- 1Department of Geography, National University of Singapore, Singapore, Singapore
- 2Centre for Nature-Based Climate Solutions, National University of Singapore, Singapore, Singapore
Extreme weather events are a cause of mangrove forest loss and degradation globally. Almost half of the world’s mangroves are found in the tropical cyclone belt, and forests often experience disturbance in structure, functioning and ecosystem service provision. Understanding the factors that increase the vulnerability of mangroves to such disturbances is a challenge. Using a novel remote sensing analysis combining water class change with vegetation classification, we showed that mangrove loss across multiple cyclone events is influenced by previous erosion history, suggesting that the prior state of the coastline affects susceptibility to future disturbance events. During Cyclone Amphan in May 2020, more than 1,200 km2 of mangroves were damaged and 40.6 km2 of shoreline was lost. Cyclone Amphan caused the most damage out of three recent cyclones, with the most mangrove loss (18.8%) experienced along shorelines that were eroding over the past 35 years. This can be explained by the long-term effect of erosion on the overall intertidal morphology of the shoreline. Landscape-scale mangrove management, particularly of sediment budgets is essential to switch previously eroding mangroves to a state where they can withstand cumulative storm impacts.
Introduction
Extreme weather events account for 11% of contemporary global mangrove forest loss, with their proportion in the 21st century increasing relative to human drivers of deforestation (Goldberg et al., 2020). Approximately 40% of the world’s mangrove forests are distributed in areas prone to cyclone activity; while evidence on the impacts of cyclones on mangrove biomass at the global scale is mixed (Simard et al., 2019; Rovai et al., 2021), cyclones have clear impacts on mangrove forests at the landscape scale in regions where they occur. Cyclones cause a range of disturbances on mangrove forest structure, functioning, and geomorphology, including immediate defoliation and short-term biomass loss, changes in carbon and nutrient cycling, peat collapse, and eventual marine transgression (Castañeda-Moya et al., 2010; Jones et al., 2019; Krauss and Osland, 2020). This leads to observable impacts on the ecosystem services that mangrove forests provide, such as their ability to store and sequester carbon to regulate the global climate (Friess et al., 2020; Peneva-Reed et al., 2021).
Despite adaptation to repeated disturbances, mangrove forests can suffer losses due to extreme weather events when interacting environmental stressors are present. Under certain environmental disturbances, mangrove forests can lose resistance to tropical cyclones which may increase the impact a single cyclone can cause (Vogt et al., 2012; Lewis et al., 2016). Past human disturbances to mangrove forest structures can alter the way they respond to or are impacted by cyclones (Krauss and Osland, 2020). However, it is not clear if past shoreline dynamics influence their vulnerability to cyclone damage and shoreline loss. Understanding the factors contributing to mangrove damage and recovery in response to cyclones is important to ensure that shorelines are protected from further damage and are resilient to disturbances. Such information will allow us to evaluate the suitability of mangrove forests as a tool for ecological disaster risk reduction in cyclone-prone areas.
The Sundarbans mangrove forest is a suitable area to test hypotheses of the role that cyclone history plays in determining future disturbance vulnerability; the Sundarbans are considered one of the largest contiguous mangrove areas in the world, and its location in the Bay of Bengal means it is particularly exposed to frequent high-magnitude tropical storm and cyclone events. Despite the potential ability of the Sundarbans mangroves to protect local communities from natural hazards (Akber et al., 2018), the mangroves are themselves damaged by winds, waves, and storm surges associated with cyclones in the Bay of Bengal. The Sundarbans have experienced permanent, seasonal, and ephemeral changes to their shoreline over the past 35 years (Bhargava et al., 2020), and long-term mangrove degradation and changes in forest density have been observed (Quader et al., 2017), particularly in response to category three or higher cyclones (Mandal and Hosaka, 2020). Anecdotally, on-the-ground observations suggest substantial mangrove damage occurred after Cyclone Amphan, the most recent cyclone to affect the area in May 2020 (Sud and Rajaram, 2020).
We determined the relationship between historic shoreline dynamics and mangrove susceptibility to contemporary cyclone activity, using the ∼10,000 km2 Sundarbans mangrove forest as a model. The long-term trends are used to contextualize the results of short-term cyclone impact. The short-term cyclone impact is estimated using remote sensing classification of images collected just before and after the impact. We then investigated the relationship between mangrove damage and current shoreline losses, and historical shoreline retreat trajectories. The spatial extent of this study is transboundary and covers the entire Sundarbans across both India and Bangladesh. The temporal extent of this study covers three major cyclones that occurred between 2019 and 2020: Cyclone Fani (May 2019, Category 4), Cyclone Bulbul (November 2019, Category 3), and Cyclone Amphan (May 2020, Category 5).
Materials and Methods
Study Site Description
The Sundarbans are a cluster of low-lying islands covering ∼10,000 km2, with an elevation of fewer than 5 m located in the Ganga, Brahmaputra, and the Meghna river delta. The Sundarbans are shared between India (38%) and Bangladesh (62%). The mangrove forests of the Sundarbans have been protected as a UNESCO World Heritage Site in both countries since 1987 due to its rich biodiversity of flora and fauna, including the Royal Bengal Tiger (Panthera tigris), the Ganga River Dolphin (Platanista gangetica), and the critically endangered endemic River Terrapin (Batagur baska). Approximately 14 million people depend on the varied ecosystem services of the Sundarbans, making it crucial for the region (Ortolano et al., 2016; Islam et al., 2018). The delta is fed in by substantial volumes of sediment, mainly from the Ganga River, and receives an annual rainfall of ∼1,700 mm.
The Sundarbans experience 5–6 cyclones annually, of which two can be of severe category. Over the last 40 years, the Bay of Bengal region has experienced 255 cyclonic storms ranging from low to severe categories (Singh, 2007). Cyclone intensity has increased over the last 100 years. Between 2019 and 2020, three cyclones in the Bay of Bengal region impacted the Sundarbans (Figure 1 and Table 1). Cyclone Fani (May 2019) was a category 4 cyclone and one of the most severe in the Indian state of Odisha since 1999. Cyclone Fani impacted approximately 1 million people from India and Bangladesh, with total mortality of 89 and damage amounting to USD 8.1 billion. The next severe cyclone in 2019 was Cyclone Bulbul (November 2019) was a category 3 cyclone that occurred during the post-monsoon cyclone season. Total fatalities across India and Bangladesh were 41 with the damage of about USD 3.37 billion. The most recent cyclone that impacted the Sundarbans region was Cyclone Amphan (May 2020); the first category five cyclone to affect the Sundarbans in the past two decades. Anecdotally, Amphan had the highest impact in India and Bangladesh of the three cyclones, with a loss of 128 lives and >USD 13 million in damages (Sud and Rajaram, 2020).
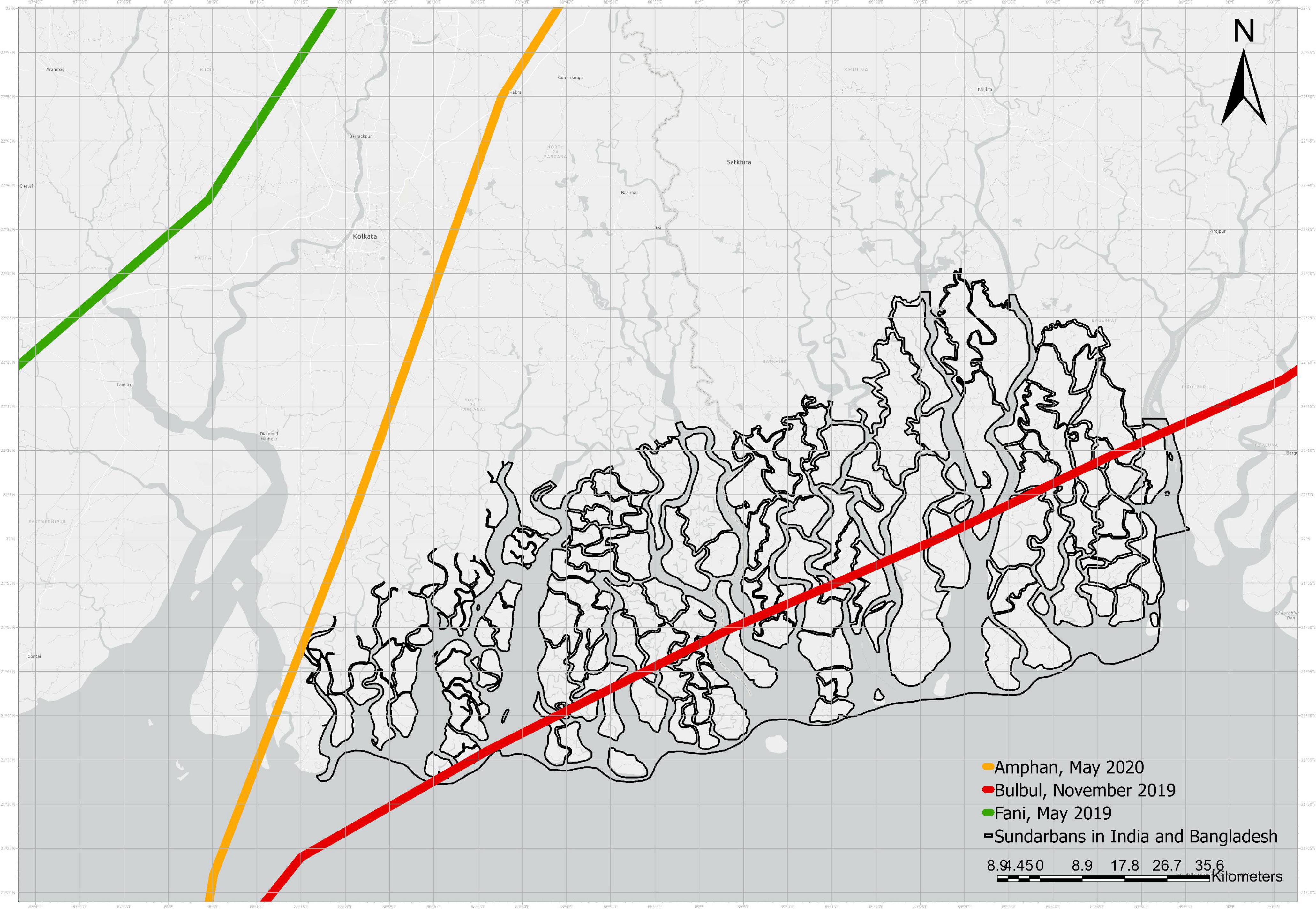
Figure 1. Cyclone distribution and occurrence across the Sundarbans in India and Bangladesh. The white dot on the inset map is the location of the Sundarbans in India and Bangladesh. The gray lines are the trajectory of Cyclone Fani (Category 4, 3-min sustained highest winds: 215 km/h, 1-min sustained highest winds 280 km/h, lowest pressure 932 hPa), Bulbul (Category 3, 3-min sustained highest winds: 140 km/h, 1-min sustained highest winds 195 km/h, lowest pressure 976 hPa), and Amphan (Category 5, 3-min sustained highest winds: 240 km/h, 1-min sustained highest winds 280 km/h, lowest pressure 920 hPa).
Defining Shoreline, Loss, and Damage
The shoreline is defined using the Mentaschi et al. (2018) dataset and Bhargava et al. (2020) as a base for analysis. These studies used the Global Surface Water Exchange Dataset (GSWE; Pekel et al., 2016) to create interpretations of shoreline gain or loss. Mentaschi et al. (2018) created virtual orthogonal transects of varying lengths starting from permanent water to permanent land (from GSWE) covering a significant area of the coastline to analyse shoreline dynamics. Bhargava et al. (2020) used this interpretation of the shoreline to extract shoreline dynamics for the Sundarbans, and this manuscript used the results from Bhargava et al. (2020) to define dynamic shoreline along with a 60 m buffer area to capture changes due to concurrent cyclones (described in detail below).
Any changes from permanent water to land and land to permanent water in the impact of cyclones on mangroves was divided into loss and damage. Damage was defined in this study as loss of a pixel defined as “mangrove” from the remote sensing classification process (detailed below). This is attributed to the visible impact caused by cyclones on mangrove forests structure where the mangrove landmass is not lost, but the mangrove canopy is disturbed. The disturbance to the mangrove canopy could happen due to various factors including stem defoliation, stem breaking, bark shredding, or complete loss of a tree.
Loss of mangrove shoreline is defined as a loss in mangrove pixel and gain in water pixel during the change detection and classification process. The appearance of a water pixel instead of a mangrove pixel denotes that mangroves in that area were lost to water. This cannot mean the area is seasonally flooded as the pixel of 25 m tall mangrove trees in a 30 m-by-30 m plot would be classified as a mangrove pixel, not a water pixel.
Data Compilation and Processing
Several different datasets were compiled to understand shoreline erosion dynamics and mangrove loss in the Sundarbans (Figure 2). Sentinel 1 and 2 images which are satellite imagery collecting Synthetic Aperture Radar (SAR) data were used for water and mangrove classifications, respectively (Table 2), and for accuracy assessment. Bunting et al. (2018) dataset classifies mangrove forests at a global scale for the year 2016 by using machine learning techniques (accuracy 89%, kappa-value 0.87; Thomas et al., 2017) was used to train the classifier for mangrove classification. For the identification of areas with a history of shoreline dynamics, data from Bhargava et al. (2020) [accuracy 92%, kappa-value 0.83, based on GSWE Pekel et al. (2016) and Mentaschi et al. (2018)] were used. The spatial scale of analysis was 30 m. As multiple datasets were used with varying resolutions (GSWE-30 m, Landsat-30 m, Sentinel 2-some bands at 20 m, some at 10 m, and Sentinel 1-10 m), the minimum resolution was 30 m which is then used for the overall analysis.
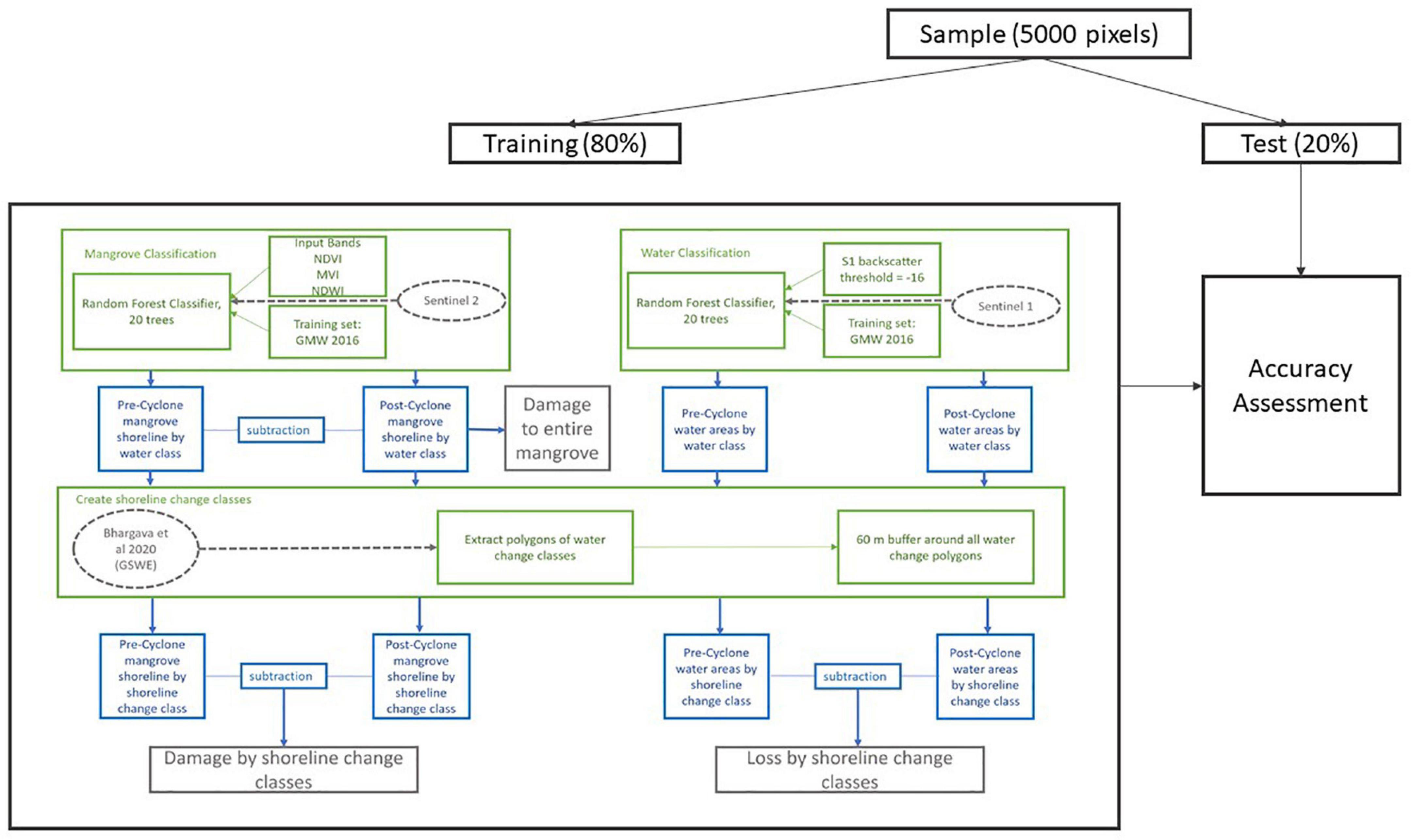
Figure 2. Data collection and analysis framework for assessing mangrove forest and shoreline retreat.
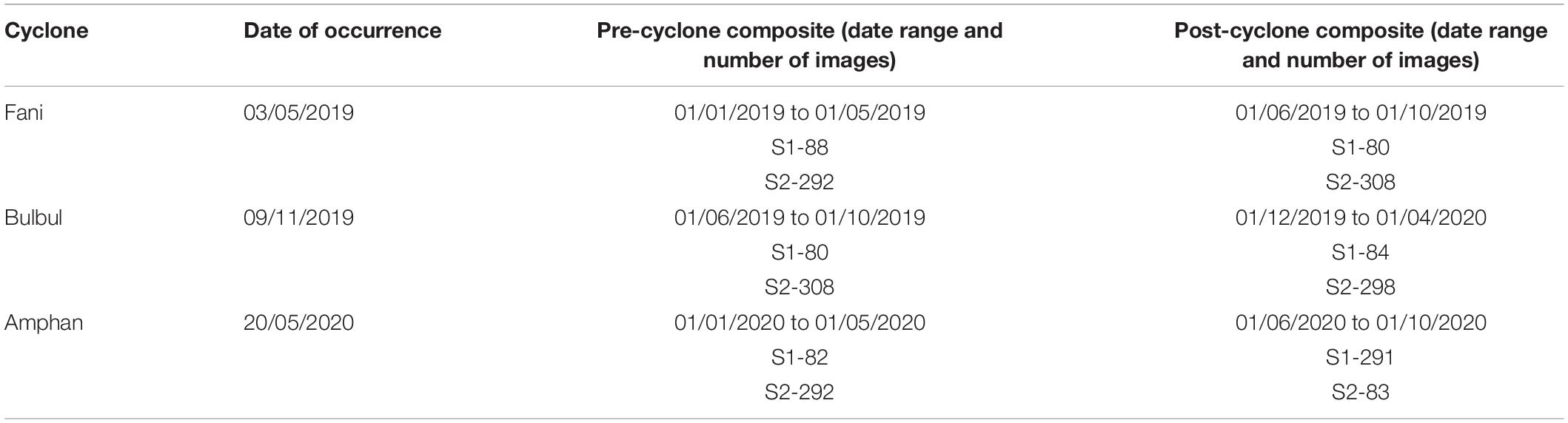
Table 2. Range and number of Sentinel 1 (S1) and Sentinel 2 (S2) imageries used to create the pre-and post-cyclone composite scenes (for specific dates refer to Supplementary Table A).
Meteorological data on the three cyclones, including cyclone track points, were collected from International Best Track Archive for Climate Stewardship (IBTrACS) database (Knapp et al., 2010) and Global Disaster Alert and Coordination System (GDACS) which collects information on the trajectory, time, location, speed, etc., for each cyclone. Google Earth Engine (GEE) was used for image processing and data analysis (explained below). The estimation of cyclone impact (damage and loss) on mangroves was performed (as described below) for the three cyclones in 2019 and 2020, namely-Cyclone Fani, Cyclone Bulbul, and Cyclone Amphan for the entire mangrove forest extent of the Sundarbans (Figure 1).
Classification and Change Detection
Mangrove areas in the Sundarbans were identified through supervised classification using a Random Forest (RF) Classifier (Breiman, 2001), a machine learning algorithm used for the classification of pixels based on a decision tree that is trained on known areas. RF is widely used in the classification of large-scale satellite data for land cover studies (Rodriguez-Galiano et al., 2012). This study calculated the Mangrove Vegetation Index (Baloloy et al., 2020), Normalized Difference Vegetation Index (NDVI), Normalized Difference Water Index (NDWI), and GMW (Bunting et al., 2018) raster image to train the classifier. Finally, a composite of Sentinel 2 images 4 months before a cyclone was created and labeled as pre-cyclone, and similarly, images from 4 months after a cyclone were used to create a composite of post-cyclone images. In this way, three sets of pre-cyclone and post-cyclone composites (Table 2) were created, which were classified as mangrove or non-mangrove using the RF classifier. Due to high cloud cover during the cyclone period, image mosaics were created by averaging various images from across 4 to 5 months period before and after the cyclone.
Pre- and post-cyclone water classification images were created with Sentinel-1 for the same periods as specified above. The S1 backscattering threshold of −16 (sensitivity 4%, Supplementary Figures 14, 15) was used and all the pixels less than −16 were labeled as water. The threshold was selected based on the highest accuracy in comparison with the training dataset.
Next, using the output from Bhargava et al. (2020), areas that went through different types of shoreline dynamics between 1985 and 2018 were converted into polygons. A buffer of 60 m was created around each polygon to capture areas adjacent to the dynamic shoreline. A buffer was created so that mangrove areas adjacent to the shoreline could be analyzed. Thus, the definition of shoreline in this study included a 60 m buffer around the water classes. This area is called the shoreline area in this manuscript. As the resolution of the final dataset was 30 m, a buffer of 60 m captured at least 2 pixels worth of change in the nearby areas.
To estimate the change in terms of damage of mangroves due to a cyclone, classified mangrove images were clipped to the extent of the shoreline described above, and then the pre-cyclone image was subtracted from the post-cyclone image to get the resultant change image. Finally, pixels falling under the different water change classes were aggregated for each cyclone. Similar steps of creating pre and post-cyclone images were followed for the water classification image to estimate the change in terms of loss.
Accuracy Assessment
The accuracy assessment was conducted by dividing the sample (5,000 pixels) into training (80%) and test (20%) sets. The training was performed using the RF classifier and bands discussed above and the same classification method was used on the test (validation) set. To test the accuracy of the classification method, the results from training and test were compared using a confusion matrix. Accuracy statistics from the training set showed how well the classifier (model) interprets the image (mangrove and water classes- presented in Table 3 as classification accuracy), whereas the accuracy statistics from the test set showed how well the classifier (model—presented in Table 3 as model accuracy) fits unknown data (Maxwell et al., 2021). The test data are randomized, independent, and unbiased samples that do not overlap with training samples. The training accuracy statistics should be used to assess the accuracy in interpreting the mangrove and water classes used in this study, and the test accuracy statistics should be used to assess the accuracy of the classifier when applied to a different set of data (Hoeser et al., 2020; Hoeser and Kuenzer, 2020).

Table 3. Consumers accuracy, producers accuracy, and kappa-values of classification (training sample) and model (test sample).
For the test set, a confusion matrix was generated which compares the prediction with the true class, whereas for the training set a confusion matrix was created. Using the confusion matrices, overall accuracy, kappa-value, consumer accuracy, and producer accuracy were calculated (Table 3 and Supplementary Table A).
The average overall accuracy of the interpretation of mangrove and water classes (training set) is 97.5% with a minimum kappa-value of 0.93 meaning that the overall training accuracy assessment was 93% better than a chance occurrence. Additionally, the overall accuracy of the model on new data would be an average of 86.9% with a minimum kappa-value of 0.57 meaning that the overall test accuracy assessment was 57% better than chance. Hence, the results presented in the following sections should be interpreted with a minimum accuracy of 93% and if the model used to classify the data is replicated on new data, then it is cautioned that the minimum accuracy would be 58%. The users would have to utilize additional measures like changing band ratios to improve the model for their specific dataset.
Mapping Historical Shoreline Dynamics
Historical shoreline dynamics maps were adopted from Bhargava et al. (2020) where shoreline dynamics was defined as changes in the presence of land to water and vice versa. The classification for historical shoreline dynamics was adopted from the GSWE (Pekel et al., 2016) where each pixel was classified into land and water. The water pixels were further classified into permanent, seasonal, and ephemeral classes. The signature of these changes over the 1985–2018 period. If permanent and seasonal changes are only present during a short time in the 35 years, then it was classified as either ephemeral permanent change or ephemeral seasonal change. Lastly, changes from permanent waters to seasonal waters or seasonal waters to permanent waters are also included. The shorelines that did not change were labeled as either permanent water or seasonal water depending on the permanence of water occurrence near that shore. Finally, change from land to water is defined as erosion (permanent and seasonal), and change from water to land is defined as progradation (permanent and seasonal) (Table 4). These classes were used to label different shoreline types in the analysis. Image pixels falling under different classes were aggregated to obtain the total pixel area covered by each class.
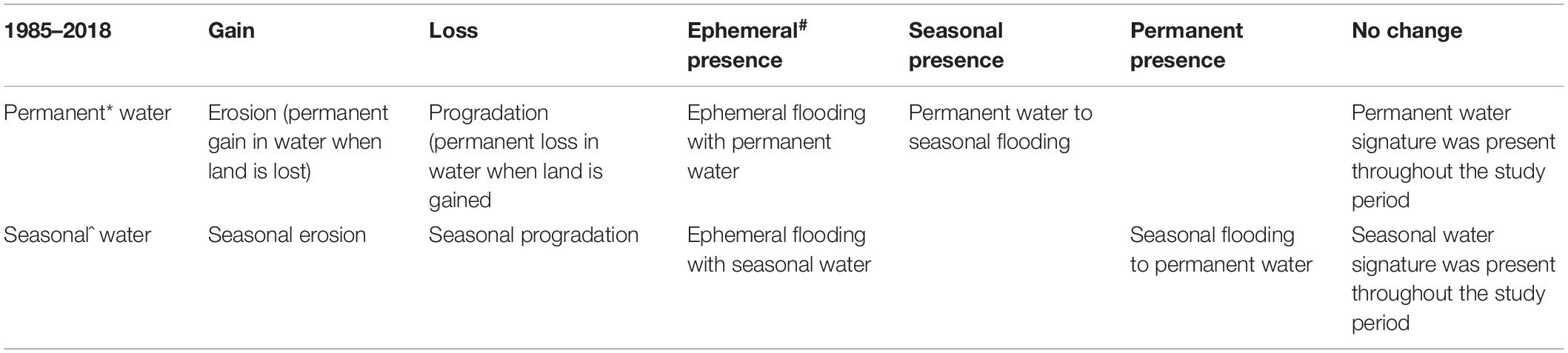
Table 4. Shoreline retreat classes [*Permanent—Water signature is present in all the months for the 35 years analysis period, ^Seasonal—reoccurrence of water within a year, in other words, presence of water for less than 12 months each year for the 35-year analysis period, #Ephemeral- water signature present for any given time during the 35-year analysis period, adopted from Pekel et al. (2016)].
Shoreline retreats were not limited to permanent loss or gain, there was multiple seasonal and ephemeral dynamism active along the shore. Understanding these short-term changes is important, especially in terms of understanding the shoreline vulnerability to extreme weather events. Therefore, the short-term cyclone damage and loss were understood with the previous shoreline dynamics to understand and compare spatial vulnerability.
The benefit of using the GSWE dataset and adopting results from Bhargava et al. (2020) was the ability to capture seasonal and ephemeral changes that qualitatively accounts for the effects of tides, waves, storm surges, and river floods. Since the analysis in this manuscript was based on the GSWE dataset, explicitly extracting tidal areas was not necessary. However, a disadvantage of using GSWE was that it does not generate a current or changed location of a shoreline or a coastline.
Results
Net Erosion and Cyclone Damage on Mangroves and Mangrove Shorelines
Cyclone Amphan was the most powerful cyclone of those studied, and caused the highest damage across the entire Sundarbans, followed by Cyclone Fani and Cyclone Bulbul (Figure 3). A similar pattern of damage was observed in shoreline areas. Across all three cyclones, more than one-third of the total damage was in the shoreline mangrove areas. The net erosion of shoreline was highest during Cyclone Bulbul and lowest during Cyclone Fani, additionally, erosion as a proportion to shoreline damage was highest (14.47%) during Cyclone Bulbul and least for Cyclone Amphan (9.96%). More than 13.2% of the damaged shoreline (457.87 km2 in total) eroded as a cumulative impact of the three cyclones.
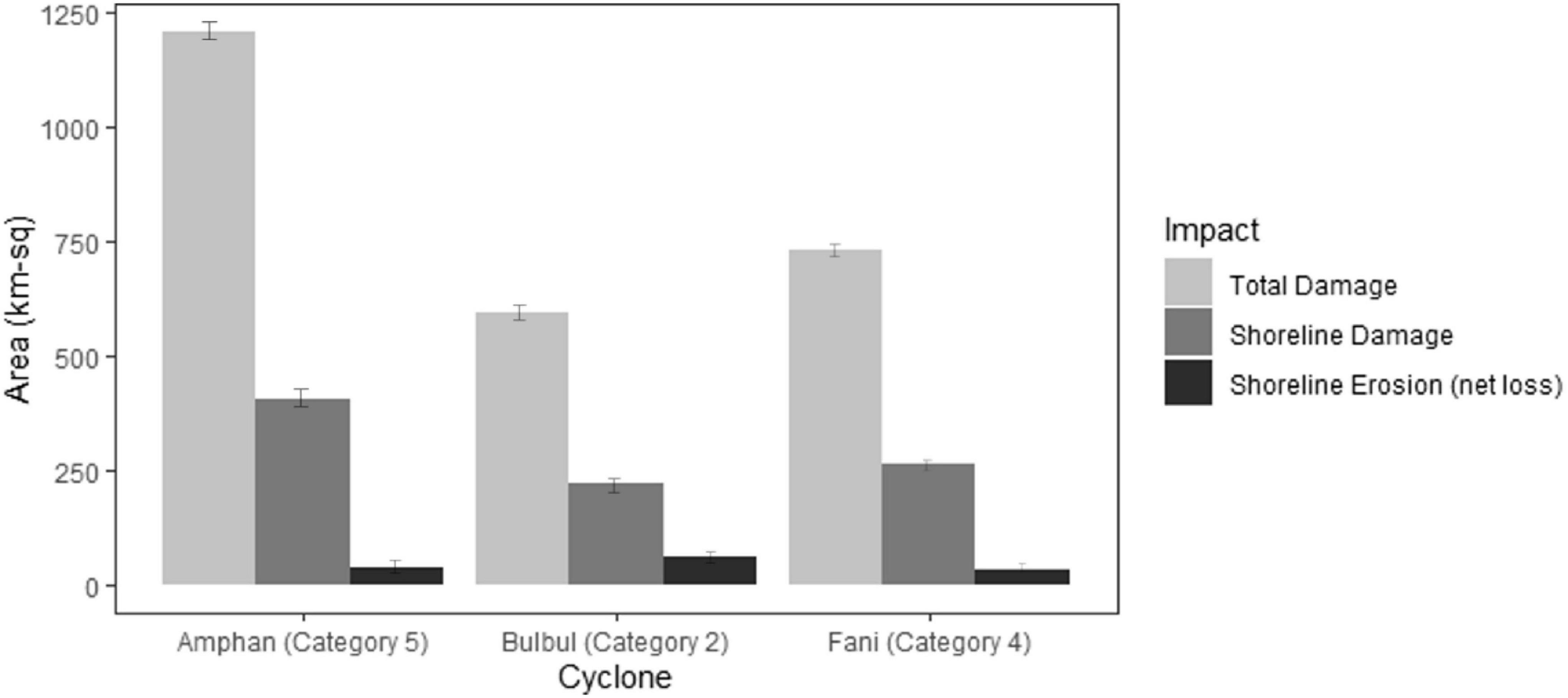
Figure 3. Area of shoreline impact due to Cyclone Amphan (error = 6.7%), Cyclone Bulbul (error = 5.9%), and Cyclone Fani (error = 6.15%) categorized into total damage to entire mangrove extent, the total damage to shoreline mangroves and erosion of shoreline (net loss).
The impact to the entire extent of the Sundarbans and the shoreline mangrove areas were observed in the same areas persistently through the three cyclones (Figure 4). Eastern Sundarbans (Bangladesh side) was the hotspot areas of damage. Finally, the hotspot area of loss is in the west-central and east toward the landward side of the forest.
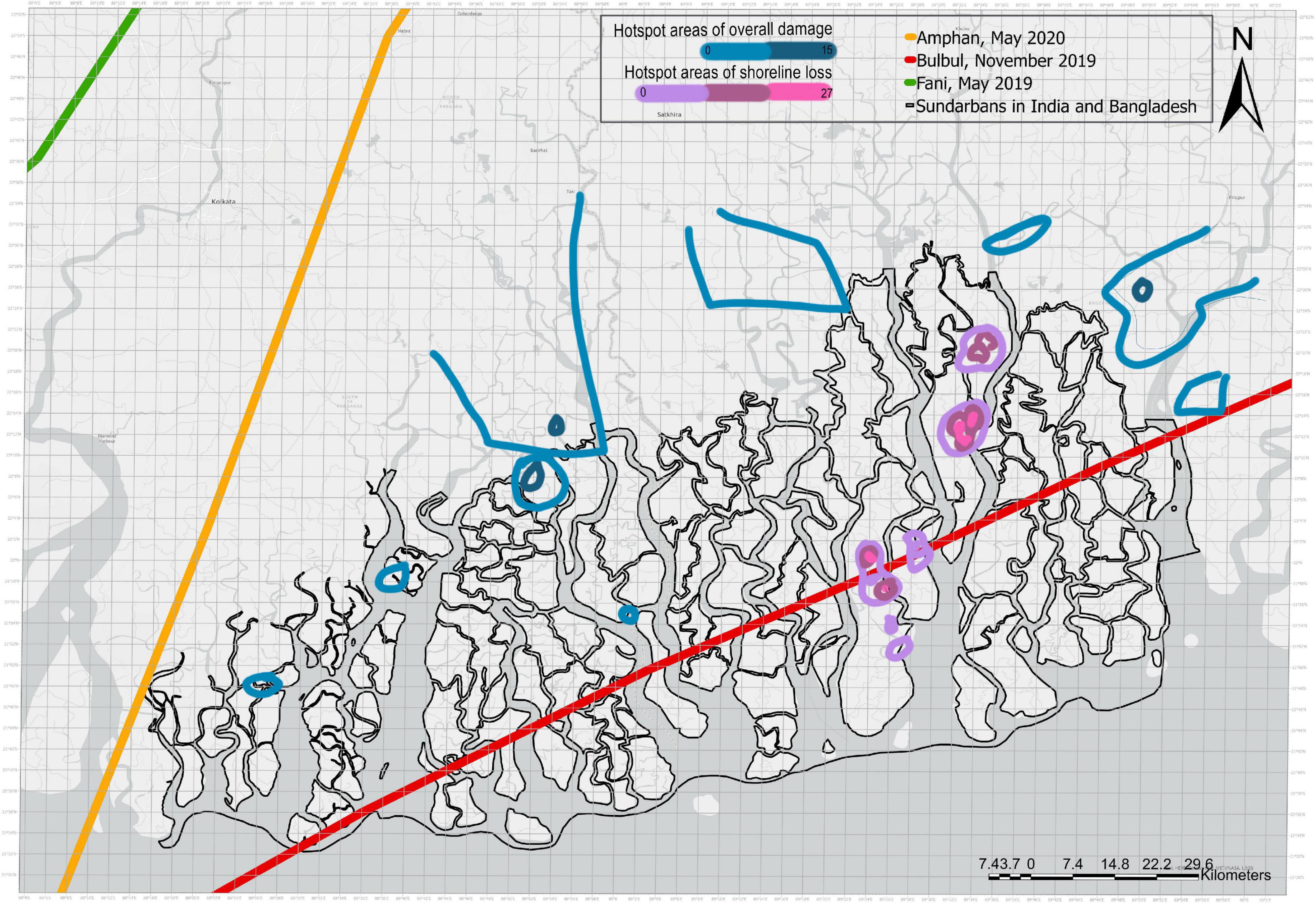
Figure 4. Spatial distribution of hotspot areas of damage (entire mangrove and shoreline) and shoreline loss (erosion) in the Sundarbans as a cumulative impact of Cyclone Amphan, Bulbul, and Fani. The white dot in the inset image is the location of the Sundarbans in India and Bangladesh. The color scale ranging shows the hotspot areas of mangrove forest area damage throughout the Sundarbans and loss along with the shoreline areas. The orange areas show areas with higher cumulative damages across the three cyclones and the pink areas show higher cumulative losses in the shorelines areas across the three cyclones. The scale is a unitless normalized value based on loss in the area. This image is a simplified version of a 10,000 km2 area. The hotspot areas that appear inland, are along river channels.
Influence of Historical Shoreline Dynamics on Mangrove Resilience
Cumulative cyclone damage was highest in the areas with a history of seasonal water (15.01% of total damage), prograding (14.15%) and other seasonally changing areas whereas loss were highest in historically eroding areas (18.81%) followed by seasonally eroding areas (17.2%) and other seasonally changing areas. Historically prograding areas with the highest damage during Cyclone Bulbul shows a different relationship as compared to the trends of the other two cyclones (Figure 5). The trend of damage and loss of shoreline mangroves follow a similar pattern across the historic shoreline classes persistently during the three cyclones (Figures 5, 6).
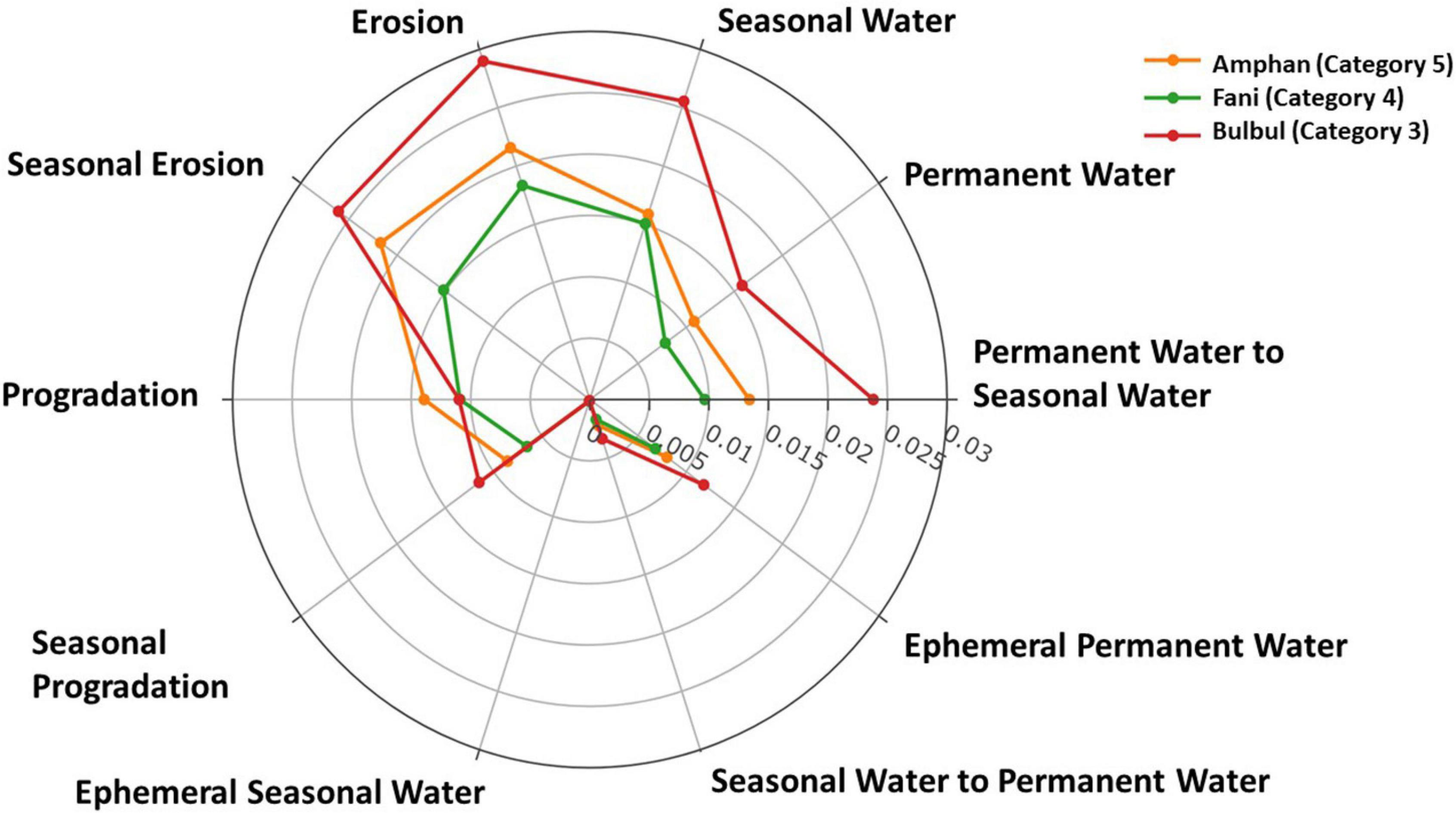
Figure 5. Damage to different shoreline classes due to Cyclone Amphan (Orange), Cyclone Bulbul (Red), and Cyclone Fani (Green).
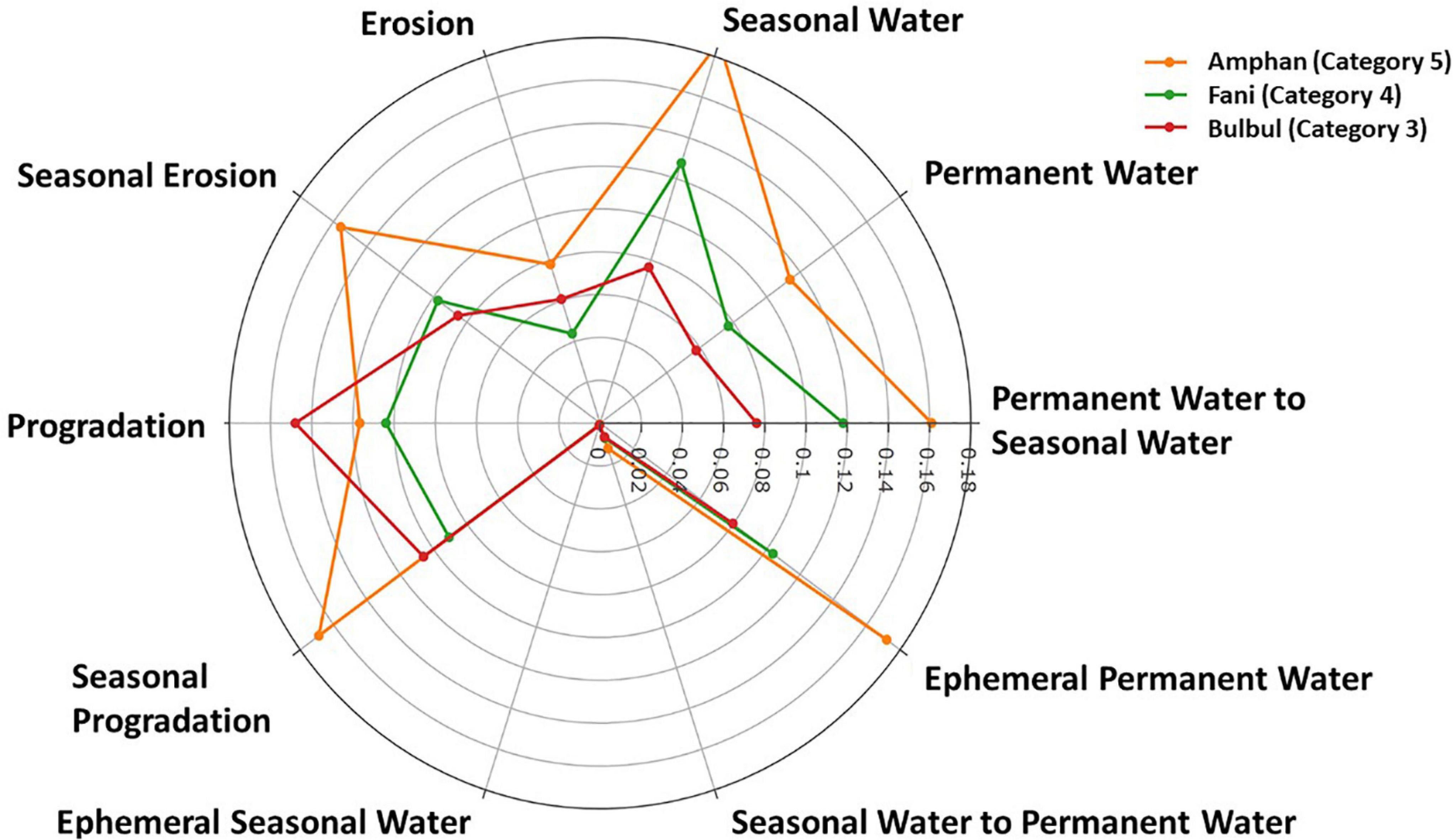
Figure 6. Loss of different shoreline classes due to Cyclone Amphan (Orange), Cyclone Bulbul (Red), and Cyclone Fani (Green).
The amount of damages are highest for most classes during Cyclone Amphan, followed by Cyclone Fani, and least for most classes during Cyclone Bulbul. In comparison to Cyclone Fani, Cyclone Bulbul caused higher damages in the seasonally prograding, prograding, and eroding classes. As compared to Cyclone Amphan, Cyclone Bulbul suffered higher losses in the prograding class. However, the amount of losses was highest during Cyclone Bulbul across all the classes except for the prograding class. Cyclone Amphan and Fani caused a similar trend of losses across all the classes.
Areas with a history of seasonal waters and seasonally eroding areas suffered the most amount of damages and losses, additionally, eroding areas suffered a lesser proportion of damage but a higher proportion of loss across the three cyclones. Losses due to Cyclone Amphan and Fani followed a similar pattern, however, the dip in the pattern in the progradation class during Cyclone Bulbul is showcasing a different trend. In addition, the progradation class does not follow a pattern across the three cyclones in the damage pattern.
Discussion
Net Erosion and Cyclone Damage on Mangroves and Mangrove Shorelines
Cyclone Amphan is one of the most powerful storms to affect the Sundarbans in recent years and has been classified by some as a super cyclone, with strong winds and rainfall exceeding 600 mm per day (Mishra and Vanganuru, 2020). Anecdotal reports suggest 1,200 km2 of mangrove forest was severely damaged after Cyclone Amphan (Basu, 2020), which is comparable to the amount of damage reported in this study (1210.50 km2). Mandal and Hosaka (2020) showed that among the 21 cyclones that affected the Sundarbans between 1988 and 2016, Cyclone Sidr (November 2007; category 5) had the most severe impact, affecting about 1,290 km2 of total mangrove area which is also comparable to the Category 5 Cyclone Amphan. Additionally, Cyclone Rashmi (October 2008; tropical storm) and Cyclone Aila (May 2009; Category 1) damaged mangroves in similar areas as reported in this study (Dutta et al., 2015). Based on these observations, the west-central and eastern sides of the Sundarbans are the most vulnerable to cyclone damage and losses. This trend is despite different cyclone trajectories (Figure 1). However, the trend of cyclone damage can be influenced by species, diameter at breast height (DBH) of the species, and/or distance from the coast (Halder et al., 2021).
Influence of Historical Shoreline Dynamics on Mangrove Resilience
Areas with a history of erosion are the most vulnerable to loss during a cyclone. Although several classes (like seasonally changing and prograding) were damaged at least twice as much as eroding areas, overall losses were highest in eroding areas. For instance, 15.01% (highest) of total damage was in areas with a history of seasonal waters but only 14.43% of total damage to seasonal water areas translated into loss, whereas, eroding areas suffered 7% of total damage and 37.72% was translated into a loss. This can be explained by the long-term effect of erosion on the overall intertidal morphology of the shoreline (Strahler, 1952). Eroding shorelines are typically characterized by an unstable concave cross-shore profile, which allows waves to reach further landward, eroding further. This positive feedback means that shorelines with a previously eroding trajectory become more susceptible to future erosion (Winterwerp et al., 2013) unless sediment budgets can be increased to switch the intertidal profile to an accreting convex profile. When eroding shorelines are exposed to extreme weather events like cyclones, they are lost at an accelerated rate. This is important not only for historically eroding shorelines but also for seasonally eroding shorelines which suffered excessive damage during cyclones. In the future, with excessive erosion and mangrove degradation, these vulnerable shorelines would develop a concave-up morphology which would lead to a negative feedback loop for further erosion and mangrove loss.
The damage due to Cyclone Bulbul was highest in historically prograding areas as compared to all the other areas. The process of tidal buffering can explain why prograding areas suffered relatively small amounts of losses during Cyclone Amphan and Fani as compared to Cyclone Bulbul. Category 4 and 5 cyclones can produce a higher storm surge compared to category 3 cyclones. Under a higher storm surge alongside rising tidal conditions, the low-lying mangrove areas like the prograding areas are submerged. The submergence protects them from damage due to wind bursts and excessive wave action during a cyclone (Ferwerda et al., 2007). This buffering effect has been observed in the Exmouth Gulf (Paling et al., 2008) in Australia. Therefore, in the case of more intense cyclones under high tide conditions, prograding areas are shielded and suffer fewer losses as might have been in the case of Cyclone Amphan and Fani, but during Cyclone Bulbul where the storm surge was lower, and the tidal height was also falling (The Indian Express, 2019), the low-lying prograding areas were not shielded from cyclone effect and experienced greater damage.
Mangrove Erosion as a Cause of Global Mangrove Loss
Mangrove loss due to erosion is an increasing cause of global concern for mangrove conservation. Currently, damage triggered by extreme weather events accounts for 11% of the global mangrove loss (Goldberg et al., 2020). With the increase in cyclone activity due to global warming, this proportion is likely to increase in the Sundarbans and across the world. As mangroves are commonly found in cyclone-prone areas, their increasing vulnerability to damage and loss due to a cyclone is a critical problem to mangrove conservation.
The permanence of the impact of cyclones on mangroves depends on the resilience of the ecosystem. Between 1985 and 2018 the Sundarbans lost 136.77 km2 of mangroves to erosion and gained 62.2 km2 (Bhargava et al., 2020) due to progradation; however, in 2019 and 2020 alone there was a further net erosion of 133.47 km2 due to the three cyclones. Mangroves are susceptible to such disturbances and have shown recovery as rapidly as a year after cyclone damage (Walker, 1991; Bhowmik and Cabral, 2011; Barr et al., 2012). For example, in Puerto Rico cyclone impacted mangrove forests saw 72% canopy cover recovery within 11 months of the 2017 hurricane season (Branoff, 2020) and in Myanmar, approximately 60% of mangroves recovered and stayed intact after 4 years of Cyclone Nargis (Aung et al., 2013). Similarly, after Typhoon Haiyan in the Philippines, mangrove forests with low, mid, and severe impacts recovered within 18 months of cyclone damage (Long et al., 2016). On the contrary, even after 14 years of cyclone damage severely impacted mangroves in Mozambique saw no recovery (Macamo et al., 2016). These patterns are also dependent on the frequency of cyclones in a region, where multiple cyclones could lead to excess mangrove damage (Taillie et al., 2020). Such differences in post-cyclone mangrove recovery can occur due to the cumulative impact of multiple stressors in addition to weather extremities (Smith et al., 2009; Sippo et al., 2018).
The historical water trajectory of the shoreline can affect its susceptibility to cyclone damage. Initial system properties define how mangroves are impacted by cyclones and how mangroves will regrow after cyclone impact (Krauss and Osland, 2020). Additionally, cumulative cyclone impact can reduce cyclone recovery and worsen the impact of each consecutive cyclone (Taillie et al., 2020). Mangroves are also known to regenerate upon cyclonic disturbances under specific conditions (Walker, 1991; Bhowmik and Cabral, 2011; Barr et al., 2012). For instance, mangroves in the well-drained areas regenerated with a year of cyclone damage but mangroves in the poorly drained inland areas suffered about 10,000 ha of cyclone triggered die back (Lagomasino et al., 2021). Additionally, when mangrove shorelines experience dynamic changes over a long time in addition to long-term anthropogenic stressors and increasing cyclone intensity, mangroves can become less resilient to extreme weather events.
Implications for Managing and Conserving Cyclone-Prone Shorelines
The intensity of extreme weather events such as cyclones is likely to increase in the future, with region-specific concomitant impacts on mangrove forests (Ward et al., 2016). Understanding the influence of previous shoreline dynamics on contemporary sensitivity to cyclones is important when making projections of future vulnerability or mangrove loss. Such information can contribute to participatory assessments of mangrove vulnerability to natural hazards and climate change (Ellison, 2016). Vulnerability models are useful to highlight landscapes and shorelines where preemptive management may be required to reduce vulnerability. In the Sundarbans, shorelines with low resilience and in need of urgent management interventions are identified in the east-central (Bangladesh) and north-western (India).
Once vulnerable shorelines have been identified based on their previous erosion dynamics, they can be targeted with specific management interventions that aim to flip the system from an eroding to a prograding trajectory. For minerogenic systems, this is commonly done through reduction of wave exposure combined with larger-scale changes in sediment budget. Interventions such as permeable dams aim to reduce water flows and encourage sediment deposition behind them (Winterwerp et al., 2020), to change the intertidal profile from an erosive concave profile to a prograding convex profile. This approach has been deployed to arrest long-term erosive shoreline trajectories in locations such as Indonesia, Thailand, and Suriname, with mixed effectiveness (Winterwerp et al., 2020).
Conclusion
A substantial proportion of the world’s mangrove forests are impacted by cyclonic activity, and while we have an improved understanding of mangrove dynamics and response to storms at the stand level (Krauss and Osland, 2020), this study contributes insights to how mangrove forests respond to cyclones at the landscape level. Importantly, landscape-scale mangrove response to cyclone activity is influenced temporally, and system resilience is in part a response to the historical trajectory of the shoreline where historically erosion-prone shorelines become more vulnerable to losses due to a cyclone. Cyclone Amphan caused the most damage out of three recent cyclones, and overall, the most mangrove loss was along shorelines that were eroding over the past 35 years. This can be explained by the long-term effect of erosion on the overall intertidal morphology of the shoreline. Thus, the history of shoreline disturbances can impact future susceptibility to cyclones. This has important implications for regions such as South Asia that experience frequent and regular cyclones, suggesting that mangrove management should include the landscape-level differences in shoreline retreat history and response to cyclones and shoreline-specific measures should be taken to support resilient mangrove ecosystems.
Data Availability Statement
The original contributions presented in the study are included in the article/Supplementary Material, further inquiries can be directed to the corresponding author.
Author Contributions
RB: conceptualization, methodology, software, validation, formal analysis, investigation, resources, data curation, writing—original draft, writing—review and editing, and visualization. DF: conceptualization, methodology, resources, writing—original draft, writing—review and editing, visualization, supervision, and project administration. Both authors contributed to the article and approved the submitted version.
Funding
RB was partially supported by the National Geographic Society Early Career Grant (EC-78131C-21) and the National University of Singapore President’s Graduate Fellowship (funded by the Lee Kong Chian Scholarship). Software support (ArcGIS license) was provided by the Geospatial Analysis Lab at the University of San Francisco.
Conflict of Interest
The authors declare that the research was conducted in the absence of any commercial or financial relationships that could be construed as a potential conflict of interest.
Publisher’s Note
All claims expressed in this article are solely those of the authors and do not necessarily represent those of their affiliated organizations, or those of the publisher, the editors and the reviewers. Any product that may be evaluated in this article, or claim that may be made by its manufacturer, is not guaranteed or endorsed by the publisher.
Acknowledgments
We acknowledge Dipto Sarkar (Carleton University) for his contribution to the shoreline retreat class dataset (Bhargava et al., 2020) and Nicole Cormier (Macquarie University) for insightful inputs on a previous draft. The Mangrove Lab and Tropical Environmental Change Group (National University of Singapore) provided comments on an initial version of this manuscript. The West Bengal Forest Department is thanked for its support throughout this project.
Supplementary Material
The Supplementary Material for this article can be found online at: https://www.frontiersin.org/articles/10.3389/fmars.2022.814577/full#supplementary-material
References
Akber, M. D. A., Patwary, M. M., Islam, M. D. A., and Rahman, M. R. (2018). Storm protection service of the Sundarbans mangrove forest, Bangladesh. Nat. Hazards 94, 405–418. doi: 10.1007/s11069-018-3395-8
Aung, T. T., Mochida, Y., and Than, M. M. (2013). Prediction of recovery pathways of cyclone-disturbed mangroves in the mega delta of Myanmar. For. Ecol. Manag. 293, 103–113. doi: 10.1016/j.foreco.2012.12.034
Baloloy, A. B., Blanco, A. C., Ana, R. R. C. S., and Nadaoka, K. (2020). Development and application of a new mangrove vegetation index (MVI) for rapid and accurate mangrove mapping. ISPRS J. Photogramm. Remote Sens. 166, 95–117. doi: 10.1016/j.isprsjprs.2020.06.001
Barr, J. G., Engel, V., Smith, T. J., and Fuentes, J. D. (2012). Hurricane disturbance and recovery of energy balance, CO2 fluxes and canopy structure in a mangrove forest of the Florida Everglades. Agric. For. Meteorol. 153, 54–66. doi: 10.1016/j.agrformet.2011.07.022
Basu, J. (2020). Plant 50 million mangroves in the Sundarbans? Improbable, say experts. Down to Earth. Available Online at: https://www.downtoearth.org.in/news/forests/plant-50-million-mangroves-in-the-sundarbans-improbable-say-experts-71977 (accessed June 24, 2021).
Bhargava, R., Sarkar, D., and Friess, D. A. (2020). A cloud computing-based approach to mapping mangrove erosion and progradation: case studies from the Sundarbans and French Guiana. Estuar. Coast. Shelf Sci. 248:106798. doi: 10.1016/j.ecss.2020.106798
Bhowmik, A., and Cabral, P. (2011). “Damage and Post-cyclone Regeneration Assessment of the Sundarbans Botanic Biodiversity caused by the Cyclone Sidr,” in The 1st World Sustainability Forum session Remote Sensing for Sustainable Management of Land and Biodiversity, (Basel, Switzerland: Sciforum). doi: 10.3390/wsf-00566
Branoff, B. L. (2020). Mangrove Disturbance and Response Following the 2017 Hurricane Season in Puerto Rico. Estuaries Coasts 43, 1248–1262. doi: 10.1007/s12237-019-00585-3
Bunting, P., Rosenqvist, A., Lucas, R. M., Rebelo, L.-M., Hilarides, L., Thomas, N., et al. (2018). The global mangrove watch—A new 2010 global baseline of mangrove extent. Remote Sens. 10:1669. doi: 10.3390/rs10101669
Castañeda-Moya, E., Twilley, R. R., Rivera-Monroy, V. H., Zhang, K., Davis, S. E., and Ross, M. (2010). Sediment and Nutrient Deposition Associated with Hurricane Wilma in Mangroves of the Florida Coastal Everglades. Estuaries Coasts 33, 45–58. doi: 10.1007/s12237-009-9242-0
Dutta, D., Das, P. K., Paul, S., Sharma, J. R., and Dadhwal, V. K. (2015). Assessment of ecological disturbance in the mangrove forest of Sundarbans caused by cyclones using MODIS time-series data (2001–2011). Nat. Hazards 79, 775–790. doi: 10.1007/s11069-015-1872-x
Ellison, J. C. (2016). Mangrove vulnerability assessment methodology and adaptation prioritisation. Malays. For. 79, 95–108.
Ferwerda, J. G., Ketner, P., and McGuinness, K. A. (2007). Differences in regeneration between hurricane damaged and clear-cut mangrove stands 25 years after clearing. Hydrobiologia 591:35. doi: 10.1007/s10750-007-0782-7
Friess, D. A., Krauss, K., Taillardat, P., Adame, M. F., Yando, E. S., Cameron, C., et al. (2020). Mangrove blue carbon in the face of deforestation, climate change, and restoration. Annu. Plant Rev. 3:427456. doi: 10.1002/9781119312994.apr0752
Goldberg, L., Lagomasino, D., Thomas, N., and Fatoyinbo, T. (2020). Global declines in human-driven mangrove loss. Glob. Chang. Biol. 26, 5844–5855. doi: 10.1111/gcb.15275
Halder, N. K., Merchant, A., Misbahuzzaman, K., Wagner, S., and Mukul, S. A. (2021). Why some trees are more vulnerable during catastrophic cyclone events in the Sundarbans mangrove forest of Bangladesh? For. Ecol. Manag. 490:119117. doi: 10.1016/j.foreco.2021.119117
Hoeser, T., Bachofer, F., and Kuenzer, C. (2020). Object detection and image segmentation with deep learning on earth observation data: a review—part II: applications. Remote Sens. 12:3053. doi: 10.3390/rs12183053
Hoeser, T., and Kuenzer, C. (2020). Object detection and image segmentation with deep learning on earth observation data: a review-part I: evolution and recent trends. Remote Sens. 12:1667. doi: 10.3390/rs12101667
Islam, M. M., Sunny, A. R., Hossain, M. M., and Friess, D. A. (2018). Drivers of mangrove ecosystem service change in the Sundarbans of Bangladesh: ecosystem services Bangladesh Sundarbans. Singap. J. Trop. Geogr. 39, 244–265. doi: 10.1111/sjtg.12241
Jones, M. C., Wingard, G. L., Stackhouse, B., Keller, K., Willard, D., Marot, M., et al. (2019). Rapid inundation of southern Florida coastline despite low relative sea-level rise rates during the late-Holocene. Nat. Commun. 10:3231. doi: 10.1038/s41467-019-11138-4
Knapp, K. R., Kruk, M. C., Levinson, D. H., Diamond, H. J., and Neumann, C. J. (2010). The International Best Track Archive for Climate Stewardship (IBTrACS): unifying Tropical Cyclone Data. Bull. Am. Meteorol. Soc. 91, 363–376. doi: 10.1175/2009BAMS2755.1
Krauss, K. W., and Osland, M. J. (2020). Tropical cyclones and the organization of mangrove forests: a review. Ann. Bot. 125, 213–234. doi: 10.1093/aob/mcz161
Lagomasino, D., Fatoyinbo, T., Castañeda-Moya, E., Cook, B. D., Montesano, P. M., Neigh, C. S. R., et al. (2021). Storm surge and ponding explain mangrove dieback in southwest Florida following Hurricane Irma. Nat. Commun. 12:4003. doi: 10.1038/s41467-021-24253-y
Lewis, R. R., Milbrandt, E. C., Brown, B., Krauss, K. W., Rovai, A. S., Beever, J. W., et al. (2016). Stress in mangrove forests: early detection and preemptive rehabilitation are essential for future successful worldwide mangrove forest management. Mar. Pollut. Bull. 109, 764–771. doi: 10.1016/j.marpolbul.2016.03.006
Long, J., Giri, C., Primavera, J., and Trivedi, M. (2016). Damage and recovery assessment of the Philippines’ mangroves following Super Typhoon Haiyan. Mar. Pollut. Bull. 109, 734–743. doi: 10.1016/j.marpolbul.2016.06.080
Macamo, C. C. F., Massuanganhe, E., Nicolau, D. K., Bandeira, S. O., and Adams, J. B. (2016). Mangrove’s response to cyclone Eline (2000): what is happening 14 years later. Aquat. Bot. 134, 10–17. doi: 10.1016/j.aquabot.2016.05.004
Mandal, M. S. H., and Hosaka, T. (2020). Assessing cyclone disturbances (1988–2016) in the Sundarbans mangrove forests using Landsat and Google Earth Engine. Nat. Hazards 102, 133–150. doi: 10.1007/s11069-020-03914-z
Maxwell, A. E., Warner, T. A., and Guillén, L. A. (2021). Accuracy assessment in convolutional neural network-based deep learning remote sensing studies—part 2: recommendations and best practices. Remote Sens. 13:2591. doi: 10.3390/rs13132591
Mentaschi, L., Vousdoukas, M. I., Pekel, J.-F., Voukouvalas, E., and Feyen, L. (2018). Global long-term observations of coastal erosion and accretion. Sci. Rep. 8:12876. doi: 10.1038/s41598-018-30904-w
Mishra, A. K., and Vanganuru, N. (2020). Monitoring a tropical super cyclone Amphan over Bay of Bengal and nearby region in May 2020. Remote Sens. Appl. Soc. Environ. 20:100408. doi: 10.1016/j.rsase.2020.100408
Ortolano, L., Sánchez-Triana, E., Paul, T., and Ferdausi, S. (2016). Managing the Sundarbans region: opportunities for mutual gain by India and Bangladesh. Int. J. Environ. Sustain. Dev. 15, 16–31. doi: 10.1504/IJESD.2016.073331
Paling, E. I., Kobryn, H. T., and Humphreys, G. (2008). Assessing the extent of mangrove change caused by Cyclone Vance in the eastern Exmouth Gulf, northwestern Australia. Estuar. Coast. Shelf Sci. 77, 603–613. doi: 10.1016/j.ecss.2007.10.019
Pekel, J.-F., Cottam, A., Gorelick, N., and Belward, A. S. (2016). High-resolution mapping of global surface water and its long-term changes. Nature 540, 418–422. doi: 10.1038/nature20584
Peneva-Reed, E. I., Krauss, K. W., Bullock, E. L., Zhu, Z., Woltz, V. L., Drexler, J. Z., et al. (2021). Carbon stock losses and recovery observed for a mangrove ecosystem following a major hurricane in Southwest Florida. Estuar. Coast. Shelf Sci. 248:106750. doi: 10.1016/j.ecss.2020.106750
Quader, M. A., Agrawal, S., and Kervyn, M. (2017). Multi-decadal land cover evolution in the Sundarban, the largest mangrove forest in the world. Ocean Coast. Manag. 139, 113–124. doi: 10.1016/j.ocecoaman.2017.02.008
Rodriguez-Galiano, V., Ghimire, B., Rogan, J., Chica-Olmo, M., and Rigol-Sanchez, J. (2012). An assessment of the effectiveness of a random forest classifier for land-cover classification. ISPRS J. Photogramm. Remote Sens. 67, 93–104. doi: 10.1016/j.isprsjprs.2011.11.002
Rovai, A. S., Twilley, R. R., Castañeda-Moya, E., Midway, S., Friess, D. A., Trettin, C., et al. (2021). Testing Macroecological Patterns and Drivers of Mangrove Carbon Stocks Across Biogeographic Regions and Coastal Morphologies.
Simard, M., Fatoyinbo, L., Smetanka, C., Rivera-Monroy, V. H., Castañeda-Moya, E., Thomas, N., et al. (2019). Mangrove canopy height globally related to precipitation, temperature and cyclone frequency. Nat. Geosci. 12, 40–45. doi: 10.1038/s41561-018-0279-1
Singh, O. P. (2007). Long-term trends in the frequency of severe cyclones of Bay of Bengal: observations and simulations. Mausam 58, 59–66.
Sippo, J. Z., Lovelock, C. E., Santos, I. R., Sanders, C. J., and Maher, D. T. (2018). Mangrove mortality in a changing climate: an overview. Estuar. Coast. Shelf Sci. 215, 241–249. doi: 10.1016/j.ecss.2018.10.011
Smith, T. J., Anderson, G. H., Balentine, K., Tiling, G., Ward, G. A., and Whelan, K. R. T. (2009). Cumulative impacts of hurricanes on Florida mangrove ecosystems: sediment deposition, storm surges and vegetation. Wetlands 29:24. doi: 10.1672/08-40.1
Strahler, A. N. (1952). Hypsometric (Area-Altitude) Analysis Of Erosional Topography. GSA Bull. 63, 1117–1142. doi: 10.1130/0016-7606(1952)63[1117:haaoet]2.0.co;2
Sud, V., and Rajaram, P. (2020). Cyclone Amphan caused an estimated $13.2 billion in damage: government source. Atlanta: CNN.
Taillie, P. J., Roman-Cuesta, R., Lagomasino, D., Cifuentes-Jara, M., Fatoyinbo, T., Ott, L. E., et al. (2020). Widespread mangrove damage resulting from the 2017 Atlantic mega hurricane season. Environ. Res. Lett. 15:064010. doi: 10.1088/1748-9326/ab82cf
The Indian Express (2019). Mangroves, low tide made Cyclone Bulbul less devastating. Noida: The Indian Express.
Thomas, N., Lucas, R., Bunting, P., Hardy, A., Rosenqvist, A., and Simard, M. (2017). Distribution and drivers of global mangrove forest change, 1996–2010. PLoS One 12:e0179302. doi: 10.1371/journal.pone.0179302
Vogt, J., Skóra, A., Feller, I. C., Piou, C., Coldren, G., and Berger, U. (2012). Investigating the role of impoundment and forest structure on the resistance and resilience of mangrove forests to hurricanes. Aquat. Bot. 97, 24–29. doi: 10.1016/j.aquabot.2011.10.006
Walker, L. R. (1991). Tree Damage and Recovery From Hurricane Hugo in Luquillo Experimental Forest, Puerto Rico. Biotropica 23, 379–385. doi: 10.2307/2388255
Ward, R. D., Friess, D. A., Day, R. H., and MacKenzie, R. A. (2016). Impacts of climate change on mangrove ecosystems: a region by region overview. Ecosyst. Health Sustain. 2:e01211. doi: 10.1002/ehs2.1211
Winterwerp, J. C., Albers, T., Anthony, E. J., Friess, D. A., Mancheño, A. G., Moseley, K., et al. (2020). Managing erosion of mangrove-mud coasts with permeable dams – lessons learned. Ecol. Eng. 158:106078. doi: 10.1016/j.ecoleng.2020.106078
Keywords: hurricane, Typhoon, India, Bangladesh, erosion, Bay of Bengal, remote sensing, Google Earth Engine (GEE)
Citation: Bhargava R and Friess DA (2022) Previous Shoreline Dynamics Determine Future Susceptibility to Cyclone Impact in the Sundarban Mangrove Forest. Front. Mar. Sci. 9:814577. doi: 10.3389/fmars.2022.814577
Received: 13 November 2021; Accepted: 21 February 2022;
Published: 11 March 2022.
Edited by:
Achilleas G. Samaras, Democritus University of Thrace, GreeceReviewed by:
Theocharis A. Plomaritis, University of Cádiz, SpainGiuseppe Barbaro, Mediterranea University of Reggio Calabria, Italy
Copyright © 2022 Bhargava and Friess. This is an open-access article distributed under the terms of the Creative Commons Attribution License (CC BY). The use, distribution or reproduction in other forums is permitted, provided the original author(s) and the copyright owner(s) are credited and that the original publication in this journal is cited, in accordance with accepted academic practice. No use, distribution or reproduction is permitted which does not comply with these terms.
*Correspondence: Radhika Bhargava, cmFkaGlrYV9iaGFyZ2F2YUB1Lm51cy5lZHU=