- 1University of California San Diego Scripps Institution of Oceanography, Marine Biology Research Division, La Jolla, CA, United States
- 2Center for Marine Biodiversity and Conservation, University of California (UC) San Diego, La Jolla, CA, United States
- 3Stanford Center for Ocean Solutions, Stanford University, Palo Alto, CA, United States
Small-scale fisheries (SSFs) and the foods they produce are extremely important, contributing 25–50% of global seafood landed for direct consumption. In some cases, SSFs provide seafoods with an exceptionally low carbon footprint, but like all food, it is important to understand the factors that regulate that footprint in the face of increasing demand and a worsening climate-ecological crisis. We utilize long-term fisheries monitoring data from Northwest Mexico to generate novel stock assessments and, subsequently, test the relationship between underlying fishery biomass and fuel intensity observed among several motorized SSFs. Using fuel data from over 4,000 individual fishing trips, in combination with estimated biomass data for 19 regional stocks, we show that the fuel footprint per kilogram of seafood increases sharply as the stock’s underlying annual biomass (B) falls below its estimated biomass at Maximum Sustainable Yield (BMSY). We find an inverse relationship between B/BMSY and fuel intensity using a test for simple correlation between the two (r= -0.44), a linear regression analysis (R2adj. = 0.17), and a mixed-effects model with gear type, year, and genus modelled as random effects. These results indicate that efforts to end overfishing, rebuild fishery stocks, and/or minimize intensive fishing practices will help to decrease the carbon emissions generated by motorized wild-catch fishing. We anticipate that this study will contribute an important “missing link” to discussions on how best to secure climate-resilient fisheries and, ideally, help SSF stakeholders garner recognition and support for SSFs in this context.
Introduction
Fisheries are an integral source of animal protein to nearly 1-in-5 of the world’s roughly 7.2 billion people (FAO, 2017; FAO SOFIA, 2018), making them a key element in all considerations related to global food security. While the production of wild-caught seafood has reached something of a plateau in terms of annual landed biomass (Pauly and Zeller, 2016), demand for seafood (and other animal products) has been steadily increasing over the last several decades and is projected to grow for the foreseeable future (Kearny, 2010; FAO SOFIA, 2018; Costello et al., 2020). Because seafood, like all food, bears some environmental footprint, it is important to understand the factors that maintain, diminish, or grow that footprint in the face of increasing demand and a worsening climate-ecological crisis (IPBES, 2019; IPCC, 2022).
Fishing is inextricably linked to nearly all marine landscapes, flora, and fauna — and the act of overfishing has been shown to have deleterious effects on abiotic and biogenic habitat, dramatically altering species abundance and the preservation of biodiversity, with often negative impacts on coastal communities of people (e.g., Turner et al., 1999; Worm et al., 2006; Sumaila and Tai, 2020; Sumaila et al., 2021). In the 1990’s, scientists began to document the serial depletion of fisheries stocks worldwide (e.g., Pauly et al., 1998), namely at the hands of industrial/large-scale fisheries, and several high-profile papers drew public attention to the fact that a large proportion of the world’s fish populations were experiencing overfishing or were already overfished (Pauly et al., 1998; Worm et al., 2006). Since then, numerous governmental and non-governmental policy agendas have been dedicated to ending overfishing and rebuilding stocks (FAO SOFIA, 2018). While these efforts have been met with some success (Duarte et al., 2020; Hilborn et al., 2020), overfishing remains a serious problem (FAO SOFIA, 2018), particularly for stocks which lack formal assessments (Costello et al., 2012; Hilborn et al., 2020).
SSFs produce anywhere from 25–50% of all seafood landed for direct consumption (Pauly and Zeller, 2016; FAO, 2017; FAO SOFIA, 2018; Greer et al., 2019) and, in some cases, serve as a source of food with an exceptionally low carbon footprint (Nijdam et al., 2012; Hilborn et al., 2018; Ferrer et al., 2021). SSFs can be motorized or non-motorized, targeting thousands of taxa and supporting millions of jobs in both the fishing and post-production sectors (FAO, 2017; FAO SOFIA, 2018). At the same time, SSFs tend to be “data-poor,” and often lack formal fishery stock assessments (Costello et al., 2012; FAO SOFIA, 2018; Hilborn et al., 2020). This makes them often difficult to manage (Costello et al., 2012; FAO SOFIA, 2018; Hilborn et al., 2020) and notoriously underrepresented in socio-political discussions surrounding (sustainable) global food production (e.g., Cohen et al., 2019). Given their diversity, there does not exist a universal definition for SSFs (Hidden Harvest Report, 2012; Smith and Basurto, 2019), but for the purposes of this article, we define SSFs to be those fisheries targeted by vessels < 12m in length (FAO, 2022).
Key considerations vis-à-vis “sustainable” seafood are the existence or non-existence of overfishing within that fishery/particular region, as well as gear type, and extent of fishing effort. Ending overfishing is a valuable endeavor in its own right, with several co-benefits, such as increased ecosystem biomass and avoided damages to aquatic habitat (Sumaila and Tai, 2020). A decrease in the total carbon footprint and/or fuel intensity of fisheries are two additional co-benefits that have been theorized by fisheries scientists; the logic behind this idea being that an end to overfishing would require a decrease in overcapacity of the world’s fishing fleets, shrinking the total fuel footprint of fisheries while growing available biomass across a number of stocks, and effectively increasing the Catch per Unit Effort (CPUE) (The World Bank, 2017; Sumaila and Tai, 2020). This idea is compelling and largely intuitive, yet the relationship between overfishing and emissions is supported by a limited number of empirical studies and relevant inquiries focused on SSFs are noticeably scant (see Parker and Tyedmers, 2015; Rousseau et al., 2019; Bloor et al., 2021).
Here, we utilized long-term fisheries monitoring data from Northwest Mexico to generate novel stock assessments and to, subsequently, test the relationship between underlying stock status and fuel intensity among several SSFs in the region [note that we use fishery biomass as a proxy for stock status]. Like others have alluded to (e.g., The World Bank, 2017; Sumaila and Tai, 2020), we hypothesize that there exists an inverse relationship between fishery biomass (B/BMSY) and fuel intensity — that is to say, as fishery biomass decreases, the fuel required to land one unit of seafood increases. To substantiate this hypothesis, we utilized two independent fishery databases, in combination with methods for “data-poor” stock assessment (developed by Froese et al., 2017), to explore the relationship between estimated B/BMSY and fuel intensity across 19 Stocks, and 39 “Stock-Years of Interest” (defined in Section 2.3). We end with a discussion on the theoretical climate-fishing feedbacks that have been proposed in the literature to date, and discuss the importance of our results in the context of overlapping social, climate, and biodiversity objectives.
Methods
To test the relationship between fishery biomass (B/BMSY) and fuel intensity, we draw from the following independent data sources: (i) fuel and catch data from the Gulf of California Marine Program (GCMP) Fisheries Monitoring Network, hereafter referred to as “the GCMP database” (Mascareñas-Osorio et al., 2017); and (ii) fisheries landings data supplied by the Mexican governmental agency known as Comisión Nacional de Acuacultura y Pesca (CONAPESCA), hereafter referred to as “the CONAPESCA database” (Mascareñas-Osorio et al., 2018). Both of these databases are updated periodically, and the data used herein reflect observations from the GCMP database recorded through March, 2018, and from the CONAPESCA database recorded through December, 2019. See Figure 1 for a stylized representation of our methodology, and consult the Supplementary Material section to access relevant dataframes. All analyses were performed using Microsoft Excel in combination with R version 4.0.3 (R Core Team, 2020).
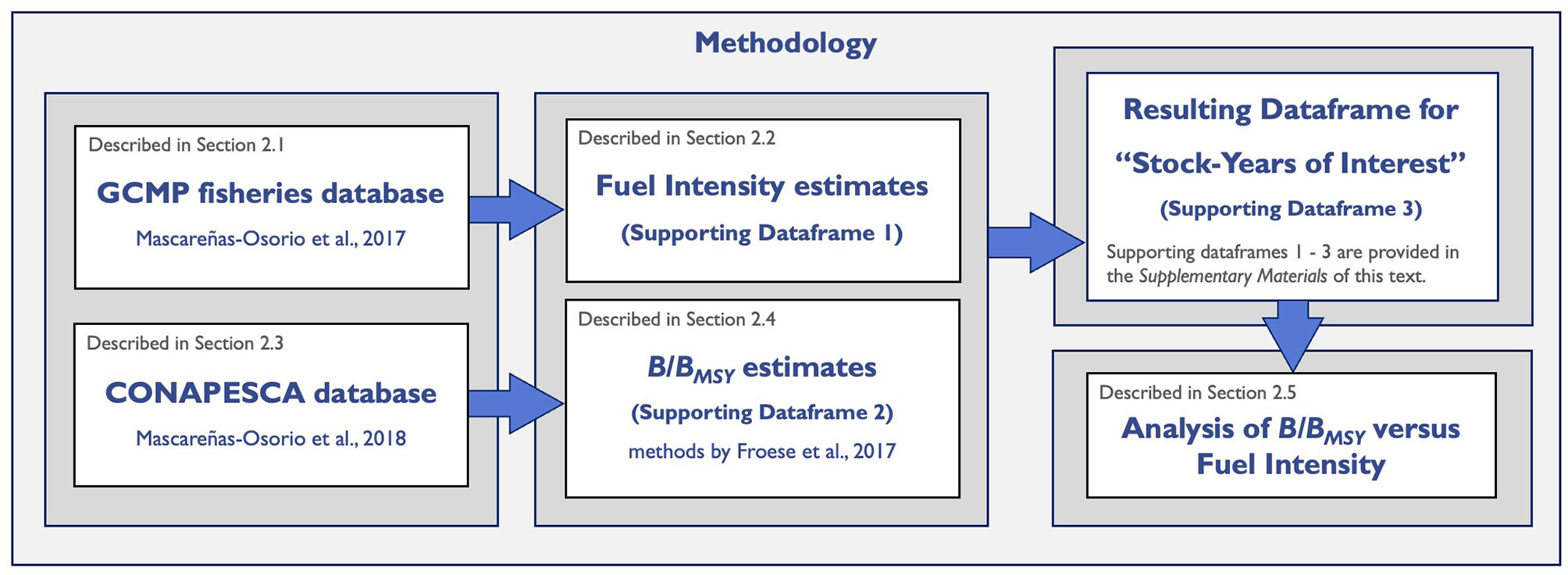
Figure 1 Infographic showing our methodological flow from Section 2.1 to 2.5. Here, “GCMP” stands for the Gulf of California Marine Program, and “CONAPESCA” stands for the Comisión Nacional de Acuacultura y Pesca.
Collecting High-Resolution Catch and Fuel Data From SSFs in Northwest Mexico (Utilizing “the GCMP Database”)
Northwest Mexico is home to two of the country’s most productive fishery zones: the southern extension of the California Current Ecosystem, and the Gulf of California (GoC) (Cisneros-Mata, 2010, FAO, 2022). Indeed, from our own interrogation of the CONAPESCA database, we found that from 2006 to 2014, SSFs in Northwest Mexico contributed 52% of the total biomass generated by the nation’s marine SSFs (Ferrer et al., 2021).
Understanding the critical importance of SSFs in and around the GoC, colleagues with the GCMP and local small-scale fishers have worked collaboratively for over a decade to collect high-resolution fisheries monitoring data from in and around the Baja California Peninsula (see http://gulfprogram.ucsd.edu/slider-home/projects/). This monitoring program employs portable GPS tracking devices to populate a database with thousands of fishing tracks (> 25,000), about 5,000 of which are further appended with information related to catch and fuel consumption. These records within the GCMP database comprise the universe of data we used to generate the fuel intensity estimates described below.
Fuel Intensity Estimates From the GCMP Database
A fuel intensity estimate (FIE) is a measure of fuel efficiency, telling us the fuel consumption per unit of X, where in this case X is one kilogram of wet weight1, 2 seafood. To generate FIEs, we divided the gasoline consumption (in liters) by the wet weight of catch reported for each single-species fishing trip identified/located in the GCMP database. To avoid the numerous uncertainties associated with partitioning fuel consumption among trips targeting multiple species (Vázquez-Rowe et al., 2012), we constrained our analysis to records for single-species trips. We also excluded any records that were obviously duplicated, incomplete, split, or erroneous (e.g., average boat speed > 80 km/hr). The resulting dataframe (nrecords= 4,795) is included in the Supplementary Material section of this text, entitled “Supporting Dataframe 1”. The raw data for this analysis (Mascareñas-Osorio et al., 2017) are available upon consultation at dataMares.ucsd.edu.
Extracting Time Series Data From the CONAPESCA Database Based on Two “Criteria for Inclusion”
To generate stock assessment profiles (described in Section 2.4), we first needed to extract catch time series from the CONAPESCA database (Mascareñas-Osorio et al., 2018). The particular version of the CONAPESCA database that we used is a repository consisting of tens of thousands of records for small-scale and industrial fishing activities, targeting over 500 taxa, reported in all of Mexico’s Pacific states. Thus, to extract the relevant time series from this rather large database, we needed to identify the “Stocks of Interest” for which we would eventually require estimates of B/BMSY (Section 2.4); we did so based on the definition of a “Stock,” and the two “Criteria for Inclusion,” we describe below.
We define a “Stock” (S) as any genus (G) living in fishing zone (Z), such that S = GZ. Subsequently, we define a “Stock-Year” (SY) as a stock (S) in a specific year (Y), such that one SY = GZ,Y. So, for example, we consider Callinectes-Lower Pacific-2015 and Callinectes-Lower Pacific-2016 as two separate “Stock-Years” borne from the same “Stock” of swimming crab (Callinectes sp.). We defined fishing zones (Z) a priori, based on expert knowledge of the area and a large geographic separation of recorded ports (Figure 2). The fishing zones relevant to our analyses are as follows: (1) the “Central Pacific” (CP), which includes ports circa Punta Eugenia; (2) the “Lower Pacific” (LP), which includes major ports on the Pacific side of Baja California Sur, near Bahía Magdalena; (3) the “Lower Gulf” (LG), which we’ve defined as the ports in and around La Paz, south of Loreto; and finally, (4) the “Upper Gulf” (UG), which spans the region from the Colorado River Delta to the Midriff Islands in the GoC. Note that, on average, small-scale fishers in the region only travel about 63 kilometers in a given fishing trip (see “Supporting Dataframe 1”), and it is unlikely — though not impossible — that fishers in, for example, the LP would land and register their catch in the LG.
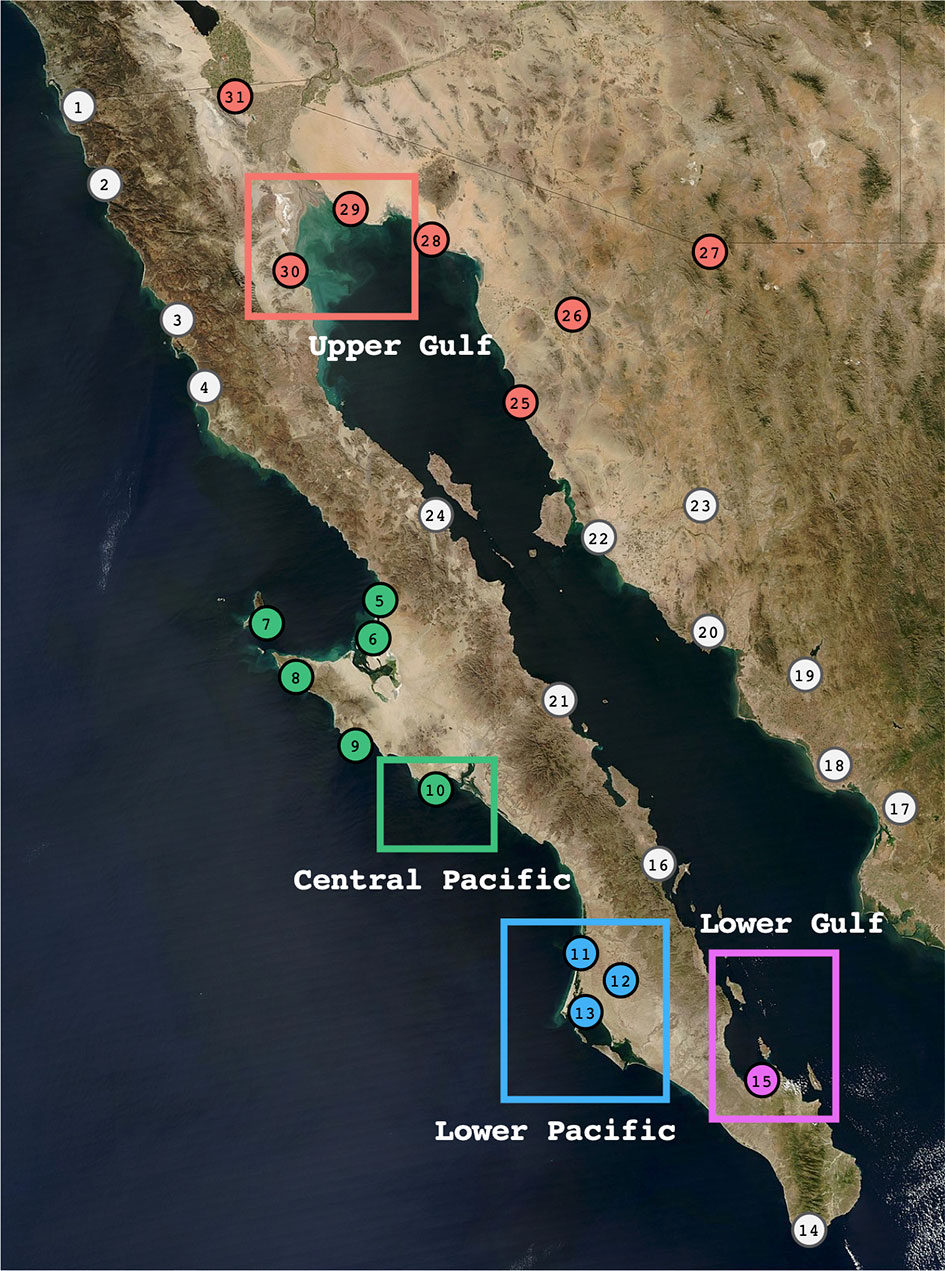
Figure 2 Map of Baja California, where each circle represents a fishery office recorded in the CONAPESCA database, and each square demarcates the approximate spatial footprint of fishing trips for which there exist records of fuel consumption in the GCMP (Gulf of California Marine Program) database. For a complete roster of CONAPESCA office names, see Table S1. Respectively, the offices shown in green (#5 - #10), blue (#11 - #13), purple (#15), and red (#25 - #31), represent those with fishery reports that we used to generate stock assessment profiles for “Stocks of Interest” in the “Central Pacific”, “Lower Pacific”, “Lower Gulf”, and “Upper Gulf” (as described in Section 2.4). Conversely, we did not utilize CONAPESCA landings data reported by those offices shown in grey. (Underlying map of Baja California is courtesy of the MODIS Rapid Response Team and NASA, and is available for download at: https://commons.wikimedia.org/wiki/File:Baja_peninsula_(mexico)_250m.jpg).
With these definitions in mind, we decided upon two “Criteria for Inclusion,” that is, two criteria used to determine whether or not a stock would be included in our final statistical analyses and therefore in need of assessment. First, we stipulated that a stock (S) can be found in the CONAPESCA database with ≥ 10-years’ worth of landings data; and second, that that stock has at least one Stock-Year (SY) represented in the GCMP database with ≥ 10 paired observations of fuel consumption and catch. Ultimately, these “Criteria for Inclusion” produced a list of 19 “Stocks of Interest” for which we were able to extract landings time series from the CONAPESCA database, and which are associated with the 39 “Stock-Years of Interest” listed in Table S2.
The reason for our first “Criteria of Inclusion,” in which we have stipulated that n years of CONAPESCA catch data must be ≥ 10, has to do with the number of years required to generate a reliable stock assessment. According to Froese et al. (2017), it is sometimes possible to generate an stock assessment profile with as little as five-year’s worth of catch data, however, the fewer the number of years, the larger the uncertainty. Moreover, for species with low or very-low intrinsic population growth (e.g., ocean whitefish Caulolatilus princeps), about 10 years’ worth of landings data are required to generate an informed prior about the fishery’s catchability and, in turn, its Maximum Sustainable Yield (Froese et al., 2017). The second criteria we have defined, that n paired observations of fuel use & catch in the GCMP database must be ≥ 10, has to do with the methods we used to test the relationship between B/BMSY and fuel intensity among “Stock-Years of Interest” (described in Section 2.5); summarily, we wanted to include only those Stock-Years for which it would be possible to generate a reliable estimate of mean fuel intensity.
Methods for Stock Assessment: Synthesizing B/BMSY Values for “Stock-Years of Interest”
B/BMSY is often used as a measure for stock status and a proxy for “overfishing,” where B is the current (available) stock biomass, and BMSY is the estimated stock biomass at “Maximum Sustainable Yield” (MSY). MSY is the theoretical level of extraction equal to the rate of added population growth over an indefinite period of time — the highest level at which it is possible to extract fish from a population while still maintaining that stock’s standing biomass. By fishing a stock at MSY, the biological stability, economic value, and contribution to fishers’ livelihoods (derived from that stock) are more likely to be preserved in the long-term (Giron-Nava et al., 2021). Meanwhile, fishing a stock at levels above MSY is considered “overfishing,” which, if allowed to persist, will eventually diminish or crash that population (e.g., New England Cod; Pershing et al., 2015).
Herein lies a key distinction between “overfishing” and “overfished”. Overfishing, estimated as the current fishing mortality versus fishing mortality at MSY (F/FMSY), is the actual act of fishing beyond MSY at any given point in time. In contrast, B/BMSY tells us if a stock is currently overfished, which, if it is, is usually the direct result of habitual or chronic overfishing. We contend that, in this respect, B/BMSY carries some amount of “memory” vis-à-vis fisher behavior in response to stock status over time. We have therefore elected to use B/BMSY as our proxy for overfishing, where biomass (B) exists on a gradient from “pristine” (B/BMSY = 2) to “overfished” (B/BMSY ≤ 0.5), such that when B is precisely equal to biomass at MSY, the ratio of B/BMSY is equal to 1.
To generate B/BMSY estimates for the 39 “Stock-Years of Interest” described above (in Section 2.3), we applied a data-poor method for fishery stock assessment developed by Froese et al. (2017) to the catch time series we extracted from the CONAPESCA database. Summarily, this method, which Froese et al. (2017) dub the “Simple CMSY,” applies a Bayesian model to fisheries-dependent time series to generate most-likely values for B/BMSY over time. It does so based on the landings data combined with informed priors about the stock’s intrinsic population growth (r) and starting biomass (B0). To better understand the theoretical and mathematical underpinnings of the Simple CMSY method, we recommend reviewing the original research by Froese et al. (2017) as well as a subsequent application of their methods to SSFs in Mexico executed by Giron-Nava et al. (2019). The resulting stock assessment profiles we generated using this method are included in Supplementary Materials - “Supporting Dataframe 2”.
Examining the Relationship(s) Between B/BMSY and Fuel Intensity
To test for a relationship between B/BMSY and fuel intensity across the 39 “Stock-Years of Interest,” we employed three complementary statistical analyses. We reasoned that if there exists a strong (linear) relationship between B/BMSY and fuel intensity across SSFs, it would be apparent via a simple correlation test and, likely, a simple linear model. Thus, we began with (i) a Pearson’s correlation test between estimated values for B/BMSY and mean values for fuel intensity (Fuel Intensitymeans). We then constructed (ii) a simple linear regression, where Fuel Intensitymean values are predicted by B/BMSY. Each Fuel Intensitymean value is calculated as the arithmetic mean of all FIEs associated with that particular Stock-Year. Here, we compared B/BMSY values to Fuel Intensitymean values in an effort to control against the influence of uneven sample sizes across Stock-Years (sample sizes for each are listed in Table S2).
The third and final analysis we conducted was (iii) a mixed effects model where any individual estimate for fuel intensity is predicted by its corresponding value for B/BMSY as a fixed effect, along with three random effects: gear type, genus, and year. One might reasonably expect that the fuel-use per kilogram of landed seafood on any given trip is borne from a number of factors beyond the fishery’s underlying stock biomass which could cause or covary with B/BMSY. We know, for instance, that fishing patterns, fleet (over)capacity, management schemes, underlying ecosystem dynamics, weather patterns, and changes in climate are all important factors that can determine fishery outcomes (e.g., Worm et al., 2006; Pershing et al., 2015; Schuhbauer et al., 2017; Hilborn et al., 2020; Giron-Nava et al., 2021). For the most part, these dynamics lie beyond the scope of this paper, or the data are not available at this time. We can, however, begin control for some of this complexity — both observed and unobserved — by incorporating the additional information we have access to via the GCMP database about gear type, genus, and year (at the trip-level).
We controlled for gear type as a random effect based on findings from previous studies, which indicate that gear type plays an important role in the overall emissions borne from fishing activities (Parker and Tyedmers, 2015; Parker et al. 2018; Ferrer et al., 2021) . Likewise, we included genus and year as random effects since target taxa and unobserved changes over time likely play important roles in predicting fuel expenditures. We chose to model these three factors as random effects because, while we anticipate that they do matter, we are not explicitly interested in the fixed effects of gear type, genus, nor temporal factors on the observed discrepancies among individual estimates for fuel intensity. Rather, our primary interest lies in understanding the generalized relationship between B/BMSY (a fixed effect) and fuel intensity (a continuous output) across gear types, target genera, and years.
We generated the mixed effects model using the “lme4” package in R (Bates et al., 2015), and utilized the R “lmerTest” package to generate associated p values (Kuznetsova et al., 2017). Notably, the “lmerTest” package applies “Satterthwaite’s method” (Fai and Cornelius, 1996) to estimate degrees of freedom, covariance, and significance values for unbalanced samples in mixed effects models. Thus, in contrast to our first and second analyses, it was not necessary to test B/BMSY against Fuel Intensitymean values here, due to the very nature of the mixed effects model itself. Instead, we compared estimates for B/BMSY against individual estimates for fuel intensity, generated over 4,491 single-species fishing trips. (Data associated with each of these trips can be accessed in Supplementary Materials - “Supporting Dataframe 3.”)
Results
Stock Assessment Profiles
We have included the results from our stock assessments in “Supporting Dataframe 2”. Specifically, we report estimated values and confidence intervals for B/BMSY and F/FMSY for all 19 “Stocks of Interest” over time (2001–2019), as generated by the Simple CMSY method.
Stocks that are both overfished and experiencing overfishing are those with a B/BMSY < 1 and a F/FMSY > 1 (e.g., Giron-Nava et al., 2019; see also UW - Sustainable Fishing 101: https://sustainablefisheries-uw.org/seafood-101/overfished-overfishing-rebuilding-stocks/). Conversely, stocks that are fished “sustainably” are those that are neither overfished (B/BMSY > 1) nor experiencing overfishing (F/FMSY < 1). There are many cases in between, where stocks can be overfished but recovering (B/BMSY < 1, F/FMSY < 1), or not yet overfished but experiencing overfishing (B/BMSY > 1, F/FMSY > 1). With these definitions in mind, we found evidence of at least some overfishing in every year for which we were able to generate assessment data. We also found evidence that many of these stocks are currently overfished.
In 2019, the most recent year for which we were able to generate assessment data, we found that out of the 19 stocks, nine (47%) were overfished and experiencing overfishing (B/BMSY < 1, F/FMSY > 1); zero (0%) were overfished and recovering (B/BMSY < 1, F/FMSY < 1); two (11%) were not overfished but were experiencing overfishing (B/BMSY > 1, F/FMSY > 1); and eight stocks (42%) were being fished sustainably (B/BMSY > 1, F/FMSY < 1). Of the eight stocks that were fished sustainably that year, six of them were located in the LP, one in the LG, and one in the UG.
Our results for B/BMSY, on which we’ve based our assessment of overfishing, are largely consistent with anecdotal evidence, ecological research (e.g., Lluch-Cota et al., 2007), and local ecological knowledge about the region’s fish populations (e.g., Sáenz-Arroyo et al., 2005). For example, as of 2016, Giron-Nava et al. (2019) found that in the GoC, 69% of the stocks analyzed (n = 121) were overfished and still being fished at unsustainable levels; 13% were overfished but recovering; 11% were not overfished but were being fished at unsustainable levels; and just 7% were fished “sustainably,” neither overfished nor experiencing overfishing.
Relationship Between B/BMSY and Fuel Intensity
A visual assessment of the data (Figures 3A, B) suggests that, the relationship between B/BMSY and fuel intensity is inverse and exponential of some type, and we therefore report our results for B/BMSY against log10(Fuel Intensity) or log10(Fuel Intensitymean) values where appropriate. We’ve elected to report the results of our first two analyses in terms of B/BMSY versus Fuel Intensitymean for reasons explained above (in Section 2.5), however, the interpretation of our results does not change if we compare values of B/BMSY to demeaned values for fuel intensity (Figure S1).
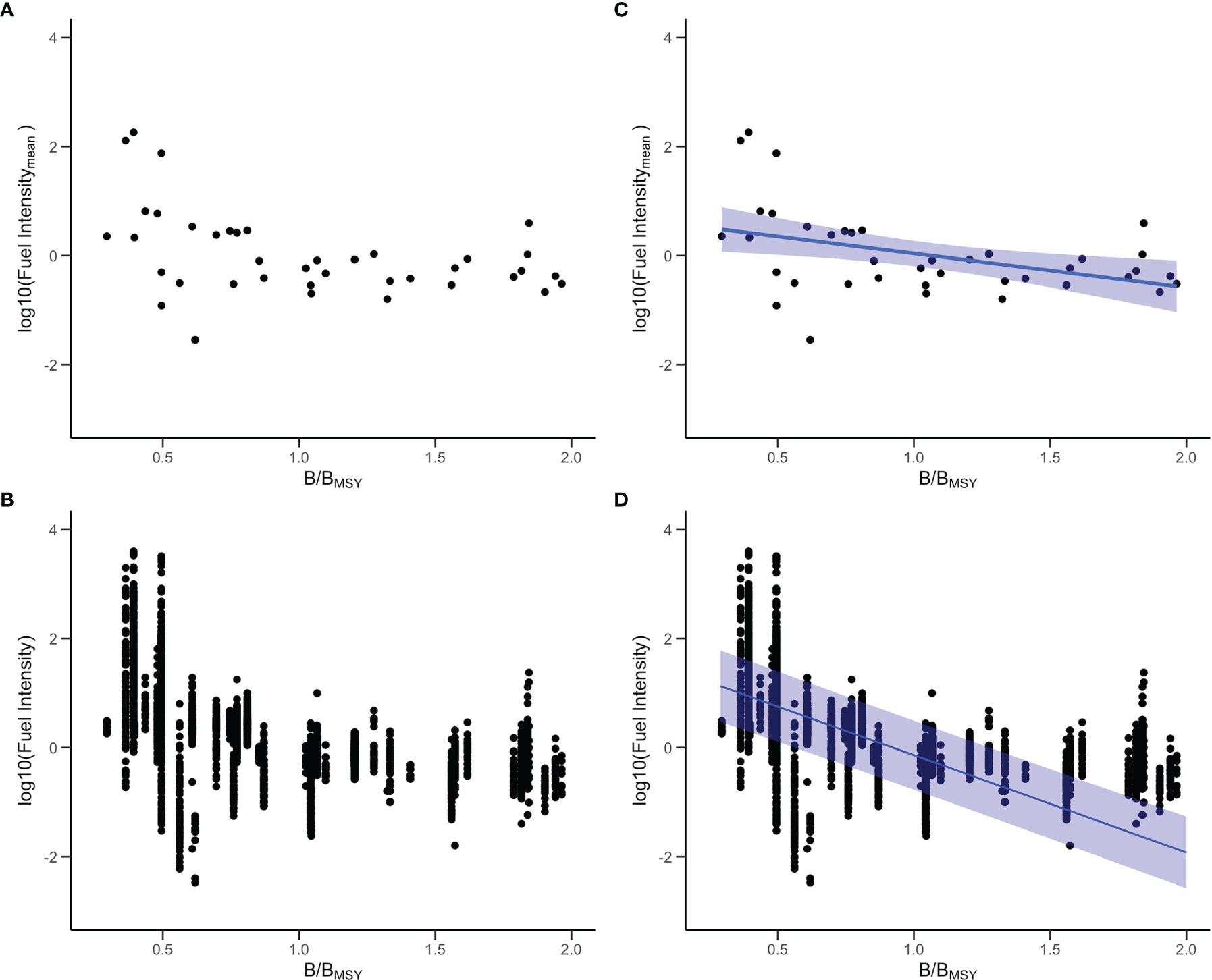
Figure 3 A plot of the raw data showing (A) mean and (B) demeaned values for estimates of fuel intensity. On the x-axis, B/BMSY is plotted from 0.25 to 2.0, and on the y-axis, the appropriate measure of fuel intensity is plotted in log10 scale. In panels (B) and (D), each point represents a unique B/BMSY-fuel intensity combination derived from an individual fishing trip, and in panels (A) and (C), each point represents a unique B/BMSY-Fuel Intensitymean combination associated with a particular Stock-Year. Panel (C) shows the linear regression of B/BMSY versus fuel intensity, where the blue line represents the predicted relationship, and the band around the line depicts 95% confidence intervals; note that the R2adj. for this simplified linear relationship is 0.17 (p value < 0.01). Finally, panel (D) shows the results of our mixed effects model, where fuel intensity is predicted by B/Bmsy (statistically-significant fixed effect), as well as “gear type”, “genus”, and “year” modelled as random effects; the blue line represents the relationship predicted by our mixed effects model, and the band around the line depicts 95% confidence intervals predicted by the “effects” package in R (Fox et al., 2020).
We found a statistically-significant negative correlation between B/BMSY and log10(Fuel Intensitymean) using a Pearson’s test for correlation, where r(df = 37) = -0.44 (p value < 0.01). Subsequently, we conducted a simple linear regression, where log10(Fuel Intensitymean) was predicted by B/BMSY with an R2adj. = 0.17 (df = 37, p value < 0.01) (Figure 3C). Finally, the existence of an inverse relationship between B/BMSY and fuel intensity was further corroborated by the results of our mixed effects model (Figure 3D), where B/BMSY is a statistically-significant predictor of log10(Fuel Intensity) (p value < 0.01), when accounting for gear type, genus, and year as random effects. According to this model parameterization, the association between B/BMSY and log10(Fuel Intensity) has a negative correlation of r = -0.36. Notably, B/BMSY remained a significant predictor of both fuel intensity and Fuel Intensitymean under a variety of controls and robustness checks (Figure S1).
Discussion
Projections indicate that as the effects of climate change continue to manifest, many fisheries (though not all) will be negatively impacted (Allison et al., 2009; Free et al., 2019). As fisheries change or degrade, fishing effort may increase while, on net, CPUE declines. As net CPUE declines, fishers working in both small-scale and large-scale fishery settings may burn more fuel in an effort to land the same amount of fish, contributing more CO2 to the atmosphere along with other fossil fuel-derived pollutants. Burning more fuel is likely to increase the costs of production, while depleting regional air and water quality2, and potentially contributing to other social and environmental consequences (Aburto-Oropeza et al., 2018). To sustain their livelihoods, fishers may expend more effort fishing — be it in the form of more time spent on the water, gear deployed, etc. — increasing the expense/labor associated with fishing as well as the likelihood that stocks will become overfished. Thus, one can hypothesize a “positive” feedback loop (albeit small and asymmetrical) where, as overfishing increases, the fuel intensity of seafood grows and climate change continues, resulting in increasingly negative fishery outcomes (Sumaila and Tai, 2020).
Our results indicate that at least one aspect of this theoretical feedback loop very likely exists, in that the fuel intensity per kilogram of seafood appears inversely related to underlying stock biomass among several SSFs in Northwest Mexico. One cannot necessarily infer causation from our results, however, the relationship between B/BMSY and fuel intensity proves suggestive and predictive under a variety of controls. Given the inverse and log-linear nature of this relationship, fuel intensity increases sharply for those seafood products generated by fisheries with a B/BMSY < 1. This lends credence to the theories we described earlier (The World Bank, 2017; Sumaila and Tai, 2020), which posit that the act of habitual overfishing contributes to the carbon footprint associated with fishing.
Our results give us some idea of the carbon emissions that could be avoided with even slight improvements to stock biomass. Given that the majority of carbon emissions associated with wild-caught seafood are generated by burning fossil fuels while fishing (Vázquez-Rowe et al., 2012; Parker and Tyedmers, 2015), we can devise a short back-of-the-envelope calculation as follows: the average Fuel Intensitymean of seafood landed among Stock-Years with a B/BMSY ≥ 1 is 0.66 L fuel/kg catch, and for those with a B/BMSY < 1 is 22.31 L fuel/kg catch. Thus, if we assume a fuel-to-emissions conversion factor of 2.3 kg CO2-equivalent per liter of gasoline (Natural Resources Canada, 2014)3, the carbon footprint generated by Stock-Years examined herein with a B/BMSY < 1 contributes (on average) an additional 50 kilograms of CO2-equivalent per kilogram of wet-weight seafood, compared to those with a B/BMSY ≥ 1.
Evidence of this nature might motivate climate-oriented policies designed to restore fisheries and alleviate fishing pressure, or perhaps generate novel possibilities for those countries that seek to better incorporate the ocean and blue carbon into their Nationally Determined Contributions under the Paris Agreement (“NDCs”) (see Gallo et al., 2017). Indeed, our results expand our understanding of, and appreciation for, the ways in which the protection of biodiversity and a stable climate are inextricably linked: abating climate change will help to conserve healthy fish populations, and, vice versa, the conservation of healthy fish populations may help to mitigate climate change (IPBES, 2019; IPCC, 2022). The conservation of marine ecosystem services (IPBES, 2019) and fish populations as “blue carbon” sinks (e.g., Mariani et al., 2020) are two popular examples of how ending overfishing might be considered a form of climate action. Now, we have evidence to suggest that, in addition to conserving ecosystem services and blue carbon sinks, ending overfishing is likely to make it more carbon-efficient to supply seafood security going forward.
To be clear, SSFs, in all their importance, diversity, and abundance, likely account for a relatively small portion of greenhouse gas emissions borne from total seafood production. While the carbon footprints of SSFs are heterogenous and can be high (see Purcell et al., 2018, Ferrer et al., 2021), Greer et al. (2019) estimate that SSFs land about a quarter of the world’s wild catch for a little less than a quarter of all fishery emissions. At the same time, we know that the carbon emissions borne from the fishing sector in general are non-negligible (accounting for ~1% of all global CO2 emissions; Sumaila and Tai, 2020), and it’s possible that the relationship we’ve identified here is generalizable to the fishing sector more broadly. While this remains a largely open question, we posit that, independent of the answer, investing time and resources towards ending overfishing presents a prime opportunity for stakeholders with varied interests (e.g., fisheries management, fisheries livelihood, blue carbon) to collaborate in protecting and rebuilding healthy fish populations.
To this end, we argue that it is important to continue designing and investing in culturally-relevant and participatory management schemes that support fishers in fishing sustainably (e.g., Bloor et al., 2021; Gómez and Maynou, 2021; see also FAO SOFIA, 2018). For fisheries in Northwest Mexico, successful community-based efforts to ensure sustainable fishing among SSFs are already underway, and have been for some time. The Community Catch Monitoring Program in the Upper GoC (see Juárez, 2021), and the SCPPPA cooperative that governs fishing in the town of Punta Abreojos (Cota-Nieto et al., 2018), are just a two notable examples. For the former, Juárez (2021) describes how local rights-based management efforts have helped to stabilize the Gulf Corvina fishery over the last decade — and for the later, Cota-Nieto et al. (2018) describe how a number of strategic, participatory management actions taken by fishers and other community members have ensured the town’s fishing success over multiple generations.
Strategies for ending overfishing more broadly include: eliminating harmful fishing subsidies while supporting those that are beneficial, particularly among SSFs (Schuhbauer et al., 2017; Sumaila et al., 2021); protecting SSFs from exclusionary or exploitative fishing and management practices, including those conducted by industrial fisheries and other large-scale industry interests (see Schuhbauer et al., 2017; Cohen et al., 2019); improving our fishery assessments among historically “data-poor” stocks (Costello et al., 2012; Hilborn et al., 2020) and prioritizing SSF data needs (e.g., Smith and Basurto, 2019); working to alleviate illegal, unreported, and unregulated fishing (IUU) (World Bank, 2017); closing tax loopholes and shuttering tax havens that undermine biodiversity objectives and have, in some cases, been shown to contribute to IUU (Dempsey et al., 2021); and finally, investing in the restoration of ecosystems upon with healthy fisheries depend (Sumaila et al., 2012; Duarte et al., 2020).
Data Availability Statement
The original contributions presented in the study are included in the article/Supplementary Material. Further inquiries can be directed to the corresponding author.
Author Contributions
EF conceived of the initial idea for this article and implemented its execution. AG-N helped established the methods underlying this article, contributed to analysis, and edited the manuscript. OA-O helped contribute to the vision, methods, and execution of this research, including production of the results and manuscript. All authors contributed to the article and approved the submitted version.
Funding
EF is supported by the NSF GRFP under Grant No. DGE-2038238, and the University of California, San Diego. The GCMP’s Fisheries Monitoring Program benefits from funding from The David and Lucile Packard Foundation, The Walton Family Foundation, Fondo Mexicano para la Conservacion de la Naturaleza, and CONACyT. Funds from Our Fish, administered by The University of British Columbia, covered the costs of this publication.
Conflict of Interest
Author EF received an honorarium from the non-governmental organization Our Fish to cover the costs of this article’s publication, and for some minor consult and presentations related to this topic. Our Fish was not involved in the design of this study, data collection, analysis, interpretation of the data, writing of this article, nor the authors’ decision to submit it for publication.
The remaining authors declare that the research was conducted in the absence of any commercial or financial relationships that could be construed as a potential conflict of interest.
Publisher’s Note
All claims expressed in this article are solely those of the authors and do not necessarily represent those of their affiliated organizations, or those of the publisher, the editors and the reviewers. Any product that may be evaluated in this article, or claim that may be made by its manufacturer, is not guaranteed or endorsed by the publisher.
Acknowledgments
EF would like to thank those who supplied helpful feedback on this research, including Drs. Lisa A. Levin, Brice X. Semmens, M. A. Jackson, and W. D. Howden, plus the two reviewers and handling editors who carried out peer-review. EF would also like to thank Dr. Rashid Sumaila for the invitation to participate in this special issue, as well as Rebecca Hubbard, Mike Walker, and other friends of Our Fish for the many stimulating conversations on this topic. The GCMP’s Fisheries Monitoring Program and the Aburto Lab at University of California San Diego benefits from collaboration with members of MAREA (NSF CNH‐L: DEB‐1632648). We give thanks to additional members of the GCMP and dataMares, including Catalina López‐Sagástegui, Victoria Jiménez-Esquivel, J. José Cota-Nieto, Ismael Mascareñas-Osorio, and Santiago Dominguez‐Sánchez, for their work. Finally, a special thank you to the fishermen who work with the GCMP, without whom this science would not be possible; Un agradecimiento muy especial a los pescadores que trabajan con el GCMP, sin los cuales esta ciencia no serıá posible.
Supplementary Material
The Supplementary Material for this article can be found online at: https://www.frontiersin.org/articles/10.3389/fmars.2022.768784/full#supplementary-material
Footnotes
- ^ Key assumption: About half of the fishing records we analyzed reported total landings in terms of wet weight (kilograms), while the other half were marked as having been been butchered or prepared in some way. We assumed that, in most cases, prepared weight accounted for 40% of total wet weight (Nijdam et al., 2012), such that wet weight = prepared weight • (0.40)-1, with two notable exceptions to this rule: beheaded shrimps, where we assumed that the rest of the shrimp’s body accounted for 65% of total wet weight (https://louisianadirectseafood.com/seafood-handbook/), and shark / ray fins ("aleta"), which we assumed accounted for just 5% of total wet weight (Cortes & Neer, 2006). For fishing trips where the style of preparation was not explicitly noted, we assumed that the catch was reported in terms of wet weight. Note that the statistical significance and interpretation of our results appears robust to alternative conversion factors of prepared-to-wet weight, where some conversion factors actually increased the statistical-significance and effect size of our results.
- ^ See: https://www.epa.gov/nutrientpollution/sources-and-solutions-fossil-fuels
- ^ This conversion factor only accounts for the quantity of greenhouse gases emitted directly by the burning of gasoline. It does not, for example, account for the upstream emissions associated with the production of fossil fuels, nor the downstream emissions associated with seafood production and transport.
References
Aburto-Oropeza O., Johnson A. F., Agha M., Allen E. B., Allen M. F., Arellano Gonzáles A., et al. (2018). Harnessing Cross-Border Resources to Confront Climate Change. Environ. Sci. Policy 87, 128–132. doi: 10.1016/j.envsci.2018.01.001
Allison E. H., Perry A. L., Badjeck M.-C., Adger W. N., Brown K., Conway D., et al. (2009). Vulnerability of National Economies to the Impacts of Climate Change on Fisheries. Fisheries Fisheries 10, 173–196. doi: 10.1111/j.1467-2979.2008.00310.x
Bates D., Mächler M., Bolker B., Walker S. (2015). Fitting Linear Mixed-Effects Models Using Lme4. J. Stat. Softw. 67 (1), 1–48. doi: 10.18637/jss.v067.i01
Bloor I. S. M., Duncan P. F., Dignan S. P., Emmerson J., Beard D., Gell F. R., et al. (2021). Boom Not Bust: Cooperative Management as a Mechanism for Improving the Commercial Efficiency and Environmental Outcomes of Regional Scallop Fisheries. Mar. Policy 132 (104649), 1–10. doi: 10.1016/j.marpol.2021.104649
Cisneros-Mata M. A. (2010). The Importance of Fisheries in the Gulf of California and Ecosystem-Based Sustainable Co-Management for Conservation (Tucson, AZ, USA: University Arizona Press).
Cohen P. J., Allison E. H., Andrew N. L., Cinner J., Evans L. S., Fabinyi M., et al. (2019). Securing a Just Space for Small-Scale Fisheries in the Blue Economy. Front. Mar. Sci. 6–171. doi: 10.3389/fmars.2019.00171
Cortes E., Neer J. A. (2006). Preliminary Reassessment of the Validity of the 5% Fin to Carcass Weight Ratio for Sharks. ICCAT Collective Volume Sci. Papers 59 (3), 1025–1036.
Costello C., Cao L., Gelcich S., Cisneros-Mata M. Á., Free C. M., Froehlich H. E., et al. (2020). The Future of Food From the Sea. Nature 588, 95–100. doi: 10.1038/s41586-020-2616-y
Costello C., Ovando D., Hilborn R., Gaines S. D., Deschenes O., Lester S. E. (2012). Status and Solutions for the World’s Unassessed Fisheries. Science 338 (6106), 517–520. doi: 10.1126/science.1223389
Cota-Nieto J. J., Erisman B., Aburto-Oropeza O., Moreno-Báez M., Hinojosa-Arango G., Johnson A. F. (2018). Participatory Management in a Small-Scale Coastal Fishery - Punta Abreojos, Pacific Coast of Baja California Sur, Mexico. Regional Stud. Mar. Sci. 18, 68–79. doi: 10.1016/j.rsma.2017.12.014
Dempsey J., Irvine-Broque A., Bigger P., Christiansen J., Muchhala B., Nelson S., et al. (2021). Biodiversity Targets Will Not be Met Without Debt and Tax Justice. Nat. Ecol. Evol. 6, 237–239. doi: 10.1038/s41559-021-01619-5
Duarte C. M., Agusti S., Barbier E., Britten G. L., Castilla J. C., Gattuso J.-P., et al. (2020). Rebuilding Marine Life. Nature 580, 39–51. doi: 10.1038/s41586-020-2146-7
Fai A. H., Cornelius P. L. (1996). Approximate F-Tests of Multiple Degree of Freedom Hypotheses in Generalised Least Squares Analyses of Unbalanced Split-Plot Experiments. J. Stat. Comput. Simulation 54 (4), 363–378. doi: 10.1080/00949659608811740
FAO (Food and Agriculture Organization of the United Nations). (2017). Workshop on Improving Our Knowledge on Small-Scale Fisheries: Data Needs and Methodologies (Rome, Italy: FAO Fisheries and Aquaculture Proceedings No. 55).
FAO (Food and Agriculture Organization of the United Nations) (2018). The State of the World Fisheries and Aquaculture (SOFIA) 2018. Eds. Alder J., Barg U., Funge-Smith S., Mannini P., Taconet M., Plummer J., Barange M. (Rome, Italy: FAO Secretariat). Available at: http://www.fao.org/publications/sofia/en/.
FAO (Food and Agriculture Organization of the United Nations) (2022). Fishery and Aquaculture Country Profiles - Mexico. Country Profile Fact Sheets (Rome, Italy: Fisheries and Aquaculture Division). Available at: https://www.fao.org/fishery/en/facp/mex?lang=en.
Ferrer E. M., Aburto-Oropeza O., Jimenez-Esquievel V., Cota-Nieto J., Mascareñas-Osorio I., López-Sagástegui C. (2021). Mexican Small-Scale Fisheries Reveal New Insights Into Low-Carbon Seafood and “Climate-Friendly” fisheries management. Fisheries 46 (6), 277–287. doi: 10.1002/fsh.10597
Fox J., et al. (2020) Effect Displays for Linear, Generalized Linear, and Other Models. Available at: https://cran.r-project.org/web/packages/effects/index.html.
Free C. M., Thorson J. T., Pinsky M. L., Oken K. L., Wiedenmann J., Jensen O. P. (2019). Impacts of Historical Warming on Marine Fisheries Production. Science 363, 979–983. doi: 10.1126/science.aau1758
Froese R., Demirel N., Coro G., Kleisner K. M., Winker H. (2017). Estimating Fisheries Reference Points From Catch and Resilience. Fish Fisheries 18, 506–526. doi: 10.1111/faf.12190
Gallo N. D., Victor D. G., Levin L. A. (2017). Ocean Commitments Under the Paris Agreement. Nat. Clim. Change 7, 833–838. doi: 10.1038/nclimate3422
Giron-Nava A., Johnson A. F., Cisneros-Montemayor A. M., Aburto-Oropeza O. (2019). Managing at Maximum Sustainable Yield Does Not Ensure Economic Well-Being for Artisanal Fisher. Fish Fisheries 20 (2), 214–223. doi: 10.1111/faf.12332
Giron-Nava A., Lam V. W. K., Aburto-Oropeza O., Cheung W. W. L., Halpern B. S., Sumaila R. U., et al. (2021). Sustainable Fisheries are Essential But Not Enough to Ensure Well-Being for the World’s Fishers. Fish Fisheries 22 (4), 812–821. doi: 10.1111/faf.12552
Gómez S., Maynou F. (2021). Balancing Ecology, Economy and Culture in Fisheries Policy: Participatory Research in the Western Mediterranean Demersal Fisheries Management Plan. J. Environ. Manage. 291 (112728), 1–12. doi: 10.1016/j.jenvman.2021.112728
Greer K., Zeller D., Woroniak J., Coulter A., Winchester M., Palomares M. L. D., et al. (2019). Global Trends in Carbon Dioxide (CO2) Emissions From Fuel Combustion in Marine Fisheries From 1950 to 2016. Mar. Policy 107 (103382), 1–9. doi: 10.1016/j.marpol.2018.12.001
Hilborn R., Banobi J., Hall S. J., Pucylowski T., Walsworth T. E. (2018). The Environmental Cost of Animal Source Foods. Front. Ecol. Environ. 16 (6), 329–335. doi: 10.1002/fee.1822
Hilborn R., Amoroso R. O., Anderson C. M., Baum J. K., Branch T. A., Costello C., et al. (2020). Effective Fisheries Management Instrumental in Improving Fish Stock Status. Proc. Natl. Acad. Sci. 11 (4), 2218–2224. doi: 10.1073/pnas.1909726116
IPBES (Intergovernmental Panel on Biodiversity and Ecosystem Services) (2019). Summary for Policy Makers of the Global Assessment Report on Biodiversity and Ecosystem Services of the Intergovernmental Science-Policy Platform on Biodiversity and Ecosystem Services. Eds. Brondizio E. S., Settele J., Díaz S., Ngo H. T. (Bonn, Germany: IPBES Secretariat). Available at: https://ipbes.net/sites/default/files/ipbes_7_10_add.1_en_1.pdf.
IPCC (2022). “Summary for Policymakers,” in Climate Change 2022: Impacts, Adaptation, and Vulnerability. Contribution of Working Group II to the Sixth Assessment Report of the Intergovernmental Panel on Climate Change. Eds. Pörtner H.-O., Roberts D. C., Poloczanska E. S., Mintenbeck K., Tignor M., Alegría A., Craig M., Langsdorf S., Löschke S., Möller V., Okem A. (Cambridge, United Kingdom: Cambridge University Press).
Juárez A. (2021) How a Community-Based Fishery Program is Bringing Sustainability to Mexico’s Upper Gulf of California. Environmental Defense Fund Blog. Available at: https://blogs.edf.org/edfish/2021/04/20/how-a-community-based-fishery-program-is-bringing-sustainability-to-mexicos-upper-gulf-of-california/.
Kearny J. (2010). Food Consumption Trends and Drivers. Philos. Trans. R. Soc. B 365, 2793–2807. doi: 10.1098/rstb.2010.0149
Kuznetsova A., Brockhoff P. B., Christensen R. H. B. (2017). Lmertest Package: Tests in Linear Mixed Effects Models. J. Stat. Softw. 82 (13), 1–26. doi: 10.18637/jss.v082.i13
Lluch-Cota S. E., Aragón-Noriega E. A., Arreguín-Sánchez F., Aurioles-Gamboa D., Bautista-Romero J. J., Brusca R. C., et al. (2007). The Gulf of California: Review of Ecosystem Status and Sustainability Challenges. Prog. Oceanogr. 73, 1–26. doi: 10.1016/j.pocean.2007.01.013
Mariani G., Cheung W. W. L., Lyet A., Sala E., Mayorga J., Velez L., et al. (2020). Let More Big Fish Sink: Fisheries Prevent Blue Carbon Sequestration—Half in Unprofitable Areas. Sci. Adv. 6 (44), 1–8. doi: 10.1126/sciadv.abb4848
Mascareñas-Osorio I., Cota-Nieto J. J., Jimenez V., Lopez-Sagastegui C. (2017)Fisheries Monitoring Program: Gulf of California Artisanal Fisheries Data. In: Datamares: Fisheries (University of California – San Diego Library Digital Collections) (Accessed April, 2018).
Mascareñas-Osorio I., Giron-Nava A., Aburto-Oropeza O. (2018)Mexico’s National Fisheries Statistics. In: Datamares: Fisheries (University of California – San Diego Library Digital Collections) (Accessed May, 2021).
Natural Resources Canada (2014) Learn the facts: fuel consumption and CO2. Available at: https://bit.ly/3kWJEYf.
Nijdam D., Rood T., Westhoek H. (2012). The Price of Protein: Review of Land Use and Carbon Footprints From Assessments of Animal Food Products and Their Substitutes. Food Policy 37 (6), 760–770. doi: 10.1016/j.foodpol.2012.08.002
Parker R. W. R., Blanchard J. L., Gardner C., Green B. S., Hartmann K., Tyedmers P. H., et al. (2018). Fuel Use and Greenhouse Gas Emissions of World Fisheries. Nat. Climate Change 8, 333–337. doi: 10.1038/s41558-018-0117-x
Parker R. W. R., Tyedmers P. H. (2015). Fuel Consumption of Global Fishing Fleets: Current Understanding and Knowledge Gaps. Fish Fisheries 16 (4), 684–696. doi: 10.1111/faf.12087
Pauly D., Christensen V., Dalsgaard J., Froese R., Torres F. Jr., et al. (1998). Fishing Down Marine Food Webs. Science 279 (5352), 860–863. doi: 10.1126/science.279.5352.860
Pauly D., Zeller D. (2016). Catch Reconstructions Reveal That Global Marine Fisheries Catches Are Higher Than Reported and Declining. Nat. Comm. 7 (10244), 1–9. doi: 10.1038/ncomms10244
Pershing A. J., Alexander M. A., Hernandez C. M., Kerr L. A., Le Bris A., Mills K. E., et al. (2015). Slow Adaptation in the Face of Rapid Warming Leads to Collapse of the Gulf of Maine Cod Fishery. Science 350, 809–812. doi: 10.1126/science.aac9819
Purcell S. W., Lalavanua W., Cullis B. R., Cocks N. (2018). Small-Scale Fishing Income and Fuel Consumption: Fiji’s Artisanal Sea Cucumber Fishery. ICES J. Marine Sci. 75 (5), 1758–1767. doi: 10.1093/icesjms/fsy036
R Core Team (2020). R: A Language and Environment for Statistical Computing (Vienna, Austria: R Foundation for Statistical Computing). Available at: https://www.R-project.org/.
Rousseau Y., Watson R. A., Blanchard J. L., Fulton E. A. (2019). Evolution of Global Marine Fishing Fleets and the Response of Fished Resources. Proc. Natl. Acad. Sci. 116 (25), 12238–12243. doi: 10.1073/pnas.1820344116
Sáenz-Arroyo A., Roberts C. M., Torre J., Cariño-Olvera M., Enríquez-Andrade R. R. (2005). Rapidly Shifting Environmental Baselines Among Fishers of the Gulf of California. Proc. R. Soc. B 272, 1957–1962. doi: 10.1098/rspb.2005.3175
Schuhbauer A., Chuenpagdee R., Chueng W. W. L., Greer K., Sumaila U. R. (2017). How Subsidies Affect the Economic Viability of Small-Scale Fisheries. Mar. Policy 82, 114–121. doi: 10.1016/j.marpol.2017.05.013
Smith H., Basurto X. (2019). Defining Small-Scale Fisheries and Examining the Role of Science in Shaping Perceptions of Who and What Counts: A Systematic Review. Front. Mar. Sci. 6 (236). doi: 10.3389/fmars.2019.00236
Sumaila U. R., Cheung W., Dyck A., Gueye K., Huang L., Lam V., et al. (2012). Benefits of Rebuilding Global Marine Fisheries Outweigh Costs. PLoS One 7 (7), 1–12. doi: 10.1371/journal.pone.0040542
Sumaila U. R., Skerritt D. J., Schuhbauer A., Villasante S., Cisneros-Montemayor A. M., Sinan H., et al. (2021). WTO Must Ban Harmful Fisheries Subsidies. Science 374 (6567), 544–544. doi: 10.1126/science.abm1680
Sumaila U. R., Tai T. C. (2020). End Overfishing and Increase the Resilience of the Ocean to Climate Change. Front. Mar. Sci. 7 (523). doi: 10.3389/fmars.2020.00523
The World Bank (2017). The Sunken Billions Revisited: Progress and Challenges in Global Marine Fisheries (Washington, DC: World Bank). doi: 10.1596/978-1-4648-0919-4
The World Bank with FAO, WorldFish, and ARD (2012) Hidden Harvest Report: The Global Contribution of Capture Fisheries. Available at: http://documents.worldbank.org/curated/en/515701468152718292/pdf/664690ESW0P1210120HiddenHarvest0web.pdf.
Turner S. J., Thrush S. F., Hewitt J. E., Cummings V. J., Funnell G.. (1999). Fishing Impacts and the Degradation of Loss of Habitat Structure. Fisheries Manage. Ecol. 6, 401–420. doi: 10.1046/j.1365-2400.1999.00167.x
Vázquez-Rowe I., Hospido A., Moreira M. T., Feijoo G. (2012). Best Practices in Life Cycle Assessment Implementation in Fisheries. Improving and Broadening Environmental Assessment for Seafood Production Systems. Trends Food Sci. Technol. 28, 116–131. doi: 10.1016/j.tifs.2012.07.003
Keywords: artisanal fisheries, carbon footprint, fuel intensity, management, maximum sustainable yield, Mexico, overfishing, small-scale fisheries
Citation: Ferrer EM, Giron-Nava A and Aburto-Oropeza O (2022) Overfishing Increases the Carbon Footprint of Seafood Production From Small-Scale Fisheries. Front. Mar. Sci. 9:768784. doi: 10.3389/fmars.2022.768784
Received: 01 September 2021; Accepted: 13 May 2022;
Published: 11 July 2022.
Edited by:
Steven W. Purcell, Southern Cross University, AustraliaReviewed by:
Asuncion Bina De Guzman, Independent researcher, Naawan, PhilippinesCharlotte De Fontaubert, World Bank Group, United States
Copyright © 2022 Ferrer, Giron-Nava and Aburto-Oropeza. This is an open-access article distributed under the terms of the Creative Commons Attribution License (CC BY). The use, distribution or reproduction in other forums is permitted, provided the original author(s) and the copyright owner(s) are credited and that the original publication in this journal is cited, in accordance with accepted academic practice. No use, distribution or reproduction is permitted which does not comply with these terms.
*Correspondence: Erica M. Ferrer, ZW1mZXJyZXJAdWNzZC5lZHU=