- 1Division of Marine Science and Conservation, Duke University Marine Laboratory, Beaufort, NC, United States
- 2Geospatial Ecology of Marine Megafauna Lab, Marine Mammal Institute, Oregon State University, Newport, OR, United States
- 3Department of Statistical Science, Duke University, Durham, NC, United States
- 4Department of Ecology and Evolutionary Biology, University of California (UC), Santa Cruz, CA, United States
- 5Department of Ocean Sciences, University of California (CA), Santa Cruz, CA, United States
- 6Zoophysiology, Department of Biology, Aarhus University, Aarhus, Denmark
- 7The University of Western Australia (UWA) Oceans Institute and School of Agriculture and Environment, The University of Western Australia, Albany, WA, Australia
- 8Biology Department, Woods Hole Oceanographic Institution, Woods Hole, MA, United States
- 9Department of Biology, Hopkins Marine Station of Stanford University, Pacific Grove, CA, United States
Most baleen whales are capital breeders that use stored energy acquired on foraging grounds to finance the costs of migration and reproduction on breeding grounds. Body condition reflects past foraging success and can act as a proxy for individual fitness. Hence, monitoring the seasonal gain in body condition of baleen whales while on the foraging grounds can inform how marine mammals support the costs of migration, growth, and reproduction, as well as the nutritional health of the overall population. Here, we use photogrammetry from drone-based imagery to examine how the body condition of humpback whales (Megaptera novaeangliae) changed over the foraging season (November to June) along the Western Antarctic Peninsula (WAP) from 2017 to 2019. This population (IWC stock G) is recovering from past whaling and is growing rapidly, providing an opportunity to study how whales store energy in a prey-rich environment. We used a body area index (BAI) to estimate changes in body condition and applied a Bayesian approach to incorporate measurement uncertainty associated with different drone types used for data collection. We used biopsy samples to determine sex and pregnancy status, and a length-based maturity classification to assign reproductive classes (n = 228; calves = 31, juveniles = 82, lactating females = 31, mature males = 12, mature unknown sex = 56, non-pregnant females = 12, pregnant females = 3, pregnant & lactating females = 1). Average BAI increased linearly over the feeding season for each reproductive class. Lactating females had lower BAI compared to other mature whales late in the season, reflecting the high energetic costs of nursing a calf. Mature males and non-pregnant females had the highest BAI values. Calves and juvenile whales exhibited an increase in BAI but not structural size (body length) over the feeding season. The body length of lactating mothers was positively correlated with the body length of their calves, but no relationship was observed between the BAI of mothers and their calves. Our study establishes a baseline for seasonal changes in the body condition for this humpback whale population, which can help monitor future impacts of disturbance and climate change.
1 Introduction
A species’ life history describes how an organism maximizes its fitness by appropriate allocation of resources toward growth, health, and reproduction (Partridge and Harvey, 1988; Stephens et al., 2009). The eastern South Pacific humpback whale (Megaptera novaeangliae) population, identified as stock G by the International Whaling Commission (IWC), travels over 16,000 km round trip from the Western Antarctic Peninsula (WAP) to the coastal waters off Ecuador, Colombia, and Costa Rica (Acevedo et al., 2013; Avila et al., 2020; Modest et al., 2021). This extreme migratory behavior allows them to exploit the seasonal abundance of Antarctic krill (Euphausia superba, hereafter referred to as “krill”) and store energy reserves to finance the costs of migration and reproduction on the breeding grounds (Lockyer, 1981; Savoca et al., 2021). This life history strategy places a particularly large burden on reproductive females, as they must supply enough energy to support gestation and lactation in addition to their own health (Young, 1976; Partridge and Harvey, 1988; Jönsson, 1997) and larger females are able to better provision their offspring (Christiansen et al., 2018). Hence, monitoring the seasonal gain in energy reserves of these whales on the foraging grounds can reveal how they support the costs of migration, growth, health, and reproduction, and how these costs and gains vary between different reproductive classes. Here we illustrate such monitoring by quantifying changes in humpback whale body condition throughout the foraging season.
Recent evidence suggests that stock G humpback whales are recovering from their near extinction from 20th century commercial whaling, with an estimated annual population growth rate of 5.1% between 2000 (~7,000 individuals) to 2020 (~19,107 individuals) (Johnston et al., 2011; Rocha et al., 2014; Pallin et al., 2018a; Johannessen et al., 2022). Rapid population growth is typically observed in vertebrate populations recovering from catastrophic perturbation when there is a lack of competition and an adequate food supply (Choquenot, 1991; Foley and Faust, 2010; Leopold, 2018). The recovery of this humpback whale population has likely been facilitated by the high abundance of krill along the WAP (Nowacek et al., 2011; Johnston et al., 2012) and resource partitioning with sympatric baleen whales species (Friedlaender et al., 2009; Herr et al., 2016; Friedlaender et al., 2021). Despite this recovery, there are long-term concerns for this population, as the WAP region has experienced significant warming since the 1950s that has coincided with declines in krill density and recruitment, and a contraction of krill distribution southward and closer toward Antarctic continental shelves (Atkinson et al., 2004; Atkinson et al., 2019), which may be negatively impacting other humpback whale populations (Marcondes et al., 2017). In addition to environmental stressors, recent anthropogenic stressors are becoming more present. The WAP region has experienced dramatic increases in tourism since the 1990s (Cavanagh et al., 2021; Johannessen et al., 2022) and recent evidence suggests vessel noise, including whale watching vessels, can cause disturbance to cetaceans (Sprogis et al., 2020; Arranz et al., 2021). Furthermore, the WAP region has experienced increased activities by the krill fishery, which overlap with key foraging areas for humpback whales (Nicol and Foster, 2016; Weinstein et al., 2017; Reisinger et al., 2022).
Throughout the season, humpback whales adjust their foraging behavior and spatial distribution along the WAP to reflect the seasonal behavior of krill (Curtice et al., 2015). Humpback whale distribution is linked closely to areas of high krill abundance in which whales do not forage below a minimum threshold of prey density (Friedlaender et al., 2011; Tyson et al., 2016). In the early austral summer, humpback whales distribute themselves broadly across the continental shelf, becoming more concentrated in bays and fjords during autumn (Curtice et al., 2015; Weinstein and Friedlaender, 2017), where they may exploit super-aggregations of krill (Nowacek et al., 2011; Johnston et al., 2012; Cade et al., 2022). Humpback whales adjust their foraging behavior based on depth, density, and size of their prey, and follow the diel vertical migration of krill – foraging mostly at night and resting during the day, especially late in the season (Friedlaender et al., 2016; Cade et al., 2022; Nichols et al., 2022). Humpback whale foraging behavior also reflects seasonal differences in krill behavior; in the summer, humpback whales forage predominately on shallow prey in all light conditions and at high feeding rates, whereas in autumn, feeding becomes progressively deeper, nocturnal, and at lower rates (Nichols et al., 2022). The plasticity and efficiency of foraging behavior in humpback whales along the WAP likely enables the successful accumulation of energy stores over the foraging season on an abundant but ephemeral prey species.
An animal’s body condition represents its relative energy reserves and can reflect foraging success and provide information on habitat quality and reproductive status (Green, 2001; Stevenson and Woods, 2006; Peig and Green, 2010). It is challenging to obtain morphological measures of body condition of baleen whales, as these animals often occur in remote locations, spend little time at the surface, and their large size precludes capture. Photogrammetry from unoccupied aircraft systems (UAS, UAVs, or drones) is an effective non-invasive tool for obtaining measurements of cetaceans in remote environments (Durban et al., 2015). UAS have been used to measure body condition in blue (Balaenoptera musculus), gray (Eschrichtius robustus), and northern (Eubalaena glacialis) and southern right whales (Eubalaena australis) (Christiansen et al., 2016; Durban et al., 2016; Christiansen et al., 2018; Lemos et al., 2020; Christiansen et al., 2021; Stewart et al., 2022; Torres et al., 2022). For humpback whales, studies have described trends in body condition along the migration routes (Christiansen et al., 2020b; Russell et al., 2022), the North Atlantic feeding grounds (Aoki et al., 2021), and the Western Australian breeding grounds (Christiansen et al., 2016).
In this study, we used UAS imagery to measure the body condition of humpback whales throughout the foraging season along the WAP. Our objectives of this study were to examine: 1) how body condition changes over the course of the foraging season for this population of humpback whales; 2) differences in how body condition changes as a function of reproductive class; and 3) the relationship of body size (length and condition) between mother-calf pairs. We incorporate measurement uncertainty associated with multiple UAS types used in these efforts to accurately compare length and body condition estimates (Bierlich et al., 2021b; Bierlich et al., 2021a). This work establishes a foundational understanding of how a rapidly growing baleen whale population increase energy stores across the foraging season. Furthermore, this study sets a baseline for monitoring future impacts of regional warming, as well as increased anthropogenic pressures from the krill fishery and tourism activities.
2 Materials and methods
2.1 Field operations
2.1.1 UAS data collection
High resolution images of humpback whales were collected along the WAP between November and June during 2017–2019 as part of the Palmer Long Term Ecological Research (LTER) project (Figure 1). Field work was conducted from Palmer Station, the ARSV Laurence M. Gould, and tour ships as platforms of opportunity (Table S1). UAS were launched and recovered from a Zodiac Mark V rigid-hulled inflatable boat (Zodiac) following methods described in Gough et al. (2019). Whales were either first spotted from land or the vessel and then approached for sampling in the Zodiac, or were spotted and then approached directly from the Zodiac. When working from tour ships, field operations were conducted separate from the passengers.
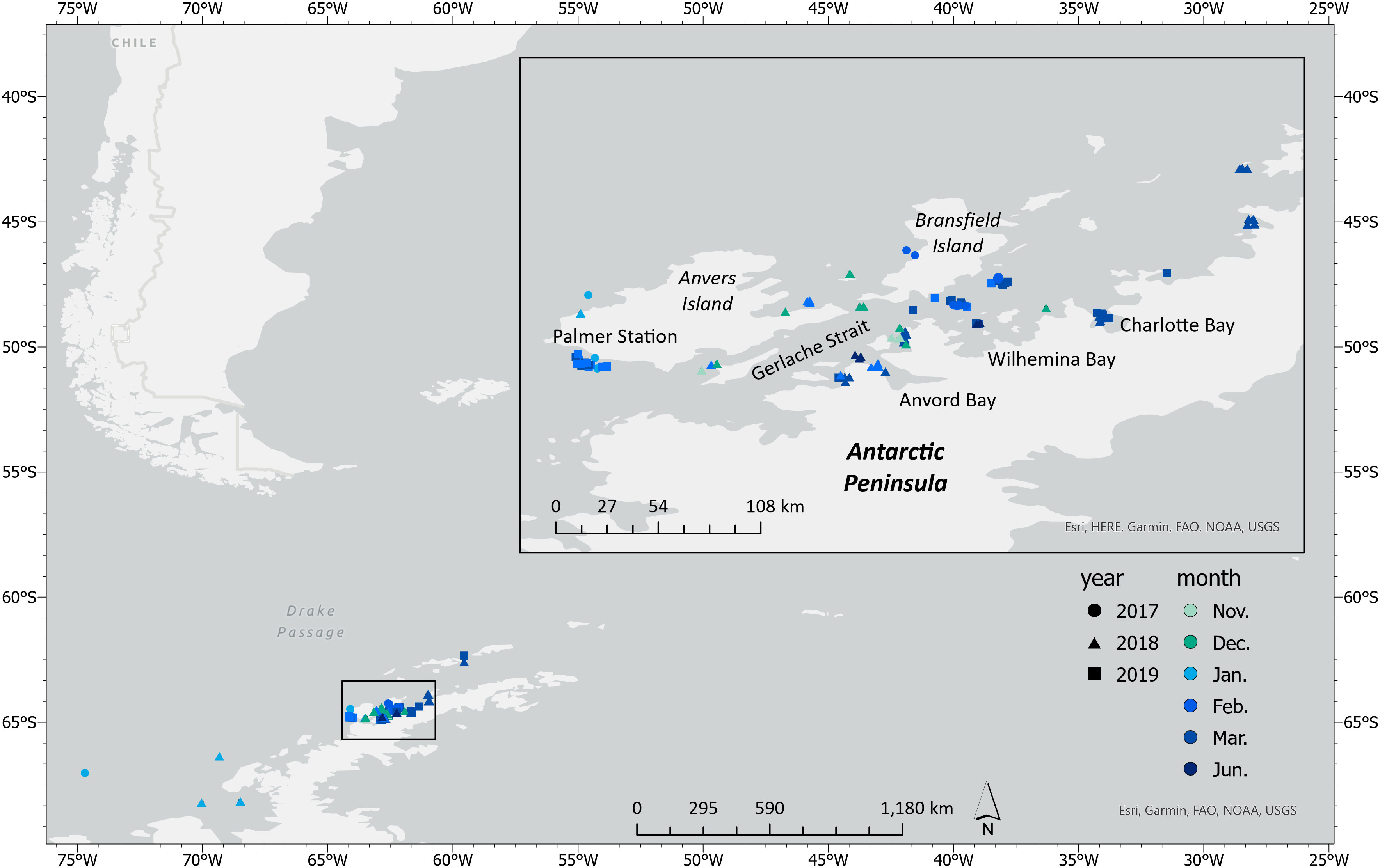
Figure 1 Locations of UAS flights along the Western Antarctic Peninsula (WAP) that collected aerial imagery of humpback whales (n = 228) throughout the austral foraging season (Spring–Autumn).
Images were acquired using a DJI Inspire 1 Pro quadcopter (I1P), a FreeFly Alta 6 hexacopter (Alta), and a Mikrokopter-based LemHex-44 hexacopter (LemHex). The I1P was fitted with a DJI Zenmuse X5 micro four-thirds camera (17.3 x 23.5 mm sensor), 4608 x 3456 pixel resolution, and an Olympus M. Zuiko a 25 mm f1.8 focal length. The LemHex-44 and Alta 6 both incorporated a Sony Alpha a5100 camera with an APS-C (23.5 x 15.6 mm) sensor, 6000 x 4000 pixel resolution, and either a 35 or 50 mm f1.8 Sony SEL focal length lens. Each UAS contained an onboard barometer and was also fitted with a Lightware SF11/C laser altimeter. The two hexacopters had the laser altimeter and camera co-located on a 2-axis gimbal with pitch angle controlled via remote control to ensure image collection at nadir. The I1P also had the camera on a 2-axis gimbal but instead had the laser altimeter fixed on the aircraft frame, and the pitch and roll of the aircraft were accounted for in order to calculate vertical altitude (Dawson et al., 2017; Christiansen et al., 2018). The LemHex and Alta collected still images of whales in bursts, while the I1P collected videos and then later extracted still frames using VLC Media Player Software (Versions 3.08 VideoLAN).
2.1.2 Biopsy sampling, demographic analysis, and photo-identification
Whenever possible, skin and blubber biopsy samples were collected in tandem along with UAS imagery of individual humpback whales using standard techniques (Lambertsen, 1987; Palsbøll et al., 1991). We used a crossbow with modified bolts and 40 mm stainless steel cutting tips (CetaDart) to obtain samples from a distance of 10–30 m when the whale surfaced to breathe, targeting the area of the body below the dorsal fin. Samples were stored frozen whole at -20° C until used for analysis. Supplementary Data (i.e., location, group size, group composition) were also recorded with every biopsy event.
A standard DNA profile, including sex-specific markers and microsatellite genotypes, was used to identify individual whales following the methods described in Pallin et al. (2018b). To assign pregnancy, we extracted the sex steroid hormone progesterone from the blubber portion of the biopsy sample and compared it to a control model built from female humpbacks of known pregnancy status (Pallin et al., 2018b). We identified individual whales based on unique features (coloration patterns, markings, and scars) from boat- and UAS-based images (Kaufman et al., 1987). UAS images of each individual were used to segment the body into 24 different regions and identify unique features to confirm individuals and review potential matches (see Figure S1).
2.2 Data processing
2.2.1 Image and data filtering
UAS images for each individual were ranked for quality in measurability following Christiansen et al. (2018), where a score of 1 (good quality), 2 (medium quality), or 3 (poor quality) was applied to seven attributes: camera focus, straightness of body, body roll, body arch, body pitch, total length measurability and body width measurability. Images with a score of 3 in any attribute were removed from analysis, as well as any images that received a score of 2 in both roll and arch, roll and pitch, or arch and pitch (Christiansen et al., 2018). Measurements from up to five images were used per individual. For images with an altitude difference >10% between the barometer and laser altimeter, barometer values were changed to NA (Bierlich et al., 2021b; Bierlich et al., 2021a).
2.2.2 Photogrammetry and uncertainty quantification
We used MorphoMetriX open-source photogrammetry software to measure (in pixels) the total length (TL, tip of rostrum to fluke notch) and perpendicular widths in 5% increments of the total length measurement (Torres and Bierlich, 2020) (Figure 2). MorphoMetriX outputs were collated using CollatriX (Bird and Bierlich, 2020).
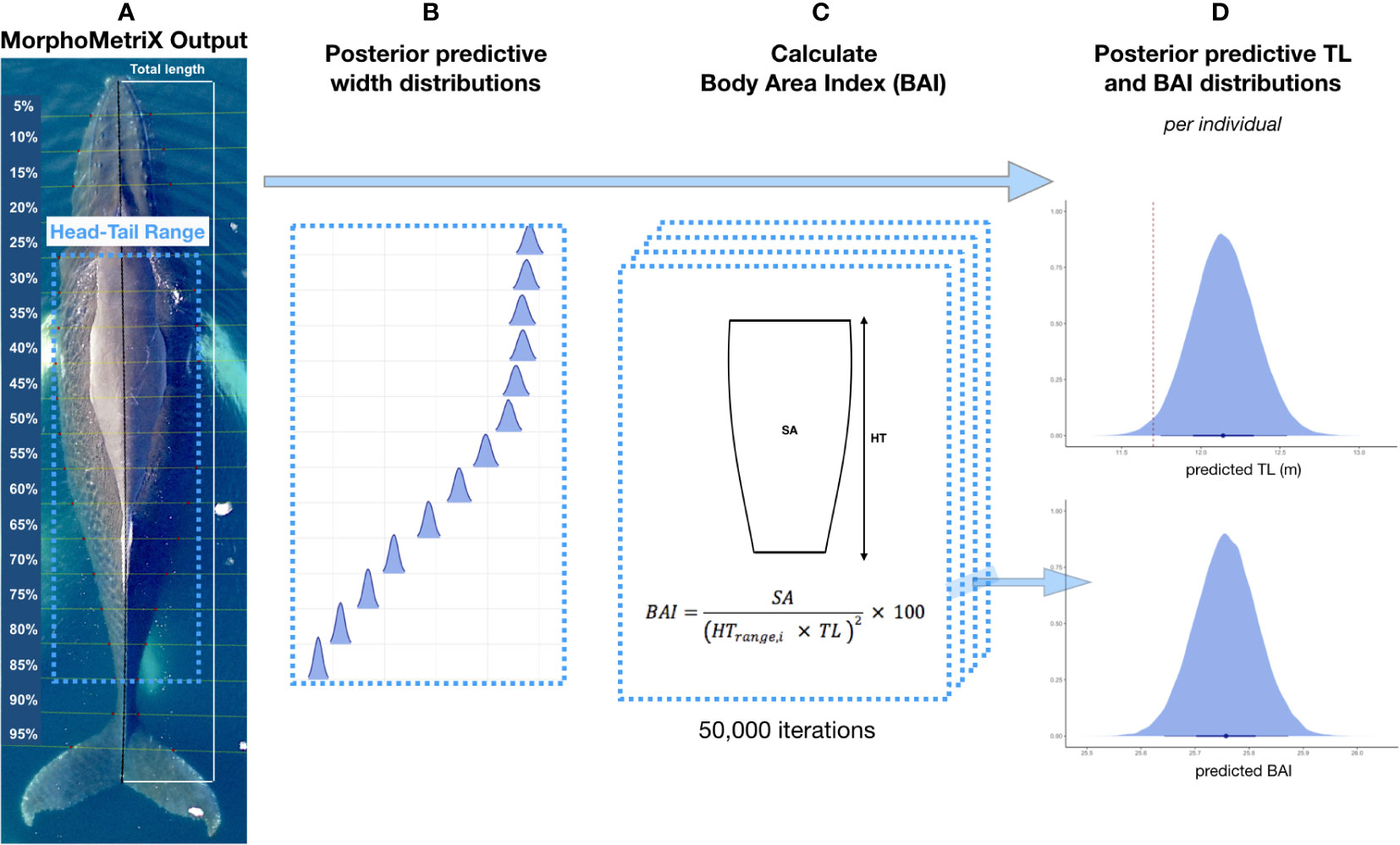
Figure 2 Overview of Bayesian framework for incorporating uncertainty associated with total length (TL) and Body Area Index (BAI). (A) An example of a MorphoMetriX output (Torres and Bierlich, 2020) from a UAS image of a humpback whale. TL is measured as rostrum to fluke notch with perpendicular widths in 5% increments of TL. The Head-Tail Range represents the region of the body used to calculate BAI, and excludes the head, tail, and fins (juvenile and adults = 25-85%, calves = 20-85%). (B) Posterior predictive distributions for each 5% width included in the Head-Tail Range are generated and used to calculate BAI. (C) BAI is calculated for each iteration in the Markov Chain Monte Carlo output of each posterior predictive distribution for TL and widths using CollatriX (Bird and Bierlich, 2020). (D) An example of a posterior predictive distribution for TL and BAI for a single individual. On the x-axis, the black dot represents the mean value and uncertainty is represented by the 95% highest posterior density (HPD) intervals (longer black bars) and the 65% HPD intervals (thicker shorter black bars). The red dashed line represents the maturity cutoff length (female = 11.73, male = 11.20, unknown = 11.47 m). Figure adapted from Bierlich et al., 2021a and Torres et al., 2022.
Photogrammetric uncertainty varies with different cameras, focal length lenses, altimeters, and the altitude of the UAS (Bierlich et al., 2021b). To quantify and incorporate measurement uncertainty associated with each UAS, we used the Bayesian statistical model developed by Bierlich et al. (2021b) that uses measurements of known-sized objects at various altitudes as training data to predict the length and widths of unknown-sized whales. For the Alta and LemHex, we used training data of known-sized calibration objects (length = 1.48 m, 1.33 m, or 1.40 m) floating at the surface (described in Bierlich et al., 2021b; Bierlich et al., 2021a). For the I1P, we used measurements of a known sized mat (length = 1.45 m) flown on land (described in Christiansen et al., 2018). We applied the Bayesian statistical model to each individual whale using the corresponding UAS training data associated with that individual.
Rather than a single point estimate, the Bayesian statistical model outputs a posterior distribution for each length and width measurement, which is combined to estimate body condition, and properly account for the uncertainty inherent in the observation process (Bierlich et al., 2021a) (Figure 2). We measured body condition as Body Area Index (BAI), which is a unitless and scale-invariant metric standardized by total length (Burnett et al., 2018). BAI is useful for comparing body condition across individuals, as it has been shown to have low measurement uncertainty with high precision (Bierlich et al., 2021a; Torres et al., 2022) (Figure 2). We calculated BAI for each individual using a Head-Tail Range of 25-85% for juvenile and mature humpback whales and 20-85% for calves (Figure 2) (Bierlich et al., 2021a).
We summarized uncertainty by calculating the variance, 95% highest posterior density (HPD) intervals, and the coefficient of variation (CV%) of the posterior predictive distributions for total length and BAI for each individual. We used the CV% to compare measurement uncertainty associated with each UAS, which compares the relative width of each individual’s posterior distribution, with a lower CV% translating to a more precise estimated measurement (Bierlich et al., 2021a; Torres et al., 2022).
2.2.3 Assigning maturity and reproductive class
To examine how body condition changes throughout the foraging season for each reproductive class, each individual was assigned to one of ten reproductive classes based on biopsy results, field observations, and length estimates: calf, juvenile female, juvenile male, juvenile unknown sex, mature male, mature unknown sex, lactating female, pregnant female, pregnant & lactating female, or non-pregnant mature female. Calves and lactating females were classified based on their relative size to each other, as well as their consistent association (Christiansen et al., 2016). Following Bierlich et al. (2021b), females and males were assigned as sexually mature if at least 50% of the posterior distribution for TL was greater than the mean length at maturity for each sex; 11.73 m for females (Chittleborough, 1955b) and 11.20 m for males (Chittleborough, 1955a) (Figure 2). If sex was unknown, we used the average length between the mean length at maturity for males and females (11.47 m) as the maturity cutoff length.
2.3 Statistical analyses
2.3.1 Seasonal change in total length & body condition
The humpback whale foraging season in the Southern Hemisphere spans the austral spring and autumn, with peak densities typically between December and February (Lockyer, 1981). We calculated the seasonal duration as the number of days since the start of the foraging season, which we set as November 1 since the beginning of November was the earliest we observed whales feeding (Table S1). The date and time of each flight was used to determine the time within the season that each individual was sampled. Since individuals vary on their timing of arrival to and departure from the feeding grounds (Dawbin, 1966; Modest et al., 2021), which can influence an individual’s overall body condition (Irvine et al., 2017b), we do not assume that the number of days since November 1 is equal to the number of days spent foraging for each individual. Though, once individuals arrive to the WAP, they often feed for several months between the austral spring and fall (Bierlich et al., 2018; Pallin et al., 2018a; Friedlaender et al., 2021). We then evaluated how BAI and TL changed over the course of the foraging season for each reproductive class.
We first investigated whether BAI increased linearly or nonlinearly with respect to time over the course of the season for each reproductive class by comparing the fit between a linear regression model and a quadratic polynomial regression model. Similar to Torres et al. (2022), we used Monte Carlo methods to propagate uncertainty associated with BAI into the regression analysis by averaging the results of 1,000,000 replications each of a linear or quadratic model, where reproductive class and date within season (i.e., 10, 11, 12 days since start of season, etc.) are the predictor variables and BAI is the response variable. For each model replicate, we sampled each whale’s BAI measurement from a normal distribution parameterized with the posterior mean and variance for that whale’s posterior distribution for BAI. We then used a nested ANOVA test to evaluate whether there was a significant difference between the linear and quadratic models (i.e., a general linear test; Kutner et al., 2004, Section 2.8), where the average of the p-values from the replicate ANOVA tests serves as the overall Monte Carlo p-value to evaluate the best fitting model. The Monte Carlo average coefficients and HPDs were calculated for the best model (i.e., linear or quadratic). We then illustrate how each reproductive class increases BAI over the course of the season by evaluating the expected BAI for two time points covering early and late season.
To investigate potential differences in migratory timing of different sized individuals, as well as if energy is invested toward skeletal growth, we determined whether the TL of individuals sampled within a reproductive class varied across the season (Craig et al., 2003; Irvine et al., 2017b). We followed similar Monte Carlo methods as described above, where reproductive class and seasonal duration are the predictor variables and TL is the response variable.
2.3.2 Body size between mother-calf pairs
To investigate if a positive relationship exists between the body size of mother-calf pairs at the feeding grounds, we analyzed how the TL and BAI of lactating females influenced the TL and BAI of their dependent calves. We used a Deming regression to account for measurement uncertainty in both the predictor variable (mother’s TL or BAI) and response variable (calf’s TL or BAI) (Deming, 1943). We used the “deming” package in R (Therneau, 2018) and accounted for measurement uncertainty using the standard error of each individual’s posterior predictive distribution for TL and BAI.
3 Results
3.1 Data collection
We collected UAS Images from 461 humpback whales between November and June of 2017-2019. After filtering for image quality, 228 individuals remained in the analysis (Table 1). Each individual had measurements from up to five images, totaling 602 images (9 individuals had 5 images, 13 had 4, 121 had 3, 53 had 2, and 36 had 1). The sample size of each reproductive class varied by month, with no data collected during the months of April and May (Table 1). No individual was sampled more than once, so our dataset represents a cross-sectional sample of the population to describe broad trends for each reproductive class. We observed no behavioral response from the whales toward the UAS during flight operations.
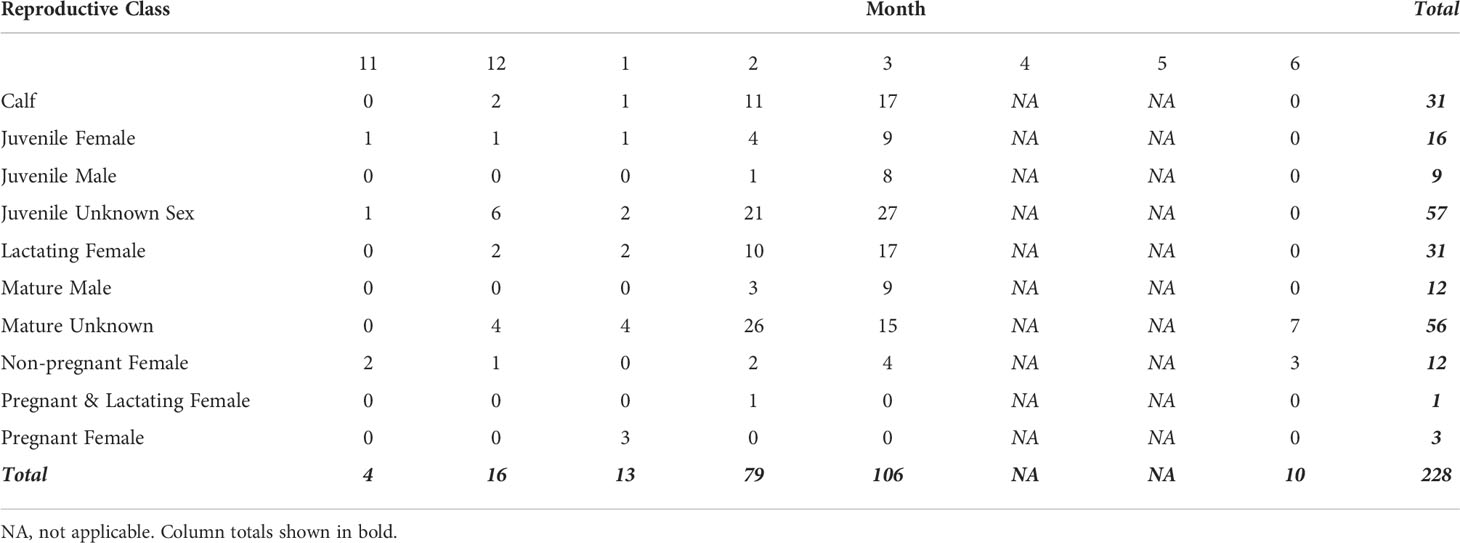
Table 1 Number of samples for each demographic unit by month used in analysis (after quality filtering). No data were collected in months 4 (April) and 5 (May).
3.2 Morphometric measurements and photogrammetric uncertainty
Each UAS displayed similarly low levels of measurement uncertainty for BAI, described here as the CV% of the posterior predictive distribution for each individual, with a mean value < 0.64% across all UAS (Figure S2, Table S2). Measurement uncertainty associated with TL varied slightly more amongst each UAS, with Alta w/50 mm focal length (f.l.) having the least uncertainty (CV%: n = 51, mean = 3.52%, standard deviation (sd) = 2.73%), followed by I1Pro (CV%: n = 52, mean = 3.95%, sd = 2.56%), LemHex w/50 mm f.l. (CV%: n = 34, mean = 4.23%, sd = 3.06%), LemHex w/35 f.l. (CV%: n = 49, mean = 5.03, sd = 3.93), and Alta w/35 mm f.l. (CV%: n = 42, mean = 6.31%, sd = 5.08%) (Figure S2, Table S2).
The TL and BAI for each individual is described as the mean of their respective posterior predictive distributions (Figure 2, Table 2). Across all reproductive classes, BAI ranged from 19.74 to 31.39 (Table 2). The single lactating & pregnant female had a BAI of 25.76, similar to the other pregnant females (mean = 26. 03, sd = 1.92) (Table 2).
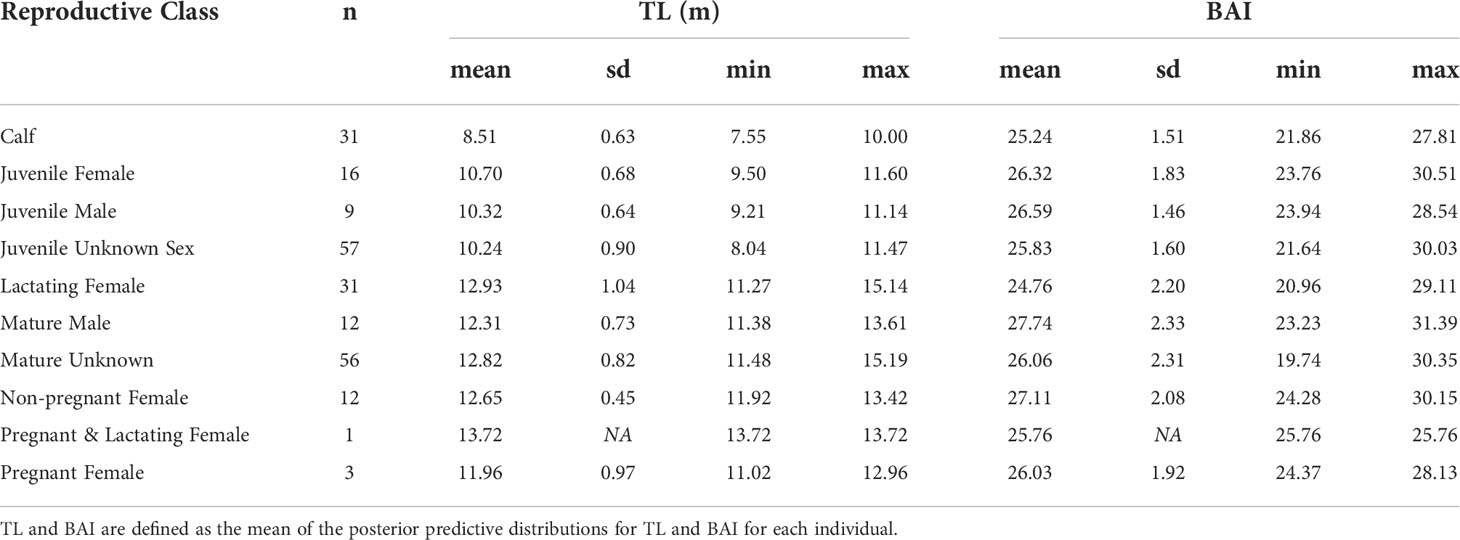
Table 2 Summary statistics of the total length (TL) and Body Area Index (BAI) for each reproductive class.
3.3 Seasonal increase in body condition
Juvenile males, mature males, pregnant females, and lactating & pregnant females were excluded from analyses looking at seasonal changes in TL and BAI, due to uneven sampling across months (Table 1). TL did not significantly change across the season for any reproductive class (Table S3, Figure S3).
BAI increased over the course of the season for each reproductive class (Figure 3). The Monte Carlo quadratic polynomial with respect to days-in-season did not significantly reduce the unexplained variation in BAI measurements relative to the Monte Carlo linear model (nested ANOVA general linear test, p = 0.13). Lacking statistically significant differences between the models, we used the Monte Carlo linear model to describe BAI increase over the foraging season as it was more parsimonious (Figure 3). To help describe how each reproductive class increased BAI over the course of the season, we used the Monte Carlo linear model to compare the expected BAI for the average whale in each class between early and late season. Dec. 12 (Day 54 from November 1) and Mar 26 (Day 146 from November 1) were the earliest and latest dates where each reproductive class was sampled, respectively, so we compared the expected BAI for each class at the beginning of December and April (Table 3, Figure 4). Calves and lactating females had the lowest expected BAI in the beginning of the season (Table 3, Figure 4). Despite this lower BAI at the start of the season, calves experienced the greatest increase in BAI from early to late season, 22.5 [95% HPD: 20.3, 24.7] to 26.8 [95% HPD: 25.5, 28.2] (Table 3, Figure 4). All reproductive classes reached similar levels of BAI by the end of the season, but lactating females had the lowest BAI in the late season, 25.7 [95% HPD: 24.4, 27.0] (Table 3, Figure 4). Non-pregnant females had the highest early season BAI (26.5 [HPD: 25.0, 28.1] and experienced the least amount of increase in BAI by late season (27.3 [HPD: 26.2, 28.4]) (Table 3, Figure 4).
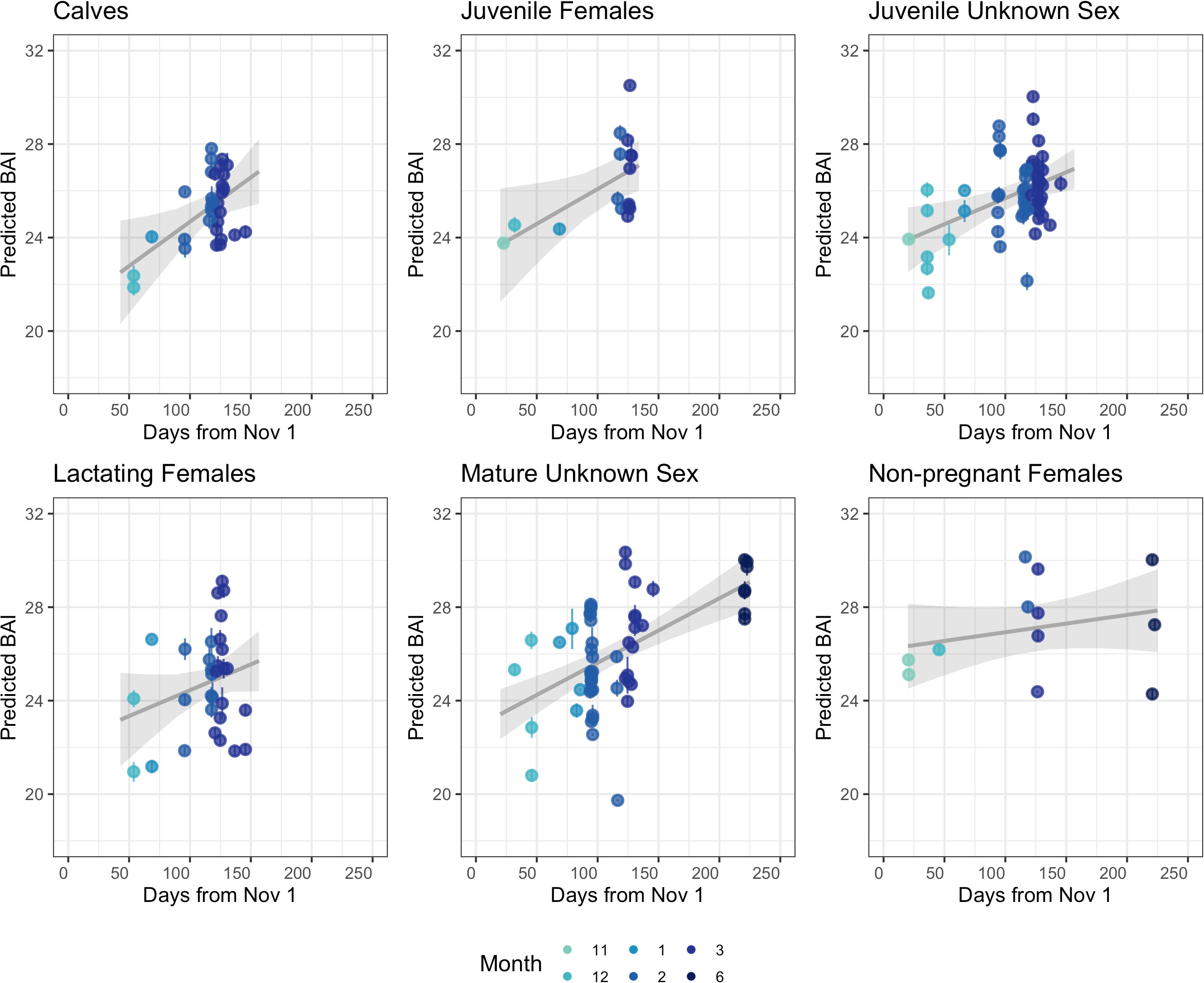
Figure 3 BAI change over the foraging season for each reproductive class of humpback whales sampled along the WAP. Each point represents the mean of the posterior predictive distribution for BAI for an individual whale and the error bars represent uncertainty as the 95% highest posterior density (HPD) intervals. The grey line is the estimated linear relationship, with the grey band indicating the 95% credible interval for the estimated relationship. Mature males, pregnant females, and the single pregnant & lactating female are not shown due to low samples sizes across each month (Table 1).
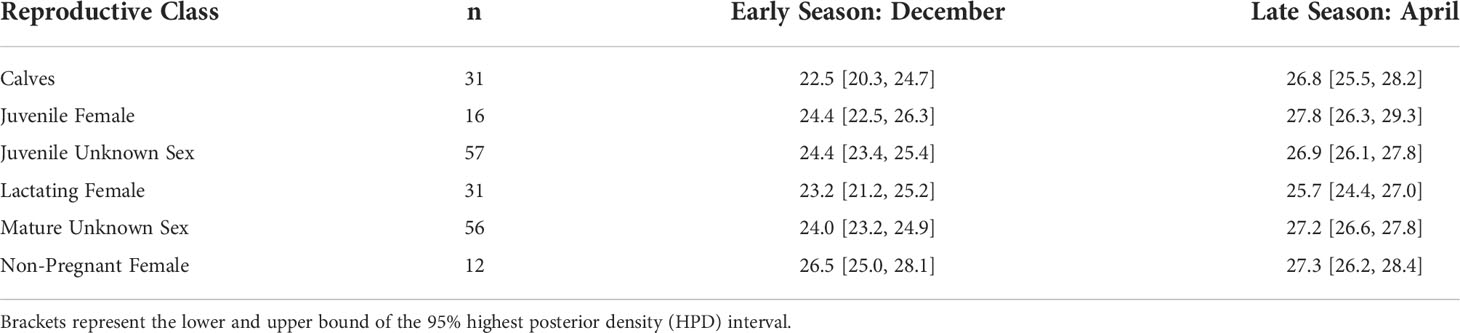
Table 3 Expected Body Area Index (BAI) for each reproductive class based on the best fitting Monte Carlo linear model.
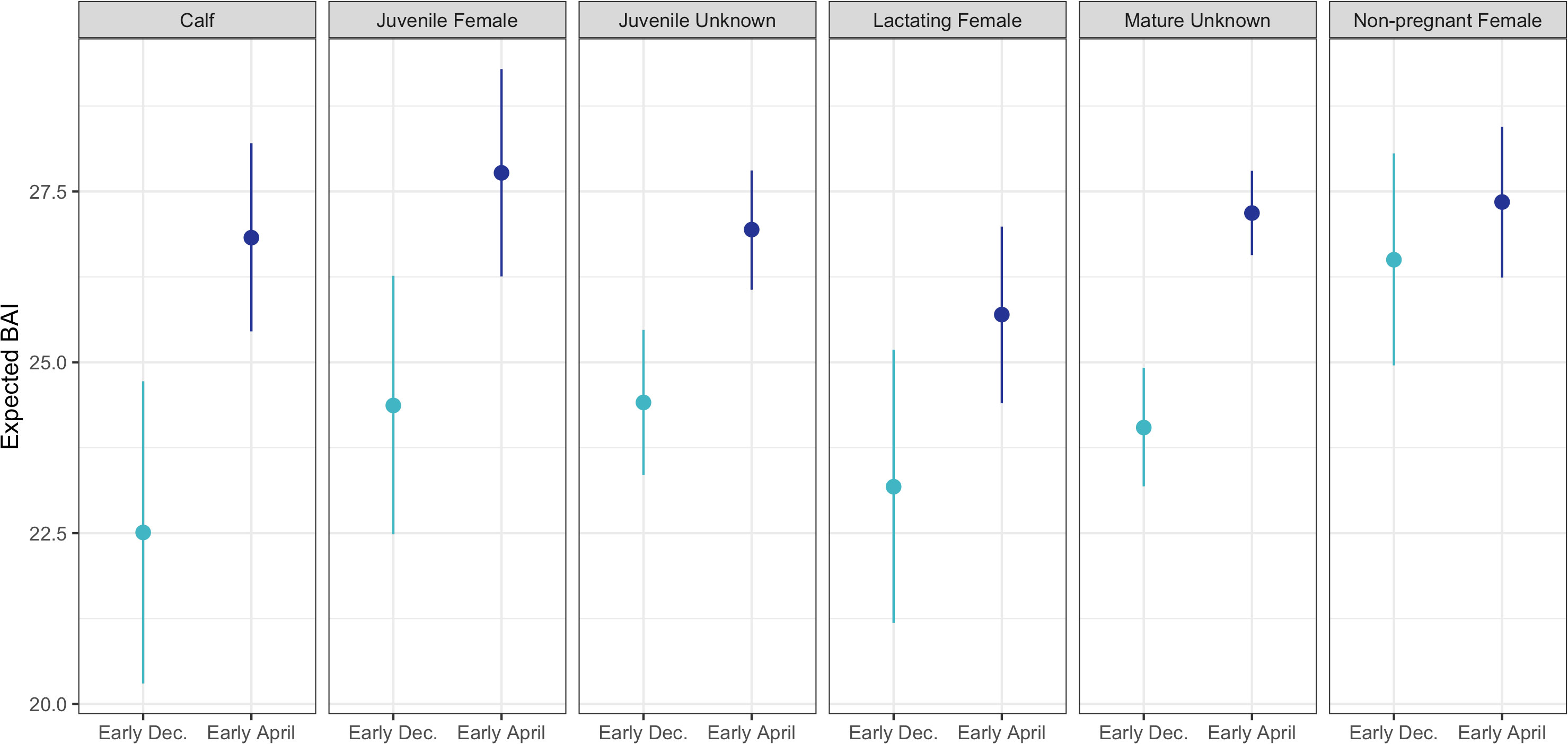
Figure 4 The expected Body Area Index (BAI) for the average whale in each reproductive class for early (beginning of December, lighter blue) and late (beginning of April, darker blue) season. Each point represents the mean expected BAI for that reproductive class, and the bars represent the 95% highest posterior density (HPD) intervals.
3.4 Mother-calf pairs
We measured TL and BAI in 28 total mother-calf pairs between December and March (Table S4). The TL of the mothers in the mother-calf pair had a significant positive relationship with the TL of their calves (Deming regression: slope = 0.49 [95% HPD: 0.30, 0.67], intercept = 2.11 [95% HPD: -0.28, 4.51]) (Figure 5). This suggests that longer mothers produce longer calves (Figure 5). We found no relationship between the mother’s BAI and their calf’s BAI (Deming regression: slope = -1.43 [95% HPD: -11.72, 8.85], intercept = 60.82 [95% HPD: -191.86, 131.50]), or the mother’s BAI and their calf’s TL (Deming regression: slope = -0.01 [95% HPD: -0.27, 0.25], intercept = 8.55 [95% HPD: 2.00, 15.11]).
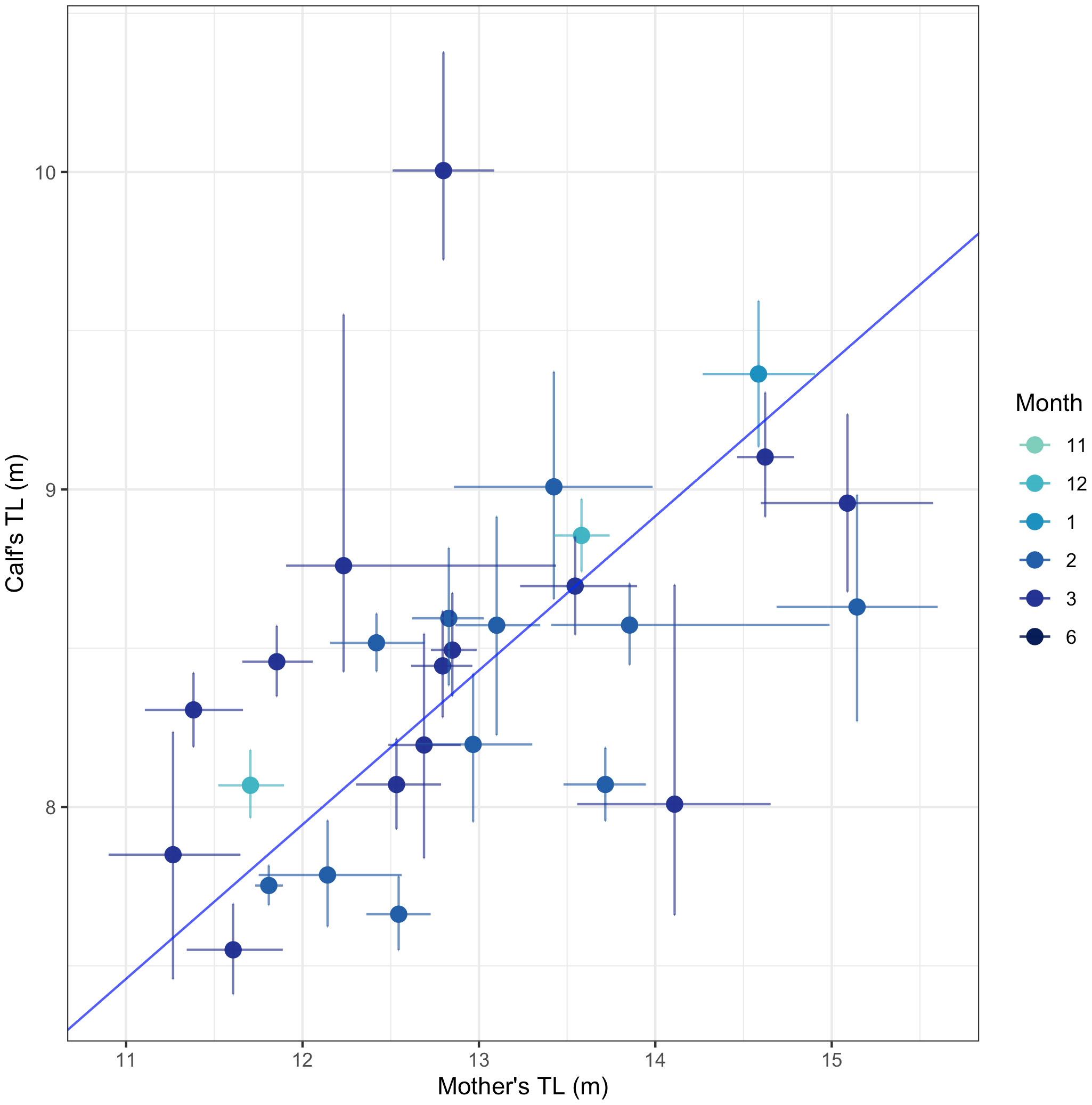
Figure 5 The total length (TL) between mother-calf pairs. Each point represents the mean of the posterior predictive distribution for TL for each mother and calf in a mother-calf pair. The solid lines represent the uncertainty as the 95% highest posterior density (HPD) intervals. The blue solid line indicates the best fit from a Deming regression (slope = 0.49 [95% HPD: 0.30, 0.67], intercept = 2.11 [95% HPD: -0.28, 4.51]).
4 Discussion
Our results provide new insight into how humpback whales increase their energy reserves while foraging along the WAP. By incorporating photogrammetric uncertainty associated with different UAS used in data collection, we ensure the results are robust and comparable across platforms. In general, we found linear increases in body condition over the course of the foraging season for each reproductive class. We also found evidence that longer mothers tend to have longer calves.
4.1 Intra-seasonal increase in body condition
In the early feeding season, soon after their arrival to the WAP, humpback whales exhibit extremely high feeding rates throughout all hours of the day (Nichols et al., 2022). As the season progresses into autumn, feeding rates significantly drop and become more nocturnal (Nichols et al., 2022). Despite these reduced feeding rates into autumn, our results indicate that humpback whales continue to accumulate substantial mass into the late foraging season. Similar linear increases in body condition between summer and autumn were also observed across reproductive classes in North Atlantic fin (Balaenoptera physalus), sei (Balaenoptera borealis), minke (Balaenoptera acutorostrata), and humpback whales (Lockyer, 1987; Vikingsson, 1995; Næss et al., 1998; Christiansen et al., 2013; Aoki et al., 2021). The rate of increase in body condition over the foraging season appears closely related to prey abundance, distribution, and quality (Lockyer, 1986; Lockyer, 1987; Aguilar and Borrell, 1990; Williams et al., 2013). Víkingsson (1997) noted that fin whales caught off of Iceland between 1967 – 1989 likely had lower feeding rates in late summer and autumn compared to early summer that coincided with unreduced rates of energy deposition. The observed continued increase in humpback whale body condition implies a change in prey density and/or quality into autumn to compensate for lower feeding rates. Krill density and biomass increase by over an order of magnitude along the inshore waters of the WAP from summer to winter, while the offshore areas experience significant declines (Reiss et al., 2017). Furthermore, in the Weddell Sea, krill undergo substantial increases in total lipid content over the foraging season, with dry mass increasing from 10.5% +/- 4.0% in spring to 39.2% +/- 5.6% by autumn (Hagen et al., 1996). Hence, humpback whales likely compensate for their lower feeding rates in the late season by feeding closer inshore on denser and higher quality krill, thereby increasing efficiency in energy deposition.
In contrast to the linear increases in body condition we observed, some studies on North Atlantic fin whales have documented non-linear increases in body condition. Body condition increases steadily in early season and then begins to asymptote or decrease by autumn prior to migration, demonstrating a density-dependent response to decreasing food availability (Aguilar and Borrell, 1990; Williams et al., 2013). Our study did not find statistically significant evidence for a non-linear increase in body condition. The increase in body condition likely coincided with denser and higher quality krill into late season, suggesting humpbacks are not yet constrained by prey availability. Future studies should test this constraint hypothesis by examining how foraging activity from tagged whales correlates with body condition and prey distribution across repeated samples of known individuals throughout the foraging season. Continual observation between and across seasons may also provide evidence to identify potential non-linear relationships in seasonal body condition changes.
In addition to food availability and quality, rapid mass gain may also be facilitated by a suite of endocrine factors regulating metabolic rate and satiation. For example, an increase in body mass is normally associated with increases in leptin, which acts on receptors in the hypothalamus to reduce appetite and increase metabolic rate, restoring normal energy balance (Sahu, 2004). However, during the pre-hibernating and pre-migrating period of rapid fattening in mammals, elevated leptin does not seem to inhibit feeding nor stimulate metabolism (Florant and Healy, 2012). This has been observed in several mammals including racoon dogs (Nyctereutes procyonoides) (Nieminen et al., 2001), little brown bats (Myotis lucifugus) (Townsend et al., 2008), and even bowhead whales (Balaena mysticetus) (Ball et al., 2017). These studies suggest that pre-hibernating and pre-migrating mammals are able to gain a surplus in their energy reserves via temporal leptin resistance that decreases hypothalamic sensitivity to the anorexigenic effects of leptin during fattening. Analyzing leptin from biopsy samples paired with UAS-based body condition measurements on repeated individuals over the season could help confirm if leptin resistance also occurs in humpback whales.
4.2 Variation in body condition increase amongst reproductive classes
BAI increase for humpback whales along the WAP varied with reproductive status. Lactating females had the poorest measures of condition across the season, reflecting the high energetic demands of lactation (Lockyer, 1981; Miller et al., 2011; Christiansen et al., 2018). Conversely, non-pregnant females had the highest BAI at the start of the season compared to all other classes, likely reflecting the energy saved and recovered from skipping breeding (Figure 4) (Lockyer, 1986; Lockyer, 1987; Aoki et al., 2021). This same trend was also observed in Pacific Coast Feeding Group gray whales and North Atlantic humpback, sei, and fin whales, where lactating females increased their body condition during the foraging season but still remained lower than mature males and non-pregnant females (Lockyer, 1987; Lemos et al., 2020; Aoki et al., 2021). On the breeding grounds, Christiansen et al. (2016) reported that lactating female humpback whales had the greatest rate of decline in body condition, which explains why lactating females on the WAP have such low BAI in early season, while non-pregnant females had the highest BAI (Figure 4).
Immature whales (calves and juveniles) experienced rapid increases in body condition over the course of the foraging season, reaching similar levels of BAI as mature whales by the beginning of April (Table 3, Figure 4). Immature whales likely invest the stored energy accumulated on the foraging grounds toward skeletal growth during the breeding season, whereas mature individuals invest in reproduction (Lockyer, 1981; Christiansen et al., 2013; Christiansen et al., 2016; Irvine et al., 2017b; Christiansen et al., 2020b; Russell et al., 2022). It is important to note that BAI is a relative measure of body condition (energy stores) and mature whales have larger absolute measures of body condition compared to immature whales, as their larger body sizes with smaller surface area to volume ratios enable greater capacity for absolute energy storage and a lower mass-specific metabolic rate.
While we found similar levels in expected BAI between immature and mature whales by April (Figure 4), it is also plausible that prolonged residency times along the WAP further increases BAI for mature whales. Indeed, large aggregations of humpbacks have been documented along the WAP in late autumn (Nowacek et al., 2011; Cade et al., 2022), and while our sample size is small, we observed only mature individuals in late autumn (n = 10 in June) that showed continued increases in BAI (Table 1, Figure 3). A study examining the number of barrels of oil from humpback whales taken from commercial whaling found that whales caught in late season had greater total body lipid stores compared to individuals caught earlier in the season, possibly reflecting differences in residency times between age-classes in the Antarctic foraging grounds (Irvine et al., 2017b). Furthermore, it is well documented that juvenile humpback whales are typically the first to arrive to the breeding grounds, followed by adults and pregnant females, indicating differences in phenology between reproductive classes (Dawbin, 1966; Franklin et al., 2011; Avila et al., 2020). Thus, it is likely that some individuals stay longer on the foraging grounds to further increase their body condition based on their reproductive status.
The calves sampled during this study were born in the austral winter prior to arriving to the WAP. As such, these samples represent the calves’ first foraging season along the WAP. Calves showed an increase in BAI over the course of the foraging season, but not in their TL. The opposite was observed on their lower latitude breeding grounds, where 32 humpback whale calves (TL range: 4.14-7.76 m, thus classified as n = 30 post-neonate, n = 2 neonate, see Irvine et al., 2017a) increased their TL, but not their body condition (Christiansen et al., 2016). Calves may first prioritize investing energy towards growth on the breeding grounds to reduce heat loss via reducing their surface area to volume ratio, to enhance breath holding ability (Ejrnæs and Sprogis, 2022), and to avoid predation by killer whales (Orcinus orca) and sharks (Pitman et al., 2015). Then, as they enter their high latitude foraging grounds, they begin to invest more energy toward fat and blubber storage. Nevertheless, calves likely still continue to grow in length on the foraging grounds to some extent, as newly weaned humpbacks are larger than the largest dependent calves just prior to departing the feeding grounds (Christiansen et al., 2016). The majority of calves in this present study were sampled between February and March (28 of 31 individuals) (Table 1), which may be too short of a timescale to detect an overall increase in TL, especially since baleen whales typically experience reduced rates in somatic growth after 6 months of age (Lockyer, 1981; Agbayani et al., 2020; Christiansen et al., 2022) and likely start prioritizing energy toward fat and blubber.
4.3 Body size of mother-calf pairs
We examined how the body size (BAI and TL) of lactating mothers’ influences the body size of their respective calves’. In this study, humpback whale calves ranged in length from 7.5 to 10 m (mean= 8.5 m), while on the breeding grounds, humpback whale calves ranged between 4 and 8 m in length, with a presumed birth length between 3.96 and 4.57 m (Clapham et al., 1999; Ejrnæs and Sprogis, 2022; Ransome et al., 2022). We found that calves’ TLs were positively related to their mothers’ TLs, meaning longer calves were associated with longer mothers (Figure 5). This same trend was observed in humpback whales in Eastern and Western Australia (Christiansen et al., 2016; Russel et al., 2022), as well as in Eastern North Pacific gray whales (Christiansen et al., 2021) and southern and North Atlantic right whales (Best and Rüther, 1992; Christiansen et al., 2018; Stewart et al., 2022). In general, longer body lengths are associated with increased calf survivorship, as well as increased reproductive success in baleen whales (Pack et al., 2009; Stewart et al., 2022).
We found no relationship between calves’ BAIs and their mothers’ BAIs. On the breeding grounds, lactating female humpback whales in better body condition had calves that were also in better body condition, supporting the hypothesis that females with insufficient energy reserves reduce their energetic investment toward their offspring in favor of their own survival (Christiansen et al., 2016). This was confirmed in southern right whales on the breeding grounds, where maternal loss in body condition was proportional to investment in her calf (Christiansen et al., 2018). The weaning process is presumed to begin while on the WAP foraging grounds, as calves are typically not fully weaned until afterwards, with some calves even returning to the breeding grounds as yearlings with their mothers from May onwards (Chittleborough, 1958; Baker et al., 1987; Avila et al., 2020). Synchronous foraging events between mother and calf pairs have been observed on the WAP, with reported observations of mother’s presumably teaching their calves to feed on krill (Dawbin, 1966; Tyson et al., 2012). It is likely that calf BAI starts to become independent of its mother’s BAI as the weaning process begins – when mothers begin nursing less and their calves start to gain foraging independence.
4.4 Caveats and considerations
We were able to describe broad trends in body condition for each reproductive class, but we were unable to collect repeated measurements of known individuals throughout the season. Future studies should aim to obtain repeated measurements of known individuals to help better understand the rate of change in BAI over the course of the season, as well as variation across seasons and life history events. Though, body condition measurements from a cross-sectional sample has been shown to be strongly correlated with body condition measurements from repeated individuals (Christiansen et al., 2020a). Additionally, increased sampling of body condition in the early (November and December) and late (April – June) foraging season would resolve some uncertainty in the differences in mass gain rates amongst reproductive classes. We also did not take into account environmental variability across seasons, which can influence inter-annual differences in baleen whale body condition (i.e., Ichii et al., 1998; Williams et al., 2013; Torres et al., 2022). The timing of migration to and from the foraging grounds and the number of days spent foraging along the WAP was also unknown for each individual, which can influence an individual’s body condition (Irvine et al., 2017b) and likely explains some of the variation in BAI observed within each reproductive class (Table 2). Combined, these factors will be especially important in monitoring how variation in body condition is related to changes in sea ice, krill abundance, individual residency times, the krill fishery, and tourism. Linking repeated measurements of known individuals over time with vital rates will characterize the energetic demands of each reproductive class and help monitor population response to these environmental and anthropogenic disturbances (Pirotta et al., 2018; Pirotta et al., 2022).
It is also important to note that while BAI yields low measurement uncertainty with high precision for capturing differences in body condition amongst individuals (Bierlich et al., 2021a), BAI does not capture body condition change in the vertical plane of the body, which also contributes to energy storage (Lockyer et al., 1985). Imagery of animals rolling on their side throughout the season could be incorporated into a volumetric model (Christiansen et al., 2020b; Hirtle et al., 2022) to help determine whether changes in the horizontal and vertical plane are consistent across reproductive classes, and such a model could also be used to estimate body mass (Christiansen et al., 2019).
While no behavioral response from the whales towards the UAS was observed, it is possible that our data may include a bias towards healthier whales that are less evasive near the various platforms used in field operations (i.e., field stations, research vessels, and tour ships). If this bias towards healthier animals existed, it would be the same throughout the study period and likely would not affect intra-seasonal trends. However, this likely had little effect on our analysis, as we observed a wide range in BAI values across the season (Table 2, Figure 3).
4.5 Future monitoring
It is expected that regional warming along the WAP will continue and may negatively impact future prey availability (Richerson et al., 2018; Atkinson et al., 2019), so it is important to continue to monitor the body condition of these whales in the future. Furthermore, there is concern for 1) the impacts of increased tourism along the WAP and migration routes (Avila et al., 2020; Sprogis et al., 2020; Arranz et al., 2021; Cavanagh et al., 2021) and 2) the krill fishery in key foraging areas (Weinstein et al., 2017). The commercial krill fishery has negatively affected krill-eating penguin populations (Watters et al., 2020; Krüger et al., 2021; Reisinger et al., 2022), and recent changes in the fishery’s operation places it in greater spatiotemporal overlap with foraging humpback whales around the WAP. Our work provides a foundational baseline for seasonal changes in body condition of this population, which can be used for monitoring any negative impacts from increasing environmental and anthropogenic stressors in the future and enact greater protective measures for humpback whales and other krill predators in the region.
Data availability statement
The body area index (BAI) and total length (TL) dataset used in this analysis can be accessed via FigShare: 10.6084/m9.figshare.21528801. Further inquiries can be directed to the corresponding author.
Ethics statement
This study was conducted under NMFS permits 14809, ACA Permits 2015-011 and 2016-024, and UCSC IACUC Friea1706, a Cetacean permit from the Australian Department of the Environment and Energy (C2018-0002), and an animal ethics permit from Murdoch University, Australia (R3018/18). Biopsy samples originating from outside the US jurisdiction were imported under the Convention on International Trade in Endangered Species (CITES) import permit numbers 16US50849B/9 through 19US504849/B.
Author contributions
All authors significantly contributed toward development of the manuscripts. KB, AF, DJ, JG, RS, AR, and JD contributed to conception and design of study. KB, JD, FC, GL, LP, RN, KS, MS, CB, AF, DJ, and JG all contributed to data collection. KB, CB, LP, and AD contributed to data processing and organization. KB and AD measured the whales. KB, JH, and RS contributed to model development and statistical analyses. KB, JH, RS, AF, DJ, JG, and AR contributed to interpretation of results. KB wrote the manuscript. All authors contributed to the article and approved the submitted version.
Funding
This work was supported in part by World Wildlife Fund, California Ocean Alliance, and One Ocean Expeditions. UAS imagery and biopsy samples were collected as part of the National Science Foundation Office of Polar Programs Grants 1643877 and 1440435 to AF.
Acknowledgments
We thank Taylorann Smith, Kendall Jeffreys, Katie Knotek, and Melissa Baldino for assistance in processing data. We are grateful to One Ocean Expeditions and staff, NSF USAP science support crew, Marine Robotics and Remote Sensing (MaRRS) Lab, and the Duke University Marine Lab for project support.
Conflict of interest
The authors declare that the research was conducted in the absence of any commercial or financial relationships that could be construed as a potential conflict of interest.
Publisher’s note
All claims expressed in this article are solely those of the authors and do not necessarily represent those of their affiliated organizations, or those of the publisher, the editors and the reviewers. Any product that may be evaluated in this article, or claim that may be made by its manufacturer, is not guaranteed or endorsed by the publisher.
Supplementary material
The Supplementary Material for this article can be found online at: https://www.frontiersin.org/articles/10.3389/fmars.2022.1036860/full#supplementary-material
References
Acevedo J., Haro D., Dalla Rosa L., Aguayo-Lobo A., Hucke-Gaete R., Secchi E., et al. (2013). Evidence of spatial structuring of eastern south pacific humpback whale feeding grounds. Endanger Species Res. 22, 33–38. doi: 10.3354/esr00536
Agbayani S., Fortune S. M. E., Trites A. W. (2020). Growth and development of north pacific gray whales (Eschrichtius robustus). J. Mammal 101, 742–754. doi: 10.1093/jmammal/gyaa028
Aguilar A., Borrell A. (1990). Patterns of lipid content and stratification in the blubber of fine whales (Balaenoptera physalus). J. Mammal 71, 544–554. doi: 10.2307/1381793
Aoki K., Isojunno S., Bellot C., Iwata T., Kershaw J., Akiyama Y., et al. (2021). Aerial photogrammetry and tag-derived tissue density reveal patterns of lipid-store body condition of humpback whales on their feeding grounds. Proc. R. Soc B Biol. Sci. 288, 20202307. doi: 10.1098/rspb.2020.2307
Arranz P., de Soto N. A., Madsen P. T., Sprogis K. R. (2021). Whale-watch vessel noise levels with applications to whale-watching guidelines and conservation. Mar. Policy 134, 104776. doi: 10.1016/j.marpol.2021.104776
Atkinson A., Hill S. L., Pakhomov E. A., Siegel V., Reiss C. S., Loeb V. J., et al. (2019). Krill (Euphausia superba) distribution contracts southward during rapid regional warming. Nat. Clim. Change 9 (2), 1–8. doi: 10.1038/s41558-018-0370-z
Atkinson A., Siegel V., Pakhomov E., Rothery P. (2004). Long-term decline in krill stock and increase in salps within the southern ocean. Nature 432, 100–103. doi: 10.1038/nature02996
Avila I. C., Dormann C. F., Garcia C., Payán L. F., Zorrilla M. X. (2020). Humpback whales extend their stay in a breeding ground in the tropical Eastern pacific. ICES J. Mar. Sci. 77, 109–118. doi: 10.1093/icesjms/fsz251
Baker C. S., Perry A., Herman L. M. (1987). Reproductive histories of female humpback whales (Megaptera novaeangliae) in the north pacific. int-res.com 41, 103–114. doi: 10.3354/meps041103
Ball H. C., Londraville R. L., Prokop J. W., George J. C., Suydam R. S., Vinyard C., et al. (2017). Beyond thermoregulation: Metabolic function of cetacean blubber in migrating bowhead and beluga whales. J. Comp. Physiol. B 187, 235–252. doi: 10.1007/s00360-016-1029-6
Best P. B., Rüther H. (1992). Aerial photogrammetry of southern right whales, eubalaena australis. J. Zool. 228, 595–614. doi: 10.1111/j.1469-7998.1992.tb04458.x
Bierlich K. C., Hewitt J., Bird C. N., Schick R. S., Friedlaender A., Torres L. G., et al. (2021a). Comparing uncertainty associated with 1-, 2-, and 3D aerial photogrammetry-based body condition measurements of baleen whales. Front. Mar. Sci. 8, e02574-17. doi: 10.3389/fmars.2021.749943
Bierlich K. C., Miller C., DeForce E., Friedlaender A. S., Johnston D. W., Apprill A. (2018). Temporal and regional variability in the skin microbiome of humpback whales along the Western Antarctic peninsula. Appl. Environ. Microbiol. 84, e02574-17. doi: 10.1128/AEM.02574-17
Bierlich K., Schick R., Hewitt J., Dale J., Goldbogen J., Friedlaender A., et al. (2021b). Bayesian Approach for predicting photogrammetric uncertainty in morphometric measurements derived from drones. Mar. Ecol. Prog. Ser. 673, 193–210. doi: 10.3354/meps13814
Bird C., Bierlich K. C. (2020). CollatriX: A GUI to collate MorphoMetriX outputs. J. Open Source Software 5, 2323–2328. doi: 10.21105/joss.02328
Burnett J. D., Lemos L., Barlow D., Wing M. G., Chandler T., Torres L. G. (2018). Estimating morphometric attributes of baleen whales with photogrammetry from small UASs: A case study with blue and gray whales. Mar. Mammal Sci. 35, 108–139. doi: 10.1111/mms.12527
Cade D. E., Kahane-Rapport S. R., Wallis B., Goldbogen J. A., Friedlaender A. S. (2022). Evidence for size-selective predation by Antarctic humpback whales. Front. Mar. Sci. 9. doi: 10.3389/fmars.2022.747788
Cavanagh R. D., Melbourne-Thomas J., Grant S. M., Barnes D. K. A., Hughes K. A., Halfter S., et al. (2021). Future risk for southern ocean ecosystem services under climate change. Front. Mar. Sci. 7. doi: 10.3389/fmars.2020.615214
Chittleborough R. G. (1955a). Aspects of reproduction in the Male humpback whale, megaptera nodosa (Bonnaterre). Mar. Freshw. Res. 6, 1–29. doi: 10.1071/MF9550001
Chittleborough R. G. (1955b). Puberty, physical maturity, and relative growth of the female humpback whale, megaptera nodosa (Bonnaterre), on the Western Australian coast. Mar. Freshw. Res. 6, 315. doi: 10.1071/MF9550315
Chittleborough R. G. (1958). The breeding cycle of the female humpback whale, megaptera nodosa(Bonnaterre). Mar. Freshw. Res. 9, 1. doi: 10.1071/MF9580001
Choquenot D. (1991). Density-dependent growth, body condition, and demography in feral donkeys: Testing the food hypothesis. Ecology 72, 805–813. doi: 10.2307/1940583
Christiansen F., Bejder L., Burnell S., Ward R., Charlton C. (2022). Estimating the cost of growth in southern right whales from drone photogrammetry data and long-term sighting histories. Mar. Ecol. Prog. Ser. 687, 173–194. doi: 10.3354/meps14009
Christiansen F., Dawson S., Durban J., Fearnbach H., Miller C., Bejder L., et al. (2020a). Population comparison of right whale body condition reveals poor state of the north Atlantic right whale. Mar. Ecol. Prog. Ser. 640, 1–16. doi: 10.3354/meps13299
Christiansen F., Dujon A. M., Sprogis K. R., Arnould J. P. Y., Bejder L. (2016). Noninvasive unmanned aerial vehicle provides estimates of the energetic cost of reproduction in humpback whales. Ecosphere 7, e01468–18. doi: 10.1002/ecs2.1468
Christiansen F., Rodríguez-González F., Martínez-Aguilar S., Urbán J., Swartz S., Warick H., et al. (2021). Poor body condition associated with an unusual mortality event in gray whales. Mar. Ecol. Prog. Ser. 658, 237–252. doi: 10.3354/meps13585
Christiansen F., Sironi M., Moore M. J., Di Martino M., Ricciardi M., Warick H. A., et al. (2019). Estimating body mass of free-living whales using aerial photogrammetry and 3D volumetrics. Methods Ecol. Evol. 10, 2034–2044. doi: 10.1111/2041-210X.13298
Christiansen F., Sprogis K. R., Gross J., Castrillon J., Warick H. A., Leunissen E., et al. (2020b). Variation in outer blubber lipid concentration does not reflect morphological body condition in humpback whales. J. Exp. Biol. 223 (8), jeb.213769. doi: 10.1242/jeb.213769
Christiansen F., Vikingsson G. A., Rasmussen M. H., Lusseau D. (2013). Minke whales maximise energy storage on their feeding grounds. J. Exp. Biol. 216, 427–436. doi: 10.1242/jeb.074518
Christiansen F., Vivier F., Charlton C., Ward R., Amerson A., Burnell S., et al. (2018). Maternal body size and condition determine calf growth rates in southern right whales. Mar. Ecol. Prog. Ser. 592, 267–281. doi: 10.3354/meps12522
Clapham P. J., S.E. W., Smith T. D., Mead J. G. (1999). Length at birth and at independence in humpback whales. J. Cetacean Res. Manage. 1, 141–146.
Craig A. S., Herman L. S., Gabriele C. M., Pack A. A. (2003). Migratory timing of humpback whales (Megaptera novaeangliae) in the central north pacific varies with age, sex and reproductive status. Behaviour 140, 981–1001. doi: 10.1163/156853903322589605
Curtice C., Johnston D. W., Ducklow H., Gales N., Halpin P. N., Friedlaender A. S. (2015). Modeling the spatial and temporal dynamics of foraging movements of humpback whales (Megaptera novaeangliae) in the Western Antarctic peninsula. Mov Ecol. 3, 1508–1509. doi: 10.1186/s40462-015-0041-x
Dawbin W. H. (1966). “The seasonal migratory cycle of humpback whales,” in Whales, dolphins, and porpoises (Berkeley, CA: University of California Press), 145–170.
Dawson S. M., Bowman M. H., Leunissen E., Sirguey P. (2017). Inexpensive aerial photogrammetry for studies of whales and Large marine animals. Front. Mar. Sci. 4. doi: 10.3389/fmars.2017.00366
Durban J. W., Fearnbach H., Barrett-Lennard L. G., Perryman W. L., Leroi D. J. (2015). Photogrammetry of killer whales using a small hexacopter launched at sea. J. Unmanned Veh Syst. 3, 131–135. doi: 10.1139/juvs-2015-0020
Durban J. W., Moore M. J., Chiang G., Hickmott L. S., Bocconcelli A., Howes G., et al. (2016). Photogrammetry of blue whales with an unmanned hexacopter. Mar. Mammal Sci. 32, 1510–1515. doi: 10.1111/mms.12328
Ejrnæs D. D., Sprogis K. R. (2022). Ontogenetic changes in energy expenditure and resting behaviour of humpback whale mother-calf pairs examined using unmanned aerial vehicles. Wildl Res. 49, 34–45. doi: 10.1071/WR20186
Florant G. L., Healy J. E. (2012). The regulation of food intake in mammalian hibernators: a review. J. Comp. Physiol. B 182, 451–467. doi: 10.1007/s00360-011-0630-y
Foley C. A. H., Faust L. J. (2010). Rapid population growth in an elephant loxodonta africana population recovering from poaching in tarangire national park, Tanzania. Oryx 44, 205–212. doi: 10.1017/S0030605309990706
Franklin T., Franklin W., Brooks L., Harrison P., Baverstock P., Clapham P. (2011). Seasonal changes in pod characteristics of eastern Australian humpback whales (Megaptera novaeangliae), hervey bay 1992-2005. Mar. Mammal Sci. 27, 134–152. doi: 10.1111/j.1748-7692.2010.00430.x
Friedlaender A. S., Johnston D. W., Fraser W. R., Burns J., Patrick N H., Costa D. P. (2011). Ecological niche modeling of sympatric krill predators around Marguerite bay, Western Antarctic peninsula. Deep Sea Res. Part II Top. Stud. Oceanogr 58, 1729–1740. doi: 10.1016/j.dsr2.2010.11.018
Friedlaender A. S., Johnston D. W., Tyson R. B., Kaltenberg A., Goldbogen J. A., Stimpert A. K., et al. (2016). Multiple-stage decisions in a marine central-place forager. R. Soc Open Sci. 3, 160043. doi: 10.1098/rsos.160043
Friedlaender A., Joyce T., Johnston D., Read A., Nowacek D., Goldbogen J., et al. (2021). Sympatry and resource partitioning between the largest krill consumers around the Antarctic peninsula. Mar. Ecol. Prog. Ser. 669, 1–16. doi: 10.3354/meps13771
Friedlaender A. S., Lawson G. L., Halpin P. N. (2009). Evidence of resource partitioning between humpback and minke whales around the western Antarctic peninsula. Mar. Mammal Sci. 25, 402–415. doi: 10.1111/j.1748-7692.2008.00263.x
Gough W. T., Segre P. S., Bierlich K. C., Cade D. E., Potvin J., Fish F. E., et al. (2019). Scaling of swimming performance in baleen whales. J. Exp. Biol. 222, jeb204172–11. doi: 10.1242/jeb.204172
Green A. J. (2001). Mass/length residuals: measures of body condition or generators of spurious results? Ecology 82, 1473–1483. doi: 10.1890/0012-9658(2001)082[1473:MLRMOB]2.0.CO;2
Hagen W., Van Vleet E. S., Kattner G. (1996). Seasonal lipid storage as overwintering strategy of Antarctic krill. Mar. Ecol. Prog. Ser. 134, 85–89. doi: 10.3354/meps134085
Herr H., Viquerat S., Siegel V., Kock K.-H., Dorschel B., Huneke W. G. C., et al. (2016). Horizontal niche partitioning of humpback and fin whales around the West Antarctic peninsula: evidence from a concurrent whale and krill survey. Polar Biol. 39, 799–818. doi: 10.1007/s00300-016-1927-9
Hirtle N. O., Stepanuk J. E. F., Heywood E. I., Christiansen F., Thorne L. H. (2022). Integrating 3D models with morphometric measurements to improve volumetric estimates in marine mammals. Methods Ecol. Evol. 2022, 1–13. doi: 10.1111/2041-210X.13962
Ichii T., Shinohara N., Fujise Y., Nishiwaki S., Matsuoka K. (1998). Interannual changes in body fat condition index of minke whales in the Antarctic. Mar. Ecol. Prog. Ser. 175, 1–12. doi: 10.3354/meps175001
Irvine L. G., Thums M., Hanson C. E., McMahon C. R., Hindell M. A. (2017a). Evidence for a widely expanded humpback whale calving range along the Western Australian coast. Mar. Mammal Sci. 34, 294–310. doi: 10.1111/mms.12456
Irvine L. G., Thums M., Hanson C. E., McMahon C. R., Hindell M. A. (2017b). Quantifying the energy stores of capital breeding humpback whales and income breeding sperm whales using historical whaling records. R. Soc Open Sci. 4, 160290. doi: 10.1098/rsos.160290
Johannessen J. E. D., Biuw M., Lindstrøm U., Ollus V. M. S., Martín López L. M., Gkikopoulou K. C., et al. (2022). Intra-season variations in distribution and abundance of humpback whales in the West Antarctic peninsula using cruise vessels as opportunistic platforms. Ecol. Evol. 12, 1–13. doi: 10.1002/ece3.8571
Johnston D. W., Friedlaender A. S., Read A. J., Nowacek D. P. (2012). Initial density estimates of humpback whales (Megaptera novaeangliae) in the inshore waters of the western Antarctic peninsula during the late autumn. endanger. Species Res. 18, 63–71. doi: 10.3354/esr00395
Johnston S., Zerbini A. N., Butterworth D. S. (2011). A Bayesian approach to assess the status of southern hemisphere humpback whales (Megaptera novaengliae) with an applicatoin to breeding stock G. J. Cetacean Res. Manag 3, 309–317.
Jönsson K. I. (1997). Capital and income breeding as alternative tactics of resource use in reproduction. Oikos 78, 57. doi: 10.2307/3545800
Kaufman G., Smultea M., Forestell P. (1987). Use of lateral body pigmentation patterns for photographic identification of east Australian (Area V) humpback whales. Cetus 7, 5–13.
Krüger L., Huerta M. F., Santa Cruz F., Cárdenas C. A. (2021). Antarctic Krill fishery effects over penguin populations under adverse climate conditions: Implications for the management of fishing practices. Ambio 50, 560–571. doi: 10.1007/s13280-020-01386-w
Kutner M. H., Nachtsheim C. J., Neter J., William L. (2004). Applied linear statistical models. Fifth (New York: McGraw-Hill/Irwin).
Lambertsen R. (1987). A biopsy system for large whales and its use for cytogenetics. J. Mammal 68, 443–445. doi: 10.2307/1381495
Lemos L. S., Burnett J. D., Chandler T. E., Sumich J. L., Torres L. G. (2020). Intra- and inter-annual variation in gray whale body condition on a foraging ground. G Ecosphere 11, e03094. doi: 10.1002/ecs2.3094
Lockyer C. (1981). Growth and energy budgets of Large baleen whales from the southern hemisphere. Food Agric. Organ 3, 379–487.
Lockyer C. (1986). Body fat condition in northeast Atlantic fin whales, balaenoptera physalus, and its relationship w/reproduction and food resource. J. Fish Aquat Sci. 43, 142–147. doi: 10.1139/f86-015
Lockyer C. H. (1987). Evaluation of the role of fat reserves in relation to the ecology of north Atlantic fin and sei whales. In: Huntley A. C., Costa D. P., Worthy G. A. J., Castellini M. A., eds. Approaches to marine mammal energetics (Lawrence, KS: Special Publication Number 1, The Society for Marine Mammalogy), 183–203.
Lockyer C. H., McConnell L. C., Waters T. D. (1985). Body condition in terms of anatomical and biochemical assessment of body fat in north Atlantic fin and sei whales. Can. J. Zool. 63, 2328–2338. doi: 10.1139/z85-345
Marcondes M. C. C., Colosio A. C., Ramos H. G., Coelho I. P. (2017). Krill decreasing in south Georgia could explain humpback whale strandings in Brazil? (Natal, Brazil: VIII ENCOPEMAQ B).
Miller C. A., Reeb D., Best P. B., Knowlton A. R., Brown M. W., Moore M. J. (2011). And Blubber thickness in right whales eubalaena glacialis and eubalaena australis related with reproduction, life history status and prey abundance. Mar. Ecol. Prog. Ser. 438, 267–283. doi: 10.3354/meps09174
Modest M., Irvine L., Andrews-Goff V., Gough W. T., Johnston D. W., Nowacek D. P., et al. (2021). First description of migratory behavior of humpback whales from an Antarctic feeding ground to a tropical breeding ground. Anim Biotelemetry 9, 1–16. doi: 10.1186/s40317-021-00266-8
Næss A., Haug T., Nilssen E. M. (1998). Seasonal variation in body condition and muscular lipid contents in northeast Atlantic minke whale (Balaenoptera acutorostrata). Sarsia 83, 196–218. doi: 10.1080/00364827.1998.10413682
Nichols R., Cade D. E., Kahane-Rapport S., Goldbogen J., Simpert A., Nowacek D., et al. (2022). Intra-seasonal variation in feeding rates and diel foraging behavior in a seasonally fasting mammal, the humpback whale. Open Sci. 9 (7), 211674. doi: 10.1098/rsos.211674
Nieminen P., Asikainen J., Hyvrinen H. (2001). Effects of seasonality and fasting on the plasma leptin and thyroxin levels of the raccoon dog (Nyctereutes procyonoides) and the blue fox (Alopex lagopus). J. Exp. Zool. 289, 109–118. doi: 10.1002/1097-010X(20010201)289:2<109::AID-JEZ4>3.0.CO;2-I
Nowacek D. P., Friedlaender A. S., Halpin P. N., Hazen E. L., Johnston D. W., Read A. J., et al. (2011). Super-aggregations of krill and humpback whales in Wilhelmina bay, Antarctic peninsula. PloS One 6, e19173. doi: 10.1371/journal.pone.0019173
Pack A. A., Herman L. M., Spitz S. S., Hakala S., Deakos M. H., Herman E. Y. K. (2009). Male Humpback whales in the Hawaiian breeding grounds preferentially associate with larger females. Anim Behav. 77, 653–662. doi: 10.1016/j.anbehav.2008.11.015
Pallin L. J., Baker C. S., Steel D., Kellar N. M., Robbins J., Johnston D. W., et al. (2018a). High pregnancy rates in humpback whales (Megaptera novaeangliae) around the Western Antarctic peninsula, evidence of a rapidly growing population. R. Soc Open Sci. 5, 180015–180017. doi: 10.1098/rsos.180017
Pallin L., Robbins J., Kellar N., Bérubé M., Friedlaender A. (2018b). Validation of a blubber-based endocrine pregnancy test for humpback whales. Conserv. Physiol. 6, 323. doi: 10.1093/conphys/coy031
Palsbøll P., Larsen F., Sigurd-Hansen E. (1991). Sampling of skin biopsies from free-ranging large cetaceans at West Greenland: Development of biopsy tips and new designs of bolts. Rep. Int. Whal Commun. 13, 71–79.
Partridge L., Harvey P. H. (1988). The ecological context of life history evolution. Science 80. 241, 1449–1455. doi: 10.1126/science.241.4872.1449
Peig J., Green A. J. (2010). ). the paradigm of body condition: a critical reappraisal of current methods based on mass and length. Funct. Ecol. 24, 1323–1332. doi: 10.1111/j.1365-2435.2010.01751.x
Pirotta E., Booth C. G., Costa D. P., Fleishman E., Kraus S. D., Lusseau D., et al. (2018). Understanding the population consequences of disturbance. Ecol. Evol. 24, 712. doi: 10.1002/ece3.4458
Pirotta E., Thomas L., Costa D. P., Hall A. J., Harris C. M., Harwood J., et al. (2022). Understanding the combined effects of multiple stressors: A new perspective on a longstanding challenge. Sci. Total Environ. 821, 153322. doi: 10.1016/j.scitotenv.2022.153322
Pitman R. L., Totterdell J. A., Fearnbach H., Ballance L. T., Durban J. W., Kemps H. (2015). Whale killers: Prevalence and ecological implications of killer whale predation on humpback whale calves off Western Australia. Mar. Mammal Sci. 31, 629–657. doi: 10.1111/mms.12182
Ransome N., Bejder L., Jenner M., Penfold G., Brosig V. J., Kitson C., et al. (2022). Observations of parturition in humpback whales (Megaptera novaeangliae) and occurrence of escorting and competitive behavior around birthing females. Mar. Mammal Sci. 38, 408–432. doi: 10.1111/mms.12864
Reisinger R., Trathan P. N., Johnson C. M., Joyce T. W., Durban J. W., Pitman R. L., et al. (2022). Spatiotemporal overlap of baleen whales and krill fisheries in the Antarctic peninsula region. Front. Mar. Sci 9. doi: 10.3389/fmars.2022.914726
Reiss C. S., Cossio A., Santora J. A., Dietrich K. S., Murray A., Greg Mitchell B., et al. (2017). Overwinter habitat selection by Antarctic krill under varying sea-ice conditions: Implications for top predators and fishery management. Mar. Ecol. Prog. Ser. 568, 1–16. doi: 10.3354/meps12099
Richerson K., Driscoll R., Mangel M. (2018). Increasing temperature may shift availability of euphausiid prey in the southern ocean. Mar. Ecol. Prog. Ser. 588, 59–70. doi: 10.3354/meps12460
Rocha R., Clapham P. J., Ivashchenko Y. V. (2014). Emptying the oceans: a summary of industrial whaling catches in 20th century. Mar. Fish Rev. 76, 37–48. doi: 10.7755/MFR.76.4.3
Russell G., Colefax A., Christiansen F., Russell G., Fowler Z., Cagnazzi D. (2022). Do fatter whales migrate earlier? body condition and migratory timing of east Australian humpback whales. Mar. Ecol. Prog. Ser. 692, 169–183. doi: 10.3354/meps14075
Sahu A. (2004). Minireview: A hypothalamic role in energy balance with special emphasis on leptin. Endocrinology 145, 2613–2620. doi: 10.1210/en.2004-0032
Savoca M. S., Czapanskiy M. F., Kahane-Rapport S. R., Gough W. T., Fahlbusch J. A., Bierlich K. C., et al. (2021). Baleen whale prey consumption based on high-resolution foraging measurements. Nature 599, 85–90. doi: 10.1038/s41586-021-03991-5
Sprogis K. R., Videsen S., Madsen P. T. (2020). Vessel noise levels drive behavioural responses of humpback whales with implications for whale-watching. Elife 9, 1–17. doi: 10.7554/eLife.56760
Stephens P. A., Boyd I. L., McNamara J. M., Houston A. I. (2009). Capital breeding and income breeding: Their meaning, measurement, and worth. Ecology 90, 2057–2067. doi: 10.1890/08-1369.1
Stevenson R. D., Woods W. A. (2006). Condition indices for conservation: New uses for evolving tools. Integr. Comp. Biol. 46, 1169–1190. doi: 10.1093/icb/icl052
Stewart J. D., Durban J. W., Europe H., Fearnbach H., Hamilton P. K., Knowlton A. R., et al. (2022). Larger females have more calves: Influence of maternal body length on fecundity in north Atlantic right whales Mar. Ecol. Prog. Ser. 689, 179–189. doi: 10.3354/meps14040
Therneau T. (2018). Deming: Deming, theil-sen, passing-bablock and total least squares regression. R package version 1.4. Available at: https://cran.r-project.org/package=deming.
Torres W., Bierlich K. C. (2020). MorphoMetriX: A photogrammetric measurement GUI for morphometric analysis of megafauna. J. Open Source Software 5, 1825–1826. doi: 10.21105/joss.01825
Torres L. G., Bird C. N., Rodríguez-González F., Christiansen F., Bejder L., Lemos L., et al. (2022). Range-wide comparison of Gray whale body condition reveals contrasting Sub-population health characteristics and vulnerability to environmental change. Front. Mar. Sci. 9. doi: 10.3389/fmars.2022.867258
Townsend K. L., Kunz T. H., Widmaier E. P. (2008). Changes in body mass, serum leptin, and mRNA levels of leptin receptor isoforms during the premigratory period in myotis lucifugus. J. Comp. Physiol. B 178, 217–223. doi: 10.1007/s00360-007-0215-y
Tyson R. B., Friedlaender A. S., Nowacek D. P. (2016). Does optimal foraging theory predict the foraging performance of a large air-breathing marine predator? Anim Behav. 116, 223–235. doi: 10.1016/j.anbehav.2016.03.034
Tyson R. B., Friedlaender A. S., Ware C., Stimpert A. K., Nowacek D. P. (2012). Synchronous mother and calf foraging behaviour in humpback whales (Megaptera novaeangliae): Insights from multi-sensor suction cup tags. Mar. Ecol. Prog. Ser. 457, 209–220. doi: 10.3354/meps09708
Vikingsson G. A. (1995). Body condition of fin whales during summer off Iceland. Elsevier Sci. 4, 361–369. doi: 10.1016/S0163-6995(06)80037-5
Víkingsson G. A. (1997). Feeding of fin whales (Balaenoptera physalus) off Iceland - diurnal and seasonal variation and possible rates. J. Northwest Atl. Fish Sci. 22, 77–89. doi: 10.2960/J.v22.a7
Watters G. M., Hinke J. T., Reiss C. S. (2020). Long-term observations from Antarctica demonstrate that mismatched scales of fisheries management and predator-prey interaction lead to erroneous conclusions about precaution. Sci. Rep. 10, 2314. doi: 10.1038/s41598-020-59223-9
Weinstein B. G., Double M., Gales N., Johnston D. W., Friedlaender A. S. (2017). Identifying overlap between humpback whale foraging grounds and the Antarctic krill fishery. Biol. Conserv. 210, 184–191. doi: 10.1016/j.biocon.2017.04.014
Weinstein B. G., Friedlaender A. S. (2017). Dynamic foraging of a top predator in a seasonal polar marine environment. Oecologia 185, 427–435. doi: 10.1007/s00442-017-3949-6
Williams R., Vikingsson G. A., Gislason A., Lockyer C., New L., Thomas L., et al. (2013). Evidence for density-dependent changes in body condition and pregnancy rate of north Atlantic fin whales over four decades of varying environmental conditions. ICES J. Mar. Sci. 70, 1273–1280. doi: 10.1093/icesjms/fst059
Keywords: drones (unmanned aerial vehicles or UAVs), body condition, humpback whale (Megaptera novaeangliae), Western Antarctic Peninsula (WAP), photogrammetry, baleen whales, Bayesian
Citation: Bierlich KC, Hewitt J, Schick RS, Pallin L, Dale J, Friedlaender AS, Christiansen F, Sprogis KR, Dawn AH, Bird CN, Larsen GD, Nichols R, Shero MR, Goldbogen J, Read AJ and Johnston DW (2022) Seasonal gain in body condition of foraging humpback whales along the Western Antarctic Peninsula. Front. Mar. Sci. 9:1036860. doi: 10.3389/fmars.2022.1036860
Received: 05 September 2022; Accepted: 01 November 2022;
Published: 21 November 2022.
Edited by:
Michele Thums, Australian Institute of Marine Science (AIMS), AustraliaReviewed by:
Salvatore Siciliano, Escola Nacional de Saúde Pública Sergio Arouca, Fundação Oswaldo Cruz (Fiocruz), BrazilLyn Irvine, University of Tasmania, Australia
Robert L. Brownell Jr, Southwest Fisheries Science Center (NOAA), United States
Copyright © 2022 Bierlich, Hewitt, Schick, Pallin, Dale, Friedlaender, Christiansen, Sprogis, Dawn, Bird, Larsen, Nichols, Shero, Goldbogen, Read and Johnston. This is an open-access article distributed under the terms of the Creative Commons Attribution License (CC BY). The use, distribution or reproduction in other forums is permitted, provided the original author(s) and the copyright owner(s) are credited and that the original publication in this journal is cited, in accordance with accepted academic practice. No use, distribution or reproduction is permitted which does not comply with these terms.
*Correspondence: K. C. Bierlich, a2V2aW4uYmllcmxpY2hAb3JlZ29uc3RhdGUuZWR1