- 1Florida Fish and Wildlife Conservation Commission, Fish and Wildlife Research Institute, St. Petersburg, FL, United States
- 2Fisheries and Aquatic Sciences Program, University of Florida, Gainesville, FL, United States
- 3St. Johns River Water Management District, Palm Bay, FL, United States
- 4St. Johns River Water Management District, Palatka, FL, United States
- 5Florida Fish and Wildlife Conservation Commission, Fish and Wildlife Research Institute, Melbourne, FL, United States
The Indian River Lagoon (IRL), located on the east coast of Florida, is a complex estuarine ecosystem that is negatively affected by recurring harmful algal blooms (HABs) from distinct taxonomic/functional groups. Enhanced monitoring was established to facilitate rapid quantification of three recurrent bloom taxa, Aureoumbra lagunensis, Pyrodinium bahamense, and Pseudo-nitzschia spp., and included corroborating techniques to improve the identification of small-celled nanoplankton (<10 μm in diameter). Identification and enumeration of these target taxa were conducted during 2015–2020 using a combination of light microscopy and species-specific approaches, specifically immunofluorescence flow cytometry as well as a newly developed qPCR assay for A. lagunensis presented here for the first time. An annual bloom index (ABI) was established for each taxon based on occurrence and abundance data. Blooms of A. lagunensis (>2 × 108 cells L–1) were observed in all 6 years sampled and across multiple seasons. In contrast, abundance of P. bahamense, largely driven by the annual temperature cycle that moderates life cycle transitions and growth, displayed a strong seasonal pattern with blooms (105–107 cells L–1) generally developing in early summer and subsiding in autumn. However, P. bahamense bloom development was delayed and abundance was significantly lower in years and locations with sustained A. lagunensis blooms. Pseudo-nitzschia spp. were broadly distributed with sporadic bloom concentrations (reaching 107 cells L–1), but with minimal concentrations of the toxin domoic acid detected (<0.02 μg L–1). In summer 2020, multiple monitoring tools characterized a novel nano-cyanobacterium bloom (reaching 109 cells L–1) that coincided with a decline in A. lagunensis and persisted into autumn. Statistical and time-series analyses of this spatiotemporally intensive dataset highlight prominent patterns in variability for some taxa, but also identify challenges of characterizing mechanisms underlying more episodic yet persistent events. Nevertheless, the intersect of temperature and salinity as environmental proxies proved to be informative in delineating niche partitioning, not only in the case of taxa with long-standing data sets but also for seemingly unprecedented blooms of novel nanoplanktonic taxa.
Introduction
Harmful algal blooms (HABs) have occurred in the Indian River Lagoon (Florida, United States) for decades and can negatively affect water quality and ecosystem function and, in turn, hinder restoration efforts. Effects resulting from IRL HABs include low dissolved oxygen (Diaz and Rosenberg, 2008), decreases in light availability, losses of seagrass (Morris et al., 2018; Lapointe et al., 2020), fisheries closures related to toxins (Landsberg et al., 2006), and negative health effects on wildlife and shellfish resources (Gobler et al., 2013; Fire et al., 2015; Adams et al., 2019). Although blooms of harmful algae are not a recent development in the IRL, the past 10 years have exhibited a measurable shift to more frequent and higher biomass bloom events dominated by nano- and picoplankton (Phlips et al., 2021).
Recurring blooms of saxitoxin-producing Pyrodinium bahamense have caused regular shellfish harvest closures in the IRL system since the early 2000s (Anderson et al., 2021), and these blooms can reach high concentrations and often dominate summer algal biomass (Phlips et al., 2011). Blooms of diatoms from the genus Pseudo-nitzschia (some of which can produce the toxin domoic acid) can also sometimes reach concentrations greater than 106 cells L–1 in the IRL, but are rarely dominant in terms of biomass, and the only domoic acid-related closures of shellfish harvests in Florida have occurred in the Panhandle region (Bates et al., 2018). In the IRL in 2011, a still taxonomically unclassified small flagellate co-occurred with picocyanobacteria and exceeded 100 μg L–1 chlorophyll-a, which was higher than biomass levels typically observed during other blooms (SJRWMD, 2012). This “2011 superbloom” lasted 7 months and is thought to have contributed to the loss of over 40% of established seagrass beds in the area (SJRWMD, 2012; Lapointe et al., 2015; Phlips et al., 2015). Since then, high concentrations of other nanophytoplankton taxa (∼2–10 μm diameter) have been reported nearly annually and sometimes coincidentally or sequentially with other HABs, presenting new challenges to management, conservation, monitoring, and restoration efforts in the IRL (FWC-FWRI HAB Monitoring Database; Gobler and Sunda, 2012; Kang et al., 2015; Barile, 2018). Specifically, in 2012, the first documented brown tide caused by the nanophytoplankton Aureoumbra lagunensis further affected the northern IRL system with maximum recorded biomass of ∼200 μg L–1 chlorophyll-a surpassing values measured during the 2011 superbloom event. Subsequent brown tide events were recorded throughout the system in 2013 and from 2016 through 2020, causing further seagrass losses, extensive fish kills in 2016, and negative effects on seagrass-dependent fisheries and wildlife (Morris et al., 2018; Lapointe et al., 2020).
Pyrodinium bahamense can be readily identified by light microscopy, while Pseudo-nitzschia and A. lagunensis each present specific challenges for routine identification by light microscopy. The identification of the former is straightforward at the genus level by gross morphology (typically delineated by width and/or length), but electron microscopy and/or molecular tools are required to distinguish species; therefore, the preferred taxonomic designation Pseudo-nitzschia spp. is most commonly used. Teasing Aureoumbra cells apart from other non-descript spherical nanoplankton cells requires specialized expertise in identification using light microscopy along with secondary confirmation. To expand, light microscopy-based enumeration of A. lagunensis-like cells is feasible once it is documented to occur in a particular region, though it shares morphometric traits with other taxa, and it is critical that identification be verified by complementary techniques such as antigen- and nucleic acid-based detection methods specific to the species—for example in this study an immunofluorescent flow cytometry assay (Lopez-Barrerio et al., 1998; Koch et al., 2014) and by a qPCR assay presented here for the first time.
These HAB taxa, A. lagunensis (pelagophyte), P. bahamense (dinoflagellate), and Pseudo-nitzschia (diatom), belong to distinct taxonomic/functional phytoplankton groups, each with particular affinities and tolerances to myriad environmental parameters. Characterizing ecological niches that these HAB taxa occupy will help improve our predictive abilities and develop better management strategies (Karasiewicz et al., 2020). To that end, we explore a high resolution 6-year time series (2015–2020) of observations in different sub-basins of the IRL to portray a detailed picture of the fine-scale spatial and temporal variability in their occurrence and abundance. Hutchinson (1957) defined the “fundamental niche” as the “n-dimensional hypervolume” (based on n number of limiting factors) that a species can occupy, and described the “realized niche,” which we invoke here, as the portion of the fundamental niche that a species occupies in nature due to restrictions imposed by ecological interactions. Numerous factors, including temperature, salinity, nutrient concentrations, light availability, life cycle dynamics, grazing, water circulation patterns, and water residence times can all influence the distribution and proliferation of phytoplankton within the IRL (Badylak and Phlips, 2004; Hargraves, 2020). For the purpose of this observational study, however, we focus on quantifying relationships of taxon abundance and two easily measured primary predictors of phytoplankton distributions: temperature and salinity (Irwin et al., 2012). An advantage of of using these parameters to estimate niches is that they are routinely measured and generally available in real-time from continuous monitoring programs, so these characterizations may be more readily applied in various scenarios to meet management needs (Melo-Merino et al., 2020). From statistical relationships with each taxon, we can infer their distribution, or realized niche, as bounded by these environmental parameters in the IRL (Brun et al., 2015). We also interpret our results in the context of available information on life cycle dynamics, functional traits of taxonomic groups (such as nutrient affinity and flexibility), water residence time of basins, and large scale disturbances, such as hurricanes, and discuss how these factors may interact to modify these hypothesized niches (Karasiewicz et al., 2017) and perhaps create conditions within the IRL that may allow opportunistic, small-celled species to proliferate episodically relative to more predictable, and typically easier to identify, larger-celled species that have been tracked in the system for decades. This concept is particularly relevant given that, during this study, an unexpected bloom of a novel cyanobacterium began in the northern IRL in 2020. Due to our ongoing extensive sampling scheme and the suite of monitoring tools already in use for A. lagunensis, we were in a unique position to track the development and demise of this event that, although relatively short-lived and not originally part of our 6-year time-series, provided additional insights and hypotheses for future investigations of HAB dynamics in the IRL.
Materials and Methods
Study Area Description
The IRL, within the longest barrier island complex in the United States, comprises three distinct water bodies which include the Mosquito Lagoon, the Indian River Lagoon proper, and the Banana River Lagoon. Extending from Ponce de Leon Inlet in the Mosquito Lagoon south to Jupiter Inlet near West Palm Beach, this ∼250-km long estuary is a semi-enclosed water body with limited exchange of saline water from the Atlantic Ocean and freshwater from upland/riverine sources. This estuary harbors high biological diversity because of the unique transition zone between the region’s temperate and sub-tropical biological zones (Steward and Green, 2007). Ecologically distinct regions exist within the lagoon, and the salinity, tidal influence, and degree of flushing characteristic of a particular portion of the IRL is largely influenced by its distance from an inlet and by freshwater inputs from adjacent streams, rivers, ditches, and canals (Virnstein, 1990; Sheng and Davis, 2003; Steward et al., 2005). For our analyses, we focused on the IRL system north of Vero Beach and sub-divided the ∼170-km long study area into four, hydrologically distinct sub-basins (Kleppel, 1996; Phlips et al., 2002; Steward et al., 2005): Mosquito Lagoon, Banana River Lagoon, Northern IRL, and Central IRL (Figure 1). The sub-basins are shallow, with an average depth ranging from 0.8 m (Mosquito Lagoon) to 1.9 m (Northern IRL). There are two main connections between the Atlantic Ocean and the IRL system, at the Sebastian Inlet and, south of our study area, at the Ft. Pierce Inlet, and there is an intermittent inlet connection with the Banana River Lagoon through the Port Canaveral navigational lock (Figure 1). The northern IRL is also connected to the Mosquito Lagoon through the Haulover Canal and to the Banana River Lagoon through the Barge Canal and the southern opening of the Banana River Lagoon (Steward and Green, 2007; Morris et al., 2018). Of these sub-basins, Central IRL is characterized by the shortest flushing time (estimated < 10 days for 50% exchange), and the Banana River Lagoon by the longest (estimated ∼70–156 days for 50% exchange Kim, 2003; Steward et al., 2005; Reyier et al., 2008) based on their distance from the nearest inlets.
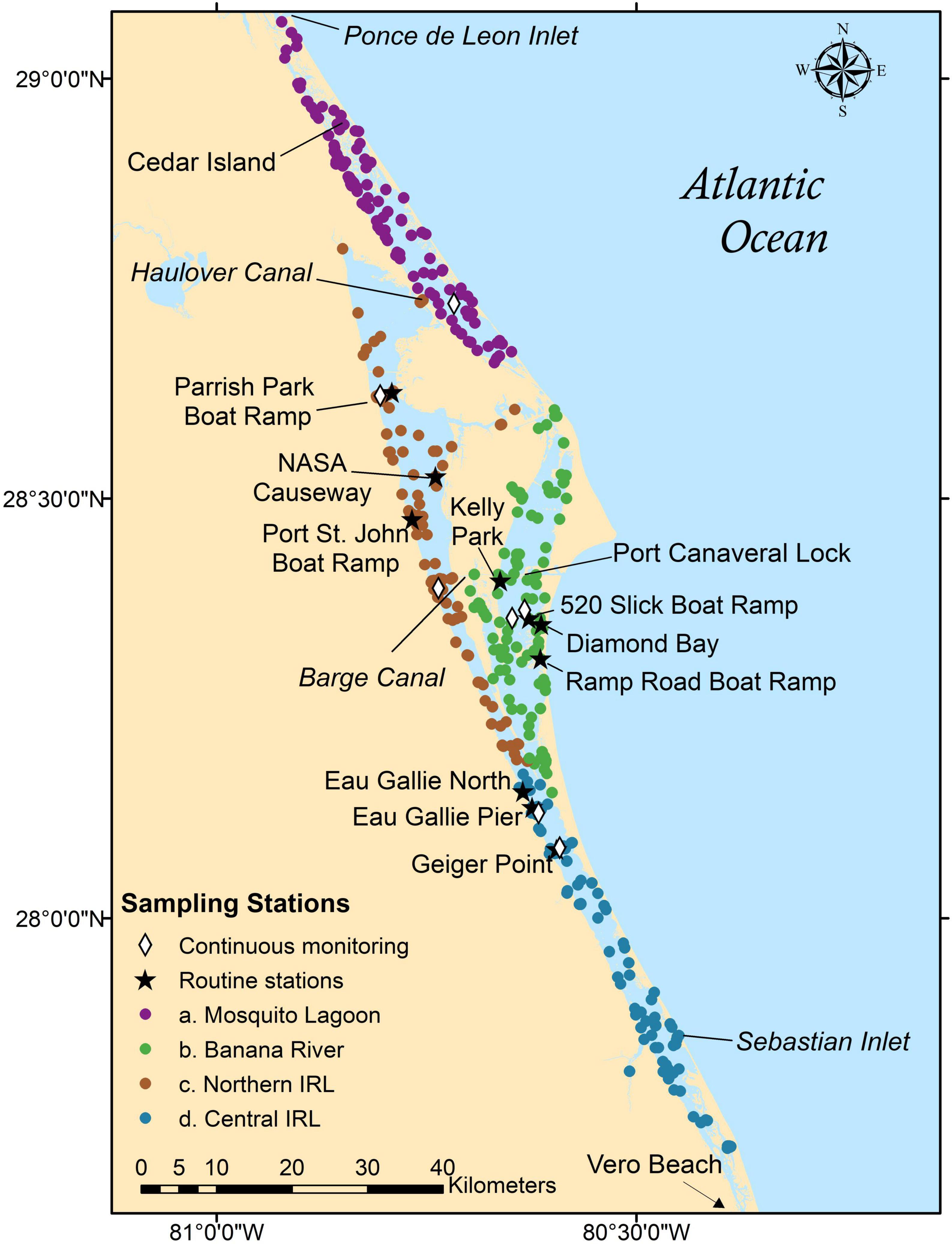
Figure 1. Map of the Indian River Lagoon (IRL) system showing the four sub-basins monitored: Mosquito Lagoon (purple), Banana River Lagoon (green), Northern IRL (brown), and Central IRL (blue). Filled circles represent all sampling locations over the 2015–2020 time period, and black stars represent the ten routine fixed stations that were sampled on a regular basis over the course of this time period (Parrish Park Boat Ramp, NASA Causeway, Port St. John Boat Ramp, Kelly Park, 520 Slick Boat Ramp, Diamond Bay, Ramp Road Boat Ramp, Eau Gallie North, Eau Gallie Pier, and Geiger Point). White diamonds represent the St. Johns River Water Management District continuous monitoring stations used in this study.
Sample Collection and Analysis
During 2015–2020, multiple partners and volunteers collected water samples for phytoplankton identification and enumeration at various time intervals and locations in the four sub-basins and, beginning in 2016, at weekly intervals at 10 routine fixed stations (Figure 1, Table 1, and Supplementary Table 1). Sample locations covered a broad spatial area and included both vessel-based and shore-based sampling. Live and Lugol’s preserved surface water samples (n = 4,417) were collected from discrete sample depths (98% of samples were collected from 0.1 to 1.0 m, Supplementary Table 1), and salinity and temperature were consistently recorded for a subset (n = 2,273) of these water samples (multiparameter sondes, YSI Inc./Xylem Inc.). Samples for microscopic analyses were collected into 125-mL amber NalgeneTM HDPE bottles and preserved in neutral Lugol’s solution at 2% final concentration. Live water samples were collected into 500-mL polypropylene or HDPE bottles. Live and Lugol’s preserved water samples were stored at ambient temperature and shipped overnight to FWC-Fish and Wildlife Research Institute (FWRI).
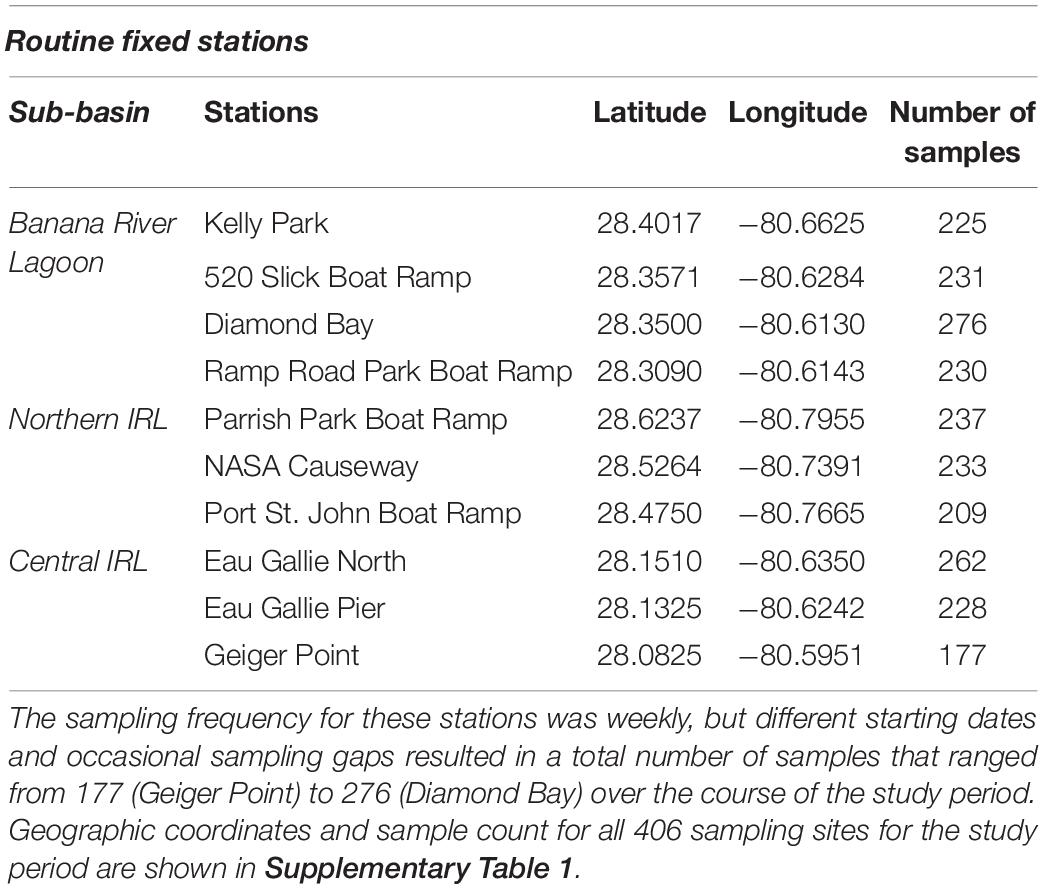
Table 1. The geographic coordinates of the 10 routine fixed stations monitored in the different sub-basins of the IRL are shown along with the total number of samples collected at each.
Lugol’s preserved water samples were analyzed by the FWC-FWRI HAB monitoring program using inverted light microscopes to identify and enumerate phytoplankton. Sub-samples (3 mL) were settled in Nunc® chambers (adapted from Edler and Elbrachter, 2010) and analyzed using a combination of bright field and phase contrast illuminations under 200× final magnification for P. bahamense and Pseudo-nitzschia spp., and 400–630× final magnification in targeted samples for nanoplankton and smaller taxa. All samples (n = 4,417) were inspected for P. bahamense and Pseudo-nitzschia spp.; for select samples (n = 2,992), FWC-FWRI also inspected for A. lagunensis-like cells by light microscopy based on size and gross morphology. For a subset of live samples, collection for definitive A. lagunensis identification (immuno-flow cytometry, qPCR) was conducted either at the time of sampling or once samples reached FWC-FWRI [see sections “Immunofluorescence flow cytometry for A. lagunensis” and “Quantitative Polymerase Chain Reaction (qPCR) for A. lagunensis”]. Domoic acid and saxitoxins were analyzed in target samples based on cell concentrations (see section “Particulate domoic acid in seawater” and “Particulate saxitoxins in seawater”).
Phytoplankton abundance data generated by the University of Florida (UF) were used to supplement FWC-FWRI data in Mosquito Lagoon to increase temporal coverage in that sub-basin. This subset of water samples (n = 141) was collected by UF and St. Johns River Water Management District (SJRWMD) using a depth-integrated vertical sampler (capturing water from the surface to 0.1 m from the bottom) and were quantified microscopically by UF as described in Phlips et al. (2010).
Daily mean air temperature and precipitation data were obtained from the Florida Climate Center1 and represent historical temperature and precipitation from National Weather Service (NWS) cooperative stations in Titusville, Florida (Coop IDs 088942, Titusville, and 088947, Titusville 7E). Continuous sensor-based monitoring data (EXO multiparameter sondes, YSI Inc.) for water temperature and salinity were obtained from the SJRWMD continuous monitoring stations2 in the Northern IRL (station IDs 33954622, 37375724), Mosquito Lagoon (station ID 33814526), Banana River Lagoon (station IDs 33844736, 33964621), and Central IRL (station IDs 34094983, 33944620). The continuous temperature and salinity data were used to compare trends among years and sub-basins and to calculate temperature-based predictions of P. bahamense germination dates. All other data analysis herein used discrete salinity and temperature measured upon water sample collection.
Immunofluorescence Flow Cytometry for A. lagunensis
FWC-FWRI water samples for identification of A. lagunensis via immunofluorescence flow cytometry (n = 561) were fixed and frozen within 6 h of sample receipt. Samples were pre-filtered through nylon mesh (with a 64-μm pore size) and then aliquots (1.2 mL) were preserved in polypropylene cryogenic storage vials (Corning or Nalgene, United States) in duplicate with a 1% final glutaraldehyde concentration. Additional samples for flow cytometry were collected by SJRWMD and UF (n = 341) via a vertically integrated water sampler; samples were preserved in the field in 1% glutaraldehyde in cryovials, stored on ice, and shipped overnight. All preserved samples were stored in the dark at 4°C until analysis.
For targeted detection of A. lagunensis, a specific antibody initially developed by Lopez-Barrerio et al. (1998) and later optimized for fluorometric flow cytometry (Gobler et al., 2013; Koch et al., 2014) was used. From 2015 to 2019, the FITC-conjugated primary antibody developed by Koch et al. (2014) was used (provided by C. Gobler, Stony Brook University), while for 2020, the original antiserum (provided by T. Villareal, University of Texas) was used to generate and purify additional conjugated antibody (Envigo, United States), following the methods in Koch et al. (2014).
All flow cytometric analyses were performed with an Accuri C6 (BD Biosciences, United States) or Attune NxT (ThermoFisher, United States) flow cytometer, both equipped with a 488-nm laser, and data were processed using FCS Express software (De Novo Software). Fluorescent antibody sample staining was conducted following Koch et al. (2014). Fixed and stained samples were shaken or mixed by aspiration immediately before analyzing at flow rates of 66 μL min–1 or 200 μL min–1 (Accuri C6 and Attune NxT, respectively). For data analysis, the following 3-parameter gating protocol, informed by additional validation with culture and field material (see Supplementary Figures 1, 2), was used: (1) cell size-selection proxy using forward light scatter (FSC) signal between 2.5 and 10 μm (typical of A. lagunensis), (2) green fluorescence signal (Accuri C6: 518–548 nm; Attune: 515–545 nm) to identify cells bound with the FITC-conjugated antibody, and (3) red autofluorescence signal (Accuri C6: > 670 nm Attune NxT: 675–715 nm) to identify cells based on chlorophyll-a fluorescence. The FSC size-proxy signal was calibrated against a polystyrene bead size standard (range 0.58–14.8 μm, Life Technologies & Spherotech Inc). Gating was always verified by eye before final estimation of A. lagunensis population abundance.
Quantitative Polymerase Chain Reaction for A. lagunensis
DNA from cultures and environmental samples was extracted using the QiaCube DNA extraction robot (Qiagen, Germany) with the Qiagen DNeasy Plant Mini Kits following the manufacturer’s instructions with minor modifications. Small volumes (typically 1–20 mL) of sample were filtered onto 0.45-μm pore-size 25-mm PVDF membrane filters (Millipore Sigma, United States) and frozen in cryovials (Nalgene, United States) at –80°C until extraction. Two minutes of bead beating at 50 Hz with ∼100 μL of a 1:1 mix of 0.1/0.5 mm glass beads (with a Tissue Lyser LT, Qiagen, Germany) in Qiagen’s lysis buffer (“AP1”) was used to maximize cell lysis. DNA was eluted into 150 μL of Qiagen AE buffer. Extraction efficiency and PCR inhibition were quantified by the addition of 2 ng of a synthetic DNA fragment (500 bp, random sequence) into the lysis buffer of 11 and 25 independent extractions, respectively.
For initial A. lagunensis qPCR-assay development, the 18S rRNA gene was sequenced in two cultured strains of A. lagunensis (CCMP1503 and UTEX LB 2796, hereafter UTEX2796), along with an archived sample collected from the Mosquito Lagoon during the 2012 brown tide event. Universal eukaryotic primers were used for bidirectional sequencing of unialgal cultures (Medlin et al., 1988; Weekers et al., 1994) at Eurofins Genomics (Eurofins Genomics, KY, United States). Global pairwise sequence alignment (1,577 bp each) between CCMP1503 and UTEX2796 was conducted using Needle (V6.6.0 EMBOSS, Rice et al., 2000) to quantify their percent sequence identity. This alignment was used to design specific qPCR primers (Supplementary Table 2), targeting a conserved region of the 18S rRNA gene. The forward primer was highly specific to A. lagunensis whereas the reverse primer was designed to be pelagophyte specific. Three additional field DNA samples from subsequent sampling in 2016, 2018, and 2020 were also directly sequenced from PCR products to re-confirm A. lagunensis identity and the specificity of newly designed primers by aligning newly obtained culture and field DNA sequences in Sequencher (Gene Codes Corporation, United States) along with closely related taxa (sequenced by FWC-FWRI or retrieved from NCBI GenBank). Primer specificity was further validated by screening culture and field DNA samples by PCR and agarose gel electrophoresis, and gradient PCRs were used to optimize annealing temperature. Assays for qPCR were performed in 10 μL reactions with 1X PowerUP SYBR Green master mix (Life Technologies, United States) on a QuantStudio 5 thermocycler with a 384-well block [93 environmental DNA (eDNA) samples] or on a Stratagene MX3005p with 96-well block (61 eDNA samples). Post-run analyses included an automated baseline subtraction and ROX normalization, with subsequent crossing point (Cq) value determination at a constant threshold across plates run on the same instrument. eDNA sample Cq values were converted to copies/reaction using a standard curve prepared using a 500-bp gBlock gene fragment (synthesized by IDT, United States). Copies per reaction were converted to A. lagunensis cells L–1 by accounting for all sample manipulations and using an empirically derived cellular rRNA copy number of 16 copies cell–1 and DNA extraction efficiency of 42%. The assay was finally evaluated by regressing qPCR-determined A. lagunensis abundance against counts made by immunofluorescence flow cytometry, and by microscopy. Furthermore, qPCR was used to assess whether the unusual insertion, present within the 18S rRNA gene of the isolate sequenced by DeYoe et al. (1997), was consistently present in two IRL eDNA samples from each of the following 3 years, 2016, 2018, and 2020. Lastly, RT-PCR using a Two-Step Cells-to-Ct SYBR Green Kit (Invitrogen, United States) and agarose gel electrophoresis assessed whether the insertion region was present in rRNA.
Initial Sequencing of a Novel Cyanobacterium
Direct PCR sequencing was applied to aid identification of the novel cyanobacterium that bloomed in summer–autumn 2020. Bloom samples collected at Parrish Park Boat Ramp and Eau Gallie Pier stations (Table 1) on September 10, 2020, were extracted in duplicate as described above for one replicate and without bead beating for the second replicate. Microscopic evaluation suggested this organism was a cyanobacterium based on autofluorescence. Accordingly, 16S rRNA genes were amplified using cyanobacteria-specific primer sets (Nübel et al., 1997): cya106F-cya781R(a) and cya359F-cya781R(b). PCR products were verified by gel electrophoresis, purified with Exo-SAP-IT Express reagent (Life Technologies, United States), and sent to Eurofins Genomics (Eurofins Genomics, KY, United States) for bi-directional Sanger sequencing. Subsequently, two new primers (nanocyano_p8_F and nanocyano_p8_R, Supplementary Table 2) were designed to be specific to the new sequence obtained from the cya359F-cya781R(b) amplicon. These primers were used in combination with a variety of universal 16S rRNA primers [8F and 1492R, Turner et al., 1999; 340R, Iteman et al., 2000; and a modified 16S-1247f, Rocap et al. (2002) wherein the antepenultimate base is an S instead of a C]. This sequence was subjected to BLASTn and SILVA database searches to identify the most closely related taxa. Finally, the putative novel cyanobacterium sequence abundance was compared to A. lagunensis abundance using qPCR for the Parrish Park Boat Ramp and Eau Gallie Pier samples.
Particulate Domoic Acid in Seawater
A select number of live water samples (n = 133, 200–250 mL), within those that reached the established operational bloom threshold for Pseudo-nitzschia spp. (see section “Data Analysis and Statistics”), were filtered onto Whatman® GF/F filters and placed into individual 15-mL polypropylene centrifuge tubes for domoic acid analysis. Domoic acid was extracted by adding 5 mL of 20% aqueous MeOH (ACS grade, Thermo Fisher Scientific, Waltham, MA, United States) to each tube, vortexing for 2 min, and centrifuging at 4°C and 3500 × g for 15 min. The supernatant was then transferred to a glass vial and stored in the dark at –20°C until analysis.
Extracts were filtered through 0.22-μm PVDF syringe filters and analyzed using an Acquity ultra-high performance liquid chromatographic (UPLC) system coupled to a Quattro MicroTM API triple quadrupole mass spectrometer (Waters, Milford, MA, United States). Separations were performed on an Acquity UPLC BEH C18 1.7-μm column (2.1 × 100 mm). The injection volume was 10 μL, with a flow rate of 0.4 mL min–1 and a column oven temperature of 40°C. Mobile phase A consisted of a 0.1% formic acid solution in water, and mobile phase B was acetonitrile with 0.1% formic acid (LC-MS grade, Thermo Fisher Scientific, Waltham, MA, United States). The initial gradient condition was 5% mobile phase B for 0.3 min, followed by a linear gradient to 40% B at 2.5 min before returning to initial conditions at 3 min and reconditioning the column for 1 min. The mass spectrometer was operated in positive ionization mode (ESI +) with the following parameters: capillary voltage 3.5 kV, cone voltage 28 V, desolvation temperature 500°C, and desolvation gas flow 850 L/h. Multiple reaction monitoring (MRM) transitions from the protonated domoic acid ion were monitored for the following transitions: m/z 312 > 193, m/z 312 > 248, and m/z 312 > 266; and the collision energies were optimized for each precursor/product pair. A certified reference standard solution of domoic acid (National Research Council, Halifax, Canada) was used to generate an 8-point standard curve for quantification of domoic acid.
Particulate Saxitoxins in Seawater
A select number of live water samples (n = 456) were filtered for saxitoxins onto Whatman® GF/D filters and placed into 15-mL polypropylene centrifuge tubes (Falcon brand, Corning, United States) at –20°C until further processing. Filters for samples collected in 2015 were thoroughly homogenized in a Tenbroeck grinder (7 mL) with 1 mL of 1% acetic acid, and the slurry was poured into a fresh 15-mL centrifuge tube, and 1 mL of 1% acetic acid was used to wash the grinder and combined with the slurry in the tube. Tubes were centrifuged at 4000 rpm (3600× g) for 10 min and supernatants were placed into cryovials. Filters for samples collected in 2016 were extracted either by freeze-boil or freeze-thaw methods; 2 mL of 1% acetic acid was added to the filters in centrifuge tubes, which were then vortexed for 30 s before being placed at –80°C. Tubes were removed from –80°C after 20 min and thawed in a boiling water bath or at room temperature for 10 min. After thawing, each tube was vortexed for 30 s before being placed at –80°C again. These steps were repeated three times before filtering the slurry using 0.45-μm nylon filters. Filters for samples collected from 2017 to 2020 were extracted in 7-mL Omni plastic tubes with ∼1.5 g of 2.8-mm diameter ceramic beads along with 2 mL of 1% acetic acid. These tubes were homogenized in a bead mill homogenizer (Bead Ruptor Elite, Omni International-PerkinElmer, Inc., United States) at a speed of 4 m s–1 for 3 cycles of 30 s with a dwell interval of 10 s between each cycle. Once homogenized, these tubes were centrifuged at 4000 rpm (3600× g) for 10 min and supernatant was transferred into a cryovial. All extracts were stored at –20°C until analysis and were analyzed within 1 y. Saxitoxin congeners (decarbamoyl saxitoxin, gonyautoxin-5, and saxitoxin) were analyzed by HPLC using the prechromatographic oxidation method described in Lawrence et al. (2005). Toxins were quantified by a 5-point calibration method using standards prepared with certified reference materials obtained from the National Research Council (Halifax, Canada).
Data Analysis and Statistics
Operational bloom thresholds for each taxon were defined and used in data analysis and visualization. The threshold for A. lagunensis was defined as > 2 × 108 cells L–1 based upon levels used in bloom reporting (FWC-FWRI HAB Monitoring Database). The threshold for Pseudo-nitzschia spp. and P. bahamense was defined as > 105 cells L–1; for P. bahamense, it was based on cell abundance levels that correspond to target chlorophyll-a concentration exceedances (Lopez et al., 2021), and for Pseudo-nitzschia spp. it was based on levels that denoted substantial elevation above the majority (∼90%) of observations in the dataset. A descriptor for the persistence of blooms over time was developed by calculating the percent of weeks each year that an individual taxon was detected above the bloom threshold, and this metric is hereafter referred to as the annual bloom index (ABI). Using SJRWMD in situ water temperature from continuous monitoring stations averaged for all sub-basins (see footnote 2), the predicted peak germination date for P. bahamense resting cysts in spring was defined as the date when accumulated days with mean water temperatures below 25°C over the prior autumn/winter equaled 125 days, indicative of when a majority of P. bahamense resting cysts will have transitioned from dormancy (Lopez et al., 2019).
Kruskal-Wallis one way analysis of variance on ranks was used to compare differences among years and sub-basins for continuously monitored temperature and salinity data, and Spearman rank order correlations were used to compare relationships between taxa and salinity and temperature (SigmaPlot14.0). Then, using the most restricted subset of data, the one for which samples included both environmental parameters and corresponding cell enumeration for all three taxa (final n = 2,017), a matrix was developed that summarized A. lagunensis, Pseudo-nitzschia spp., and P. bahamense, by averaging cell concentrations in each sub-basin, per season (winter: December–February; spring: March–May; summer: June–August; autumn: September–November), for each of the years within the study period. After matrix transformation (log [x + 1]) to approach normalization and account for large number of zeros, permutation-based hypothesis was tested by analysis of similarity (ANOSIM) to examine for differences between groups of samples (in this case, sub-basins or seasons or years) according to the contribution of the three taxa in question (via Primer 6 with Permanova; Clarke and Gorley, 2006; Anderson et al., 2008).
Negative binomial regression (“nbinom1” parameterization; Hardin and Hilbe, 2007) was used to evaluate the influence of temperature, salinity, and the presence of an A. lagunensis bloom on Pseudo-nitzschia spp. and P. bahamense cell abundance at four of the fixed locations sampled weekly (Figure 1): Diamond Bay (Banana River Lagoon), Eau Gallie Pier and Eau Gallie North (central IRL), and NASA Causeway (northern IRL, P. bahamense only). Negative binomial regression was deemed appropriate for this analysis because of the discrete nature of the cell abundance response variable; additionally, preliminary analyses indicated evidence of overdispersion, which precluded the use of a more conventional Poisson regression for analyzing discrete count data. For each location, the total length of the weekly time series was restricted such that there was never a gap of data longer than 2 weeks, and gaps of data of 1 and 2 weeks were interpolated by averaging temperature, salinity, and cell abundances immediately prior to and following the gap in data (<10% of observations were interpolated). For model fitting, a global model was first developed that included all predictor variables hypothesized to be important drivers of variability in cell abundance: temperature, salinity, the presence of an A. lagunensis bloom, a temperature × salinity interaction term, and quadratic effects of temperature and salinity (temperature2 and salinity2). Variance inflation factors were calculated to test for collinearity of variables included in the global model. Additionally, the previous week’s Pseudo-nitzschia spp. or P. bahamense cell abundance was included to account for temporal autocorrelation (i.e., non-independence) among weekly cell abundance observations at each location. For each species and location, eight candidate negative binomial regression models were fitted, each representing a different combination of the predictor variables in the global model described above. Akaike’s information criterion (AIC; Akaike, 1973) was used with a small-sample bias-adjustment (Hurvich and Tsai, 1989) and Akaike weights (Burnham and Anderson, 2002) to rank the relative support of the candidate models. To account for model selection uncertainty (Burnham and Anderson, 2002), a confidence model set was identified for each location and taxon (P. bahamense and Pseudo-nitschia spp.), defined as those models with Akaike weights that were at least 10% of the best-approximating model, which is similar to Royall’s 1/8th rule of thumb for evaluating strength of evidence (Royall, 1997). The confidence model set for each location and taxon was then used to calculate model-averaged predictions of their respective time series to demonstrate the ability of the negative binomial regression models to characterize the temporal dynamics at each location, along with associated uncertainty. Lastly, goodness of fit was assessed for each model using a simulation-based approach implemented in the R package ‘DHARMa’ (Hartig, 2021). All model fits were conducted in R v.4.0.3 (R Core Team, 2020) using the packages ‘glmmTMB’ (model fitting; Brooks et al., 2017) and ‘MuMIn’ (model selection and model-averaged predictions; Barton, 2020). The goodness-of-fit assessment of negative binomial regression of model residuals did not reveal evidence of lack of fit for any of the candidate models fitted to the taxon- and location-specific time series.
Kernel density estimates for bloom occurrence of each taxon in temperature and salinity space were computed and plotted in R package ‘ggplot2.’
Results
Molecular Analyses and Method Comparisons for A. lagunensis Quantification
A variety of sequencing was performed to confirm the identity of A. lagunensis in cultures and eDNA samples. For two A. lagunensis isolates from Laguna Madre, TX, United States (CCMP1503 and UTEX2796), global pairwise 18S rRNA gene sequence alignment showed 97.2% sequence identity over 1577 bp. Except for four ambiguously called bases, the 18S rRNA gene sequences derived from 2012, 2016, 2018, and 2020 IRL DNA samples were identical to the UTEX2796 isolate (1577 bp, except for 2012 which was only 903 bp, GenBank accession numbers MW812272-MW812281). The UTEX2796 isolate and all IRL sequences contained a ∼420-bp insertion that was not present in the CCMP1503 isolate (and that was excluded from estimates of sequence similarity). Subsequent qPCR testing with 2016, 2018, and 2020 IRL DNA found that both the insertion and the non-insertion regions were present at similar levels across all years tested (mean delta Cq = –0.48 ± 0.21). For UTEX2796, RT-PCR revealed that the insertion region was only very weakly present as RNA compared to the 18S rRNA amplicon without the insertion (Supplementary Figures 3, 4).
Specificity testing (by PCR and agarose gel electrophoresis) of the A. lagunensis qPCR assay against 20 cultured algae indicated (1) strong amplification with A. lagunensis UTEX2796 and IRL DNA; (2) weak amplification for the two most closely related non-target pelagophytes, Aureococcus anophagefferens and A. lagunensis CCMP1503 (Supplementary Figure 5), which both yielded very minor amplification and were clearly discriminable from the target DNA in melt-curve data (Supplementary Figure 6); and (3) no amplification with other non-target species (Supplementary Figure 5). It was estimated that A. lagunensis contains 16 ± 2.6 copies of 18S rRNA gene per cell.
The qPCR assay for A. lagunensis UTEX2796 showed an efficiency of 87.7% based on average slopes of individual reactions in exponential-phase, using gDNA as template (n = 21, via LinRegPCR, Ramakers et al., 2003), 83.7% using a 10-fold dilution series of gDNA, and 88.2% using a synthetic 500-bp rRNA gene fragment. Extraction efficiency excluding variability in cell lysis, determined as a percent recovery of a 500-bp synthetic gene fragment, was 42% (±10, n = 11). PCR inhibition above a twofold inhibition was present in 1 out of 25 IRL DNA samples tested. Based on good replication among triplicate qPCR reactions, the limit of quantification was set at 10 copies per 10 μL qPCR reaction (Cq = 33.1 ± 1.0), which, from a typical IRL DNA sample, represents 145,103 cells L–1.
Loge transformed results of cell abundance quantified by qPCR and immunofluorescence flow cytometery were correlated with loge transformed microscopic counts of A. lagunensis-like cells (Figure 2). The log-log relationship between qPCR and microscopy had a slope of 1.13 (slope 95% C.I. 1.05–1.21, R2 of 0.85, Figure 2A), though abundance measured by qPCR was generally lower than microscopic counts when cell abundances were below the bloom threshold. In contrast, abundance measurements by flow cytometry were generally higher than microscopic counts at lower cell abundances in FWC-FWRI samples (slope = 0.76, slope 95% CI 0.70–0.81, R2 = 0.59, Figure 2B) and UF/SJRWMD samples (slope = 0.81, slope 95% CI 0.74–0.87, R2 = 0.81, Figure 2D). For those samples which had both qPCR and flow cytometry performed (n = 78), the log-log relationship was comparable with a slope of 1.10 (slope 95% CI 0.99–1.22, R2 = 0.82, Figure 2C). In evaluating all three methods together (qPCR/flow cytometry/microscopy), certain sample-weeks showed consistent discrepancies between the microscopy counts and the two molecular tools (Supplementary Figure 7), indicating a small subset of anomalous data points (2% of samples) that were eliminated from the data presented in Figures 2A,B.
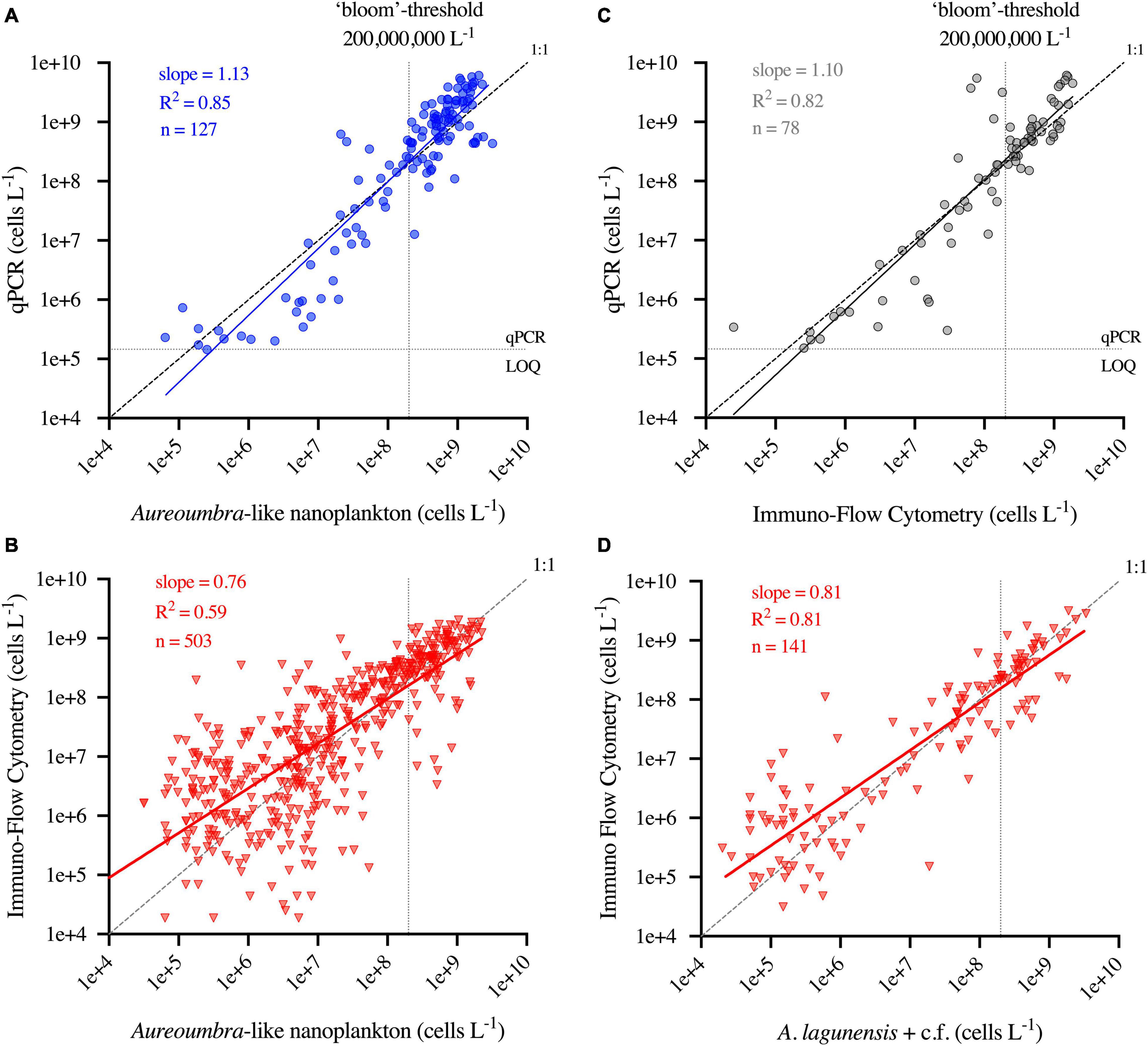
Figure 2. (A) Regression between qPCR and microscopy counts for Aureoumbra-like cells. (B) Regression between immunofluorescence flow cytometry and microscopy counts for Aureoumbra-like cells. (C) Regression between qPCR and immunofluorescence flow cytometry. (D) Regression between immunofluorescence flow cytometry and summed microscopy counts of A. lagunensis + A. lagunensis c.f. from SJRWMD samples.
Environmental Data
Salinity data from continuous monitoring were significantly different among all sub-basins [Kruskal–Wallis, H(3) = 3783, p < 0.001] and most years [Kruskal–Wallis, H(5) = 1159, p < 0.001; Figure 3]. By sub-basins, the highest median salinities recorded at continuous monitoring stations occurred in the Mosquito Lagoon (31.2 psu) and the lowest in the Banana River Lagoon (22.5 psu) and Central IRL (21.4 psu); a gap in data in 2018 occurred in Central IRL in situ salinity. Among years, the highest median salinity (30.2 psu) occurred in 2017 and was significantly higher than all other years; 2017 was also a drier than average year until September when heavy rainfall was associated with a rapid salinity decline following Hurricane Irma (Figure 3 and Supplementary Figure 8). Salinities were significantly lower in the latter 3 years (∼22–23 psu) compared to the first 3 years (∼27–30 psu). This observed shift to lower salinity in late 2017 was most evident at the Banana River Lagoon continuous monitoring station where it persisted in subsequent years (Figure 3B) but was also observed in discrete measurements of salinity (Figures 4–7).
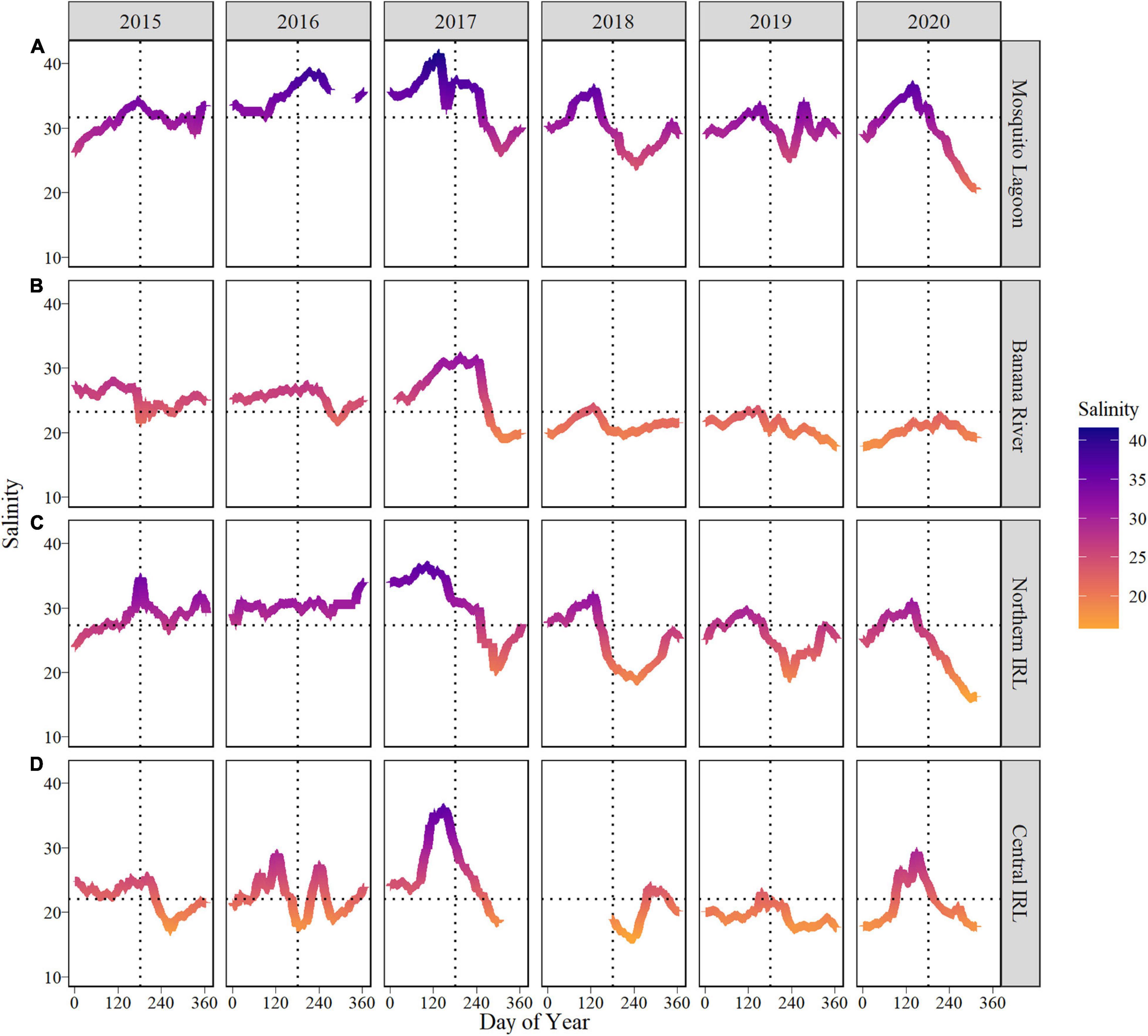
Figure 3. Weekly rolling average of in situ salinity collected at SJRWMD continuous monitoring stations for (A) Mosquito Lagoon, (B) Banana River Lagoon, (C) Northern IRL, and (D) Central IRL. Line color indicates the salinity gradient of the weekly rolling average, ranging from the lowest salinities (orange) to the highest salinities (blue). The horizontal lines represent the mean daily salinity for all years for each respective basin. The vertical dotted line represents JD 182 (July 1, non-leap years).
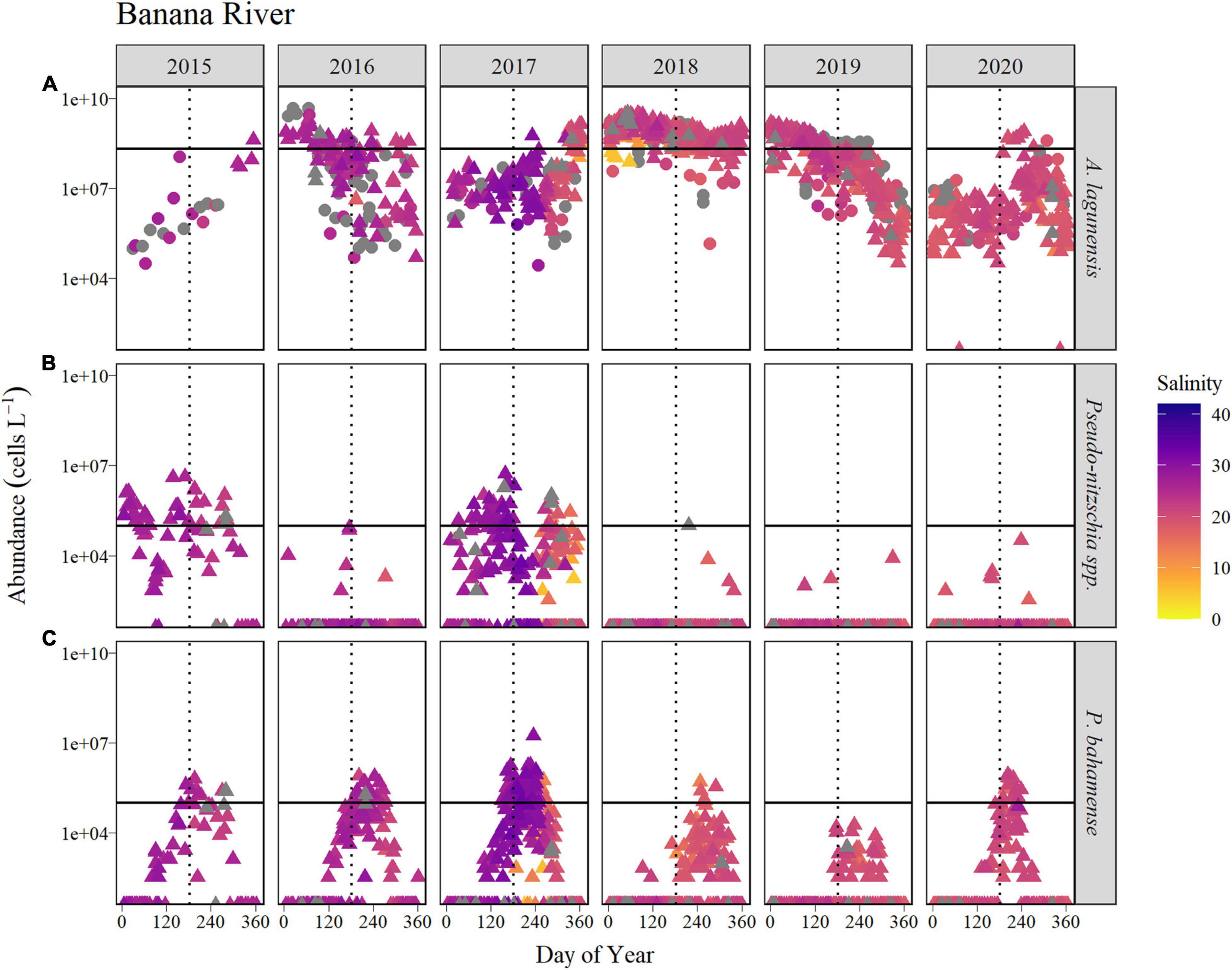
Figure 4. Time course of abundance of (A) A. lagunensis cells, (B) Pseudo-nitzschia spp., and (C) P. bahamense presented on a logarithmic scale for the Banana River Lagoon sub-basin for the years 2015 to 2020 (horizontal panels). Symbol color indicates salinity in corresponding discrete samples, shown as a gradient from the lowest salinities (orange) to the highest (blue). Gray symbols represent samples in which no discrete salinity was recorded. For A. lagunensis, abundance was determined by FWC-FWRI microscopic enumeration (filled triangles) and immunofluorescence flow cytometry (filled circles). The solid horizontal line indicates the bloom levels for each taxon (A. lagunensis = 2 × 108 cells L–1, Pseudo-nitzschia spp. and P. bahamense = 105 cells L–1). The vertical dotted line represents JD 182 (July 1, non-leap years).
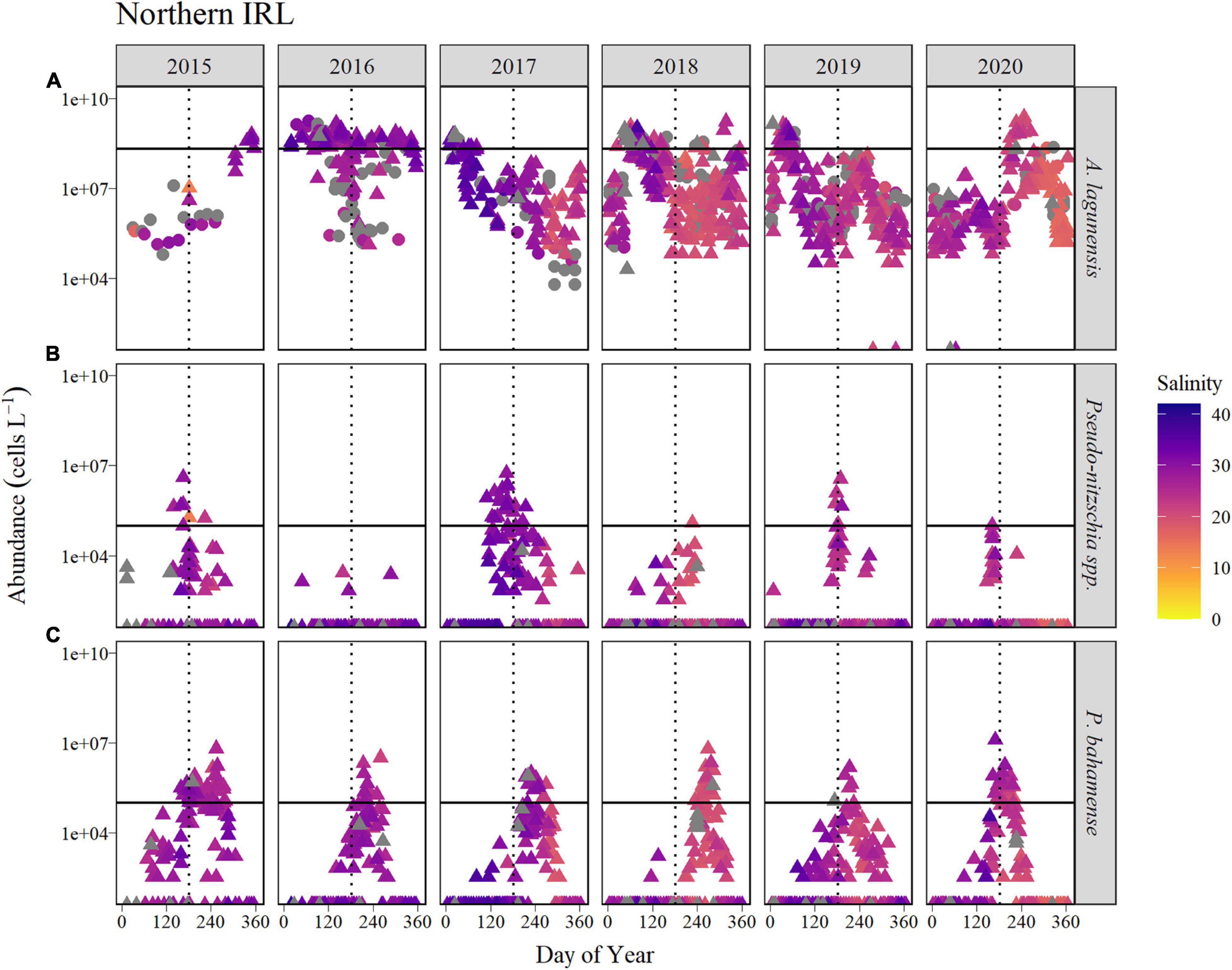
Figure 5. Time course of abundance of (A) A. lagunensis cells, (B) Pseudo-nitzschia spp., and (C) P. bahamense presented on a logarithmic scale for the Northern IRL sub-basin for the years 2015 to 2020 (horizontal panels). Symbol color indicates salinity in corresponding discrete samples, shown as a gradient from the lowest salinities (orange) to the highest (blue). Gray symbols represent samples in which no discrete salinity was recorded. For A. lagunensis, abundance was determined by FWC-FWRI microscopic enumeration (filled triangles) and immunofluorescence flow cytometry (filled circles). The solid horizontal line indicates the bloom levels for each taxon (A. lagunensis = 2 × 108 cells L–1, Pseudo-nitzschia spp., and P. bahamense = 105 cells L–1). The vertical dotted line represents JD 182 (July 1, non-leap years).
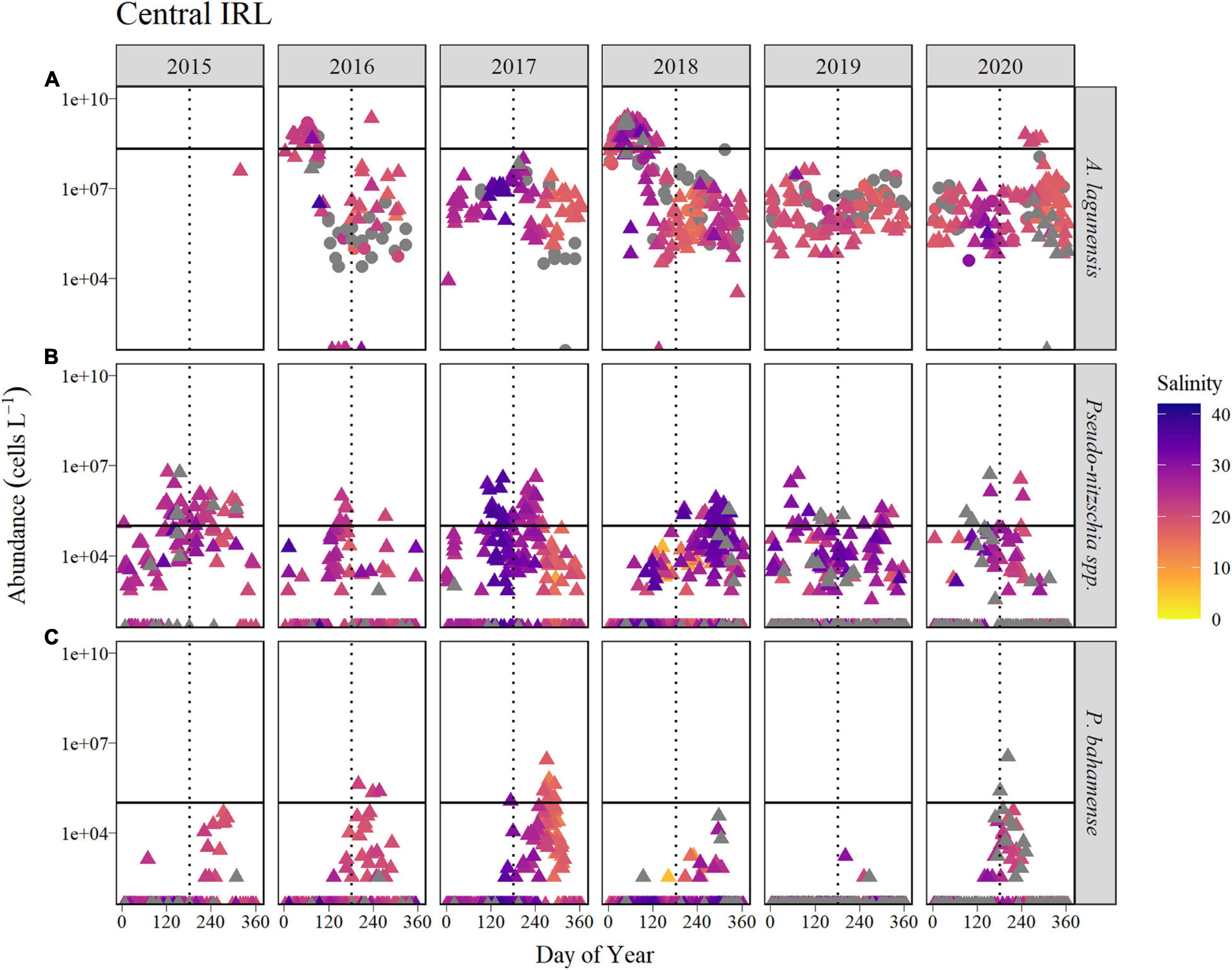
Figure 6. Time course of abundance of (A) A. lagunensis cells, (B) Pseudo-nitzschia spp., and (C) P. bahamense presented on a logarithmic scale for the Central IRL sub-basin for the years 2015 to 2020 (horizontal panels). Symbol color indicates salinity in corresponding discrete samples, shown as a gradient from the lowest salinities (orange) to the highest (blue). Gray symbols represent samples in which no discrete salinity was recorded. For A. lagunensis, abundance was determined by FWC-FWRI microscopic enumeration (filled triangles) and immunofluorescence flow cytometry (filled circles). The solid horizontal line indicates the bloom levels for each taxon (A. lagunensis = 2 × 108 cells L–1, Pseudo-nitzschia spp., and P. bahamense = 105 cells L–1). The vertical dotted line represents JD 182 (July 1, non-leap years).
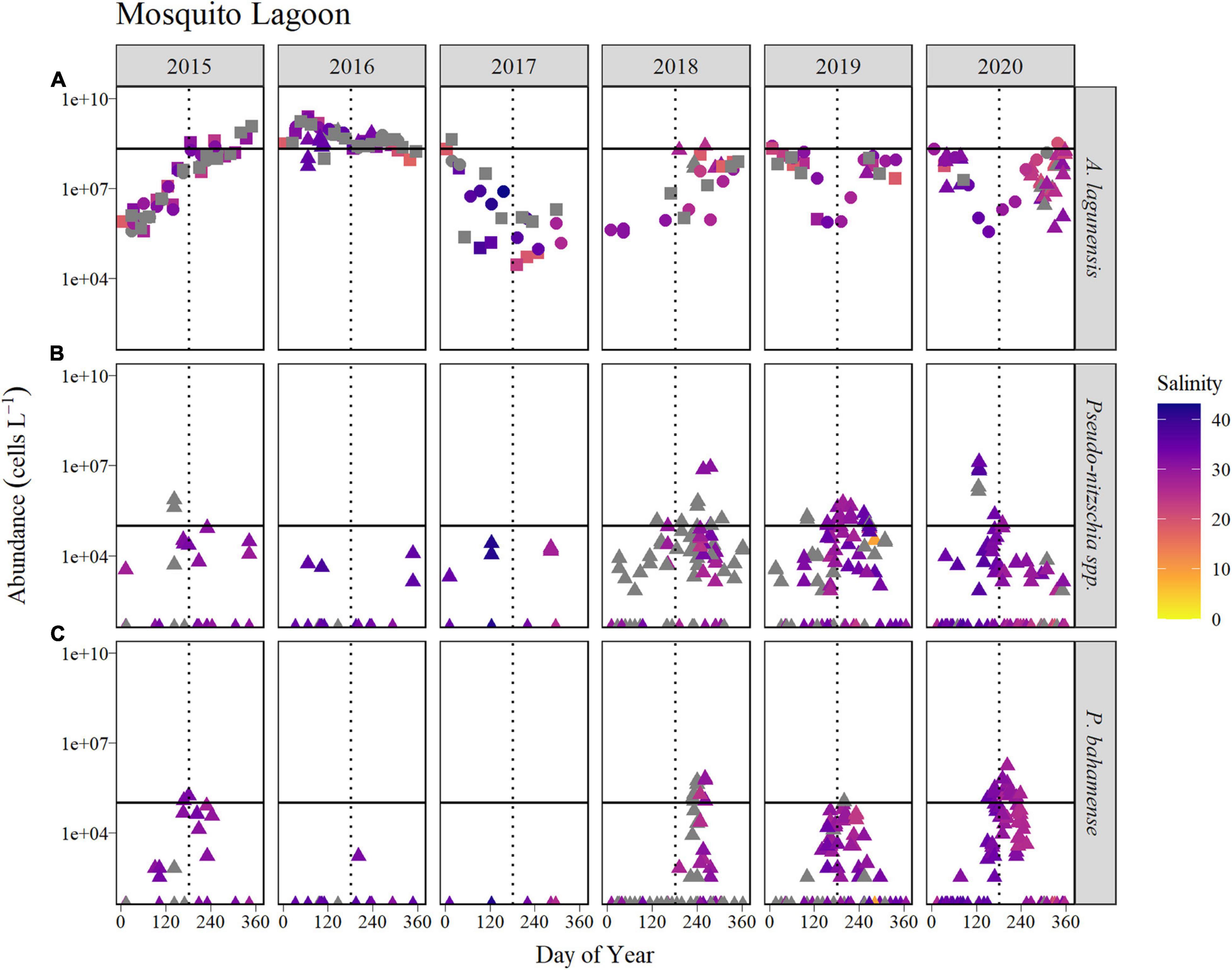
Figure 7. Time course of abundance of (A) A. lagunensis cells, (B) Pseudo-nitzschia spp., and (C) P. bahamense presented on a logarithmic scale for the Mosquito Lagoon sub-basin for the years 2015 to 2020 (horizontal panels). Symbol color indicates salinity in corresponding discrete samples, shown as a gradient from the lowest salinities (orange) to the highest (blue). Gray symbols represent samples in which no discrete salinity was recorded. For A. lagunensis, abundance was determined by microscopic enumeration (FWC-FWRI, filled triangles; UF, filled squares) and by immunofluorescence flow cytometry (filled circles). The solid horizontal line indicates the bloom levels for each taxon (A. lagunensis = 2 × 108 cells L–1, Pseudo-nitzschia spp., and P. bahamense = 105 cells L–1). The vertical dotted line represents JD 182 (July 1, non-leap years).
Water temperature measured at continuous monitoring stations was similar among basins except for the Central IRL, which had a significantly higher median temperature (26.9°C) than other basins (26.0–26.2°C, Kruskal–Wallis, p < 0.01). Water temperatures were generally similar to the 7-day moving average of mean air temperature, and there were no significant differences in median yearly temperature among years (Kruskal–Wallis, p = 0.246, Supplementary Figure 9). In general, summer temperatures were consistent among years with the highest interannual variation occurring in the winter and transition months (November–April). For example, mean water temperatures in February were cooler in 2015 (14.6°C) and 2016 (15.6°C) compared to later years (18.5–21.2°C, p < 0.001), but were followed by warmer mean temperatures in March, and 2015 had the warmest April of all years. Two years, 2015 and 2020, also had a warmer November (i.e., delayed cooling) compared to other years, which resulted in those 2 years having a longer period of warmer temperatures than other years. In situ water temperatures in the autumn and winter—and moreover, the number of days below 25°C—were used to calculate the predicted date for maximum potential for P. bahamense resting cyst germination (based on the transition from dormancy) for the following spring. Thus, delayed cooling in 2015 and 2020 translated to delayed maximum predicted germination in 2016 and 2021 relative to other years (Table 2).
Harmful Algal Blooms Dynamics in the Banana River Lagoon Sub-Basin
In the Banana River Lagoon, A. lagunensis blooms displayed no clear seasonal trend, and bloom timing and persistence varied from year to year (Figure 4). In 2015, A. lagunensis reached bloom levels (2 × 108 cells L–1) in December and remained high in some locations within the sub-basin through July 2016, resulting in the ABI exceeding 50% for 2016 (Table 2). In 2017, A. lagunensis did not reach bloom levels again until November (Julian day, JD 319), but then abundance remained high for almost 22 months—exceeding the bloom threshold every single week of 2018 and for more than half of 2019 (Figure 4 and Table 2). From the end of 2017 until mid-2019, higher cell concentrations coincided with relatively lower and stable salinity levels in this sub-basin compared to earlier in the study period. Cell concentrations finally declined at all sampling sites by September 2019 (∼JD 270) and remained at lower levels for most of 2020, except for a short-lived (∼5–6 weeks) A. lagunensis bloom in late summer of that year.
During the period of study, Pseudo-nitzschia spp. exceeded 105 cells L–1 in the Banana River Lagoon only in 2015 (ABI of 50%) and 2017 (ABI of 42%), and in both years these blooms occurred at times when A. lagunensis concentrations were lower (Figure 4 and Table 2). During other years, the Pseudo-nitzschia spp. were found only sporadically and in low abundance in this sub-basin.
Patterns in P. bahamense abundance were marked by an annually recurring summer bloom with cell abundances exceeding > 105 cells L–1 in most years (Figure 4). From 2015 to 2017, blooms were present between 18 and 34% of the year (Table 2) compared to 2018, when bloom levels were only observed for 8% of the year (4 weeks), and to 2019, when the bloom threshold was never reached (Figure 4 and Table 2). The relatively lower P. bahamense abundance in 2018 and 2019 coincided with the time period affected by the 22-month A. lagunensis bloom and with the lower salinity levels in this sub-basin. Furthermore, the timing of the seasonal increase in P. bahamense abundance above bloom levels each year varied between JD 149 and 183 (i.e., end of May to the beginning of July) for all years with two exceptions: bloom abundance in 2018 were not observed until substantially later in the year (early September, JD 248) and there was no bloom observed in 2019 (Table 2). While the predicted peak germination date for P. bahamense resting cysts was similar among all years (earliest was JD 51 in 2017 and latest was JD 74 in 2016), there was a late cool spell in March 2018 that occurred after the predicted maximum germination date (JD 62, Supplementary Figure 9). The date of bloom decline among years ranged from late-August (in 2020) to mid-October (in 2018).
Harmful Algal Blooms Dynamics in the Northern IRL Sub-Basin
In the Northern IRL sub-basin, the pattern of A. lagunensis abundances varied slightly from that in the Banana River Lagoon, with blooms declining on different timescales in some years. For example, A. lagunensis cell abundances in 2016 remained above the bloom threshold for a longer time period (ABI of 76%, Figure 5 and Table 2) than in the Banana River Lagoon. Conversely, the number of weeks above bloom threshold during 2018–2019 was lower in the Northern IRL (40% in 2018) due to a mid-summer decline in both years that was not observed in the Banana River Lagoon (Figure 4). Like the Banana River Lagoon, a noticeable drop in salinity was also observed in both discrete and continuous monitoring data in the Northern IRL at the end of 2017.
Pseudo-nitzschia spp. abundance in the Northern IRL reached and surpassed bloom levels in 2015, 2017, and briefly in 2019 (6% or 3 weeks of the year), all at times when A. lagunensis levels were lower (Figure 5). In other years, Pseudo-nitzschia spp. abundance in water samples was sporadic; the highest abundances detected tended to be associated with higher salinities.
Abundance of P. bahamense in the Northern IRL displayed a recurring seasonal pattern of summertime blooms for the 2015–2020 time period (Figure 5). The first exceedance of bloom thresholds varied among years and occurred as early as June (JD 159) in 2015 and as late as August (JD 233) in 2018 (Table 2). The ABI based on bloom duration was longest in 2015 (34% of the year) and shortest in 2019 (8% of the year). In 2019 and 2020, the bloom declined in mid-August—earlier than in other years, when it declined from mid-September (2016) to mid-October (2018).
Harmful Algal Blooms Dynamics in the Central IRL Sub-Basin
Abundance of A. lagunensis generally was lower in the Central IRL sub-basin relative the other sub-basins examined, with peaks above the bloom threshold observed in winter/spring months of both 2016 (20% of the year) and 2018 (37% of the year), and for a shorter period in the autumn of 2020 (Figure 6 and Table 2).
Of the four sub-basins considered in this study, Pseudo-nitzschia spp. occurred most frequently in the Central IRL, based on year-round presence and the annual occurrence of blooms. While peak abundance generally occurred in warmer months, there was not a clear pattern of recurrence. There were fewer weeks with bloom levels in 2016 and 2020 (<10% of the year), compared to other years when bloom levels were detected between 21 and 39% of the year (Table 2).
Cell abundance of P. bahamense in the Central IRL was lower and presence less sustained than in the Banana River Lagoon and Northern IRL sub-basins (Figure 6); however, a similar seasonal pattern characterized by a summer bloom was still observed. Bloom levels were only briefly reached in three of the 6 years: 2016 (2% of the year), 2017 (12% of the year), and 2020 (4% of the year, Table 2). In 2019, P. bahamense remained barely detectable (Figure 6).
Harmful Algal Blooms Dynamics in the Mosquito Lagoon Sub-Basin
In the Mosquito Lagoon, sampling was less frequent than in the other basins, i.e., approximately monthly from 2015 to 2017. However, bloom concentrations observed for A. lagunensis in 2015 and 2016 reflected patterns observed in the Northern IRL sub-basin (Figure 7). The longest duration of A. lagunensis above bloom levels occurred in 2016 (ABI of 89%). In other years, A. lagunensis was present mostly at lower abundances with sporadic observations of bloom levels, surpassed for only 3–7% of the year.
The cell abundance for Pseudo-nitzschia spp. and P. bahamense showed a similar seasonal pattern to each other, characterized by summer blooms in four of the 6 years (Figure 7). In those 4 years, the longest Pseudo-nitzschia spp. bloom occurred in 2019 (ABI of 23%) and the longest P. bahamense bloom occurred in 2020 (ABI of 17%, Table 2). Samples for which salinity data were available showed that these higher abundances were typically associated with salinities > 27 psu (Figure 7).
Occurrence of a Novel Cyanobacterium in 2020
In August of 2020, greenish water discoloration was detected in the IRL and associated with a bloom of a nano-sized cyanobacterium. Microscopic inspection of available archived samples revealed that this taxon had been present since at least June 2020 (JD 175, Figure 8). Bloom concentrations (>2 × 108 cells L–1) were first observed in the Banana River Lagoon and Northern IRL in late-July to early-August and persisted in all sub-basins until early December 2020. Bloom levels of this cyanobacterium overlapped with bloom levels of A. lagunensis at some locations in the Northern IRL and Banana River Lagoon (August and September 2020) and in the Central IRL (late-September to mid-October 2020). The abrupt decline in abundance in December 2020 coincided with a decline in water temperature below 20°C (Figure 8).
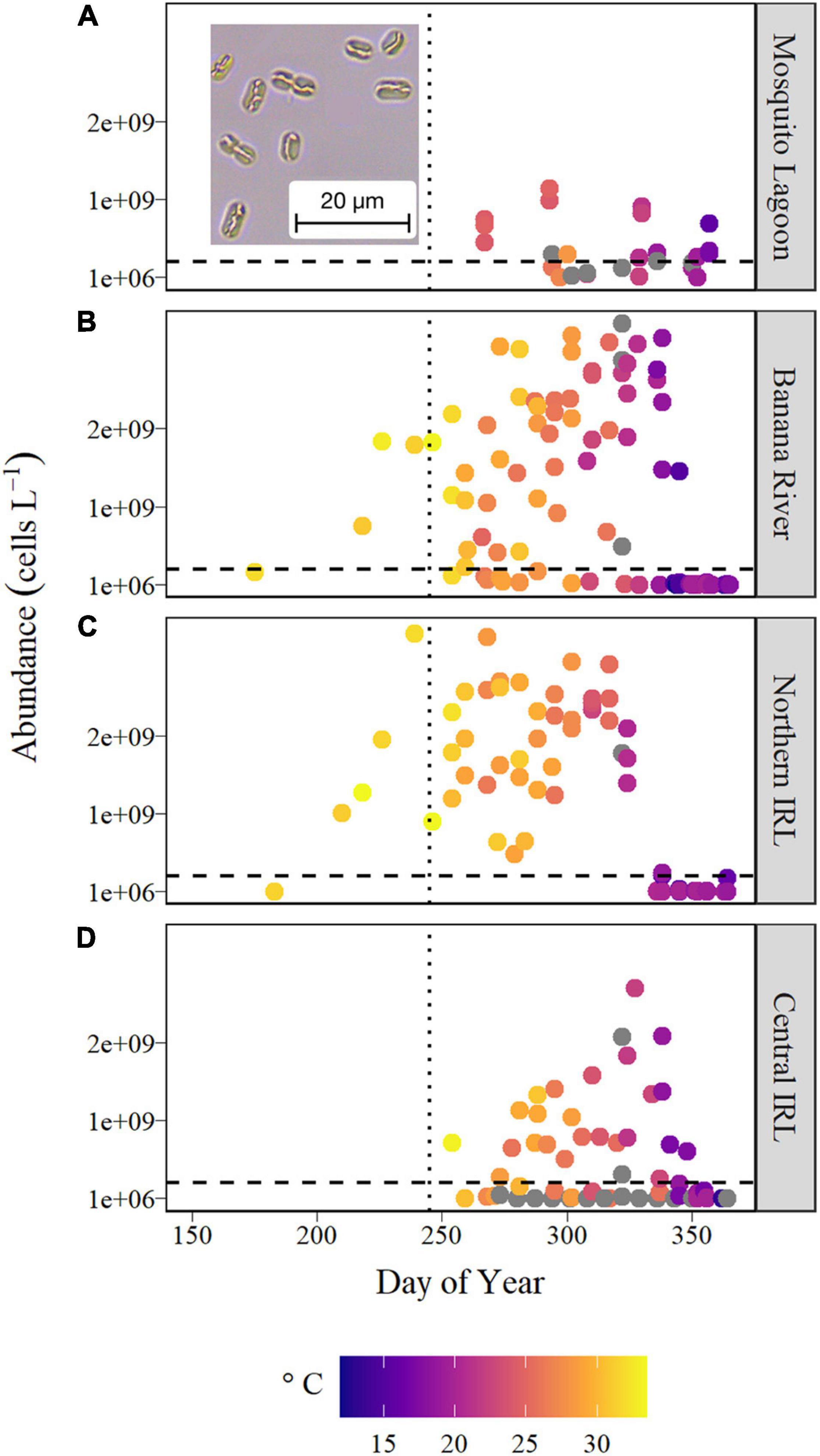
Figure 8. Cell abundance of the novel cyanobacterium shown on a linear scale for (A) Mosquito Lagoon, (B) Banana River Lagoon, (C) Northern IRL, and (D) Central IRL for June through December 2020. Symbol color indicates temperature recorded at discrete depths of samples, shown as a gradient from the lowest temperature (blue) to the highest (yellow), and gray symbols represent samples in which no discrete temperature was recorded. The dashed horizontal line represents 2 × 108 cells L–1 and the dotted vertical line represents JD 245 (September 1). (Inset) Phase contrast light microscopy image of live cells of the novel cyanobacterium, depicting a refringent structure inside each cell, the aerotope.
This taxon had unique characteristics based on gross morphology relative to known species. Cells have a round to oblong shape (3–4 × 5 μm) and were often observed as two or more cells in a chain (Figure 8A, inset). Examination of live material indicated the presence of an elongated gas vacuole that collapsed upon preservation with Lugol’s iodine solution. Flow cytometric examination confirmed relatively low chlorophyll-a (488 nm excitation, 690/40 nm emission) and high phycocyanin levels (637 nm excitation, 670/14 nm emission). Direct sequencing with cya359F-cya781R(b) was successful in the sample from Parrish Park Boat Ramp, and additional direct sequencing with a combination of specific/universal primers yielded a final contig of 2183 bp (GenBank accession number MW816502) covering most of the 16S rRNA gene, the ITS region, and a partial fragment of the 23S rRNA gene. The closest BLASTn hit was to an uncultured cyanobacterium with percent identity of 96.9% over 65% query coverage (ITS-region missing), and the first hit to a known organism was to Prochlorothrix hollandica, with a percent identity of 93.5% over 74% query coverage. Within the SILVA 16S rRNA database, this sequence was distinct from, but adjacent to, Prochlorothrix sp. Microscopy observations in the targeted Parrish Park Boat Ramp and Eau Gallie Pier samples (from September 10, 2020) indicated that this novel cyanobacterium was respectively 72- and 173-fold more abundant than A. lagunensis. Using qPCR, and without accounting for differences in rRNA gene copies per cell or qPCR efficiencies, the abundance of this sequence was approximately 20- and 700-fold greater than A. lagunensis for the same two samples.
Domoic Acid and Saxitoxins
Water samples were tested every year over the study period for the presence of domoic acid and saxitoxins. Only three samples (in 2020) showed the presence of domoic acid at concentrations varying between 0.01 and 0.02 μg L–1 (Supplementary Table 3). These samples were collected in the Mosquito Lagoon, in the vicinity of Cedar Island (Figure 1).
Paralytic shellfish toxins or saxitoxins were detected in water samples every year between 2015–2020. Decarbamoyl saxitoxin, gonyautoxin-5, and saxitoxin were the congeners detected, as has been previously reported by Landsberg et al. (2006), and results are presented as saxitoxin equivalents. The seasonality of toxins in water samples was comparable to that of P. bahamense cell abundance, with highest values of saxitoxin equivalents in surface water samples corresponding to samples with highest cell abundances (Supplementary Figure 10). For example, the highest toxicity in water samples in 2017 coincided with a cell abundance > 1.5 × 106 cells L–1 collected on August 17 in Banana River Lagoon, with high intracellular toxin levels (15.7 pg cell–1). Similarly, in 2018, the highest toxin concentration in surface water samples was detected in a Northern IRL sample collected in late September, with a high cell concentration (∼6.5 × 106 cells L–1) but low cellular saxitoxin equivalent (4.0 pg cell–1). In general, total intracellular toxin concentrations stayed below 10 pg cell–1, but concentrations as high as ∼25 pg cell–1 were detected in 2017 in the Banana River Lagoon and 2018 in the Northern IRL during late bloom season. Maximum detected toxin concentrations in water samples from 2019 and 2020 were considerably lower, peaking at 1.7 μg L–1 in 2020; however, fewer samples were analyzed during these years. Toxin per cell in 2016 was significantly lower than other years, excluding 2020 [Kruskal–Wallis, H(5) = 40.86, p < 0.001].
Statistical Analysis and Modeling of Taxon Dynamics
ANOSIM analysis suggested slightly dissimilar behavior in terms of occurrence and abundance of HAB taxa between Banana River Lagoon and Central IRL sub-basins (ρ = 0.232, p < 0.001), but variability between other basins was not significantly greater than the variability within each basin. However, there were significant seasonal differences in HAB taxa occurrence and abundance between spring and summer (ρ = 0.255, p ≤ 0.001), winter and summer (ρ = 0.679, p < 0.001), and winter and autumn (ρ = 0.283, p < 0.001). Although only marginally significant, 2017 stood out from the other years, especially in comparison with 2015 (ρ = 0.505, p = 0.002), 2016 (ρ = 0.347, p = 0.002), and 2018 (ρ = 0.353, p = 0.002).
Spearman rank order correlation was used to examine if there was a linear relationship of taxa abundance with salinity or temperature across all records. For P. bahamense, a positive association with temperature (rs = 0.49, p < 0.001) and a weak association with salinity (rs = 0.065, p < 0.001) were observed. For Pseudo-nitzschia spp., a weak association was found between abundance and temperature (rs = 0.159, p < 0.001), and the relationship with salinity (rs = 0.30, p < 0.001) was stronger than that of P. bahamense. Aureoumbra lagunensis, which was observed in all seasons, was only weakly associated with cooler temperatures (rs = –0.11, p < 0.001), and displayed no significant correlation with salinity.
The Diamond Bay station in the Banana River Lagoon provided the longest time-series for analysis compared to other sub-basins (Figure 9). For this station, the best-approximating negative binomial regression model for abundance of Pseudo-nitzschia spp. included temperature, salinity, salinity2, presence of A. lagunensis blooms, and the lagged Pseudo-nitzschia spp. count (Table 3). The best-approximating model for P. bahamense at Diamond Bay included temperature, temperature2, salinity, salinity2, presence of A. lagunensis blooms, and the lagged P. bahamense count. Model-averaged predictions of mean P. bahamense abundance generally closely followed observed abundances through the bloom development, peak, and decline phases in all years but 2018 and 2019, for which observed abundances were lower or absent in contrast to predictions (Figure 9). Parameter estimates from the best-approximating models indicated a significant non-linear (i.e., quadratic) relationship between abundance of P. bahamense and both salinity and temperature (Supplementary Table 4). Furthermore, the occurrence of bloom levels of A. lagunensis had a strong negative effect on abundance of P. bahamense, where mean abundance was 14.7 times (i.e., 1/e–2.688) lower in the presence of A. lagunensis blooms. Parameter estimates also indicated a quadratic effect of salinity and negative effects of temperature and A. lagunensis blooms on Pseudo-nitzschia spp. (Table 3); however, those results must be interpreted with caution given the rarity of Pseudo-nitzschia spp. in most years at this site.
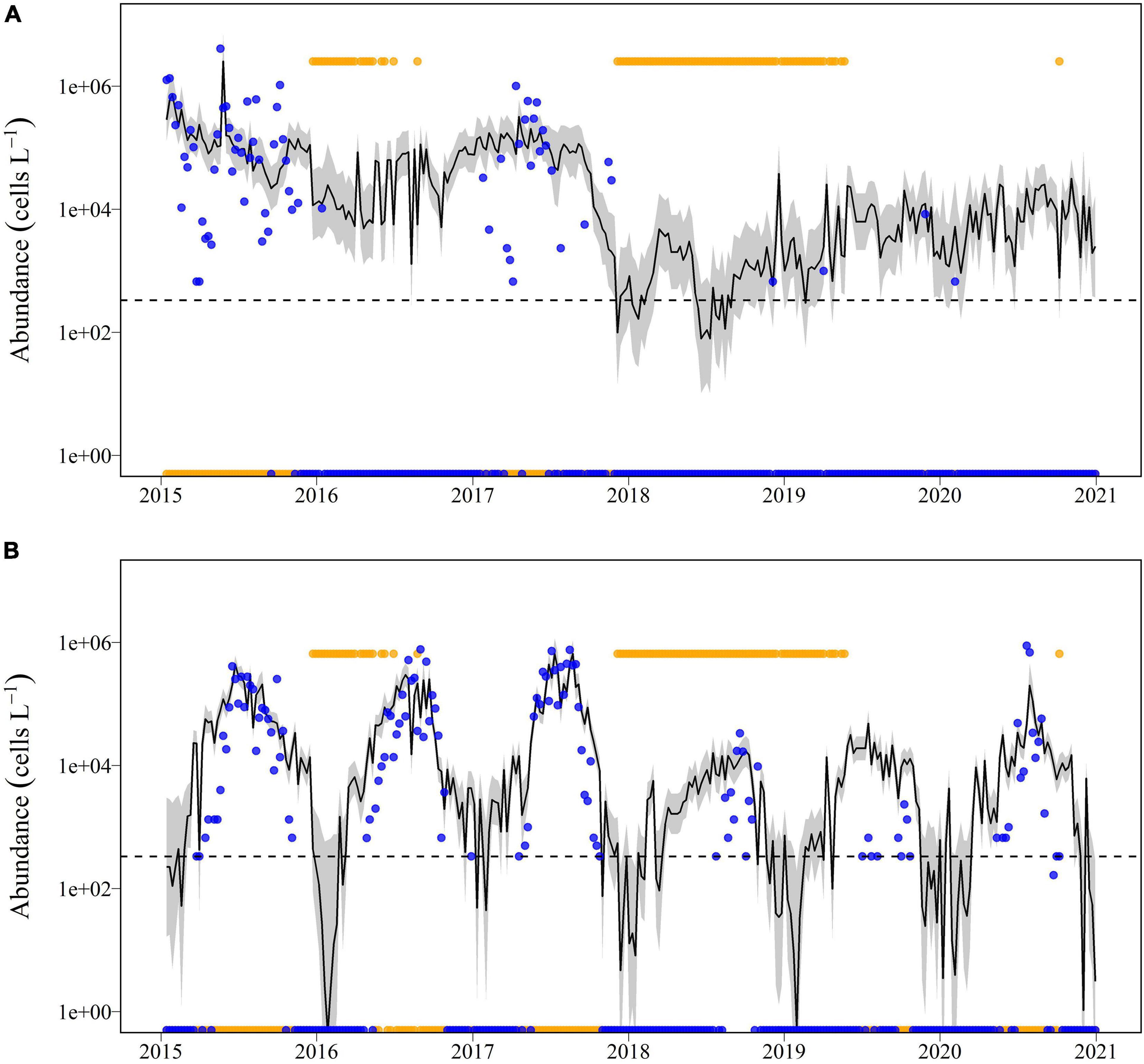
Figure 9. Model-averaged predictions of abundance (solid black line) of (A) Pseudo-nitzschia spp. and (B) P. bahamense at the Diamond Bay station for the years 2016–2020, based on the best approximating models from negative binomial regression analysis compared to observed abundances (blue dots). The gray shaded area representing the 95% confidence interval. The dashed line indicates the microscopy detection limit of 333 cells L–1 for these two taxa. The elevated horizontal yellow dots indicate the presence of A. lagunensis at bloom levels (i.e., above 2 × 108 cells L–1).
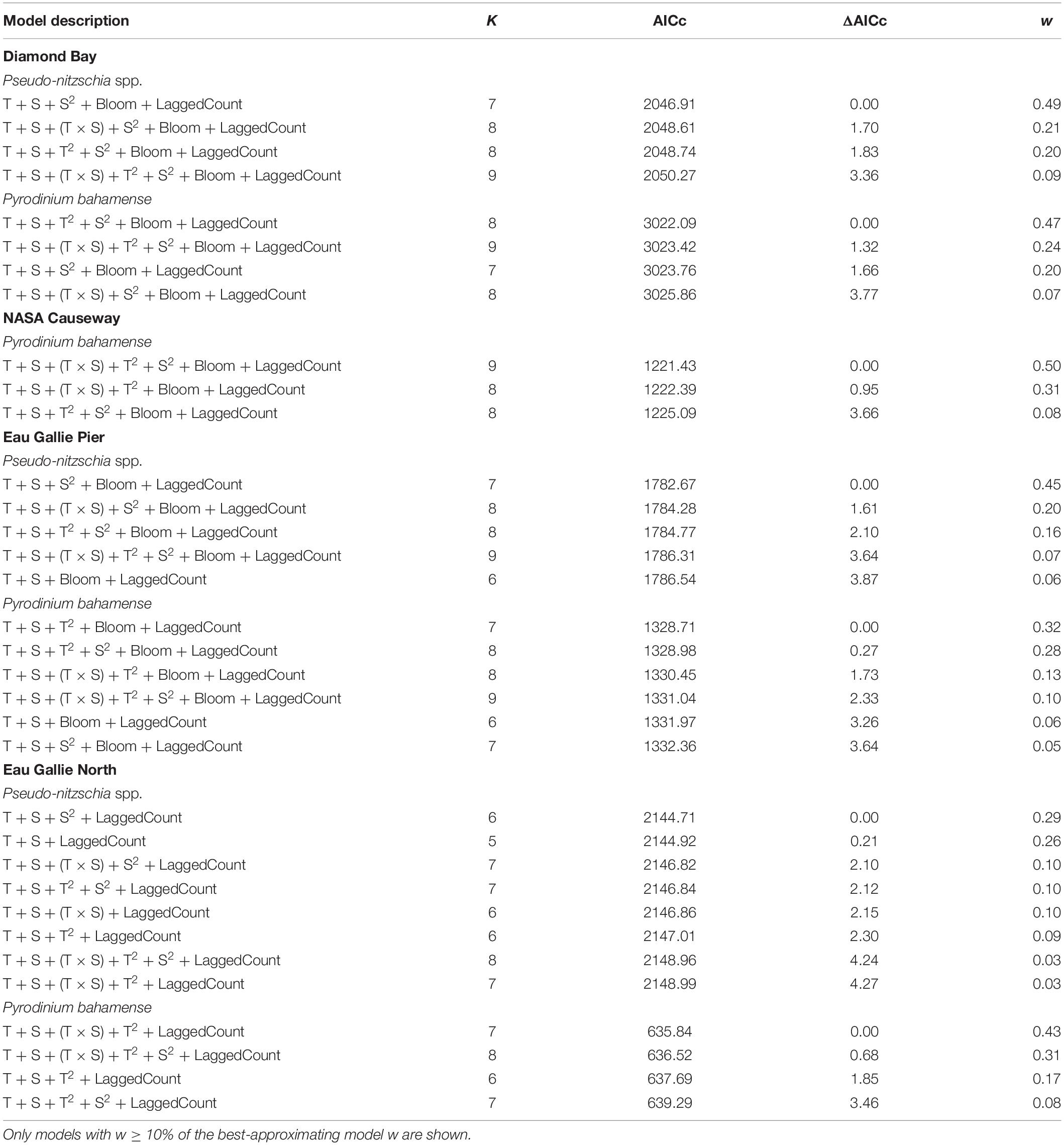
Table 3. Model parameters, number of parameters (K), AICc, ΔAICc, and AICc weights (w) for the candidate set of negative binomial regression models relating weekly Pseudo-nitzschia spp. and Pyrodinium bahamense cell counts to temperature (T), salinity (S), the presence of an Aureoumbra lagunensis bloom (Bloom), and a lagged cell count (lagged by 1 week; LaggedCount) at Diamond Bay, NASA Causeway, Eau Gallie Pier, and Eau Gallie North monitoring stations.
In the Northern IRL, the NASA causeway station time-series analysis was limited to the years 2017–2020 because of data gaps. Except for a bloom in 2017, Pseudo-nitzschia spp. rarely occurred at this site; hence, a negative binomial regression was fitted only to the P. bahamense data. The best-approximating model for P. bahamense was the global model that included temperature, temperature2, salinity, salinity2, temperature × salinity, presence of A. lagunensis blooms, and the lagged P. bahamense count (Table 3). Model-averaged predictions of mean P. bahamense abundance tracked observed abundances reasonably well in most years, with the exception of the delayed occurrence in observed cells in 2018 (Supplementary Figure 11). Parameter estimates from the best-approximating model indicated that temperature exhibited a strong quadratic effect on mean abundance of P. bahamense, and there was also a significant positive effect associated with the interaction of temperature and salinity (Supplementary Table 5). Although no significant effect of A. lagunensis blooms on P. bahamense abundance was found at this Northern IRL sites, A. lagunensis blooms were relatively rare there and this estimate should accordingly be interpreted with caution.
In Central IRL, we examined time-series from two stations—Eau Gallie Pier (located just northwest of the Eau Gallie Causeway) and Eau Gallie North (located 3-km north of the Eau Gallie Pier station, Figure 1). The best-approximating negative binomial regression model for Pseudo-nitzschia spp. at Eau Gallie Pier included temperature, salinity, salinity2, the presence of A. lagunensis blooms, and the lagged Pseudo-nitzschia spp. count; results for Pseudo-nitzschia spp. at Eau Gallie North was similar but excluded the presence of A. lagunensis blooms because of its rarity (Table 3). Parameter estimates also indicated that, unlike other time-series sites tested, the prior-week abundance of Pseudo-nitzschia spp. was not a strong predictor of Pseudo-nitzschia spp. abundance at Eau Gallie Pier, which may reflect the rapid changes in observed Pseudo-nitzschia spp. abundances from week to week at this site (Supplementary Table 6). Generally, P. bahamense abundance was lower and more poorly predicted at the Eau Gallie Pier and Eau Gallie North sites in Central IRL (Supplementary Figures 12, 13) compared to Banana River Lagoon and Northern IRL sites. At Eau Gallie Pier, the best-approximating model for P. bahamense included temperature, temperature2, salinity, and the lagged P. bahamense count; results were similar at Eau Gallie North with the addition of the temperature × salinity term (Table 3). Again, the A. lagunensis predictor variable was omitted from the Eau Gallie North model because of the rarity of A. lagunensis above bloom thresholds at this site. Parameter estimates associated with the best-approximating models for both sites indicated evidence for a quadratic relationship between temperature and P. bahamense abundance at both Eau Gallie Pier and Eau Gallie North, whereas the positive interaction between temperature and salinity appeared to be important only at Eau Gallie North (Table 3). While there was evidence for a strong quadratic relationship between temperature and abundance at both sites, model-averaged predictions showed that the timing of actual occurrence was generally delayed compared to predicted occurrence, especially at the Eau Gallie North site (Supplementary Figure 13). Furthermore, although the presence of A. lagunensis blooms could not be included in the Eau Gallie North model, it was included in the Eau Gallie Pier model and did not appear to have a significant effect on Pseudo-nitzschia spp. or P. bahamense abundance at that site. However, the absence of P. bahamense in 2019 could not be explained by the simple local predictors explored here.
Kernel density plots were used to visualize the distribution of blooms of A. lagunensis, P. bahamense, Pseudo-nitzschia spp., and the novel cyanobacterium in temperature and salinity space (Figure 10). These distributions allowed us to roughly estimate the realized niches of these taxa during our study period as bounded by these two parameters. One caveat is that the data for the novel cyanobacterium are only from June to December of 2020, so interpretations of these data must be taken with caution and regarded as a potentially incomplete characterization. Still, these comparisons are useful to understand potential for overlap of bloom levels of the different taxa in temperature-salinity space. Bloom occurrence of P. bahamense had the narrowest temperature range (on the higher end of the temperature spectrum, Figure 10B), whereas the novel cyanobacterium had the narrowest salinity range (on the lower end of the salinity spectrum, Figure 10A). Of the four taxa, A. lagunensis has the broadest temperature-salinity niche overall which support our other observations (Figure 10C). Notably, the temperature-salinity distribution of the novel cyanobacterium appeared to overlap the temperature-salinity space of highest occurrence of A. lagunensis blooms. The pattern established by the Pseudo-nitzschia indicated a higher affinity for more saline waters of the genus as a whole, as already detected in the Spearman ranking; the intersect with temperature, however, delimited different nuclei likely accounting for the presence of different taxa within the genus, some with more specific requirements for this parameter (Figure 10C). The kernel density distributions were also in general agreement with abundance relationships identified at single time-series sites via negative binomial regression models.
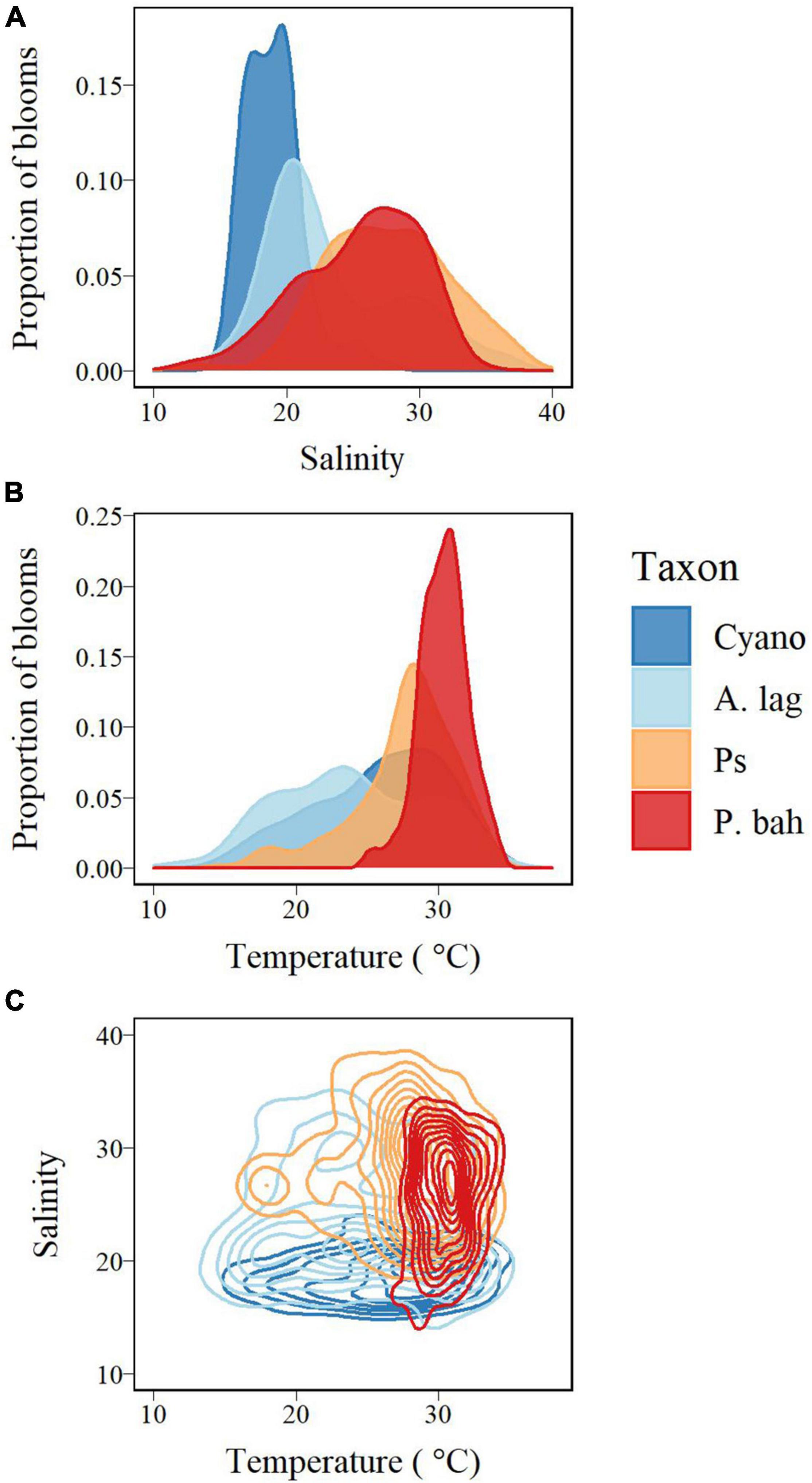
Figure 10. Kernel density plots for bloom distributions of the novel cyanobacterium (“Cyano,” dark blue), A. lagunensis (“A. lag,” light blue), Pseudo-nitzschia spp. (“Ps,” orange), and P. bahamense (“P. bah,” red) for (A) salinity, (B) temperature, and (C) salinity and temperature.
Discussion
Species-Level Tools for Understanding Bloom Dynamics
Monitoring of phytoplankton in the IRL has mostly relied on in situ chlorophyll-a fluorescence via deployed or adaptive instrumentation, bulk measures of phytoplankton biomass (e.g., chlorophyll-a concentrations), and light microscopy analysis of micro-, nano-, and picoplankton, and these approaches have limitations in terms of teasing apart and estimating nano- and picoplankton taxa of special interest, including bloom-forming species in the IRL. Measurements of chlorophyll-a cannot characterize phytoplankton community structure and may underrepresent the contribution of certain taxa (e.g., cyanobacteria) that may be responsible, at least in part, for bloom events. Microscopy can often be inadequate for small size classes of algae because target species cannot be easily distinguished from similarly sized, co-occurring algae; during routine monitoring, nano- and picoplankton are, thus, frequently placed in coarsely defined taxonomic groups (e.g., <15 μm flagellates). Antibody-based, molecular, and flow cytometric tools hold promise for routine and event response monitoring in the IRL because of their sensitivity and demonstrated ability to rapidly distinguish and enumerate small-sized phytoplankton in marine-influenced systems (Not et al., 2008; Vigil et al., 2009; Gobler et al., 2013; Koch et al., 2014). Molecular probes and spectrally selective fluorescence measurements can helpfully target unique features of harmful taxa (e.g., cellular antigens, nucleic acids, assorted pigments) and can operate at relevant taxonomic scales (e.g., population, species, genus, functional group, community). Because of this flexibility, these tools can be designed and implemented to address specific monitoring and management goals, such as identifying and tracking novel and/or specific taxa when limitations of microscopy preclude definitive identification, facilitating rapid screening of field samples, and/or characterizing transitions in community structure over relevant time scales. Many of these tools can even be adapted to screen archived material when samples are proactively preserved and stored according to appropriate protocols.
Immunofluorescence flow cytometry has been previously implemented in the IRL and other ecosystems for detecting the brown tide algae, A. lagunensis (Koch et al., 2014), and the qPCR assay described here is the first reported for this species. Both methods have advantages and disadvantages. The published methods and the availability of an antibody specific to A. lagunensis helped facilitate the integration of this approach into monitoring, and it was necessary to purify and conjugate remaining antibody stocks in 2020. Future efforts may require additional synthesis of antiserum and associated time, further use of animals, and re-validation to check for cross-reactivity, all of which carry their own costs. In contrast, qPCR probes/primers are easily obtained and synthesized, but require standards and other supplies not needed for flow cytometry, and there is greater potential for variability across users (Bustin et al., 2009; Gruselle et al., 2015); an added benefit is that archived nucleic acid samples can be readily used for subsequent assessments of other taxa. While results from all enumeration methods were correlated (Figures 2A,B), differing limits of quantification were observed for qPCR (145,000 cells L–1) vs. immunofluorescence flow cytometry (1,000 cells L–1), which limits our ability to directly compare coefficients of determination between regressions using the different methods. Despite the good correlation between methods targeting A. lagunensis, a subset of microscopy counts appeared to over- or underestimate cell abundance relative to the A. lagunensis-specific tools used, suggesting that morphological variability as well as technical biases can occasionally present meaningful discrepancies in the distinct data types. Indeed, cells resembling A. lagunensis are occasionally identified in other estuarine systems monitored by the FWC-FWRI, and the subsequent negative results provided by taxon-specific assays are invaluable (C. Tilney, E. Muhlbach, unpublished data). Given the complexity associated with identifying small-celled species, along with the need for timely data streams, the validation performed here provides further insight into the value of and how best to integrate multiple tools for monitoring these apparently non-descript, though often diverse, phytoplankton assemblages.
The A. lagunensis 18S rRNA sequence data showed that the CCMP1503 strain was genetically distinct from the UTEX2796 strain and from the dominant A. lagunensis ribotype present in the IRL in the 4 years examined (2012, 2016, 2018, 2020), which spanned different bloom events. The sequence was divergent enough to suggest a species-level difference of the CCMP1503 strain, which may be of interest for future studies of this organism. The order of magnitude decrease of immunofluorescence when probing CCMP1503 with the A. lagunensis polyclonal antibody agreed with the molecular data, showing that this isolate differs from A. lagunensis UTEX2796 (Supplementary Figure 1). The insertion region in the 18S rRNA of A. lagunensis could easily be used to discriminate these taxa via DNA-based methods, but not reliably by RNA-based methods since it appears to be spliced out.
The appearance of a novel high biomass bloom of an unidentified nano-sized cyanobacterium in 2020 provides further evidence of the shift to more frequent high biomass blooms of smaller-celled taxa in the IRL since the 2011 “superbloom” (Phlips et al., 2015, 2020, 2021). It is important to point out that the phytoplankton assemblage is rarely monospecific during blooms of these nanoplankton taxa, often including cells with morphological characters that are not readily distinguished by transmitted light microscopy used in routine analysis. Even when the dominant cell-type can be sorted out, such as in the present case, understanding the ecological requirements associated with the ability of opportunistic species in occupying likely open niches is far from being unraveled. This is particularly true for cyanobacteria, a group that has undergone extensive taxonomic restructuring in recent years, with the integration of molecular, cytomorphological, and ecophysiological approaches (Komárek et al., 2014). The closest relationship of our taxon to the genus Prochlorothrix is interesting, since the two described species (P. hollandica and P. scandica) are clearly freshwater filamentous species that lack phycobiliproteins (Burger-Wiersma et al., 1989; Pinevich et al., 1999; Velichko et al., 2013). The ability to form filaments may vary even within a species so further study of ultrastructural features, such as the arrangement of thylakoids, are needed to shed light onto the true relationship among these taxa. Likewise, molecular studies would be necessary to understand where each taxon stands in terms of having the genes associated with the expression of a particular pigment. At any rate, the clade within which our novel taxon landed depicts large genetic distances between taxa so that possible evolutionary and ecological affinities must be interpreted with caution, if at all, with the data at hand.
The high agreement between the molecular methods used here and the microscopy counts (of nanoplankton, i.e., A. lagunensis-like cells and the novel cyanobacterium) may ultimately build support for the accuracy of morphology-based microscopy enumeration of certain taxa in the IRL. However, this method is still quite time-consuming and requires dedicated taxonomic expertise. Furthermore, this supports the use of diverse methods for identifying both known and novel taxa in mixed assemblages to provide critical context that can help accurately monitor these algae and interpret their bloom dynamics.
Spatiotemporal Patterns, Ecological Niches, and Potential Modifying Factors
Of the four sub-basins, abundances of A. lagunensis and P. bahamense were highest in the Banana River Lagoon, and A. lagunensis blooms also persisted the longest in this sub-basin compared to other sub-basins. In 2018 and 2019, A. lagunensis bloom peaked and ebbed in Northern IRL and Central IRL, but it continually persisted in the Banana River Lagoon. Additionally, ANOSIM analysis supports the observation that taxon dynamics differed significantly in the Banana River Lagoon when compared to the Central IRL sub-basin. The Banana River Lagoon is a 50-km long lagoon with a 1.7-m average depth, with water exchange limited and primarily influenced by the flow of the Barge Canal and minimal flow propagating from the Sebastian Inlet to the south. There is also one direct but limited connection between the Banana River Lagoon and the Atlantic Ocean through the Port Canaveral Navigational Lock. As a result, water circulation in the Banana River Lagoon is low and influenced by winds, the water residence time is long, and the water quality is poor due to excess nutrients (Barile, 2018). Estimates to exchange 50% of this sub-basin water volume range from ∼70 to 156 d (Steward et al., 2005; Reyier et al., 2008), and this poorly flushed environment may contribute to the persistence and recurrence of blooms (Phlips et al., 2002).
Residence time of water bodies can be an important factor in bloom development, in part, because it may allow population growth to exceed losses, thus leading to biomass accumulation (Lucas et al., 2009). Lower water exchange may also foster phytoplankton resting stages to be retained and deposited in sediments, which may favor recurrence of species with resting stages in these environments. The formation of resting cysts also gives species a competitive advantage of “being in the right place at the right time” (Smayda and Reynolds, 2001) by providing a large input of cells to the water column upon germination. In Florida, P. bahamense proliferates in systems with long residence times (Phlips et al., 2006, 2011) and abundant resting cysts in sediments (Karlen and Campbell, 2012). Furthermore, a strong seasonal signal in bloom timing (summer) reflects the annual cycle of temperature that moderates P. bahamense life cycle transitions (Lopez et al., 2019) and growth rates (Usup et al., 1994). During 2015–2020, we found these relationships generally held true for the IRL, with the highest magnitude and longest duration blooms of P. bahamense occurring in the poorly flushed Banana River Lagoon, and the lowest magnitude and shortest duration blooms in Central IRL. Furthermore, we observed a strong and predictable seasonal signal in P. bahamense bloom occurrence in all sub-basins (mean bloom initiation date = JD 190 ± 8), and a narrow distribution of blooms in temperature space, which was also reflected in quadratic relationships with temperature at time series sites (Figure 10 and Table 2). On the other hand, the observed relatively broad salinity distribution for P. bahamense reflects the euryhaline nature of this species (Phlips et al., 2006; Usup et al., 2012). An additional competitive advantage of P. bahamense may be grazer deterrence through production of saxitoxins, concentrations of which can be high in the IRL and generally follow the pattern of P. bahamense abundance (Supplementary Figure 10). This capability may contribute to its success over other dinoflagellates with otherwise similar functional traits (Teegarden, 1999).
The delay or absence of P. bahamense blooms in some years or locations of our study suggest other factors can override its competitive advantages. When abundance of P. bahamense was modeled as a function of quadratic relationships with temperature and salinity, predicted values generally tracked observations—with higher abundance associated with warmer temperatures and higher salinities (up to a point before declining). Central IRL was the exception in which variability of P. bahamense abundance could not always be explained by the local predictors explored. Overall, blooms of P. bahamense in Central IRL were often delayed compared to other sub-basins and to modeled predictions, and this delay could be due to a combination of factors associated with shorter residence times. Faster transport of cells from the Central IRL sub-basin would increase cellular loss and, as a result, slow population growth and would also likely reduce the capacity for cyst accumulation in seed beds, which could in turn influence autochthonous initiation in Central IRL. Abundance of P. bahamense in Central IRL may also be positively influenced by transport of cells from other sub-basins and reflect abundance and processes in these connected sub-basins, but models that consider both transport and growth dynamics are needed to better tease apart these hypothesized dynamics in Central IRL. The presence of A. lagunensis blooms was also a significant predictor for P. bahamense and associated with an order of magnitude lower P. bahamense abundance in model predictions in Banana River Lagoon. These patterns were notable in observations across subbasins in years with high A. lagunensis abundance, where P. bahamense blooms were generally delayed and/or lower compared to other years and varied from what would be expected based on predicted germination (Figures 4–8 and Table 2). These results suggest that A. lagunensis blooms may modify the realized niche for P. bahamense through competitive interactions. Investigation of this hypothesis and potential underlying mechanisms (e.g., light or nutrient limitation, allelopathy, etc.) will be important for understanding systemic changes in the IRL and whether or not these interactions are merely episodic or have the potential for long term impacts (e.g., through disruption of the life cycle of P. bahamense).
Kernel density distributions of A. lagunensis blooms suggest that this taxon spanned a broad temperature and salinity space that overlapped those of the other prominent HAB taxa in the IRL (Figure 10). Additionally, A. lagunensis abundance was only weakly correlated with temperature (rs = –0.11, p < 0.001), blooms occurred in all seasons, and there was no evident temporal signal in abundance variability in our 6-year time series. The ∼22 month-long A. lagunensis bloom, which began late 2017 and persisted through mid-2019 in the Banana River Lagoon, proliferated after heavy rainfall associated with the passage of Hurricane Irma in 2017 and a subsequent decline in salinity. It is notable that the lower salinity in Banana River continued through 2020. In general, salinity can be influenced by the balance of rainfall and evaporation (FDEP, 2013), and also by the magnitude of groundwater contribution from both marine and terrestrial sources (e.g., Martin et al., 2007; Pandit et al., 2016); the mechanisms underlying the prevalence of low salinity in our study are unclear and cannot be determined from the data presented. Despite the observed association with the lower salinity shift in late 2017, there was no significant trend of A. lagunensis abundance with salinity, and prior studies have shown that A. lagunensis is tolerant and can proliferate over a wide range of salinities, and notably in hypersaline conditions (Buskey et al., 1998). Furthermore, the bloom of A. lagunensis that occurred prominently in Mosquito Lagoon and Northern IRL (but was observed in all sub-basins) in 2016 was associated with relatively higher salinities than in 2018. However, the 2016 A. lagunensis bloom in the IRL did notably coincide with El Niño and followed record breaking January precipitation levels (Supplementary Figure 8, see footnote 1, Phlips et al., 2020). These combined results support that A. lagunensis has a broad niche in temperature/salinity space in the IRL, which may facilitate its opportunism, but other factors associated with its functional characteristics, such as nutrient flexibility, allelopathy, and grazer resistance (Gobler et al., 2013; Kang et al., 2015), more likely influence its bloom occurrence.
The ability of A. lagunensis to take advantage of elevated ammonium concentrations (Buskey et al., 1997; DeYoe et al., 2007; Kang et al., 2015) and organic forms of nitrogen, phosphorus, and carbon (Buskey et al., 1997; Muhlstein and Villareal, 2007; Sunda and Hardison, 2007, 2010) has been attributed to bloom initiation and persistence in other locations (Buskey et al., 1998) and may help explain the proliferation success and persistence of A. lagunensis in the IRL. Analysis of these associations, however, is often confounded by the complexity of factors that may drive nutrient pulses and create conditions that allow these small-celled taxa to proliferate relative to other phytoplankton (Cira et al., 2021). For example, the Baffin Bay and Laguna Madre brown tide in the 1990’s occurred during a drought but followed fish kills (associated with a severe freezing event) that released large pulses of ammonium (DeYoe and Suttle, 1994; Buskey et al., 1997). Once high biomass blooms are established, blooms may be sustained in part by bacterial remineralization of nutrients (Gobler et al., 2013). Furthermore, additional mechanisms that limit biomass losses (such as long residence times and/or low grazing) may add layers of complexity by creating a positive feedback for sustaining bloom levels (Sunda et al., 2006). We do not present dissolved nutrient data, which is often lower resolution than is needed to evaluate the rapid processes of nutrient response and turnover (Brun et al., 2015). Our current inability to resolve short-timescale field nutrient dynamics coupled with a lack of obvious temporal patterns in A. lagunensis blooms in the IRL makes it difficult to discern relative roles of different controlling factors. However, the event-scale associations observed here and in the literature point to a likely role of nutrient pulses and disturbance in the initiation of blooms for an opportunistic taxon such as A. lagunensis.
Aureoumbra lagunensis can also enter a temporary resting stage in response to environmental stressors, such as darkness, nutrient depletion or elevated temperature, but can quickly revert back to vegetative (dividing) cells when favorable conditions return (Kang et al., 2017). The significance or role of this temporary life stage remains unclear, but it could be important for the organism’s opportunism and persistence. Kang and Gobler (2018) also reported allelopathic effects of brown tide taxa on other phytoplankton, such as reductions in the photosynthetic efficiency and growth rate as well as cell lysis and cell mortality. Aureoumbra lagunensis can also inhibit its consumption by grazers (Buskey et al., 1997; Sunda et al., 2006). These allelopathic and inhibitory qualities, in addition to nutrient flexibility/uptake, likely contribute to this alga’s ability to sustain cellular growth and minimize cellular losses, and thus, persist in an ecosystem once established. Longer time series, coupled with new detection tools and approaches that target A. lagunensis and other small-celled taxa, may reveal common associations with atypical events that help us better understand the interaction of functional traits and the mechanisms underlying bloom variability.
Pseudo-nitzschia spp. occupy a relatively broad temperature/salinity space that partially overlaps that of A. lagunensis and P. bahamense, although the most frequent distribution of blooms is in a higher salinity range than the other taxa (Figure 10). Time-series analysis also suggested that Pseudo-nitzschia spp. had a significant quadratic relationship with salinity, indicating that abundance increased as salinity increased to a certain point (i.e., the optimal threshold) and then declined at salinity levels above that point. Pseudo-nitzschia generally exhibits a cosmopolitan distribution and, even though some species may be more prevalent in either oceanic, coastal, and/or estuarine systems, the association of higher cell abundances with elevated salinities can be expected and are indicative of the marine nature of the genus (Bates et al., 2018 and references therein). The relationship of Pseudo-nitzschia spp. abundance with temperature was not consistent among basins (e.g., positive relationship in Central IRL and negative in Banana River Lagoon), and density distributions along the temperature spectrum were bimodal (Figure 10), which may be related to different temperature optima among various species of the genus.
The overall needle-shape outline of Pseudo-nitzschia is deceivingly simple. The diatom cell wall is remarkably variable from species to species at the ultrastructure level, and the elongated shape represents a relatively large surface-to-volume ratio for microphytoplankton; that is, Pseudo-nitzschia spp. from the IRL hosts several species, each with distinct abilities to respond quickly to environmental cues. Pseudo-nitzschia spp. differed from the other taxa in that blooms were observed every year in Central IRL, the sub-basin with the shortest residence times (∼ 15 times shorter than the Banana River Lagoon) due to higher tidal exchange and higher annual surface runoff (Steward and Green, 2007). Pseudo-nitzschia spp. may be more competitive in this environment, due to its marine nature and ability to respond quickly. Comparatively, other sub-basins displayed greater interannual variability in Pseudo-nitzschia spp. abundance. The longest duration blooms in Banana River Lagoon, Northern IRL, and Central IRL occurred in 2017, a drought year with a dry spring and summer and significantly higher mean salinity than other years (prior to Hurricane Irma). We note that although specific species identification of Pseudo-nitzschia was not conducted as part of our monitoring effort in the IRL, the detection of the toxin, domoic acid, in some of the water samples tested over the course of this study period points to the presence of at least some toxin-producing species in this ecosystem. Of the >56 species of Pseudo-nitzschia described to date, about half are known to produce domoic acid, and intracellular toxin content varies not only between species but is also dependent on the physiological status of any given toxin producer (Bates et al., 2018 and references therein). Domoic acid detected in the summer of 2020 in the Mosquito Lagoon was associated with cell abundances > 1.2 × 107 cells L–1 and salinity of ∼34 psu. The intersect of domoic acid and salinity variability is especially interesting to explore in coastal and estuarine systems since laboratory results indicate species-specific trends toward increased domoic acid production at the higher salinity ranges of acclimated monocultures under controlled conditions (e.g., Doucette et al., 2008; Ayache et al., 2020).
Understanding Responses to Large-Scale Perturbations
Brown tides caused by pelagophytes Aureococcus anophagefferens and Aureoumbra lagunensis can disrupt marine ecosystems and have damaging effects on marine fauna and flora (Gobler and Sunda, 2006, 2012). Blooms of these organisms not only have detrimental effects on the benthos, zooplankton, and suspension-feeding bivalves (Bricelj and Lonsdale, 1997), but also destroy seagrass meadows by limiting the light penetration in the water column (Onuf, 1996). One characteristic of these brown tides is that once blooms are established in an ecosystem, they tend to recur and can persist for several months and even years before subsiding (Buskey et al., 2001; Gobler and Sunda, 2012). While factors that select for occurrence of these blooms are not always clear (Cira and Wetz, 2019), a common thread among these brown tide blooms and those in other systems is that they occur in shallow estuarine environments with relatively long residence times and are often preceded by changes in nutrient fluxes or other atypical conditions (Buskey et al., 1998; Gobler et al., 2005, 2011; Cira and Wetz, 2019). Our results support the hypothesis that rapid environmental changes may provide an opportunity for these organisms to take hold, especially if cells are already beginning to proliferate (Buskey et al., 1998). Indeed, the dominance of this type of event-scale variability that we observe in A. lagunensis blooms (compared to variability driven by processes related to the annual climate cycle) has been associated with high nutrient environments in which “exceptional blooms” respond to a disturbance, such as a weather event (Cloern and Jassby, 2010). Large perturbations like hurricanes are often associated with rapid environmental changes, including enhanced nutrient supply which is notably important for A. lagunensis (DeYoe and Suttle, 1994; Kang et al., 2015) and may have had a role in the initiation of the 2018–2019 bloom in the IRL.
High biomass blooms of nano- and pico-sized harmful algae have been prevalent in the IRL over the last decade even though environmental conditions preceding or coinciding with these blooms have varied (Kamerosky et al., 2015; Phlips et al., 2015, 2020). That high biomass blooms are themselves large scale perturbations of the ecosystem raises the question of what is required for the system to break away from the tendency toward recurrent high-biomass plankton assemblages to low-biomass ones. The different patterns associated with target species as well as the recent entry of a novel cyanobacterial bloom across much of the IRL provides a new opportunity to evaluate factors driving the recurrence of high biomass blooms in the IRL. Understanding the drivers of this unique cyanobacterial bloom via a comprehensive suite of approaches, may help determining the physical, chemical, and biological features that facilitated this unusual bloom, and potentially reveal what may normally maintain—or reduce—A. lagunensis blooms. It is interesting to speculate whether the novel cyanobacterium was filling a similar niche that favored A. lagunensis, as potentially suggested by its 2020 environmental distribution, and simply benefited from stochastic selection (Smayda and Reynolds, 2001), or alternatively whether it had other functional traits that allowed it to fill a niche that A. lagunensis could not have optimally used. The novelty of this species might support the latter hypothesis, and continued monitoring in combination with a deeper analysis of the consortium of species in the IRL could help reveal the ecology underlying the proliferation of nanoplankton HABs. Furthermore, the extent to which these recurring bloom events represent ecosystem stressors tied to widespread mortality of seagrasses, fishes, manatees, and/or other critical biota in the IRL underscores the need for a systemic understanding that can inform management actions and changes.
A variety of approaches will be needed to understand past, present, and future ecosystem shifts in the IRL—one of these is the availability of long duration, high frequency time series of specific phytoplankton abundance. These types of data sets are relatively rare, especially for smaller sized taxa, but are gaining support from integration of new identification tools that make these types of species-level time series more attainable and will be critical for better understanding of processes driving blooms, including ecosystem responses to small- and large-scale disturbances as well as systemic changes over time.
Data Availability Statement
The sequence data presented in this study are deposited in the GenBank repository (ncbi.nlm.nih.gov/genbank), accession numbers MW812272–MW812281 and MW816502. Data on HAB abundance are available via the FWC-FWRI HAB Monitoring Database (https://myfwc.com/research/redtide/monitoring/database/). Continuous monitoring data are available via the SJRWMD data portal at (https://www.sjrwmd.com/data/). Daily mean air temperature and precipitation data are available from the Florida Climate Center (https://climatecenter.fsu.edu/).
Author Contributions
KAH, RP, DA, EM, CT, MV, and CL contributed to the concept and designed of the study. RP, DA, DE, JS, KW, AB, SL, ML, AP, LH, EP, SB, LF, and MG designed and carried out the field sampling. MV, KLH, LM, SA, SB, and EP conducted the microscopic analysis. EM and CT conducted flow cytometry and molecular analysis. LF and SS conducted the toxin analysis. CL, MV, LF, and SS synthesized field data. CS, MV, and CL conducted the statistical analyses. CL, JB, CT, MV, CS, EM, and KAH wrote the manuscript. All authors contributed to the manuscript revisions and approved the submitted version.
Funding
This study was funded by the Florida Fish and Wildlife Conservation Commission, the Saint Johns River Water Management District (in kind), grants from the Indian River Lagoon National Estuary Program, and U.S. Fish and Wildlife Service, Merritt Island National Wildlife Refuge (Contract F20AC00172).
Conflict of Interest
The authors declare that the research was conducted in the absence of any commercial or financial relationships that could be construed as a potential conflict of interest.
The handling editor is currently organizing a Research Topic with one of the author EP.
Publisher’s Note
All claims expressed in this article are solely those of the authors and do not necessarily represent those of their affiliated organizations, or those of the publisher, the editors and the reviewers. Any product that may be evaluated in this article, or claim that may be made by its manufacturer, is not guaranteed or endorsed by the publisher.
Acknowledgments
We acknowledge logistical support from Christopher Gobler, Alina Corcoran, Sue Murasko, Karen Vaughan, and Chuck Jacoby. We acknowledge additional scientific staff from FWC-FWRI, the SJRWMD, and Cape Canaveral National Seashore that assisted with field sampling and coordination, including the deployment and maintenance of in situ instrumentation. We would like to thank all of partners, including the Florida Department of Agricultural and Consumer Services, and volunteers who collected samples. Special thanks go to Marine Resources Council volunteers Jim Torpey and Tom Saam for their weekly sample collection as part of the citizen science network. We are grateful to the FWRI staff that contributed microscopy and database support, to Alicia Hoeglund, Kelsey Marvin, and others who assisted with genetic and flow cytometric analyses, and to April Granholm for domoic acid analysis. We thank Lauren Peacock for her assistance with analysis of in situ datastreams. We would also like to thank the editorial and scientific reviewers from FWRI for their helpful and constructive comments.
Supplementary Material
The Supplementary Material for this article can be found online at: https://www.frontiersin.org/articles/10.3389/fmars.2021.769877/full#supplementary-material
Footnotes
References
Adams, D. H., Tremain, D. M., Paperno, R., and Sonne, C. (2019). Florida lagoon at risk of ecosystem collapse. Science 365, 991–992. doi: 10.1126/science.aaz0175
Akaike, H. (1973). “Information theory and an extension of the maximum likelihood principle,” in Proceedings of the 2nd International Symposium on Information Theory, eds B. Petrov and F. Csáki (Budapest: Akadémiai Kiadó), 267–281.
Anderson, D. M., Fensin, E., Gobler, C. J., Hoeglund, A. E., Hubbard, K. A., Kulis, D. M., et al. (2021). Marine harmful algal blooms (HABs) in the United States: history, current status and future trends. Harmful Algae 102:101975. doi: 10.1016/j.hal.2021.101975
Anderson, M. J., Gorley, R. N., and Clarke, K. R. (2008). PERMANOVA+ for PRIMER: Guide to Software and Statistical Methods. Plymouth: PRIMER-E Ltd.
Ayache, N., Hervé, F., Lundholm, N., Amzil, Z., and Caruana, A. M. N. (2020). Acclimation of the marine diatom Pseudo-nitzschia australis to different salinity conditions: effects on growth, photosynthetic activity, and domoic acid content. J. Phycol. 56, 97–109. doi: 10.1111/jpy.12929
Badylak, S., and Phlips, E. J. (2004). Spatial and temporal patterns of phytoplankton composition in a subtropical coastal lagoon, the Indian River Lagoon. Florida, USA. J. Plankton Res. 26, 1229–1247. doi: 10.1093/plankt/fbh114
Barile, P. J. (2018). Widespread sewage pollution of the Indian River Lagoon system, Florida (USA) resolved by spatial analyses of macroalgal biogeochemistry. Mar. Pollut. Bull. 128, 557–574. doi: 10.1016/j.marpolbul.2018.01.046
Barton, K. (2020). MuMIn: Multi-Model Inference. R Package Version 1.43.17. Available online at: https://CRAN.R-project.org/package=MuMIn (accessed April 15, 2020).
Bates, S. S., Hubbard, K. A., Lundholm, N., Montresor, M., and Leawm, C. P. (2018). Pseudo-nitzschia, Nitzschia, and domoic acid: new research since 2011. Harmful Algae 79, 3–43. doi: 10.1016/j.hal.2018.06.001
Bricelj, V. M., and Lonsdale, D. J. (1997). Aureococcus anophagefferens: causes and ecological consequences of brown tides in U.S. mid-Atlantic coastal waters. Limnol. Oceanogr. 42, 1023–1038. doi: 10.4319/lo.1997.42.5_part_2.1023
Brooks, M. E., Kristensen, K., van Benthem, K. J., Magnusson, A., Berg, C. W., Nielsen, A., et al. (2017). glmmTMB balances speed and flexibility among packages for zero-inflated generalized linear mixed modeling. R J. 9, 378–400. doi: 10.32614/rj-2017-066
Brun, P., Vogt, M., Payne, M. R., Gruber, N., O’Brien, C. J., Buitenhuis, E. T., et al. (2015). Ecological niches of open ocean phytoplankton taxa. Limnol. Oceanogr. 60, 1020–1038. doi: 10.1002/lno.10074
Burger-Wiersma, T., Stal, L. J., and Mur, L. R. (1989). Prochlorothrix hollandica gen. nov., sp. nov., a filamentous oxygenic photoautotrophic procaryote containing chlorophylls a and b: assignment to Prochlorotrichaceae fam. nov. and order Prochlorales Florenzano, Balloni, and Materassi 1986, with emendation of the ordinal description. Int. J. Syst. Bacteriol. 39, 250–257. doi: 10.1099/00207713-39-3-250
Burnham, K. P., and Anderson, D. R. (2002). A Practical Information-Theoretic Approach. Model Selection and Multimodel Inference, 2nd Edn. New York, NY: Springer.
Buskey, E. J., Liu, H. B., Collumb, C., and Bersano, J. G. F. (2001). The decline and recovery of a persistent Texas brown tide algal bloom in the Laguna Madre (Texas. USA). Estuaries 24, 337–346. doi: 10.2307/1353236
Buskey, E. J., Montagna, P. A., Amos, A. F., and Whitledge, T. E. (1997). Disruption of grazer populations as a contributing factor to the initiation of the Texas brown tide algal bloom. Limnol. Oceanogr. 42, 1215–1222. doi: 10.4319/lo.1997.42.5_part_2.1215
Buskey, E. J., Wysor, B., and Hyatt, C. (1998). The role of hypersalinity in the persistence of the Texas ‘brown tide’ in the Laguna Madre. J. Plankton Res. 20, 1553–1565.
Bustin, S. A., Benes, V., Garson, J. A., Hellemans, J., Huggett, J., Kubista, M., et al. (2009). The MIQE guidelines: minimum information for publication of quantitative real-time PCR experiments. Clin. Chem. 55, 611–622. doi: 10.1373/clinchem.2008.112797
Cira, E., Palmer, T., and Wetz, M. (2021). Phytoplankton dynamics in a low-inflow estuary (Baffin Bay, TX) during drought and high-rainfall conditions associated with an El Niño event. Estuar. Coast 44, 1752–1764. doi: 10.1007/s12237-021-00904-7
Cira, E., and Wetz, M. S. (2019). Spatial-temporal distribution of Aureoumbra lagunensis (“brown tide”) in baffin bay, Texas. Harmful Algae 89:101669. doi: 10.1016/j.hal.2019.101669
Cloern, J. E., and Jassby, A. (2010). Patterns and scales of phytoplankton variability in estuarine–coastal ecosystems. Estuar. Coast 33, 230–241. doi: 10.1098/rstb.2010.0125
DeYoe, H. R., Buskey, E. J., and Jochem, F. J. (2007). Physiological responses of Aureoumbra lagunensis and Synechococcus sp. to nitrogen addition in a mesocosm study. Harmful Algae 6, 48–55. doi: 10.1016/j.hal.2006.06.001
DeYoe, H. R., Stockwell, D. A., Biolagare, R. R., Latasa, M., Johnson, P. W., Hargraves, P. E., et al. (1997). Description and characterization of the algal species Aureoumbra lagunensis eng. et sp. Nov. and referral of Aureoumbra and Aureococcus to the pelagophyceae. J. Phycol. 33, 1042–1048. doi: 10.1111/j.0022-3646.1997.01042.x
DeYoe, H. R., and Suttle, C. A. (1994). The inability of the Texas “brown tide” alga to use nitrate and the role of nitrogen in the initiation of a persistent bloom of this organism. J. Phycol. 30, 800–806. doi: 10.1111/j.0022-3646.1994.00800.x
Diaz, R. J., and Rosenberg, R. (2008). Spreading dead zones and consequences for marine ecosystems. Science 321, 926–929. doi: 10.1126/science.1156401
Doucette, G. J., King, K. L., Thessen, A. E., and Dortch, Q. (2008). The effect of salinity on domoic acid production by the diatom Pseudo-nitzschia multiseries. Nova Hedwigia 133:31. doi: 10.1111/j.1439-0485.1998.tb00451.x
Edler, L., and Elbrachter, M. (2010). “The Utermohl method for quantitative phytoplankton analysis,” in Microscopic and Molecular Methods for Quantitative Phytoplankton Analysis, IOC Manuals and Guides, no. 55, eds B. Karlson, C. Cusack, and E. Bresnan (Paris: UNESCO), 13–20. doi: 10.1002/jemt.20338
Fire, S., Flewelling, L., Stolen, M., Durden, W., Wit, M., Spellman, A. C., et al. (2015). Brevetoxin-associated mass mortality event of bottlenose dolphins and manatees along the east coast of Florida, USA. Mar. Ecol. Prog. Ser. 526, 241–251. doi: 10.3354/meps11225
FDEP (2013). Final TMDL Report: Nutrient TMDLS for Sykes Creek/Barge Canal. April 9, 2013. Tallahassee, FL: Florida Department of Environmental Protection.
Gobler, C. J., Berry, D. L., Dyhrman, S. T., Wilhelm, S. W., Salamov, A., Lobanov, A. V., et al. (2011). Niche of harmful alga Aureococcus anophagefferens revealed through ecogenomics. PNAS 108, 4352–4357. doi: 10.1073/pnas.1016106108
Gobler, C. J., Koch, F., Karig, Y., Berry, D. L., Tang, Y. Z., Lasi, M., et al. (2013). Expansion of harmful brown tides caused by the pelagophyte, Aureoumbra lagunensis DeYoe et Stockwell, to the US east coast. Harmful Algae 27, 29–41. doi: 10.1016/j.hal.2013.04.004
Gobler, C. J., Lonsdale, D. J., and Boyer, G. L. (2005). A review of the causes, effects, and potential management of harmful brown tide blooms caused by Aureococcus anophagefferens (Hargraves et Sieburth). Estuaries 28, 726–749. doi: 10.1007/bf02732911
Gobler, C. J., and Sunda, W. G. (2006). “Brown tides,” in Ecology of Harmful Algae, eds E. Granéli and J. T. Turner (Berlin: Springer), 111–123. doi: 10.1007/978-3-540-32210-8_9
Gobler, C. J., and Sunda, W. G. (2012). Ecosystem disruptive algal blooms of the brown tide species, Aureococcus anophagefferens and Aureoumbra lagunensis. Harmful Algae 14, 36–45. doi: 10.1016/j.hal.2011.10.013
Gruselle, O., Coche, T., and Louahed, J. (2015). Development of a quantitative real-time RT-PCR assay for the detection of MAGE-A3–positive tumors. J. Mol. Diagn. 17, 382–391. doi: 10.1016/j.jmoldx.2015.03.008
Hardin, J. W., and Hilbe, J. M. (2007). Generalized Linear Models and Extensions. College Station, TX: Stata Press.
Hargraves, P. E. (2020). “Microalgal diversity in the Indian River Lagoon system: how little we know,” in Proceedings of the Indian River Lagoon Symposium 2020, (Fort Pierce FL: Florida Atlantic University).
Hartig, F. (2021). DHARMa: Residual Diagnostics for Hierarchical (multi-level/mixed) Regression Models. R package Version 0.4.1. Available online at: https://CRAN.R-project.org/package=DHARMa (accessed September 28, 2021).
Hurvich, C. M., and Tsai, C. L. (1989). Regression and time series model selection in small samples. Biometrika 76, 297–307. doi: 10.1093/biomet/76.2.297
Hutchinson, G. E. (1957). Concluding remarks. Cold Spring Harb. Symp. Quant. Biol. 22, 415–427. doi: 10.1101/SQB
Irwin, A. J., Nelles, A. M., and Finkel, Z. V. (2012). Phytoplankton niches estimated from field data. Limnol. Oceanogr. 57, 787–797. doi: 10.4319/lo.2012.57.3.0787
Iteman, I., Rippka, R., Tandeau de Marsac, N., and Herdman, M. (2000). Comparison of conserved structural and regulatory domains within divergent 16S rRNA-23S rRNA spacer sequences of cyanobacteria. Microbiology 146, 1275–1286. doi: 10.1099/00221287-146-6-1275
Kamerosky, A., Cho, H., and Morris, L. (2015). Monitoring of the 2011 super algal bloom in Indian River Lagoon, FL, USA, using MERIS. Remote Sens. 7, 1441–1460. doi: 10.3390/rs70201441
Kang, Y., and Gobler, C. J. (2018). The brown tide algae, Aureococcus anophagefferens and Aureoumbra lagunensis (Pelagophyceae), allelopathically inhibit the growth of competing microalgae during harmful algal blooms. Limnol. Oceanogr. 63, 985–1003. doi: 10.1002/lno.10714
Kang, Y., Koch, F., and Gobler, C. (2015). The interactive roles of nutrient loading and zooplankton grazing in facilitating the expansion of harmful algal blooms caused by the pelagophyte, Aureoumbra lagunensis, to the Indian River Lagoon, FL, USA. Harmful Algae 49, 162–173. doi: 10.1016/j.hal.2015.09.005
Kang, Y., Tang, Y. -Z., Taylor, G. T., and Gobler, C. J. (2017). Discovery of a resting stage in the harmful, brown-tide-causing pelagophyte, Aureoumbra lagunensis: a mechanism potentially facilitating recurrent blooms and geographic expansion. J. Phycol. 53, 118–130. doi: 10.1111/jpy.12485
Karasiewicz, S., Chapelle, A., Bacher, C., and Soudant, D. (2020). Harmful algae niche responses to environmental and community variation along the French coast. Harmful Algae 93:101785. doi: 10.1016/j.hal.2020.101785
Karasiewicz, S., Dolédec, S., and Lefebvre, S. (2017). Within outlying mean indexes: refining the OMI analysis for the realized niche decomposition. PeerJ 5:e3364. doi: 10.7717/peerj.3364
Karlen, D., and Campbell, K. (2012). The Distribution of Pyrodinium Bahamense Cysts in Old Tampa Bay Sediments. Tampa Bay Estuary Program Technical Report #07-12. Tampa, FL: Environmental Protection Commission of Hillsborough County, 41.
Kim, Y. T. (2003). Water balance and flushing time in the restricted Indian River Lagoon (IRL), Florida USA. Ocean Polar Res. 25, 75–87. doi: 10.4217/OPR.2003.25.1.075
Kleppel, G. S. (1996). The State of Florida’s Estuaries and Future Needs in Estuarine Research. Florida Sea Grant College Program. The Florida Sea Grant College Program, Technical Paper 85. Gainesville, FL: University of Florida, 43–51.
Koch, F., Kang, Y., Villareal, T. A., Anderson, D. M., and Gobler, C. J. (2014). A novel immunofluorescence flow cytometry technique detects the expansion of brown tides caused by Aureoumbra lagunensis to the Caribbean Sea. Appl. Environ. Microb. 80, 4947–4957. doi: 10.1128/AEM.00888-14
Komárek, J., Kaštovský, J., Mareš, J., and Johansen, J. R. (2014). Taxonomic classification of cyanoprokaryotes (cyanobacterial genera) 2014, using a polyphasic approach. Preslia 86, 295–335.
Landsberg, J. H., Hall, S., Johannessen, J. N., White, K. D., Conrad, S. M., Abbott, J. P., et al. (2006). Saxitoxin puffer fish poisonning in the United States, with the first report of Pyrodinium bahamense as the putative toxin source. Environ. Health Perspect. 114, 1502–1507. doi: 10.1289/ehp.8998
Lapointe, B. E., Herren, L. W., Brewton, R. A., and Alderman, P. K. (2020). Nutrient over-enrichment and light limitation of seagrass communities in the Indian River Lagoon, an urbanized subtropical estuary. Sci. Total Environ. 699:134068. doi: 10.1016/j.scitotenv.2019.134068
Lapointe, B. E., Herren, L. W., Debortoli, D. D., and Vogel, M. A. (2015). Evidence of sewage-driven eutrophication and harmful blooms in Florida’s Indian River Lagoon. Harmful Algae. 43, 82–102. doi: 10.1016/J.HAL.2015.01.004
Lawrence, J. F., Niedzwiadek, B., and Menard, C. (2005). Quantitative determination of paralytic shellfish poisoning toxins in shellfish using prechromatographic oxidation and liquid chromatography with fluorescence detection: collaborative study. J. AOAC Int. 88, 1714–1732. doi: 10.1093/jaoac/88.6.1714
Lopez, C. B., Karim, A., Murasko, S., Marot, M., Smith, C. G., and Corcoran, A. A. (2019). Temperature mediates secondary dormancy in resting cysts of Pyrodinium bahamense (Dinophyceae). J. Phycol. 55, 924–935. doi: 10.1111/jpy.12883
Lopez, C. B., Shankar, S., Kaminski, S. G., Garrett, M., and Hubbard, K. A. (2021). Linking Pyrodinium Bahamense Physiology and Behavior to Population Growth and Loss in Nature and Implications for Management. TBEP Technical Report # 07-21. St. Petersburg, FL: Florida Fish and Wildlife Conservation Commission.
Lopez-Barrerio, T., Villareal, T. A., and Morton, S. L. (1998). “Development of an antibody against the Texas brown tide Aureoumbra lagunensis,” in Proceedings of the 8th International Conference on Harmful Algae Xunta de Galicia and Intergovernmental Oceanographic Commission of UNESCO, eds B. Reguera, J. Blanco, M. L. Fernandez, and T. Wyatt (Paris: UNESCO), 263–265.
Lucas, L. V., Thompson, J., and Brown, L. R. (2009). Why are diverse relationships observed between phytoplankton biomass and transport time. Limnol. Oceanogr. 54, 381–390. doi: 10.4319/lo.2009.54.1.0381
Martin, J., Cable, J., Smith, C., Roy, M., and Cherrier, J. (2007). Magnitudes of submarine groundwater discharge from marine and terrestrial sources: Indian River Lagoon, Florida. Water Resour. Res. 43:W05440. doi: 10.1029/2006WR005266
Medlin, L., Elwood, H. J., Stickel, S., and Sogin, M. L. (1988). The characterization of enzymatically amplified eukaryotic 16S-like rRNA-coding regions. Gene 71, 491–499. doi: 10.1016/0378-1119(88)90066-2
Melo-Merino, S. M., Reyes-Bonilla, H., and Lira-Noriega, A. (2020). Ecological niche models and species distribution models in marine environments: a literature review and spatial analysis of evidence. Ecol. Model. 415:108837. doi: 10.1016/j.ecolmodel.2019.108837
Morris, L., Hall, L., Chamberlain, R., and Jacoby, C. (2018). “Summary report for the Northern Indian River Lagoon,” in Seagrass Integrated Mapping and Monitoring Report No. 3. Fish and Wildlife Research Institute Technical Report TR-17 version 3, eds L. A. Yarbro and P. R. Carlson (St. Petersburg: Florida Fish and Wildlife Conservation Commission).
Muhlstein, H. I., and Villareal, T. A. (2007). Organic and inorganic nutrient effects on growth rate–irradiance relationships in the Texas brown-tide alga Aureoumbra lagunensis (Pelagophyceae). J. Phycol. 43, 1223–1226. doi: 10.1111/j.1529-8817.2007.00412.x
Not, F., Latasa, M., Scharek, R., Viprey, M., Karleskind, P., Balagué, V., et al. (2008). Protistan assemblages across the Indian Ocean, with a specific emphasis on the picoeukaryotes. Deep Sea Res. Part I Oceanogr. Res. Papers 55, 1456–1473. doi: 10.1016/j.dsr.2008.06.007
Nübel, U., Garcia-Pichel, F., and Muyzer, G. (1997). PCR primers to amplify 16S rRNA genes from cyanobacteria. Appl. Environ. Microb. 63, 3327–3332. doi: 10.1128/aem.63.8.3327-3332.1997
Onuf, C. (1996). Seagrass responses to long-term light reduction by brown tide in upper Laguna Madre, Texas: distribution and biomass patterns. Mar. Ecol. Prog. Ser. 138, 219–231. doi: 10.3354/meps138219
Pandit, A., Ali, N., Heck, H., and Mamoua, K. (2016). Estimation of submarine groundwater discharge into the Indian River Lagoon. Austin J. Irrigat. 2:08.
Phlips, E., Badylak, S., Christman, M., Wolny, J., Brame, J., Garland, J., et al. (2011). Scales of temporal and spatial variability in the distribution of harmful algae species in the Indian River Lagoon, Florida, USA. Harmful Algae 10, 277–290. doi: 10.1016/j.hal.2010.11.001
Phlips, E., Badylak, S., Lasi, M., Chamberlain, R., Green, W., Hall, L., et al. (2015). From red tides to green and brown tides: bloom dynamics in a restricted subtropical lagoon under shifting climatic conditions. Estuar. Coast. 38, 886–904. doi: 10.1007/s12237-014-9874-6
Phlips, E. J., Badylak, S., Bledsoe, E., and Cichra, M. (2006). Factors affecting the distribution of Pyrodinium bahamense var. bahamense in coastal waters of Florida. Mar. Ecol. Prog. Ser. 322, 99–115. doi: 10.3354/meps322099
Phlips, E. J., Badylak, S., Christman, M. C., and Lasi, M. A. (2010). Climatic trends and temporal patterns of phytoplankton composition, abundance and succession in the Indian River Lagoon, Florida, USA. Estuar. Coast. 33, 498–512. doi: 10.1007/s12237-009-9166-8
Phlips, E. J., Badylak, S., and Grosskopf, T. (2002). Factors affecting the abundance of phytoplankton in a restricted subtropical lagoon, the Indian River Lagoon, Florida, USA. Estuar. Coast. Shelf Sci. 55, 385–402. doi: 10.1006/ecss.2001.0912
Phlips, E. J., Badylak, S., Nelson, N. G., Hall, L. M., Jacoby, C. A., Lasi, M. A., et al. (2021). Cyclical Patterns and a regime shift in the character of phytoplankton blooms in a restricted sub-tropical Lagoon, Indian River Lagoon, Florida, United States. Front. Mar. Sci 8:730934. doi: 10.3389/fmars.2021.730934
Phlips, E. J., Badylak, S., Nelson, N. G., and Havens, K. E. (2020). Hurricanes, El Niño and harmful algal blooms in two sub-tropical Florida estuaries: direct and indirect impacts. Sci. Rep. 10:1910. doi: 10.1038/s41598-020-58771-4
Pinevich, A. V., Skulberg, O., Matthijs, H. C. P., Schubert, H., Willen, E., Gavrilova, O. V., et al. (1999). Characterization of a novel chlorophyll b-containing Prochlorothrix species (Prochlorophyta) and its photosynthetic apparatus. Microbios 100, 159–174.
R Core Team (2020). R: A Language and Environment for Statistical Computing. Vienna: Statistical R Foundation for Computing.
Ramakers, C., Ruijter, J. M., Deprez, R. H. L., and Moorman, A. F. M. (2003). Assumption-free analysis of quantitative real-time polymerase chain reaction (PCR) data. Neurosci. Lett. 339, 62–66. doi: 10.1016/s0304-3940(02)01423-4
Reyier, E., Shenker, J., and Christian, D. (2008). Role of an estuarine fisheries reserve in the production and export of ichthyoplankton. Mar. Ecol. Prog. Ser. 359, 249–260. doi: 10.3354/meps07324
Rice, P., Longden, I., and Bleasby, A. (2000). EMBOSS: the European molecular biology open software suite. Trends Genet. 16, 276–277. doi: 10.1016/s0168-9525(00)02024-2
Rocap, G., Distel, D. L., Waterbury, J. B., and Chisholm, S. W. (2002). Resolution of Prochlorococcus and Synechococcus ecotypes by using 16S-23S ribosomal DNA internal transcribed spacer sequences. Appl. Environ. Microb. 68, 1180–1191. doi: 10.1128/AEM.68.3.1180-1191.2002
SJRWMD (2012). Indian River Lagoon 2011 Superbloom Plan of Investigation. Technical Report, 26. Available online at: https://www.sjrwmd.com/static/waterways/irl-technical/2011superbloom_investigationplan_June_2012.pdf (accessed June 3, 2021).
Sheng, Y. P., and Davis, J. R. (2003). Indian River Lagoon Pollutant Load Reduction (IRLPLR) Model Development Project, Volume 1, A 3-D IRL Hydrodynamics/Salinity Model (UF-CH3D). Gainesville, FL: University of Florida.
Smayda, T. J., and Reynolds, C. S. (2001). Community assembly in marine phytoplankton: application of recent models to harmful dinoflagellate blooms. J. Plankton Res. 23, 447–461. doi: 10.1093/plankt/23.5.447
Steward, J., and Green, W. (2007). Setting load limits for nutrients and suspended solids based on seagrass depth-limit targets. Estuar. Coast. 30, 657–670. doi: 10.1007/BF02841963
Steward, J., Virnstein, R., Morris, L., and Lowe, E. (2005). Setting seagrass depth, coverage, and light targets for the Indian River Lagoon system, Florida. Estuar. Coast. 28, 923–935. doi: 10.1007/BF02696020
Sunda, W. G., Graneli, E., and Gobler, C. J. (2006). Positive feedback and the development and persistence of ecosystem disruptive algal blooms. J. Phycol. 42, 963–974. doi: 10.1111/j.1529-8817.2006.00261.x
Sunda, W. G., and Hardison, D. R. (2007). Ammonium uptake and growth limitation in marine phytoplankton. Limnol. Oceanogr. 52, 2496–2506. doi: 10.4319/lo.2007.52.6.2496
Sunda, W. G., and Hardison, D. R. (2010). Evolutionary tradeoffs among nutrient acquisition, cell size, and grazing defense in marine phytoplankton promote ecosystem stability. Mar. Ecol. Prog. Ser. 401, 63–76. doi: 10.3354/meps08390
Teegarden, G. (1999). Copepod grazing selection and particle discrimination on the basis of PSP toxin content. Mar. Ecol. Prog. Ser. 181, 163–176. doi: 10.3354/meps181163
Turner, S., Pryer, K. M., Miao, V. P. W., and Palmer, J. D. (1999). Investigating deep phylogenetic relationships among cyanobacteria and plastids by small subunit rRNA sequence analysis. J. Eukaryot. Microbiol. 46, 327–338. doi: 10.1111/j.1550-7408.1999.tb04612.x
Usup, G., Ahmad, A., Matsuoka, K., Lim, P. T., and Leaw, C. P. (2012). Biology, ecology and bloom dynamics of the toxic marine dinoflagellate Pyrodinium bahamense. Harmful Algae 14, 301–312. doi: 10.1016/j.hal.2011.10.026
Usup, G., Kulis, D. M., and Anderson, D. M. (1994). Growth and toxin production of the toxic dinoflagellate Pyrodinium bahamense var. compressum in laboratory cultures. Nat. Toxins 2, 254–262. doi: 10.1002/nt.2620020503
Velichko, N. V., Timofeyeva, A. S., Gavrilova, O. V., Averina, S. G., Ivanikova, N. V., and Pinevich, A. V. (2013). Polyphasic emended description of the filamentous prochlorophyte Prochlorothrix scandica Skulberg 2008. Algol. Stud. 141, 11–27. doi: 10.1127/1864-1318/2012/0044
Vigil, P., Countway, P. D., Rose, J., Lonsdale, D. J., Gobler, C. J., and Caron, D. A. (2009). Rapid shifts in dominant taxa among microbial eukaryotes in estuarine ecosystems. Aquat. Microb. Ecol. 54, 83–100. doi: 10.3354/ame01252
Virnstein, R. W. (1990). The large spatial and temporal biological variability of the Indian River Lagoon. Biol. Sci. 53, 249–256. doi: 10.1007/s002679910016
Keywords: brown tide, immunofluorescence flow cytometry, harmful algal blooms, qPCR, time-series, ecological niche
Citation: Lopez CB, Tilney CL, Muhlbach E, Bouchard JN, Villac MC, Henschen KL, Markley LR, Abbe SK, Shankar S, Shea CP, Flewelling L, Garrett M, Badylak S, Phlips EJ, Hall LM, Lasi MA, Parks AA, Paperno R, Adams DH, Edwards DD, Schneider JE, Wald KB, Biddle AR, Landers SL and Hubbard KA (2021) High-Resolution Spatiotemporal Dynamics of Harmful Algae in the Indian River Lagoon (Florida)—A Case Study of Aureoumbra lagunensis, Pyrodinium bahamense, and Pseudo-nitzschia. Front. Mar. Sci. 8:769877. doi: 10.3389/fmars.2021.769877
Received: 02 September 2021; Accepted: 29 October 2021;
Published: 24 November 2021.
Edited by:
Charles Alan Jacoby, St. Johns River Water Management District, United StatesReviewed by:
Yoonja Kang, Chonnam National University, South KoreaMary Lofton, Virginia Tech, United States
Copyright © 2021 Lopez, Tilney, Muhlbach, Bouchard, Villac, Henschen, Markley, Abbe, Shankar, Shea, Flewelling, Garrett, Badylak, Phlips, Hall, Lasi, Parks, Paperno, Adams, Edwards, Schneider, Wald, Biddle, Landers and Hubbard. This is an open-access article distributed under the terms of the Creative Commons Attribution License (CC BY). The use, distribution or reproduction in other forums is permitted, provided the original author(s) and the copyright owner(s) are credited and that the original publication in this journal is cited, in accordance with accepted academic practice. No use, distribution or reproduction is permitted which does not comply with these terms.
*Correspondence: Cary B. Lopez, Y2FyeS5sb3BlekBteWZ3Yy5jb20=
†Present address: Josée N. Bouchard, Centre de Recherche sur les Biotechnologies Marines, Rimouski, QC, Canada