Distinct Bottom-Water Bacterial Communities at Methane Seeps With Various Seepage Intensities in Haima, South China Sea
- 1CAS Key Laboratory of Pathogenic Microbiology and Immunology, Institute of Microbiology, Chinese Academy of Sciences, Beijing, China
- 2Department of Bioinformatics, School of Basic Medical Sciences, Southern Medical University, Guangzhou, China
- 3Key Laboratory of Ocean and Marginal Sea Geology, South China Sea Institute of Oceanology, Innovation Academy of South China Sea Ecology and Environmental Engineering, Chinese Academy of Sciences, Guangzhou, China
- 4MNR Key Laboratory of Marine Mineral Resources, Guangzhou Marine Geological Survey, Ministry of Natural Resources, Guangzhou, China
- 5Shanghai Engineering Research Center of Hadal Science and Technology, College of Marine Sciences, Shanghai Ocean University, Shanghai, China
- 6Guangdong Provincial Key Laboratory of Single Cell Technology and Application, Department of Biochemistry and Molecular Biology, School of Basic Medical Sciences, Southern Medical University, Guangzhou, China
Methane seeps are chemosynthetic ecosystems in the deep-sea environment. Microbial community structures have been extensively studied in the seepage-affected sediments and investigation in the water column above the seeping sites is still lacking. In this study, prokaryotic communities in the bottom water about 50 cm from the seabed at methane seeps with various seepage intensities in Haima, South China Sea were comparatively studied by using 16S ribosomal RNA gene sequencing. These sites were assigned based on their distinct methane content levels and seafloor landscapes as the non-seepage (NS) site, low-intensity seepage (LIS) site, and high-intensity seepage (HIS) site. The abundances of the dominant phyla Proteobacteria, Bacteroidetes, and Actinobacteria differed significantly between NS and the two seepage sites (p < 0.05). Alpha diversity differed among the three sites with the HIS site showing the lowest community diversity. Principal component analysis revealed highly divergent bacterial community structures at three sites. Many environmental variables including temperature, alkalinity, pH, methane, dissolved organic carbon (DOC), and inorganic nutrients were measured. Redundancy analysis indicated that methane content is the key environmental factor driving bacterial community variation (p = 0.001). Linear discriminant analysis effect size analysis identified various differentially enriched genera at the LIS and HIS sites. Phylogenetic analysis revealed close phylogenetic relationship among the operational taxonomic units of these genera with known oil-degrading species, indicating oil seepage may occur at the Haima cold seeps. Co-occurrence networks indicated that the strength of microbial interactions was weakest at the HIS site. This study represents a comprehensive comparison of microbial profiles in the water column of cold seeps in the SCS, revealing that the seepage intensity has a strong impact on bacterial community dynamics.
Introduction
Cold seeps are highly productive ecosystems dominated by profuse chemosynthetic microbes (Boetius and Wenzhofer, 2013; Joye, 2020). Apart from thermal maturation of organic matter at depth (Galimov, 1988), methane (CH4) is also produced through degradation of organic matter in the subsurface sediment by methanogens; sulfate-reducing bacteria and anaerobic methanotrophic (ANME) archaea consume CH4 in the sediments that is often anoxic and aerobic methanotrophic bacteria in the oxic water column oxidize CH4 seeped from sediment (Niu et al., 2018). These processes facilitate the formation of carbonates and result in high concentrations of hydrogen sulfide and oxygen depletion (Li et al., 2021). In general, these geochemical alterations may help to reconstruct the microbial communities in the waters above cold seeps. Chemosynthetic organisms in cold seeps utilize CH4 or hydrogen sulfide to sustain various faunal assemblages (Pasulka et al., 2016) such as tubeworms (Zhao et al., 2020), mussels (Sun et al., 2017; Xu et al., 2019), and bivalves.
Because abundant cold seeps are distributed in the South China Sea (SCS), it is received increasing research interest. To date, more than 30 cold seeps have been discovered on the northern continental slope of the SCS (Feng et al., 2015), but only two cold seeps are still active and identified as harboring faunal assemblages (Liang et al., 2017). One is site F and another is Haima seeps, which reported for the first time in 2015, located at a depth of approximately 1,360–1,400 m in the southern part of the Qiongdongnan Basin (Liang et al., 2017).
Although microbial community structures have been investigated at cold seeps in the SCS, nearly all the reports have focused on seepage-affected sediments (Lai et al., 2007; Zhang et al., 2012; Niu et al., 2017; Wu et al., 2018; Cui et al., 2019, 2020; Zhuang et al., 2019; Li et al., 2020). Recently, Zhang et al. (2020) investigate prokaryotes along the depth profile of the water column. These authors found heterotrophs to be the major component of the bacterial community with the most abundant functions being enriched in carbohydrate metabolism in samples from deeper water layers of the Haima seep, indicating the presence of ample organic matter. In addition, Guan et al. (2018) detected unresolved complex mixtures (UCMs) in the hydrocarbon fractions of authigenic carbonates retrieved from Haima seeps, implicating the presence of heavily biodegraded organic matter or, more likely, oil. Indeed, UCMs have frequently been observed in authigenic seep carbonates from sites where oil seepage occurs (Birgel et al., 2011; Feng et al., 2014). Apart from UCMs, lipid biomarker patterns in the authigenic carbonates also indicate oil seepage in the Haima cold seeps (Guan et al., 2021). In addition, mud diapirs and faults are well developed in the Haima cold seeps, potentially serving as pathways for the upward migration of gas fluids from deep reservoirs to the seafloor (Xie et al., 2006; Huang et al., 2016). Therefore, an oil reservoir may exist in the Haima cold seeps and microbial communities in the water column may have the ability to degrade oil.
Although the microbial community in the water column of seep sites in the SCS has been studied, a comparison of sites with various seepage activities is still lacking. To elucidate how seepage intensity shapes the prokaryotic community, we compared the prokaryotic communities of seep sites in Haima with distinct seafloor landscapes and CH4 contents. Bottom-water samples were collected from sites of the non-seepage (NS), low-intensity seepage (LIS), and high-intensity seepage (HIS). Bacterial and archaeal communities were studied by using 16S ribosomal RNA (rRNA) gene amplicon sequencing and the concentrations of geochemical factors in the seep environment were quantified. The main aims were to better understand: (i) whether intergroup variance exists among sampling sites with different seepage intensities, (ii) the correlations between environmental factors and the prokaryotic community structure, and (iii) the dominant/signature microbes in active cold seeps, especially with regard to whether oil degraders inhabit such ecosystems.
Materials and Methods
Collection of Bottom-Water Samples and Geochemical Analysis
Sampling was conducted during an expedition in May 2019 at the Haima cold seeps located in the SCS, China. Bottom-water samples were collected by using 10-L Niskin bottles fixed on Remote Operated Vehicle (ROV) Haima from depths of 1,360–1,398 m (Figure 1A). Three sample sites were selected based on distinct seafloor landscapes: a NS site outside active seepage (Figure 1B), the LIS site with dead clams and live tubeworms (Figure 1C), and the HIS site with live mussels and continuous bubbling of gas (Figure 1D). Six samples from six stations were collected from the NS and HIS site and four samples were collected from the LIS site. The distance between three sites was 1–3.7 km and the stations at each site were approximately 100 m apart between each other.
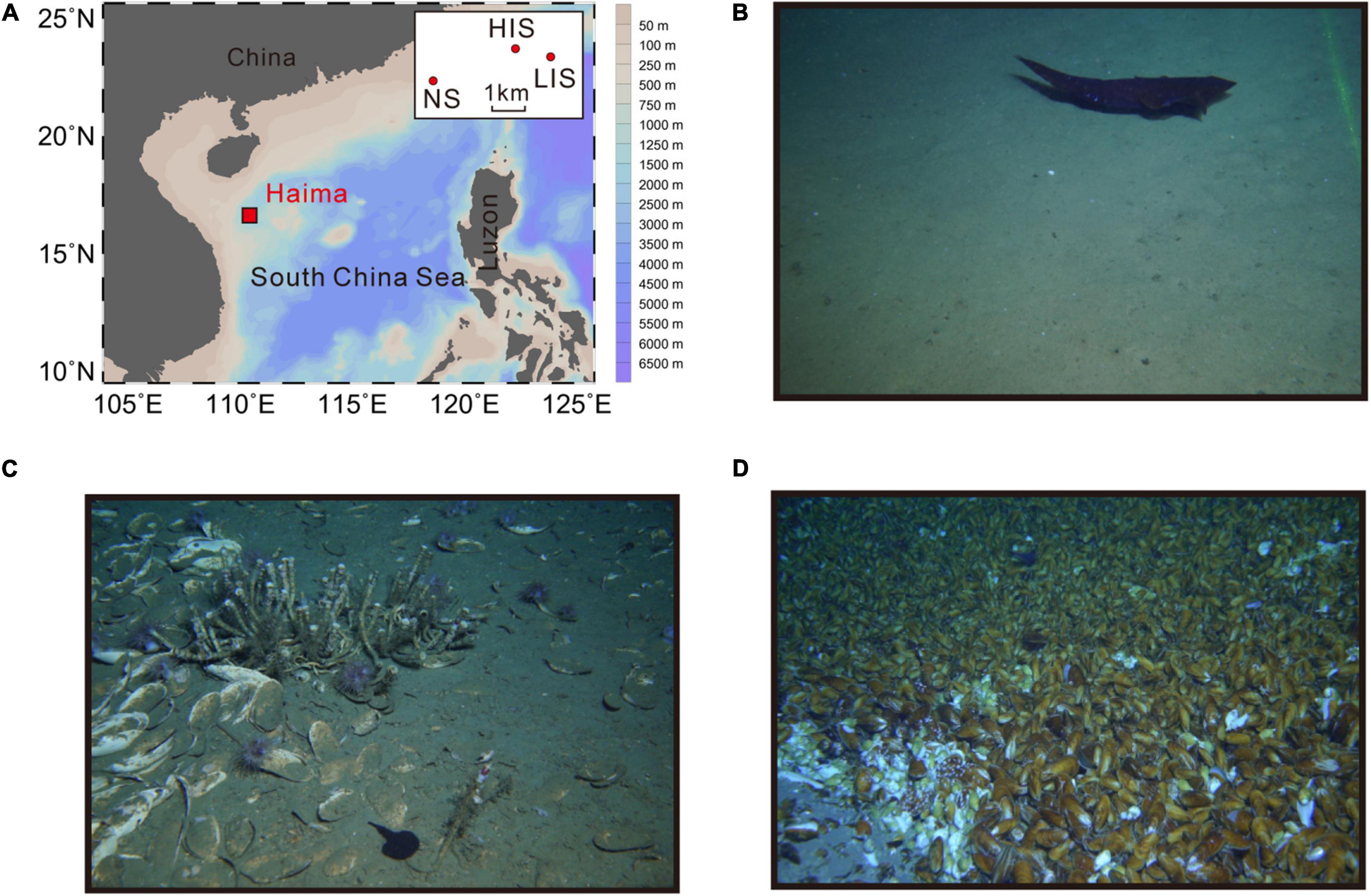
Figure 1. Location and landscapes of the sampling sites. (A) Map of the sampling sites with water depth. The white box on the top right is a zoom-in of the Haima area with the locations of three sampling sites. Landscapes of the non-seepage (NS) site (B), the low-intensity seepage (LIS) site (C), and the high-intensity seepage (HIS) site (D).
In situ CH4 concentrations were measured at each site by using the HydroCTM Methane Sensor (HYDROC GmbH, Flensburg, Germany, United Kingdom) mounted on the ROV Haima. In addition, the concentrations of inorganic nutrients, including nitrate, nitrite, ammonia, and phosphate, and the pH were quantified in reference to those described in “Specification of Oceanographic Investigation in China” (GB/T12763.4–2007, General Administration of Quality Supervision, Inspection, and Quarantine of the People’s Republic of China, 2008).1 The alkalinity was determined on board by direct titration with ∼0.006 M hydrochloric acid (HCl) by using a pH meter. A total of 4 L of collected liquid were immediately filtered through a 0.22-μm polycarbonate membrane (47-mm diameter, Millipore, MA, United States) to collect microbes. All the filtrates were stored at –80°C until further analysis.
Deoxyribonucleic Acid Extraction, PCR Amplification, and Sequencing
Total genomic DNA of microbes was extracted from the 0.22-μm polycarbonate filters by using the ALFA-SEQ Advanced Water DNA Kit (Magigene, Guangdong, China) following the instructions of the manufacturer. The extracted DNA was quantified with a NanoDrop 2000 Spectrophotometer (Thermo Scientific, Thermo Fisher Scientific Corporation, Waltham, MA) and the quality was checked via gel electrophoresis. The bacterial 16S rRNA gene V3–V4 region was amplified by using the specific forward primer 314F 5′-CCTAYGGGRBGCASCAG-3′and reverse primer 806R5′-GGACTACNNGGGTATCTAAT-3′ (Yu et al., 2005). The Archaea 16S rRNA gene V4 region was amplified with the forward primer Uni519F 5′-CAGYMGCCRCGGKAAHACC-3′ and reverse primer Arch806R 5′-GGACTACNSGGGTMTCTAAT-3′ (Zhang et al., 2015). Sample-specific 12-bp barcodes were assigned to perform multiplex sequencing. PCR was carried out by using the TaKaRa Premix Taq® version 2.0 (TaKaRa Biotechnology Corporation, Dalian, China) containing 25 μL premix Taq (2X), 1 μL (10 μM) each forward and reverse primer, 50 ng DNA template, and deionized distilled water (ddH2O) to a total of 50 μL. The “contaminant” control was performed with sterile ddH2O as the DNA template. PCR amplification was conducted as follows: 94°C for 5 min followed by 30 cycles consisting of denaturation at 94°C for 30 s, annealing at 52°C for 30 s, and extension at 72°C for 30 s and a final extension of 10 min at 72°C. PCR amplicons from PCR triplicates were mixed and examined by 1% agarose gel electrophoresis. The amplicons of each sample were pooled in equal amounts after concentration measurement with the GeneTools Analysis Software (version 4.03.05.0, SynGene, Karnataka, India) and purified with the E.Z.N.A.® Gel Extraction Kit (Omega, United States). Library construction was conducted according to the protocols of the manufacturer by using the NEBNext® UltraTM II DNA Library Prep Kit for Illumina® (New England Biolabs, United States). The amplification libraries were sequenced on the Illumina NovaSeq 6000 platform and 250 bp paired-end reads were generated (Guangdong Magigene Biotechnology Corporation Ltd., Guangzhou, China).
Quality Control, Sequence Assembly, and Taxonomy Assignment
Low quality ends of the pair-ended raw sequencing reads were trimmed with the assistance of fastp (Chen et al., 2018) in the “sliding and cut” way (–W = 4, –M = 20). The adapters were cut by using cutadapt (Martin, 2011). The pair-ended reads were merged by using the fastq_merge pairs function implemented in Ultra-fast sequence analysis (USEARCH) version 10 (Edgar, 2010) with the default parameters. The output merge reads, or namely “raw tags,” were undergone quality filter again by using fastp (–W = 4, –M = 20, average_qual = 25, and length_required = 200). The UNOISE3 function implemented in USEARCH was employed to denoise and filter chimera. After these steps, the optimized sequences, or “clean tags,” were clustered into operational taxonomic units (OTUs) at 97% sequence identity by the UPARSE (Edgar, 2013).
Taxonomy assignment was conducted by using a combination of three tools: (1) blastn (word_size = 21) embedded in standalone version of Basic Local Alignment Search Tool (BLAST+) version 2.11.0 (United States) (Camacho et al., 2009) against the SILVA 16S/18S (SSU) reference database version 138.1 (Bremen, Germany) (Quast et al., 2013); (2) OutTab function implemented in the USEARCH (identity cutoff = 97%) against its embedded Ribosomal Database Project (RDP) taxonomy 18 database; and (3) and the RDP classifier version 2.13 (confidence cutoff = 0.8; Wang et al., 2007) against SILVA SSU reference database version 138.1.
To determine valid taxonomy hits from the raw blastn output: (1) hits with identity less than 50% and coverage less than 70% of the total size of query sequence were filtered; (2) after sorting, the top 10 hits with the highest bit scores or lowest E-values were chosen to be the candidate best hits. In order to compare blast results to other tools, we introduced a statistic named “adjusted identity,” i.e., using query coverage to weight the identity. This statistics could reduce bias introduced by using only identity as cutoff (usually caused by local alignment) and provide a more intuitive statistics than E-value and bit score while comparing blast results to other tools. The adjusted identity threshold of convincing assignment by blastn was set to 97% to call a convincing assignment at genus level.
Finally, the best taxonomy assignment of all the OTUs was manually conducted based on results generated from the three tools and the inconsistence of result from these three software was manually inspected and adjusted (Supplementary Table 1).
Statistical Analysis
Rarefaction curves were generated with the online tool usearch-alpha_div_rare (version 10).2 Microbial community compositions at the phylum level are illustrated as stacked bar charts. Hierarchical clustering of the 52 most abundant genera (OTU abundance > 0.05%) was visualized as a heatmap by using “ggplot2” version 2.2.0 (Wickham, 2016). Diversity (Shannon and Simpson indices) and richness (Chao1 and abundance-based coverage estimator (ACE) indices) estimators were calculated with 97% sequence similarity as cutoff values by the corresponding algorithms embedded in Quantitative Insights Into Microbial Ecology (QIIME) (Caporaso et al., 2010).
To illustrate the distribution pattern of microbial communities, principal component analysis (PCA; Hotelling, 1933) and principal coordinate analysis (PCoA; Zuur et al., 2007) were carried out based on Euclidean distance metrics and Bray–Curtis distance matrices, respectively. To evaluate the presence of a significant difference in the microbial community among the various sampling sites, analysis of similarities (ANOSIM; Clarke, 1993) with weighted UniFrac distance metrics or analysis of Adonis (Anderson, 2001) based on Bray–Curtis distance metrics was conducted in the vegan package embedded in R. Sparse partial least squares-discriminant analysis (sPLS-DA) was applied to reveal which organisms are responsible for the dissimilarity observed in the community composition among different samples by using the “PLS-DA” function in the R package “mixOmics” version 6.3.2 (Rohart et al., 2017).
The redundancy analysis (RDA) in combination with the Monte Carlo permutation test (ter Braak, 1990) was applied to identify a subset of environmental variables that statistically explain the greatest proportions of taxon variation. The Monte Carlo permutation test showed a p-value associated with the effect of the environmental variable on the microbial composition of the samples. This analysis was performed in R with the vegan package.
Linear discriminant analysis effect size analysis (Segata et al., 2011) was performed by using the LEfSe package embedded in an online tool3 to identify dominant taxa among the three sites.
A phylogenetic tree of claimed OTUs with the known oil-degrading species was constructed. The best nucleotide substitution model was automatically calculated and selected with the assistance of Mega 7 (Kumar et al., 2016). Sequence alignment was conducted by muscle algorithm embedded in Mega 7. The phylogenetic tree was inferred by using the maximum likelihood method based on the Kimura 2-parameter model (Kimura, 1980).
To construct co-occurrence networks of the microbiota of different groups, pairwise intergenus correlations were calculated according to genus abundance and visualized by using the R package “igraph” version 1.2.6 (Csardi and Nepusz, 2006). The numbers of co-occurring microbe pairs under different Bray–Curtis distance matrices were compared.
Statistical procedures were carried out with R. For all the analyses, p < 0.05 was considered as statistically significant and significance levels were indicated as follows: ∗∗∗p < 0.001; ∗∗p < 0.01; ∗p < 0.05.
Results
Sampling Sites and Environmental Factors
The three sampling sites had distinct landscapes. The NS site is located outside any area with active seepage and the CH4 concentration at this site was 0.18–0.36 μM, which was set as the baseline (Table 1). At this site, no or few faunal assemblages were observed on the seafloor (Figure 1B). In contrast, dead clams and live tubeworms were occasionally observed at the LIS site (Figure 1C). At the HIS site, abundant live mussels and authigenic carbonates could be observed on the seafloor (Figure 1D), accompanied by continuous gas bubbling. Active seepage at the LIS and NIS sites was confirmed by the high CH4 content in the water column measured about 50 cm above the seafloor with values of 88.3–110.4 and 1,987.1–8,831.6 μM, respectively.
In addition to CH4 concentrations, other fundamental environmental variables, including temperature, alkalinity, pH, dissolved organic carbon (DOC), nitrate, nitrite, ammonium, and phosphate concentrations, were measured; the results are listed in Table 1. Temperature, alkalinity, and pH were relatively constant among the three sites. However, DOC concentrations increased from ∼0.79 μM at the NS site to ∼1.10 μM at the LIS site and were maintained at approximately 1.50 μM at the HIS site. Interestingly, the concentrations of all the inorganic nutrients, including nitrate, nitrite, ammonium, and phosphate, were maximum at the LIS site (Table 1). The DOC and inorganic nutrients possibly sourced from deep fluids associated with CH4 seepage as suggested by D’Souza et al. (2016). Furthermore, the fluid seepage intensity is controlled by flow rate, thus more nutrients could dissolve in bottom water in relatively low flow rate condition at the LIS site, while nutrients upward migration to shallow water due to high flow rate at the HIS site.
Bacterial Community Composition and Alpha Diversity at the Study Sites
Deoxyribonucleic acid of microbes in the water column was extracted and sequenced. The OTU table of blank control was checked and only a few taxa could be observed with low abundance (Supplementary Table 2). Considering the extremely low counts of contaminated OTUs in the table, we concluded bacteria and archaea OTU tables with no decontamination step. The number of sequence for each sample of bacterial and archaeal libraries was included in Supplementary Table 3. An average of 53,451 and 72,919 sequences were obtained for bacteria and archaea, respectively, in all the 16 samples. Rarefaction curves based on observed bacterial and archaeal OTUs were plotted. Both the curves reached an asymptote for bacterial and archaeal libraries; therefore, the sequencing data were sufficient to detect the microorganisms present and provided reliable results (Supplementary Figure 1).
With respect to the bacterial community composition, Proteobacteria was the most abundant phylum among all the sites followed by Bacteroidetes and Actinobacteria (Figure 2 and Supplementary Table 4). The relative abundance of these phyla was significantly different between the NS and the two CH4 seepage sites, while the LIS and HIS sites possessed similar microbial compositions with no significant difference (Supplementary Figure 2). As shown in the genus heatmap (Figure 3 and Supplementary Table 4), the distribution of top 52 abundant genera was also different between the NS and the two seepage sites, but relatively similar between the LIS and HIS sites.
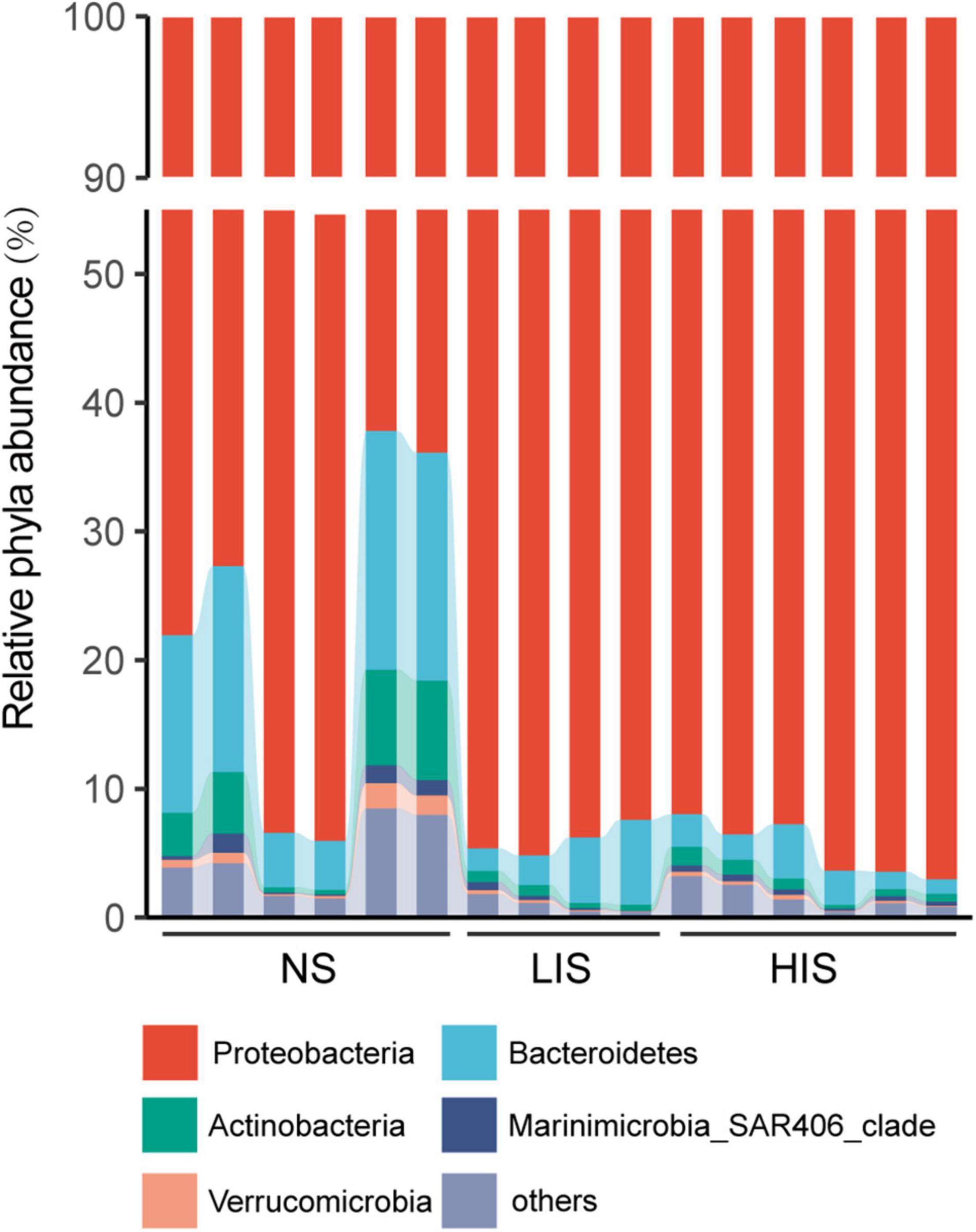
Figure 2. Bar chart of the relative abundances of phylum-level taxa in 16 samples from the three study sites. To clearly show all the phylum compositions, the percentage of dominant Proteobacteria was reduced by 45% in every sample.
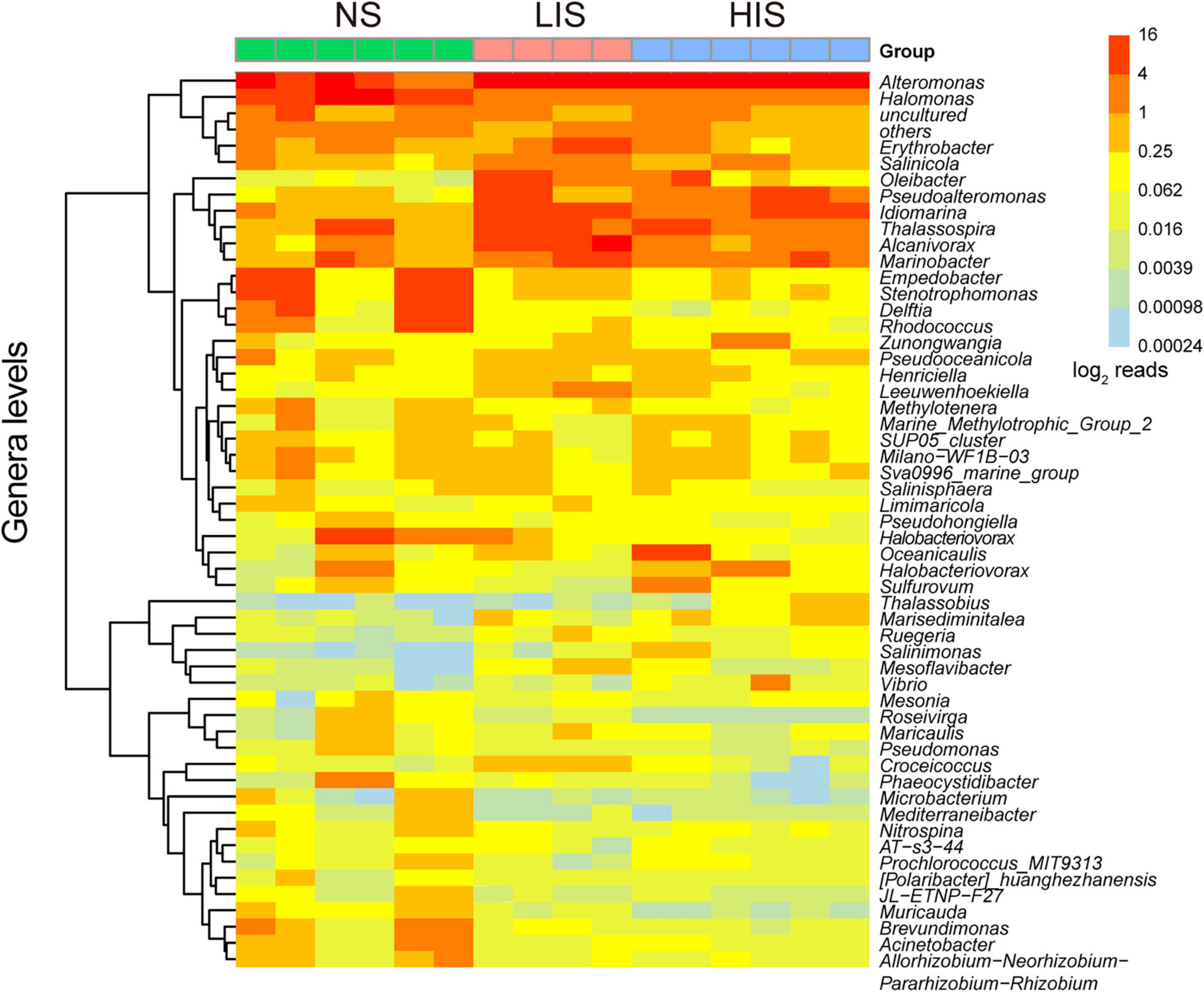
Figure 3. Heatmap based on the 52 most abundant genera at the three sites. The log2 value of the taxa read number is indicated by the color gradient from light blue (low) to red (high).
We also explored whether the alpha diversity of the bacterial community differed at seep sites with different seepage activities. Chao1 and ACE indices reveal community richness and estimate the OTU numbers in samples (O’Hara, 2005). Statistical analysis showed no significant differences among all the sites for the Chao1 index (p = 0.24) and ACE index (p = 0.19; Supplementary Figure 3). The Shannon and Simpson indices relate to community diversity (Schloss et al., 2011). Pairwise comparison showed that the Shannon index of the HIS site was different between the NS site (p = 0.0088), while the Simpson index was different between both the NS and LIS site (p = 0.0002; Supplementary Figure 3). Community diversity was lower when the Shannon index was smaller and the Simpson index was larger. Thus, the diversity of the HIS site was the lowest among the three sites.
Differences in Bacterial Community Structure at the Three Sites
To further explore the difference in bacterial community structure at the three sites, PCA based on Euclidean distance matrices for the genus-level compositional profiles of the three sites was performed. ANOSIM was used to assess the significance of the PCA grouping. All the sites were separated (R = 0.689, p = 0.001) along the PC1 direction (Supplementary Figure 4). A sequential order of the NS, LIS, and HIS associated with increased seepage activity on the PC1 axis can be observed, suggesting that a dynamic pattern of bacterial community compositions might be associated with CH4 seepage intensity. Separation was also observed in the PCoA clusters among the three sites (R2 = 0.57, p = 0.001; Supplementary Figure 5).
Next, we identified the most discriminative taxa responsible for the difference in the bacterial community at the three sampling sites by sPLS-DA. Similar to the PCA results, the grouping of all the three sites was separated from each other (Figure 4). Genera contributing to the community variance of these sites were extracted from two components. From component 1, Alteromonas best characterized the bacterial genus compositions at the LIS and HIS sites, separating them from the NS site. Alteromonas is a prevalent marine organism able to metabolize polycyclic aromatic hydrocarbons (Jin et al., 2011). For the second component, Marinobacterium and seep sulfate-reducing bacteria-2 (SEEP-SRB2) were the characteristic genera at the HIS site. In contrast, Methylophaga, Thalassolituus, Croceicoccus, Alcanivorax, Leeuwenhoekiella, Gramella, Haemophilus, and Sphingobacterium distinguished the LIS form HIS (Figure 4). Marinobacterium is a common aerobic hydrocarbon-degrading microorganism found in oil environment (Baek et al., 2018). SEEP-SRB2 is a sulfate-reducing partner in ANME consortia for anaerobic oxidation of CH4 (Kleindienst et al., 2012). Many Thalassolituus, Croceicoccus, and Alcanivorax species are oil degraders (Yakimov et al., 1998, 2004; Huang et al., 2015).
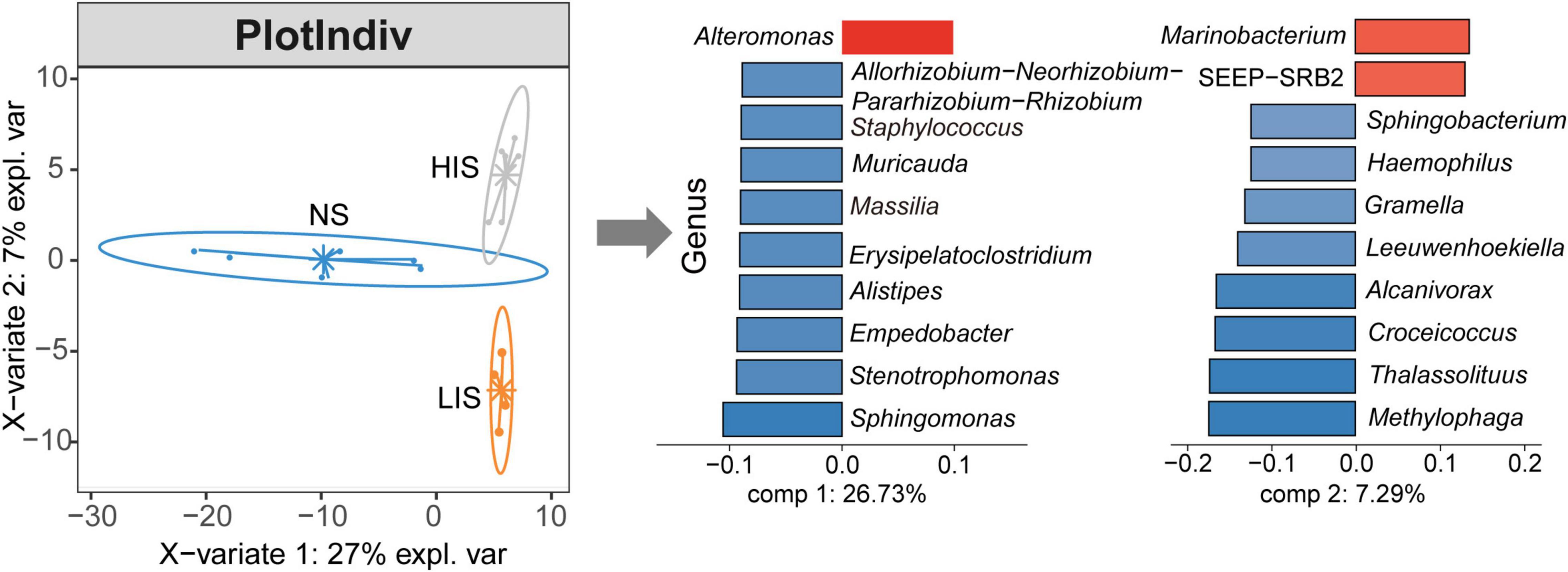
Figure 4. Sparse partial least squares-discriminant analysis (sPLS-DA) of the three study sites. From component 1, genera labeled in red discriminate the LIS and HIS sites from the NS site and genera labeled in blue are characteristic genera at the NS site. From component 2, genera contributed to the dissimilarity between the LIS and HIS sites are labeled with blue and red, respectively.
Environmental Variables Shaping Bacterial Community Structure
To explore the key environmental variables shaping the bacterial community composition, RDA in combination with the Monte Carlo permutation test was performed. Quantification of major geochemical parameters, including temperature, alkalinity, pH, CH4, DOC, nitrate, nitrite, ammonium, and phosphate, was employed and used for RDA. The Monte Carlo permutation tests indicated that CH4, DOC, nitrate, and ammonium had statistically significant (p < 0.05) contributions to explaining the variance in bacterial communities. These four variables together accounted for 76.99% of the explained variance. Importantly, CH4 was the most highly significant explanatory variable (p = 0.001) and the CH4 concentrations correlated strongly with the HIS site (Figure 5). Therefore, CH4 is the key environmental factor shaping the bacterial community structures at seep sites with various seepage activities.
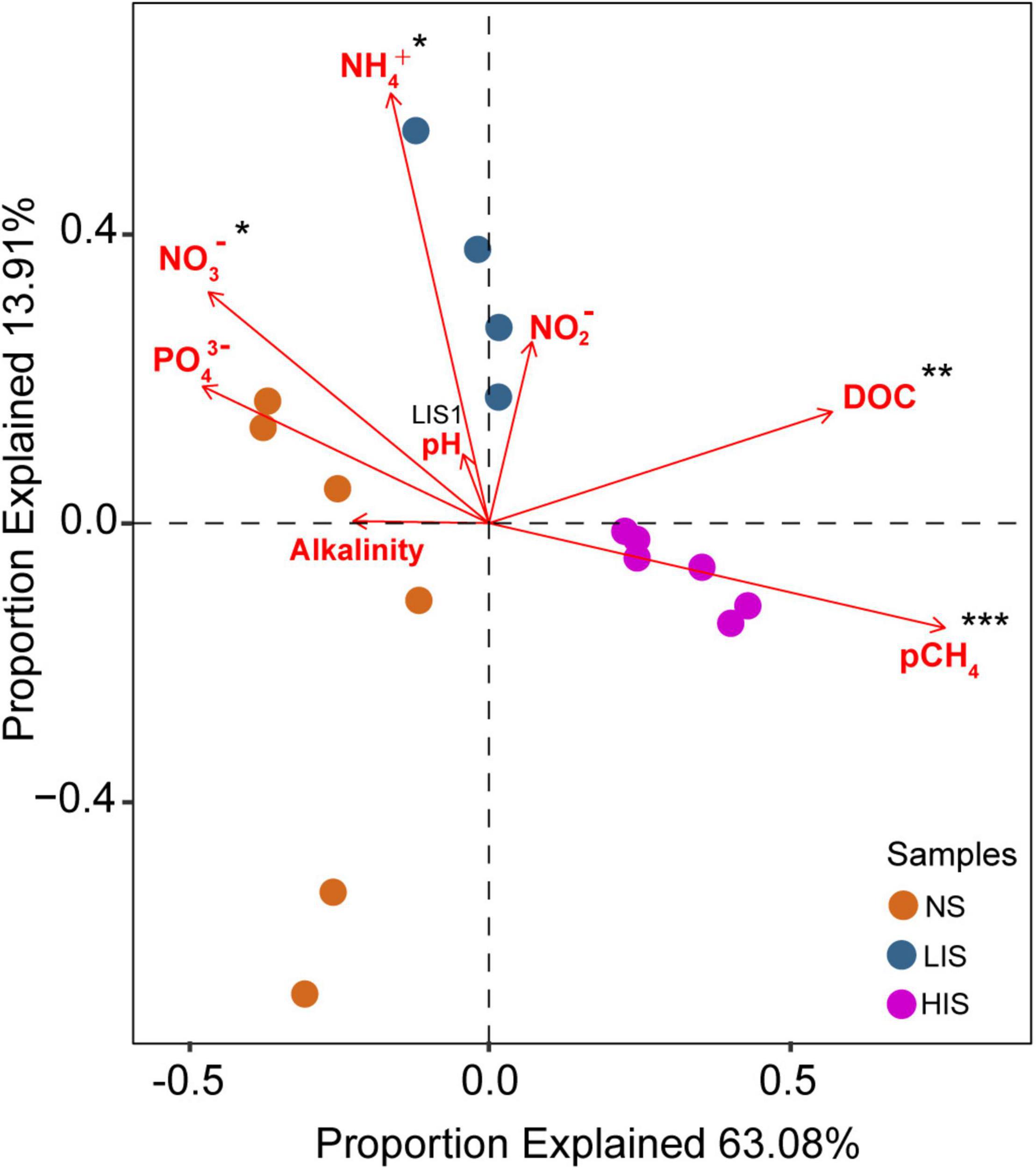
Figure 5. Redundancy analysis (RDA) correlating several environmental variables to bacterial communities. Circles represent the bacterial community of a given sample and red arrows indicate individual environmental parameters. The concentrations of methane (CH4) used for this analysis were 0.27, 99.4, and 2,207.9 μM at the NS, LIS, and HIS sites, respectively. Significance levels (marked by asterisks) were evaluated based on the Monte Carlo permutation test. ***p < 0.001; **p < 0.01; *p < 0.05.
Enriched Taxa of Study Sites With Different Seepage Activities
We applied LEfSe analysis to further determine whether specific individual bacterial taxa were differentially enriched at these sites and the results demonstrated that significantly different dominant taxa could be observed at cold seeps. Significant enrichment of genus Alcanivorax, Idiomarina, Thalassospira, Oleibacter, Marinobacter, Thalassolituus, Ruegeria, Leeuwenhoekiella, Croceicoccus, and Mesoflavibacter was identified at the LIS site, while a totally different combination of taxa, including Alteromonas, Pseudoalteromonas, Vibrio, Marinobacterium, Sulfurovum, Salinimonas, Aestuariibacter, and Thalassobius, was dominant at the HIS site (Supplementary Figure 6). Many species of the most of above genera are reported to be oil degraders, leading to the assumption of the presence of oil degraders at the two seep sites. We further confirmed this by phylogenetic analysis. We found close phylogenetic relationships appeared among known oil-degrading species with the OTUs enriched at two seepage sites belong to the genera Alcanivorax, Idiomarina, Thalassospira, Oleibacter, Marinobacter, Thalassolituus, Croceicoccus, Mesoflavibacter, Alteromonas, Pseudoalteromonas, Vibrio, Marinobacterium, Aestuariibacter, and Thalassobius. In contrast, only OTUs of four genera (Halomonas, Brevundimonas, Roseivirga, and Pseudomonas) of the 13 differentially abundant genera found at the NS site were phylogenetically closely related to oil degraders (Supplementary Figure 7). These results indicate that many putative oil degraders were enriched at the two seepage sites and natural oil seepage may occur there.
Microbial Co-occurrence Network at the Study Sites
Because bacterial communities vary from site to site, it is essential to investigate coordinated interactions of the microorganisms colonizing each CH4 seepage site. We constructed co-occurrence networks of the microorganisms by calculating pairwise intergenus correlations based on the genus-abundance profiles for every site. Microbial co-occurrence patterns were distinct at the study sites and we calculated connections (edges) between nodes under three different Bray–Curtis distance matrices. The smaller value of Bray–Curtis distance matrices is, the stronger the co-occurrence correlation is. We found that the HIS site networks contained fewest connections between nodes under all the Bray–Curtis distance matrices. Therefore, the strength of microbial co-occurrence was weakest at the HIS site (Figure 6).
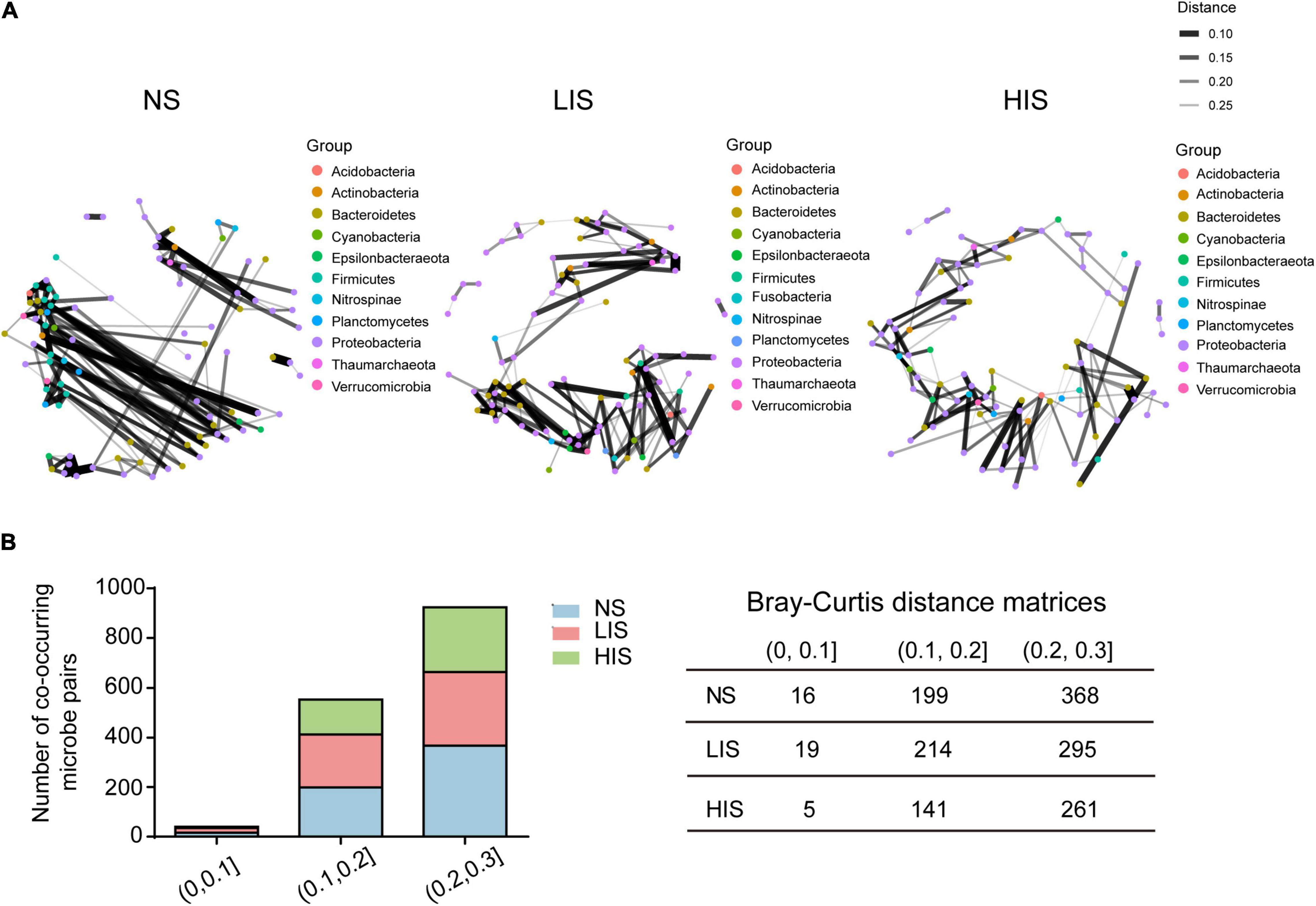
Figure 6. Microbial co-occurrence network of the three study sites. (A) Co-occurrence networks were constructed based on Bray–Curtis distance matrices (for values less than 0.3) based on the relative abundance profiles of genera among the three sites. The smaller the distance is, the stronger the co-occurrence correlation is. Each node represents a genus; genera belonging to the same phylum are shown in the same color. (B) Summary of co-occurring microbe pairs at the three sites under different Bray–Curtis distance matrices.
Archaeal Community Structure at the Three Study Sites
We also analyzed archaeal taxonomic compositions at the three sites. On average, we detected 147 OTUs in the archaeal community at the three study sites; the bacterial community contained 647 OTUs, indicating that the archaeal community structure is much simpler than the bacterial community structure. Thaumarchaeota was the most abundant phylum (average 85.7%) among all the sites followed by Euryarchaeota and Nanoarchaeaeota. The relative abundance of these phyla at the three sites was similar, except for Thaumarchaeota, the abundance of which was slightly lower at the HIS site than at the NS site (Supplementary Figure 8 and Supplementary Table 4). Circos analysis was used to visualize the corresponding abundance relationships between samples and archaeal communities, showing similar relative proportions of the 15 most abundant OTUs among the three sites (Supplementary Figure 9A and Supplementary Table 5). As shown in the genus heatmap, the distribution patterns of all the genera identified were also similar among the three sites (Supplementary Figure 9B and Supplementary Table 4).
We performed further analysis of the beta diversity of the archaeal community. Clusters of three sites presented overlaps in the PCoA plot (R2 = 0.18, p = 0.11; Supplementary Figure 10A). The PCA plot yielded similar clustering patterns with no significant separation among the three sites except for the NS and HIS sites (Supplementary Figure 10B). RDA showed that the archaeal community structure was not significantly associated with any environmental factors examined (p > 0.062; Supplementary Figure 11). Overall, the results confirmed that archaea exhibit a homogeneous distribution at different sites, indicating that they participate in general geochemical processes rather than in CH4 metabolism in the bottom water of cold seep.
Discussion
We found apart from CH4, which acts as the key environmental variable shaping the bacterial community structure, DOC, nitrate, and ammonium concentrations also correlated statistically significantly with bacterial community abundances. Because CH4 oxidation by autotrophic methanotrophs leads to DOC production (Yang et al., 2020), the effect of DOC on shaping the bacterial community may be caused by CH4 variation. Moreover, as many oil-degrading bacteria are enriched at the LIS and HIS sites, there is also the possibility of oil seepage at these sites with DOC produced through oil biodegradation by these bacteria (Orcutt et al., 2010). We observed highest concentrations of all the inorganic nutrients at the LIS site, which possibly sourced from deep fluids associated with CH4 seepage (D’Souza et al., 2016). Therefore, inorganic nutrients shape the bacterial community probably due to seepage activity at the seep sites.
At the cold seeps, CH4 oxidation likely involves communities composed of different functional guilds rather than methanotrophs alone (Dubinsky et al., 2013; Rivers et al., 2013). CH4 levels at the cold CH4 seeps vary intermittently from nanomolar to millimolar concentrations (Reeburgh, 2007; Ruff et al., 2013; Grupe et al., 2015). The concentration of oxygen in the bottom water will change concomitantly as CH4 fluctuates (Li et al., 2021) and oxygen availability can determine the composition of the microbial communities involved in CH4 oxidation (Hernandez et al., 2015). We observed distinct microbial co-occurrence patterns at the study sites with the strength of microbial correlations being weakest at the HIS site. When the CH4 concentration changes at the HIS site, the species will change and bacteria no longer present in correlation with each other; thus, the synergistic interactions between these organisms will be disrupted and results in the alteration of the topology of the co-occurrence network during CH4 fluctuations (Shi et al., 2015). In contrast, at background site and the LIS where the CH4 concentration did not fluctuate strongly, the strength of microbial co-occurrence was significantly greater.
Because methylotrophic bacteria may be enriched for CH4 consumption in CH4 seep sites, we compared canonical methanotrophs at the three sites. We found Methyloprofundus at the HIS site but not at the NS and LIS sites, while the numbers of marine methylotrophic group 2 (MMG2) and Methylotenera were similar at the three sites (Supplementary Figure 12). Members of the genus Methyloprofundus are most typically detected in sediments and within bacteriocytes of seep-associated mussels in CH4 seeps, occasionally being found in the deep-water column (Tavormina et al., 2015). This finding indicates that Methyloprofundus may be the methanotroph responsible for CH4 oxidation at the HIS site. However, the proportion of Methyloprofundus was relatively low at the HIS site (<0.1% of total bacterial reads). As reported by Zhang et al. (2020), methanotrophs were observed in the water of the Haima seeps but in low proportions. There is a possibility that CH4 may be oxidized by unrecognized methanotrophs or that it may escape the CH4 biofilter and flux to the atmosphere directly without oxidation by bacteria. Typical anaerobic cold-seep microbial groups, including methanotrophic archaea ANME-1 and ANME-2 and sulfate-reducing Desulfobulbaceae, Desulfobacteraceae, Desulfuromonadaceae, and Desulfarculaceae, were detected in extremely low proportions in our research. Similar observations were obtained in the study of Zhang et al. (2020). However, ANME-2 was found to live above a depth of approximately 600 m in the water column of the Black Sea and ANME-1 inhabited depths below 600 m; samples obtained below 600 m water depth were considered to be located in the fully anoxic water column (oxygen concentration < 1.5 μM; Schubert et al., 2006). In this study, the oxygen concentrations of bottom waters were approximately 103–109 μM (Feng et al., in preparation4). Therefore, the bottom water in the Haima seeps maintains a relatively oxic habitat that is suitable for aerobic methylotrophic bacteria for CH4 oxidization.
Natural oil seepages have been reported in cold seeps of the Gulf of Mexico. These seepages influence the structure of the bacterial community and the abundances of many oil degraders are enhanced in oiled sediments (Orcutt et al., 2010). Several studies have demonstrated that marine obligate hydrocarbonoclastic bacteria (MOHCB) are the main actors in the degradation of hydrocarbons after oil exposure (Sanni et al., 2015). We observed many bacteria enriched at the LIS and HIS sites were putative oil degraders. Phylogenetic analysis demonstrated close phylogenetic relationship among known oil-degrading species with the OTUs enriched at the two seepage sites except for those belong to the genera Ruegeria, Leeuwenhoekiella, Sulfurovum, and Salinimonas (Yoon et al., 2012; Arahal et al., 2018; Sun et al., 2020; Tahon et al., 2020). Mesoflavibacter belongs to Bacteroidia (Okai et al., 2015), whereas Thalassospira, Croceicoccus, and Thalassobius are alphaproteobacteria (Arahal et al., 2005; Kodama et al., 2008; Huang et al., 2015); all these four genera degrade aromatic hydrocarbons. Idiomarina, Marinobacter, Alcanivorax, Oleibacter, Thalassolituus, Aestuariibacter, Alteromonas, Marinobacterium, Pseudoalteromonas, and Vibrio are gammaproteobacteria genera that consume crude oil or alkanes (Yakimov et al., 1998, 2004; Hedlund and Staley, 2001; Iwabuchi et al., 2002; Pham et al., 2009; Wang et al., 2010, 2014; Kostka et al., 2011; Teramoto et al., 2011; Raddadi et al., 2017). Enrichment of these class was also observed by a study of the deep water horizon oil spill that occurred in the Gulf of Mexico in April 2010 with the major responders to oil intrusion in marine waters being members of gammaproteobacteria, alphaproteobacteria, and bacteroidia (Redmond and Valentine, 2012; Liu and Liu, 2013). In contrast, OTUs of only four genera (Halomonas, Brevundimonas, Roseivirga, and Pseudomonas) are capable of oil degradation at the NS site (Le Petit et al., 1975; Chaineau et al., 1999; Wang et al., 2007; Selvaratnam et al., 2016). Therefore, the observation of these putative oil-degrading bacteria in this study suggested that oil seepage may occur at cold seeps in the SCS.
We noted that oil-degrading bacteria were dominant at both the LIS and HIS sites with different seepage activities. Zhang et al. and Guan et al. studied two seep sites with different seepage intensities and showed that heterotrophic prokaryotes and UCMs were found at both the sites. These results suggest that oil may seep regardless of CH4 seepage activity. Members of different genera occupy distinct trophic niches with diverse oil components because different MOHCB utilize different hydrocarbons; for example, Alcanivorax degrades aliphatic hydrocarbons, whereas Cycloclasticus degrades (poly-)aromatic hydrocarbons (Head et al., 2006). We noticed that the LIS and HIS sites harbored divergent hydrocarbon degraders, probably due to differences in the oil sources and components in these two seep habitats.
In addition to microbes that participate in the carbon cycle, we noted enrichment of bacteria involved in the sulfur and nitrogen cycles at both the seep sites. It has been reported that oil exposure in deep-sea sediment can also alter other metabolic pathways such as nitrogen cycling (Mason et al., 2014). It should be noted that the abundance of the denitrifier Ruegeria increased markedly at the LIS site (Supplementary Figure 6). Additionally, the thiosulfate/sulfite oxidizer Sulfurovum was dominant at the HIS site (Supplementary Figure 6), probably due to seepage of hydrogen sulfide from the sediment resulted from the sulfate-driven anaerobic oxidation of CH4 (Lu et al., 2021).
Conclusion
In this study, bottom-water microbes were sampled from the NS site, LIS site, and HIS site in Haima, SCS. Our results showed that the three study sites harbor significantly divergent bacterial compositions and that the structures correlate significantly with the CH4 concentration. The strength of the microbial correlations was weakest at the HIS site. In contrast, the archaeal community compositions were similar among the three sites and exhibited no correlation with CH4 concentration. Diverse putative oil-degrading bacteria were enriched at the two seep sites, adding new evidence that oil seepage may occur at cold seeps in the SCS. This study represents a comprehensive comparison of microbial profiles in the water column of cold seeps in the SCS, revealing that the seepage intensity has a strong impact on bacterial community dynamics.
Data Availability Statement
The datasets presented in this study can be found in online repositories. The names of the repository/repositories and accession number(s) can be found below: NCBI [accession: PRJNA715035].
Author Contributions
YL and NL conceived and designed the research and wrote the manuscript. XL and ZD performed the experiments and analyzed the data. PD, JF, and JT provided the samples and associated the metadata. DC provided critical technical and scientific discussion. All authors agree with the final version of the manuscript.
Funding
The funding was provided by the Program of Guangdong Basic and Applied Research (Grant: 2019B030302004), the National Key R&D Program of China (2018YFC0310004), the National Natural Science Foundation of China (NSFC, 31900886, 41706053, and 41976061), the Guangzhou Basic and Applied Basic Research Foundation (202102020550), the Medical Research Foundation of Guangdong Province (A2021382), the Key Special Project for Introduced Talents Team of Southern Marine Science and Engineering Guangdong Laboratory (Guangzhou) (GML2019ZD0104), and the cold seep ecosystem research device early stage research project.
Conflict of Interest
The authors declare that the research was conducted in the absence of any commercial or financial relationships that could be construed as a potential conflict of interest.
Publisher’s Note
All claims expressed in this article are solely those of the authors and do not necessarily represent those of their affiliated organizations, or those of the publisher, the editors and the reviewers. Any product that may be evaluated in this article, or claim that may be made by its manufacturer, is not guaranteed or endorsed by the publisher.
Acknowledgments
We are grateful to the crew of the RV Haiyang-6 and ROV Haima team for their assistance in collecting the samples.
Supplementary Material
The Supplementary Material for this article can be found online at: https://www.frontiersin.org/articles/10.3389/fmars.2021.753952/full#supplementary-material
Footnotes
- ^ http://www.gb688.cn/bzgk/gb/newGbInfo?hcno=D7C5F44155DBE2C0BC40EA764A6BBF4A
- ^ http://www.drive5.com/usearch/
- ^ http://huttenhower.sph.harvard.edu/galaxy/
- ^ Feng, J. X. (in preparation). Characteristics of dissolved organic matter in the Haima Cold seep in South China Sea. Mar. Petrol Geol.
References
Anderson, M. J. (2001). A new method for non-parametric multivariate analysis of variance. Austral Ecol. 26, 32–46. doi: 10.1111/j.1442-9993.2001.01070.pp.x
Arahal, D. R., Lucena, T., Rodrigo-Torres, L., and Pujalte, M. J. (2018). Ruegeriadenitrificansspnov., a marine bacterium in the family Rhodobacteraceae with the potential ability for cyanophycin synthesis. Int. J. SystEvol.Micr. 68, 2515–2522. doi: 10.1099/ijsem.0.002867
Arahal, D. R., Macian, M. C., Garay, E., and Pujalte, M. J. (2005). Thalassobiusmediterraneus gen. nov., sp. nov., and reclassification of Ruegeriagelatinovorans as Thalassobiusgelatinovorus comb. nov. Int. J. Syst. Evol. Microbiol. 55, 2371–2376. doi: 10.1099/ijs.0.63842-0
Baek, K., Bae, S. S., Jung, J., and Chung, D. (2018). Complete genome sequence of marinobacteriumaestuarii ST58-10(T), a benzene-degrading bacterium isolated from estuarine sediment. Microbiol. Resource Announcements 7:e00971-18. doi: 10.1128/MRA.00971-18
Birgel, D., Feng, D., Roberts, H. H., and Peckmann, J. (2011). Changing redox conditions at cold seeps as revealed by authigenic carbonates from Alaminos Canyon, northern Gulf of Mexico. Chem Geol. 285, 82–96. doi: 10.1016/j.chemgeo.2011.03.004
Boetius, A., and Wenzhofer, F. (2013). Seafloor oxygen consumption fuelled by methane from cold seeps. Nat. Geosci. 6, 725–734. doi: 10.1038/NGEO1926
Camacho, C., Coulouris, G., Avagyan, V., Ma, N., Papadopoulos, J., Bealer, K., et al. (2009). BLAST+: architecture and applications. BMC Bioinformatics 10:421. doi: 10.1186/1471-2105-10-421
Caporaso, J. G., Kuczynski, J., Stombaugh, J., Bittinger, K., Bushman, F. D., Costello, E. K., et al. (2010). QIIME allows analysis of high-throughput community sequencing data. Nat. Methods 7, 335–336. doi: 10.1038/nmeth.f.303
Chaineau, C. H., Morel, J., Dupont, J., Bury, E., and Oudot, J. (1999). Comparison of the fuel oil biodegradation potential of hydrocarbon-assimilating microorganisms isolated from a temperate agricultural soil. Sci. Total Environ. 227, 237–247. doi: 10.1016/s0048-9697(99)00033-9
Chen, S. F., Zhou, Y. Q., Chen, Y. R., and Gu, J. (2018). fastp: an ultra-fast all-in-one FASTQ preprocessor. Bioinformatics 34, 884–890. doi: 10.1093/bioinformatics/bty560
Clarke, K. R. (1993). Nonparametric multivariate analyses of changes in community structure. Austral Ecol. 18, 117–143. doi: 10.1111/j.1442-9993.1993.tb00438.x
Csardi, G., and Nepusz, T. (2006). The igraph software package for complex network research. Complex Syst. 1695, 1–9.
Cui, H. P., Su, X., Chen, F., Holland, M., Yang, S. X., Liang, J. Q., et al. (2019). Microbial diversity of two cold seep systems in gas hydrate-bearing sediments in the South China Sea. Mar. Environ. Res. 144, 230–239. doi: 10.1016/j.marenvres.2019.01.009
Cui, H. P., Su, X., Liang, J. Q., Chen, F., Holland, M., Yang, S. X., et al. (2020). Microbial diversity in fracture and pore filling gas hydrate-bearing sediments at Site GMGS2-16 in the Pearl River Mouth Basin, the South China Sea. Mar. Geol. 427:106264. doi: 10.1016/J.Margeo.2020.106264
D’Souza, N. A., Subramaniam, A., Juhl, A. R., Hafez, M., Chekalyuk, A., Phan, S., et al. (2016). Elevated surface chlorophyll associated with natural oil seeps in the Gulf of Mexico. Nat. Geosci. 9, 215–218. doi: 10.1038/NGEO2631
Dubinsky, E. A., Conrad, M. E., Chakraborty, R., Bill, M., Borglin, S. E., Hollibaugh, J. T., et al. (2013). Succession of hydrocarbon-degrading bacteria in the aftermath of the deepwater horizon oil spill in the gulf of Mexico. Environ. Sci. Technol. 47, 10860–10867. doi: 10.1021/es401676y
Edgar, R. C. (2010). Search and clustering orders of magnitude faster than BLAST. Bioinformatics 26, 2460–2461. doi: 10.1093/bioinformatics/btq461
Edgar, R. C. (2013). UPARSE: highly accurate OTU sequences from microbial amplicon reads. Nat. Methods 10, 996–998. doi: 10.1038/nmeth.2604
Feng, D., Birgel, D., Peckmann, J., Roberts, H. H., Joye, S. B., Sassen, R., et al. (2014). Time integrated variation of sources of fluids and seepage dynamics archived in authigenic carbonates from Gulf of Mexico Gas Hydrate Seafloor Observatory. Chem Geol. 385, 129–139. doi: 10.1016/j.chemgeo.2014.07.020
Feng, D., Cheng, M., Kiel, S., Qiu, J. W., Yang, Q. H., Zhou, H. Y., et al. (2015). Using Bathymodiolus tissue stable carbon, nitrogen and sulfur isotopes to infer biogeochemical process at a cold seep in the South China Sea. Deep-Sea Res Pt I 104, 52–59. doi: 10.1016/j.dsr.2015.06.011
Galimov, E. M. (1988). Sources and mechanisms of formation of gaseous hydrocarbons in sedimentary rocks. Chem. Geol. 71, 77–95. doi: 10.1016/0009-2541(88)90107-6
Grupe, B. M., Krach, M. L., Pasulka, A. L., Maloney, J. M., Levin, L. A., and Frieder, C. A. (2015). Methane seep ecosystem functions and services from a recently discovered southern California seep. Mar. Ecol-EvolPersp. 36, 91–108. doi: 10.1111/maec.12243
Guan, et al. (2021). Lipid biomarker patterns reflect seepage activity and variable geochemical processes in sediments from the Haima cold seeps, South China Sea. Palaeogeography Palaeoclimatol. Palaeoecol. (accepted).
Guan, H. X., Birgel, D., Peckmann, J., Liang, Q. Y., Feng, D., Yang, S. X., et al. (2018). Lipid biomarker patterns of authigenic carbonates reveal fluid composition and seepage intensity at Haima cold seeps. South China Sea. J. Asian Earth Sci. 168, 163–172. doi: 10.1016/j.jseaes.2018.04.035
Head, I. M., Jones, D. M., and Roling, W. F. M. (2006). Marine microorganisms make a meal of oil. Nat. Rev. Microbiol. 4, 173–182. doi: 10.1038/nrmicro1348
Hedlund, B. P., and Staley, J. T. (2001). Vibrio cyclotrophicus sp. nov., a polycyclic aromatic hydrocarbon (PAH)-degrading marine bacterium. Int. J. Syst. Evol. Microbiol. 51, 61–66. doi: 10.1099/00207713-51-1-61
Hernandez, M. E., Beck, D. A. C., Lidstrom, M. E., and Chistoserdova, L. (2015). Oxygen availability is a major factor in determining the composition of microbial communities involved in methane oxidation. PeerJ 3:e801. doi: 10.7717/peerj.801
Hotelling, H. (1933). Analysis of a complex of statistical variables into principal components. J. Educ. Psychol. 24, 417–441. doi: 10.1037/h0071325
Huang, B. J., Tiam, H., Li, X. S., Wang, Z. F., and Xiao, X. M. (2016). Geochemistry, origin and accu.mulation of natural gases in the deepwater area of the Qiongdongnan Basin, South China Sea. Mar. Petrol Geol. 72, 254–267. doi: 10.1016/j.marpetgeo.2016.02.007
Huang, Y. L., Zeng, Y. H., Feng, H., Wu, Y. H., and Xu, X. W. (2015). Croceicoccusnaphthovoransspnov., a polycyclic aromatic hydrocarbons-degrading and acylhomoserine-lactone-producing bacterium isolated from marine biofilm, and emended description of the genus Croceicoccus. Int. J. Syst. Evol. Micr. 65, 1531–1536. doi: 10.1099/ijs.0.000132
Iwabuchi, N., Sunairi, M., Urai, M., Itoh, C., Anzai, H., Nakajima, M., et al. (2002). Extracellular polysaccharides of Rhodococcusrhodochrous S-2 stimulate the degradation of aromatic components in crude oil by indigenous marine bacteria. Appl. Environ. Microbiol. 68, 2337–2343. doi: 10.1128/aem.68.5.2337-2343.2002
Jin, H. M., Jeong, H., Moon, E. J., Math, R. K., Lee, K., Kim, H. J., et al. (2011). Complete genome sequence of the polycyclic aromatic hydrocarbon-degrading bacterium alteromonassp strain SN2. J. Bacteriol. 193, 4292–4293. doi: 10.1128/Jb.05252-11
Joye, S. B. (2020). The geology and biogeochemistry of hydrocarbon seeps. Annu. Rev. Earth Pl Sc. 48, 205–231. doi: 10.1146/annurev-earth-063016-020052
Kimura, M. (1980). A simple method for estimating evolutionary rate of base substitutions through comparative studies of nucleotide sequences. J. Mol. Evol. 16, 111–120.
Kleindienst, S., Ramette, A., Amann, R., and Knittel, K. (2012). Distribution and in situ abundance of sulfate-reducing bacteria in diverse marine hydrocarbon seep sediments. Environ. Microbiol. 14, 2689–2710. doi: 10.1111/j.1462-2920.2012.02832.x
Kodama, Y., Stiknowati, L. I., Ueki, A., Ueki, K., and Watanabe, K. (2008). Thalassospiratepidiphilaspnov., a polycyclic aromatic hydrocarbon-degrading bacterium isolated from seawater. Int. J. Syst. Evol. Micr. 58, 711–715. doi: 10.1099/ijs.0.65476-0
Kostka, J. E., Prakash, O., Overholt, W. A., Green, S. J., Freyer, G., Canion, A., et al. (2011). Hydrocarbon-degrading bacteria and the bacterial community response in gulf of Mexico beach sands impacted by the deepwater horizon oil spill. Appl. Environ. Microbiol. 77, 7962–7974. doi: 10.1128/AEM.05402-11
Kumar, S., Stecher, G., and Tamura, K. (2016). MEGA7: molecular evolutionary genetics analysis version 7.0 for bigger datasets. Mol. Biol. Evol. 33, 1870–1874. doi: 10.1093/molbev/msw054
Lai, X., Cao, L., Tan, H., Fang, S., Huang, Y., and Zhou, S. (2007). Fungal communities from methane hydrate-bearing deep-sea marine sediments in South China Sea. ISME J. 1, 756–762. doi: 10.1038/ismej.2007.51
Le Petit, J., Bertrand, J. C., N’Guyen, M. H., and Tagger, S. (1975). [On the taxonomy and physiology ofbacteria utilizing hydrocarbons in the sea (author’s transl)]. Ann. Microbiol. 126, 367–380.
Li, H. Z., Yang, Q. H., and Zhou, H. Y. (2020). Niche differentiation of sulfate- and iron-dependent anaerobic methane oxidation and methylotrophicmethanogenesis in deep Sea Methane Seeps. Front. Microbiol. 11:1409. doi: 10.3389/Fmicb.2020.01409
Li, N., Yang, X. Q., Peckmann, J., Zhou, Y., Wang, H. B., Chen, D. F., et al. (2021). Persistent oxygen depletion of bottom waters caused by methane seepage: evidence from the South China Sea. Ore Geol. Rev. 129:103949. doi: 10.1016/j.oregeorev.2020.103949
Liang, Q. Y., Hu, Y., Feng, D., Peckmann, J., Chen, L. Y., Yang, S. X., et al. (2017). Authigenic carbonates from newly discovered active cold seeps on the northwestern slope of the South China Sea: constraints on fluid sources, formation environments, and seepage dynamics. Deep-Sea Res Pt I 124, 31–41. doi: 10.1016/j.dsr.2017.04.015
Liu, Z. F., and Liu, J. Q. (2013). Evaluating bacterial community structures in oil collected from the sea surface and sediment in the northern Gulf of Mexico after the deepwater horizon oil spill (vol 2, pg 492, 2013). Microbiologyopen 2, 715–715. doi: 10.1002/mbo3.117
Lu, R., Gao, Z. M., Li, W. L., Wei, Z. F., Wei, T. S., Huang, J. M., et al. (2021). Asgardarchaea in the haimacold seep: spatial distribution and genomic insights. Deep-Sea Res Pt I 170:103489. doi: 10.1016/J.Dsr.2021.103489
Martin, M. (2011). Cutadapt removes adapter sequences from high-throughput sequencing reads. EMBNET J. 17, 10–12. doi: 10.14806/ej.17.1.200
Mason, O. U., Scott, N. M., Gonzalez, A., Robbins-Pianka, A., Baelum, J., Kimbrel, J., et al. (2014). Metagenomics reveals sediment microbial community response to Deepwater Horizon oil spill. ISME J. 8, 1464–1475. doi: 10.1038/ismej.2013.254
Niu, M. Y., Liang, W. Y., and Wang, F. P. (2018). Methane biotransformation in the ocean and its effects on climate change: a review. Sci. China Earth Sci. 61, 1697–1713. doi: 10.1007/s11430-017-9299-4
Niu, M. Y., Fan, X. B., Zhuang, G. C., Liang, Q. Y., and Wang, F. P. (2017). Methane-metabolizing microbial communities in sediments of the Haimacold seep area, northwest slope of the South China Sea. FEMS Microbiol. Ecol. 93. doi: 10.1093/femsec/fix101
O’Hara, R. B. (2005). Species richness estimators: how many species can dance on the head of a pin? J. Anim. Ecol. 74, 375–386. doi: 10.1111/j.1365-2656.2005.00940.x
Okai, M., Kihara, I., Yokoyama, Y., Ishida, M., and Urano, N. (2015). Isolation and characterization of benzo[a] pyrene-degrading bacteria from the Tokyo Bay area and Tama River in Japan. FEMS Microbiol. Lett. 362:fnv143. doi: 10.1093/femsle/fnv143
Orcutt, B. N., Joye, S. B., Kleindienst, S., Knittel, K., Ramette, A., Reitz, A., et al. (2010). Impact of natural oil and higher hydrocarbons on microbial diversity, distribution, and activity in Gulf of Mexico cold-seep sediments. Deep-Sea Res Pt I 57, 2008–2021. doi: 10.1016/j.dsr2.2010.05.014
Pasulka, A. L., Levin, L. A., Steele, J. A., Case, D. H., Landry, M. R., and Orphan, V. J. (2016). Microbial eukaryotic distributions and diversity patterns in a deep-sea methane seep ecosystem. Environ. Microbiol. 18, 3022–3043. doi: 10.1111/1462-2920.13185
Pham, V. D., Hnatow, L. L., Zhang, S., Fallon, R. D., Jackson, S. C., Tomb, J. F., et al. (2009). Characterizing microbial diversity in production water from an Alaskan mesothermic petroleum reservoir with two independent molecular methods. Environ. Microbiol. 11, 176–187. doi: 10.1111/j.1462-2920.2008.01751.x
Quast, C., Pruesse, E., Yilmaz, P., Gerken, J., Schweer, T., Yarza, P., et al. (2013). The SILVA ribosomal RNA gene database project: improved data processing and web-based tools. Nucleic Acids Res. 41, D590–D596. doi: 10.1093/nar/gks1219
Raddadi, N., Giacomucci, L., Totaro, G., and Fava, F. (2017). Marinobactersp from marine sediments produce highly stable surface-active agents for combatting marine oil spills. Microb Cell Fact. 16:186. doi: 10.1186/S12934-017-0797-3
Redmond, M. C., and Valentine, D. L. (2012). Natural gas and temperature structured a microbial community response to the Deepwater Horizon oil spill. Proc. Natl. Acad. Sci U S A. 109, 20292–20297. doi: 10.1073/pnas.1108756108
Reeburgh, W. S. (2007). Oceanic methane biogeochemistry. Chem. Rev. 107, 486–513. doi: 10.1021/cr050362v
Rivers, A. R., Sharma, S., Tringe, S. G., Martin, J., Joye, S. B., and Moran, M. A. (2013). Transcriptional response of bathypelagic marine bacterioplankton to the Deepwater Horizon oil spill. ISME J. 7, 2315–2329. doi: 10.1038/ismej.2013.129
Rohart, F., Gautier, B., Singh, A., and Lê Cao, K.-A. (2017). mixOmics: an R package for ‘omics feature selection and multiple data integration. PLoS Comp. Biol. 13:e1005752. doi: 10.1371/journal.pcbi.1005752
Ruff, S. E., Arnds, J., Knittel, K., Amann, R., Wegener, G., Ramette, A., et al. (2013). Microbial communities of deep-sea methane seeps at hikurangi continental margin (New Zealand). PLoS One 8:e72627. doi: 10.1371/journal.pone.0072627
Sanni, G. O., Coulon, F., and McGenity, T. J. (2015). Dynamics and distribution of bacterial and archaeal communities in oil-contaminated temperate coastal mudflat mesocosms. Environ. Sci. Pollut. R. 22, 15230–15247. doi: 10.1007/s11356-015-4313-1
Schloss, P. D., Gevers, D., and Westcott, S. L. (2011). Reducing the effects of PCR amplification and sequencing artifacts on 16S rRNA-Based studies. PLoS One 6:e27310. doi: 10.1371/journal.pone.0027310
Schubert, C. J., Coolen, M. J. L., Neretin, L. N., Schippers, A., Abbas, B., Durisch-Kaiser, E., et al. (2006). Aerobic and anaerobic methanotrophs in the Black Sea water column. Environ. Microbiol. 8, 1844–1856. doi: 10.1111/j.1462-2920.2006.01079.x
Segata, N., Izard, J., Waldron, L., Gevers, D., Miropolsky, L., Garrett, W. S., et al. (2011). Metagenomic biomarker discovery and explanation. Genome Biol. 12:R60. doi: 10.1186/Gb-2011-12-6-R60
Selvaratnam, C., Thevarajoo, S., Ee, R., Chan, K. G., Bennett, J. P., Goh, K. M., et al. (2016). Genome sequence of Roseivirga sp. strain D-25 and its potential applications from the genomic aspect. Mar. Genom. 28, 29–31. doi: 10.1016/j.margen.2016.04.004
Shi, B. C., Chang, M., Martin, J., Mitreva, M., Lux, R., Klokkevold, P., et al. (2015). Dynamic changes in the subgingivalmicrobiome and their potential for diagnosis and prognosis of periodontitis. MBIO 6:e01926-14. doi: 10.1128/mBio.01926-14
Sun, J., Zhang, Y., Xu, T., Zhang, Y., Mu, H. W., Zhang, Y. J., et al. (2017). Adaptation to deep-sea chemosynthetic environments as revealed by mussel genomes. Nat. Ecol. Evol. 1:0121. doi: 10.1038/S41559-017-0121
Sun, Q. L., Zhang, J., Wang, M. X., Cao, L., Du, Z. F., Sun, Y. Y., et al. (2020). High-Throughput sequencing reveals a potentially novel sulfurovum species dominating the microbial communities of the seawater-sediment interface of a deep-sea cold seep in South China Sea. Microorganisms 8:687. doi: 10.3390/microorganisms8050687
Tahon, G., Lebbe, L., De Troch, M., Sabbe, K., and Willems, A. (2020). Leeuwenhoekiellaaestuarii sp. nov., isolated from salt-water sediment and first insights in the genomes of Leeuwenhoekiella species. Int. J. Syst. Evol. Micr. 70, 1706–1719. doi: 10.1099/ijsem.0.003959
Tavormina, P. L., Hatzenpichler, R., McGlynn, S., Chadwick, G., Dawson, K. S., Connon, S. A., et al. (2015). Methyloprofundussedimenti gen. nov., spnov., an obligate methanotroph from ocean sediment belonging to the ‘deep sea-1’ clade of marine methanotrophs. Int. J. Syst. Evol. Micr. 65, 251–259. doi: 10.1099/ijs.0.062927-0
ter Braak, C. J. F. (1990). Update Notes: CANOCO Version 3. 10. Wageningen: Agricultural Mathematics Group Press.
Teramoto, M., Ohuchi, M., Hatmanti, A., Darmayati, Y., Widyastuti, Y., Harayama, S., et al. (2011). Oleibactermarinus gen. nov., sp. nov., a bacterium that degrades petroleum aliphatic hydrocarbons in a tropical marine environment. Int. J. Syst. Evol. Micr. 61, 375–380. doi: 10.1099/ijs.0.018671-0
Wang, L. P., Wang, W. P., Lai, Q. L., and Shao, Z. Z. (2010). Gene diversity of CYP153A and AlkB alkane hydroxylases in oil-degrading bacteria isolated from the Atlantic Ocean. Environ. Microbiol. 12, 1230–1242. doi: 10.1111/j.1462-2920.2010.02165.x
Wang, W. P., Zhang, R. Q., Shan, D. P., and Shao, Z. Z. (2014). Indigenous oil-degrading bacteria in crude oil-contaminated seawater of the Yellow sea. China. Appl. Microbiol. Biot. 98, 7281–7281. doi: 10.1007/s00253-014-5922-1
Wang, Y. N., Cai, H., Chi, C. Q., Lu, A. H., Lin, X. G., Jiang, Z. F., et al. (2007). Halomonasshengliensissp. nov., a moderately halophilic, denitrifying, crude-oil-utilizing bacterium. Int. J. Syst. Evol. Microbiol. 57, 1222–1226. doi: 10.1099/ijs.0.64973-0
Wickham, H. (2016). gplot2: Elegant Graphics for Data Analysis, 2nd Edn. New York, NY: Springer Press.
Wu, Y. Z., Qiu, J. W., Qian, P. Y., and Wang, Y. (2018). The vertical distribution of prokaryotes in the surface sediment of Jiaolongcold seep at the northern South China Sea. Extremophiles 22, 499–510. doi: 10.1007/s00792-018-1012-0
Xie, X. N., Muller, R. D., Li, S. T., Gong, Z. S., and Steinberger, B. (2006). Origin of anomalous subsidence along the Northern South China Sea margin and its relationship to dynamic topography. Mar. Petrol Geol. 23, 745–765. doi: 10.1016/j.marpetgeo.2006.03.004
Xu, T., Feng, D., Tao, J., and Qiu, J. W. (2019). A new species of deep-sea mussel (Bivalvia: Mytilidae: Gigantidas) from the South China Sea: morphology, phylogenetic position, and gill-associated microbes. Deep-Sea Res Pt I 146, 79–90. doi: 10.1016/j.dsr.2019.03.001
Yakimov, M. M., Giuliano, L., Denaro, R., Crisafi, E., Chernikova, T. N., Abraham, W. R., et al. (2004). Thalassolituusoleivorans gen. nov., spnov., a novel marine bacterium that obligately utilizes hydrocarbons. Int. J. Syst. Evol. Micr. 54, 141–148. doi: 10.1099/ijs.0.02424-0
Yakimov, M. M., Golyshin, P. N., Lang, S., Moore, E. R., Abraham, W. R., Lunsdorf, H., et al. (1998). Alcanivoraxborkumensis gen. nov., sp. nov., a new, hydrocarbon-degrading and surfactant-producing marine bacterium. Int. J. Systematic Bacteriol. 48(Pt 2), 339–348. doi: 10.1099/00207713-48-2-339
Yang, S. S., Lv, Y. X., Liu, X. P., Wang, Y. Z., Fan, Q. L., Yang, Z. F., et al. (2020). Genomic and enzymatic evidence of acetogenesis by anaerobic methanotrophicarchaea. Nat. Commun. 11:3941. doi: 10.1038/S41467-020-17860-8
Yoon, J. H., Kang, S. J., and Lee, S. Y. (2012). Salinimonaslutimarisspnov., a polysaccharide-degrading bacterium isolated from a tidal flat. Anton LeeuwInt J. G 101, 803–810. doi: 10.1007/s10482-011-9695-6
Yu, Y., Lee, C., Kim, J., and Hwang, S. (2005). Group-specific primer and probe sets to detect methanogenic communities using quantitative real-time polymerase chain reaction. Biotechnol. Bioeng. 89, 670–679. doi: 10.1002/bit.20347
Zhang, J. X., Yang, Y. Y., Zhao, L., Li, Y. Z., Xie, S. G., and Liu, Y. (2015). Distribution of sediment bacterial and archaeal communities in plateau freshwater lakes. Appl. Microbiol. Biot. 99, 3291–3302. doi: 10.1007/s00253-014-6262-x
Zhang, Y., Su, X., Chen, F., Wang, Y. Y., Jiao, L., Dong, H. L., et al. (2012). Microbial diversity in cold seep sediments from the northern South China Sea. Geosci. Front. 3, 301–316. doi: 10.1016/j.gsf.2011.11.014
Zhang, Y., Jing, H. M., and Peng, X. T. (2020). Vertical shifts of particle-attached and free-living prokaryotes in the water column above the cold seeps of the South China Sea. Mar. Pollut. Bull. 158:111472. doi: 10.1016/j.marpolbul.2020.111472
Zhao, Y., Xu, T., Law, Y. S., Feng, D., Li, N., Xin, R. J., et al. (2020). Ecological characterization of cold-seep epifauna in the South China Sea. Deep-Sea Res Pt I 163:103361. doi: 10.1016/J.Dsr.2020.103361
Zhuang, G. C., Xu, L., Liang, Q. Y., Fan, X. B., Xia, Z., Joye, S. B., et al. (2019). Biogeochemistry, microbial activity, and diversity in surface and subsurface deep-sea sediments of South China Sea. Limnol. Oceanogr. 64, 2252–2270. doi: 10.1002/lno.11182
Keywords: methane seeps, various seepage intensities, oil seepage, microbial communities, bottom water, South China Sea
Citation: Li X, Dai Z, Di P, Feng J, Tao J, Chen D, Li N and Li Y (2021) Distinct Bottom-Water Bacterial Communities at Methane Seeps With Various Seepage Intensities in Haima, South China Sea. Front. Mar. Sci. 8:753952. doi: 10.3389/fmars.2021.753952
Received: 05 August 2021; Accepted: 18 October 2021;
Published: 15 November 2021.
Edited by:
Fengping Wang, Shanghai Jiao Tong University, ChinaReviewed by:
Xiyang Dong, Sun Yat-sen University, ChinaYong Wang, Institute of Deep-Sea Science and Engineering, Chinese Academy of Sciences (CAS), China
Copyright © 2021 Li, Dai, Di, Feng, Tao, Chen, Li and Li. This is an open-access article distributed under the terms of the Creative Commons Attribution License (CC BY). The use, distribution or reproduction in other forums is permitted, provided the original author(s) and the copyright owner(s) are credited and that the original publication in this journal is cited, in accordance with accepted academic practice. No use, distribution or reproduction is permitted which does not comply with these terms.
*Correspondence: Niu Li, liniu@scsio.ac.cn; Yazi Li, liyazi@smu.edu.cn
†These authors have contributed equally to this work