- 1Department of Biochemistry and Molecular Biology, School of Basic Medical Sciences, Southern Medical University, Guangzhou, China
- 2Guangdong Provincial Key Laboratory of Single Cell Technology and Application, Southern Medical University, Guangzhou, China
- 3Guangdong-Hong Kong-Macao Greater Bay Area Center for Brain Science and Brain-Inspired Intelligence, Southern Medical University, Guangzhou, China
- 4Department of Pharmaceutical Engineering, College of Materials and Energy, South China Agricultural University, Guangzhou, China
- 5Department of Pediatrics, Nanfang Hospital, Southern Medical University, Guangzhou, China
Conopeptides from the marine cone snails are a mixture of cysteine-rich active peptides, representing a unique and fertile resource for neuroscience research and drug discovery. The ConoServer database includes 8,134 conopeptides from 122 Conus species, yet many more natural conopeptides remain to be discovered. Here, we identified 517 distinct conopeptide precursors in Conus quercinus using de novo deep transcriptome sequencing. Ten of these precursors were verified at the protein level using liquid chromatography-mass spectrometry/mass spectrometry (LC-MS/MS). The combined gene and protein analyses revealed two novel gene superfamilies (Que-MNCLQ and Que-MAMNV), and three other gene superfamilies (N, P, and I1) were reported for the first time in C. quercinus. From the Que-MAMNV superfamily, a novel conotoxin, Que-0.1, was obtained via cloning and prokaryotic expression. We also documented a new purification process that can be used to induce the expression of conopeptides containing multiple pairs of disulfide bonds. The animal experiments showed that Que-0.1 strongly inhibited neuroconduction; the effects of Que-1.0 were 6.25 times stronger than those of pethidine hydrochloride. In addition, a new cysteine framework (CC-C-C-C-C-C-CC-C-C-C-C-C) was found in C. quercinus. These discoveries accelerate our understanding of conopeptide diversity in the genus, Conus and supply promising materials for medical research.
Introduction
Carnivorous marine gastropod mollusks in the genus Conus produce a venomous cocktail for predation, defense, or competition. Any significant amount of Conus venom may contain hundreds of different peptides, each of which generally comprised of 12–50 residues with multiple pairs of disulfide bonds; these peptides are called conotoxins or conopeptides (Gao et al., 2017; Terlau and Olivera, 2017). Owing to the high selectivity of certain ion channels and nerve receptors, conotoxins have gradually become a useful tool for neuroscience research and have great potential utility for the development of novel drugs. Conus species can be roughly divided into three groups based on diet: vermivorous, molluscivorous, and piscivorous (Gao et al., 2017). Of these, insectivorous Conus are the most common, accounting for about 70% of all the Conus species worldwide (Gao et al., 2017). Insect-eating Conus have dominated throughout the evolution of this genus (Gao et al., 2017).
Several recent studies of conotoxins have focused on their strong pharmacological activity as potential analgesics (Terlau and Olivera, 2017; Shen et al., 2019). The conotoxin MVIIA (Ziconotide), a selective N-type voltage-sensitive calcium channel blocker that contains the cysteine framework C-C-CC-C-C, is approved by the US Food and Drug Administration (FDA) to treat the intractable pain (Miljanich, 2004; Wermeling, 2005). Similarly, the conotoxin MrIA, a norepinephrine transporter inhibitor, is under phase II clinical trials as a potential pain treatment (Lovelace et al., 2006; Paczkowski et al., 2007; Wan et al., 2016). Yet, additional conopeptide materials are still required from which to screen the desired drugs.
In general, the conopeptide signal peptide region is a relatively well conserved (Gao et al., 2017). Based on the sequence similarities among the signal peptides, ConoServer1 currently classifies the conopeptide-encoding genes into 40 superfamilies. Of these, 27 gene superfamilies (A, B1, B2, B3, C, D, E, F, G, H, I1, I2, I3, J, K, L, M, N, O1, O2, O3, P, Q, S, T, V, and Y) are widely recognized (Kaas et al., 2008, 2010, 2012; Puillandre et al., 2012; Ye et al., 2012; Aguilar et al., 2013; Dutertre et al., 2013; Luo et al., 2013; Lu et al., 2014; Fu et al., 2018; Yao et al., 2019; Zhang et al., 2019; Li et al., 2020). The remaining 13 gene superfamilies are listed as temporary in the ConoServer database (Espiritu et al., 2001; Biggs et al., 2010; Kaas et al., 2010, 2012). However, in the ConoServer database, 31 distinct cysteine frameworks are described (Bernáldez et al., 2013). The recent studies have reported more than the 30 novel temporarily gene superfamilies (Lavergne et al., 2015; Peng et al., 2016; Prashanth et al., 2016). The genomic or proteomic analyses of a single Conus specimen may reveal hundreds of unique conopeptides (Biass et al., 2009; Davis et al., 2009; Dutertre et al., 2013; Lavergne et al., 2015). We anticipate that many natural conopeptides remain to be identified, and that these as-yet unknown compounds have great potential utility.
Herein, we used transcriptome sequencing to identify a series of novel putative conopeptides from the three venom ducts of the vermivorous cone snail Conus quercinus. Five novel gene superfamilies were further verified using liquid chromatography-mass spectrometry/mass spectrometry (LC-MS/MS). One of the newly discovered conotoxins, Que-0.1, which has a novel cysteine framework, was prepared using the genetic engineering techniques. The nerve-blocking potential of this conotoxin was analyzed using the electrophysiological methods.
Results
Transcriptome Sequencing
A summary of the transcriptome assemblies of the three venom ducts is given in Supplementary Table 1. Illumina sequencing of Que-1-VD, Que-2-VD, and Que-3-VD generated approximately 8.39, 9.21, and 7.19 Gb of clean data, respectively. Based on these data, full-length transcript sequences were assembled using Trinity (Fu et al., 2015). The total numbers of potential transcripts in Que-1-VD, Que-2-VD, and Que-3-VD were 134,610, 113,063, and 97,027, respectively. The percentage of Q30 clean bases in Que-1-VD, Que-2-VD, and Que-3-VD were 93.2, 96.52, and 93.21%, respectively. The GC contents of the potential transcripts in Que-1-VD, Que-2-VD, and Que-3-VD were 43.46, 44, and 42.97%, and the average lengths of potential transcripts were 434.94, 366.95, and 414.24 bp. For Que-1-VD, Que-2-VD, and Que-3-VD, the ratio of pair mapping was 79.09, 70.31, and 83.39%, and the total numbers of open reading frames (ORFs) were 15,364, 12,141, and 10,068, respectively.
We used the Venn diagrams to visualize the numbers of annotated genes. In the databases, non-redundant nucleotide sequences (Nt), Swiss-Prot, and non-redundant protein sequences (Nr), there were 3,071, 14,918, 9,983, and 19,980 hits, respectively, for Que-1-VD (Supplementary Figure 1A); 2,964, 14,608, 8,185, and 18,828 hits, respectively, for Que-2-VD (Supplementary Figure 1B); and 2,419, 11,463, 6,884, and 14,971, respectively, for Que-3-VD (Supplementary Figure 1C). The major functional Gene Ontology Consortium (GO) terms in the biological process category were cellular process, single-organism process, and metabolic process (Supplementary Figure 1D).
The cytochrome c oxidase subunit I (COI) gene is often used as a DNA barcode for animal species identification (Hebert et al., 2003). From the NCBI EST database, we obtained 22 COI nucleotide sequences from eight Conus species: C. tribblei (Barghi et al., 2015), C. lenavati (Li et al., 2017), C. episcopatus (Prashanth et al., 2016), C. marmoreus (Dutertre et al., 2013), C. geographus (Safavi-Hemami et al., 2014), C. eburneus (Liu et al., 2012), C. flavidus (Davis et al., 2009), C. litteratus (Lu et al., 2014), and C. quercinus. We aligned these sequences with the COI nucleotide sequences of Que-1-VD, Que-2-VD, and Que-3-VD. The resulting evolutionary tree indicated that the genetic distances among the COI genes from the nine Conus species were 0.01–0.011. On the contrary, the genetic distances among the two previously published COI sequences (MN389188.1 and KJ606021) and three of the COI sequences collected from the five C. quercinus specimens were 0.000–0.003. This evolutionary tree was consistent with the traditional morphological identifications and classifications. Thus, we identified Que-1-VD, Que-2-VD, and Que-3-VD as C. quercinus (Figure 1).
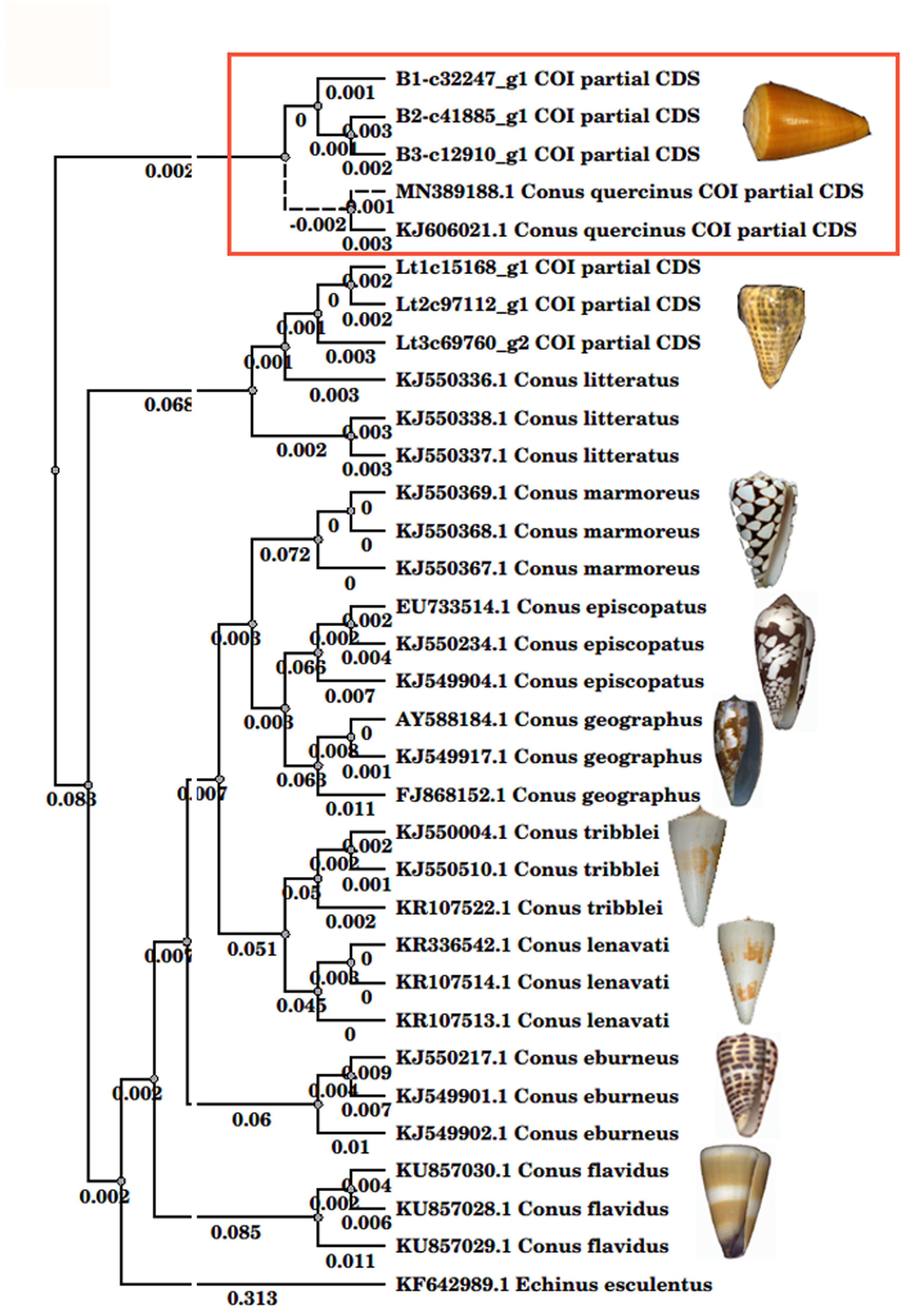
Figure 1. The phylogenetic relationship of Conus species. The phylogenetic tree was inferred from the partial sequences of cytochrome oxidase I. Tree building method: PHYLIP Neighbor Joining in the UGENE-1.25.0 program. The Distance matrix model: FB4. Transition/transversion ratio: 2.00. Some species pictures were cited in the literature (Zhang et al., 2019).
Eukaryotic Orthologous Group Functional Classification of the Open Reading Frames
To further explore the possible functions, we screened the identified ORFs against the eukaryotic orthologous groups (KOGs) database. In Que-1-VD, Que-2-VD, and Que-3-VD, we successfully assigned the KOG functional annotations to 9,910, 8,114, and 6,753 sequences, respectively, these annotations were grouped into 25 functional categories (Supplementary Figure 2). Across all the annotations, 3,669 sequences (14.81%) were annotated as “general function prediction”; 3,143 sequences (12.69%) were annotated as “signal transduction mechanisms”; and 2,567 sequences (10.36%) were annotated as “posttranslational modification, protein turnover, and chaperones.” The functions “intracellular trafficking, secretion, and vesicular transport,” “transcription,” “translation, ribosomal structure, and biogenesis,” and “RNA processing and modification” were associated with similar numbers of sequences: 5.11% (1,266 sequences), 5.17% (1,282 sequences), 5.00% (1,236 sequences), and 6.92% (1,715 sequences). In contrast, “defense mechanisms” and “cell motility” were associated with the fewest sequences: 0.73% (180 sequences) and 0.35% (78 sequences), respectively. This pattern of KOG enrichment is similar to that of the sequences from the venom ducts of C. litteratus (Puillandre et al., 2012). Our results showed that many genes involved in the signal transduction and post-translational modification are active in the venom ducts.
Putative Conopeptide Precursors Predicted With ConoSorter
ConoSorter, a machine-learning tool that predicts toxin genes, is widely used to identify and classify the conotoxin gene precursors (Lavergne et al., 2013, 2015; Phuong et al., 2016). Owing to the risk of over-fitting that is associated with machine learning, we adjusted the hydrophobicity threshold of the analysis, which reduced noise while improving the accuracy and efficiency of the predictions. This analysis gave reliable output when conopeptide precursors predicted with ConoSorter was further screened using ConoServer (Kaas et al., 2012) and artificial correction (Safavi-Hemami et al., 2015).
According to the ConoServer database (see text footnote 1), C. quercinus contains 133 mature conotoxins in 13 gene superfamilies (A, B1, I2, Insin, K, M, O1, O2, O3, Q, T, Y, and divergent M—L-LTVA). Using ConoSorter (Materials and methods), we predicted and annotated 273, 180, and 188 conopeptide precursor sequences from Que-1-VD, Que-2-VD, and Que-3-VD, respectively (Supplementary Table 2). The Venn diagrams were used to visualize the relationships among the annotated conopeptide precursor sequences (Figure 2A). There were 194, 115, and 106 unique sequences in Que-1-VD, Que-2-VD, and Que-3-VD, respectively, while 42 sequences were shared between Que-1-VD and Que-2-VD, 45 sequences were shared between Que-2-VD and Que-3-VD, and 59 sequences were shared between Que-1-VD and Que-3-VD.
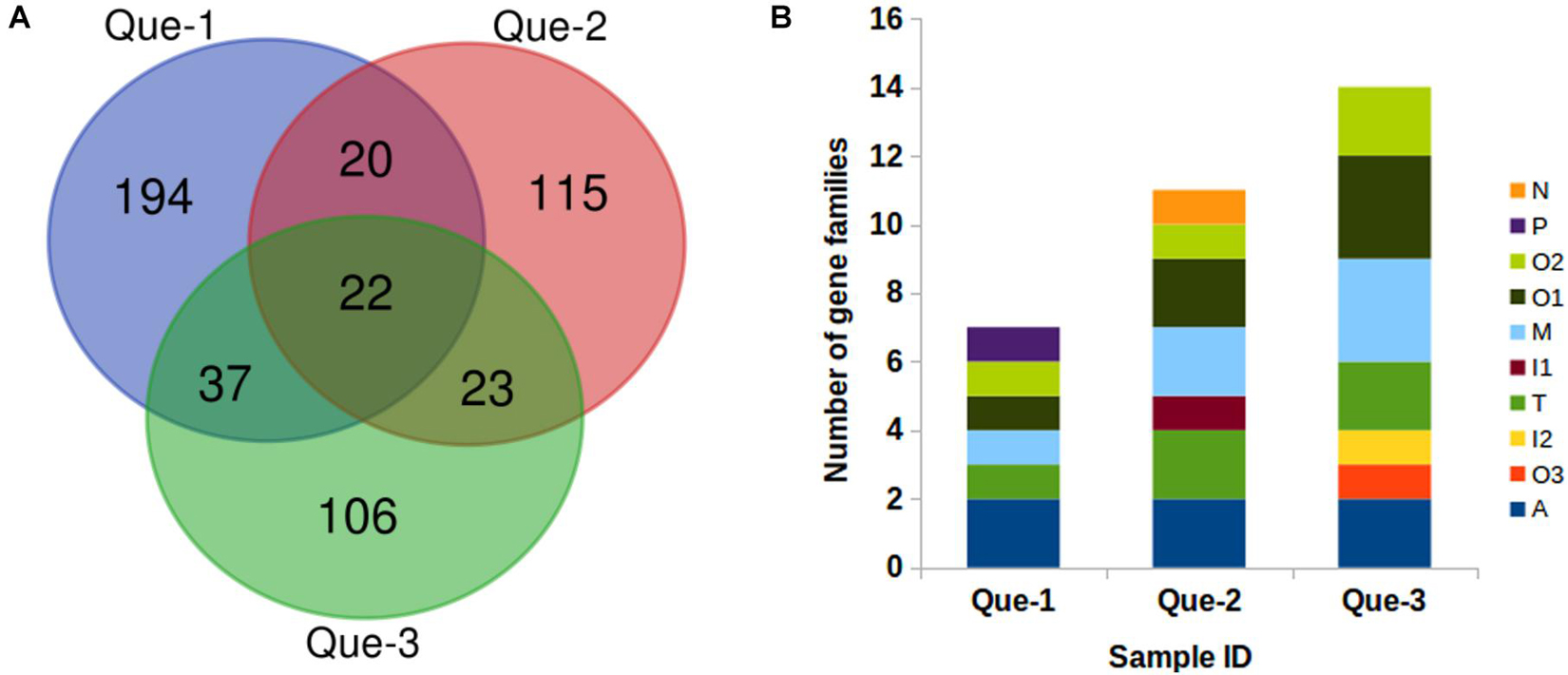
Figure 2. The annotated conopeptides predicted from transcriptome sequencing. (A) Relationship of the annotated conopeptides predicted by ConoSorter from the Que-1-VD, Que-2-VD, and Que-3-VD. (B) Number of the identified conopeptide precursors previously reported for Conus quercinus.
We identified 17 previously reported conopeptides in Que-1-VD, belonging to seven gene superfamilies (A, M, O1, O2, O3, P, and T); 12 previously reported conopeptides in Que-2-VD, belonging to seven gene superfamilies (A, I1, M, N, O1, O2, and T); and 14 previously reported conopeptides in Que-3-VD, belonging to seven gene superfamilies (A, I2, M, O1, O2, O3, and T). In total, 517 conopeptide genes were identified, of which 26 unique precursors were previously reported (Figure 2B). The 26 unique precursors were Qc5.2, Qc5.1, Qc3.1, Qc3-YDG01, Qc1.2, Qc1.1b, Qc1.18, Qc1.16, Qc1.11, MrIA, Mr5.1b, Mr15.3, Mr11.2, MaIr137, LtXVIA, LtXVA, LtVIC, LtVIA, LtIIIA, Lt9a variant, Lt6d/Lt6e, Lt5b, Lt11.2, Eu6.6, Contryphan-Lo, and Contryphan-G. Of these 26 precursors, 5 were previously identified in the molluscivorous species C. marmoreus (MrIA, Mr5.1b, Mr15.3, Mr11.2, and MaIr137) (Duda and Palumbi, 2000; Luo et al., 2006; Pi et al., 2006; Liu et al., 2012), and 10 were previously identified in the vermivorous species C. litteratus (LtXVIA, LtXVA, LtXVA, LtVIC, LtVIA, LtIIIA, Lt9a variant, Lt6d/Lt6e, Lt5b, and Lt11.2) (Duda and Palumbi, 2000; Luo et al., 2006; Pi et al., 2006; Liu et al., 2012). The Eu6.6 precursors from the related vermivorous species C. ebraeus (Hu et al., 2012) were also predicted by ConoSorter. Notably, the N, P, and I1 gene superfamilies, which were previously reported in this genus, were identified herein for the first time in the vermivorous C. quercinus.
Distribution of Cysteine Frameworks
Cysteine bridges improve the stability of mature conopeptide domains and provide resistance to enzymatic degradation, while the cysteine frameworks with disulfide connectivity are more pharmacologically relevant (Pakkala et al., 2010; Then et al., 2016). Across the 517 conopeptide genes in vermivorous C. quercinus, the most common cysteine frameworks were XIV (C-C-C-C), VI/VII (C-C-CC-C-C), and I (CC-C-C): these frameworks were found in 40, 36, and 26 genes, respectively (Supplementary Figure 3). In addition, 14 genes contained framework V (CC-CC), 10 genes contained framework XVI (C-C-CC), and 10 genes contained framework III (CC-C-C-CC). The frameworks IX (C-C-C-C-C-C), XII (C-C-C-C-CC-C-C), XV (C-C-CC-C-C-C-C), and XXIV (C-CC-C) were all found in seven genes. The remaining nine cysteine frameworks were found in between 2 and 5 genes each. These results further demonstrated the diversity of cysteine frameworks.
Conopeptide Precursors Verified by Liquid Chromatography-Mass Spectrometry/Mass Spectrometry
The total proteins from the venom duct were sequenced using a Thermo LTQ Orbitrap Elite (Thermo Fisher Scientific, MA, United States). LC-MS/MS sequencing generated 177, 138, and 153 Mb of raw ion current traces from Que-1-VD, Que-2-VD, and Que-3-VD, respectively. The total ion current traces for the three venom ducts of C. quercinus are shown in Figure 3A. We identified three conopeptide gene superfamilies (F, A, and M) (Supplementary Table 3) and 10 unique conopeptides (Figure 3B). In addition, Qu1.8 was identified by LC-MS/MS. Que-1-c61524_g2_4_1, Que-2-c19299_g2_2_1, and Que-3-c57791_g1_4_1 were identified as the same toxin, and the mature peptide was renamed Que-0.1. The mature region of the aligned sequence is shown in Figure 3C. The signal regions of Que-0.1, Que-2-c57915_g1_5, and Que-3-c11961_g1_6_5 did not cluster with any known groups. An evolutionary analysis showed that these three novel conopeptide precursors belonged to two new gene superfamilies. We named these gene superfamilies Que-MNCLQ and Que-MAMNV based on their signal peptide sequences (MNCLQLLLVLLLISTIAA and MAMNVWMTISVLVVVVMATAVTG, respectively, Figure 4). In addition, we identified the cysteine framework CC-C-C-C-C-C-CC-C-C-C-C-C for the first time in cone snails. We also found disulfide links at the cysteine residue sites Cys50, Cys56, Cys64, and Cys68 in the cysteine framework of Que-1-c61524_g2_4_1.
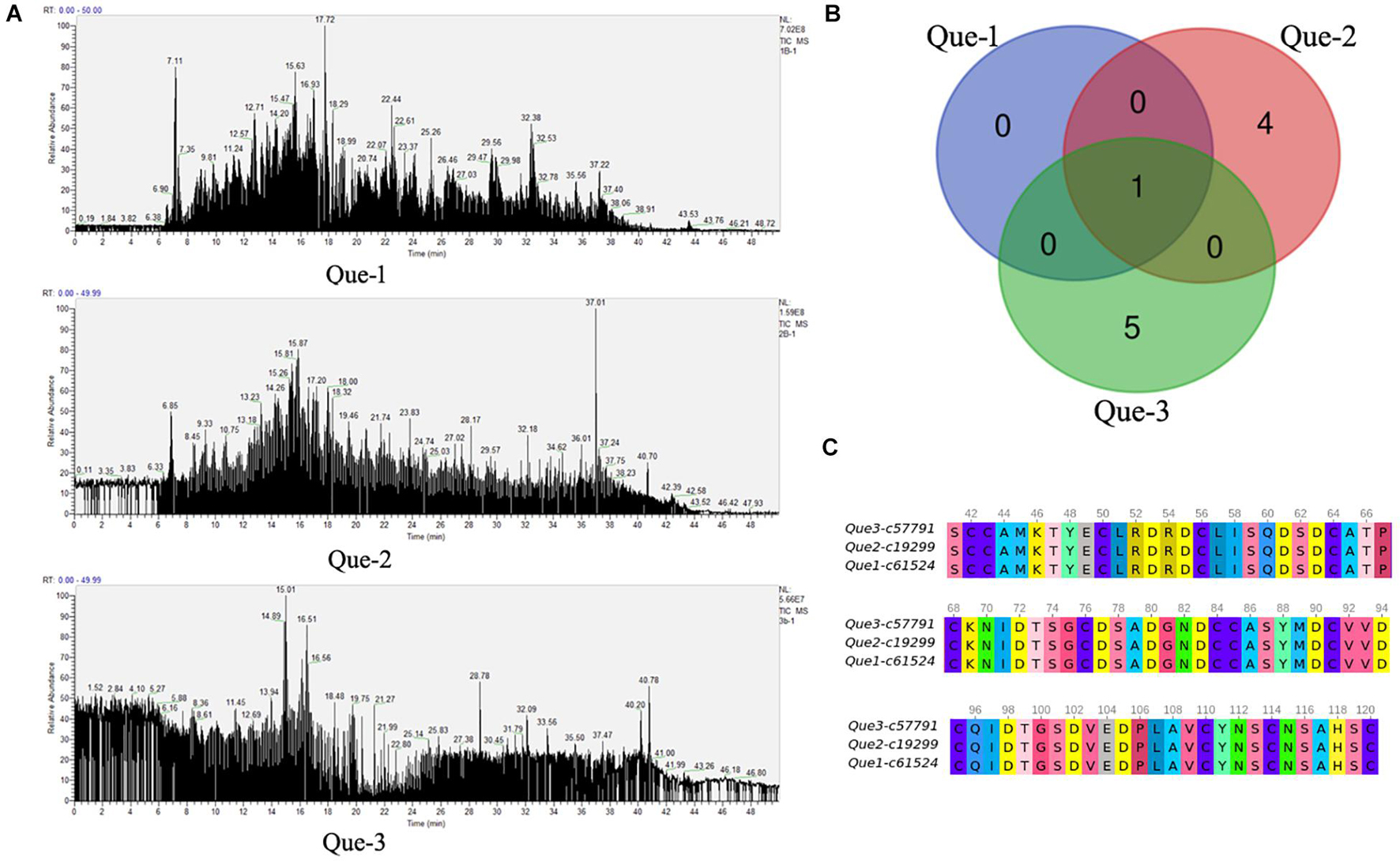
Figure 3. The results of LC-MS/MS analysis. (A) Total ion current traces of the venom ducts of three C. quercinus. Liquid chromatography-mass spectrometry/mass spectrometry (LC-MS/MS) runs using an LTQ Orbitrap Elite System. (B) The Venn diagrams of the identified conopeptides at the protein level from the Que-1-VD, Que-2-VD, and Que-3-VD. (C) The sequence alignment of a novel conopeptide Que-0.1 from the Que-1-VD, Que-2-VD, and Que-3-VD.
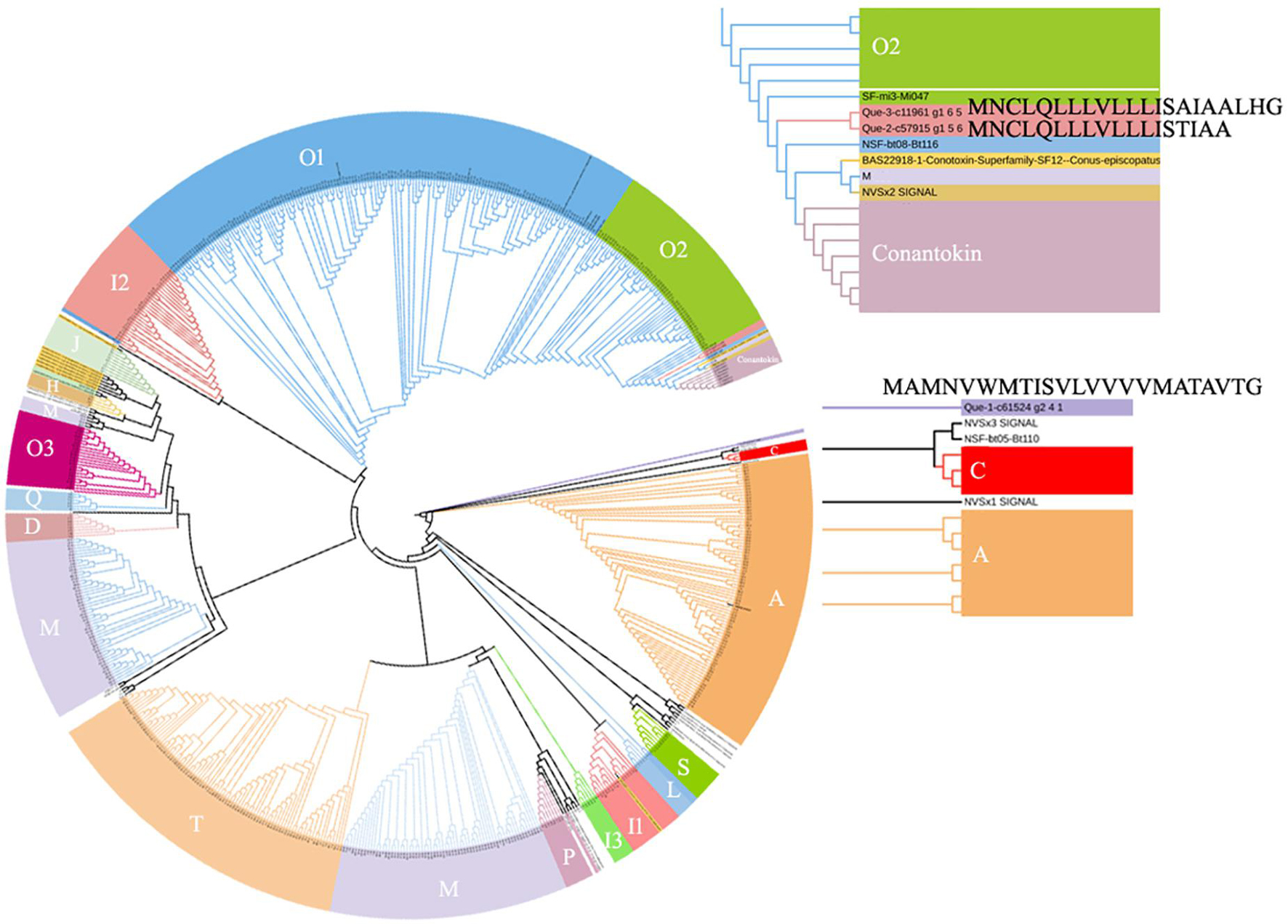
Figure 4. Phylogenetic tree of signal sequences from three new precursor conopeptides (Supplementary Table 3) and 63 signal sequences. The amino acid sequences were aligned and a guide tree were constructed using the CLUSTALW 2.1. Tree building method: PHYLP Neighbor Joining. The guide tree was decorated using iTOL (http://itol.embl.de/).
Expression, Purification, and Identification of Que-0.1
The length of the Que-0.1 peptide sequence was 80 amino acids. Owing to the multiple pairs of disulfide bonds in Que-0.1, we selected the expression vector PET32a (+) with ampicillin resistance. The N-terminal of this vector contains the tag protein thioredoxin (TrxA) for auxiliary oxidative folding and the six histidine (His) tag sequence for purification. The sequence is the toxin gene, the restriction endonuclease MscI, the spacer sequence, and the intestinal kinase (EK) recognition site from 5′ end to 3′ end. The termination codon and the restriction endonuclease Xho I were added to the 3′ end of the Que-0.1 gene. The recombinant Que-0.1 gene was synthesized directly. The map of the constructed PET32a-Que-0.1 expression vector and the Sanger sequencing peak map of Que-0.1 gene are shown in Supplementary Document.
The PET32a-Que-0.1 expression vector was successfully constructed and transferred into Escherichia coli (E. coli) BL21 (DE3) strain for induction and expression. The optimal temperature, IPTG (Isopropyl β-D-thiogalactoside) concentration, and time used to induce the expression of the fusion peptides were explored to maximize the product yield while retaining the biological activity. The final induction conditions were as follows: culture at 37°C until an OD600 of 0.6, add IPTG to a final concentration of 0.1 mM, and culture at 18°C for 10 h. On average, 3 mg of recombinant Que-0.1 conotoxin were obtained from l L culture.
To obtain high-purity recombinant Que-0.1, we first purified the recombinant Que-0.1 product with six histidines (His). After Ni affinity purification, the resultant recombinant Que-0.1 product was of high purity. After the N-terminal TrxA label in the purified product was removed by EK, Que-0.1 was further purified using the AKTA Explorer chromatography system. The molecular weight of the purified product was consistent with the theoretical molecular weight of Que-0.1 (8.52 kDa), as shown by the sodium dodecyl sulfate polyacrylamide gel electrophoresis (SDS-PAGE) analysis (Figure 5A). The crude recombinant Que-0.1 was again purified using reversed phase high performance liquid chromatography (RP-HPLC). The 2 min peak, which was the target peptide (Figure 5B), was collected. To verify that the product was recombinant Que-0.1, we validated it using mass spectrometry. The two peptides of recombinant Que-0.1 were successfully identified (Figure 5C). The products were stored separately and lyophilized for the activity test.
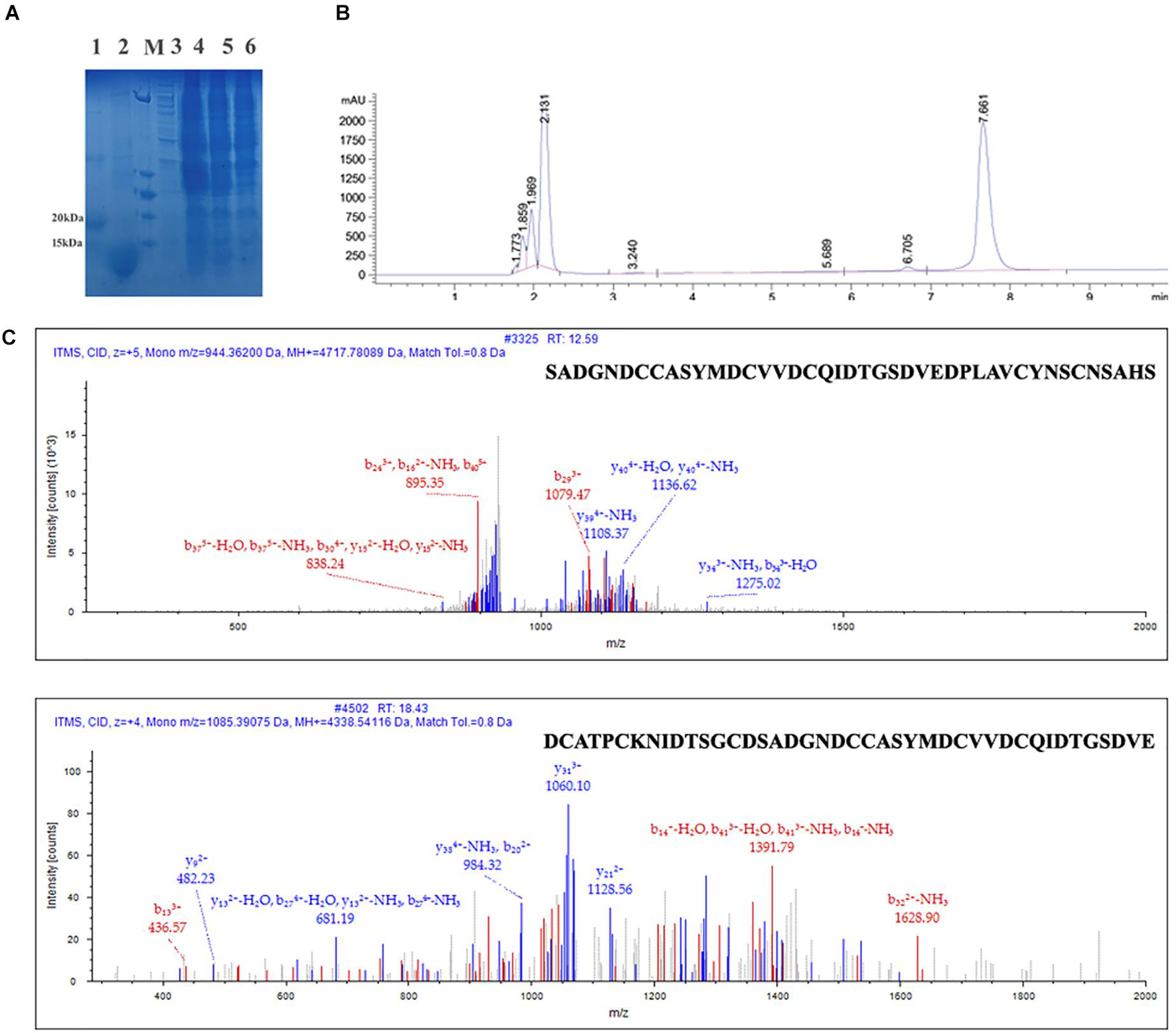
Figure 5. Expression, purification, and identification of Que-0.1. (A) Sodium dodecyl sulfate polyacrylamide gel electrophoresis (SDS-PAGE) analyze of expression of recombinant Que-0.1; 1: sample of the fusion protein TrxA-QcMAMN0.1; 2: sample of the fusion protein TrxA-Que-0.1 digested by enterokinase; 3: the standard protein marker. (B) Reverse phase high-performance liquid chromatography (HPLC) purification of purified conopeptide Que-0.1. (C) LC-MS/MS of Que-0.1.
Biological Activity of Que-0.1
To test the biological activity of the recombinant Que-0.1 toxin, we designed the model (Figure 6) described in section “Materials and Methods.” We used the clinical pethidine hydrochloride anesthetic as the positive control group and the recombinant Que-0.1 toxin as the experimental group.
We recorded eight electrical stimulation signals in the positive control group before drug administration, eight signals when 5 μg/μl pethidine hydrochloride was applied, and six signals after the drug was washed out. The peaks were 0.08, 0.01, and 0.02 mV, respectively, and the troughs were -0.08, -0.02, and -0.04 mV, respectively (Figure 7). In the experimental group, we recorded six electrical stimulation signals in the positive control group before drug administration, six signals when 5 μg/μl pethidine hydrochloride was applied, and six signals after the drug was washed out. The peaks were 0.18, 0.09, and 0.12 mV, respectively, and the troughs were -0.10, -0.11, and -0.07 mV, respectively (Figure 8).
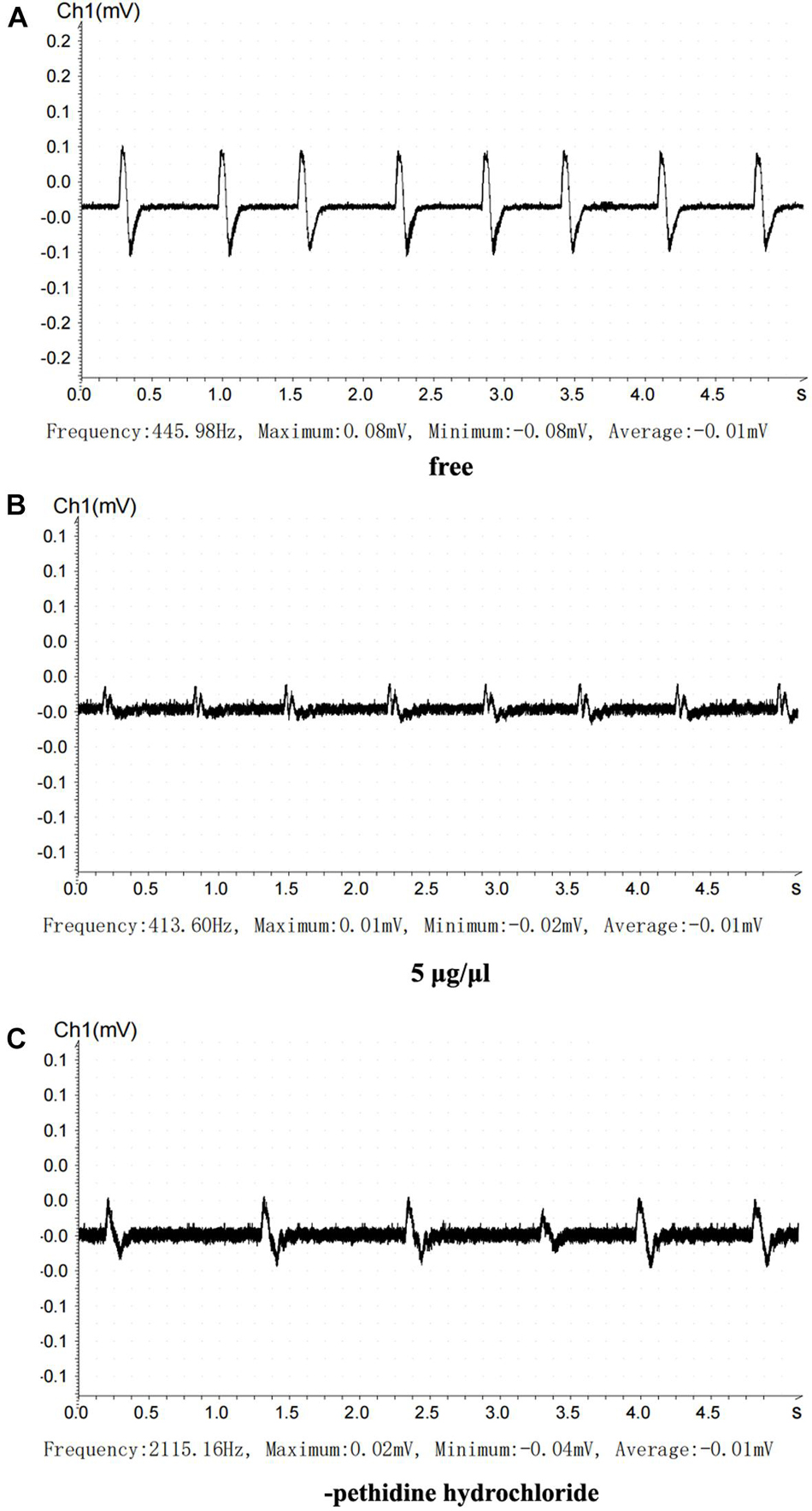
Figure 7. Inhibitory action of the recombinant Que-0.1 on neuromuscular transmission. (A) Before treatment. (B) 0.5 μg/μl recombinant Que-0.1. (C) Cleaning recombinant Que-0.1 with Ren’s solution.
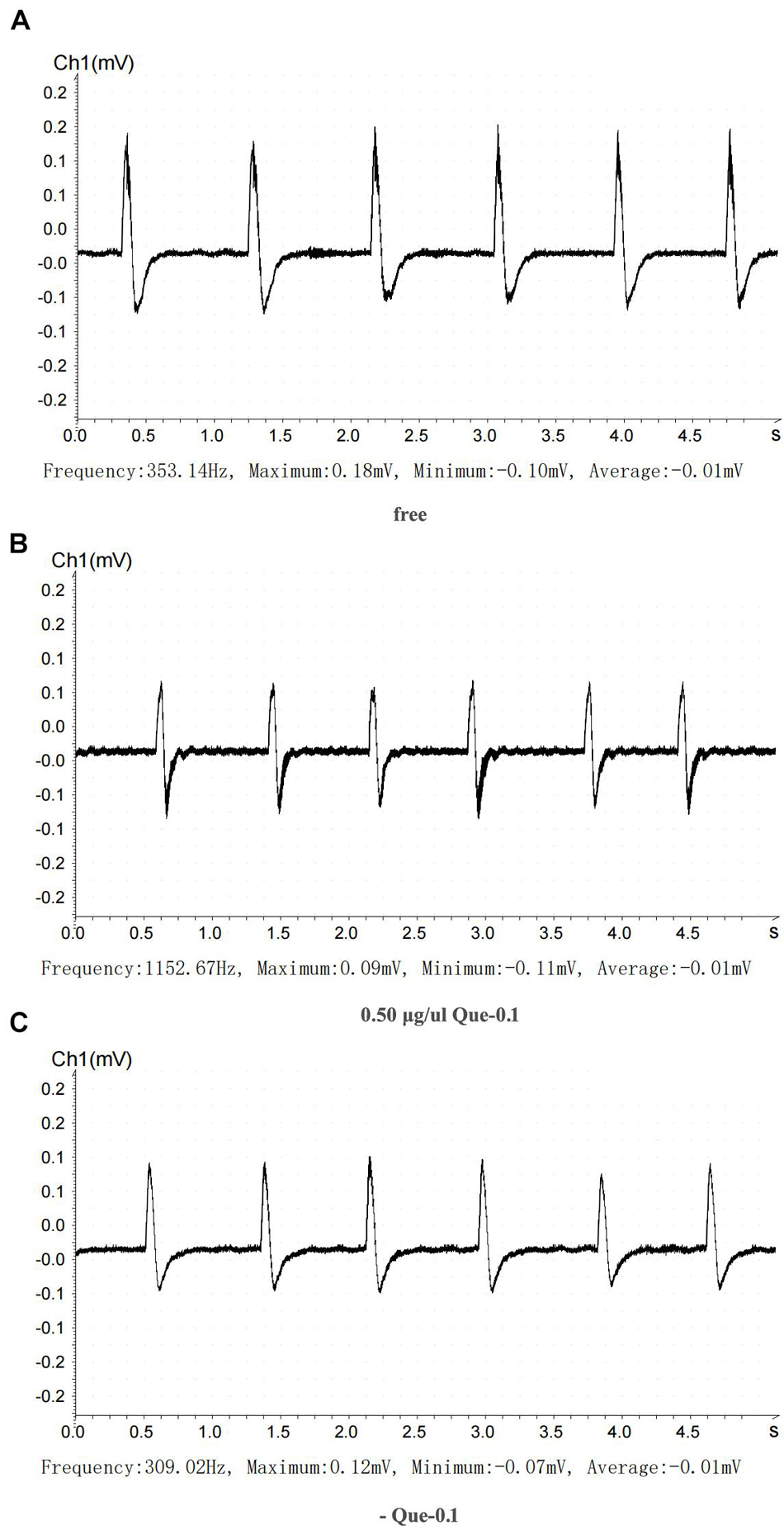
Figure 8. Inhibitory action of pethidine hydrochloride on neuromuscular transmission. (A) Before treatment. (B) 5 μg/μl pethidine hydrochloride. (C) Cleaning pethidine hydrochloride with Ren’s solution.
Using the statistics described in the Materials and methods, we found that the treatment with 5 μg/μl pethidine hydrochloride inhibited neural signal transduction by 20%, which recovered by 60% after the pethidine hydrochloride was washed out. Importantly, treatment with 0.5 μg/μl recombinant Que-0.1 inhibited neural signal transduction by 50%. When the toxin was washed out, neural signal transduction recovered to 66.67%. At equivalent doses, Que-0.1 had a stronger inhibitory effect on neuromuscular conduction than pethidine hydrochloride; specifically, the inhibitory effect of Que-0.1 was 6.25 times greater than that of pethidine hydrochloride.
Discussion
The toxin resources of cone snails are rich and diverse. To date, more than 700 species of cone snail have been described, but the transcriptome and proteome data are available for only a few of these species (Violette et al., 2012; Dutertre et al., 2013; Safavi-Hemami et al., 2014; Jin et al., 2015; Lavergne et al., 2015). The transcriptomic and proteomic approaches have different advantages in the identification of novel conotoxins. To uncover conopeptide diversity in the venom of vermivorous species and to explore the potential pharmacological applications of natural conopeptides, we systematically analyzed the conopeptide genes and proteins in the venom ducts of the vermivorous species C. quercinus. The phenetic and phylogenetic relationships of the specimens were analyzed based on the shell morphology, COI sequences, and internal transcribed spacer 2 (ITS2) sequences. We used these analyses to distinguish this new species from other species (Nam et al., 2009; Puillandre et al., 2014a, b).
The COI gene is an excellent marker gene for species classification in Conus. We extracted the COI genes from transcriptome of C. quercinus. We then constructed an evolutionary tree based on these sequences and previously published the COI gene sequences from eight other cone snails. We found that the genetic distances among the COI genes of the analyzed Conus species were 0.01–0.011. Using these data, we correctly identified C. quercinus. Furthermore, the KOG enrichment analysis showed that the venom ducts were highly enriched in the signal transduction and post-translational modification genes, presumably due to the large number of conopeptides that are synthesized and processed in this organ.
Many conotoxins are identified in various Conus species, such as 215 distinct conopeptides in C. betulinus (Peng et al., 2016), 136 unique conopeptides in C. tribblei (Barghi et al., 2015), and 3,305 novel conopeptides in C. episcopatus (Lavergne et al., 2015). Here, we identified 517 full-length putative conopeptide precursors in total, encompassing 20 known cysteine frameworks. Thus, the accurate identification of conotoxin types provide key clues for the biological research and clinical medicine (Dao et al., 2017). Our analyses of conopeptide genes among the venom ducts of three snails demonstrated that many conopeptide gene sequences differed among the individual C. quercinus. The N, P, and I1 gene superfamilies, which have been previously identified in other Conus species, are reported here for the first time from the vermivorous C. quercinus. In addition, a small set of conopeptide genes with identical sequences were shared among C. quercinus, C. marmoreus, C. litteratus, and C. ebraeus, reflecting the complexity of gene origin and evolution in this genus.
The proteomic sequences reflect the final secreted conopeptides. Using the proteome analysis in combination with transcriptome analysis, we identified 10 unique conopeptides at the protein level, encompassing five conopeptide gene superfamilies: F, A, M, Que-MNCLQ, and Que-MAMNV. The cysteine framework CC-C-C-C-C-C-CC-C-C-C-C-C was identified for the first time in C. quercinus at both the mRNA and the protein levels. The Que-0.1 precursor, which contained seven pairs of disulfide bonds and which was found in all the three individuals, was used for the functional study.
The in vitro preparation of functionally active conopeptides containing multiple pairs of disulfide bonds is challenging (Heimer et al., 2018). Here, we successfully generated the conopeptide Que-0.1 for the first time by constructing an expression system using the tag TrxA. TrxA plays a key role in the oxidative folding of Que-0.1, which is biologically active due to its seven pairs of disulfide bonds. Via a series of trials, we successfully optimized the parameters for efficient induction and purification of recombinant Que-0.1. Further explorations of Que-0.1 activity using the animal models showed that Que-0.1 inhibited neural signal transduction at low concentrations. Specifically, the inhibitory effects of laboratory-generated Que-0.1 on neural signal transduction were more than six times stronger than those of pethidine hydrochloride. This suggests that Que-0.1 may represent a potential biological anesthetic derived from the natural products.
At present, the analgesic drugs used in clinic, such as morphine, pethidine, and dizocine, have side effects, such as easy addiction and poisoning, while conotoxin has the advantages of novel structure, high diversity, strong activity, strong specificity, non-addiction, and few side effects (Duda and Palumbi, 2000; Luo et al., 2006; Pi et al., 2006; Hu et al., 2012; Terlau and Olivera, 2017; Shen et al., 2019). It can specifically bind to a variety of membrane proteins and has become an important treasure house in the field of analgesic research and development (Elisabetta et al., 2017; Gao et al., 2017; Shen et al., 2019). For example, Ziconotide, an analgesic drug listed in the United States, can specifically target Ca2+ channel (Cav2.2) (Wermeling, 2005). Other clinical pipelines, such as conotoxin κ-PVIIA (CGX-1051) targeting K+ channel (Kv1) (Kwon et al., 2016) and conotoxin αO-conotoxin GeXIV targeting α9α10 nicotinic acetylcholine receptor (Zhangsun et al., 2017). However, ω-CVID targeting Ca2+ channel (Cav2.2) has completed a preclinical study, but it is temporarily in phase II observation test due to high cytotoxicity (Adams et al., 2003). In the future conotoxin research, the cytotoxicity of conotoxin Que-0.1 and animal safety test can be carried out. In addition, the conotoxin Que-0.1 may contain seven pairs of disulfide bonds, while the other four conotoxins (κ-PVIIA, Kwon et al., 2016; GeXIV, Zhangsun et al., 2017; and ω-CVID Adams et al., 2003) have only three pairs of disulfide bonds. Disulfide connectivity in the peptides bearing intramolecular disulfide bonds is highly important for the structure and the biological activity of the peptides (Massonnet et al., 2018). It may be that Que-0.1 is more stable and can prevent degradation during in vivo administration. The Que-0.1 has better analgesic effect and lower dosage than the clinical anesthetics pethidine in this study. It may be developed into the analgesic drugs for chronic pain and neuropathic pain in the future.
In summary, this study updated our knowledge of the Conus toxins, not only deepening our understanding of conopeptide diversity, but also providing a valuable framework for the future investigations of conotoxin activity. Analgesia has been a long-term effort of humankind, but there is long way to go. The discovery of neuroblocking conotoxin Que-0.1 is helpful to identify its specific analgesic molecular targets.
Materials and Methods
RNA Extraction
Three adult specimens of C. quercinus were collected from the South China Sea. The venom ducts were immediately removed from each specimen and designated Que-1-VD, Que-2-VD, and Que-3-VD. Each tissue sample was separated into two parts and stored at −80°C until use. RNA was extracted from one part of each sample, while the remainder was used for LC-MS/MS. Total RNA was extracted using TRIzol, following the instructions of the manufacturer.
Transcriptome Sequencing and Bioinformatics Analysis
The transcriptomes of the C. quercinus species were sequenced on an Illumina HiSeq platform (125-bp paired-end, Illumina Inc., San Diego, CA, United States). The raw data were filtered using the NGS QC Toolkit (Patel and Jain, 2012) and Trimmomatic-0.60 (Bolger et al., 2014). The filtering criteria and analysis pipeline have been described previously (Puillandre et al., 2012).
Screening New Conotoxins With ConoSorter
ConoSorter is a machine learning program for the large-scale identification and classification of conopeptide precursors (Lavergne et al., 2013). The analysis process was as follows: first, ConoSorter was used to analyze the unique transcripts directly. Sequences 40–147 amino acids long were selected. The predicted conopeptide precursors with an N-ter hydrophobicity rate ≥ 65% were selected as candidate conopeptides for further analysis. Finally, according to the prediction of ConoServer, the sequence without signal peptide was removed.
Protein Fractionation and Preparation for Liquid Chromatography-Mass Spectrometry/Mass Spectrometry Analysis
The venom duct samples were stored at −80°C. The sample preparation and analysis methods were preformed following the protocols of the Thermo Scientific EASY-nLC 1000 system, the Thermo LTQ Orbitrap Elite mass spectrometer, and our previously published studies (Puillandre et al., 2012). The sample was solubilized in lysis buffer (8 M urea, pH 8.00) containing 5 mM DTT at 60°C for 45 min, followed by alkylation at 25°C for 45 min in the presence of 25 mM iodoacetamide in the dark. The obtained protein solutions were reconstituted in 50 mM ammonium bicarbonate with 0.5 M urea, pH 7.8, and digested (trypsin: protein = 1:100) for 12 h at 37°C. The pH of the samples was adjusted using a 0.1% formic acid solution before MS sequencing. The test time for each sample was 50 min.
Proteomic Data Analysis
The peak lists were generated and the sequences were identified by searching the Thermo LTQ Orbitrap Elite mass spectra against candidate conopeptide databases using the Thermo Scientific Proteome Discoverer Version 1.3.0.339 (Supplementary Table 2). For the alkylated samples, the fixed modification was set as carbamidomethyl (+57.021 Da). The MS instrument was Collision-induced dissociation- Ion Trap Mass Spectrometer (CID-ITMS), the precursor tolerance was 20 ppm, and the fragment tolerance was 20 ppm. The dynamic modification was set to oxidation (+15.995 Da, methionine). The maximum modifications per peptide were set to 4, the precursor mass tolerance was set to 20 ppm, the fragment mass tolerance was set to 20 ppm, up to 3 missed cleavages were allowed, the enzyme was trypsin, the FDR was set to 1%, the peptide mass was 600–10,000 Da, and the peptide length was 6–100 amino acids.
Phylogenetic Tree
The signal sequences of the 27 superfamilies in ConoServer (A, B1, B2, B3, C, D, E, F, G, H, I1, I2, I3, J, K, L, M, N, O1, O2, O3, P, Q, S, T, V, and Y) as well as the 13 temporary gene superfamilies were referenced (Liu et al., 2012; Bernáldez et al., 2013; Safavi-Hemami et al., 2014; Lavergne et al., 2015). In addition, the signal sequences from the 35 novel superfamilies were described over the past three years (Duda and Palumbi, 2000; Luo et al., 2006; Pi et al., 2006), and those from the three newly identified precursor conopeptides were added. The amino acid sequences were aligned by CLUSTALW 2.1 software.2 All the distances were between 0.0 and 1.0. We chose PHYLP Neighbor Joining as tree building method in the UGENE1.25 software.3
Recombinant Expression of the Que-0.1 Conotoxin
The Que-0.1 conopeptide was prepared using the genetic engineering. The codon of the toxin gene was optimized based on the nucleotide sequence of the conotoxin gene encoding the mature peptide, in combination with the polyclonal site of PET32a (+) vector and the restriction endonuclease site of toxin gene. The toxin gene was short and was synthesized using the direct synthesis method. Ni2 + column affinity purification was performed using the ATAK Chromatographic system (chromatographic column: Chelating Sepharose Fast Flow, Sephadex G25, Sephadex G-50, Amersham Pharmacia Biotech, Amersham, United Kingdom). The Que-0.1 gene was sequenced using the Applied BioSystems 3730xl DNA Analyzer (Applied Biosystems, Carlsbad, CA, United States). The sequencing results were viewed using UGENE-1.25.0 (see text footnote 3). The basic procedure for induced expression and product purification of the recombinant Que-0.1 was as follows. (1) Single colonies were cultured in 15 ml 2YT medium and vibrated at 37°C for 12 h as seed. They were inoculated in 1 L 2YT medium at the ratio of 1:50 and cultured at 37°C until the OD value reached at 0.6–0.7. The stock solution 24 mg/ml IPTG and 5% glucose solution were separately added to the culture under the ratio of 1:1,000 and cultured at 18°C for 10 h. (2) The bacteria were collected after centrifugation at 4°C at 8,000 rpm at 4°C for 10 min. The ultrasonic supernatant was collected after crushing the bacteria at 4°C, followed by 12,000 rpm centrifugation for 20 min. (3) Cleaned the ethanol-sealed Ni2+-Chelating Sepharose affinity chromatography column (GE AKTA explorer100, GE Electric Company, MA, United States) with five times the column bed volume of deionized water, bound the Ni2+ to the column matrix with two times the column bed volume of 0.2 M NiSO4, washed with 10 times the column bed volume of deionized water to remove the free NiSO4, and balance the column with an Ultrasonic Buffer (50 mM Tris, 0.5 M NaCl, 20 mM imidazole, and pH 8.0) at five times the column bed volume (4). The supernatant after ultrasound treatment and centrifugation was loaded on the column and washed with ultrasonic buffer until the UV absorption value reached the baseline, then the gradient elution was carried out with the Elution Buffer (50 mM Tris, 0.5 M NaCl, 0.5 M imidazole, and pH8.0). The solutions of the cross-flow peaks and elution peaks were collected for SDS-PAGE detection. (5) For the fusion polypeptide solution obtained using Ni2+ affinity chromatography, a 3 K ultrafiltration tube was used to replace the original buffer with EK enzyme digestion buffer, during which the rotational speed did not exceed 7,500 rpm/min. The volume ratio of the EK enzyme to the fusion peptide solution was 1:100, and the digestion was performed at room temperature for 18 h. (6) The digested product was loaded to a fresh prepared column, and the second round of affinity purification was carried out by repeating the step 3–step 4 above. (7) The crude product obtained above was passed through a C18 reversed-phase column for RP-HPLC detection, during which the salt ions and other miscellaneous peptides and proteins were removed simultaneously. The volume of each sample was 50–100 μl. Linear gradient elution was performed for 30 min using solution A (deionized water + 0.1% TFA) and solution B (acetonitrile + 0.1% TFA): solution A, 95– > 5%; solution B, 5– > 95%. Double wavelength detection was performed at 215 and 280 nm, and the elution peaks were collected for MS detection. After RP-HPLC purification, the collected polypeptide peaks were again vacuum freeze-dried and stored at −20°C until use. After preliminary purification, the Que-0.1 conopeptide was analyzed using LC-MS-MS.
Bullfrog Sciatic Nerve Bioelectric Activity
In this experiment, the bullfrog sciatic nerve bioelectric activitywas used to detect whether the conotoxin blocked neuromuscularconduction (Cymerman and Gottlieb, 1970; Gaffey and Tenforde, 1983). The bullfrog sciatic nerve was prepared as follows: decapitation, destruction of spinal cord, stripping of specimen hind limb, separation of two hind limbs, and examination of specimen. During the specimen preparation, the peeled specimen was frequently moistened with Ren’s solution. The sciatic nerve of the prepared specimen was gently touched with the two poles of the zinc copper arch. If the gastrocnemius muscle contracted immediately, we considered the specimen to have good excitability. Next, the specimen was transferred to Ren’s solution, and the experiment was performed after the excitability of the specimen stabilized. The tension transducer was connected to the root muscle of the gastrocnemius tendon, and the sciatic nerve was placed on the stimulation electrode. The tension transducer and the stimulation electrode were connected to a BL-420S biological function experimental system. To assess the nerve blocking abilities of recombinant Que-0.1, we recorded the signals using the BL-420 biological signaling system. The animals used were bullfrogs purchased at the university market. When the toxin was given, 6–10 electrical stimulations were performed, and at least six signals were collected each time. The time interval between the electrical stimulations was 0.8 s, and the peak was recorded each time. All the electrical stimulations for each model were completed within 5 min. The concentration of the conotoxin was 0.5 μg/μl. The dosage was 50 μl. To calculate the experimental results, at least five peaks were collected, and the maximum of each peak was extracted. The peak represents the maximum pulling force, and the average value of the peak was calculated. The inhibition rate of the neural circuit was calculated as (mean value after administration/mean value before administration) × 100%. The recovery rate of the neural circuit after drug removal was calculated as (mean value after drug removal/mean value before drug administration) × 100% - inhibition of the neural circuit.
Data Availability Statement
Raw read data are available at the National Center for Biotechnology Information Sequence Read Archive (https://www.ncbi.nlm.nih.gov/sra, Accession Numbers: SRR15195159, SRR15195160, SRR15195161). Proteomic raw data were submitted to ProteomeXchange via the PRIDE database (http://proteomecentral.proteomexchange.org/, PXD027478).
Author Contributions
HZ, LW, XW, and XP designed the research. HZ, XY, and YQ performed the research. HZ, YQ, and ZD analyzed the data. HZ, XW, and XP wrote the manuscript. All authors have read and agreed to the published version of the manuscript.
Funding
This work was in part supported by the grants from the National Key R&D Program of China (2018YFA0507800), the National Nature Science Foundation of China (81770173), the Guangdong Natural Science Foundation (2018B030308004), the Open Fund Programs of Shenzhen Bar Laboratory (SZBL2020090501003), and the Pearl River Talents Program Local Innovative and Research Teams (2017BT01S131). We are grateful to the Sun Yat-sen University and the Shenzhen Science and Technology Industry and Information committee (ZD20111108120A) for partial material support.
Conflict of Interest
The authors declare that the research was conducted in the absence of any commercial or financial relationships that could be construed as a potential conflict of interest.
Publisher’s Note
All claims expressed in this article are solely those of the authors and do not necessarily represent those of their affiliated organizations, or those of the publisher, the editors and the reviewers. Any product that may be evaluated in this article, or claim that may be made by its manufacturer, is not guaranteed or endorsed by the publisher.
Acknowledgments
We thank LetPub (www.letpub.com) for its linguistic assistance during the preparation of this manuscript.
Supplementary Material
The Supplementary Material for this article can be found online at: https://www.frontiersin.org/articles/10.3389/fmars.2021.766792/full#supplementary-material
Footnotes
- ^ http://www.conoserver.org
- ^ http://www.clustal.org/download/current/clustalw-2.1.tar.gz
- ^ http://ugene.net/
References
Adams, D. J., Smith, A. B., Schroeder, C. I., Yasuda, T., and Lewis, R. J. (2003). Omega-conotoxin CVID inhibits a pharmacologically distinct voltage-sensitive calcium channel associated with transmitter release from preganglionic nerve terminals. J. Biol. Chem. 278, 4057–4062. doi: 10.1074/jbc.M209969200
Aguilar, M. B., Ortiz, E., Kaas, Q., López-Vera, E., Becerril, B., Possani, L. D., et al. (2013). Precursor De13.1 from conus delessertii defines the novel G gene superfamily. Peptides 41, 17–20. doi: 10.1016/j.peptides.2013.01.009
Barghi, N., Concepcion, G. P., Olivera, B. M., and Lluisma, A. O. (2015). High conopeptide diversity in conus tribblei revealed through analysis of venom duct transcriptome using two high-throughput sequencing platforms. Mar. Biotechnol. 17, 81–98. doi: 10.1007/s10126-014-9595-7
Bernáldez, J., Román-González, S. A., Martínez, O., Jiménez, S., Vivas, O., Arenas, I., et al. (2013). A conus regularis conotoxin with a novel eight-cysteine framework inhibits Cav2.2 channels and displays an anti-nociceptive activity. Mar. Drugs 11, 1188–1202. doi: 10.3390/md11041188
Biass, D., Dutertre, S., Gerbault, A., Menou, J.-L., Offord, R., Favreau, P., et al. (2009). Comparative proteomic study of the venom of the piscivorous cone snail conus consors. J. Proteom. 72, 210–218. doi: 10.1016/j.jprot.2009.01.019
Biggs, J. S., Watkins, M., Puillandre, N., Ownby, J.-P., Lopez-Vera, E., Christensen, S., et al. (2010). Evolution of conus peptide toxins: analysis of conus californicus reeve, 1844. Mol. Phylogenet. Evol. 56, 1–12. doi: 10.1016/j.ympev.2010.03.029
Bolger, A. M., Lohse, M., and Usadel, B. (2014). Trimmomatic: a flexible trimmer for illumina sequence data. Bioinformatics 30, 2114–2120.
Cymerman, A., and Gottlieb, S. F. (1970). Effects of increased oxygen tensions on bioelectric properties of frog sciatic nerve. Aerospace Med. 41, 36–39.
Dao, F. Y., Yang, H., Su, Z. D., Yang, W., Wu, Y., Hui, D., et al. (2017). Recent advances in conotoxin classification by using machine learning methods. Molecules 22:1057.
Davis, J., Jones, A., and Lewis, R. J. (2009). Remarkable Inter- and intra-species complexity of conotoxins revealed by Lc/Ms. Peptides 30, 1222–1227. doi: 10.1016/j.peptides.2009.03.019
Duda, T. F., and Palumbi, S. R. (2000). Evolutionary diversification of multigene families: allelic selection of toxins in predatory cone snails. Mol. Biol. Evol. 17, 1286–1293. doi: 10.1093/oxfordjournals.molbev.a026412
Dutertre, S., Jin A-h, Kaas, Q., Jones, A., Alewood, P. F., and Lewis, R. J. (2013). Deep venomics reveals the mechanism for expanded peptide diversity in cone snail venom. Mol. Cell. Proteom. MCP 12, 312–329. doi: 10.1074/mcp.M112.021469
Elisabetta, T., Boni, R., and Gallo, A. (2017). micro-Conotoxins modulating sodium currents in pain perception and transmission: a therapeutic potential. Mar. Drugs 15:295. doi: 10.3390/md15100295
Espiritu, D. J., Watkins, M., Dia-Monje, V., Cartier, G. E., Cruz, L. J., and Olivera, B. M. (2001). Venomous cone snails: molecular phylogeny and the generation of toxin diversity. Toxicon 39, 1899–1916.
Fu, S., Tarone, A. M., and Sze, S.-H. (2015). Heuristic pairwise alignment of de bruijn graphs to facilitate simultaneous transcript discovery in related organisms from Rna-Seq data. BMC Genomics 16(Suppl. 11):S5. doi: 10.1186/1471-2164-16-S11-S5
Fu, Y., Li, C., Dong, S., Wu, Y., Zhangsun, D., and Luo, S. (2018). Discovery methodology of novel conotoxins from conus species. Mar. Drugs 16:E417.
Gaffey, C. T., and Tenforde, T. S. (1983). Bioelectric properties of frog sciatic nerves during exposure to stationary magnetic fields. Radiation Environ. Biophys. 22, 61–73. doi: 10.1007/BF01323761
Gao, B., Peng, C., Yang, J., Yi, Y., Zhang, J., and Shi, Q. (2017). Cone snails: a big store of conotoxins for novel drug discovery. Toxins 9:397. doi: 10.3390/toxins9120397
Hebert, P. D. N., Ratnasingham, S., and deWaard, J. R. (2003). Barcoding animal life: cytochrome c oxidase subunit 1 divergences among closely related species. Proc. Biol. Sci. 270(Suppl. 1), S96–S99.
Heimer, P., Schmitz, T., Bäuml, C. A., and Imhof, D. (2018). Synthesis and structure determination of μ-Conotoxin PIIIA isomers with different disulfide connectivities. J. Visual. Exp. JoVE 140, 58368. doi: 10.3791/58368
Hu, H., Bandyopadhyay, P. K., Olivera, B. M., and Yandell, M. (2012). Elucidation of the molecular envenomation strategy of the cone snail conus geographus through transcriptome sequencing of its venom duct. BMC Genomics 13:284. doi: 10.1186/1471-2164-13-284
Jin, A.-H., Vetter, I., Himaya, S. W. A., Alewood, P. F., Lewis, R. J., and Dutertre, S. (2015). Transcriptome and proteome of conus planorbis identify the nicotinic receptors as primary target for the defensive venom. Proteomics 15, 4030–4040. doi: 10.1002/pmic.201500220
Kaas, Q., Westermann, J.-C., and Craik, D. J. (2010). Conopeptide characterization and classifications: an analysis using conoserver. Toxicon 55, 1491–1509. doi: 10.1016/j.toxicon.2010.03.002
Kaas, Q., Westermann, J.-C., Halai, R., Wang, C. K. L., and Craik, D. J. (2008). Conoserver, a database for conopeptide sequences and structures. Bioinformatics 24, 445–446.
Kaas, Q., Yu, R., Jin, A.-H., Dutertre, S., and Craik, D. J. (2012). Conoserver: updated content, knowledge, and discovery tools in the conopeptide database. Nucleic Acids Res. 40, D325–D330. doi: 10.1093/nar/gkr886
Kwon, S., Bosmans, F., Kaas, Q., Cheneval, O., Conibear, A. C., Rosengren, K. J., et al. (2016). Efficient enzymatic cyclization of an inhibitory cystine knot-containing peptide. Biotechnol. Bioeng. 113, 2202–2212. doi: 10.1002/bit.25993
Lavergne, V., Dutertre, S., Jin A-h, Lewis, R. J., Taft, R. J., and Alewood, P. F. (2013). Systematic interrogation of the conus marmoreus venom duct transcriptome with conosorter reveals 158 novel conotoxins and 13 new gene superfamilies. BMC Genomics 14:708. doi: 10.1186/1471-2164-14-708
Lavergne, V., Harliwong, I., Jones, A., Miller, D., Taft, R. J., and Alewood, P. F. (2015). Optimized deep-targeted proteotranscriptomic profiling reveals unexplored conus toxin diversity and novel cysteine frameworks. Proc. Natl. Acad. Sci. U S A. 112, E3782–E3791.
Li, Q., Barghi, N., Lu, A., Fedosov, A. E., Bandyopadhyay, P. K., Lluisma, A. O., et al. (2017). Divergence of the venom exogene repertoire in two sister species of turriconus. Genome Biol. Evol. 9, 2211–2225. doi: 10.1093/gbe/evx157
Li, X., Chen, W., Zhangsun, D., and Luo, S. (2020). Diversity of conopeptides and their precursor genes of conus litteratus. Mar. Drugs 18:E464.
Liu, Z., Li, H., Liu, N., Wu, C., Jiang, J., Yue, J., et al. (2012). Diversity and evolution of conotoxins in conus virgo, conus eburneus, conus imperialis and conus marmoreus from the South China Sea. Toxicon 60, 982–989. doi: 10.1016/j.toxicon.2012.06.011
Lovelace, E. S., Armishaw, C. J., Colgrave, M. L., Wahlstrom, M. E., Alewood, P. F., Daly, N. L., et al. (2006). Cyclic mria: a stable and potent cyclic conotoxin with a novel topological fold that targets the norepinephrine transporter. J. Med. Chem. 49, 6561–6568. doi: 10.1021/jm060299h
Lu, A., Yang, L., Xu, S., and Wang, C. (2014). Various conotoxin diversifications revealed by a venomic study of conus flavidus. Mol. Cell. Proteom. MCP 13, 105–118. doi: 10.1074/mcp.M113.028647
Luo, S., Christensen, S., Zhangsun, D., Wu, Y., Hu, Y., Zhu, X., et al. (2013). A novel inhibitor of A 9α10 nicotinic acetylcholine receptors from conus vexillum delineates a new conotoxin superfamily. PLoS One 8:e54648. doi: 10.1371/journal.pone.0054648
Luo, S., Zhangsun, D., Zhang, B., Quan, Y., and Wu, Y. (2006). Novel alpha-conotoxins identified by gene sequencing from cone snails native to hainan, and their sequence diversity. J. Peptide Sci. 12, 693–704.
Massonnet, P., Haler, J., Upert, G., Smargiasso, N., Mourier, G., Gilles, N., et al. (2018). Disulfide connectivity analysis of peptides bearing two intramolecular disulfide bonds using MALDI In-Source decay. J. Am. Soc. Mass Spectrometry 29, 1995–2002. doi: 10.1007/s13361-018-2022-y
Miljanich, G. P. (2004). Ziconotide: neuronal calcium channel blocker for treating severe chronic pain. Curr. Med. Chem. 11, 3029–3040. doi: 10.2174/0929867043363884
Nam, H. H., Corneli, P. S., Watkins, M., Olivera, B., and Bandyopadhyay, P. (2009). Multiple genes elucidate the evolution of venomous snail-hunting Conus species. Mol. Phylogenet. Evol. 53, 645–652. doi: 10.1016/j.ympev.2009.07.013
Paczkowski, F. A., Sharpe, I. A., Dutertre, S., and Lewis, R. J. (2007). Chi-Conotoxin and tricyclic antidepressant interactions at the norepinephrine transporter define a new transporter model. J. Biol. Chem. 282, 17837–17844. doi: 10.1074/jbc.M610813200
Pakkala, M., Weisell, J., Hekim, C., Vepsäläinen, J., Wallen, E. A. A., Stenman, U.-H., et al. (2010). Mimetics of the disulfide bridge between the N- and C-Terminal cysteines of the Klk3-Stimulating Peptide B-2. Amino Acids 39, 233–242. doi: 10.1007/s00726-009-0433-6
Patel, R. K., and Jain, M. (2012). Ngs Qc toolkit: a toolkit for quality control of next generation sequencing data. PLoS One 7:e30619. doi: 10.1371/journal.pone.0030619
Peng, C., Yao, G., Gao, B.-M., Fan, C.-X., Bian, C., Wang, J., et al. (2016). High-Throughput identification of novel conotoxins from the chinese tubular cone snail (conus betulinus) by multi-transcriptome sequencing. GigaScience 5:17. doi: 10.1186/s13742-016-0122-9
Phuong, M. A., Mahardika, G. N., and Alfaro, M. E. (2016). Dietary breadth is positively correlated with venom complexity in cone snails. BMC Genomics 17:401. doi: 10.1186/s12864-016-2755-6
Pi, C., Liu, J., Peng, C., Liu, Y., Jiang, X., Zhao, Y., et al. (2006). Diversity and evolution of conotoxins based on gene expression profiling of conus litteratus. Genomics 88, 809–819.
Prashanth, J. R., Dutertre, S., Jin, A. H., Lavergne, V., Hamilton, B., Cardoso, F. C., et al. (2016). The role of defensive ecological interactions in the evolution of conotoxins. Mol. Ecol. 25, 598–615. doi: 10.1111/mec.13504
Puillandre, N., Bouchet, P., Duda, T. F. Jr., Kauferstein, S., Kohn, A. J., Olivera, B. M., et al. (2014a). Molecular phylogeny and evolution of the cone snails (Gastropoda, Conoidea). Mol. Phylogenet. Evol. 78, 290–303.
Puillandre, N., Stöcklin, R., Favreau, P., Bianchi, E., Perret, F., Rivasseau, A., et al. (2014b). When everything converges: integrative taxonomy with shell, DNA and venomic data reveals Conus conco, a new species of cone snails (Gastropoda: Conoidea). Mol. Phylogenet. Evol. 80, 186–192. doi: 10.1016/j.ympev.2014.06.024
Puillandre, N., Koua, D., Favreau, P., Olivera, B. M., and Stöcklin, R. (2012). Molecular phylogeny, classification and evolution of conopeptides. J. Mol. Evol. 74, 297–309.
Safavi-Hemami, H., Gajewiak, J., Karanth, S., Robinson, S. D., Ueberheide, B., Douglass, A. D., et al. (2015). Specialized insulin is used for chemical warfare by fish-hunting cone snails. Proc. Natl. Acad. Sci. U S A. 112, 1743–1748. doi: 10.1073/pnas.1423857112
Safavi-Hemami, H., Hu, H., Gorasia, D. G., Bandyopadhyay, P. K., Veith, P. D., Young, N. D., et al. (2014). Combined proteomic and transcriptomic interrogation of the venom gland of conus geographus uncovers novel components and functional compartmentalization. Mol. Cell. Proteom. MCP 13, 938–953. doi: 10.1074/mcp.M113.031351
Shen, H., Liu, D., Wu, K., Lei, J., and Yan, N. (2019). Structures of human Nav1.7 channel in complex with auxiliary subunits and animal toxins. Science 363, 1303–1308. doi: 10.1126/science.aaw2493
Terlau, H., and Olivera, B. M. (2017). Conus venoms: a rich source of novel ion channel-targeted peptides. Physiol. Rev. 84, 41–68. doi: 10.1152/physrev.00020.2003
Then, J., Wei, R., Oeser, T., Gerdts, A., Schmidt, J., Barth, M., et al. (2016). A disulfide bridge in the calcium binding site of a polyester hydrolase increases its thermal stability and activity against polyethylene terephthalate. FEBS Open Bio 6, 425–432. doi: 10.1002/2211-5463.12053
Violette, A., Biass, D., Dutertre, S., Koua, D., Piquemal, D., Pierrat, F., et al. (2012). Large-Scale discovery of conopeptides and conoproteins in the injectable venom of a fish-hunting cone snail using a combined proteomic and transcriptomic approach. J. Proteom. 75, 5215–5225. doi: 10.1016/j.jprot.2012.06.001
Wan, J., Brust, A., Bhola, R. F., Jha, P., Mobli, M., Lewis, R. J., et al. (2016). Inhibition of the norepinephrine transporter by X -conotoxin dendrimers. J. Pept. Sci. 22, 280–289. doi: 10.1002/psc.2857
Wermeling, D. P. (2005). Ziconotide, an intrathecally administered N-Type calcium channel antagonist for the treatment of chronic pain. Pharmacotherapy 25, 1084–1094. doi: 10.1592/phco.2005.25.8.1084
Yao, G., Peng, C., Zhu, Y., Fan, C., Jiang, H., Chen, J., et al. (2019). High-Throughput identification and analysis of novel conotoxins from three vermivorous cone snails by transcriptome sequencing. Mar. Drugs 17:E193. doi: 10.3390/md17030193
Ye, M., Khoo, K. K., Xu, S., Zhou, M., Boonyalai, N., Perugini, M. A., et al. (2012). A helical conotoxin from conus imperialis has a novel cysteine framework and defines a new superfamily. J. Biol. Chem. 287, 14973–14983. doi: 10.1074/jbc.M111.334615
Zhang, H., Fu, Y., Wang, L., Liang, A., Chen, S., and Xu, A. (2019). Identifying novel conopepetides from the venom ducts of conus litteratus through integrating transcriptomics and proteomics. J. Proteom. 192, 346–357. doi: 10.1016/j.jprot.2018.09.015
Zhangsun, D., Zhu, X., Kaas, Q., Wu, Y., Craik, D. J., and McIntosh, J. M. (2017). αO-Conotoxin GeXIVA disulfide bond isomers exhibit differential sensitivity for various nicotinic acetylcholine receptors but retain potency and selectivity for the human α9α10 subtype. Neuropharmacology 127, 243–252. doi: 10.1016/j.neuropharm.2017.04.015
Keywords: diversity, conopeptides, de novo sequencing, LC-MS/MS, pethidine hydrochloride
Citation: Zhang H, Wang L, Yang X, Lian Z, Qiu Y, Dong Z, Wu X and Pan X (2021) Identification of Novel Conopeptides and Distinct Gene Superfamilies in the Marine Cone Snail Conus quercinus. Front. Mar. Sci. 8:766792. doi: 10.3389/fmars.2021.766792
Received: 30 August 2021; Accepted: 07 October 2021;
Published: 12 November 2021.
Edited by:
Ana Rotter, National Institute of Biology (NIB), SloveniaReviewed by:
Angela Cuttitta, National Research Council, Consiglio Nazionale delle Ricerche (CNR), ItalyGianfranco Santovito, University of Padua, Italy
Copyright © 2021 Zhang, Wang, Yang, Lian, Qiu, Dong, Wu and Pan. This is an open-access article distributed under the terms of the Creative Commons Attribution License (CC BY). The use, distribution or reproduction in other forums is permitted, provided the original author(s) and the copyright owner(s) are credited and that the original publication in this journal is cited, in accordance with accepted academic practice. No use, distribution or reproduction is permitted which does not comply with these terms.
*Correspondence: Xuedong Wu, eHVlZG9uZ3d1QDE2My5jb20=; Xinghua Pan, UGFuVmljdG9yQHNtdS5lZHUuY24=