015jg002931
Frère, L., Paul-Pont, I., Rinnert, E., Petton, S., Jaffré, J., Bihannic, I., et al. (2017). Influence of environmental and anthropogenic factors on the composition, concentration and spatial distribution of microplastics: a case study of the Bay of Brest (Brittany, France). Environ. Pollut. 225, 211–222. doi: 10.1016/j.envpol.2017.03.023
Galafassi, S., Nizzetto, L., and Volta, P. (2019). Plastic sources: a survey across scientific and grey literature for their inventory and relative contribution to microplastics pollution in natural environments, with an emphasis on surface water. Sci. Total Environ. 693:133499. doi: 10.1016/j.scitotenv.2019.07.305
Genc, A. N., Vural, N., and Balas, L. (2020). Modeling transport of microplastics in enclosed coastal waters: a case study in the Fethiye Inner Bay. Mar. Pollut. Bull. 150:110747. doi: 10.1016/j.marpolbul.2019.110747
Geyer, R., Jambeck, J. R., and Law, K. L. (2017). Production, use, and fate of all plastics ever made. Sci. Adv. 3:e1700782. doi: 10.1126/sciadv.1700782
Haidvogel, D. B., Arango, H., Budgell, W. B., Cornuelle, B. D., Curchitser, E., Di Lorenzo, E., et al. (2008). Ocean forecasting in terrain-following coordinates: formulation and skill assessment of the regional ocean modeling system. J. Computational Phys. 227, 3595–3624. doi: 10.1016/j.jcp.2007.06.016
Hardesty, B. D., Harari, J., Isobe, A., Lebreton, L., Maximenko, N., Potemra, J., et al. (2017). Using numerical model simulations to improve the understanding of micro-plastic distribution and pathways in the marine environment. Front. Mar. Sci. 4:30. doi: 10.3389/fmars.2017.00030
Hinson, K. E., Friedrichs, M. A. M., St-Laurent, P., Da, F., and Najjar, R. G. (2021). Extent and causes of Chesapeake Bay warming. J. Am. Water Resour. Assoc. 20, 1–20.
Hirai, H., Takada, H., Ogata, Y., Yamashita, R., Mizukawa, K., Saha, M., et al. (2011). Organic micropollutants in marine plastics debris from the open ocean and remote and urban beaches. Mar. Pollut. Bull. 62, 1683–1692. doi: 10.1016/j.marpolbul.2011.06.004
Hood, R. R., Wang, H. V., Purcell, J. E., Houde, E. D., and Harding, L. W. Jr. (1999). Modeling particles and pelagic organisms in Chesapeake Bay: convergent features control plankton distributions. J. Geophys. Res.: Oceans 104, 1223–1243. doi: 10.1029/1998jc900020
Hutchings, J. A., Bianchi, T. S., Najjar, R. G., Herrmann, M., Kemp, W. M., Hinson, A. L., et al. (2020). Carbon deposition and burial in estuarine sediments of the contiguous United States. Glob. Biogeochem. Cycles 34:e2019GB006376.
Irby, I. D., and Friedrichs, M. A. M. (2019). Evaluating confidence in the impact of regulatory nutrient reduction on Chesapeake Bay water quality. Estuaries Coasts 42, 16–32. doi: 10.1007/s12237-018-0440-5
Isobe, A., Kubo, K., Tamura, Y., Kako, S. I, Nakashima, E., and Fujii, N. (2014). Selective transport of microplastics and mesoplastics by drifting in coastal waters. Mar. Pollut. Bull. 89, 324–330. doi: 10.1016/j.marpolbul.2014.09.041
Ivar do Sul, J. A. (2021). Why it is important to analyze the chemical composition of microplastics in environmental samples. Mar. Pollut. Bull. 165:112086. doi: 10.1016/j.marpolbul.2021.112086
Iwasaki, S., Isobe, A., Kako, S. I, Uchida, K., and Tokai, T. (2017). Fate of microplastics and mesoplastics carried by surface currents and wind waves: a numerical model approach in the Sea of Japan. Mar. Pollut. Bull. 121, 85–96. doi: 10.1016/j.marpolbul.2017.05.057
Jalón-Rojas, I., Wang, X. H., and Fredj, E. (2019). A 3D numerical model to Track Marine Plastic Debris (TrackMPD): sensitivity of microplastic trajectories and fates to particle dynamical properties and physical processes. Mar. Pollut. Bull. 141, 256–272. doi: 10.1016/j.marpolbul.2019.02.052
Jambeck, J. R., Geyer, R., Wilcox, C., Siegler, T. R., Perryman, M., Andrady, A., et al. (2015). Plastic waste inputs from land into the ocean. Science 347, 768–771. doi: 10.1126/science.1260352
Kane, I. A., Clare, M. A., Miramontes, E., Wogelius, R., Rothwell, J. J., Garreau, P., et al. (2020). Seafloor microplastic hotspots controlled by deep-sea circulation. Science 368:1140. doi: 10.1126/science.aba5899
Kemp, W. M., Boynton, W. R., Adolf, J. E., Boesch, D. F., Boicourt, W. C., Brush, G., et al. (2005). Eutrophication of Chesapeake Bay: historical trends and ecological interactions. Mar. Ecol. Progr. Ser. 303, 1–29. doi: 10.3354/meps303001
Khatmullina, L., and Isachenko, I. (2017). Settling velocity of microplastic particles of regular shapes. Mar. Pollut. Bull. 114, 871–880. doi: 10.1016/j.marpolbul.2016.11.024
Kvale, K., Prowe, A. E. F., Chien, C. T., Landolfi, A., and Oschlies, A. (2020). The global biological microplastic particle sink. Sci. Rep. 10:16670.
Law, K. L., and Thompson, R. C. (2014). Microplastics in the seas. Science 345, 144–145. doi: 10.1126/science.1254065
Lebreton, L., Egger, M., and Slat, B. (2019). A global mass budget for positively buoyant macroplastic debris in the ocean. Sci. Rep. 9:12922.
Lebreton, L. C. M., van der Zwet, J., Damsteeg, J.-W., Slat, B., Andrady, A., and Reisser, J. (2017). River plastic emissions to the world’s oceans. Nat. Commun. 8:15611.
Lett, C., Verley, P., Mullon, C., Parada, C., Brochier, T., Penven, P., et al. (2008). A Lagrangian tool for modelling ichthyoplankton dynamics. Environ. Modell. Softw. 23, 1210–1214. doi: 10.1016/j.envsoft.2008.02.005
Liro, M., Emmerik, T. V., Wyżga, B., Liro, J., and Mikuś, P. (2020). Macroplastic storage and remobilization in rivers. Water 12:2055. doi: 10.3390/w12072055
Liubartseva, S., Coppini, G., Lecci, R., and Clementi, E. (2018). Tracking plastics in the Mediterranean: 2D Lagrangian model. Mar. Pollut. Bull. 129, 151–162. doi: 10.1016/j.marpolbul.2018.02.019
Luznik, L., and Flack, K. A. (2010). “Observations of turbulent flow fields in the Chesapeake Bay estuary for tidal energy conversion,” in Oceans 2010 MTS/IEEE Seattle, 1–5. doi: 10.1109/OCEANS.2010.5664375
Martin, C., Baalkhuyur, F., Valluzzi, L., Saderne, V., Cusack, M., Almahasheer, H., et al. (2020). Exponential increase of plastic burial in mangrove sediments as a major plastic sink. Sci. Adv. 6:eaaz5593. doi: 10.1126/sciadv.aaz5593
McGlathery, K. J., Sundbäck, K., and Anderson, I. (2007). Eutrophication in shallow coastal bays and lagoons: the role of plants in the coastal filter. Mar. Ecol.-Progr. Ser. - Mar. Ecol.-Progr. Ser. 348, 1–18. doi: 10.3354/meps07132
Moore, C., Guignet, D., Maguire, K. B., Dockins, C., and Simon, N. B. (2018). Valuing ecological improvements in the Chesapeake Bay and the importance of ancillary benefits. J. Benefit-Cost Analys. 9, 1–26. doi: 10.1017/bca.2017.9
Moore, C., Lattin, G., and Zellers, A. F. (2011). Quantity and type of plastic debris flowing from two urban rivers to coastal waters and beaches of Southern California. J. Integr. Coastal Zone Manage. 11, 65–73. doi: 10.5894/rgci194
Moriarty, J. M., Friedrichs, M. A. M., and Harris, C. K. (2021). Seabed resuspension in the Chesapeake Bay: implications for biogeochemical cycling and hypoxia. Estuaries Coasts 44, 103–122. doi: 10.1007/s12237-020-00763-8
Murphy, R., Robinson, M., Landry, B., Wardrop, D., Luckenbach, M., Grubert, K., et al. (2019). Microplastics in the Chesapeake Bay and its Watershed: State of the Knowledge, Data Gaps and Relationship to Management Goals. Edgewater, MD: Stac.
Najjar, R. G., Herrmann, M., Alexander, R., Boyer, E. W., Burdige, D. J., Butman, D., et al. (2018). Carbon budget of tidal wetlands, estuaries, and shelf waters of Eastern North America. Glob. Biogeochem. Cycles 32, 389–416. doi: 10.1002/2017gb005790
Olivelli, A., Hardesty, B. D., and Wilcox, C. (2020). Coastal margins and backshores represent a major sink for marine debris: insights from a continental-scale analysis. Environ. Res. Lett. 15:074037. doi: 10.1088/1748-9326/ab7836
Pabortsava, K., and Lampitt, R. S. (2020). High concentrations of plastic hidden beneath the surface of the Atlantic Ocean. Nat. Commun. 11:4073.
Parada, C., Van Der Lingen, C. D., Mullon, C., and Penven, P. (2003). Modelling the effect of buoyancy on the transport of anchovy (Engraulis capensis) eggs from spawning to nursery grounds in the southern Benguela: an IBM approach. Fish. Oceanogr. 12, 170–184. doi: 10.1046/j.1365-2419.2003.00235.x
Peliz, A., Marchesiello, P., Dubert, J., Marta-Almeida, M., Roy, C., and Queiroga, H. (2007). A study of crab larvae dispersal on the Western Iberian Shelf: physical processes. J. Mar. Syst. 68, 215–236. doi: 10.1016/j.jmarsys.2006.11.007
Pires, R. F. T., Peliz, A., Pan, M., and dos Santos, A. (2020). There and back again” - How decapod megalopae find the way home: a modelling exercise for Pachygrapsus marmoratus. Progr. Oceanogr. 184:102331. doi: 10.1016/j.pocean.2020.102331
Pverley, B. N., and Clett. (2020). Ichthyop/Ichthyop 3.3.6 (Version 3.3.6) [Online]. Zenodo. Available online at: http://doi.org/10.5281/zenodo.4244484 (accessed February 1, 2021).
Riascos, J. M., Valencia, N., Peña, E. J., and Cantera, J. R. (2019). Inhabiting the technosphere: the encroachment of anthropogenic marine litter in Neotropical mangrove forests and its use as habitat by macrobenthic biota. Mar. Pollut. Bull. 142, 559–568. doi: 10.1016/j.marpolbul.2019.04.010
Rummel, C. D., Jahnke, A., Gorokhova, E., Kühnel, D., and Schmitt-Jansen, M. (2017). Impacts of biofilm formation on the fate and potential effects of microplastic in the aquatic environment. Environ. Sci. Technol. Lett. 4, 258–267. doi: 10.1021/acs.estlett.7b00164
Ryan, P. G., Weideman, E. A., Perold, V., and Moloney, C. L. (2020). Toward balancing the budget: surface macro-plastics dominate the mass of particulate pollution stranded on beaches. Front. Mar. Sci. 7:575395. doi: 10.3389/fmars.2020.575395
Santos, B. S., Kaplan, D. M., Friedrichs, M. A. M., Barco, S. G., Mansfield, K. L., and Manning, J. P. (2018). Consequences of drift and carcass decomposition for estimating sea turtle mortality hotspots. Ecol. Indicators 84, 319–336. doi: 10.1016/j.ecolind.2017.08.064
Schernewski, G., Radtke, H., Hauk, R., Baresel, C., Olshammar, M., Osinski, R., et al. (2020). Transport and behavior of microplastics emissions from urban sources in the baltic sea. Front. Environ. Sci. 8:579361. doi: 10.3389/fenvs.2020.579361
Schmidt, C., Krauth, T., and Wagner, S. (2017). Export of plastic debris by rivers into the sea. Environ. Sci. Technol. 51, 12246–12253. doi: 10.1021/acs.est.7b02368
Schubel, J. R., and Kennedy, V. S. (1984). “The estuary as a filter: an introduction,” in The Estuary As a Filter, ed. V. S. Kennedy (Cambridge: Academic Press), 1–11. doi: 10.1016/b978-0-12-405070-9.50007-4
Schulte, J. A., Najjar, R. G., and Lee, S. (2017). Salinity and streamflow variability in the Mid-Atlantic region of the United States and its relationship with large-scale atmospheric circulation patterns. J. Hydrol. 550, 65–79. doi: 10.1016/j.jhydrol.2017.03.064
Schulte, J. A., Najjar, R. G., and Li, M. (2016). The influence of climate modes on streamflow in the Mid-Atlantic region of the United States. J. Hydrol.: Regional Stud. 5, 80–99. doi: 10.1016/j.ejrh.2015.11.003
Schwarz, A. E., Ligthart, T. N., Boukris, E., and van Harmelen, T. (2019). Sources, transport, and accumulation of different types of plastic litter in aquatic environments: a review study. Mar. Pollut. Bull. 143, 92–100. doi: 10.1016/j.marpolbul.2019.04.029
Sharqawy, M. H., Lienhard, J. H., and Zubair, S. M. (2010). Thermophysical properties of seawater: a review of existing correlations and data. Desalination Water Treat. 16, 354–380. doi: 10.5004/dwt.2010.1079
Shchepetkin, A. F., and McWilliams, J. C. (2005). The regional oceanic modeling system (ROMS): a split-explicit, free-surface, topography-following-coordinate oceanic model. Ocean Modell. 9, 347–404. doi: 10.1016/j.ocemod.2004.08.002
Shchepetkin, A. F., and McWilliams, J. C. (2009). “Computational kernel algorithms for fine-scale, multiprocess, longtime oceanic simulations,” in Handbook of Numerical Analysis, eds R. M. Temam and J. J. Tribbia (Amsterdam: Elsevier), 121–183. doi: 10.1016/s1570-8659(08)01202-0
Shenk, G. W., and Linker, L. C. (2013). Development and application of the 2010 Chesapeake Bay watershed total maximum daily load model. JAWRA J. Am. Water Resour. Assoc. 49, 1042–1056. doi: 10.1111/jawr.12109
Sterl, M. F., Delandmeter, P., and van Sebille, E. (2020). Influence of barotropic tidal currents on transport and accumulation of floating microplastics in the global open ocean. J. Geophys. Res.: Oceans 125:e2019JC015583.
St-Laurent, P., Friedrichs, M. A. M., Najjar, R. G., Shadwick, E. H., Tian, H., and Yao, Y. (2020). Relative impacts of global changes and regional watershed changes on the inorganic carbon balance of the Chesapeake Bay. Biogeosciences 17, 3779–3796. doi: 10.5194/bg-17-3779-2020
Stow, C. A., Jolliff, J., McGillicuddy, D. J. Jr., Doney, S. C., Allen, J. I., Friedrichs, M. A. M., et al. (2009). Skill assessment for coupled biological/physical models of marine systems. J. Mar. Syst. : J. Eur. Assoc. Mar. Sci. Techniques 76, 4–15. doi: 10.1016/j.jmarsys.2008.03.011
Sutton, R., Franz, A., Gilbreath, A., Lin, D., Miller, L., Sedlak, M., et al. (2019). Understanding Microplastic Levels, Pathways, and Transport in the San Francisco Bay Region. Richmond, CA: San Francisco Estuary Institute.
The ROMS/TOMS Group (2021). The ROMS/TOMS Group. Available online at: https://www.myroms.org/index.php?page=License_ROMS (accessed February 1, 2021).
Tramoy, R., Gasperi, J., Colasse, L., Silvestre, M., Dubois, P., Noûs, C., et al. (2020). Transfer dynamics of macroplastics in estuaries – New insights from the Seine estuary: part 2. Short-term dynamics based on GPS-trackers. Mar. Pollut. Bull. 160:111566. doi: 10.1016/j.marpolbul.2020.111566
Turner, J. S., St-Laurent, P., Friedrichs, M. A. M., and Friedrichs, C. T. (2021). Effects of reduced shoreline erosion on Chesapeake Bay water clarity. Sci. Tot. Environ. 769:145157. doi: 10.1016/j.scitotenv.2021.145157
Umlauf, L., and Burchard, H. (2003). A generic length-scale equation for geophysical turbulence models. J. Mar. Res. 61, 235–265. doi: 10.1357/002224003322005087
Vermeiren, P., Muñoz, C. C., and Ikejima, K. (2016). Sources and sinks of plastic debris in estuaries: a conceptual model integrating biological, physical and chemical distribution mechanisms. Mar. Pollut. Bull. 113, 7–16. doi: 10.1016/j.marpolbul.2016.10.002
Vims Comprehensive Coastal Inventory (2018). “Virginia Shoreline Inventory Database”, (ed.) Center for Coastal Resources Management. 01 January 2013 ed. (Gloucester Point, Virginia: Virginia Institute of Marine Science, College of William and Mary. Available online at: http://www.vims.edu/ccrm/research/inventory/index.php (accessed July 24, 2020).
Wardrop, D., Bott, C., Criddle, C., Hale, R., McDevitt, J., Morse, M., et al. (2016). Technical Review of Microbeads/Microplastics in the Chesapeake Bay. Edgewater, MD: Stac.
Warner, J. C., Sherwood, C. R., Arango, H. G., and Signell, R. P. (2005). Performance of four turbulence closure models implemented using a generic length scale method. Ocean Modell. 8, 81–113. doi: 10.1016/j.ocemod.2003.12.003
Watkins, L., Sullivan, P. J., and Walter, M. T. (2019). A case study investigating temporal factors that influence microplastic concentration in streams under different treatment regimes. Environ. Sci. Pollut. Res. 26, 21797–21807. doi: 10.1007/s11356-019-04663-8
Wichmann, D., Delandmeter, P., and van Sebille, E. (2019). Influence of near-surface currents on the global dispersal of marine microplastic. J. Geophys. Res.: Oceans 124, 6086–6096. doi: 10.1029/2019jc015328
Woodall, L. C., Sanchez-Vidal, A., Canals, M., Paterson, G. L. J., Coppock, R., Sleight, V., et al. (2014). The deep sea is a major sink for microplastic debris. R. Soc. Open Sci. 1:140317. doi: 10.1098/rsos.140317
Xu, J., Long, W., Wiggert, J. D., Lanerolle, L. W. J., Brown, C. W., Murtugudde, R., et al. (2012). Climate forcing and salinity variability in Chesapeake Bay, USA. Estuar. Coasts 35, 237–261. doi: 10.1016/j.scitotenv.2020.140947
Yonkos, L. T., Friedel, E. A., Perez-Reyes, A. C., Ghosal, S., and Arthur, C. D. (2014). Microplastics in four estuarine rivers in the Chesapeake Bay, U.S.A. Environ. Sci. Technol. 48, 14195–14202. doi: 10.1021/es5036317
Zhang, K., Hamidian, A. H., Tubić, A., Zhang, Y., Fang, J. K. H., Wu, C., et al. (2021). Understanding plastic degradation and microplastic formation in the environment: a review. Environ. Pollut. 274:116554. doi: 10.1016/j.envpol.2021.116554
Zhang, Z., Wu, H., Peng, G., Xu, P., and Li, D. (2020). Coastal ocean dynamics reduce the export of microplastics to the open ocean. Sci. Tot. Environ. 713:136634. doi: 10.1016/j.scitotenv.2020.136634
Keywords: ROMS (Regional Ocean Modeling System), Ichthyop model, Chesapeake Bay (United States), microplastics, estuaries
Citation: López AG, Najjar RG, Friedrichs MAM, Hickner MA and Wardrop DH (2021) Estuaries as Filters for Riverine Microplastics: Simulations in a Large, Coastal-Plain Estuary. Front. Mar. Sci. 8:715924. doi: 10.3389/fmars.2021.715924
Received: 27 May 2021; Accepted: 03 August 2021;
Published: 26 August 2021.
Edited by:
Tim van Emmerik, Wageningen University & Research, NetherlandsReviewed by:
Sarah Piehl, Leibniz Institute for Baltic Sea Research (LG), GermanyRomain Tramoy, Laboratoire Eau Environnement et Systèmes Urbains, École des ponts ParisTech, France
Lixin Zhu, East China Normal University, China
Copyright © 2021 López, Najjar, Friedrichs, Hickner and Wardrop. This is an open-access article distributed under the terms of the Creative Commons Attribution License (CC BY). The use, distribution or reproduction in other forums is permitted, provided the original author(s) and the copyright owner(s) are credited and that the original publication in this journal is cited, in accordance with accepted academic practice. No use, distribution or reproduction is permitted which does not comply with these terms.
*Correspondence: Alexander G. López, YXhsNTc1OEBwc3UuZWR1