Pelagic connectivity of European sea bass between spawning and nursery grounds
- 1Centre for Environment, Fisheries and Aquaculture Science (Cefas), Lowestoft, United Kingdom
- 2School of Environmental Sciences, University of East Anglia, Norwich, United Kingdom
- 3Instituto Español de Oceanografía (IEO, CSIC), Centro Oceanográfico de A Coruña, A Coruña, Spain
- 4Helford Marine Conservation Group, Helford Voluntary Marine Conservation Area, United Kingdom
- 5School of Biological and Marine Sciences, University of Plymouth, Plymouth, United Kingdom
- 6Inland Fisheries Ireland, Dublin, Ireland
The sea bass stock around the UK (northern stock) has declined rapidly over the past decade, likely due to a combination of both overfishing and low recruitment. Understanding mechanisms that drive the number of young reaching nursery grounds is important for explaining observed recruitment variability and therefore developing effective management strategies. An individual-based model (IBM) has been developed here to investigate factors affecting sea bass settlement on nursery grounds for the northern stock. Simulations considered seven years with varying environmental conditions and year class strength, and outputs were compared to empirical data from monitoring of nursery areas in the UK and Ireland. The IBM includes hydrodynamics to simulate the drift of eggs and larvae, temperature-dependent growth and mortality, and behavior affecting position in the water column. Comparison between the model and ICES assessment abundance of age class zero fish showed similar temporal variability, but with higher recruitment predicted for 2018, and a lower peak recruitment in 1997. The model reproduced general patterns of interannual variability for the Thames estuary, but performed less well for some other regions. Further investigation is needed to understand differences between modelled supply and observations, as these could be due to uncertainties in reproducing the physical environment (e.g., currents, temperature) or biology (e.g., spawning, behavior, mortality), as well as uncertainty in recruitment derived from stock assessments or data collected from nursery grounds. Nevertheless, the model provides a useful demonstration of connectivity between spawning and nursery grounds, showing spawning regions that are likely to have greatest influence on recruitment in different estuaries, and how these may vary interannually. For example, sources from western Channel and Celtic Sea can supply larvae to many areas, leading to connectivity across the Channel and Celtic Sea, and into the Irish Sea. However, other regions may depend on more local areas. For example, with the Solent estuary dependent on sources within the Channel each year. With the temperature-dependence on growth, and therefore duration of the pelagic stage, results show the potential influence of spawning timing, in relation to ocean temperatures, for connectivity between spawning and nursery grounds.
1 Introduction
The relationship between spawning and the number of fish recruited into that stock is a key metric for implementing successful fisheries management. However, despite decades of research, predicting this relationship continues to be challenging (Subbey et al., 2014). High spawning stock biomass (SSB) does not necessarily result in high levels of recruitment, due to the large number of interacting biological and physical drivers integrated over the early life stages. The pelagic larval phase has been studied in numerous freshwater (Ludsin et al., 2014; Stocks et al., 2021) and marine systems (e.g., coral reefs (Schlaefer et al., 2018), temperate rocky shores (Borges et al., 2007), and commercial fisheries (Bolle et al., 2009). In the marine environment, mortality rates (e.g., Stige et al., 2019) as well as larval dispersal to settlement sites (e.g., Gaines et al., 2007; van der Molen et al., 2007), are likely to be key drivers in varying year class strength for many important commercial fish stocks (e.g., van der Veer et al., 2000).
European sea bass (Dicentrarchus labrax; hereafter “sea bass”) is an important target species for commercial and recreational fishers around the UK (Armstrong et al., 2013; Hyder et al., 2017; Hyder et al., 2018a; Radford et al., 2018). However, after decades of exploitation and minimal regulation (Pickett and Pawson, 1994), assessments have shown that the stock declined rapidly between 2010 and 2018 (ICES, 2021). This decline was attributed to a combination of poor recruitment and fishing mortality, which led to the implementation of emergency management measures in 2015. Stringent harvest restrictions for both commercial and recreational fisheries have continued until present day (ICES, 2021). However, despite these measures, recruitment levels have remained lower than those typically observed prior to 2010, with the underlying drivers behind variability in stock levels still uncertain.
In the ICES defined northern stock (ICES, 2021), sea bass are slow growing and long lived, maturing between 4-6 years (35-49cm) with a maximum age of 30 years (Pickett and Pawson, 1994). Sea bass are both highly eurythermic and euryhaline, with these traits facilitating the complex life cycle and migratory behavior seen in this stock (Pickett and Pawson, 1994). Between February and June, northern stock mature sea bass undertake a spawning migration and form spawning aggregations in the Celtic Sea and southern North Sea. The geographic extent of these spawning distributions is thought to be bounded by a minimum temperature of 9°C, meaning distribution can expand in warmer years and as the season progresses (Pickett and Pawson, 1994). During spawning, sea bass release up to three batches of eggs over a three-week period (Mayer et al., 1990). The pelagic early life history stage then lasts between 2 and 4 months as eggs and larvae drift from the offshore spawning grounds to the coastline around the British Isles (Jennings and Ellis, 2015). Temperature is thought to influence not only the spatial extent of the spawning area but also recruitment, as lower recruitment is apparent in cooler years (ICES, 2012). This latter phenomenon may be due to both temperature affecting survival of juveniles in nursery areas, and the associated meteorological processes that drive egg and larval trajectories and survival.
Particle tracking models for fish eggs and larvae, which combine hydrodynamics and individual dynamics (e.g., van der Veer et al., 1998; van der Molen et al., 2007; Savina et al., 2010) are a useful method for investigating pelagic stages of fish and the drivers of year class strength. Critically, these models are spatially and temporally explicit and can be used to assess both the spatial and temporal distribution of pelagic life stages and connectivity between spawning areas and larval settlement. Particle tracking models are often coupled with individual based models (IBMs) and use a bottom-up approach to simulate a population of discreet individuals (i.e., eggs and larvae), with a combination of individual state (e.g., growth, mortality rate) and environmental variables (e.g., sea surface temperature, current velocity) that influence individual behavior (i.e., movement) (DeAngelis and Grimm, 2014). These models when coupled with IBMs have been used in pelagic phase studies of many fish species including European anchovy (Engraulis encrasicolus) in the northwest Mediterranean (Ospina-Alvarez et al., 2012), important flat fish species in the North Sea (Savina et al., 2010; Hufnagl et al., 2013; Barbut et al., 2019) and the European sea bass (Beraud et al., 2018).
The objective of this study was to investigate the factors influencing pelagic connectivity between spawning and nursery grounds, and how this relates to variability in year class strength within the northern sea bass stock. To do so, this study builds on the numerical modelling approach developed within Beraud et al. (2018), providing further developments to the IBM as well as extending the model domain to consider spawning within the entire northern stock. To assess drivers of variability, simulations considered years with varying environmental conditions and year class strength. A comparison with time series of settlement reported in stock assessments, as well as field studies in UK and Irish nursery areas, demonstrates the applicability of the model to reproduce (or predict) observed variability within the northern stock as well as individual nursery grounds. This study then provides an overview of the mechanisms responsible for varying connectivity and recruitment within the IBM, including how these relate to observed variability in year class strength across the stock region.
2 Methods
The pelagic phase model used here was built on the approach developed by Beraud et al. (2018) for sea bass in the northern stock (North Sea, English Channel, Celtic Sea, and Irish Sea; ICES Statistical Areas 4b-c, 7a, d-h). This previous study combined a Lagrangian particle-tracking model and a hydrodynamic model, to account for physical transport by currents as well as the behavior of the early life stages of sea bass within an IBM. This model was extended here to include a larger domain and later particle release timings to account for spawning in the North Sea. Predicted settlement rates were compared between years to assess whether the model could account for variability observed in both the regional stock assessment and field studies of specific nursery areas in England and Ireland.
2.1 Hydrodynamic model and Lagrangian particle tracking
Hydrodynamic conditions were obtained from the General Estuarine Transport Model, GETM v2.4 (www.getm.eu; Burchard and Bolding, 2002), using a northwest European Shelf configuration, with 0.08°C x 0.05°C horizontal resolution (~ 5.5 km) and 25 vertical sigma levels. The model was forced at the open ocean boundaries with tidal elevations obtained from Topex-Poseidon (Le Provost et al., 1998) and with temperatures and salinities from the global hindcast European Centre for Medium-Range Weather Forecast - Ocean Reanalysis System 4 (ECMWF-ORAS4) (Mogensen et al., 2012; Balmaseda et al., 2013). Atmospheric forcing was provided from the ERA5 global reanalysis product (ECMWF fifth generation reanalysis; Hersbach et al., 2020). River runoff volumes are provided from a range of gauge datasets from the UK, France, the Netherlands, Germany, and Norway. Further details of this model configuration can be found in van der Molen et al. (2018). Current velocity, diffusivity, and water temperature were all saved at hourly frequency, to resolve the effect of tides on circulation. These simulations were used to force the particle tracking model offline, providing the environmental conditions required to determine the trajectories and behavior of each individual particle.
The particle tracking model, General Individual Transport Model (GITM 2.0), was used to simulate the potential supply of sea bass to nursery grounds. GITM combines physical diffusion of particles with biological development and behavior, through defined development stages (e.g., Wolk, 2003; Tiessen et al., 2014; van der Molen et al., 2016). For this study, the trajectories of sea bass early life stages consider the particle behavior in terms of buoyancy, vertical migration patterns, growth, and settlement. This behavior and development, as a function of temperature, were implemented in GITM using the framework initially presented by Beraud et al. (2018), but with further developments here discussed in Sections 2.3 and 2.4.
2.2 Characterization of spawning and nursery areas
The northern sea bass stock includes the North Sea, English Channel, Celtic Sea, and Irish Sea (ICES fishing areas 4b-c and 7a, d-h). For this study, the model domain covered the area between 48.25°N to 56.2°N and 9.82°W to 9.06°E (Figure 1), extending further to the south, west, and north than Beraud et al. (2018). This allows for additional spawning and settlement around the Irish coast and within the North Sea. Extension further south also allows for spawning in the Biscay stock that may drift into the northern sea bass stock area. However, for the purpose of this study, focus will be on particles spawning within the northern stock region. The release sites do not extend to the northernmost limit of the stock region, however the relative contribution to the stock from any spawning in this area would be negligible given the lower temperatures expected in the deeper northern North Sea (in relation to spawning thresholds, discussed further below). To mimic spawning, particles were released across every three model grid cells in the longitudinal direction, and every two model grid cells in the latitudinal direction, giving a total of 4,785 release points within the domain (Figure 1). To manage the number of particles (and computational expense), particles were released from each point every three days, from February to July, giving a total of 291,885 particles as potential spawning sites for each simulation.
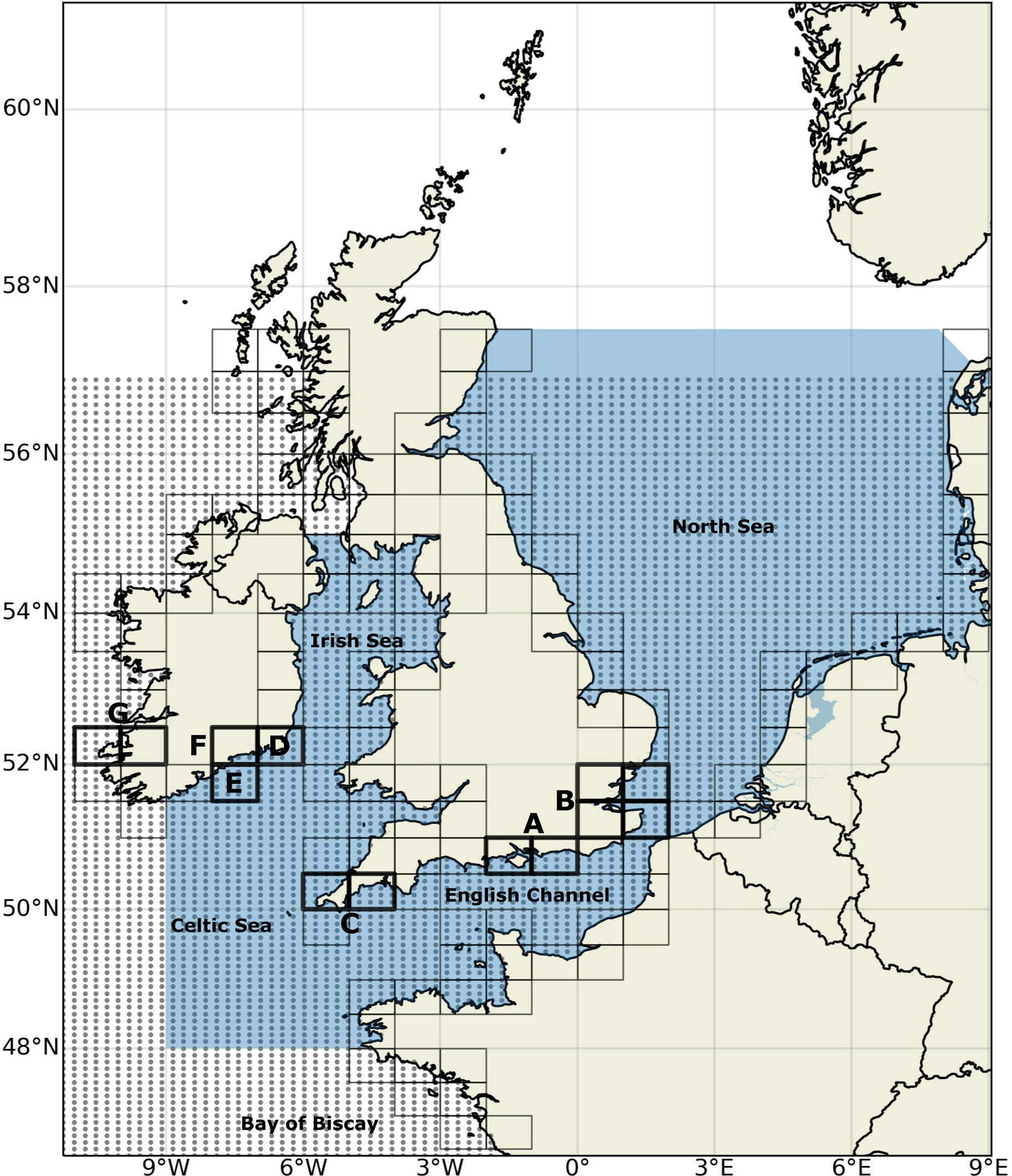
Figure 1 Model domain used for analysis. Grey dots indicate all potential spawning locations where eggs were released. Blue shading shows the northern sea bass stock region, comprising ICES Areas 4b-c and 7a, d-h. Coastal ICES rectangles (1° x 0.5° subdivisions of ICES areas; thin black lines) used to assess supply to nursey grounds, with relevant estuaries used for model comparison (thick black lines): (A) Solent; (B) Thames; (C) Fal and Helford; (D) Slaney; (E) Munster Blackwater; (F) New Ross Port; (G) Lee.
The resulting spawning area and timing was then dependent on temperature, with the 9°C isotherm used as the threshold for spawning (Pickett and Pawson, 1994). Compared with previous studies, spawning timing was extended here to occur up to July, to allow for spawning in the North Sea (e.g., van Damme et al., 2011a; van Damme et al., 2011b) where the 9°C isotherm threshold is typically passed in late April-May. It is worth noting that despite the temperature continuing to be above 9°C later into the summer, populations across the wider stock region would be unlikely to continue spawning at the same rate (Mayer et al., 1990). To account for this, each particle is assigned an egg supply that is dependent on the time of spawning, as well as the spawning stock biomass for that year (discussed further in Section 2.5).
Sea bass nursery grounds can be found in estuaries and saltmarshes, as well as other sheltered coastal sites (e.g., Pickett and Pawson, 1994). In the 1990s, 37 sites were designated as official Bass Nursery Areas (BNAs) for protection of juvenile sea bass around England and Wales (Bass (Specified Sea Areas) (Prohibition of Fishing) Order 1990: S.I. 1990/1156). However, juveniles have been found in many other coastal areas around the UK and northwest Europe (e.g., Laffaille et al., 2001; Cardoso et al., 2015; Hyder et al., 2018b). For this study, nursery grounds were then defined broadly as any coastal area. This included nursery grounds outside the northern stock area in order to assess potential connectivity between stocks. However, the north coast of Scotland was excluded as sea bass are unlikely to settle in this area (Figure 1). Settlement was considered to have been successful when a fry of length 15 mm or above (Jennings and Ellis, 2015) arrive within coastal ICES rectangles at a depth of less than 30 m. This depth threshold was chosen to account for the resolution of the hydrodynamic model, which is unable to fully resolve shallow estuarine regions. The connectivity between spawning and nursery areas was then analyzed at the spatial resolution of the ICES rectangles across the whole model domain.
2.3 Development and behavior
The early life stages of sea bass were split into one egg and three larval stages (hatchling, larva, and fry), as summarized in Table 1. The size, growth and behavior associated with each stage were based initially on those found to best represent sea bass within Beraud et al. (2018). These behaviors are outlined here, along with description of any differences to previous studies.
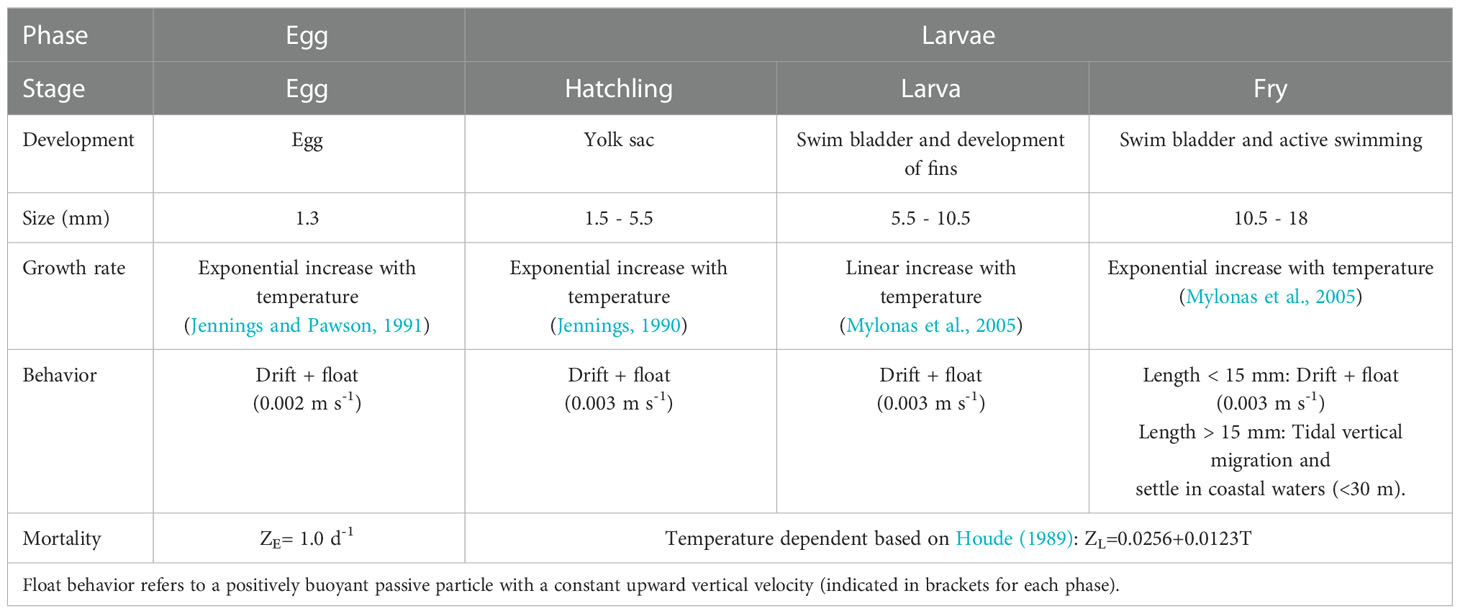
Table 1 Parameters used to model growth and behavior of sea bass at different pelagic developmental stages, and corresponding sizes (modified from Beraud et al., 2018).
The development of eggs was defined using the same relationship as previous studies (Beraud et al., 2018), with the duration of this stage (DE) dependent on temperature:
where a and b were defined for each of the egg sub-substages following Jennings and Pawson (1991). After hatching, the first larval phase (the hatchling) also followed the same development and behavior as defined in Beraud et al. (2018), with the duration of this stage in days (DH) depending on temperature according to:
where a=1.89 and b=0.077, following Jennings (1990).
The development of the subsequent larval phases differed here to the constant rate of growth that was assumed in Beraud et al. (2018). Temperature-dependent growth rates were based on analysis from Mylonas et al. (2005), where larvae were exposed to three different temperatures (13, 17, and 21°C) and length recorded over time. Based on Mylonas et al. (see their Figure 3), the duration of the larva (length 5.5–10.5 mm) phase (DL) was assumed to vary linearly with temperature (T), such that:
with DL given in days. During the subsequent stage, for fry (length 10.5–18 mm), phase duration (DF) varied non-linearly with temperature. Limited data were available to determine a best fitting curve, so for consistency with hatchling phase, the same relationship as Equation (2) was chosen, with a=4.37 and b=0.069. The resulting growth rates and durations defined for larva and fry phases are similar to the 0.2 mm/day used in Beraud et al. (2018), but crucially allow for temperature-dependent variability in this study.
Vertical behavior of each of the stages was defined as buoyant for the hatchling and larva phases, followed by tidal migration during the final development stage (when looking to settle; Table 1). These behaviors were selected as the scenario that best replicated the observed settlement in 1996 and 1997 in Beraud et al. (2018). Horizontal swimming was not accounted for in the model as movement is negligible in comparison with current speed, so was unlikely to impact on the outcomes of particles (Leis et al., 2012). Settlement was considered to have been successful when fry of length exceeding 15 mm (Jennings and Ellis, 2015) arrived in a coastal area (depth < 30 m).
2.4 Mortality
Very little information is available on the mortality of sea bass eggs and larvae during their early pelagic stages. The majority of information is available from laboratory experiments (e.g., Johnson and Katavic, 1984; Devauchelle and Coves, 1988; Carrillo et al., 1989; Pepin, 1991; Pope et al., 2014) or adult farmed fish (e.g., El-Shebly, 2009), however, these neglect the potential influence of predation and starvation. Therefore, a uniform instantaneous mortality rate was assumed for the egg phase (ZE). However, mortality during the larval stage (ZL) was based on a temperature-dependent relationship presented in Houde (1989). Sea bass was not included in this study, but the results are considered applicable given the variety of species in the analyses.
Daily mortality rates for both the egg and larval stages (Table 1) were applied during the post-processing stage of analysis, such that the survival rate for each individual particle (Si) was calculated as follows:
where the product operator loops over all time steps (t) corresponding to the larval duration of each simulated particle. Average survival during the pelagic phase (S), for a given settlement location, was then calculated as the median survival rate across all particles settling at that location.
2.5 Model simulations and analysis
Particle tracking simulations were carried out for seven years: 1996, 1997, 2004, 2005, 2010, 2015 and 2018. These were chosen as they included differing environmental conditions (Figures 2A, 3), and differing observed settlement (Figure 4). The North Atlantic Oscillation (NAO) index describes the distribution of mean sea level pressure between the polar and subtropical North Atlantic. This index was then taken to be a proxy for varying environmental conditions, given its influence on both wind stress and temperature over the northwest European shelf (e.g., Hurrel and Deser, 2010; Holt et al., 2012). The number of particles that met the spawning criteria depended on the local temperature according to Section 2.2, and was determined during postprocessing, along with calculations of the average survival rate (Section 2.4). The number of spawned particles settling in assumed nursery grounds and the corresponding supply of fry for recruitment were then assessed.
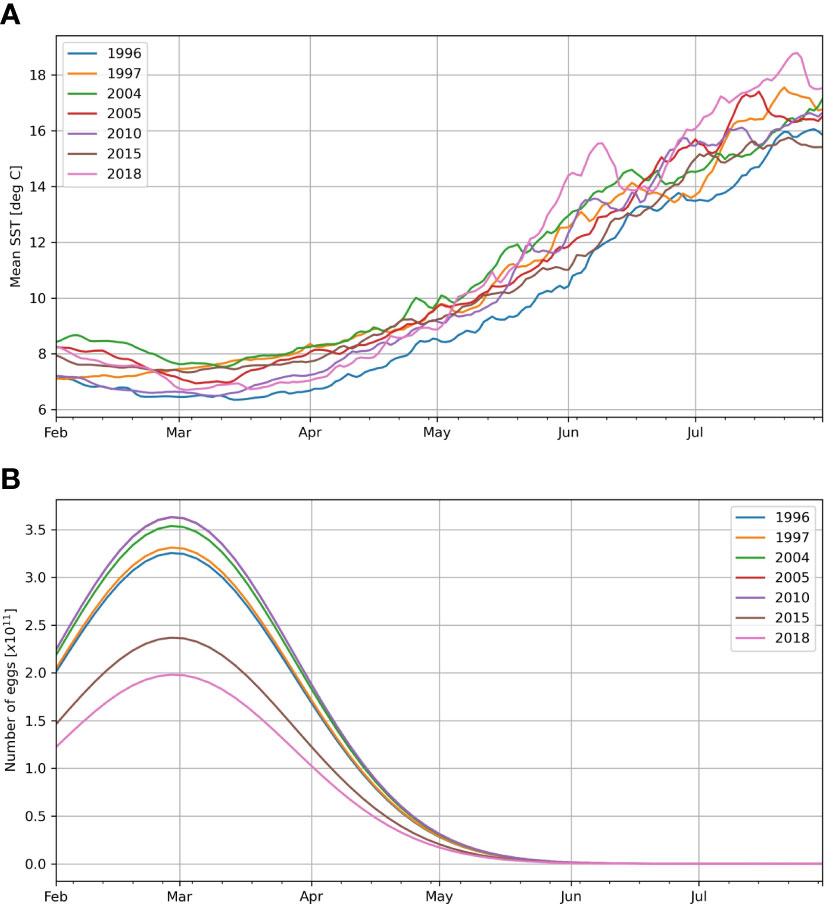
Figure 2 (A) Mean daily sea surface temperature (SST), [°C] averaged across 9°W-9°E, 48-57.5°N, for each chosen year of analysis. (B) Temporal distribution of eggs released, scaled by total available within each year of analysis. This truncated normal distribution has a mean of 1st March, and a standard deviation of 28 days.
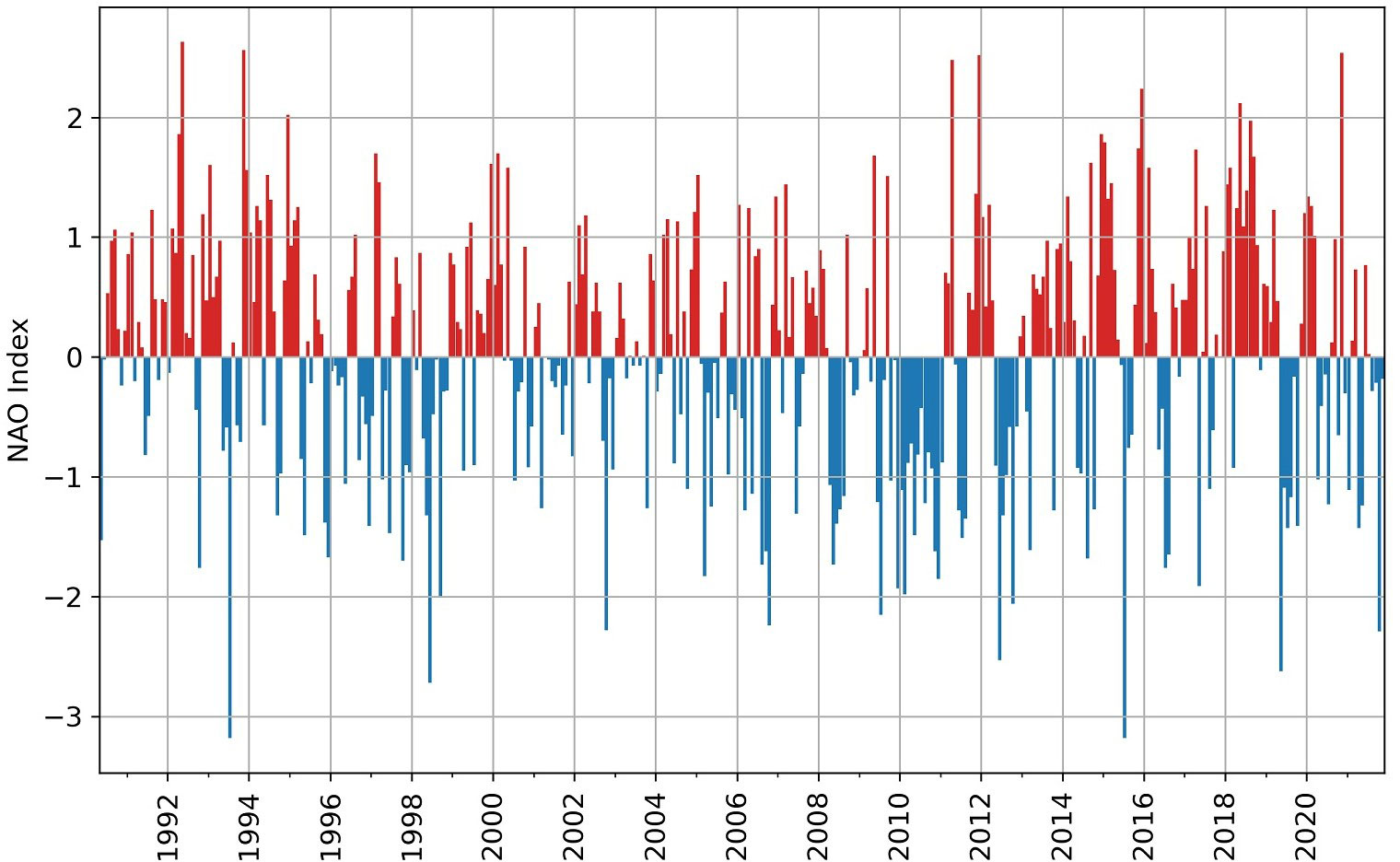
Figure 3 Monthly North Atlantic Oscillation (NAO) index (data obtained from NOAA Climate Prediction Center, 2022), for the period 1990-2021.
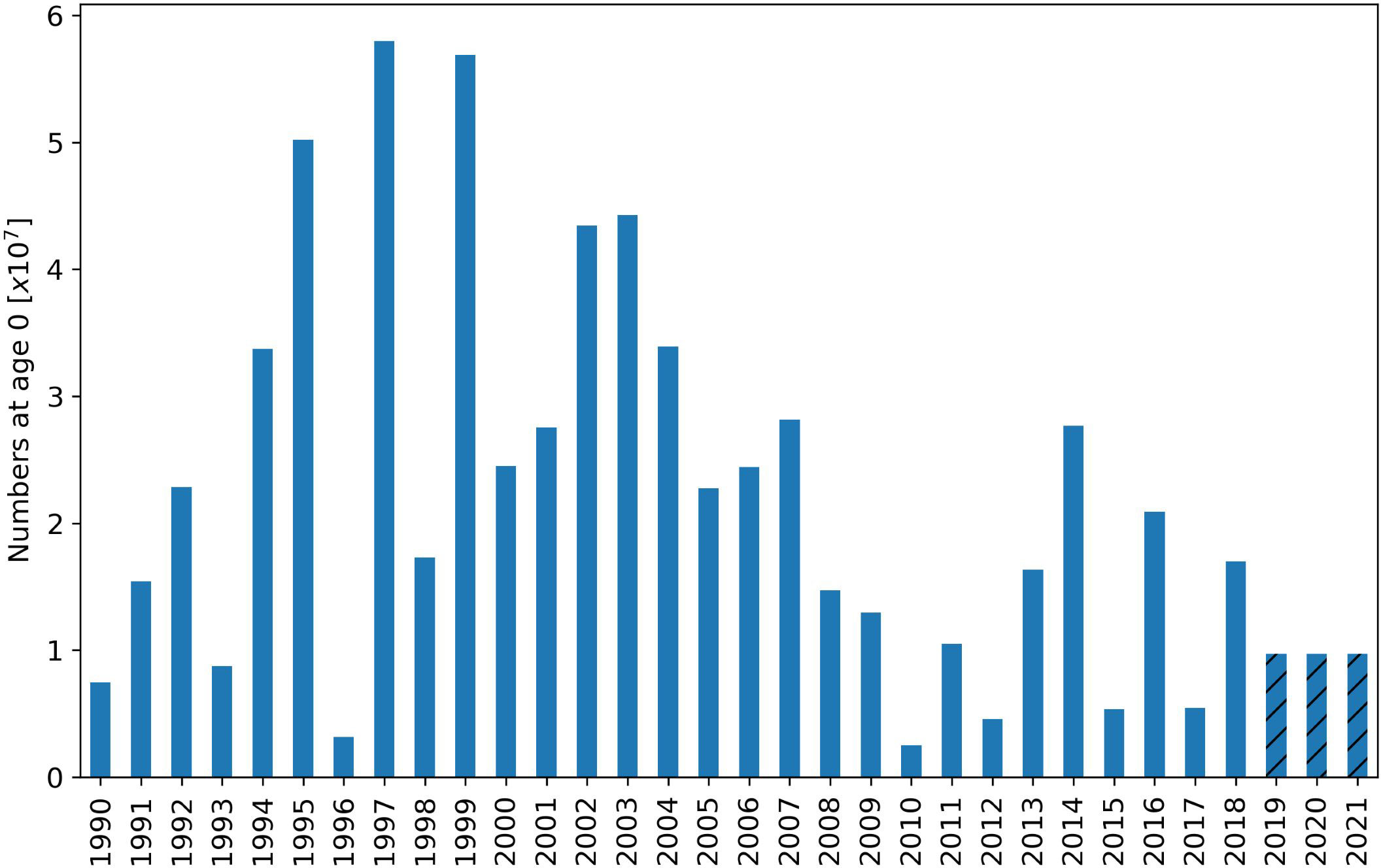
Figure 4 Numbers at age-class 0 for the period 1990-2021, from ICES (2021). Hatched shading for 2019-2021 indicates that values are based on mean recruitment over 2009-2018.
The total supply of fry to nursery grounds derived from the model results (Rm) was calculated from the proportion of spawned particles settling (p) obtained from the Lagrangian model, survival across the pelagic phase (S, Equation 4), and egg production (E), according to the following equation:
This shows that each spawned particle is considered to represent a proportion of the total number of eggs available within the stock area. Total egg production (E) was calculated from the number of females at age and the weight at age obtained from the stock assessment (ICES, 2020a), and an estimation of the absolute fecundity (eggs produced per kg per female per year) (Mayer et al., 1990). The distribution of egg production through the year was based on a truncated normal distribution, scaled by the total number of eggs for each year. This differed to the method used in Beraud et al. (2018), where eggs were assumed to be distributed regularly throughout the February-April spawning period. It was necessary to update the method here to account for the longer duration of spawning, and therefore large number of potential spawning locations in May to July.
A truncated distribution was chosen based on the sea bass spawning potentially beginning in December and January further south, outside of the Northern stock area, prior to the February start date used here (e.g., Dambrine et al., 2021). The distribution assumed that the stock would reach peak spawning in March each year, with reduced spawning rate later in the year. Different parameters were tested for the mean and standard deviation, considering a mean spawning time of either early or mid-March, and a standard deviation of either six or four weeks, based on the expected timing and duration of batch spawning (e.g., Lopez et al., 2015). Figure 2B shows the final distribution used in the results presented here, with the earlier, narrower distribution (mean of 1st March and a standard deviation of 28 days) chosen to represent a higher proportion of spawning occurring in the southwest, during February-April. For each ICES rectangle, the settlement index was calculated for each three day spawning window, based on the proportion of particles originally spawned and the corresponding egg availability within that window. This was then integrated over the entire simulation period to give a total number of eggs (and hence fry) supplied to the nursery grounds. As the number of spawned particles within each window increases later in the simulation (with temperature >9°C throughout the domain), and the number of eggs decreases, there are therefore fewer eggs likely attributable to each particle later in the season, and within the North Sea.
2.6 Stock assessments and observed settlement indices
To assess model performance, comparisons were made between the particle tracking model outputs and the age-class zero abundance from the stock assessment and long-term field surveys described below. For each of the data sets there are some caveats and assumptions that need to be understood when they are used to validate model outputs. For each of the sampling sites or stock assessments considered here, it is not possible to compare absolute numbers (e.g., due to differing spatial scales, assumptions, and methods of sampling). Instead, comparison is based on the ability of the model to reproduce patterns of interannual variability. Due to the resolution of the model, and position of ICES rectangles in relation to the estuaries of interest, a pair or group of rectangles was sometimes chosen to represent each site, rather than simply to nearest rectangle (as shown in Figure 1). For the purpose of this evaluation, although spawning could occur within the wider model domain, only spawning within the northern stock region was considered (due to dependence on the total egg production within the northern stock region).
To assess the overall abundance of age zero sea bass, the number of individuals in year class zero were taken from the 2021 stock assessment for ICES areas 4.b-c and 7.a, d-h (ICES, 2021). The sea bass stock assessment has been carried out each year using Stock Synthesis (SS) (Methot and Wetzel, 2013) that was tuned using a settlement index, generating a series of estimated numbers of age-class zero fish and their associated errors for the period from 1985–2021 (ICES, 2021). The SS model takes all the available observations from surveys and literature to assess the state of the stock (ICES, 2021) and outputs modelled ‘data’. Comparing this study’s model outputs to another model’s outputs is suboptimal, but in the absence of the extensive long-term field data, outputs from SS remain the best option for validation.
For the spatial element of the particle tracking model, the model derived settlement indices (supply of fry, Equation 5) were compared with existing settlement series (Thames and Solent recruitment indices; and numbers at age zero for Fal and Helford, Slaney, Munster Blackwater, New Ross Port, and Lee) for years that survey data were available. For two of the settlement areas in England, annual surveys of juvenile sea bass are available from the Thames and Solent estuaries (Walmsley, 2006; Brown, 2017; Brown, 2018). In the Thames estuary, trawl surveys were conducted each November between 1989 and 2009, with an additional survey in 2018. In the Solent, surveys were conducted in May and/or September, from 1979 to present (Pickett et al., 2002). Using sea bass age and length data collected during the trawl surveys, an annual recruitment index was calculated for both the Thames and Solent estuaries (see Pickett et al., 2002 for further details; note that only autumn surveys were used for the Solent index in our analysis, to reflect the settlement within a particular year). The recruitment index for the Thames estuary was based on the abundance of juveniles between the ages of zero and three (ICES, 2014). Conversely, the recruitment index for the Solent was based on the abundance of juveniles between the ages of two and four, as age zero and one sea bass were not well sampled in this area (Brown, 2017; Brown, 2018). It is then worth noting that processes affecting survival on the nursery ground (beyond age zero) may have dampened or altered any signs of variability resulting from settlement patterns.
Additional settlement areas considered in England were the Fal and Helford estuaries (located on the south coast of Cornwall), which are both known to shelter juvenile sea bass (Catherall, 2020). To assess sea bass year class strength, an annual seine net survey has been conducted here by volunteers since 1997. The main netting period is May to September, with July being excluded to protect newly settled age zero fish from net damage. Efforts were made to sample three main areas of the Fal and Helford systems consistently on at least two occasions each summer. Two different methods are typically used for sampling, where the net is either hauled up a gulley or inlet (sampling per unit area), or held across the tide (sampling per unit time) (Catherall, 2020). To address issues with patchy or inconsistent sampling methodologies, here we use data from just the “held” samples during 2005-2018, with the abundances of sea bass then aggregated across the Fal and Helford to ensure temporal coverage over the entire timeseries. However, it is important to note that inconsistencies in sampling locations within the aggregated data could still influence annual recruitment estimates.
To validate the model results for Ireland we make use of pre-recruit sea bass surveys in four rivers in the south of Ireland. Sampling was carried out using seine nets in August each year in the Rivers Lee at Tralee (2015–18, 2020), New Ross Port (2013–20), Slaney (2013–20), and Munster Blackwater (2013–20). On the Slaney, New Ross Port, and Lee waterbodies, a minimum of five beach seine net hauls were used to capture fish in littoral areas. On the Munster Blackwater, a seine was used to cut off a backwater which was positioned at high tide and monitored until low tide. All sea bass lengths were measured, with the numbers at each age group primarily determined by length. Scales from a subsample of these length groups were taken to verify their age. Here it is important to note that time series of the Irish surveys are shorter than the English surveys and as such this limits the timeframe for which the model can be validated.
3 Results
3.1 Drivers of settlement
There was variability in the numbers of particles derived from spawning and settling over the northern stock area between each of the years simulated (Figure 5A). This was initially assessed before applying egg production and mortality to investigate the impact of purely physical drivers (e.g., temperatures, windspeed, currents). As spawning was dependent on temperature, interannual variability in climate affected the number of particles spawned. The highest number of particles spawned occurred in 2004, whereas the lowest number spawned in 1996, with 120,727 and 99,707 particles, respectively. This is consistent with difference in temperature between these two years, with lower temperatures experienced in 1996 resulting in later start of spawning across the region, when compared with the warmer conditions in 2004 (Figure 2A). Looking at the spatial and temporal variability of spawning during 1996 and 2004, both years show increasing spawning from the southwest to the North Sea, as the simulation progresses (Supplementary Figures 1, 2), but there are clear differences between these two years. While spawning is widespread in the North Sea by May during 2004, there are far fewer particles spawned here during May 1996. Note also that while both these figures show an increasing number of particles spawning later in the year (as temperature exceeds the spawning threshold over a wider area), the number of eggs assigned to each particle will decrease from March-July (Figure 2B). This is illustrated for 2004 in Supplementary Figure 3, with far fewer eggs assigned to each particle spawned in June and July.
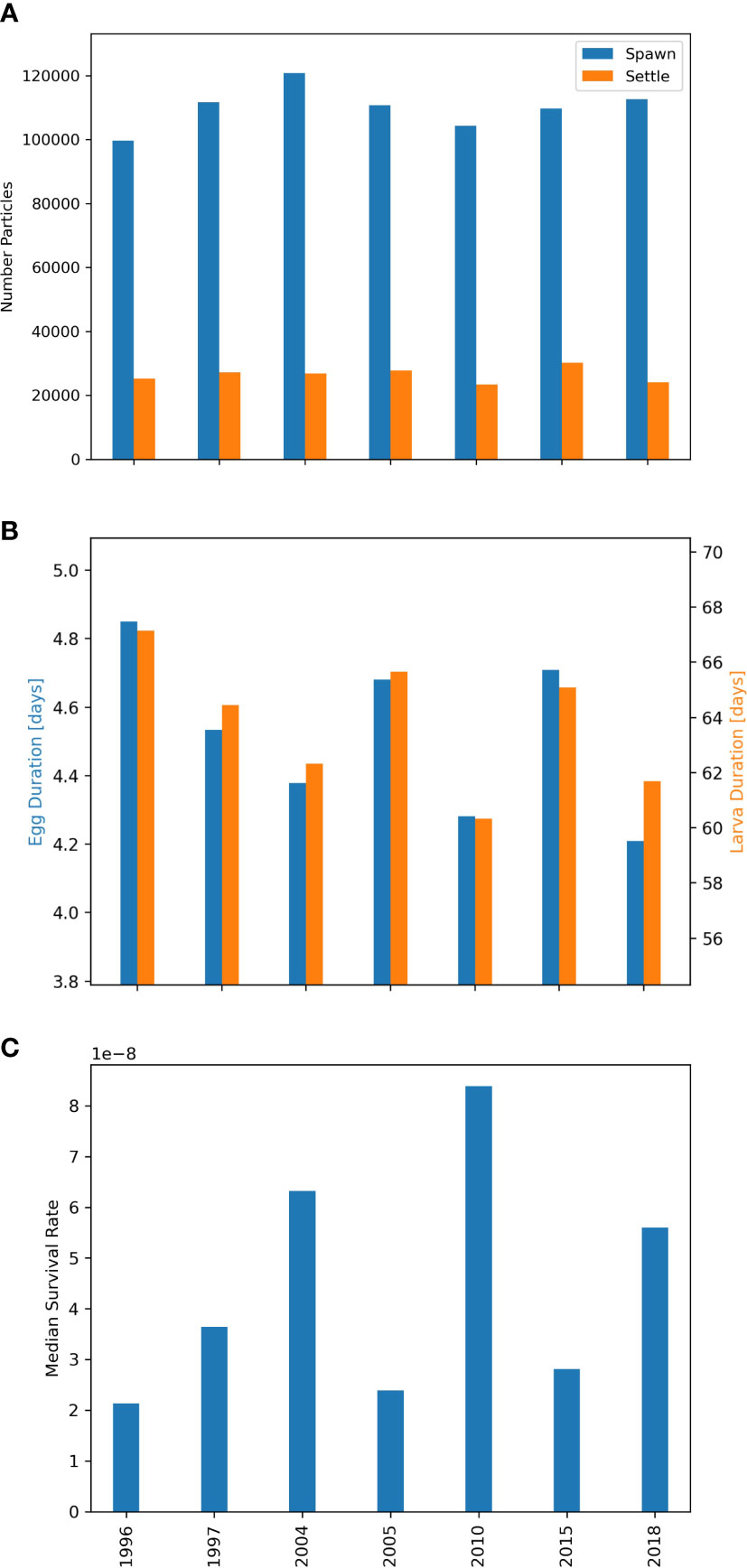
Figure 5 For each year of analysis: (A) Number of particles spawning within the northern stock region (blue) and settling within coastal ICES rectangles (orange); (B) Mean egg duration (blue) and larval duration (orange) [days], over the total number of settled particles; (C) Average survival rate (proportion of particles/eggs that survive, Equation 4), calculated as median across all settling particles.
A higher number of particles spawned does not necessarily translate to higher numbers of particles settling. This shows that current pathways, determining particle trajectories, are also likely to have an impact on interannual variability in settlement. The highest number settled occurred in 2015, whereas the lowest number settled was found in 2010, with 30,178 and 23,445 particles, equivalent to 27% and 22% of spawned particles, respectively (Figure 5A). The likelihood of settlement may depend on the location and timing of spawning during that year. For example, particles spawning further from coastal nursery areas (e.g., along the shelf-break or in the central North Sea) may be less likely to settle, and variability in wind stress has the potential to influence strength and direction of currents that determine connectivity. This variability can be separate to the mechanisms determining temperature across the region. For 2010, reduced temperatures earlier in the season lead to fewer particles spawning (Figure 2A), and there was also a strong negative phase of the North Atlantic Oscillation (NAO; Figure 3), which is typically associated with weaker westerly winds over the UK (e.g., Hurrell and Deser, 2010).
In addition to influencing spawning, temperature also determined the growth rate of eggs and larvae, and therefore the likely duration of each stage of development. There was variation between years, with mean egg duration between 4.2 and 4.8 days, and larval duration between 60 and 67 days (Figure 5B). However, there is also significant variation within each year. As growth rate increases with temperature, the average duration decreases with spawning permitted later into the year (Figure 6). Where spawning occurs earlier in the year, this typically leads to longer stages of development, as opposed to much shorter development periods for spawning later in the year. Therefore, the mean duration over the whole simulation is dependent on the temporal distribution of spawning in relation to temperature variability. For example, a longer mean duration in 1996 is consistent with lower average temperatures throughout the simulation, whereas later spawning in 2010 leads to a shorter mean duration due to increased temperatures later in the year (Figures 2A, 5B).
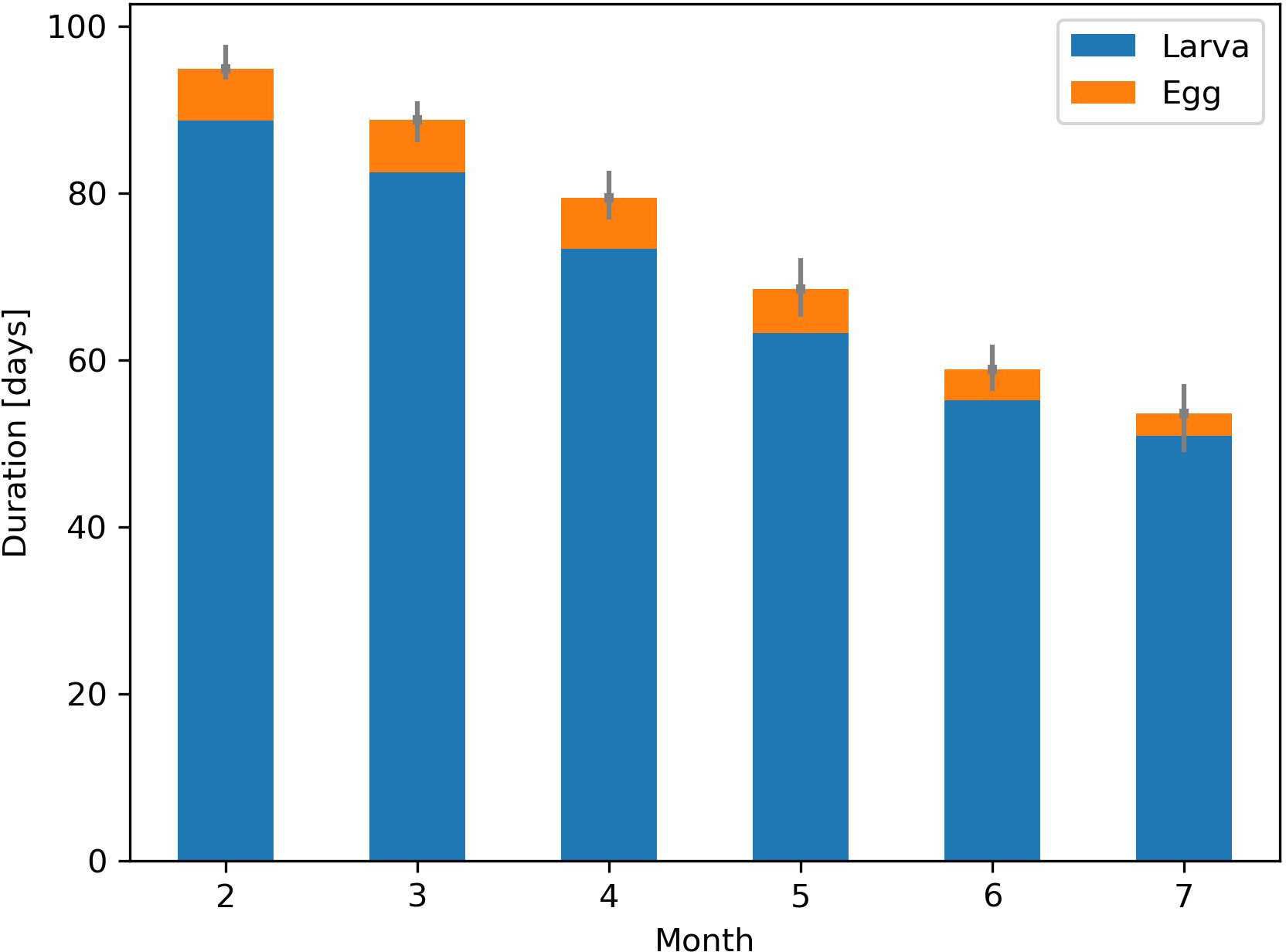
Figure 6 Monthly mean duration of egg and larva phases of development. Grey bars indicate the range in mean total duration (combined egg and larva) for all years of analysis.
Survival of each particle was dependent on both its mortality rate and the time taken to reach the nursery ground (pelagic phase duration). As mortality was dependent on temperature, survival was then also influenced by both the timing of spawning, and the temperature variability during the pelagic phase. The highest median survival across all particles was found in 2010, coinciding with a shorter pelagic phase duration (Figures 5B, C). However, it is worth noting that temperature and currents will vary across the stock area, therefore leading to regional variability in duration and survival through the pelagic phase. The resulting settlement and year class strength will then be influenced by both the total number of particles settling and the source location of those particles.
3.2 Comparison of model with settlement indices
Comparing the total supply to nursery grounds (number of fry settling) predicted in the model with the ICES assessment abundance of age class zero fish (Figure 7) shows that interannual variability in recruitment was not purely determined by the number of particles settling in nursery areas (Figure 5). Variability results from a combination of all the factors outlined above (Section 3.1) and the distribution of egg production (Figure 2B). Comparisons with the ICES assessment showed a similar pattern of interannual variability for most years, apart from the large increase in model recruitment for 2018 (Figure 7). The model also did not reproduce the large increase in recruitment shown in the ICES assessment for 1997 (Figure 7).
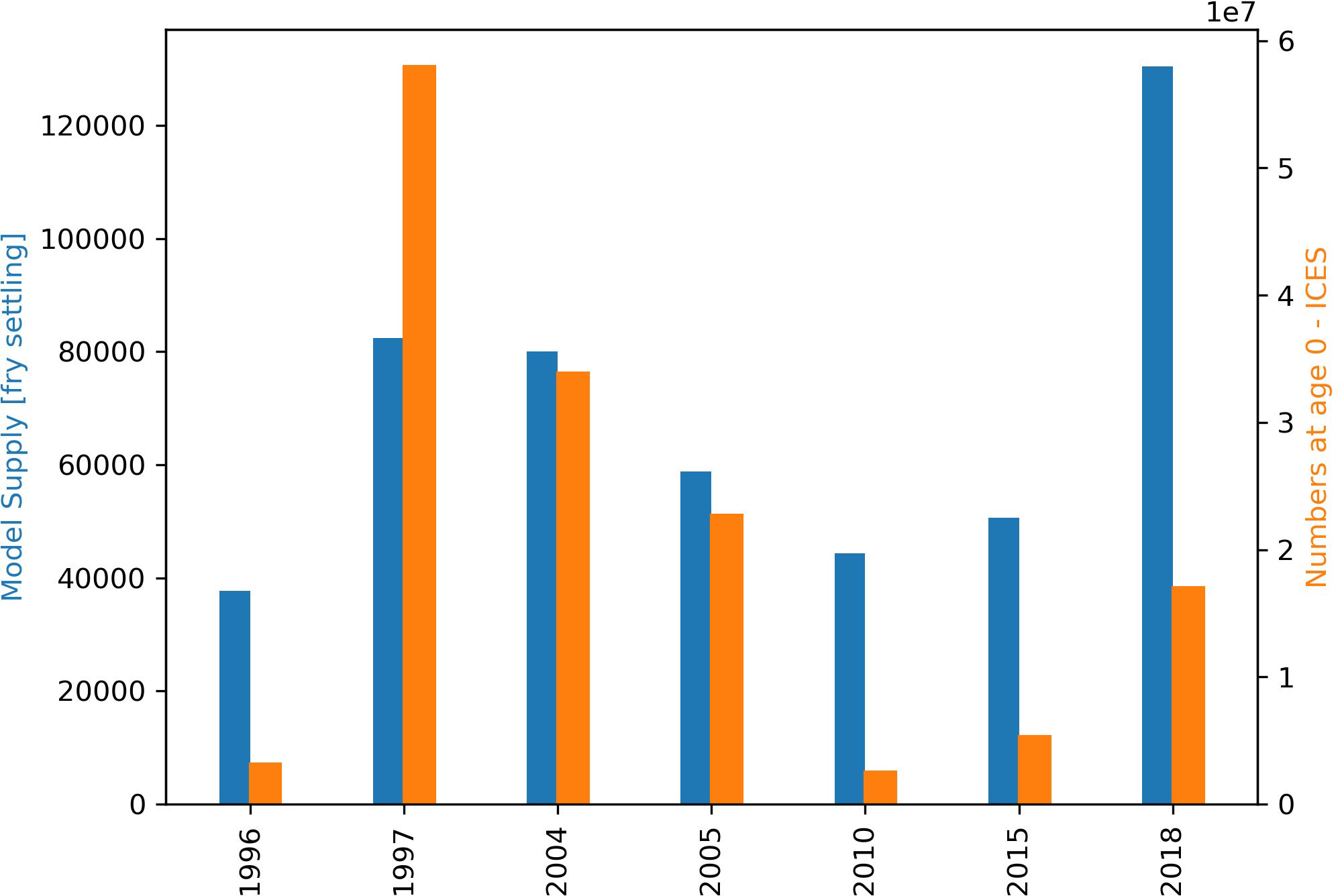
Figure 7 Comparison between model supply to coastal nursery ground (blue; number of fry settling) with ICES recruitment index (orange), for each year of analysis.
On the scale of surveys from individual nursery grounds, there were also differences between model predictions and estimates of year class strength. Model predictions for the Solent showed some similarity with the observed recruitment index in terms of variability between years, apart from a large overprediction in 2004 (Figure 8A). Similarities appeared greater between the model and observations at the Thames (Figure 8B). For the Fal and Helford, the model showed a large underprediction in 2015, but performed reasonably well in 2005, 2010 and 2018 (Figure 8C). Overall, the model results also indicate that supply to the Solent is less than those seen for the other estuaries (Figure 8).
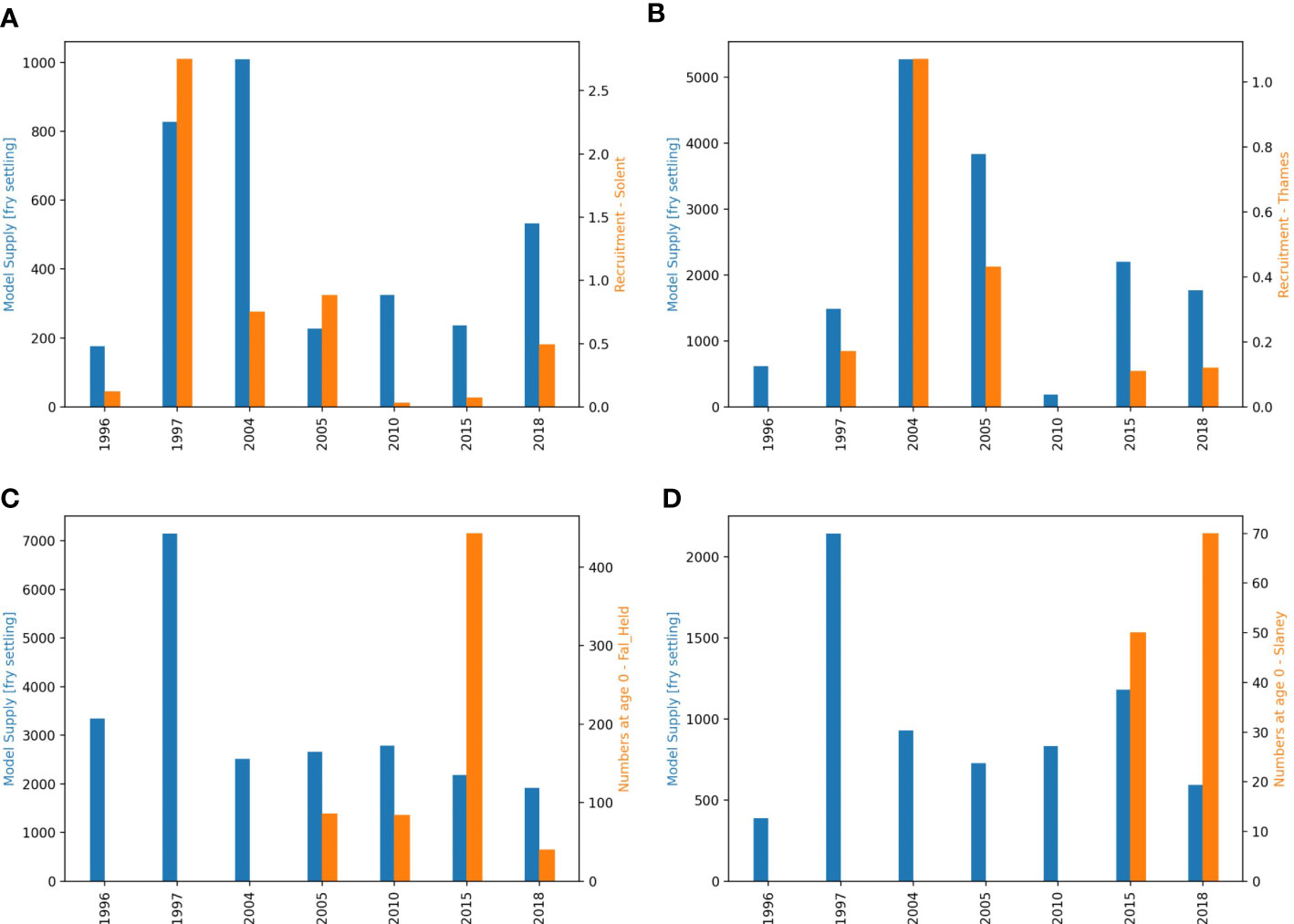
Figure 8 Comparison between model supply to nursery grounds and observed recruitment indices for each year of analysis, for (A) Solent, (B) Thames; or numbers at age 0 for (C) Fal, (D) Slaney. Recruitment indices are age aggregated and generated during analysis of the survey data (see Section 2.6).
Although the years available for comparison were limited, the model appeared to perform worse in terms of variability in the southeast of Ireland (Figure 8D). Comparing 2015 and 2018, the model shows a higher supply to nursery grounds in 2015, contrary to the observed numbers at age zero for Slaney, New Ross Port, and Munster Blackwater (Figure 8D and Supplementary Figure 4). The model also showed the same behavior in the Lee (Supplementary Figure 4), however it’s worth noting that poor comparison here may be a result of the estuary being outside the northern stock area, and dependent on additional larval sources towards the shelf-break, as part of the West Coast of Scotland and Ireland stock (ICES 6.a, 7.b, 7.j).
3.3 Connectivity between spawning areas and nursery grounds
To assess the relative importance of source areas for different nursery grounds, connectivity matrices were constructed to show the number of particles that settle either within or outside their source area (Figure 9). While the connectivity of particles themselves show the influence of physical processes, the connectivity of fry supplied is also compared, to investigate the influence of spawning egg distributions assigned to each particle (Figure 10). These connectivity matrices do not take into account the different sizes of ICES areas, therefore some weighting may result from different numbers of particles released (or lengths of coastline within each available for settling). For further spatial detail, Supplementary Figures 5–12 show the source regions for each year of analysis, for either the whole coastal area or individual nursery grounds. Supplementary Figure 13 also shows example trajectories for particles that settled within nursery grounds.
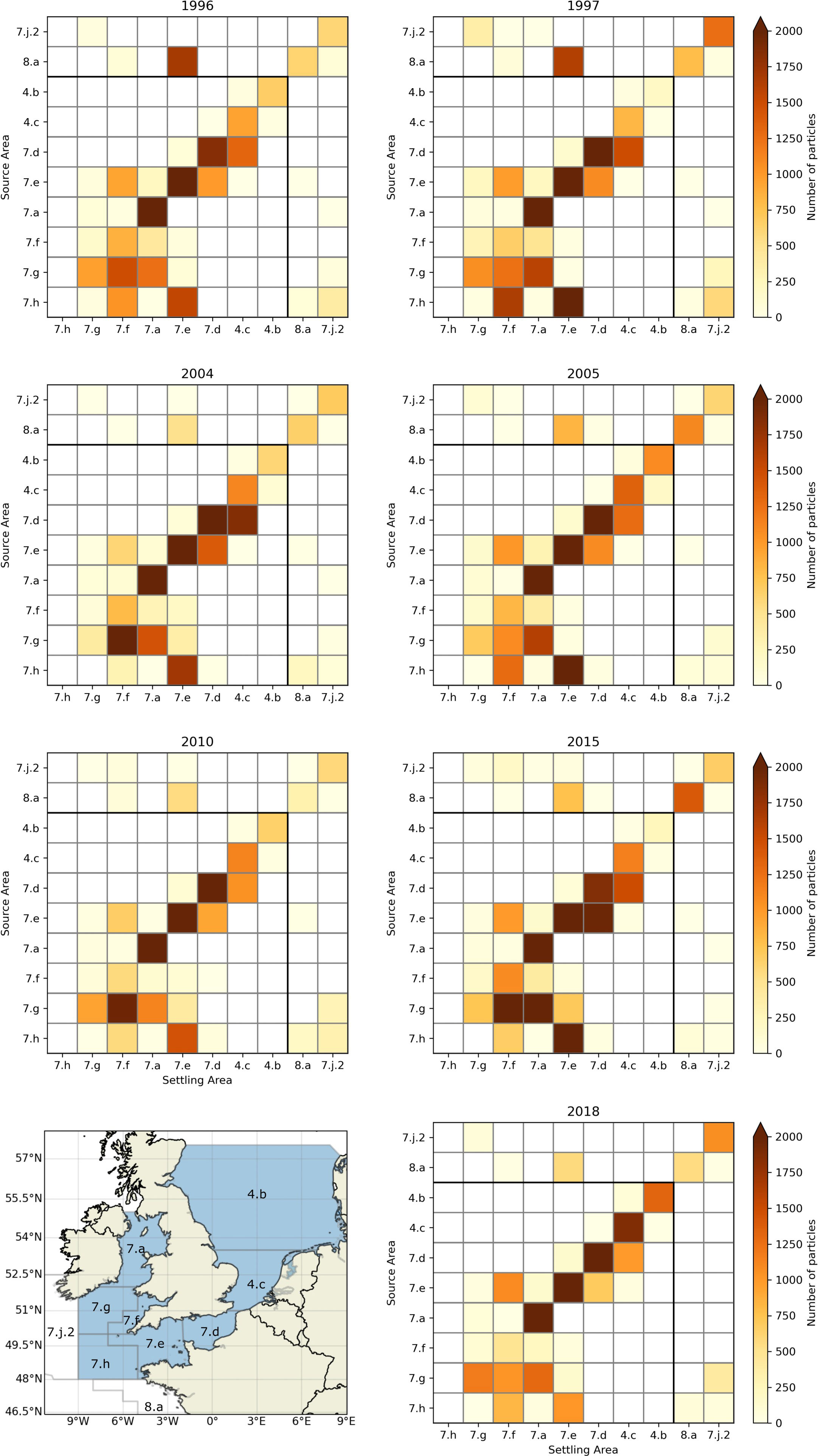
Figure 9 Connectivity matrix, showing number of particles sourced and settled within ICES areas for the northern stock (7.a, d-h, 4.b-c), bounded by black lines, and adjacent areas of interest (8.a and 7.j.2). Location of each area is shown in lower left panel. Values along the diagonal indicate spawning and settling within the same area. White boxes indicate zero particles present.
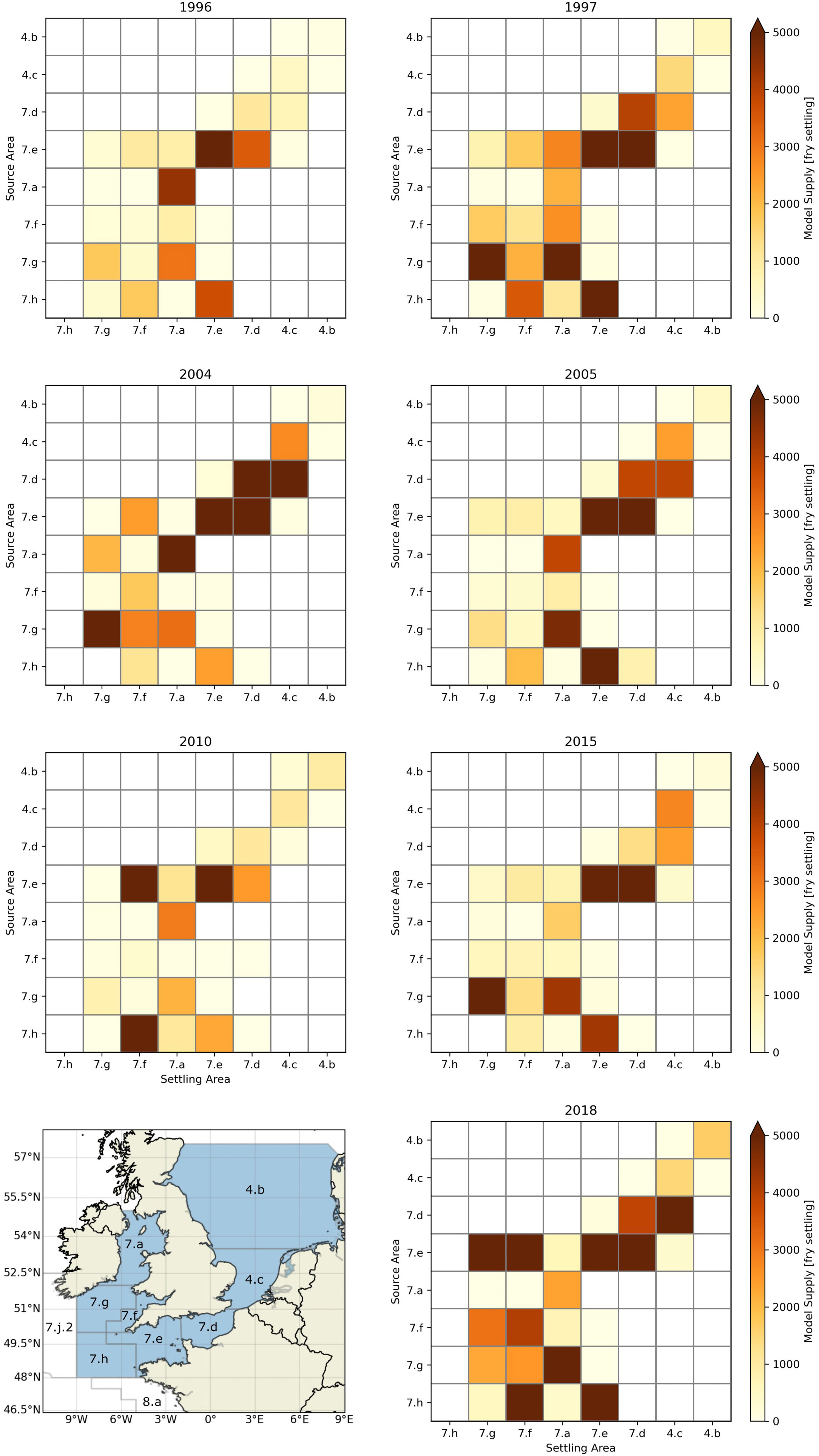
Figure 10 Connectivity matrix, showing model supply of fry sourced (as eggs, attributed to each particle) and settling within ICES areas for the northern stock (7.a, d-h, 4.b-c). Location of each area is shown in lower left panel (shaded in blue). Values along the diagonal indicate spawning and settling within the same area. White boxes indicate zero particles present.
The majority of particles that settled spawned in the same ICES area, but there was also significant transport between certain areas, with connections varying in strength between years (Figure 9). The southwest and Celtic Sea (7.e, 7.g, and 7.h) spawning areas tended to supply the Irish Sea (7.a) and the western Channel (7.e). Across the whole northern stock region, the western Channel (7.e) was the most important spawning location in terms of number of particles reaching coastal nursery areas each year (Figure 9 and Supplementary Figure 5). Particles spawned in the western Channel settled predominantly within this area, as well as the eastern Channel (7.d) and Bristol Channel (7.f), with weaker connections also to the wider Celtic and Irish Sea. A high number of particles also spawn further west, in the Celtic Sea (7.g and 7.h), but the importance of this region is seen to vary between years, for example with increased sources in 2015, and weaker in 2018 (Figure 9 and Supplementary Figure 5). The northern Celtic Sea (7.g) was an important source for adjacent areas, including the Bristol Channel (7.f) and Irish Sea (7.a), with weaker connections to the western Channel. Eastern Channel particles were also found to settle in the southern North Sea, with a consistently strong connection from 7.d to 4.c, but relatively little connection between the northern and southern North Sea (Figure 9). Outside the northern stock, the Biscay area (8.a) is a source for settling in the western Channel (7.e). For southwest Ireland (7.j.2, including the Lee estuary), there were sources from the Celtic Sea (7.g and 7.h). However, the larger sources were within this area itself, as well as further south along the shelf-break (outside the areas shown here).
Comparison between Figures 9, 10 then demonstrates the influence of egg distribution and mortality on connectivity for model supply of fry, in addition to the number of particles spawned and settled. There is greater connectivity between different areas when considering the resultant supply of fry (Figure 10), as opposed to just the number of particles (Figure 9). The western Channel (7.e) is again a consistently strong source for the northern stock region (Figure 10). This area supplies both itself and the eastern Channel, but there can also be increased supply to the Celtic and Irish Seas, with the relative influence varying interannually. For the Irish Sea (7.a), there is strong supply from within the area, but the dominant source for some years can be from further south (7.g). There is also strong connectivity between the Bristol Channel (7.f) and wider areas of the Celtic and Irish seas and western Channel (Figure 10). For this region, supply comes predominantly from outside rather than within the area itself. Fewer eggs are sourced within the North Sea, with most years showing a larger source for the southern North Sea (4.c) arriving from the eastern Channel (7.d; Figure 10). However, this connection does vary between years, with a particularly weak connection in 2010. This variability is less visible when considering just the number of particles (Figure 9), but is consistent with a lower recruitment in the Thames in 2010 (Figure 8).
3.4 Variability in model supply
When considering supply to individual nursery grounds, the dominance of different areas will vary through the simulation, depending on the time of spawning. As the duration of the pelagic phase is seen to decrease through the year (Figure 6), spawning later in the year will tend to support supply within the same area, due to shorter trajectories. Spawning earlier in the year will allow for longer trajectories between spawning and settlement areas, supporting greater connectivity between different areas. Spawning during February and March also coincides with the peak in egg availability for the stock region (Figure 2B). This dependence on spawning timing for both egg supply and connectivity can help to explain the regional dominance of the southwest for model supply, and the interannual variability seen in these simulations.
As spawning typically begins during February in the southwest (Celtic Sea and Western Channel), increased egg availability can account for the increased supply from this area during the simulations. Longer trajectories for particles spawning in February-March also account for increased connectivity from these areas to the wider domain. During years when spawning occurs earlier over a wider area, this can lead to increased supply and connectivity from those areas. Similarly, for years with reduced spawning in February-March, this likely accounts for reduced supply across the region.
For the year with the largest model supply, 2018, there was a significantly larger supply from the western Channel (Figures 7, 10). While the Celtic Sea was still a key source, warmer temperatures in February allowed for early spawning in the Channel, and therefore a large supply from this region (Supplementary Figure 13). As temperatures decrease into March, the higher recruitment predicted for this year is therefore likely due to the increased number of particles spawned in February (which then also settle within the northern stock region), rather than a sustained increase throughout the year.
The highest mean surface temperatures for February occurred in 2004 (Figure 2A), but this does not translate to higher supply across the region. Therefore, while increased temperatures can allow for early spawning, connectivity to nursery grounds is still a key factor in supply and therefore recruitment. A strong positive phase of the NAO occurred in 2018 (Figure 3), so stronger westerly winds could contribute to increased connection of particles spawned in the Celtic Sea towards the western Channel and Bristol Channel. Despite increased supply to the Channel, it is worth noting that the large increase in model recruitment for 2018 was not seen at the scale of individual estuaries, including the Fal and Solent (Figure 8). Further analysis showed that this increase occurs predominantly on the French rather than English side of the Channel, around the Cotentin Peninsula (not shown).
For the year with the largest recruitment shown in the stock assessment, 1997, the model does predict an increased supply, but appears to underestimate this increase when compared with other years (Figure 7). The model suggests a similar level of supply for both 1997 and 2004, whereas the ICES stock assessment suggests a significantly higher recruitment in 1997. Both these years have spawning in February, but the warmer conditions in 2004 allow for earlier spawning over a wider area, leading to increased supply and connectivity from areas outside the southwest. For 1997, spawning in February occurs predominantly within the Celtic Sea, and western Channel, accounting for increased supply to the Fal and Helford as well as southwest Ireland (Figure 8 and Supplementary Figure 4). During 1997, there was a positive phase of the NAO (Figure 3). While not as strong as 2018, the increased westerly winds during the spawning season would support increased supply from particles spawned further west (Beraud et al., 2018).
During 2004, there is increased supply particularly from within the Channel (in both 7.e and 7.d; Figure 10). The earlier spawning and increased egg supply within the eastern Channel allows for increased connectivity into the southern North Sea and Thames estuary (Supplementary Figure 13), with 2004 then showing the largest recruitment at this location (Figure 8B). For the Solent, while the stock assessment suggests the largest recruitment in 1997, the model suggests the largest supply in 2004 (Figure 8A), likely resulting from the earlier spawning within the Channel during this year. However, the resolution of this model may not allow for coastal circulation patterns around the Solent that may influence both connectivity and self-seeding within this area.
The lowest recruitment years, for both the ICES stock assessment and the model supply, occurred in 1996 and 2010. These years also showed reduced model supply on the scale of individual nursery grounds (e.g., Figure 8). As discussed in Section 3.1, both 1996 and 2010 were colder years, with negative phases of the NAO, leading to the lowest number of particles spawned or settled, respectively. For 1996, while the total number of particles settling is not significantly lower than other years, the delayed start to spawning across the region leads to a reduced egg supply available within the model. For 2010, reduced westerly winds, as well as the reduction in spawning and egg supply, likely contribute to reduced connectivity from spawning grounds in the Celtic Sea, as suggested by reduced sources from 7.g in Figure 10.
4 Discussion
The seven years chosen for simulation in this study covered variation in both the physical conditions (e.g., temperature and wind stress) and year class strengths. The model developed here was not expected to capture all the processes responsible for stock recruitment, but can still be useful for investigating the relative influence of physical or biological processes in determining interannual variability. Where the model does not reproduce observed variability, differences could be due to uncertainties in reproducing the physical environment (e.g., currents, temperature) or biological assumptions (e.g., spawning, behavior, mortality), as well as uncertainty in data collection or stock assessment.
It was clear that predictions from the model were able to capture some of the patterns both at the stock and individual estuary levels, but there were differences between the stock assessments or surveys and model predictions. For example, the model showed a similar pattern of interannual variability for most years in terms of recruitment over the whole northern stock region, apart from much larger recruitment in 2018. The model was not able to predict reduced settlement for 2004 in the Solent, or increased settlement for 2015 in Fal and Helford. However, the model generally performed better for the Thames, representing most patterns of interannual variability. Performance was generally poorer for the Irish nursery grounds. However, with only two years for comparison, it is not possible to draw any significant conclusions from this analysis as to what might cause this difference.
As the model appeared to perform better in some regions than others, this means that there were likely processes that were not captured or assumptions that were not valid across the scale of the model domain. For example, the connectivity matrices showed that particles spawned in the Biscay region could be a key source for settling in the western Channel. As these particles were sourced from outside the northern stock region, they were not included in the model supply here. This could potentially account for some of the difference between the model and observed recruitment in the Fal and Helford analysis. Supply to the Solent was also found to be smaller than other areas in this study. As the Solent was shown to be dependent predominantly on spawning sources within the Channel, the distribution of particles released in this study may have limited the potential supply from this region. Studies that consider higher densities of particles released from likely spawning areas in the Channel (e.g., Dambrine et al., 2021), could be useful to further assess interannual variability at this location.
Previous studies have shown that environmental variability partly explains the variability in successful settlement observed for sea bass (e.g., Beraud et al., 2018), sole (Lacroix et al., 2013), and other multi-annual studies (e.g., van der Molen et al., 2018; Barbut et al., 2019). Settlement success was shown to be driven by wind direction and temperature in previous models of the pelagic phase of sea bass (Beraud et al., 2018), and such processes were also shown to influence interannual variability here.
Lower recruitment was found in 1996 and 2010, for both the model and ICES recruitment. Both these years coincide with a negative phase of the NAO (Figure 3), which is typically associated with weaker westerly winds over the UK (e.g., Hurrell and Deser, 2010). The resulting impact on surface currents could mean a reduced likelihood of particles spawning in the Celtic Sea, or further in the southwest, reaching the coastal nursery grounds. Reduced temperatures also lead to a reduced number of particles spawned during these simulations. However, this study demonstrates that such comparisons with temperature do not necessarily apply to every year, for example with the warmest year (2004) having increased number of particles spawned within the model, but not the largest recruitment rates (in either the model or ICES stock assessments). In contrast, 2018 saw highest recruitment within the model, coinciding with a strong positive phase of the NAO. The increased westerly winds during both this year and the spawning season in 1997 could also support increased supply from particles spawned further west (consistent with results from Beraud et al., 2018). However, many different factors impact the resulting supply and connectivity to nursery grounds.
The physical environment nevertheless has a large impact on settlement through both the initial spawning criteria (temperature thresholds) and the transport of particles throughout the domain. The physical conditions were here set by the GETM hydrodynamic model (Burchard and Bolding, 2002). This model has been validated for use in previous studies (e.g., van der Molen et al., 2016; van der Molen et al., 2018) and has been shown to reproduce large scale climate and transport variability across the northwest shelf. However, it has a resolution of about 5 km, so may struggle to resolve transport in the coastal regions that are needed for analysis of settlement. To mitigate this, a wide region was chosen for comparison around each estuary, rather than expecting to resolve recruitment into individual estuaries. However, future work should investigate the impact of higher resolution models (e.g., Graham et al., 2018), as improving resolution around the coast may be a significant factor in determining variability in particle trajectories (e.g., Dauhajre et al., 2019; Bedington et al., 2022). For example, supply to the Solent may be increased through improved resolution of flow around the region. The larval behaviors used here were shown to best represent settlement in the Solent in previous studies (Beraud et al., 2018). However, it would be beneficial to reassess the sensitivity of the model to different behaviors under increased model resolution.
The influence of biological factors on settlement success relates to spawning (egg production, timing, and distribution), behavior of larval stages, and mortality. Previous models of sea bass have assumed constant spawning across the whole domain bounded only by temperature of 9–15 °CC in the period of February and April (Beraud et al., 2018). Here, spawning timing was extended to occur between February and July to allow for spawning in the North Sea (e.g., van Damme et al., 2011a; b). No time or temperature cut-off was applied to spawning locations, as this could lead to unusual dynamics in spatial patterns of spawning. However, egg production was assumed to be normally distributed, to generate more eggs in the center of the spawning distribution, and therefore reduced influence of spawning later in the year (Fahy et al., 2000). Egg production was split equally between all areas where particles were released at each time period. It is possible that the spawning period will vary between years due to temperature (Politikos et al., 2015) and latitudinal variations in day length (Vinagre et al., 2009). The current approach then represents an improvement from previous studies, but further work is needed to develop representations of timing, location, and magnitude of spawning across the region, with observations required to support modelling approaches.
Temperature-dependent growth rates and mortality were also updated here from previous work (Beraud et al., 2018). These again represent a significant improvement in terms of including the influence of environmental conditions. With the temperature-dependence on growth, and therefore duration of the pelagic stage, results here show the potential influence of timing and its relation to warming ocean temperatures, for connectivity between spawning and settling locations. However, it is likely that spatial and temporal variation in the early life stages would also include factors such as the presence of food (Cushing, 1996) and the abundance of pelagic predators (Hyder and Nash, 1998), both of which are currently omitted from this study. While very few observations exist with which to constrain such model behavior (e.g., spatial impacts of predation would be very difficult to predict), potential future development could look towards including an energy budget based on chlorophyll concentrations (e.g., Dambrine et al., 2020; Watson et al., 2022).
There was uncertainty in the nursery area surveys and stock assessment predictions of year-class strength, both of which affect the assessment of model performance. The stock assessment predictions of age class zero requires many assumptions and fitting of a complex model to sparse data sets, which results in uncertainty (ICES, 2020a). In addition, the stock assessment estimates of settlement are tuned to age class abundances from the Solent survey (ages two to four; ICES, 2020b), so are most likely to mimic patterns observed in the Solent. While data for comparison was limited, the model evaluation appears to show some improved representation of variability within the Thames as opposed to other regions. This may indicate that discreet regional variations in recruitment are not being captured, either within the stock assessment or the model analysis. Comparisons were made with indices of year class strength from the Thames and Solent, which were generated from multiple year of surveys. This was done to account for the efficiency of the survey at catching specific age classes that are driven by sampling location and gear efficiency (Solent - ages two to four; Thames - ages zero to three). As these surveys are based on older sea bass, it is possible that density-dependent processes occurring on the nursery ground (e.g., Beverton and Iles, 1992; Beverton, 1995; Geffen et al., 2011) may have dampened or altered any signs of variability from settlement patterns. Finally, the spatial and temporal extent of the time series is limited, so it would be beneficial to have longer time series and include data from more estuaries (e.g., Hyder et al., 2018b).
The definition of biological stocks of sea bass in the northeast Atlantic has proved elusive (ICES, 2012; ICES, 2018). Adult sea bass show strong site fidelity in the non-spawning period (Pawson et al., 2008) and then undertake annual migrations of widely differing distances depending on their location to reach water of appropriate temperature for spawning (Quayle et al., 2009; de Pontual et al., 2018). Given the uncertainty on timing and extent of spawning regions, the IBM scenarios explored here used a uniform distribution of spawning sites, rather than targeting release from specified areas. However, the results showed that known nursery areas around southwest England, southwest Wales and coastal sites in northwest Brittany and southeast Ireland, are likely to have high settlement rates with relatively short larval transport connections possible from the main spawning sites in the western English Channel and Celtic Sea. Spatial management measures that reduce targeting of spawning aggregations in specific areas, with the greatest connectivity to nursery areas, have the potential to have a disproportionate benefit on settlement of young sea bass. Ultimately, consideration of spatial management will need an understanding of where fish are spawning and migrating.
5 Conclusions
An individual-based model (IBM) has been extended to investigate factors affecting sea bass settlement on nursery grounds for the northern sea bass stock. The IBM included hydrodynamics to simulate the drift of eggs and larvae, temperature dependent development, mortality, and behavior affecting position in the water column. To assess the relative impact of environmental conditions on recruitment, simulations focused on years with both varying environmental conditions and year class strength.
Outputs from the model have been compared with monitoring from nursery areas in the UK and Ireland. This shows that the model can reproduce aspects of observed interannual variability across the northern stock region, and for the Thames estuary, but performed less well on the scale of individual estuaries for some other locations. Regional differences in performance could be a result of model performance in either the physical environment (e.g., currents, temperature) or biology (e.g., spawning, behavior, mortality), or uncertainty in data collection or stock assessment.
This model provides a useful tool for understanding the factors driving sea bass year-class strength, demonstrating spatial patterns in connectivity between spawning and nursery grounds. The region of influence shows varying extent for different nursery grounds. For example, sources for eastern Channel (including Solent estuary) come predominantly from a relatively local area, within either the eastern or western Channel. However, for the western Channel (including Fal & Helford) there is a wider range of source locations, from the wider Celtic Sea and further south into the Biscay stock region. Thames estuary also shows a large source from within the Channel as well as the southern North Sea. The connectivity between various spawning and settling areas have been shown to vary interannually, depending on the environmental conditions and timing of spawning across the region.
The model demonstrates the influence of physical processes, egg supply and mortality in determining the key source locations. Temperature and circulation patterns have a significant impact on connectivity within the region. Throughout the pelagic stages, growth is dependent on temperature within the model. When spawning occurs earlier in the year, lower temperatures lead to slower growth, longer trajectories, and therefore greater connectivity between different areas. This impact, in addition to the spawning distribution across the shelf (egg supply), has a large influence on interannual variability seen at different nursery grounds across the stock region.
Where differences do occur between the model predictions and stock assessments (or site surveys), this helps to highlight where either physical or biological processes may be unresolved, or require further investigation. For example, considering availability of food supply for growth, or impacts of predation on mortality. Further work is needed to both develop this model and improve monitoring of recruitment within nursery areas. However, spatial management measures that reduce targeting of spawning aggregations with the greatest connectivity to nursery areas have the potential to have a particular benefit on settlement of young sea bass.
Data availability statement
The raw data supporting the conclusions of this article will be made available by the authors, without undue reservation.
Author contributions
Manuscript preparation has been led by JG, with significant contributions also from JW and KH. JG, LG, KB, RN, WR and KH provided model development, experimental design and analysis of results. RB, MB, BC, DG, WR and CW provided survey data from nursery grounds for comparison, as well as guidance on interpretation of this data. All authors contributed to the article and approved the submitted version.
Funding
This work was funded by UK Department for Environment Food and Rural Affairs (DEFRA, FRD009).
Acknowledgments
The research presented in this paper was carried out on the High Performance Computing Cluster supported by the Research and Specialist Computing Support service at the University of East Anglia. We would like to thank all the citizen science volunteers that have participated in the annual surveys within the Fal and Helford estuaries. We would also like to thank Tiago Silva (Cefas) for his useful discussions and assistance in both the modelling work and review of this manuscript.
Conflict of interest
The authors declare that the research was conducted in the absence of any commercial or financial relationships that could be construed as a potential conflict of interest.
Publisher’s note
All claims expressed in this article are solely those of the authors and do not necessarily represent those of their affiliated organizations, or those of the publisher, the editors and the reviewers. Any product that may be evaluated in this article, or claim that may be made by its manufacturer, is not guaranteed or endorsed by the publisher.
Supplementary material
The Supplementary Material for this article can be found online at: https://www.frontiersin.org/articles/10.3389/fmars.2022.1046585/full#supplementary-material
References
Armstrong M., Brown A., Hargreaves J., Hyder K., Munday M., Proctor S., et al. (2013). Sea Angling 2012 – a Survey of Recreational Sea Angling Activity and Economic Value in England. Department for Food, Environment and Rural Affairs, London, United Kingdom.16 pp
Balmaseda M. A., Mogensen K., Weaver A. T. (2013). Evaluation of the ECMWF ocean reanalysis system ORAS4. Q. J. R. Meteorological Soc. 139, 1132–1161. doi: 10.1002/qj.2063
Barbut L., Groot Grego C., Delerue-Ricard S., Vandamme S., Volckaert F. A. M., Lacroix G. (2019). How larval traits of six flatfish species impact connectivity. Limnology Oceanography 64, 1150–1171. doi: 10.1002/lno.11104
Bedington M., García-García L. M., Sourisseau M., Ruiz-Villarreal M. (2022). Assessing the performance and application of operational Lagrangian transport HAB forecasting systems. Front. Mar. Sci. 9. doi: 10.3389/fmars.2022.749071
Hersbach H., Bell B., Berrisford P., Hirahara S., Horányi A., Muñoz-Sabater J., et al. (2020). The ERA5 global reanalysis. Q. J. R. Meteorological Soc. 146, 1999–2049. doi: 10.1002/qj.3803
Beraud C., van der Molen J., Armstrong M., Hunter E., Fonseca L., Hyder K. (2018). The influence of oceanographic conditions and larval behaviour on settlement success — the European sea bass dicentrarchus labrax (L.). ICES J. Mar. Sci. 75, 455–470. doi: 10.1093/icesjms/fsx195
Beverton R. J. H. (1995). Spatial limitation of population size — the concentration hypothesis. Netherlands J. Sea Res. 34, 1–6. doi: 10.1016/0077-7579(95)90010-1
Beverton R. J. H., Iles T. C. (1992). Mortality rates of O-group plaice (Pleuronectes platessa l.), dab (Limanda limanda l.) and turbot (Scophthalmus maximus l.) in European waters. III. density-dependence of mortality rates of O-group plaice and some demographic implications. Netherlands J. Sea Res. 29, 61–79. doi: 10.1016/0077-7579(92)90008-3
Bolle L. J., Dickey-Collas M., Van Beek J. K. L., Erftemeijer P. L. A., Witte J. I., van der Veer H. W., et al. (2009). Variability in transport of fish eggs and larvae. III. effects of hydrodynamics and larval behaviour on recruitment in plaice. Mar. Ecol. Prog. Ser. 390, 195–211. doi: 10.3354/meps08177
Borges R., Ben-Hamadou R., Chícharo M. A., Ré P., Gonçalves E. J. (2007). Horizontal spatial and temporal distribution patterns of nearshore larval fish assemblages at a temperate rocky shore. Estuarine, Coastal and Shelf Science 71, 412–428. doi: 10.1016/j.ecss.2006.08.020.
Brown M. (2017). Cefas solent bass survey in 2017, and update of bass recruitment index time series (Lowestoft, UK: Cefas Report), 7.
Brown M. (2018). Cefas solent bass survey in 2018, and update of bass recruitment index time series (Lowestoft, UK: Cefas Report), 9.
Burchard H., Bolding K. (2002). GETM, a General Estuarine Transport Model. EUR 20253 EN. European Commission. JRC23237
Cardoso J., Freitas V., Quilez I., Jouta J., Witte J., Van Der Veer H. (2015). The European sea bass dicentrarchus labrax in the Dutch wadden Sea: From visitor to resident species. J. Mar. Biol. Assoc. United Kingdom 95 (4), 839–850. doi: 10.1017/S0025315414001714
Carrillo M., Bromage N., Zanuy S., Serrano R., Prat F. (1989). The effect of modifications in photoperiod on spawning time, ovarian development and egg quality in the sea bass (Dicentrarchus labrax l.). Aquaculture 81, 351–365. doi: 10.1016/0044-8486(89)90159-2
Catherall H. (2020). Inter-annual variation in the abundance of juvenile European sea bass (Dicentrarchus labrax) in estuarine nursery habitats in Cornwall, UK, University of Plymouth, Plymouth
Cushing D. H. (1996). Towards a science of recruitment in fish populations (Luhne, Germany: Ecology institute), 175.
Dambrine C., Huret M., Woillex M., Pecquerie L., Allal H., Servili A., et al. (2020). Contribution of a bioenergetics model to investigate the growth and survival of European seabass in the bay of Biscay – English channel area. Ecol. Model. , 423, 109007. doi: 10.1016/j.ecolmodel.2020.109007
Dambrine C., Woillex M., Huret M., de Pontual H. (2021). Characterising essential fish habitat using spatio-temporal analysis of fishery data: A case study of the European seabass spawning areas. Fisheries Oceanography 30, 413–428. doi: 10.1111/fog.12527
Dauhajre D. P., McWilliams J. C., Renault L. (2019). Nearshore Lagrangian connectivity: Submesoscale influence and resolution sensitivity. J. Geophysical Res. 124, 5180–5204. doi: 10.1029/2019JC014943
DeAngelis D. L., Grimm V. (2014). Individual-based models in ecology after four decades. F1000Prime Rep. 6, 39. doi: 10.12703/P6-39
de Pontual H., Lalire M., Fablet R., Laspougeas C., Garren F., Martin S., et al. (2018). New insights into behavioural ecology of European seabass off the West coast of France: Implications at local and population scales. ICES J. Mar. Sci. 76, 501–515. doi: 10.1093/icesjms/fsy086
Devauchelle N., Coves D. (1988). The characteristics of sea bass (Dicentrarchus labrax) eggs: Description, biochemical composition and hatching performances. Aquat. Living Resour. 1, 223–230. doi: 10.1051/alr:1988022
El-Shebly A. A. (2009). Aquaculture potential of Sea bass (Dicentrarchus labrax) in brackish water fish farms in Egypt. J. Appl. Sci. Res. 5, 765–769.
Fahy E., Forrest N., Shaw U., Green P. (2000). Observations on the status of bass dicentrarchus labrax stocks in Ireland in the late 1990s. Irish Fisheries Investigations 5, 27.
Gaines S. D., Gaylord B., Gerber L. R., Hastings A., Kinlan B. (2007). Connecting places. the ecological consequences of dispersal in the sea. Oceanography 20, 90–99. doi: 10.5670/oceanog.2007.32
Geffen A. J., Nash R. D. M., Dau K., Harwood A. J. P. (2011). Sub-Cohort dynamics of O-group plaice, pleuronectes platessa l., in the northern Irish Sea: Settlement, growth and mortality. J. Exp. Mar. Biol. Ecol. 400, 108–119. doi: 10.1016/j.jembe.2011.02.030
Graham J. A., O’Dea E., Holt J., Polton J., Hewitt H. T., Furner R., et al. (2018). AMM15: A new high-resolution NEMO configuration for operational simulation of the European north-west shelf. Geoscientific Model. Dev. 11, 681–696. doi: 10.5194/gmd-11-681-2018
Holt J. T., Hughes S. L., Hopkins J., Wakelin S. L., Holliday N. P., Dye S. R., et al. (2012). Multi-decadal variability and trends in the temperature of the northwest European continental shelf: A model-data synthesis. Prog. Oceanography 106, 96–117. doi: 10.1016/j.pocean.2012.08.001
Houde E. D. (1989). Comparative growth, mortality, and energetics of marine fish larvae: temperature and implied latitudinal effects. Fishery Bull. 87, 471–495.
Hufnagl M., Peck M. A., Nash R. D. M., Pohlmann T., Rijnsdorp A. D. (2013). Changes in potential north Sea spawning grounds of plaice (Pleuronectes platessa l.) based on early life stage connectivity to nursery habitats. J. Sea Res. 84, 26–39. doi: 10.1016/j.seares.2012.10.007
Hurrell J. W., Deser C. (2010). North Atlantic climate variability: The role of the north Atlantic oscillation. J. Mar. Syst. 79(3–4), 231–244. doi: 10.1016/j.jmarsys.2009.11.002
Hyder K., Nash R. D. M. (1998). Variations in settlement pattern of Irish Sea plaice (Pleuronectes platessa l.) as determined from a simulation model. J. Sea Res. 40, 59–71. doi: 10.1016/S1385-1101(98)00018-5
Hyder K., Radford Z., Prellezo R., Weltersbach M. S., Lewin W. C., Zarauz L., et al. (2017). Research for PECH committee - marine recreational and semi-subsistence fishing - its value and its impact on fish stocks. European parliament, policy department for structural and cohesion policies, Brussels. 134. Brussels, Belgium: European Parliament, Policy Department for Structural and Cohesion Policies
Hyder K., Scougal C., Couce E., Fronkova L., Waugh A., Brown M., et al. (2018b). Presence of European sea bass (Dicentrarchus labrax) and other species in proposed bass nursery areas. (Lowestoft, UK: Cefas report), 120.
Hyder K., Weltersbach M. S., Armstrong M., Ferter K., Townhill B., Ahvonen A., et al. (2018a). Recreational sea fishing in Europe in a global context-participation rates, fishing effort, expenditure, and implications for monitoring and assessment. Fish Fisheries 19, 225–243. doi: 10.1111/faf.12251
ICES (2012). European Seabass in the northeast atlantic. ICES advice 2012, book 9 (Copenhagen Denmark: ICES), 14.
ICES (2014). Report of the working group on recreational fisheries surveys (WGRFS) (Copenhagen, Denmark: ICES), 62. ICES CM 2014/ACOM:37.
ICES (2018). Report of the benchmark workshop on seabass (WKBASS), 20-24 February 2017 and 21-23 February 2018 (Copenhagen, Denmark: ICES), 287. ICES CM 2018/ACOM:44.
ICES (2020a). Stock annex for Sea bass (Dicentrarchus labrax) in divisions 4.b–c, 7.a, and 7.d–h (central and southern north Sea, Irish Sea, English channel, Bristol channel, and celtic Sea) (Copenhagen, Denmark: ICES Stock Annex), 111.
ICES (2020b). ICES workshop on unavoidable survey effort reduction (WKUSER) (ICES Scientific Reports, 2:72: 92).
ICES (2021). “Seabass (Dicentrarchus labrax) in Divisions 4.b-c, 7.a, and 7.d-h (central and southern North Sea, Irish Sea, English Channel, Bristol Channel, and Celtic Sea)” doi: 10.17895/ices.advice.7733. In Report of the ICES Advisory Committee, 2021. ICES Advice 2021, bss.27.4bc7ad-h.
Jennings S. (1990). Population dynamics of larval and juvenile bass Dicentrarchus labrax (L.) (Swansea, UK: University of Wales, Swansea), 253.
Jennings S., Ellis J. R. (2015). “Bass (Moronidae),” in Fish atlas of the celtic Sea, north Sea, and Baltic Sea. Eds. Heessen H. J. L., Daan N., Ellis J. R. (Netherlands: Wageningen Academic Publishers / KNNV Publishing), 324–327. doi: 10.3920/978-90-8686-878-0
Jennings S., Pawson M. G. (1991). The development of bass, dicentrarchus labrax, eggs in relation to temperature. J. Mar. Biol. Assoc. United Kingdom 71, 107–116. doi: 10.1017/S0025315400037425
Johnson D. W., Katavic I. (1984). Mortality, growth and swim bladder stress syndrome of sea bass (Dicentrarchus labrax) larvae under varied environmental conditions. Aquaculture 38, 67–78. doi: 10.1016/0044-8486(84)90138-8
Lacroix G., Maes G. E., Bolle L. J., Volckaert F. A. M. (2013). Modelling dispersal dynamics of the early life stages of a marine flatfish (Solea solea l.). J. Sea Res. 84, 13–25. doi: 10.1016/j.seares.2012.07.010
Laffaille P., Lefeuvre J.-C., Schricke M.-T., Feunteun E. (2001). Feeding ecology of 0-group Sea bass, Dicentrarchus labrax, in salt marshes of mont saint Michel bay (France). Estuaries 24 (1), 116–125. doi: 10.2307/1352818
Leis J. M., Balma P., Ricoux R., Galzin R., de Perpignan U. (2012). Ontogeny of swimming ability in the European Sea bass, dicentrarchus labrax (L.) (Teleostei: Moronidae). Mar. Biol. Res. 8, 265–272. doi: 10.1080/17451000.2011.616898
Le Provost C., Lyard F., Molines J. M., Genco M. L., Rabilloud F. (1998). A hydrodynamic ocean tide model improved by assimilating a satellite altimeter-derived data set. J. Geophysical Res. 103, 5513. doi: 10.1029/97JC01733
López R., De Pontual H., Bertignac M., Mahévas S. (2015). What can exploratory modelling tell us about the ecobiology of European sea bass (Dicentrarchus labrax): A comprehensive overview. Aquatic Living Resources 28(2-4), 61–79. doi: 10.1051/alr/2015007
Ludsin S. A., De Vanna K. M., Smith R. E. H. (2014). Physical-biological coupling and the challenge of understanding fish recruitment in freshwater lakes. Canadian Journal of Fisheries and Aquatic Sciences. 71 (5), 775–794. doi: 10.1139/cjfas-2013-0512
Mayer I., Shackley S. E., Witthames P. R. (1990). Aspects of the reproductive biology of the bass, dicentrarchus labrax l. II. fecundity and pattern of oocyte development. J. Fish Biol. 36, 141–148. doi: 10.1111/j.1095-8649.1990.tb05590.x
Methot R. D., Wetzel C. R. (2013). Stock synthesis: A biological and statistical framework for fish stock assessment and fishery management. Fisheries Res. 142, 86–99. doi: 10.1016/j.fishres.2012.10.012
Mogensen K., Balmaseda M. A, Weaver A.T. (2012). The NEMOVAR ocean data assimilation system as implemented in the ECMWF ocean analysis for system 4. ECMWF. Tech. Memoranda 668, 61. doi: 10.21957/x5y9yrtm
Mylonas C. C., Anezaki L., Divanach P., Zanuy S., Piferrer F., Ron B., et al. (2005). Influence of rearing temperature during the larval and nursery periods on growth and sex differentiation in two Mediterranean strains of dicentrarchus labrax. J. Fish Biol. 67, 652–668. doi: 10.1111/j.0022-1112.2005.00766.x
NOAA Climate Prediction Center (2022) Monthly mean NAO index since January 1950. Available at: https://www.cpc.ncep.noaa.gov/products/precip/CWlink/pna/nao.shtml (Accessed 1st February 2022).
Ospina-Alvarez A., Parada C., Palomera I. (2012). Vertical migration effects on the dispersion and recruitment of European anchovy larvae: From spawning to nursery areas. Ecol. Model. 231, 65–79. doi: 10.1016/j.ecolmodel.2012.02.001
Pawson M. G., Brown M., Leballeur J., Pickett G. D. (2008). Will philopatry in sea bass, dicentrarchus labrax, facilitate the use of catch-restricted areas for management of recreational fisheries? Fisheries Res. 93, 240–243. doi: 10.1016/j.fishres.2008.03.002
Pepin P. (1991). Effect of temperature and size on development, mortality, and survival rates of the pelagic early life history stages of marine fish. Canadian. J. Fisheries Aquat. Sci. 48(503), 518. doi: 10.1139/f91-065
Pickett G. D., Brown M., Harley B., Dunn M. R. (2002). Surveying fish populations in the solent and adjacent harbours using the CEFAS bass trawl. Cefas science series technical report, Vol. 118. 16. (Lowestoft, UK: Cefas Report)
Pickett G. D., Pawson M. G. (1994). Sea Bass. biology, exploitation and conservation (London UK: Chapman & Hall), 342.
Politikos D., Somarakis S., Tsiaras K. P., Giannoulaki M., Petihakis G., Machias A., et al. (2015). Simulating anchovy’s full life cycle in the northern Aegean Sea (eastern mediterranean): A coupled hydro-biogeochemical-IBM model. Prog. Oceanography 138, 399–416. doi: 10.1016/j.pocean.2014.09.002
Pope E. C., Ellis R. P., Scolamacchia M., Scolding J. W. S., Keay A., Chingombe P., et al. (2014). European Sea bass, dicentrarchus labrax, in a changing ocean. Biogeosciences 11, 2519–2530. doi: 10.5194/bg-11-2519-2014
Quayle V. A., Righton D., Hetherington S., Pickett G. (2009). Observations of the behaviour of European Sea bass (Dicentrarchus labrax) in the north sea. reviews: Methods and technologies in fish biology and fisheries (Netherlands, Dordrecht: Springer), 104–119.
Radford Z., Hyder K., Zarauz L., Mugerza E., Ferter K., Prellezo R., et al. (2018). The impact of marine recreational fishing on key fish stocks in European waters. PloS One 13, e0201666. doi: 10.1371/journal.pone.0201666
Savina M., Lacroix G., Ruddick K. (2010). Modelling the transport of common sole larvae in the southern north Sea: Influence of hydrodynamics and larval vertical movements. J. Mar. Syst. 81, 86–98. doi: 10.1016/j.jmarsys.2009.12.008
Schlaefer J. A., Wolanski E., Lambrechts J., Kingsford M. J. (2018). Wind Conditions on the Great Barrier Reef Influenced the Recruitment of Snapper (Lutjanus carponotatus). Front. Mar. Sci. 5, 193. doi: 10.3389/fmars.2018.00193
Stige L. C., Rogers L. A., Neuheimer A. B., Hunsicker M. E., Yaragina N. A., Ottersen G., et al. (2019). Density- and size-dependent mortality in fish early life stages. Fish Fisheries 20, 962–976. doi: 10.1111/faf.12391
Stocks J. R., Davis S., Anderson M. J., Asmus M. W., Cheshire K. J. M., van der Meulen D. E., et al. (2021). Fish and flows: Abiotic drivers influence the recruitment response of a freshwater fish community throughout a regulated lotic system of the Murray-Darling Basin, Australia. Aquatic Conservation: Marine and Freshwater Ecosystems 31(11), 3228–3247. doi: 10.1002/aqc.3636
Subbey S., Devine J. A., Schaarschmidt U., Nash R. D. M. (2014). Modelling and forecasting stock–recruitment: Current and future perspectives. ICES J. Mar. Sci. 71, 2307–2322. doi: 10.1093/icesjms/fsu148
Tiessen M. C. H., Fernard L., Gerkema T., van der Molen J., Ruardij P., van der Veer H. W. (2014). Numerical modelling of physical processes governing larval transport in the southern north Sea. Ocean Sci. 10, 357–376. doi: 10.5194/os-10-357-2014
van Damme C. J. G., Hoek R., Beare D., Bolle L. J., Bakker C., van Barneveld E., et al. (2011a). Shortlist master plan wind monitoring fish eggs and larvae in the southern north Sea: Final report part a. report number C098/11 (Wageningen UR, Netherlands: IMARES), 56.
van Damme C. J. G., Hoek R., Beare D., Bolle L. J., Bakker C., van Barneveld E., et al. (2011b). Shortlist master plan wind monitoring fish eggs and larvae in the southern north Sea: Final report part b. report number C098/11 (Wageningen UR, Netherlands: IMARES), 377.
van der Molen J., García-García L. M., Whomersley P., Callaway A., Posen P. E., Hyder K. (2018). Connectivity of larval stages of sedentary marine communities between hard substrates and offshore structures in the north Sea. Sci. Rep. 8, 14772. doi: 10.1038/s41598-018-32912-2
van der Molen J., Rogers S. I., Ellis J. R., Fox C. J., McCloghrie P. (2007). Dispersal patterns of the eggs and larvae of spring-spawning fish in the Irish Sea, UK. J. Sea Res. 58, 313–330. doi: 10.1016/j.seares.2007.07.003
van der Molen J., Ruardij P., Greenwood N. (2016). Potential environmental impact of tidal energy extraction in the pentland firth at large spatial scales: results of a biogeochemical model. Biogeosciences 13, 2593–2609. doi: 10.5194/bg-13-2593-2016
van der Veer H., Berghahn R., Miller J., Rijnsdorp A. (2000). Recruitment in flatfish, with special emphasis on north Atlantic species: Progress made by the flatfish symposia. ICES J. Mar. Sci. 57, 202–215. doi: 10.1006/jmsc.1999.0523
van der Veer H. W., Ruardij P., Van den Berg A. J., Ridderinkhof H. (1998). Impact of interannual variability in hydrodynamic circulation on egg and larval transport of plaice pleuronectes platessa l. in the southern north Sea. J. Sea Res. 39, 29–40. doi: 10.1016/S1385-1101(97)00008-7
Vinagre C., Ferreira T., Matos L., Costa M. J., Cabral H. N. (2009). Latitudinal gradients in growth and spawning of sea bass, dicentrarchus labrax, and their relationship with temperature and photoperiod. Estuarine Coast. Shelf Sci. 81, 375–380. doi: 10.1016/j.ecss.2008.11.015
Watson J. W., Boyd R., Dutta R., Vasdekis G., Walker N. D., Roy S., et al. (2022). Incorporating environmental variability in a spatially-explicit individual-based model of European sea bass. Ecol. Model. 466, 109878. doi: 10.1016/j.ecolmodel.2022.109878
Keywords: European sea bass (Dicentrarchus labrax), European Northwest shelf, pelagic life stages, particle tracking, larval connectivity, interannual variability, Individual Based Model (IBM)
Citation: Graham JA, Watson JW, García García LM, Bradley K, Bradley R, Brown M, Ciotti BJ, Goodwin D, Nash RDM, Roche WK, Wogerbauer C and Hyder K (2023) Pelagic connectivity of European sea bass between spawning and nursery grounds. Front. Mar. Sci. 9:1046585. doi: 10.3389/fmars.2022.1046585
Received: 16 September 2022; Accepted: 12 December 2022;
Published: 19 January 2023.
Edited by:
Francois Bastardie, Technical University of Denmark, DenmarkReviewed by:
Vânia Baptista, University of Algarve, PortugalEsther Goldstein, National Oceanic and Atmospheric Administration, United States
Copyright © 2023 Graham, Watson, García García, Bradley, Bradley, Brown, Ciotti, Goodwin, Nash, Roche, Wogerbauer and Hyder. This is an open-access article distributed under the terms of the Creative Commons Attribution License (CC BY). The use, distribution or reproduction in other forums is permitted, provided the original author(s) and the copyright owner(s) are credited and that the original publication in this journal is cited, in accordance with accepted academic practice. No use, distribution or reproduction is permitted which does not comply with these terms.
*Correspondence: Jennifer A. Graham, jennifer.graham@cefas.gov.uk