- 1Department of Engineering Cybernetics, Norwegian University of Science and Technology, Trondheim, Norway
- 2Fisheries and new Biomarine Industry, SINTEF Ocean, Trondheim, Norway
- 3Department of Biological Sciences, University of Bergen, Bergen, Norway
- 4Norwegian Institute for Nature Research (NINA), Bergen, Norway
- 5GAGN AS consulting, Ålesund, Norway
There is increasing interest in utilizing fishers’ knowledge to better understand the marine environment, given the spatial extent and temporal resolution of fishing vessel operations. Furthermore, fishers’ knowledge is part of the best available information needed for sustainable harvesting of stocks, marine spatial planning and large-scale monitoring of fishing activity. However, there are difficulties with integrating such information into advisory processes. Data is often not systematically collected in a structured manner and there are issues around sharing of information within the industry, and between industry and research partners. Decision support systems for fishing planning and routing can integrate relevant information in a systematic way, which both incentivizes vessels to share information beneficial to their operations and capture time sensitive big datasets for marine research. The project Fishguider has been developing such a web-based decision support tool since 2019, together with partners in the Norwegian fishing fleet. The objectives of the project are twofold: 1) To provide a tool which provides relevant model and observation data to skippers, thus supporting sustainable fishing activity. 2) To foster bidirectional information flow between research and fishing activity by transfer of salient knowledge (both experiential and data-driven), thereby supporting knowledge creation for research and advisory processes. Here we provide a conceptual framework of the tool, along with current status and developments, while outlining specific challenges faced. We also present experiential input from fishers’ regarding what they consider important sources of information when actively fishing, and how this has guided the development of the tool. We also explore potential benefits of utilizing such experiential knowledge generally. Moreover, we detail how such collaborations between industry and research may rapidly produce extensive, structured datasets for research and input into management of stocks. Ultimately, we suggest that such decision support services will motivate fishing vessels to collect and share data, while the available data will foster increased research, improving the decision support tool itself and consequently knowledge of the oceans, its fish stocks and fishing activities.
1. Introduction
There is a global movement towards better understanding and utilization of data and experience of fishers in order to inform research activity and management decisions (Johannes et al., 2008; Stephenson et al., 2016; Dyrset et al., 2022). This is due to an increasing awareness that it is advantageous to consider fishers’ knowledge, as the quantity of information available to modern fleets is vast given the temporal and spatial extent of global fisheries operations, which is estimated at four times the spatial extent of agriculture (Kroodsma et al., 2018). Such knowledge includes the experiences of fishers themselves and information processing systems onboard, and is considered part of the best available information (Stephenson et al., 2016). This information can be used in stock assessment, marine spatial planning and mapping species abundance and distribution (Holm and Soma, 2016). Modern applications to real-time monitoring of vessel tracks can screen for illegal fishing activity and map the global footprint of effort (de Souza et al., 2016; Kroodsma et al., 2018). Remote sensing of environmental variables may be a cost-effective method of supporting fishing activities (Santos, 2000). The recent paper from Jones et al. (2022) demonstrates how high resolution data from the US reference fleet has contributed to abundance indices for several stocks, while footprints of fishing vessels can inform planning of offshore wind projects. A similar Norwegian reference fleet program found that gathering species and age composition data from fishing vessels is a cost-effective method of sampling and producing CPUE time series for cod, haddock and redfish (Hjelle et al., 2021).
Given the multitude benefits of using fishers’ knowledge to inform policy, it begs the question why it’s underutilized? For catch data, there is the issue of bias in samples for density estimates, as catch logs exclusively record instances of fishing activity, neglecting areas not targeted by fishers, which biases predictions of species distributions (Karp et al., 2022). Also, given the unsystematic way much of fishers’ knowledge is handled, it is often neglected (Hind, 2015). This means that although the quantity of information is high, the quality is highly variable and potentially skewed. It’s challenging to filter from individual knowledge claims to scientific input that is legitimate and salient for decision-making (Brattland, 2013; Röckmann et al., 2015). Regardless, there is the charge that biologists don’t take fishers ecological knowledge seriously, where such information can avert collapses of spawning stocks (Johannes et al., 2008).
In addition to the benefits to decision makers of incorporating fishers’ knowledge, there are increasingly clear incentives for fishers to contribute in meaningful ways. The historical trajectory of the Norwegian fishing industry has been to long-term sustainable harvesting. For example, advances in fish finding equipment, with the uptake of echosounders and sonar, has improved vertical and horizontal profiling of fish and led to more offshore and targeted exploitation of stocks (Nakken, 2008; Gordon and Hannesson, 2015). Advances in mechanical winches for trawling gear reduced the labour involved in hauling nets, and introduction of non-rotting synthetic fibres made nets pressure resistant, increasing catch efficiency (Hamre and Nakken, 1971; Jennings et al., 2001). A modern purse seiner makes particularly effective use of the listed advances, and is relatively fuel efficient, using approximately 0.1kg of fuel per kilo of fish (Schau et al., 2009). In addition to technological developments, structural changes to the fleet, from introduction of tradeable quotas, decommissioning schemes and general movement of labour away from the industry, have reduced overcapacity and increased operating margins (Standal and Asche, 2018; Fisheries Directorate, 2021). However, such technological advances are a double-edged sword. The cumulative impact of technological innovation, especially mechanical hauling, led to increased catch rates and the collapse of the North-East Atlantic herring stocks in the 1970s (Fiksen and Slotte, 2002; Gordon and Hannesson, 2015; Standal and Asche, 2018). Therefore, prudent management of stocks is essential alongside such developments.
Such modernization of the industry means vessels spend long periods at sea with advanced equipment such as echo-sounders and sonars, covering vast geographical areas, and thus, have access to large quantities of information. To utilize such information effectively, collaboration between researchers and fishers is important. Increased knowledge of the environment fishers operate within can contribute to achieving long-term objectives. In this work, the first objective is to supply fishers with information that reduces time spent searching for fishing grounds, while simultaneously reducing fuel use of vessels. The second objective is to build a system that automatically captures and stores data gathered while vessels are at sea.
Decision support systems (DSS) are tools that can integrate knowledge sources to achieve these objectives. Formulations of DSS include: manufacturing DSS that help deliver products and services to customers, clinical DSS used to improve healthcare delivery using clinical knowledge and patient information, and organizational DSS used to inform decisions on complex activities within a large organization (e.g. governmental body), through integration of knowledge such as norms and roles in the organization (Jacob and Pirkul, 1992; Sala et al., 2019; Sutton et al., 2020).
In the maritime context, the major application of DSS tools have been in the shipping industry, aimed mainly towards optimizing speed and routes of vessels and avoiding collisions between vessels (Lazarowska, 2014; Bal Beşikçi et al., 2016; Lee et al., 2018). As described in Gilman et al. (2022), forms of shipping DSS can be applied to support fishing route optimization. In this article we will refer to such computer based tools in the context of supporting stakeholder decisions in the fishing industry specifically. In this context, DSS that have been applied to support management decisions in spatial allocation of effort and bycatch management (Truong et al., 2005; Granado et al., 2021). Moreover, they have been used to provide model estimates on presence and size of fishing banks directly to fishers, thus reducing time and fuel spent on fishing operations (Iglesias et al., 2007). There are a wide range of actors who may benefit from such tools, from managers to ship owners and skippers. As researchers, it’s important that research knowledge is integrated with the needs of industry to facilitate uptake of tools Röckmann et al. (2015). In this way, DSS can provide a vital link between research and the fishing industry, where two way information transfer can garner interest in results of research as directed towards their operation, while at the same time encouraging more engagement between parties.
The Fishguider project began in 2019 as a science-industry research collaboration aimed at both reducing fuel use and search time of the Norwegian fishing fleet and fostering two-way information transfer between fishers and researchers. Importantly, this was an industry directed project, where an umbrella organization of motivated fishing companies was founded to partially fund work activities, under the name of the North Atlantic Institute for Sustainable Fishing (NAIS). In consultation between NAIS and researchers, a DSS tool was conceived of as an appropriate method to co-create knowledge necessary to achieve long-term objectives of industry. Such co-creation of knowledge between research and industry is an effective way of building mutual trust between researchers and fishers (Holm and Soma, 2016). Additionally, the delivery of such a software solution is well placed for systematically capturing and sharing data between participants, and supporting management decisions through production of salient and legitimate knowledge. A key component of the project is the participation of fishers in the pilot program to ascertain the feasibility of the DSS tool. There is evidence suggesting that participation can increase in science-industry collaborations if results are perceived to be positive for industry (Calderwood et al., 2021).
In this article, we present the conceptual framework for the DSS tool being developed as part of Fishguider and it’s current status, reflecting on similarities to other DSS tools mentioned above. The capacity to systematically capture and share information through a user interface is explored and we discuss how data-driven input and experiential knowledge inform the development of this interface. In addition, a questionnaire is presented, detailing fishers’ experiences of which factors are most relevant when considering when and where to fish. Finally, we consider challenges in interpreting, capturing and sharing knowledge through this project.
2. Literature on DSS tools in the fisheries context
DSS tools are described as computer-based programs that integrate diverse information sources in order to support complex decision-making processes (Truong et al., 2005; Bal Beşikçi et al., 2016; Granado et al., 2021; Gilman et al., 2022). In a DSS, computer output virtually represents the real fisheries system, reducing uncertainties that constrain decision making (Truong et al., 2005). Decisions that require support systems usually address problems where there are competing interest groups, such as fishing effort allocation. Therefore, human participation and intervention are essential in the process (Bal Beşikçi et al., 2016; Gilman et al., 2022). In this way, DSS plays a supporting role in decision-making, rather than an executive role. Regardless, there are a multitude of areas where they can give insight, as shown in Table 1. The two broad applications are within fisheries management and industry-related optimization. A diverse range of inputs are used, from data-driven input such as remote sensing and vessel speed to knowledge based input from interdisciplinary collaboration and stakeholder engagements.
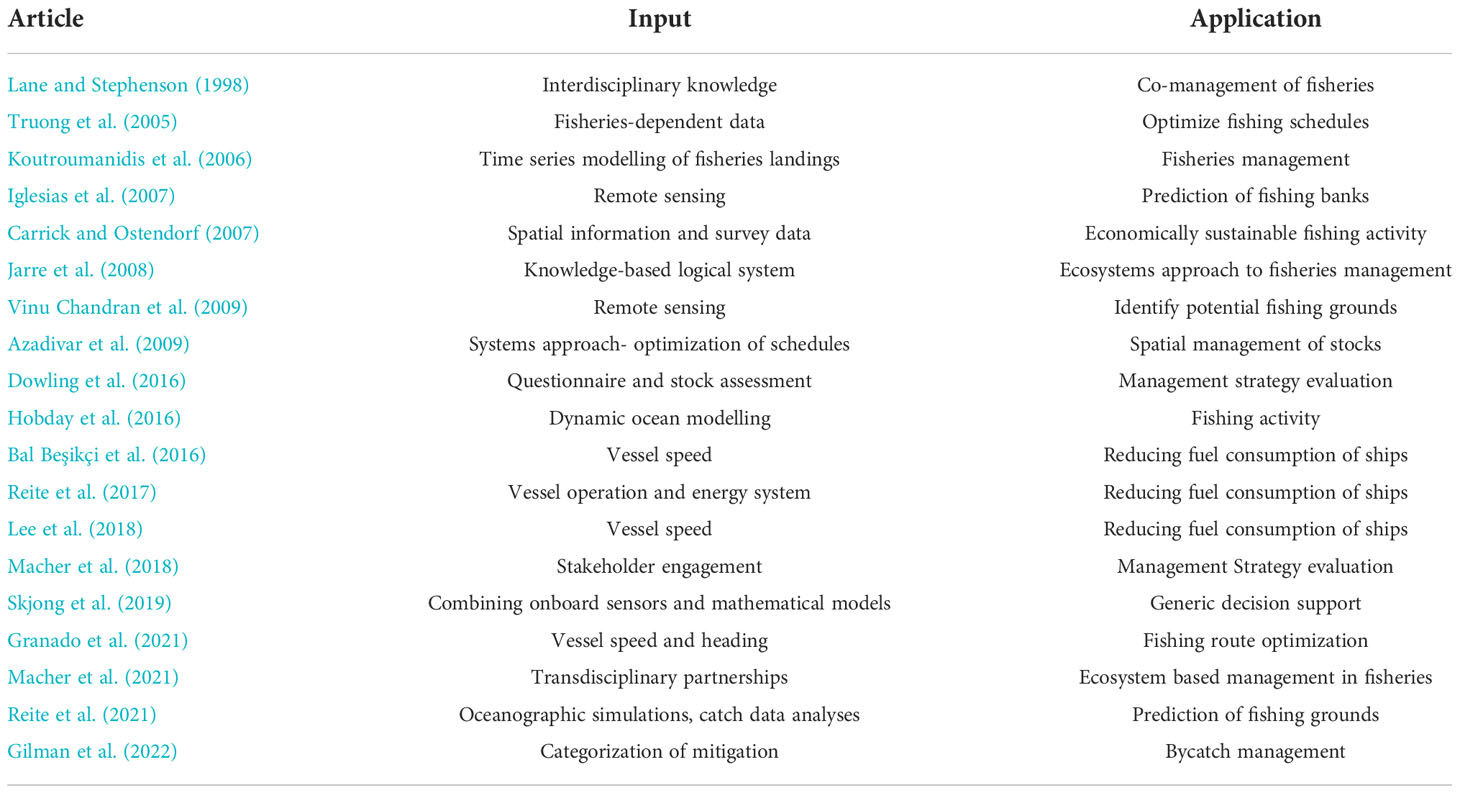
Table 1 A selection of literature sorted chronologically on decision support in fisheries and shipping, describing the input used and the area of application.
Fishers face many practical issues when searching for fishing grounds, such as uncertainties in weather conditions, quality and location of fish, and prices and costs being variable. In the face of these issues, they must make concrete decisions on how to organise fishing activities. The scales of fishing activity decisions can be separated based on duration into three categories: strategic, tactical and operational decisions. Strategic decisions (weeks to months to years) refers to long-term planning of location and timing of fishing based on expectations of both the market and fishing possibilities (Reite et al., 2021). Tactical decisions (hours to days) are decisions about which fishing grounds to visit, the number of grounds to visit and where and when to return to port to land catches (Granado et al., 2021). Long-term tactical decisions may involve, for example, planning of whether to target herring or mackerel based on market prices (Reite et al., 2021). Short-term tactical decisions include motion planning of fishing vessels and controlling position and course of vessels relative to schools of fish (Haugen and Imsland, 2019; Haugen and Kyllingstad, 2021; Kyllingstad et al., 2021). Operational decisions (near real-time) involve immediate control of the vessel, such as speed and heading of fishing vessels between waypoints defined through tactical decisions Granado et al. (2021). Assuming waypoints are clearly defined, operational decisions can be informed through routing optimization, which has been addressed using DSS tools in the shipping industry to reduce fuel consumption (Bal Beşikçi et al., 2016; Granado et al., 2021). However, defining strategic and tactical decisions is a complex task for fishing vessels searching for fish, given the uncertainties in stock distribution and abundance at these scales and therefore, the Fishguider DSS tool is designed to support these decisions.
DSS tools are designed with of a number of interconnected components. Fundamentally, they require high quality data sources, where data can be obtained from remote sensing of environmental variables such as sea surface temperature, weather archive data, information systems on board such as positional data, as well as manual input from ship operators (Iglesias et al., 2007; Bal Beşikçi et al., 2016; Lee et al., 2018). Data can also be gathered from national or global databases, such as historical catch data, where the data is directly relevant to fishers operations and can improve their situational awareness. This data is uploaded to a database, where information is compiled and can be queried directly by the user. There is also typically a model solver which takes input and produces estimates of relevant information. Often the problems are complex and require pattern detection through machine learning and data mining algorithms, where artificial neural networks have been particularly effective (Bal Beşikçi et al., 2016).
This information is mapped to a user interface, where the user (fisher or manager) may query databases directly (Bal Beşikçi et al., 2016). User interfaces are typically tuned to the experience and requirements of the user. Information is often displayed in interactive layers which compile the most salient knowledge for decision making. For example, (Granado et al., 2021) describes decision layers developed for fishers to display routes based on an optimization algorithm which allows for interaction with the user. In addition, explicit costs associated with decisions may be displayed, such as in management decisions where there are multiple conflicting objectives such as safety and economic viability (Gilman et al., 2022).
3. Case study: The fishguider DSS tool
3.1. Description
The Fishguider DSS tool was requested by fishing companies working together in an umbrella organization called North Atlantic Institute for Sustainable Fishing (NAIS), who spend much time and fuel searching for fishing grounds, while lacking systemized knowledge to assist in making informed decisions on where and when to fish. The system desired should aid in communication of information between fishing vessels and allow them to both contribute and ascertain relevant information to minimize uncertainties when operating. Importantly, the fishers involved are motivated to collaborate with researchers and understand the ecosystem they operate within. The interested parties wish to build a knowledge base to ensure present and future sustainable harvesting. Specifically, a DSS system may aid in handling decisions made in light of the complexities of climate change, the potential shifts in distributions of fish stocks and instabilities in fuel prices. Improving the situational awareness through knowledge co-creation will help the fishers meet these demands, specifically aiding with strategic and tactical decision making.
The DSS is currently designed as a proof of concept which can be refined and scaled for industrial use. The scaling of the system relies in part on connecting more vessels to the project. Therefore a pilot programme of vessels is underway, where they are now utilizing the system during the fishing season. Participants are from a variety of fisheries, targeting both demersal and pelagic species, with different gears, quotas and sizes of vessels. At the time of writing, there are 19 vessels involved with the pilot project. Of those 6 are classed as coastal vessels, 3 large coastal, 7 ocean-going trawlers and 3 ocean-going purse seiner. In addition, 16 of these vessels are above 21m in length. These classes determine the quotas and areas where the vessel operates. For example, ocean-going vessels cannot operate within fjords without special permission. For pelagic species, the fishers are most active from October to December when herring overwinter near the coast and in Northern fjords and then again January to March during the spawning migration and spawning for the herring, while mackerel are mainly targeted during their wintering cycle in southern Norway from September to December when the market prices are highest, although there is inter-annual variability (Varpe et al., 2005; Nøttestad et al., 2016; Ølmheim, 2021; Reite et al., 2021). The following sections describe the DSS tool according to its data sources, model-based inputs and the user interface (Figure 1).
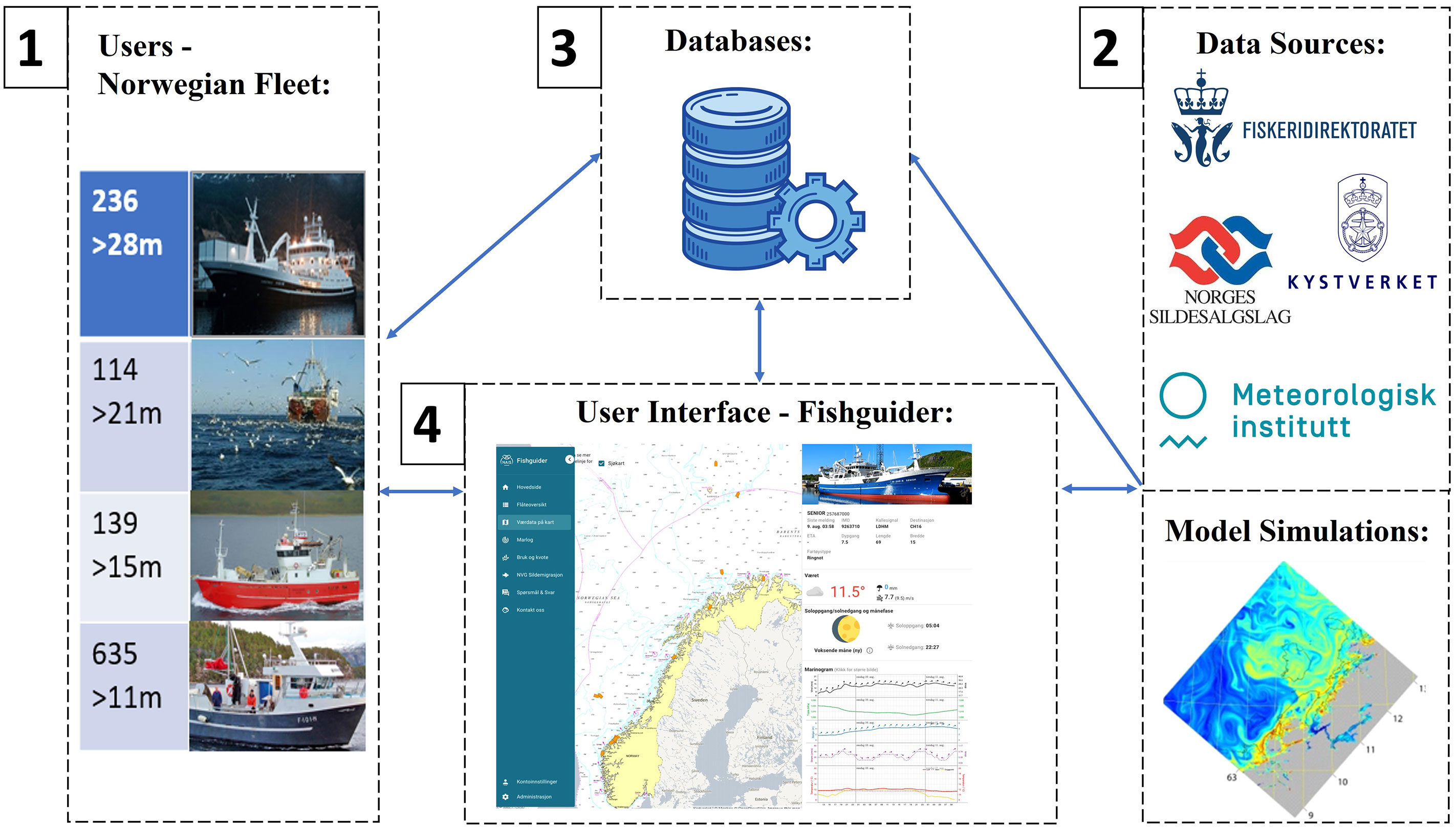
Figure 1 Conceptual model of the Fishguider tool: 1) The Norwegian Fleet of vessels over 11m in length who may contribute information, both from experiential knowledge and from information systems onboard vessels (such as satellite and acoustic data). 2) Fishers can access external information, such as meteorological forecasts, real-time auction prices and relevant model output. 3) The data sources are collected in databases developed in conjunction with the project. 4) The final user interface is a web portal that displays relevant layers to the skipper. The design of the interface is largely driven by the requests of participating fishers.
3.2. Knowledge sources for DSS
3.2.1. Experiential
In an effort to build a tool that is useful for the fishers, a survey in the form of a questionnaire was designed and 13 of the skippers in NAIS responded. The questionnaire was conducted by phone in 2020 in Norwegian and answers were translated into English. An online or paper-based solution were not possible due to logistic challenges with communication. The skippers surveyed are the most actively involved in the project. They target both pelagic and demersal species, but we learned from project meetings that they perceive the most immediate use of the tool in targeting herring and mackerel. Therefore, the questionnaire focused on these two species.
There were two categories of questions asked. The first related to the importance of a variety of factors in deciding when and where the new fishing season should begin (Figure 2). This set of questions corresponded to strategic decisions. The second related to to the importance of factors during the season (Figure 3). This set of questions corresponded to tactical decisions. The survey was designed to gauge the information fishers in NAIS consider important, regardless of availability, in making decisions to choose fishing grounds. Questions were chosen based on wide-ranging project meetings between researchers and active fishers in NAIS. Fishers expressed the importance of a full ecosystem understanding in decision-making, from plankton to whales, and therefore, questions of this nature were included.
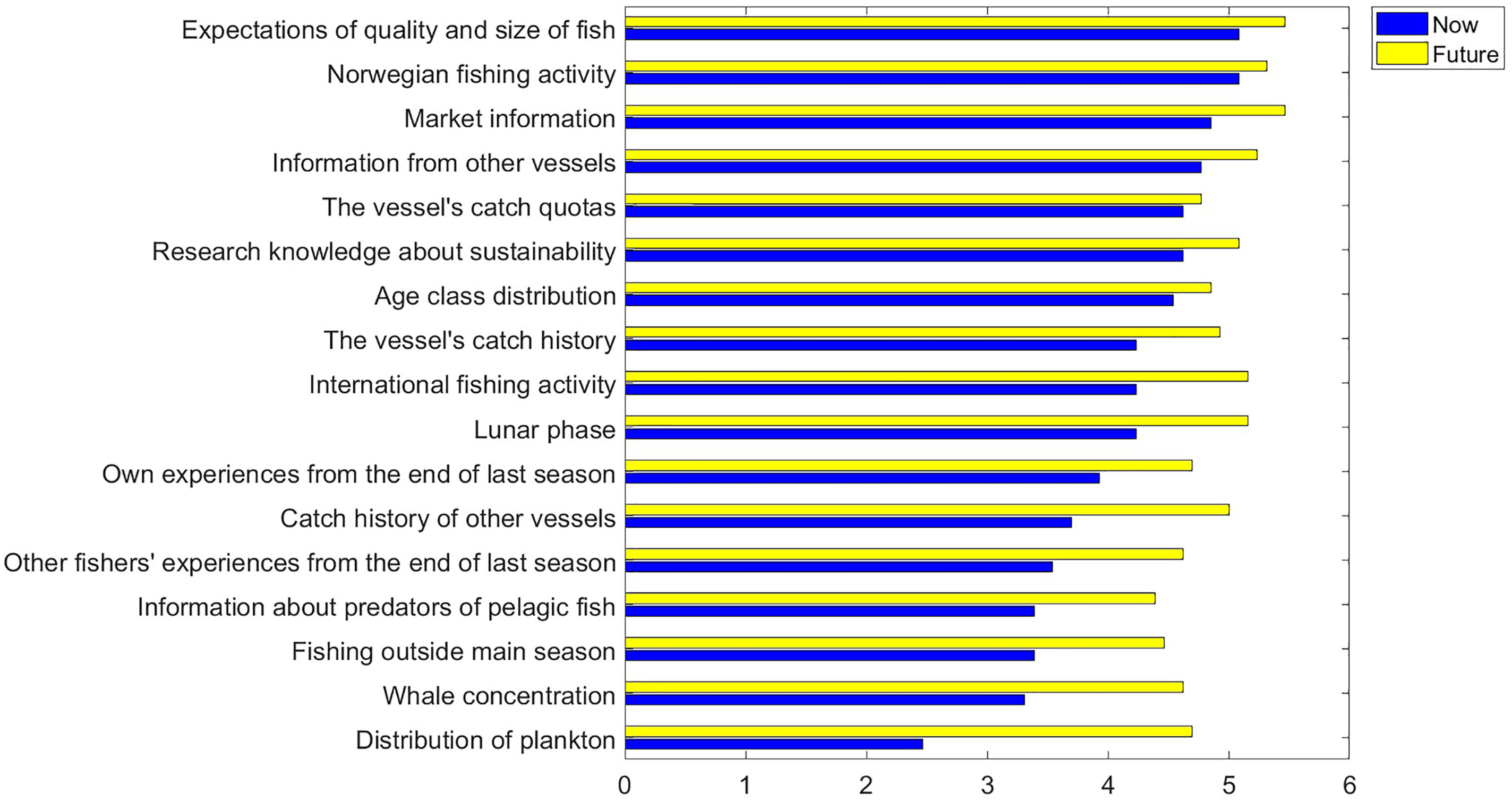
Figure 2 Response to Question: How important are the following factors when deciding when and where the new fishing season should begin? The blue bars indicate how important they are now, while the yellow bars signal the importance of better information in the future. The bars display the mean value (N = 13).
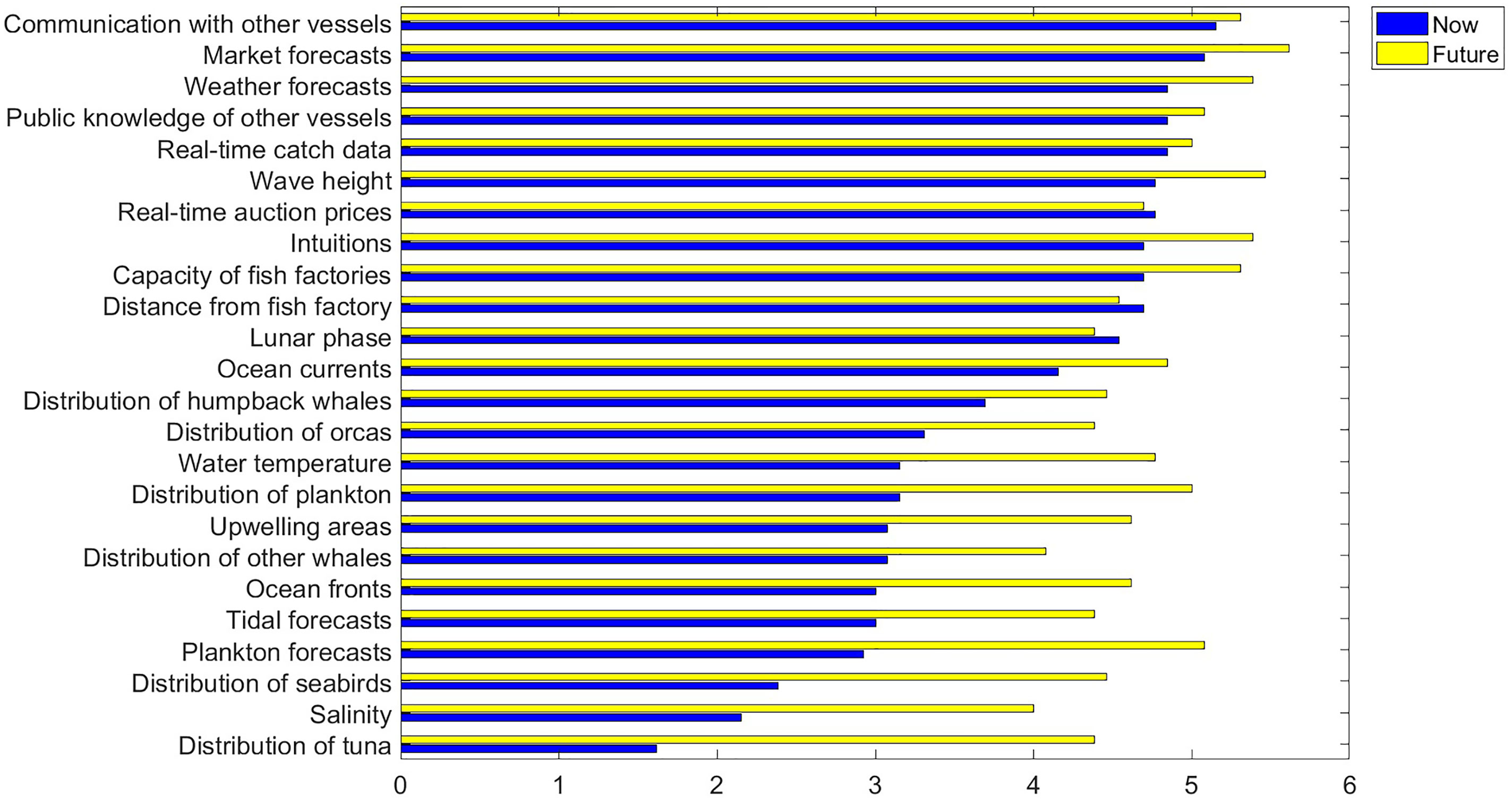
Figure 3 Response to Question: How important are the following factors for choosing a fishing spot during the season? The blue bars indicate how important they are now, while the yellow bars signal the importance of better information in the future. The bars display the mean value (N=13).
Respondents were asked to rate the importance of items from both categories on an evaluative rating scale from 1 to 6, 6 being the highest value. Items were categorized based on their importance to fishers now and their potential importance in the future. The questionnaires displayed are the results for questions related to the targeting of herring. The mean values for the 13 respondents are displayed in the horizontal barplots (Figure 2 and 3). Given the sample surveyed, we don’t assume this is completely representative of the fishing industry as a whole, especially given the number of large vessels involved. Additionally, social factors such as business structures and working rhythm may influence strategic and tactical decisions (Schadeberg et al., 2021). Nevertheless, the survey offered relevant input to the design of the support tool in order to make it relevant for industry implementation, which was our main objective. A table of questionnaire responses for both herring and mackerel can be found in the appendix, with additional informal commentary from respondents included (Appendix A).
Generally, practical considerations such as the vessel’s quota, catch history and Norwegian fishing activity are important now and are considered important in the future in strategic decision making (Figure 2). Ecological information such as whale concentration, plankton forecasts and information about predators are not strategically utilized now, but attaining such information is perceived as useful in the future.
Similarly, when asked what factors are important in tactical decision making, plankton forecasts and distribution of seabirds and whales are not utilized now, but such information may be valuable in the future (Figure 3). It must be noted that the perspective of fishers on the data they use today is likely based on their ongoing assessment of the quality of data available, while the question of future utility is made under the assumption that high quality data may be readily available. In real-time fishing activity, communication with other vessels, market forecasts and weather forecasts are seen as the most important factors to consider.
The questionnaire, complimented by meetings with fishers, has informed the development of the web portal over the past two years. Many of the information sources fishers deem important are publicly available and a major part of the work is compiling these in one place. Currently, communication between fishers is being facilitated through messaging options in the portal, weather forecasts are attained from the meteorological institute, such as wind speeds and swell at the vessels’ location, and oceanographic data (particularly ocean currents), plankton and fish distribution data from model simulations are included. In addition, based on project meetings, it was discovered that fishers deemed the lunar phase an indicator of the timing of the initiation of herring spawning migrations. This factor was thus included in the questionnaire, and has been integrated into the support tool (Figure 4). Finally, the catch history of vessels, market information such as auction prices and vessel quotas, and the trajectories of individual vessels are now being connected to the portal. In the next sections, we explore the major knowledge sources available for the DSS tool.
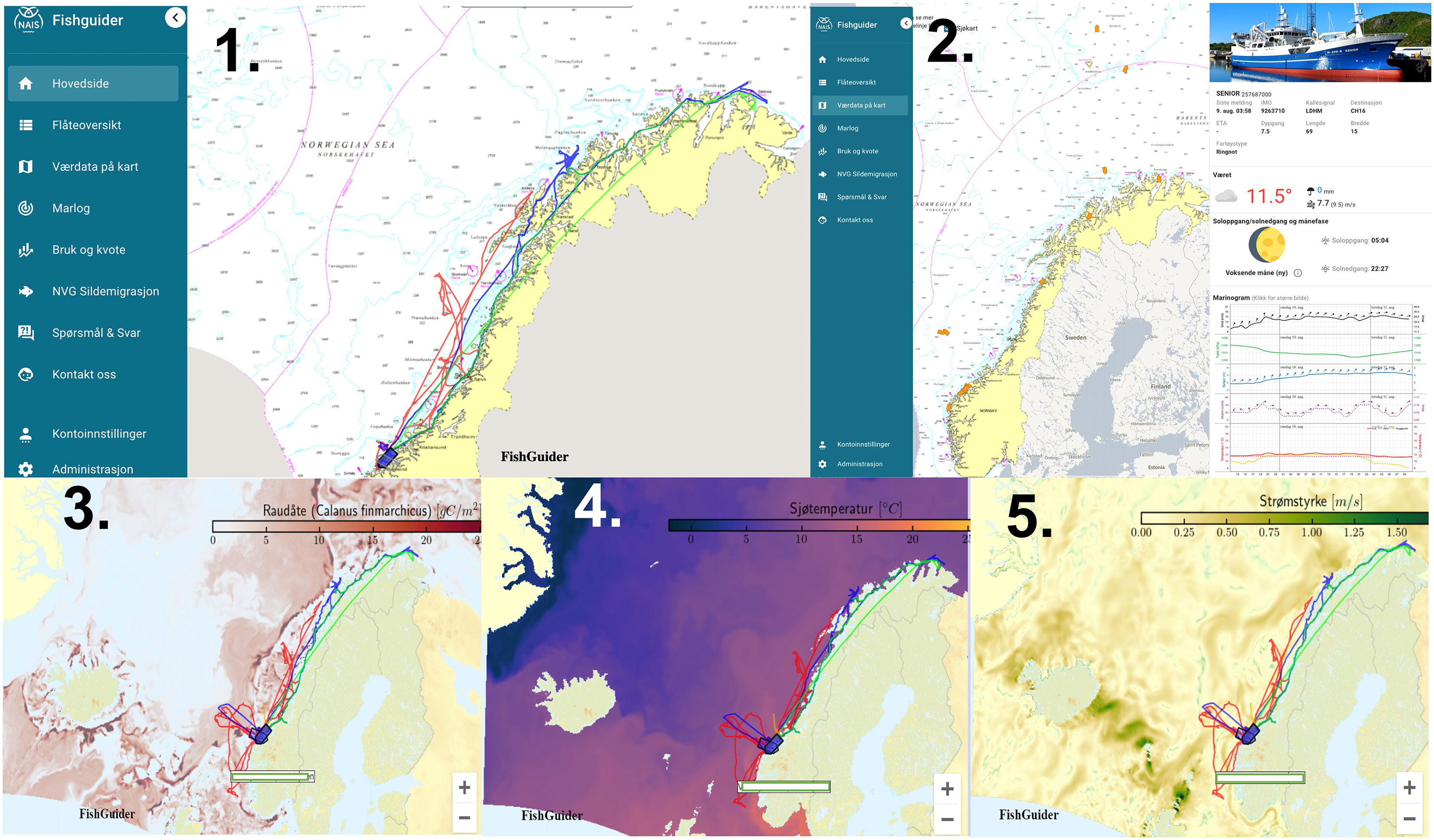
Figure 4 A selection of output layers in the Fishguider portal, with Norwegian text: 1) Homepage with tabs for various layers centered on the Norwegian Sea. The red, blue and green lines are the tracks of individual vessels based on GPS coordinates. 2) Weather data and forecast from the position of one of the NAIS vessels based on meterological institute data. 3) Modelled Calanus finmarchicus distribution and abundance in grams of carbon per meter squared 4) Sea surface temperature output on a single day in degrees Celsius. 5) Horizontal components of current velocities in meters per second.
3.2.2. Data-driven
In addition to the fishers’experiences as obtained from the questionnaire, data is being gathered from several sources. The catch and activity reporting (ERS) and Vessel Monitoring System (VMS) are electronic reporting systems for fisheries data provided by the Norwegian Directorate of Fisheries (https://www.fiskeridir.no/English/Fisheries/Electronic-Reporting-Systems). Whereas ERS data includes vessel positions for fishing activities such as ‘in catch operation’, ‘pumping’ and ‘steaming’ to and from harbour, VMS data includes more detailed position data for all types of vessels with a length of 15 meters and above, logged at minimum one hour sampling frequency. The ERS logs replaced physical logs of catches in 2005 where there is a principle of reporting all Norwegian fishing activity, with widespread adoption (https://www.fiskeridir.no/English/Fisheries/Electronic-Reporting-Systems).
Automatic identification systems data (AIS) are, like VMS data, detailed position data for all types of vessels. There are many sources available such as Marine Traffic (https://www.marinetraffic.com/en/ais/) and The Norwegian Coastal Administration (https://www.kystverket.no/en/navigation-and-monitoring/ais/access-to-ais-data/), which provides AIS data in real time, either as raw data or online traffic information displayed in charts. AIS data is primarily used by coastal administration to avoid shipping collisions and locating a given vessel quickly in an emergency situation. Given the time sensitivity needed to avoid collisions or respond to emergencies, data is transmitted approximately every 10 seconds. ERS, VMS and AIS data are complementary data for monitoring fishing vessel movements which are being integrated in the DSS tool.
The Norwegian Fishers’ Sales Organization for Pelagic Fish (or Norges Sildesalslag in Norwegian: https://www.sildelaget.no/) is a fisher-owned sales organization that trades fish through an electronic auction. Fresh catches are offered to buyers while vessels are at sea, after the catch is registered over phone, and a commission price on the value of each catch is payed by the fisher (0.65 percent of each catch). Real-time auction prices are highly relevant to direct decisions on fishing, as reflected in the questionnaire responses, and will be integrated in the DSS tool (Figure 3).
Finally, vessels in the Norwegian fleet continuously gather observations using sonar and echosounder, but this data is usually discarded. A future version of Fishguider is expected to collect, aggregate and make decision support based on a fleet supplying such observations, and this work has begun.
3.2.3. Model simulations
Model simulations of conditions alongshore and offshore the Norwegian coast are currently being integrated into the DSS tool. Ocean model estimates of sea surface temperature, current and salinity are loaded from a model called SINMOD (Slagstad and McClimans, 2005). The output from this model has a 4km resolution and is centered on the Norwegian Sea. The model has a time resolution of 10 seconds. An eulerian model of the copepod species Calanus finmarchicus has been coupled to the SINMOD model, where plankton distributions are mainly driven by atmospheric fields including wind, air temperature and precipitation, river discharge, and bottom topography (bathymetry) (Wassmann et al., 2006). This species is a key prey item for many pelagic stocks in the Norwegian Sea. In addition, a model of the spawning migration of herring is coupled to SINMOD, where information on current, temperature and bathymetry are used to drive the fish motion towards their spawning areas (Kelly et al., 2022). All model ouputs are loaded to the web portal in near real-time.
Minimizing the gap between the true system and the model estimates depends on integrating as many vessels into the project. Capturing of data by vessels included in the project can strengthen input to these models, which improves their predictive capacity. An Ensemble Kalman Filter setup has been designed to allow assimilation of observation data into the migration model (Kelly et al., submitted). In the long-term this can develop larger datasets for studying effects of climate change, understanding life cycles and migrations of fish, and providing input into stock assessment.
3.3. Databases
Both national and international databases are being integrated into the web portal. FishGuider is currently being integrated with FiskInfo (https://fhf-prod.azurewebsites.net), Kystverkets NAIS service (https://nais.kystverket.no/), BarentsWatch (https://www.barentswatch.no/en), Marine Traffic (https://www.marinetraffic.com/en/ais/), Ocean Resource Watch (https://resourcewatch.org/dashboards/ocean-watch) and other complementary information tools.
3.4. User interface
The user interface of Fishguider web portal provides layers of information tailored to the needs of the fisher. As mentioned, it has been curated according to the experiences of fishers, considering important features when planning fishing operations and in real-time (Figures 2, 3). The web portal has a fleet overview tab, which details the vessels involved in the project and their specifications. There are also messaging possibilities and contact points for the fishers, if they have any difficulties with usability of the tool. The tracks that are displayed in the interface are based on GPS transmitters installed onboard, which are being trialed (Figure 4). This interface has facilitated information flow between fishers and researchers, where fishers now have access to spatiotemporal data on current, temperature, nitrate, plankton and herring from research-based models developed, while researchers have access to observations from vessels which provide input to the models (Figure 4). This input can allow improvement of the accuracy of the model predictions, while also correcting errors in model output.
4. Takeaways
4.1. Knowledge co-creation
Such collaborations between industry and research may rapidly produce extensive, structured datasets for research and input into management of stocks. Involving enough fishers and/or vessels improves collaboration and will give more access to quality information. In general, the more vessels involved, the better. The vessels involved are representative of a subset of the coastal and oceanic fleet in Norway. The project results are presented at project meetings and industry conferences, such as Norfishing and The Midsund Conference (Midsundkonferansen). In this way, both participants and industry at large can provide input and feedback on the design of the tool. Additionally, as fishing companies are partly funding the project, key results are communicated to these larger audiences. Fishers seem interested to participate and share data on condition that the platform will yield useful input in guiding operations.
Sharing of information between researchers and fishers is key to achieving this. Given that the fishers themselves are interested in this work, they have been quite open to sharing vessel data. Due to competition between fishers there is a potential scepticism in sharing information, but this issue has become less important the last decade, as individual vessel quotas are the main limiting factor, less vessels participate and there is more transparency because of open data sources (AIS, VMS, ERS). By limiting the spread of information to those who contribute, this should not be a big issue for this project in the future. Furthermore, engagement with the DSS tool will develop the user experience and friendliness of the application, which in turn will encourage more participants to join the project.
The questionnaire results give insight into what fishers deem important factors in strategic and tactical decision making. However, the small sample size and evaluative scale used means we cannot gauge the prioritization of factors by fishers. Further work should consider ranking factors and matching available knowledge based on this. It is important to avoid the inclusion of all desired sources in the DSS tool at the cost of adequate user experience.
4.2. Research-based inputs
Collaboration between fishers and scientists has provided direct results that are salient for decisions regarding fishing activity. For example, fishing routes can be optimized to meet strategic, tactical and operational decisions (Granado et al., 2021). Spatially and temporally explicit maps of fish distribution are particularly useful for planning operations, and can be obtained through analysing remote sensing data (Iglesias et al., 2007). In our work, a migration model has been implemented to estimate the development of the herring spawning migration (Kelly et al., 2022). Lifting the modelling of fish migration, implementation and visualisation of the model to a level that gives the fishers useful additional information and promotes more active engagement with the tool.
Coordinating the various ideas and requirements from the diverse set of fishers is challenging, as there can be variability in the problems they face, depending on the target stock, vessel size and fuel consumption. Additionally, when asked about the utility of various factors in the future, almost all were considered useful in some way, especially research output which is not capitalized upon today (Figure 2 and Figure 3). Therefore, continuous dialogue and soliciting of feedback from fishers is central to qualifying the true importance of information for decision making. Understanding the behaviour of fishing vessels themselves is also important and progress has been made on categorizing activities automatically based on position, speed and heading of vessels (de Souza et al., 2016).
4.3. Advisory processes
Finally, DSS tools can contribute to advisory processes by reducing uncertainties involved in executive decision making. For example, offshore wind farms are planned along the coast of Norway, and the potential conflicts with industry may be anticipated and captured through understanding the movements of fishing vessels. Firstly, the formalized knowledge of fishers is relevant input into decision-making on management of stocks throughout the season. Fine grain information about individual vessels can improve CPUE indices, an important input for stock assessments (Campbell, 2004). Secondly, the legality of fishing activity can be monitored through automatic detection of vessel activities (Arasteh et al., 2020). Automatic monitoring of activity from data they can contribute, may be more desirable and less invasive than physical monitoring through observers or drones. Thirdly, collaboration between researchers, fishers and managers can improve decision-making on these issues. Of crucial importance is that the knowledge base is considered legitimate to decision-makers (Röckmann et al., 2015).
5. Conclusion
The Fishguider project has developed a functional pilot of a DSS tool which is being used for testing and development of the interface, databases and models, while simultaneously helping connect more vessels to the project. Currently, only small number of companies are involved, but the entire Norwegian fleet of fishing vessels are seen as potential participants. Fishguider was setup to primarily facilitate environmentally sustainable fishing activity by reducing search time and fuel consumption of fishing vessels. As the project has evolved, fuel prices have risen, and concerns about climate change have grown, making DSS tools like this one even more crucial. The knowledge being created should therefore be central to fishing activity, marine research and management going forward.
Data availability statement
The original contributions presented in the study are included in the article/Supplementary Material. Further inquiries can be directed to the corresponding author.
Ethics statement
Ethical review and approval was not required for the study on human participants in accordance with the local legislation and institutional requirements. Written informed consent from the participants was not required to participate in this study in accordance with the national legislation and the institutional requirements.
Author contributions
CK: Wrote the manuscript and created figures and tables. FM: Provided details about model and observations inputs. KR: Provided information about the fishing industry and decision support literature. JK: Proofread the manuscript and provided additional references. ØV: Proofread the manuscript, provided feedback and added discussion points. AB. Provided details and images of the Fishguider tool. MA: Gave detailed feedback. All authors were involved editing a shared version of the manuscript in overleaf. All authors contributed to the article and approved the submitted version.
Funding
The work is part of the FishGuider project, which is funded by the project participants and the Norwegian Research Council (project number 296321).
Acknowledgments
We greatly acknowledge the support, input and feedback from the project participants: NTNU, SINTEF, NAIS and UiB.
Conflict of interest
Author AB is employed by GAGN AS consulting.
The remaining authors declare that the research was conducted in the absence of any commercial or financial relationships that could be construed as a potential conflict of interest.
Publisher’s note
All claims expressed in this article are solely those of the authors and do not necessarily represent those of their affiliated organizations, or those of the publisher, the editors and the reviewers. Any product that may be evaluated in this article, or claim that may be made by its manufacturer, is not guaranteed or endorsed by the publisher.
Supplementary material
The Supplementary Material for this article can be found online at: https://www.frontiersin.org/articles/10.3389/fmars.2022.1051879/full#supplementary-material
References
Arasteh S., Tayebi M. A., Zohrevand Z., Glässer U., Shahir A. Y., Saeedi P., et al. (2020). “Fishing vessels activity detection from longitudinal AIS data,” in Proceedings of the 28th international conference on advances in geographic information systems (Seattle WA USA: ACM), 347–356. doi: 10.1145/3397536.3422267
Azadivar F., Truong T., Jiao Y. (2009). A decision support system for fisheries management using operations research and systems science approach. Expert Syst. Appl. 36, 2971–2978. doi: 10.1016/j.eswa.2008.01.080
Bal Beşikçi E., Arslan O., Turan O., Ölçer A. (2016). An artificial neural network based decision support system for energy efficient ship operations. Comput. Operations. Res. 66, 393–401. doi: 10.1016/j.cor.2015.04.004
Brattland C. (2013). Proving fishers right. effects of the integration of experience-based knowledge in ecosystem-based management. Acta Borealia. 30, 39–59. doi: 10.1080/08003831.2013.768053
Calderwood J., Marshall C. T., Haflinger K., Alfaro-Shigueto J., Mangel J. C., Reid D. G. (2021). An evaluation of information sharing schemes to identify what motivates fishers to share catch information. ICES. J. Mar. Sci., 1–22. doi: 10.1093/icesjms/fsab252
Campbell R. A. (2004). CPUE standardisation and the construction of indices of stock abundance in a spatially varying fishery using general linear models. Fish. Res. 70, 209–227. doi: 10.1016/j.fishres.2004.08.026
Carrick N., Ostendorf B. (2007). Development of a spatial decision support system (DSS) for the Spencer gulf penaeid prawn fishery, south Australia. Environ. Model. Software. 22, 137–148. doi: 10.1016/j.envsoft.2005.07.025
de Souza E. N., Boerder K., Matwin S., Worm B. (2016). Improving fishing pattern detection from satellite AIS using data mining and machine learning. PloS One 11, e0158248. doi: 10.1371/journal.pone.0158248
Dowling N., Wilson J., Rudd M., Babcock E., Caillaux M., Cope J., et al. (2016). “FishPath: A decision support system for assessing and managing data- and capacity- limited fisheries,” in Assessing and managing data-limited fish stocks. Eds. Quinn T. II, Armstrong J., Baker M., Heifetz J., Witherell D. (Alaska, United States: Alaska Sea Grant, University of Alaska Fairbansk). doi: 10.4027/amdlfs.2016.03
Dyrset G., Margaryan L., Stensland S. (2022). Local knowledge, social identity and conflicts around traditional marine salmon fisheries – a case from mid-Norway. Fish. Manage. Ecol. 29, 131–142. doi: 10.1111/fme.12522
Fiksen Ø., Slotte A. (2002). Stock-environment recruitment models for norwegian spring spawning herring (clupea harengus). Can. J. Fish. Aquat. Sci. 59, 211–217. doi: 10.1139/f02-002
Fisheries Directorate (2021). Profitability survey of the Norwegian fishing fleet 2019 (Norway: Fisheries Directorate).
Gilman E., Hall M., Booth H., Gupta T., Chaloupka M., Fennell H., et al. (2022). A decision support tool for integrated fisheries bycatch management. Rev. Fish. Biol. Fish. 32, 441–472. doi: 10.1007/s11160-021-09693-5
Gordon D. V., Hannesson R. (2015). The Norwegian winter herring fishery: A story of technological progress and stock collapse. Land. Econ. 91, 362–385. doi: 10.3368/le.91.2.362
Granado I., Hernando L., Galparsoro I., Gabiña G., Groba C., Prellezo R., et al. (2021). Towards a framework for fishing route optimization decision support systems: Review of the state-of-the-art and challenges. J. Cleaner. Prod. 320, 128661. doi: 10.1016/j.jclepro.2021.128661
Hamre J., Nakken O. (1971). “Technological aspects of the modern norwegian purse seine fisheries,” in Modern fishing gear of the world, vol. 3. Ed. Kristjonsson H. (Oxford: Fishing News (Books) Ltd).
Haugen J., Imsland L. (2019). Optimization-based motion planning for trawling. J. Mar. Sci. Technol. 24, 984–995. doi: 10.1007/s00773-018-0600-0
Haugen J., Kyllingstad L. T. (2021). Course planning for purse seiners (Norway: SINTEF). Available at: https://hdl.handle.net/11250/2991650.
Hind E. J. (2015). A review of the past, the present, and the future of fishers’ knowledge research: a challenge to established fisheries science. ICES. J. Mar. Sci. 72, 341–358. doi: 10.1093/icesjms/fsu169
Hjelle H., Gundersen S., Nedreaas K., Vølstad J. H., Kolding J. (2021). “Fleet composition, fishing effort and contributions to science,” in The coastal reference fleet 2007-2019 (Norway: Institute of Marine Research).
Hobday A. J., Spillman C. M., Paige Eveson J., Hartog ,. J. R. (2016). Seasonal forecasting for decision support in marine fisheries and aquaculture. Fish. Oceanography. 25, 45–56. doi: 10.1111/fog.12083
Holm P., Soma K. (2016). Fishers’ information in governance — a matter of trust. Curr. Opin. Environ. Sustainabil. 18, 115–121. doi: 10.1016/j.cosust.2015.12.005
Iglesias A., Dafonte C., Arcay B., Cotos J. (2007). Integration of remote sensing techniques and connectionist models for decision support in fishing catches. Environ. Model. Software. 22, 862–870. doi: 10.1016/j.envsoft.2006.05.017
Jacob V. S., Pirkul H. (1992). Organizational decision support systems. Int. J. Man-Machine. Stud. 36, 817–832. doi: 10.1016/0020-7373(92)90074-U
Jarre A., Paterson B., Moloney C. L., Miller D. C., Field J. G., Starfield ,. A. M. (2008). Knowledge-based systems as decision support tools in an ecosystem approach to fisheries: Comparing a fuzzy-logic and a rule-based approach. Prog. Oceanography. 79, 390–400. doi: 10.1016/j.pocean.2008.10.010
Jennings S., Kaiser M. J., Reynolds ,. J. D. (2001). Marine fisheries ecology (Oxford ; Malden, MA, USA: Blackwell Science).
Johannes R., Freeman M., Hamilton R. (2008). Ignore fishers’ knowledge and miss the boat. Fish. Fish. 1, 257–271. doi: 10.1111/j.1467-2979.2000.00019.x
Jones A. W., Burchard K. A., Mercer A. M., Hoey J. J., Morin M. D., Gianesin G. L., et al. (2022). Learning from the study fleet: Maintenance of a Large-scale reference fleet for northeast U.S. fisheries. Front. Mar. Sci. 9. doi: 10.3389/fmars.2022.869560
Karp M. A., Brodie S., Smith J. A., Richerson K., Selden R. L., Liu O. R., et al. (2022). Projecting species distributions using fishery-dependent data. Fish. Fish. 1–22 doi: 10.1111/faf.12711
Kelly C., Michelsen F. A., Kolding J., Alver M. O. (2022). Tuning and development of an individual-based model of the herring spawning migration. Front. Mar. Sci. 8. doi: 10.3389/fmars.2021.754476
Koutroumanidis T., Iliadis L., Sylaios G. K. (2006). Time-series modeling of fishery landings using ARIMA models and fuzzy expected intervals software. Environ. Model. Software. 21, 1711–1721. doi: 10.1016/j.envsoft.2005.09.001
Kroodsma D. A., Mayorga J., Hochberg T., Miller N. A., Boerder K., Ferretti F., et al. (2018). Tracking the global footprint of fisheries. Science 359, 904–908. doi: 10.1126/science.aao5646
Kyllingstad L. T., Haugen J., Tenningen M., Breen M. (2021). Catch control in seine fisheries targeting pelagic species (Norway: SINTEF). Available at: https://hdl.handle.net/11250/2991646.
Lane D. E., Stephenson R. L. (1998). Fisheries co-management: Organization, process and decision support. J. Northwest. Atlantic. Fishery. Sci. 23, 251–265. doi: 10.2960/J.v23.a16
Lazarowska A. (2014). Ant colony optimization based navigational decision support system. Proc. Comput. Sci. 35, 1013–1022. doi: 10.1016/j.procs.2014.08.187
Lee H., Aydin N., Choi Y., Lekhavat S., Irani Z. (2018). A decision support system for vessel speed decision in maritime logistics using weather archive big data. Comput. Operations. Res. 98, 330–342. doi: 10.1016/j.cor.2017.06.005
Ølmheim O. (2021). Economic and biological figures from Norwegian fisheries – 2020 (Norway: Fishery Directorate).
Macher C., Bertignac M., Guyader O., Frangoudes K., Frésard M., Le Grand C., et al. (2018). The role of technical protocols and partnership engagement in developing a decision support framework for fisheries management. J. Environ. Manage. 223, 503–516. doi: 10.1016/j.jenvman.2018.06.063
Macher C., Steins N. A., Ballesteros M., Kraan M., Frangoudes K., Bailly D., et al. (2021). Towards transdisciplinary decision-support processes in fisheries: experiences and recommendations from a multidisciplinary collective of researchers. Aquat. Living. Resour. 34, 13. doi: 10.1051/alr/2021010
Nøttestad L., Utne K. R., Óskarsson G. J., Jónsson S. T., Jacobsen J. A., Tangen Ø., et al. (2016). Quantifying changes in abundance, biomass, and spatial distribution of northeast Atlantic mackerel ( scomber scombrus ) in the Nordic seas from 2007 to 2014. ICES. J. Mar. Sci.: J. du. Conseil. 73, 359–373. doi: 10.1093/icesjms/fsv218
Nakken O. (Ed.) (2008). Norwegian Spring-spawning herring & northeast Arctic cod: 100 years of research management (Trondheim: Tapir Academic Press). OCLC: ocn262434637.
Reite K.-J., Haugen J., Michelsen F., Aarsæther K. (2021). Sustainable and added value small pelagics fisheries pilots. In: C Södergård, T Mildorf, E Habyarimana, A. J. Berre, J. A. Fernandes, C Zinke-Wehlmann (eds) Big data in bioeconomy (Cham: Springer). doi: 10.1007/978-3-030-71069-9_30
Reite K. J., Ladstein J., Haugen J. (2017). “Data-driven real-time decision support and its application to hybrid propulsion systems,” in International conference on offshore mechanics and Arctic engineering, vol. 57748. (Trondheim, Norway: American Society of Mechanical Engineers), V07BT06A024.
Röckmann C., van Leeuwen J., Goldsborough D., Kraan M., Piet G. (2015). The interaction triangle as a tool for understanding stakeholder interactions in marine ecosystem based management. Mar. Policy 52, 155–162. doi: 10.1016/j.marpol.2014.10.019
Sala R., Pezzotta G., Pirola F., Huang G. Q. (2019). Decision-support system-based service delivery in the product-service system context: Literature review and gap analysis. Proc. CIRP. 83, 126–131. doi: 10.1016/j.procir.2019.03.140
Santos A. P. (2000). Fisheries oceanography using satellite and airborne remote sensing methods: a review. Fish. Res. 49, 1–20. doi: 10.1016/S0165-7836(00)00201-0
Schadeberg A., Kraan M., Hamon K. G. (2021). Beyond métiers: social factors influence fisher behaviour. ICES. J. Mar. Sci. 78, 1530–1541. doi: 10.1093/icesjms/fsab050
Schau E. M., Ellingsen H., Endal A., Aanondsen S. A. (2009). Energy consumption in the Norwegian fisheries. J. Cleaner. Prod. 17, 325–334. doi: 10.1016/j.jclepro.2008.08.015
Skjong S., Kyllingstad L. T., Reite K.-J., Haugen J., Ladstein J., Aarsæther K. G. (2019). “Generic on-board decision support system framework for marine operations,” in Ocean engineering, vol. Volume 7A. (Glasgow, Scotland, UK: American Society of Mechanical Engineers), V07AT06A032. doi: 10.1115/OMAE2019-95146
Slagstad D., McClimans T. A. (2005). Modeling the ecosystem dynamics of the barents sea including the marginal ice zone: I. physical and chemical oceanography. J. Mar. Syst. 58, 1–18. doi: 10.1016/j.jmarsys.2005.05.005
Standal D., Asche F. (2018). Hesitant reforms: The Norwegian approach towards ITQ’s. Mar. Policy 88, 58–63. doi: 10.1016/j.marpol.2017.11.005
Stephenson R. L., Paul S., Pastoors M. A., Kraan M., Holm P., Wiber M., et al. (2016). Integrating fishers’ knowledge research in science and management. ICES. J. Mar. Sci. 73, 1459–1465. doi: 10.1093/icesjms/fsw025
Sutton R. T., Pincock D., Baumgart D. C., Sadowski D. C., Fedorak R. N., Kroeker K. I. (2020). An overview of clinical decision support systems: benefits, risks, and strategies for success. NPJ Digital. Med. 3, 17. doi: 10.1038/s41746-020-0221-y
Truong T., Rothschild B., Azadivar F. (2005). “Decision support system for fisheries management,” in Proceedings of the winter simulation conferenc (Orlando, FL. USA: IEEE), 2107–2111. doi: 10.1109/WSC.2005.1574494
Varpe Ø., Fiksen Ø., Slotte A. (2005). Meta-ecosystems and biological energy transport from ocean to coast: the ecological importance of herring migration. Oecologia 146, 443–451. doi: 10.1007/s00442-005-0219-9
Vinu Chandran R., Jeyaram A., Jayaraman V., Manoj S., Rajitha K., Mukherjee C. K. (2009). Prioritization of satellite-derived potential fishery grounds: an analytical hierarchical approach-based model using spatial and non-spatial data. Int. J. Remote Sens. 30, 4479–4491. doi: 10.1080/01431160802577980
Keywords: decision support, interface, knowledge, experience, observations, model
Citation: Kelly C, Michelsen FA, Reite KJ, Kolding J, Varpe Ø, Berset AP and Alver MO (2022) Capturing big fisheries data: Integrating fishers’ knowledge in a web-based decision support tool. Front. Mar. Sci. 9:1051879. doi: 10.3389/fmars.2022.1051879
Received: 23 September 2022; Accepted: 21 November 2022;
Published: 12 December 2022.
Edited by:
Nathalie A. Steins, Wageningen Marine Research IJmuiden, NetherlandsReviewed by:
Julia Calderwood, Marine Institute, IrelandMartin Pastoors, Pelagic Freezer-trawler Association, Netherlands
Copyright © 2022 Kelly, Michelsen, Reite, Kolding, Varpe, Berset and Alver. This is an open-access article distributed under the terms of the Creative Commons Attribution License (CC BY). The use, distribution or reproduction in other forums is permitted, provided the original author(s) and the copyright owner(s) are credited and that the original publication in this journal is cited, in accordance with accepted academic practice. No use, distribution or reproduction is permitted which does not comply with these terms.
*Correspondence: Cian Kelly, Y2lhbi5rZWxseUBudG51Lm5v