- 1Interdisciplinary Centre of Marine and Environmental Research (CIIMAR/CIMAR), University of Porto, Porto, Portugal
- 2Centre of Territory, Environment and Construction (CTAC), Department of Civil Engineering, University of Minho, Braga, Portugal
- 3Faculty of Engineering, University of Porto, Porto, Portugal
- 4Department of Geosciences Environment and Spatial Planning, Faculty of Sciences, University of Porto, Porto, Portugal
Numerical models are essential tools for the study and analysis of the hydrodynamics of estuarine systems. However, the model results contain uncertainties, which need to be minimized to increase the accuracy of predictions. In this work, the ensemble technique is proposed as a solution to improve hydrodynamic forecasts for estuarine regions. Two numerical models, openTELEMAC-MASCARET and Delft3D, were considered for the application of this technique to two Portuguese estuaries. Superensembles for three scenarios (summer, winter, and extreme event) were built to assess the effectiveness of the technique in improving water level prediction. Various weighing techniques were tested in the construction of the ensembles. Weighing techniques that consider the previous performance of each model alone outperformed other techniques. This was observed for all scenarios considered, at all sampling points and in both studied estuaries. The effect of the ensemble size was also analyzed. It was found that the size of the set is directly related to the prediction accuracy, with the best results provided by the superensembles with the highest number of elements. It is concluded that the combined use of several hydrodynamic models reduces the uncertainty of the results and increases the reliability and consistency of predictions for estuarine regions.
Introduction
Coastal regions, particularly the estuarine areas, are of strategic importance from an environmental, economic, and social point of view. They provide multiple ecosystem services, such as fishery and other food resources, leisure and tourism, energy, water, and raw materials. Coasts are generally densely populated and highly urbanized. Approximately 40% of the world population lives at a distance less than 100 km from the coast, and that percentage is increasing. This implies an increase in economic activities, coastal infrastructures, and urban intensification (Dangendorf et al., 2012; IPCC, 2012; Hallegatte et al., 2013; Moser et al., 2014; Bell et al., 2018). Estuaries are particularly dynamic coastal systems, with strong variations in salinity, currents, stratification, and water quality. They are subject to the influence of meteo-oceanographic and geomorphological phenomena such as wind, tides, waves, currents, river flows, transport of sediments, erosion, and accretion. In terms of ecosystems, they are highly productive, serving as habitats for numerous species. They usually present vast urban areas due to their privileged situation in terms of resources and accessibility. The massive occupation entails the artificialization of the banks, which leads to a loss of biodiversity, affecting both the physical and environmental properties of the water/land interface. This increases the vulnerability of the system and, therefore, the risk to populations and their assets, with adverse effects on the economy (Peixoto, 2016). Considering the current context of climate change, an increase in the frequency and intensity of extreme events is expected (IPCC, 2012), with serious consequences for society and the environment, and with impacts on populations, infrastructures, habitats, and ecosystem services (Vose et al., 2014; Bell et al., 2018).
There is, therefore, an urgent need for accurate scientific and technical information to support sustainable coastal management, to reduce the exposure and vulnerability of estuarine areas, to mitigate the risks associated with different climate scenarios and to promote the adaptation and the resilience of communities to potential adverse impacts (Coelho et al., 2009; Veloso-Gomes, 2016). To provide this accurate information, it is necessary to understand, in a comprehensive way, all the hydrodynamic processes that take place in estuaries, and their interactions with anthropogenic activities and ecosystems. In situ measurements can help to understand these phenomena, enabling the representation of the current state of the systems and their main historical evolutionary trends. However, obtaining this type of data is not simple, as it requires detailed planning and constant monitoring, which is not always feasible. As a consequence, there is a huge lack of continuous and long-term observations. The limited knowledge, related to a lack of systematic monitoring, in combination with the complexity of estuarine systems, leads to a high degree of uncertainty about future scenarios and the effects they will have on populations and ecosystems (Iglesias et al., 2020b).
Numerical models are valuable tools that make it possible to overcome shortcomings in information (Horritt and Bates, 2002; Liu et al., 2008; Chen et al., 2015a,b; van Maren et al., 2015; Pereira, 2016). The efforts of the scientific community and the developments in computational techniques allowed to increase the simulation capacity, improve the accuracy and resolution of the numerical models and reduce the computational processing time. Numerical simulation of hydrodynamic processes in estuarine systems is essential to evaluate the effects of forcing terms, through the imposition of dynamic boundary conditions, the inclusion of topo-bathymetric characteristics, and the presence of anthropic structures (Bastos et al., 2016; Teng et al., 2017). However, each numerical model has its own strengths and simplifications, and its results and uncertainties are dependent on many factors, such as its conceptual framework, approach to physical processes, initial and boundary conditions, and horizontal and vertical spatial resolutions. The assumptions made to define and quantify those factors are also a relevant source of the uncertainty associated with a model (Feng et al., 2011).
Next to the natural randomness associated with the temporal and spatial variation of natural processes, there are other sources of numerical model uncertainties. They can result from model initialization, due to, for example, incomplete required data, measurement errors or errors in data processing, or reside in the model itself, due to imprecise parametrization of physical processes, inaccuracies in the definition of parameters, the existence of unresolved scales, or errors in the boundary conditions (Palmer, 2003; Buizza et al., 2005; Weigel et al., 2008). Uncertainties in the construction and application of these numerical models can be grouped into: initial conditions uncertainties, model boundary conditions and model parameters uncertainties, and structural uncertainties (Tebaldi and Knutti, 2007). Furthermore, it must not be forgotten that any attempt to represent nature in a set of equations will always be a simplification of reality that inevitably introduces uncertainties. Although the partial differential equations that govern estuarine hydrodynamic phenomena are well known, they must be truncated to a finite-dimensional set of linear equations to be computationally integrated and solved. The uncertainties associated with this process can propagate and produce effects across the entire spectrum of scales predicted by the model (Palmer et al., 2004; Hagedorn et al., 2005). To produce a perfect forecast, a sound knowledge of each process and state of the system would be needed, and this should be perfectly implemented in the model. However, this can only be done in an approximate way, as even the actual quantification of the system is accompanied by inherent uncertainties. All uncertainties, even minor ones, propagate through the model processes, adding up and eventually leading to a large forecast error (Mohan Das et al., 2017; Deutscher Wetterdienst, 2021). The need for accurate forecasts, where errors of this type are minimized, highlights the importance of finding and implementing new solutions to deliver better numerical results. In this context, ensemble modeling is considered as one of the best solutions because it can minimize the combined uncertainty in input data, model parameters and model structure, improving the performance of the models (Viney et al., 2009; Suh et al., 2012; Mohan Das et al., 2017).
Given that the ensemble technique has not yet been fully implemented for the assessment of estuarine hydrodynamics, the main objective of this study is the implementation and testing of an ensemble technique for estuarine regions to improve hydrodynamic forecasts and demonstrate its effectiveness in reducing the uncertainty of the results. The technique will be selected and tested considering various scenarios, different estuarine regions, diverse locations inside the estuaries and several statistical techniques for ensemble construction. In the following sections we describe the approach used for modeling the hydrodynamics of the Douro and Minho rivers estuaries using an ensemble technique.
The Ensemble Technique
A numerical model ensemble is the combination of several numerical model simulations using statistical methods. This technique consists of running different numerical models of the same natural system, or running the same model considering changes in key input parameters. The obtained results are synthesized in a single result that presents smaller bias and variance than the individual solutions, thus improving the accuracy, reliability and consistency of the final prediction (Tebaldi and Knutti, 2007; Re and Valentini, 2010). It is a technique widely applied in atmospheric and climate sciences for weather, seasonal, interannual and climate change forecasting (Gneiting and Raftery, 2005; Weigel et al., 2008; Feng et al., 2011; WMO, 2012; IPCC, 2021). Other scientific domains where ensembles techniques have been applied are hydrology (Ajami et al., 2006), morphodynamics (Thornhill et al., 2012), geography and remote sensing (Benediktsson et al., 2007), wave and coastal forecasting (Yang-Ming et al., 2013; Kourafalou et al., 2015), public health (Thomson et al., 2006), agriculture (Cantelaube and Terres, 2005), information security (Menahem et al., 2009), astronomy and astrophysics (Bazell and Aha, 2001), finance (Leigh et al., 2002), medicine (Polikar et al., 2008) and bioinformatics (Re and Valentini, 2010). In estuarine hydrodynamics, this technique has not been fully adopted and most modeling studies are based on a single model. To the best of our knowledge, few works were carried out in this area. However, a recent interest is emerging (Mohan Das et al., 2017; Iglesias et al., 2019b; Taeb and Weaver, 2019; Dinápoli et al., 2021; Khanarmuei et al., 2021).
The uncertainty in forecasts was first introduced by Lorenz (1963, 1965), who examined the uncertainties of initial states, their effect on predictions, and the so-called butterfly effect. Centered on the atmosphere, Lorenz observed that this system is chaotic due to the non-linearity of its processes. He demonstrated that, no matter how good the observations or forecasting techniques are, there will be a non-transposable limit to how far into the future it is possible to make a forecast (Roy Bhowmik and Durai, 2008). After Lorenz’s studies, the idea of combining predictions from multiple models was explored for more than 30 years in econometrics and statistics (Bates and Granger, 1969; Dickinson, 1973; Newbold and Granger, 1974). The leap into the earth sciences was taken by Epstein (1969); Leith (1974) and Thompson (1977), who included the concept of ensembles in weather forecasting, considering the perspective of the randomness of atmospheric motion. However, it was not until the 1990s that the first ensemble prediction systems were implemented at the ECMWF and at the NCEP (Feng et al., 2011).
Therefore, for the case of predictions of future behavior, an ensemble generates a forecast based on a set of forecasts, minimizing the systematic bias that occurs when a single model solution is used (Roy Bhowmik and Durai, 2010). However, a combination of different forecasts is only useful if they disagree on some inputs or if they are generated by different models. There is no gain of information from a combination of identical models with identical results (Krogh and Vedelsby, 1995). The numerical models that are members of the set should therefore include different initial conditions, boundary conditions, parameterizations, model structures, or some combination of them, to reflect the uncertainty (Buizza et al., 2005; Demeritt et al., 2007). The ensemble average of these different solutions yields a more accurate forecast than the individual forecasts of each ensemble member, while the ensemble dispersion provides quantitative information about forecast uncertainty (Wandishin et al., 2001).
Two main types of ensembles can be distinguished: those that use results from a single model and those that are computed with multiple models. Ensembles composed of a single model combine several runs with different initial and/or boundary conditions. It has long been accepted that running an ensemble of numerical forecasts from perturbed initial conditions can have a beneficial impact on prediction ability (Yang-Ming et al., 2013). The multimodel ensemble uses several numerical models with different complexities and structures that are run using similar initial and boundary conditions (Iglesias et al., 2019b,2020b).
Using several models in an ensemble is a way to take into account our lack of knowledge about the system that is being modeled, as different models make different assumptions and perform differently (Palmer et al., 2004; Doblas-Reyes et al., 2010). If multiple initial conditions are used for each model, the multimodel ensemble is a superensemble (Tebaldi and Knutti, 2007). A superensemble presents advantages when compared with a multimodel ensemble. A multimodel ensemble reduces the random component in model errors but does not provide any reduction in the initial condition uncertainty, which is taken into account in the superensemble (Doblas-Reyes et al., 2010). Furthermore, some models may provide better predictions for some conditions than for others. For example, a model might provide better predictions for summer conditions than for winter conditions. Thus better overall forecasts can be obtained using combinations of different models for different scenarios, also known as conditional ensembling (Viney et al., 2009).
Although the results of past studies are dependent on ensemble size and region, one can state that ensembles forecasts that use multiple models usually outperform single model deterministic forecasts and often outperform any combination of solutions from a single model (Krishnamurti et al., 1999; Georgakakos et al., 2004; Hagedorn et al., 2005; Palmer et al., 2005; Tebaldi and Knutti, 2007). However, and although it seems that increasing the sample size of the ensemble by pooling information from different models presumably offers a reduction in the variance of the mean of the forecast, it is not clear that such a strategy will always be effective. This is due to the linear and non-linear biases in each model (Rajagopalan et al., 2002). The ensemble’s So, its suitability has thus to be tested for estuarine hydrodynamic models.
There is a general agreement on the fact that the combination of several different forecasts provides significant improvements in the solutions. After running the models, the computed forecasts are usually collapsed into a consensus forecast or ensemble mean. There are many possible methods to obtain an ensemble result (Clemen, 1989). However, it is not clear which is the ideal method for different forecasting problems (Thomson et al., 2006; Rozante et al., 2014). Several statistical techniques can be used that can be categorized into three types: simple composite methods, weighted ensemble methods, and synthetic methods (Suh et al., 2012). The simplest technique is to use the median or the arithmetic mean, which has the effect of filtering out features of the forecast that are less predictable while retaining those features that show agreement among the members of the ensemble (Yang-Ming et al., 2013). This technique is usually selected when no in situ observations exist for comparison. However, different numerical models often have different simulation capabilities, and a variable may be better represented by one model and worse by another. To improve the ensemble solution, the predictive capability of each model should be considered as it makes sense to attribute more weight to the better performing models (Tebaldi and Knutti, 2007). Nevertheless, there is some controversy about the best way to combine the results of the models, and some previous studies in the literature found no evidence that the weighted average system provides results closer to observation than the establishment of equal or random weights (Déqué and Somot, 2010). In a weighted average method, a weighting coefficient is allocated to each model according to its forecasting performance as a single model (Feng et al., 2011). These coefficients are obtained by comparing numerical results with field observations, and generally evolve from metrics such as mean squared errors, correlation coefficients or multiple linear regressions (Woodcock and Engel, 2005; Chakraborty and Krishnamurti, 2006; Roy Bhowmik and Durai, 2010). Other more complex methods include linear regressions, non-linear regressions, principal component regression, singular value decomposition, composites, linear programming, multimodel superensembles, Supra Bayesian method or neural networks (Krishnamurti et al., 1999; Ajami et al., 2006; Roy Bhowmik and Durai, 2008, Roy Bhowmik and Durai, 2010; Viney et al., 2009; Feng et al., 2011; Kumar et al., 2012; WMO, 2012; Rozante et al., 2014; Mohan Das et al., 2017; Bernhofen et al., 2018). However, as stated by Clemen (1989), simple combinations often outperform more complex methods.
Geographical Settings
For the application of the ensembles technique, the estuaries of the rivers Minho and Douro in northern Portugal were chosen (see Figure 1). Despite being geographically close and having similar seasonal flow regimes (minimum in summer and maximum in winter), these estuaries have distinct dynamics and environmental conditions. They differ in the river average and peak discharges, as well as in terms of morphology, bathymetry, length, and banks configuration and level of urbanization (Iglesias et al., 2020b).
The river Minho reaches the Atlantic Ocean between A Guarda (Spain) and Caminha (Portugal). Its 40 km-long shallow estuary has an average depth of 4 m, although depths around 20 m can be found along stretches where the narrowing of the main channel increases current velocities and, consequently, erosion (Freitas et al., 2009; Reis et al., 2009). The freshwater inflow is controlled by the Frieira dam, located 80 km upstream from the mouth. Compared to the river Douro, the river Minho has lower flow rates, which increase the water residence time up to 1.5 days (Ferreira et al., 2005). This long residence time, in conjunction with the small estuarine area and relatively low water volumes, make the estuary vulnerable to pollutants, which can endanger its great diversity of habitats (Domínguez García et al., 2013; Ribeiro et al., 2016). It is a partially mixed estuary, where vertical stratification can occur due to a salt wedge configuration (Baeta et al., 2017). Its dynamics is strongly influenced by the tide, with a direct effect on sediment transport. The lower part of the estuary, near the mouth, presents a widening that results in a decrease in current velocity, creating favorable conditions for the deposition of sediments (Delgado et al., 2011; Portela, 2011; Melo et al., 2020). The morphodynamic patterns due to silting have caused restrictions to navigation and the appearance of islands and sandbanks during low tide (Zacarias, 2007; Reis et al., 2009; Santos et al., 2021). This fact is most notable in spring low-tide conditions, when the connection between the estuary and the sea is restricted to two shallow channels (Iglesias et al., 2019a,Iglesias et al., 2020b).
The river Douro flows into the Atlantic Ocean through an estuary surrounded by two large cities: Porto and Vila Nova de Gaia. The estuary is relatively narrow and highly dynamic, with torrential regimes that produce strong currents and recurrent floods (Bastos et al., 2012; Iglesias et al., 2020a). It has an irregular bathymetric configuration, with depths between 0 and 10 m, and some deeper regions, up to 28 m-deep, located at narrower sections, outer bends, and former sites of sediments extraction (Portela, 2008). Its dynamics is conditioned by the river flow, being highly dependent both on natural conditions and on the hydroelectric power generation schedules at the upstream Crestuma-Lever dam, which limits the estuary to 21 km in length. On the southern bank of the estuarine mouth, there is a wetland and a sand spit that partially obstructs the entrance and protects the estuary. This sand spit, formed by fluvial and maritime sediments, is conditioned by natural (wind, rain, river flows, waves, tides, and storms) and anthropic (construction of breakwaters and dams, sand extraction, and dredging) processes (Santos et al., 2010; Granja et al., 2011). In the past, this sand spit suffered erosion and a slow migration toward the interior and to the north of the estuary, partially occupying the navigation channel. To ensure safe navigation, the northern breakwater at the inlet was extended and a new detached breakwater was built. These structures, completed in 2008, interfere with the hydromorphodynamic patterns, silting up the wetland and significantly increasing the sand spit area and volume in a relatively short period (∼10 years) (Bastos et al., 2012). Historical records show ruptures of this spit during flood episodes, allowing for a rapid water discharge and reducing the risk of urban flooding. Its current configuration, more stable and robust, reduces the probability of rupture so that more severe effects are expected in terms of economic losses and structural damage during floods (Iglesias et al., 2019b).
Materials and Methods
The numerical models for the ensemble were implemented using the openTELEMAC-MASCARET (OTM) and the Delft3D (D3D) modeling suites. They solve similar formulations of the shallow water equations, considering several physical processes such as tidal forcing, tidal flats, river discharges, the rotation of the Earth, bottom friction, turbulence, sub- and supercritical flows, and water density effects (Jones and Davies, 2010; Robins and Davies, 2010; Monteiro et al., 2011; van Maren et al., 2015). These two packages were selected because they have shown to be able to accurately simulate the hydrodynamic behavior in estuarine areas, to assess the flooding risk, and to quantify the effects of climate change (Corti and Pennati, 2000; Horritt and Bates, 2002; Gomes et al., 2015; Putra et al., 2015). These OTM and D3D models were already calibrated for the Minho and Douro estuaries, and successfully demonstrated their ability to accurately represent these estuaries’ characteristic hydrodynamic patterns for both frequent and extreme events (Iglesias et al., 2019b,2020b,2021; Melo et al., 2020; Weber de Melo et al., 2022).
The ensembles were based on simulations performed with OTM and D3D modeling suites. These ensemble hindcasts, performed for a training period, were compared with historical in situ data, indicating which statistical technique is the best. An ensemble obtained as a combination of two single outputs, one from each modeling suite, would produce little improvement compared to the outputs of the individual models, as they are insufficient to resolve the inherent uncertainty. Thus, superensembles are proposed to maximize the number of members of the ensembles A set of M modified initial conditions were considered to produce a superensemble hindcast for each estuary. As two modeling suites are used, there will be a total of 2 M ensemble members, and the superensemble hindcast will be the combination of the results of all these members.
Superensembles
The models were run for historical conditions to calibrate the ensemble with in situ measurements of the water elevation at several locations, namely: stations M1, M2, M3, and M4 for the Minho estuary, and stations D1, D2, D3, and D4 for the Douro estuary (see Figure 1). The consideration of all these stations will allow to inspect whether the technique’s performance varies spatially, i.e., depending on the measuring location. The simulations, run with both modeling suites, OTM and D3D, considered three scenarios that represent three different conditions: summer, winter, and extreme event (flooding) conditions (Table 1). Measuring station M2 was only available for the winter scenario.
A deterministic approach was adopted for this study. Numerical modeling simulations were run for a single day of 2006 to proceed with the calibration of the ensemble results with in situ data. This allows the maintenance of the characteristics of each selected scenario. Each model run consisted of a 24 h-long simulation with constant river flow and water elevation boundary conditions, preceded by a spin-up period of 3 h to avoid numerical instabilities. The water elevation modeling results for ensemble construction were extracted at the end of each performed simulation when the models are stable. Given the predominant wind direction, the small size of the estuaries and the close configuration of their mouths, atmospheric (wind and sea level pressure) and wave forcing were neglected. The scenarios and respective runs are summarized in Supplementary Tables 1–3.
A superensemble was built for each of the proposed scenarios. For each scenario, runs with different combinations of river flow and oceanic water elevation were considered because they are the principal hydrodynamic drivers in the considered estuaries. The river flow rate was included as: (a) registered value, (b) registered value plus standard deviation of the simulated month, and (c) registered value minus standard deviation of the simulated month, to include possible deviations associated with inaccuracies in the river flow measurements (cf. Table 1). Standard deviations were computed based on daily mean river flows measured at Foz do Mouro (1973–2020), for the Minho estuary, and hourly mean river flows at Crestuma-Lever (1998–2020), for the Douro estuary (cf. Table 1). The water level at the ocean boundary considered the (i) maximum and (ii) minimum tide levels registered on the simulation day, as well as the (iii) annual highest tide, (iv) highest spring tide, (v) highest neap tide, (vi) annual lowest tide, (vii) lowest spring tide, and (viii) lowest neap tide (cf. Table 2). Considering these different water levels in the ensemble construction will increase the number of ensemble members to avoid inaccuracies associated with tidal amplification and asymmetry inside the estuarine regions, as well as sudden changes in depth or inaccuracies in the bathymetry included in the numerical grids. For the extreme event, since extreme events are rare, with percentiles of non-exceedance above 95% (Iglesias et al., 2021), the standard deviation of the flow was not taken into account. An additional ocean elevation of 1.10 m, associated with a storm surge, was included for both estuaries (Gama et al., 1994; Almeida et al., 2009).
Conditional Ensembling
Besides the winter and summer superensembles with their 48 members (models’ results), conditional ensembling was considered to assess the effect of the ensemble size. The numerical solutions for summer and winter were grouped into the 24 highest and 24 lowest water elevation predictions. The 48-member superensembles were calibrated with the mean water elevation observed during the selected simulation day, whereas the results of the 24-member superensembles were compared with the maximum and minimum values registered at the measuring stations. The superensembles characteristics are summarized in Table 3.
Weighting Techniques
To construct the ensembles solutions, the following techniques were selected and evaluated: the simple model average (SMA), the members’ median (Med), the trimmed mean (Trim), and weighted average methods (WAM). For the WAM technique, three weightings were analyzed: a random weighting (WAM-Rand), the absolute error (WAM-AE) and the squared error (WAM-SE).
The SMA and the Med techniques are the simplest ones and will be used as benchmarks for comparison with more sophisticated techniques. They consist of the arithmetic average and of the median of the ensemble members. These techniques are widely used for ensembles construction because there is no need for comparisons between the models’ results and the observations (Ajami et al., 2006; Viney et al., 2009; Déqué and Somot, 2010; Weigel et al., 2010; Feng et al., 2011; Suh et al., 2012). If the SMA is applied to a single forecast of each model, the technique it is called a “poor man’s” ensemble (Du et al., 2018). In both SMA and Med techniques, all model outcomes have the same weight in the final forecast. This means that they are likely to include several poor models’ results, degrading the overall results (Roy Bhowmik and Durai, 2008, Roy Bhowmik and Durai, 2010).
For the Trim technique, the outliers, which are the largest and smallest values of the dataset that might affect the arithmetic mean, are removed. Subsequently a simple average of the remaining values is calculated. Several Trim combinations were computed, considering the 36–46 centermost predictions for summer and winter scenarios, the 20–30 centermost predictions for the extreme event scenario, and the 12–22 centermost predictions for the higher and lower predictions for the summer and winter scenarios. It is expected that Trim predictions lie somewhere between SMA and Med so that the Trim ensembles with more members approach SME forecasts while the Trim ensembles with fewer members approach Med forecasts (Viney et al., 2009).
In a WAM technique, the weighting coefficients are constrained to be always positive and their sum must be equal to one. They shall not require large quantities of data for their estimation and should distinguish between better and poorer models (Woodcock and Engel, 2005). The weighting coefficients need to be derived from comparisons with the observations, taking into consideration the simulation performance of each model through some statistical property of the model’s calibration predictions (Viney et al., 2009; Suh et al., 2012). Different models have in generally different simulation capacities. At the same time, they forecast capacity may vary depending on the analyzed variables, the seasons and the geographic regions. If the different capacities of the models are taken into account through an assignment of weights (as done in WAM), the ensemble results can be closer to the observations than those obtained with the arithmetic mean method (Feng et al., 2011).
Since the SMA technique can be seen as a WAM technique with an equal weight applied to each ensemble member, random weights were considered to investigate the effectiveness of the WAM methods. In the first random method, WAM-Rand1, the run with index m gets the weight:
being M the number of simulations that integrate the ensemble (Casanova and Ahrens, 2009). For the second random method, WAM-Rand2, the weights are random numbers with a uniform distribution between 0 and 1 that are then normalized (Déqué and Somot, 2010).
For the knowledge on the performance of the models to be incorporated in the construction of the ensemble, two more techniques were considered. The WAM-AE technique, based on the absolute error metric, ℒAE, and the WAM-SE technique based on the squared error metric, LSE.
The absolute error metric is defined as:
Where Xf and Xo are the forecasted and the observed values, respectively, of the variable X. In a similar way, the squared error metric is defined as
In the WAM-AE technique, the weight wm is given by:
Where M is the number of members in the ensemble, whereas in the WAM-SE technique, the weight wm is given by:
The performance of each model and ensemble was then evaluated comparing the results with the 2006 in situ observations of water elevation. Therefore, the AE (Equation 2) was selected as the evaluation score. A flow diagram outlining the methodology performed in this study is presented in Figure 2.
Results and Discussion
Prior to the superensemble construction, the results of the individual numerical models were analyzed. The scatter plots in Figure 3 showed a strong dispersion of results for all runs and for all scenarios. This was expected, given the wide range of variation imposed in the boundary conditions (Tables 1, 2 and Supplementary Tables 1–3). Stronger dispersion was observed for the summer and winter scenarios (cf. Figures 3A,B,D,E) due to the wider range of adopted conditions when compared to the extreme event scenario (cf. Figures 3C,F), but also because, during extreme flooding events, the water level inside the estuaries is only weakly affected by any variation of the ocean tide level. Even so, the dispersion is larger for the summer scenarios than for the winter scenarios.
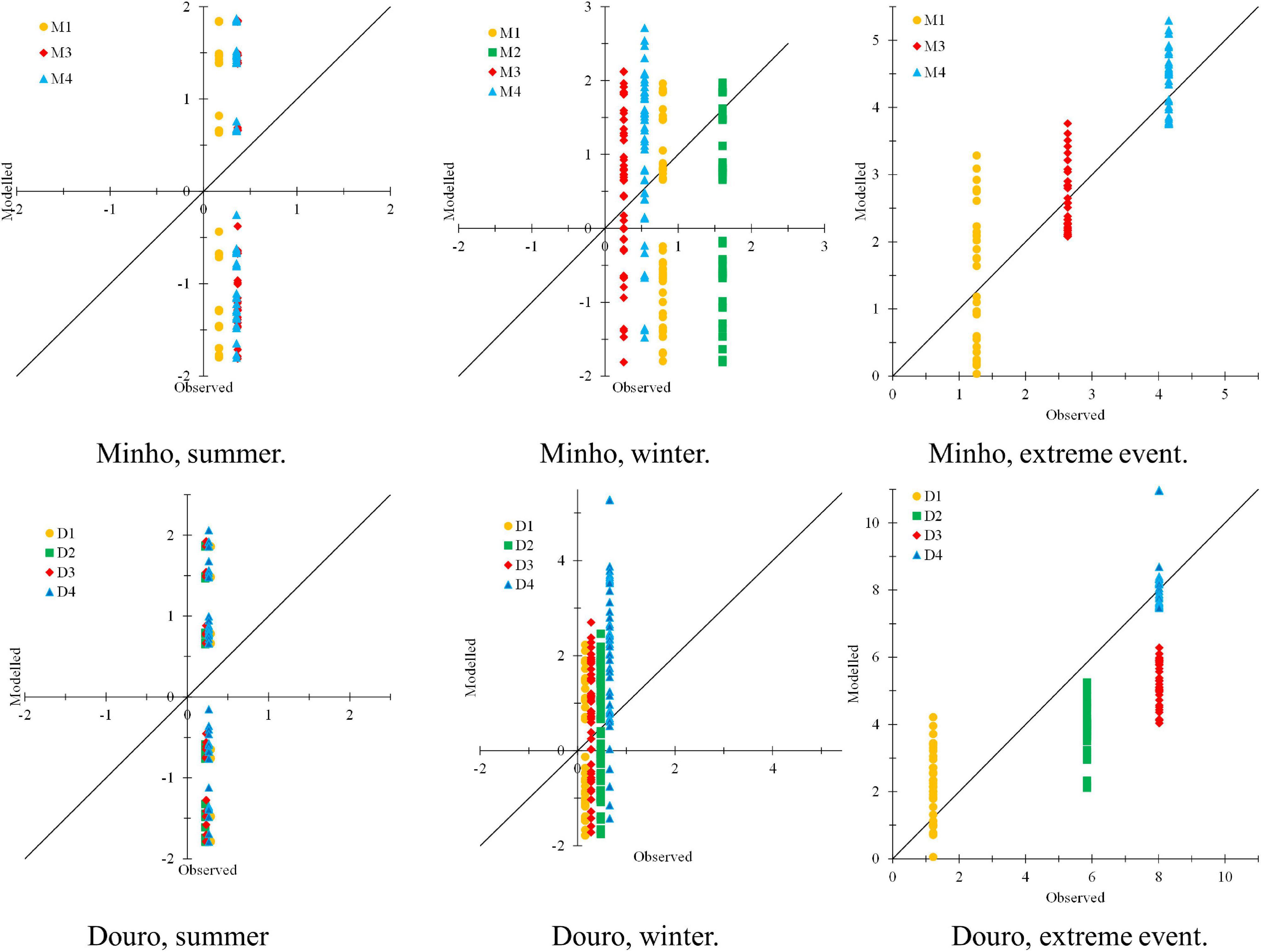
Figure 3. Scatter plots of the water elevation (in meters, referred to the MSL) for the Minho and Douro estuaries. The black line represents the perfect hindcast.
The summer scenario runs were forced with low river flows, causing the tide to become the main driver of the estuarine dynamics. For each run, the measured water levels show little variability along the length of the estuaries (cf. Figures 3A,D). The modeled water levels at both estuaries show a much wider variation due to the variations in the tide level among the various runs. Nevertheless, none of the runs provides a good solution, given that no solution is on the line of the perfect hindcast.
For the winter scenario, the differences between the upstream and downstream sampling stations are more pronounced (cf. Figures 3B,E). The highest elevations for the Minho estuary were not recorded at the upstream station M3, but at the intermediate station M2, probably due to the configuration of the estuary. Again, the modeled solutions for both estuaries feature a wide range of water levels, and for every station, there is at least one close-to-ideal solution. This means that the river flow mechanism that drives the hydrodynamic circulation during winter scenarios is better represented by the numerical models than the tide. Inaccuracies in the tidal water elevation could be related with uncertainties in the model grid, due to imprecisions in bathymetry and topography data, or inaccuracies in the definition of the models coefficients, affecting the representation of the tidal amplification and asymmetry inside the estuarine regions.
For the extreme event scenario (Figures 3C,F), the highest water levels were registered at the upstream sampling stations (M4 and D4), reaching 4 m at the Minho estuary and 8 m at the Douro estuary. As expected, the modeled solutions feature a wider range of water levels due to variations in river flow between the various runs. For the Minho estuary, there are close-to-ideal solutions for all stations. A different situation is found for in the Douro estuary, at stations D2 and D3, where the water level is always underestimated. This could be related with uncertainties in the model grid because D2 and D3 are located on the estuarine margins.
D3D hindcasts displayed higher errors, with a global average minimum error of 0.383 m, while the global average minimum error for OTM hindcasts was 0.299 m (cf. Table 4). For winter and extreme event scenarios, OTM outperformed D3D with average minimum errors of 0.145 and 0.093 m, for winter, and 0.649 and 0.444 m, for extreme event. However, for the summer scenario, D3D’s and OTM’s performances are similar, with an average minimum error of 0.389 and 0.388 m, respectively. Regarding the estuary, the Minho estuary presented more accurate hindcasts, with a global average minimum error of 0.191 and 0.153 m, for D3D and OTM, respectively. Global average minimum errors for the Douro were 0.543 and 0.420 m (Table 4). Although small, the differences between the OTM and D3D errors could be related with the numerical grid implemented for each modeling suite. While D3D uses a regular curvilinear gird, OTM uses an unstructured irregular computation grid of triangular elements. This unstructured grid suits the shape of the estuaries better, providing better results.
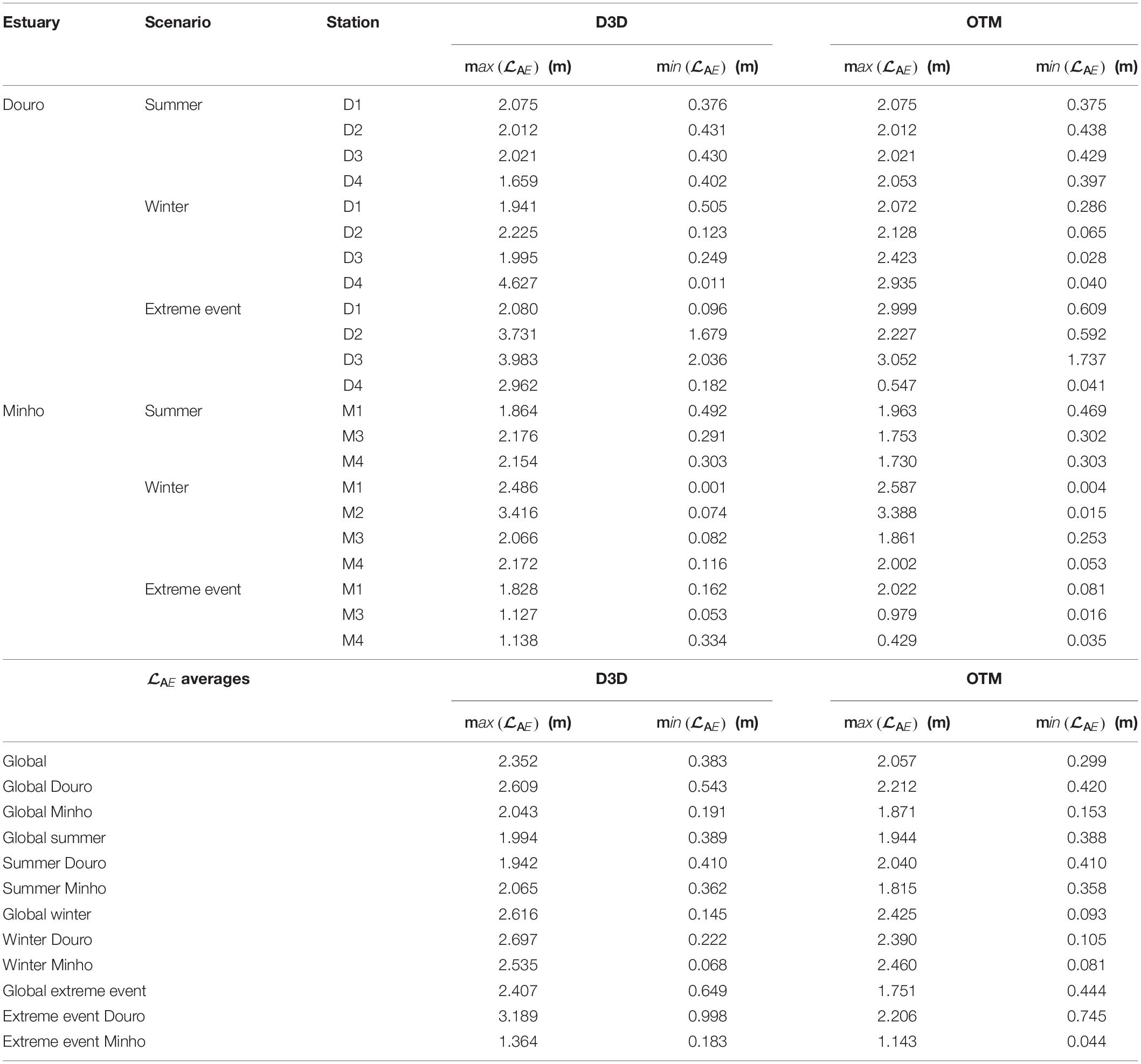
Table 4. Maximum and minimum absolute error, for each station/scenario/estuary/modeling suite, for all runs and their averages.
The highest minimum errors were calculated for the extreme event scenario at D3. Extreme events can be difficult to model due to the complexity of their dynamics, namely the strong erosive processes they give rise to, and the flooding of the riverbanks, which present a hydrodynamic behavior distinct from that of the main estuarine channel. We assumed that measured data is representing the reality. However, it cannot be ruled out that these large differences between measured and hindcasted water levels may be related to errors in the field campaigns, or to inaccuracies in the numerical models for that specific locations. Similarly to Figure 3 and Table 4 also reveals a strong dispersion in the modeling results. The average maximum absolute error for D3D was 2.352 m, but it reached a maximum value of 4.627 m for winter scenarios at D4 station. For OTM, the average maximum absolute error was 2.057 m, reaching 3.388 m for the winter scenario at M2.
Models results of the summer and winter scenarios were divided into two groups, one group for the 24 highest water level ocean boundary conditions and the other for the 24 lowest ones. The results for these two groups were compared with the highest and lowest field recorded water levels, and the absolute errors were computed and presented in Table 5. It is noticeable that OTM performs slightly better than D3D and that dispersion is now lower for both modeling suites, with most maximum absolute errors below 1.6 m and minimum dispersion values below 0.3 m.
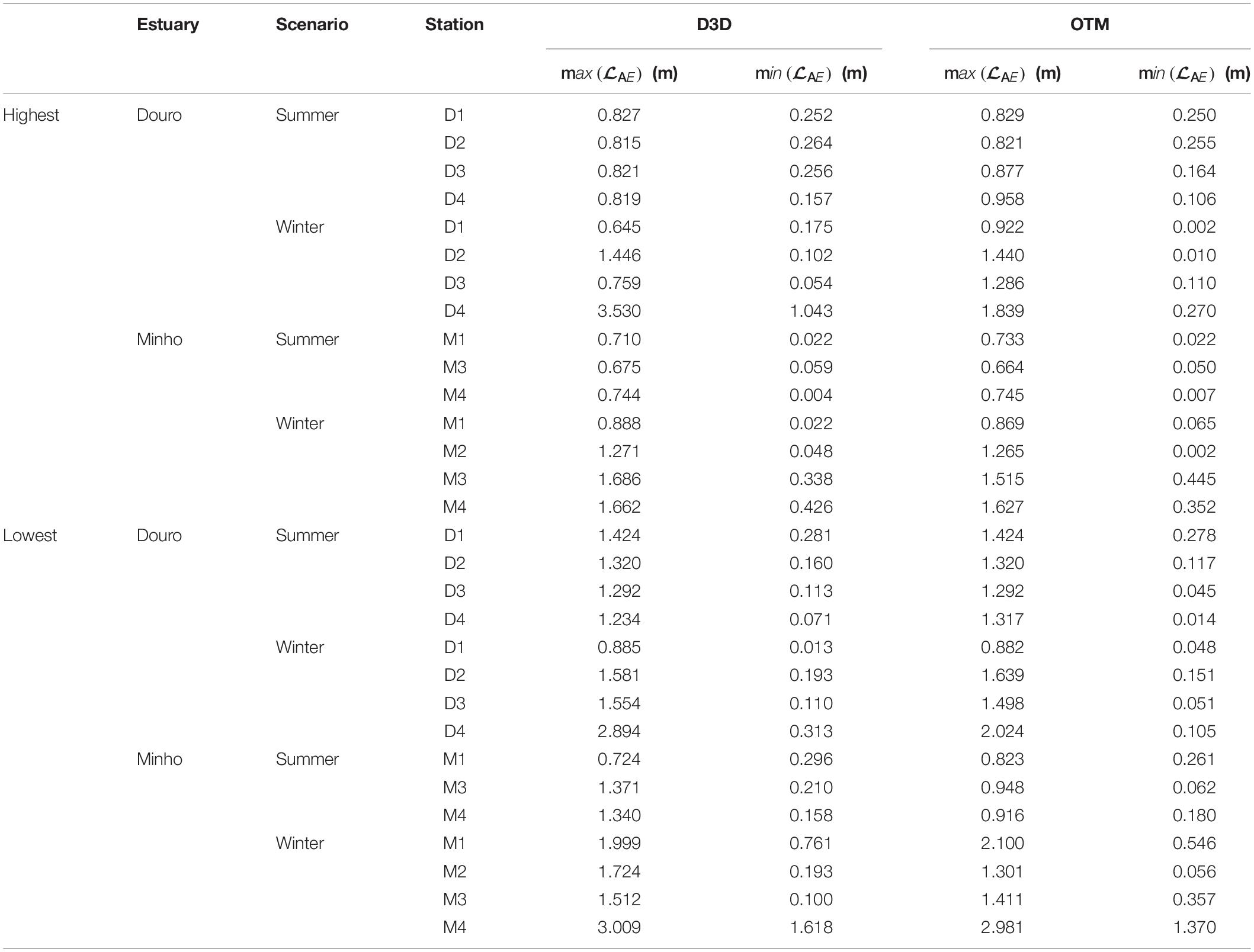
Table 5. Maximum and minimum absolute error for each station/scenario/estuary/modeling suite for the highest and lowest water levels.
The results of the techniques selected for the 48-member superensembles are displayed in Figure 4 and Table 6. The scatter plots showed that, despite the existing variability for all considered scenarios, estuaries and measuring stations, some superensembles results lie on the perfect hindcast line. The techniques that present the best hindcasts are WAM-AE and WAM-SE and the ones that present the worst hindcasts are WAM-Rand1 and WAM-Rand2. This revealed the importance of selecting a WAM method that considers the previous performance of the numerical models. The Trim, SMA and Med techniques showed an average performance, with Trim between SMA and Med, as expected.
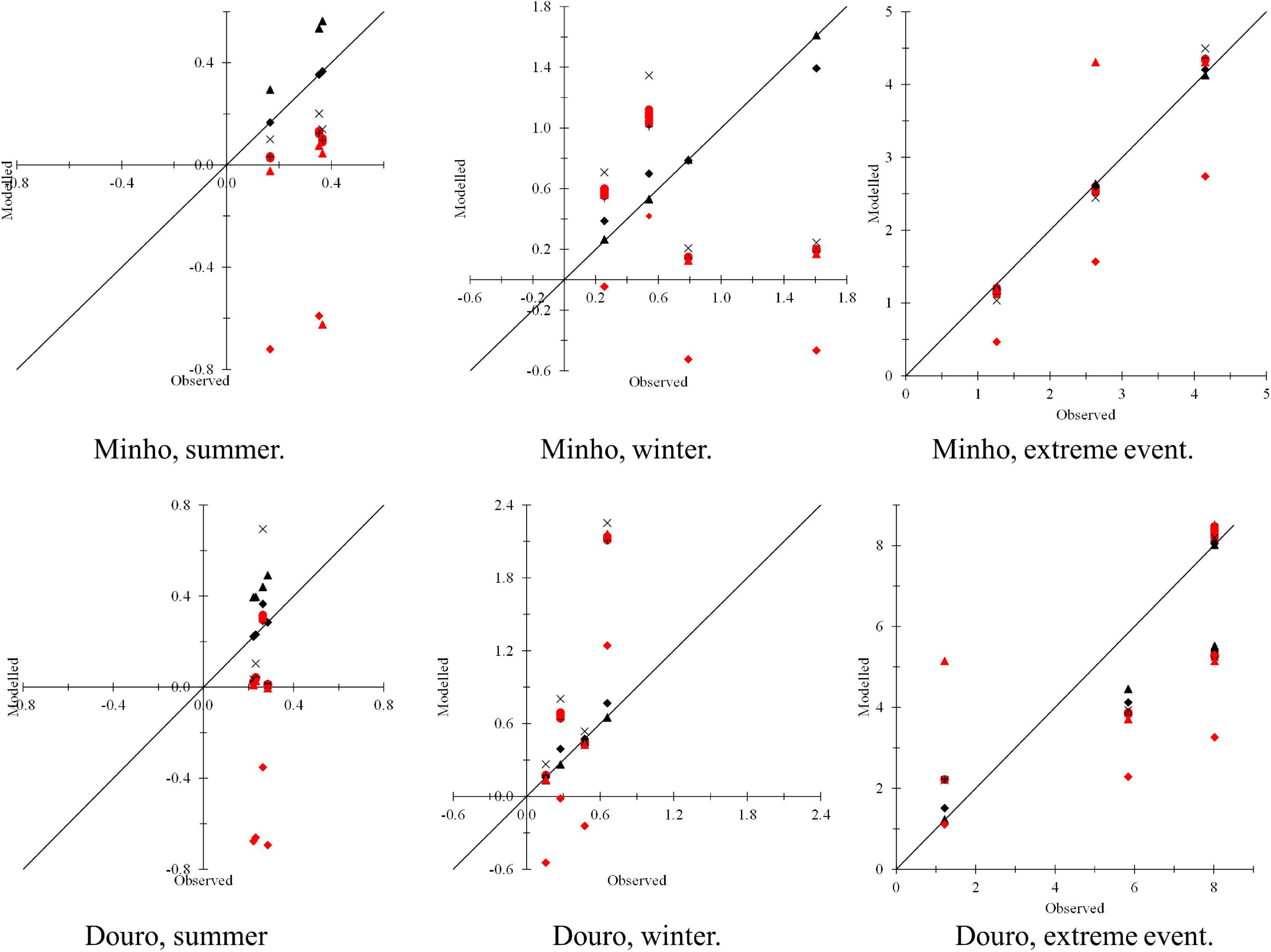
Figure 4. Scatter plots of the water elevation (in meters, referred to the MSL) for the various ensemble techniques considered: SMA (+), Med (x), Trim (), WAM-Rand1 (
), WAM-Rand2 (
), WAM-AE (◆) and WAM-SE(▲). The black line represents the perfect hindcast.
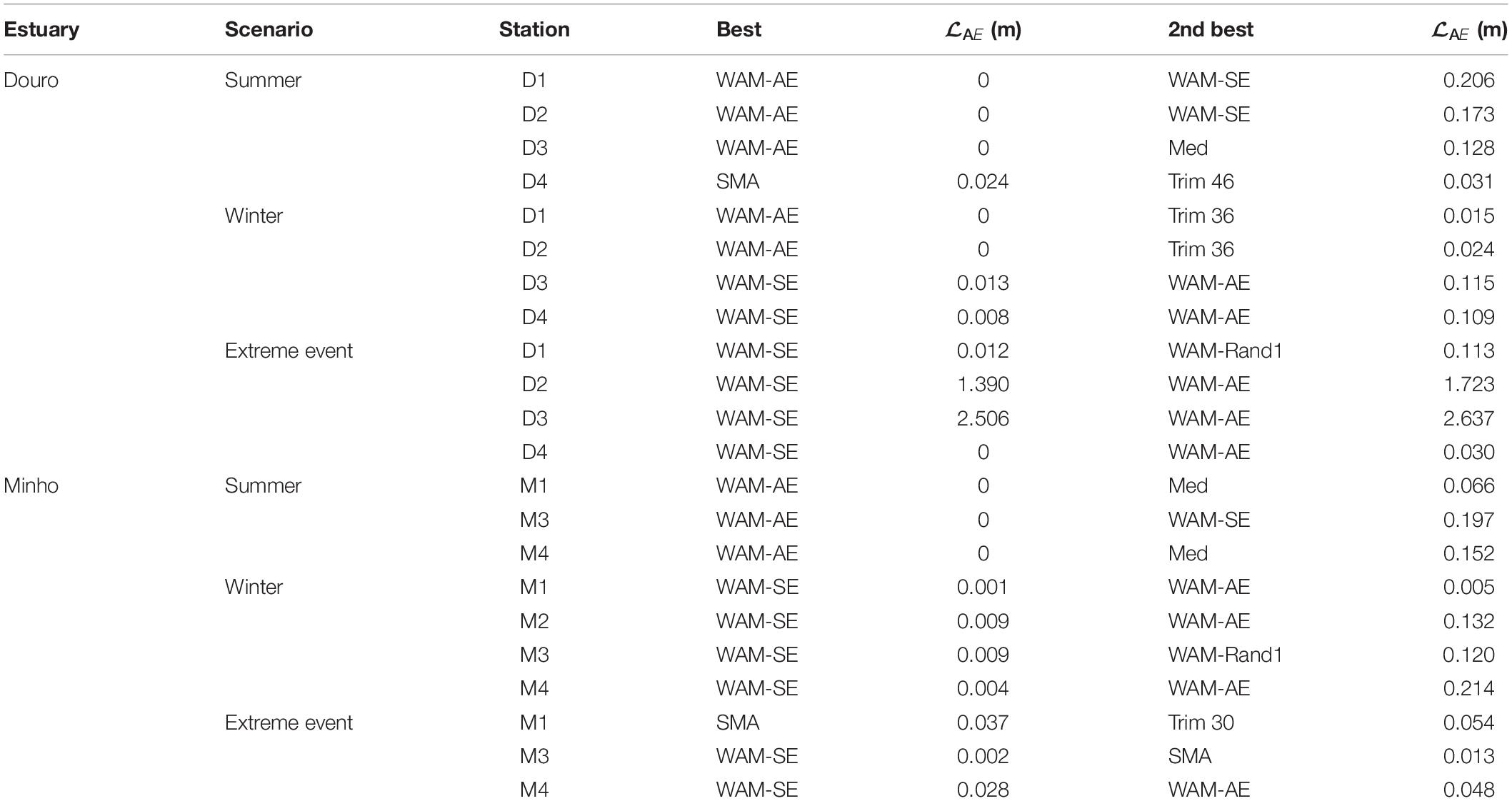
Table 6. Best and second-best ensemble technique for each measurement station/scenario/estuary and associated absolute error.
Table 6 presents the best technique for each scenario, estuary and measuring station. Notice that, for some ensembles, the absolute error is null, i.e., below 1 mm, and the hindcast can be considered perfect. WAM-SE stood out as the best technique for the high river flow scenarios (winter and extreme event), while WAM-AE performed the best for the summer scenarios. The results seem to be independent of the chosen measuring stations and the estuary under study. For all but the Douro extreme event simulations at D2 and D3, the simulations presented lower absolute errors than those obtained for the single numerical modeling runs (see Table 4). Measuring stations D2 and D3 displayed the highest absolute errors when comparing computed and recorded water levels. As pointed out before, this could have been caused by errors in the filed campaigns. For the second-best technique, WAM-SE or WAM-AE are still the prime contenders, but now other techniques can perform better (cf. Table 6). Nevertheless, for some ensembles, the second-best technique displays an absolute error larger than those computed for the single models (Table 4). These techniques are thus not recommended for ensembles construction.
The effect of the size of the ensemble can be analyzed in Figures 5, 6 and Tables 7, 8. The scatter plot constructed with the results considering the 24-highest water elevation predictions revealed a good performance of the superensembles (cf. Figure 5), with the best techniques being those that consider weights depending on the performance of the models. However, not all superensembles produced results on the perfect prediction line, revealing that the size of the ensemble affects its predictive ability.
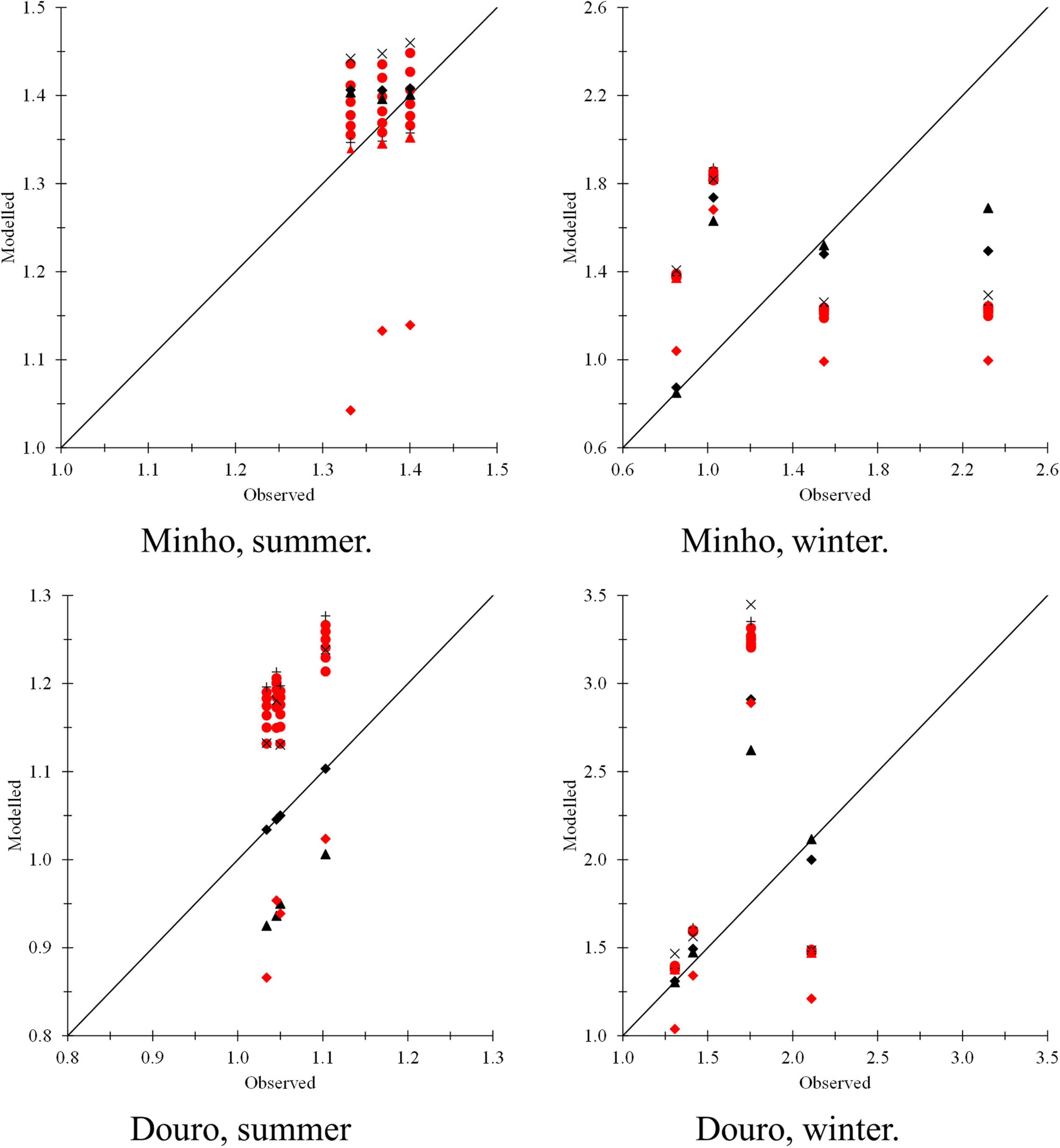
Figure 5. Scatter plots of the water elevation (in meters, referred to the MSL) for the different ensemble techniques considered: SMA (+), Med (x), Trim (), WAM-Rand1 (
), WAM-Rand2 (
), WAM-AE (◆) and WAM-SE (▲). The 24 highest forecasts were considered. The black line represents the perfect hindcast.
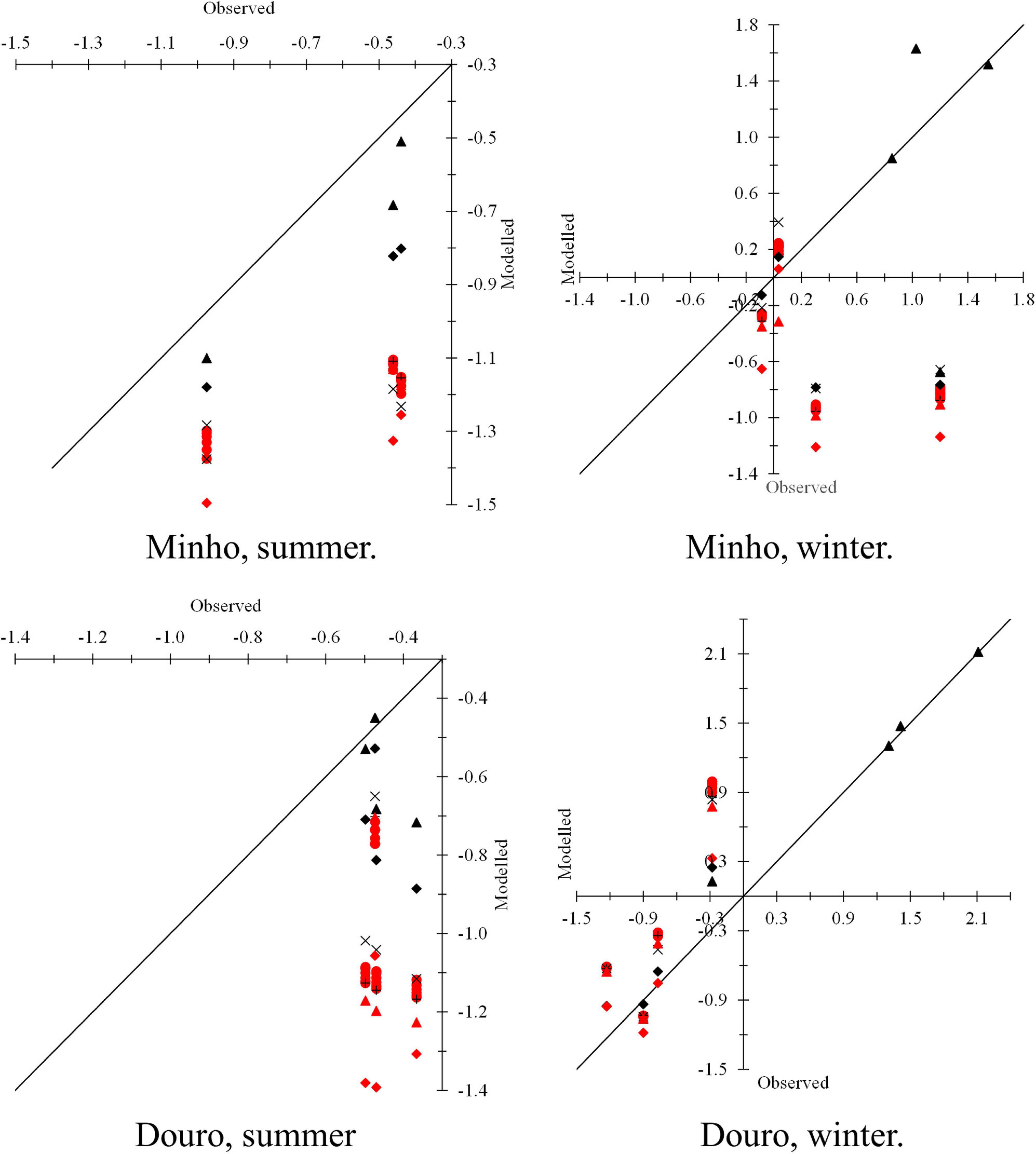
Figure 6. Scatter plots of the water elevation (in meters, referred to the MSL) for the different ensemble techniques considered: SMA (+), Med (x), Trim (), WAM-Rand1 (
), WAM-Rand2 (
), WAM-AE (◆), and WAM-SE (▲). The 24 lowest forecasts were considered. The black line represents the perfect hindcast.
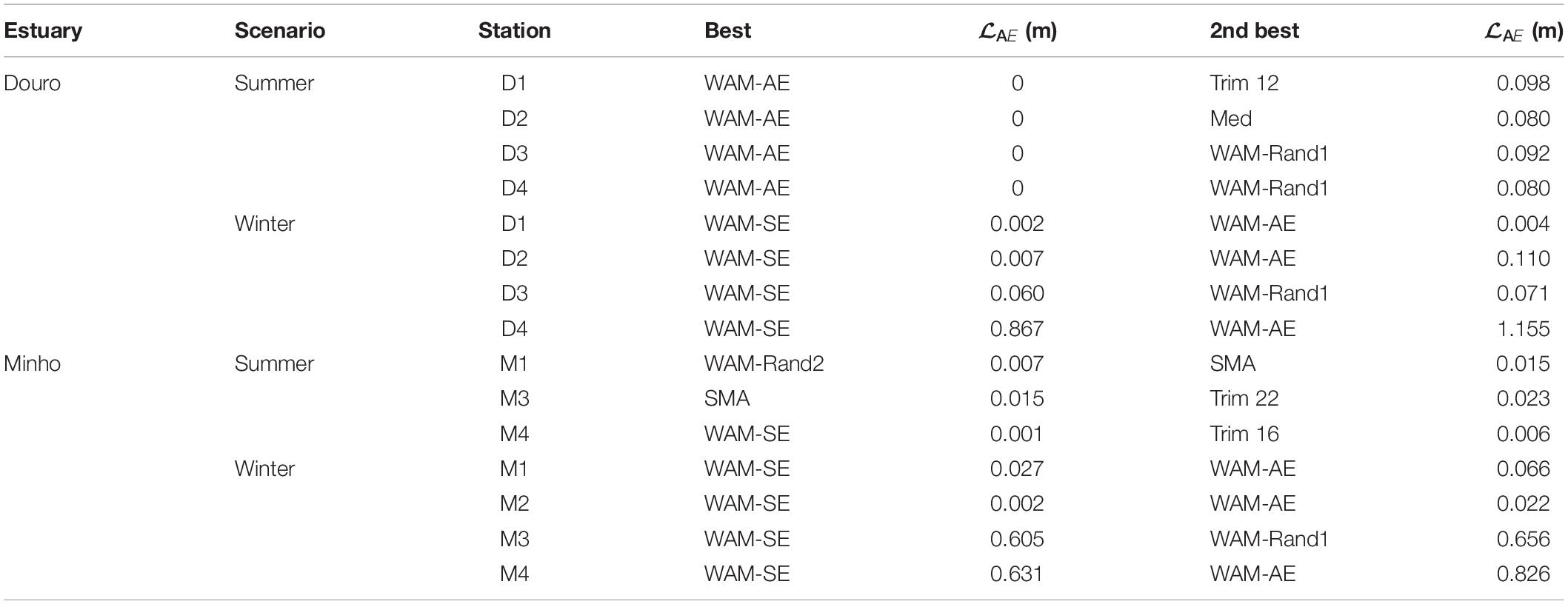
Table 7. Best and second-best ensemble technique but for the 24 highest hindcasts for each measurement station/scenario/estuary and associated absolute error.
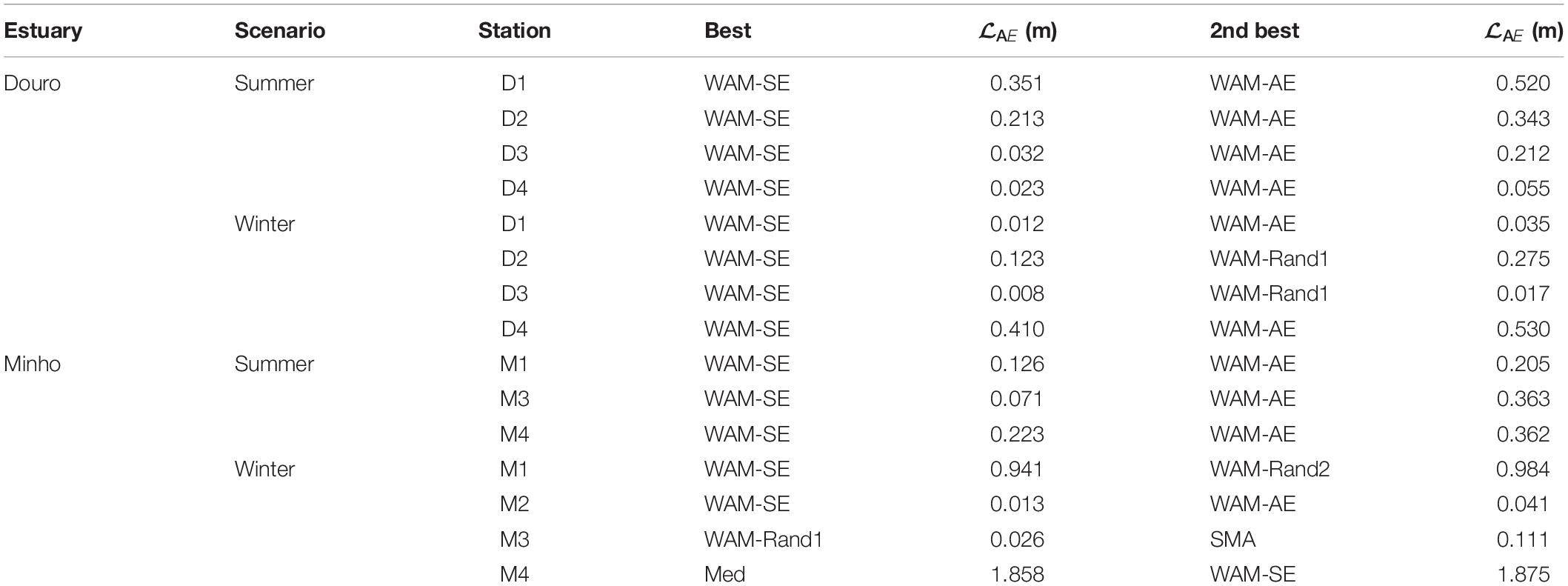
Table 8. Best and second-best ensemble technique but for the 24 lowest hindcasts for each measurement station/scenario/estuary and associated absolute error.
This fact is confirmed by the AEs (Table 7). The best technique was WAM-AE for Douro summer and WAM-SE for Minho and Douro winter. The best Minho summer ensemble technique depends on the location, which could be related with errors in the observations or numerical causalities (as, for example, WAM-Rand2). AEs were generally below 0.1 m. However, in 5 of the 15 scenarios/sampling points, ensembling did not improve the hindcast skills of the individual models, demonstrating that the ensemble size is crucial in producing the best hindcast. The second-best technique revealed even worse hindcasts, with AE values surpassing those obtained for the comparison between models results and measurements.
For the 24-member superensemble for the lower water elevation predictions, the scatter plot in Figure 6 shows a vaster dispersion of ensembles results compared to the previously analyzed superensembles (cf. Figures 4, 5). Most solutions are very distant from the perfect hindcast, which could be due to uncertainties in the modeling of low tide conditions. This suggests that the larger superensembles were able to absorb these uncertainties, providing better results, which is confirmed by the absolute error values (cf. Tables 4–6, 8). The 24-member superensembles outperform the single model hindcasts in 7 out of the 15 runs/measuring stations, with values higher than those obtained for the 48- and 32-member superensembles and for the 24-member superensembles for higher water elevation conditions. The technique that presented the best results was the WAM-SE (cf. Table 8).
Conclusion
Numerical models are essential tools for a better understanding of estuaries. They can anticipate and predict simultaneously the effects of anthropogenic interventions, extreme events, and climate change, providing the basis for an efficient estuarine management. However, as modeling results present uncertainties, mainly related to inaccuracies or assumptions in the initial and forcing conditions, we need to increase the forecasting accuracy by developing and implementing new solutions that avoid or mitigate such errors.
The approach used in this work, based on the integration of the D3D and OTM modeling suites, proved to efficiently map the response of two Portuguese open water systems —the Douro and Minho estuaries. Three scenarios were considered: summer, winter and extreme event conditions. For each estuary and scenario, a superensemble was constructed including modified conditions of river inflows and ocean surface elevation. The single model’s and the superensembles’ results were compared with in situ measurements of water elevation to assess their accuracy. It was demonstrated that the superensembles were able to circumvent the models’ inaccuracies and produce better results than the individual models, providing solutions that were closer to the observed values than those resulting from the individual models.
Several techniques of ensemble construction were implemented to find the one that yields the best results for each estuary, scenario, and measuring station. The results revealed that an adequate ensemble technique effectively improves the forecasting results of the individual models. Among the applied techniques (SMA, Med, Trim, WAM-Rand1, WAM-Rand2, WAM-AE, and WAM-SE), the best results were obtained with those that considered the hindcast capabilities of the single models (WAM-AE and WAM-SE). Namely, WAM-SE stood out as the best technique for scenarios with high river inflow (winter and extreme event scenarios), whereas WAM-AE was the best for summer scenarios. No relevant differences were found between the two estuaries or associated with the location of the field measurements, demonstrating that this technique can be widely applied to any estuarine system. However, the ensemble size affected the predictability, and the most accurate solutions were obtained with the superensemble constructed with more members.
It was demonstrated that, even for the scenarios presenting more difficulties in being hindcasted by a single model, such as extreme event scenarios, the application of the ensemble technique reduced the inaccuracy of the forecasts and improved their results. This is of upmost importance, especially for predicating future conditions under the effect of climate change, when stronger and more frequent extreme events are expected. Therefore, hydrodynamic modeling ensembles have the potential to provide accurate information for estuarine management activities and to reduce the risk and the vulnerability of populations, infrastructures, habitats and environment.
Data Availability Statement
The raw data supporting the conclusions of this article will be made available by the authors, without undue reservation.
Author Contributions
II, JP, and PA-V: conceptualization, methodology, and formal analysis. II, WM, and AB: computation. II and WM: validation. JP, PA-V, JV, AB, LB, and FV-G: resources. II, WM, and JP: data curation. II, JP, WM, PA-V, JV, AB, AG, LB, and FV-G: writing—review and editing. FV-G: supervision. II, JP, and FV-G: project administration. II, JP, PA-V, JV, AB, LB, and FV-G: funding acquisition. All authors contributed to the article and approved the submitted version.
Funding
This research was partially supported by the Strategic Funding UIDB/04423/2020 and UIDP/04423/2020 through national funds provided by FCT—Foundation for Science and Technology and European Regional Development Fund (ERDF). This work has also been funded by the project EsCo-Ensembles (PTDC/ECI-EGC/30877/2017), co-financed by NORTE 2020, Portugal 2020 and the European Union through the ERDF, and by FCT through national funds.
Conflict of Interest
The authors declare that the research was conducted in the absence of any commercial or financial relationships that could be construed as a potential conflict of interest.
Publisher’s Note
All claims expressed in this article are solely those of the authors and do not necessarily represent those of their affiliated organizations, or those of the publisher, the editors and the reviewers. Any product that may be evaluated in this article, or claim that may be made by its manufacturer, is not guaranteed or endorsed by the publisher.
Acknowledgments
The authors would like to thank project ECOIS—Estuarine Contributions to Inner Shelf dynamics, Direção Geral do Território, Instituto Hidrográfico, Energias de Portugal, and Confederación Hidrográfica Miño-Sil for the data provided.
Supplementary Material
The Supplementary Material for this article can be found online at: https://www.frontiersin.org/articles/10.3389/fmars.2022.812255/full#supplementary-material
References
Ajami, N. K., Duan, Q., Gao, X., and Sorooshian, S. (2006). Multimodel combination techniques for analysis of hydrological simulations: application to distributed model intercomparison project results. J. Hydrometeorol. 7, 755–768. doi: 10.1175/JHM519.1
Almeida, A., Taveira-Pinto, F., Veloso-Gomes, F., and Moura Paredes, G. (2009). “Análise das marés meteorológicas em leixões,” in 6as Jornadas Portuguesas de Engenharia Costeira e Portuária, (Funchal, Portugal: PIANC), 16.
Baeta, A., Vieira, L. R., Lírio, A. V., Canhoto, C., Marques, J. C., and Guilhermino, L. (2017). Use of stable isotope ratios of fish larvae as indicators to assess diets and patterns of anthropogenic nitrogen pollution in estuarine ecosystems. Ecol. Ind. 83, 112–121. doi: 10.1016/j.ecolind.2017.07.062
Bastos, L., Bio, A., and Iglesias, I. (2016). The importance of marine observatories and of RAIA in particular. Front. Mar. Sci. 3:140. doi: 10.3389/fmars.2016.00140
Bastos, L., Bio, A., Pinho, S. J. L., Granja, H., and Jorge da Silva, A. (2012). Dynamics of the douro estuary sand spit before and after breakwater construction. Estua. Coast. Shelf Sci. 109, 53–69. doi: 10.1016/j.ecss.2012.05.017
Bazell, D., and Aha, D. W. (2001). Ensembles of classifiers for morphological galaxy classification. Astrophys. J. 548, 219–223. doi: 10.1086/318696
Bell, J. E, Brown, C. L., Conlon, K., Herring, S., Kunkel, K. E., Lawrimore, J., Luber, G., et al. (2018). Changes in extreme events and the potential impacts on human health. J. Air Waste Manag. Assoc. 68, 265–287. doi: 10.1080/10962247.2017.1401017
Benediktsson, J. A., Chanussot, J., and Fauvel, M. (2007). “Multiple classifier systems in remote sensing: from basics to recent developments,” in Multiple Classifier Systems, eds H. Michal and, K. Josef and R. Fabio (Berlin: Springer Berlin Heidelberg), 501–512. doi: 10.1007/978-3-540-72523-7_50.
Bernhofen, M. V., Whyman, C., Trigg, M. A., Sleigh, P. A., Smith, A. M., Sampson, C. C., et al. (2018). A first collective validation of global fluvial flood models for major floods in nigeria and mozambique. Environ. Res. Lett. 13:104007. doi: 10.1088/1748-9326/aae014
Buizza, R., Houtekamer, P. L., Pellerin, G., Toth, Z., Zhu, Y., Wei, M., et al. (2005). A comparison of the ECMWF, MSC, and NCEP global ensemble prediction systems. Mon. Weather Rev. 133, 1076–1097. doi: 10.1175/MWR2905.1
Cantelaube, P., and Terres, J-M. (2005). Seasonal weather forecasts for crop yield modelling in Europe. Tellus A 57, 476–487. doi: 10.1111/j.1600-0870.2005.00125.x
Casanova, S., and Ahrens, B. (2009). On the weighting of multimodel ensembles in seasonal and short-range weather forecasting. Mon. Weather Rev. 137, 3811–3822. doi: 10.1175/2009MWR2893.1
Chakraborty, A., and Krishnamurti, T. N. (2006). Improved seasonal climate forecasts of the South Asian summer monsoon using a suite of 13 coupled ocean–atmosphere models. Mon. Weather Res. 134, 1697–1721. doi: 10.1175/MWR3144.1
Chen, W.-B., Liu, W.-C., and Hsu, M.-H.. (2015a). Modeling assessment of a saltwater intrusion and a transport time scale response to sea-level rise in a tidal estuary. Environ. Fluid Mech. 15, 491–514. doi: 10.1007/s10652-014-9367-y
Chen, W.-B., Liu, W.-C., Hsu, M-H., and Hwang, C.-C. (2015b). Modeling investigation of suspended sediment transport in a tidal estuary using a three-dimensional model. Appl. Math. Model. 39, 2570–2586. doi: 10.1016/j.apm.2014.11.006
Clemen, R T. (1989). Combining forecasts: a review and annotated bibliography. Int. J. Forecast. 5, 559–583. doi: 10.1016/0169-2070(89)90012-5
Coelho, C., Silva, R., Veloso-Gomes, F., and Taveira-Pinto, F. (2009). Potential effects of climate change on northwest portuguese coastal zones. ICES J. Mar. Sci. 66, 1497–1507.
Corti, S., and Pennati, V. (2000). A 3D hydrodynamic model of river flow in a delta region. Hydrol. Process. 14, 2301–2309. doi: 10.1002/1099-1085(200009)14:13<2301::AID-HYP30<3.0.CO;2-L
Dangendorf, S., Wahl, T., Hein, H., Jensen, J., Mai, S., Mudersbach, C., et al. (2012). mean sea level variability and influence of the north atlantic oscillation on long-term trends in the german bight. Water 4, 170–195. doi: 10.3390/w4010170
Delgado, A., Taveira-Pinto, F., and Silva, R. (2011). “Hydrodynamic and morphodynamic preliminary simulation of river minho estuary,” in 6a Jornadas de Hidráulica, Recursos Hídricos e Ambiente, (Porto, Portugal: Secção de Hidráulica, Recursos Hídricos e Ambiente da Faculdade de Engenharia da Universidade do Porto), 113–126.
Demeritt, D., Cloke, H., Pappenberger, F., Thielen, J., Bartholmes, J., Ramos, M., et al. (2007). Ensemble predictions and perceptions of risk, uncertainty, and error in flood forecasting. Environ. Hazard. 7, 115–127. doi: 10.1016/j.envhaz.2007.05.001
Déqué, M., and Somot, S. (2010). Weighted frequency distributions express modelling uncertainties in the ensembles regional climate experiments. Clim. Res. 44, 195–209. doi: 10.3354/cr00866
Deutscher Wetterdienst (2021). Deutscher Wetterdienst. Avaliable online at: https://www.dwd.de/EN/Home/home_node.html (accessed September, 2021).
Dickinson, J. P. (1973). Some statistical results in the combination of forecasts. J. Operat. Res.Soc. 24, 253–260. doi: 10.1057/jors.1973.42
Dinápoli, M. G., Simionato, C. G., and Moreira, D. (2021). Development and evaluation of an ensemble forecast/hindcast system for storm surges in the río de la plata estuary. Q. J. R. Meteorol. Soc. 147, 557–572. doi: 10.1002/qj.3933
Doblas-Reyes, F. J., Déqué, M., and Piedelievre, J.-P. (2010). Multi-model spread and probabilistic seasonal forecasts in PROVOST. Q. J. R. Meteorol. Soc. 126, 2069–2087. doi: 10.1002/qj.49712656705
Domínguez García, M. D., Horlings, L., Swagemakers, P., and Simón Fernández, X. (2013). Place branding and endogenous rural development. departure points for developing an inner brand of the river minho estuary. Place Brand. Public Diplom. 9, 124–140. doi: 10.1057/pb.2013.10
Du, J., Berner, J., Buizza, R., Charron, M., Houtekamer, P., Hou, D., Jankov, I., et al. (2018). “Ensemble methods for meteorological predictions,” in Handbook of Hydrometeorological Ensemble Forecasting, eds Q. Duan, F. Pappenberger, A. Wood, H. L. Cloke, and J. C. Schaake (Berlin: Springer Berlin Heidelberg), 1–52. doi: 10.1007/978-3-642-40457-3_13-1.
Epstein, E. S. (1969). Stochastic dynamic prediction. Tellus 21, 739–759. doi: 10.3402/tellusa.v21i6.10143
Feng, J., Lee, D.-K., Fu, C., Tang, J., Sato, Y., Kato, H., et al. (2011). Comparison of four ensemble methods combining regional climate simulations over Asia. Meteorol. Atmosph. Phys. 111, 41–53. doi: 10.1007/s00703-010-0115-7
Ferreira, J. G., Abreu, P. F., Bettencourt, A. M., Bricker, S. B., Marques, J. C., Melo, J. J., et al. (2005). Monitoring Plan for Portuguese Coastal Waters. Water Quality and Ecology. Development of Guidelines for the Applications of the European Union Water Framework Directive. INAG-IMAR
Freitas, V., Costa-Dias, S., Campos, J., Bio, A., Santos, P., Antunes, C., et al. (2009). Patterns in abundance and distribution of juvenile flounder, platichthys flesus, in minho estuary (NW Iberian Peninsula). Aquat. Ecol. 43, 1143–1153. doi: 10.1007/s10452-009-9237-8
Gama, C., Alveirinho Dias, J.M., Ferreira, O., and Taborda, R. (1994). Analysis of storm surge in portugal between June 1986 and May 1988. Littoral 94, 26–29
Georgakakos, K. P., Seo, D.-J., Gupta, H., Schaake, J., and Butts, M. B. (2004). Towards the characterization of streamflow simulation uncertainty through multimodel ensembles. J. Hydrol. 298, 222–241. doi: 10.1016/j.jhydrol.2004.03.037
Gneiting, T., and Raftery, A. E. (2005). Weather forecasting with ensemble methods. Science 310, 248–249. doi: 10.1126/science.1115255
Gomes, M. P., Pinho, J. L., Antunes do Carmo, J. S., and Santos, L. (2015). Hazard assessment of storm events for the battery, New York. Ocean Coast. Manag. 118, 22–31. doi: 10.1016/j.ocecoaman.2015.11.006
Granja, H., Bastos, L., Pinho, J. L., Gonçalves, J., Henriques, R. F., Bio, A., et al. (2011). “Integração de metodologias no estabelecimento de Um Programa de monitorização costeira para avaliação de Risco,” in VII Conferência Nacional de Cartografia e Geodesia, (Porto, Portugal: Colégio Nacional de Engenharia Geográfica da Ordem dos Engenheiros), 11.
Hagedorn, R., Doblas-Reyes, F. J., and Palmer, T. N. (2005). The rationale behind the success of multi-model ensembles in seasonal forecasting — I. Basic concept. Tellus A 57, 219–233. doi: 10.3402/tellusa.v57i3.14657
Hallegatte, S., Green, C., Nicholls, R. J., and Corfee-Morlot, J. (2013). Future flood losses in major coastal cities. Nat. Clim. Change 3, 802–806. doi: 10.1038/nclimate1979
Horritt, M. S., and Bates, P. D. (2002). Evaluation of 1D and 2D numerical models for predicting river flood inundation. J. Hydrol. 268, 87–99. doi: 10.1016/S0022-1694(02)00121-X
Kourafalou, V. H., Demey, P., Staneva, J., Ayoub, N., Barth, A., Chao, Y., et al. (2015). Coastal ocean forecasting: science foundation and user benefits. J. Operat. Oceanogr. 8, 147–167. doi: 10.1080/1755876X.2015.1022348
Iglesias, I., Avilez-Valente, P., Bio, A., and Bastos, L. (2019a). Modelling the main hydrodynamic patterns in shallow water estuaries: the minho case study. Water 11:1040. doi: 10.3390/w11051040
Iglesias, I., Venâncio, S., Pinho, J. L., Avilez-Valente, P., and Vieira, J. (2019b). Two models solutions for the douro estuary: flood risk assessment and breakwater effects. Estuar. Coast. 42, 348–364. doi: 10.1007/s12237-018-0477-5
Iglesias, I., Bio, A., Bastos, L., and Avilez-Valente, P. (2021). Estuarine hydrodynamic patterns and hydrokinetic energy production: the douro estuary case study. Energy 222:119972. doi: 10.1016/j.energy.2021.119972
Iglesias, I., Almeida, C. M. R., Teixeira, C., Mucha, A. P., Magalhães, A., Bio, A., et al. (2020a). Linking contaminant distribution to hydrodynamic patterns in an urban estuary: the douro estuary test case. Sci. Total Environ. 707:135792. doi: 10.1016/j.scitotenv.2019.135792
Iglesias, I., Avilez-Valente, P., Luís Pinho, J., Bio, A., Vieira, J., Bastos, L., et al. (2020b). “Numerical modeling tools applied to estuarine and coastal hydrodynamics: a user perspective,” in Coastal and Marine Environments - Physical Processes and Numerical Modelling, ed. JS. Antunes do Carmo, (London: IntechOpen), 20. doi: 10.5772/intechopen.85521.
IPCC (2012). Managing the Risks of Extreme Events and Disasters to Advance Climate Change Adaptation. Summary for Policymakers. ed Field, B. V. Barros, T. F. Stocker, D. Qin, D. J. Dokken, K. L. Ebi, etal. (Cambridge: Cambridge University Press).
IPCC (2021). IPCC. Avaliable online at: https://www.ipcc.ch/ (accessed September, 2021).
Jones, E. J., and Davies, A. M. (2010). Application of a finite element model to the computation of tides in the mersey estuary and eastern Irish Sea. Cont. Shelf Res. 30, 491–514. doi: 10.1016/j.csr.2010.01.003
Khanarmuei, M.., Mardani, N., Suara, K., Sumihar, J., Sidle, R. C., McCallum, A., et al. (2021). Assessment of an ensemble-based data assimilation system for a shallow estuary. Estuar. Coast. Shelf Sci. 257:107389. doi: 10.1016/j.ecss.2021.107389
Krishnamurti, T. N., Kishtawal, C. M., LaRow, T. E., Bachiochi, D. R., Zhang, Z., Williford, C. E., et al. (1999). Improved weather and seasonal climate forecasts from multimodel superensemble. Science 285, 1548–1550. doi: 10.1126/science.285.5433.1548
Krogh, A., and Vedelsby, J. (1995). “Neural network ensembles, cross validation, and active learning,” in Advances in Neural Information Processing Systems, (New York, NY: AMC), 7.
Kumar, A., Mitra, A. K., Bohra, A. K., Iyengar, G. R., and Durai, V. R. (2012). Multi-model ensemble (mme) prediction of rainfall using neural networks during monsoon season in india. Meteorol. Appl. 19, 161–169. doi: 10.1002/met.254
Leigh, W., Purvis, R., and Ragusa, J. M. (2002). Forecasting the NYSE composite index with technical analysis, pattern recognizer, neural network, and genetic algorithm: a case study in romantic decision support. Decis. Support Syst. 32, 361–377. doi: 10.1016/S0167-9236(01)00121-X
Leith, C. E. (1974). Theoretical skill of monte carlo forecasts. Mon. Weather Rev. 102, 409–418. doi: 10.1175/1520-04931974102<0409:TSOMCF<2.0.CO;2
Liu, W.-C., Chen, W.-B., and Kuo, J.-T. (2008). Modeling residence time response to freshwater discharge in a mesotidal estuary, Taiwan. J. Mar. Syst. 74, 295–314. doi: 10.1016/j.jmarsys.2008.01.001
Lorenz, E. N. (1963). Deterministic nonperiodic flow. J. Atmos. Sci. 20, 130–141. doi: 10.1175/1520-04691963020<0130:DNF<2.0.CO;2
Lorenz, E. N. (1965). A study of the predictability of a 28-variable atmospheric model. Tellus 17, 321-333. doi: 10.3402/tellusa.v17i3.9076
Melo, W., Pinho, J., Iglesias, I., Bio, A., Avilez-Valente, P., Vieira, J., et al. (2020). Hydro- and morphodynamic impacts of sea level rise: the minho estuary case study. J. Mar. Sci. Eng. 8:441. doi: 10.3390/jmse8060441
Menahem, E., Shabtai, A., Rokach, L., and Elovici, Y. (2009). Improving malware detection by applying multi-inducer ensemble. Comput. Stat. Data Anal. 53, 1483–1494. doi: 10.1016/j.csda.2008.10.015
Mohan Das, D., Singh, R., Kumar, A., Mailapalli, D. R., Mishra, A., and Chatterjee, C. (2017). “A multi-model ensemble approach for stream flow simulation,” in Modeling Methods and Practices in Soil and Water Engineering, eds O. N. Oakville ?and N. J. Waretown (Palm Bay, FL?: Apple Academic Press), 71–102. doi: 10.1201/b19987-5.
Monteiro, I. O., Marques, W. C., Fernandes, E. H., Gonçalves, R. C., and Möller, O. O. (2011). On the effect of earth rotation, river discharge, tidal oscillations, and wind in the dynamics of the patos lagoon coastal plume. J. Coast. Res. 27:120. doi: 10.2112/JCOASTRES-D-09-00168.1
Moser, S. C., Davidson, M. A., Kirshen, P., Mulvaney, P., Murley, J. F., Neumann, J. E.,, et al. (2014). “Coastal zone development and ecosystems,” in Climate Change Impacts in the United States: The Third National Climate Assessment, eds J. M. Melillo, T. T. C. Richmond, and G. W. Yohe (U.S. Global Change Research Program), 579–618. doi: 10.7930/J0MS3QNW
Newbold, P., and Granger, C. W. J. (1974). Experience with forecasting univariate time series and the combination of forecasts. J. R. Stat. Soc. Ser. A 137:131. doi: 10.2307/2344546
Palmer, T. N., Doblas-Reyes, F. J., Hagedorn, R., and Weisheimer, A. (2005). Probabilistic prediction of climate using multi-model ensembles: from basics to applications. Philos. Trans. R. Soc. B Biol. Sci. 360, 1991–1998. doi: 10.1098/rstb.2005.1750
Palmer, T N., Alessandri, A., Andersen, U., Cantelaube, P., Davey, M., Délécluse, P., et al. (2004). Development of an European multimodel ensemble system for seasonal-to-interannual prediction (Demeter). Bull. Am. Meteorol. Soc. 85, 853–872. doi: 10.1175/BAMS-85-6-853
Palmer, T. N. (2003). “Predictability of Weather and climate: from theory to practice - from days to decades,” in Realizing Teracomputing, Vol. 1, (Singapore: World Scientific), 1–18. doi: 10.1142/9789812704832_0001.
Peixoto, R. (2016). Modelação Hidrodinâmica Do Estuário Do Douro: Cenários de Cheia e Influência Dos Novos Molhes. Porto: University of Porto.
Pereira, H. (2016). Coupled Modelling of the Minho and Lima Estuaries: Hydrological Response to Climate Changes. Aveiro: Universidade de Aveiro.
Polikar, R., Topalis, A., Parikh, D., Green, D., and Frymiare, J., Kounios, J., and Clark, C. M. (2008). An ensemble based data fusion approach for early diagnosis of Alzheimer’s Disease. Inf. Fusion 9, 83–95. doi: 10.1016/j.inffus.2006.09.003
Portela, L.. (2008). Sediment transport and morphodynamics of the douro river estuary. Geo Mar. Lett. 28, 77–86. doi: 10.1007/s00367-007-0091-1
Portela, L. (2011). “Morphological evolution, hydrodynamics and sediment dynamics of the minho river estuary,” in 3.o Seminário Sobre Gestão de Bacias Hidrográficas. Os Estuários, 6.
Putra, S.S, van der Wegen, M., Reyns, J., Dam, A. V., Solomatine, D. P., Roelvink, J. A., et al. (2015). Multi station calibration of 3D flexible mesh model: a case study of the columbia estuary. Proc. Environ. Sci. 28: 297–306. doi: 10.1016/j.proenv.2015.07.038
Rajagopalan, B., Lall, U., and Zebiak, S. E. (2002). Categorical climate forecasts through regularization and optimal combination of multiple GCM ensembles. Mon. Weather Rev. 130, 1792–1811. doi: 10.1175/1520-04932002130<1792:CCFTRA<2.0.CO;2
Re, M., and Valentini, G. (2010). Simple ensemble methods are competitive with state-of-the-art data integration methods for gene function prediction. J. Mach. Lear. Res. W. C. Proc. 8, 98–111.
Reis, J. L, Martinho, A. S., Pires-Silva, A. A., and Silva, A. J. (2009). Assessing the influence of the river discharge on the minho estuary tidal regime. J. Coast. Res. 2009, 1405-1409.
Ribeiro, D. C, Costa, S., and Guilhermino, L. (2016). A framework to assess the vulnerability of estuarine systems for use in ecological risk assessment. Ocean Coast. Manag. 119, 267-277. doi: 10.1016/j.ocecoaman.2015.05.022
Robins, P. E., and Davies, A. G. (2010). Morphological controls in sandy estuaries: the influence of tidal flats and bathymetry on sediment transport. Ocean Dyn. 60, 503–517. doi: 10.1007/s10236-010-0268-4
Roy Bhowmik, S. K., and Durai, V. R. (2008). Multi-model ensemble forecasting of rainfall over indian monsoon region. Atmosfera 21, 225–239. doi: 10.1007/s00382-017-3841-4
Roy Bhowmik, S. K., and Durai, V. R. (2010). Application of multimodel ensemble techniques for real time district level rainfall forecasts in short range time scale over indian region. Meteorol. Atmos. Phys. 106, 19–35. doi: 10.1007/s00703-009-0047-2
Rozante, J. R, Moreira, D. S., Godoy, R. C. M., and Fernandes, A. A. (2014). Multi-model ensemble: technique and validation. Geosci. Model Dev. 7, 2333–2343. doi: 10.5194/gmd-7-2333-2014
Santos, A. I, Oliveira, A., Carinhas, D., Pinto, J. P., Freitas, and M. C. (2021). Hydrodynamic and sediment transport patterns in the minho and douro estuaries (NW Portugal) based on ADCP monitoring data: part 2—statistical interpretation of bottom moored datasets. Coasts 1, 56–72. doi: 10.3390/coasts1010004
Santos, I., Teodoro, A. C., and Taveira-Pinto, F. (2010). “Análise da evolução morfológica da restinga do rio douro,” in 5as Jornadas de Hidráulica, Recursos Hídricos e Ambiente, (Porto, Portugal: Secção de Hidráulica, Recursos Hídricos e Ambiente da Faculdade de Engenharia da Universidade do Porto), 14.
Suh, M. S., Oh, S. G., Lee, D. K., Cha, D. H., Choi, S. J., Jin, C. S., et al. (2012). Development of new ensemble methods based on the performance skills of regional climate models over south Korea. J. Clim. 25, 7067–7082. doi: 10.1175/JCLI-D-11-00457.1
Taeb, P., and Weaver, R. J. (2019). An operational coastal forecasting tool for performing ensemble modeling. Estuar. Coas. Shelf Sci. 217, 237–249. doi: 10.1016/j.ecss.2018.09.020
Tebaldi, C., and Knutti, R,. (2007). The use of the multi-model ensemble in probabilistic climate projections. Philos. Trans. R. Soc. A Math. Phys. Eng. Sci. 365, 2053–2075. doi: 10.1098/rsta.2007.2076
Teng, J., Jakeman, A. J., Vaze, J., Croke, B. F. W., Dutta, D., Kim, S., et al. (2017). Flood inundation modelling: a review of methods, recent advances and uncertainty analysis. Environ. Model. Softw. 90, 201–216. doi: 10.1016/j.envsoft.2017.01.006
Thompson, P. D. (1977). How to improve accuracy by combining independent forecasts. Mon. Weather Rev. 105, 228–229. doi: 10.1175/1520-04931977105<0228:HTIABC<2.0.CO;2
Thomson, M. C., Doblas-Reyes, F. J., Mason, S. J., Hagedorn, R., Connor, S. J., Phindela, T., et al. (2006). Malaria early warnings based on seasonal climate forecasts from multi-model ensembles. Nature 439, 576–579. doi: 10.1038/nature04503
Thornhill, G. D., Mason, D. C., Dance, S. L., Lawless, A. S., Nichols, N. K., Forbes, H. R., et al. (2012). Integration of a 3D variational data assimilation scheme with a coastal area morphodynamic model of morecambe bay. Coast. Eng. 69, 82–96. doi: 10.1016/j.coastaleng.2012.05.010
van Maren, D. S., van Kessel, T., Cronin, K., and Sittoni, L. (2015). The impact of channel deepening and dredging on estuarine sediment concentration. Cont. Shelf Res. 95, 1–14. doi: 10.1016/j.csr.2014.12.010
Veloso-Gomes, F. (2016). “Zonas Costeiras: variabilicade climática, ações antropogénicas e alterações climáticas,” in II Congresso Ibero Americano de Gestão Integrada de Áreas Litorais, 757–758.
Viney, N. R., Bormann, H., Breuer, L., Bronstert, A., Croke, B. F. W., Frede, H., et al. (2009). Assessing the impact of land use change on hydrology by ensemble modelling (LUCHEM) II: ensemble combinations and predictions. Adv. Water Resour. 32, 147-158. doi: 10.1016/j.advwatres.2008.05.006
Vose, R. S, Applequist, S., Bourassa, M. A., Pryor, S. C., Barthelmie, R. J., Blanton, B., et al. (2014). Monitoring and understanding changes in extremes: extratropical storms, winds, and waves. Bull. Am. Meteorol. Soc. 95, 377–386. doi: 10.1175/BAMS-D-12-00162.1
Wandishin, M. S., Mullen, S. L., Stensrud, D. J., and Brooks, H. E. (2001). Evaluation of a short-range multimodel ensemble system. Mon. Weather Rev. 129, 729–747. doi: 10.1175/1520-04932001129<0729:EOASRM<2.0.CO;2
Weber de Melo, W.., Pinho, J., Iglesias, I.., Bio, A., Avilez-Valente, P., Vieira, J., et al. (2022). “Flood risk assessment at the douro river estuary,” in Climate Change and Water Security. Lecture Notes in Civil Engineering, eds S. Kolathayar, A. Mondal, and S. C. Chian (Singapore: Springer), 178. doi: 10.1007/978-981-16-5501-2.
Weigel, A. P, Liniger, M. A., and Appenzeller, C. (2008). Can multi-model combination really enhance the prediction skill of probabilistic ensemble forecasts? Q. J. R. Meteorol. Soc. 134, 241–260. doi: 10.1002/qj.210
Weigel, A. P., Knutti, R., Liniger, M. A., and Appenzeller, C. (2010). Risks of model weighting in multimodel climate projections. J. Clim. 23, 4175–4191. doi: 10.1175/2010JCLI3594.1
WMO (2012). Guidelines on Ensemble Prediction Systems and Forecasting. Geneva: World Meteorological Organization.
Woodcock, F., and Engel, C. (2005). Operational consensus forecasts. Weather Forec. 20, 101–111. doi: 10.1175/WAF-831.1
Yang-Ming, F., Shunqi, P., Jia-Ming, C., and Chia Chuen, K. (2013). “Ensemble wave forecasting over typhoon period,” in 2013 MTS/IEEE OCEANS - Bergen, (Bergen: IEEE), 1–7. doi: 10.1109/OCEANS-Bergen.2013.6608029
Keywords: estuaries, hydrodynamics, ensemble, numerical models, extreme events
Citation: Iglesias I, Pinho JL, Avilez-Valente P, Melo W, Bio A, Gomes A, Vieira J, Bastos L and Veloso-Gomes F (2022) Improving Estuarine Hydrodynamic Forecasts Through Numerical Model Ensembles. Front. Mar. Sci. 9:812255. doi: 10.3389/fmars.2022.812255
Received: 10 November 2021; Accepted: 11 January 2022;
Published: 03 February 2022.
Edited by:
Wei-Bo Chen, National Science and Technology Center for Disaster Reduction (NCDR), TaiwanReviewed by:
Marta Rodrigues, National Laboratory for Civil Engineering, PortugalRobert J. Weaver, Florida Institute of Technology, United States
Copyright © 2022 Iglesias, Pinho, Avilez-Valente, Melo, Bio, Gomes, Vieira, Bastos and Veloso-Gomes. This is an open-access article distributed under the terms of the Creative Commons Attribution License (CC BY). The use, distribution or reproduction in other forums is permitted, provided the original author(s) and the copyright owner(s) are credited and that the original publication in this journal is cited, in accordance with accepted academic practice. No use, distribution or reproduction is permitted which does not comply with these terms.
*Correspondence: Isabel Iglesias, aWlnbGVzaWFzQGNpaW1hci51cC5wdA==