- Sea Mammal Research Unit, Scottish Oceans Institute, University of St Andrews, St Andrews, United Kingdom
Arrays of tidal turbines are being considered for tidally energetic coastal sites which can be important habitat for many species of marine mammal. Understanding risks to marine mammals from collisions with moving turbine blades must be overcome before regulators can issue licenses for many developments. To understand these risks, it is necessary to understand how animals move around operational turbines and to document the rate at which interactions occur. We report on the design, and performance, of a seabed mounted sensor platform for monitoring the fine scale movements of cetaceans and pinnipeds around operational tidal turbines. The system comprises two high-frequency multibeam active sonars, which can accurately track animals in the horizontal plane. By offsetting the vertical angle of the sonars, the relative intensity of targets on the two sonars can also be used to resolve a vertical component of the animal location. For regularly vocalizing species, i.e., small cetaceans, a tetrahedral array of high frequency hydrophones mounted close to the sonars is used to measure both horizontal and vertical angles to cetacean echolocation clicks. This provides additional localization and tracking information for cetaceans and can also be used to distinguish between pinnipeds and cetaceans detected in the sonar data, based on the presence or absence of echolocation clicks. The system is cabled to shore for power, data transfer, and communications using turbine infrastructure. This allows for continuous operation over many months or years, which will be required to capture what may be rare interactions. The system was tested during a series of multi-week field tests, designed to test system integrity, carry out system calibrations, and test the efficiency of data collection, analyses, and archiving procedures. Overall, the system proved highly reliable, with the PAM system providing bearing accuracies to synthetic sounds of around 4.2 degrees for echolocation clicks with a signal to noise ratio above 15 dB. The system will be deployed close to an operational turbine in early 2022.
Introduction
The global need for low-carbon energy is driving ambitious targets for wind, wave, and tidal energy in many countries. Tidal stream generators (hereafter termed “tidal turbines”) have the potential to contribute 11% of the UK’s current energy demand (Coles et al., 2021) and tidal energy has an important role to play in ensuring grid stability in a renewable energy future (Tróndheim et al., 2021). However, while wind energy is now making significant contributions to global energy supplies, tidal energy industries are currently at relatively early development stages (Ocean Energy Systems, 2020). This is in part due to the complexities of these emerging technologies, which by their nature are situated in particularly harsh marine environments. Further, concerns surrounding the potential environmental impacts that may arise from the introduction of these new technologies is a major consenting risk, forming a significant barrier to progress for some developments. Tidal turbines, which often resemble small wind turbines mounted on the sea floor, are of particular concern due to the possibility of collisions between animals and the moving rotors (Wilson et al., 2006; Copping, 2020). Critically, research has shown that collisions with moving rotors are likely to be fatal for marine mammals at rotor speeds > 5.1 ms–1 (Onoufriou et al., 2019). To inform assessments, estimates of collision risk have been derived by models of collision risk; critically, this requires direct empirical measurement of fine scale, close range, behavior of animals around turbines to validate predictions and directly estimate parameters such as avoidance rates.
At present, tidal turbine developments are proposed for areas of high tidal current which are generally close to shore. There is evidence that these sites can be important habitats for marine mammals such as harbor porpoises (Phocoena phocoena), delphinids and seals (Benjamins et al., 2017). Studying these highly mobile animals in highly energetic environments can be particularly challenging and technologies and data collection methods are limited. Many turbines are mounted on the seabed, where direct visual observation (e.g., from vessels or nearby land) of animal movements and interactions with tidal turbines is not possible. Underwater cameras have been used to some extent, but have limited fields of view, low detection ranges, and can only operate effectively in daylight. Potential alternative tools which have the capacity to detect and track marine mammals include active and passive acoustic systems (Williamson et al., 2015; Malinka et al., 2018; Hastie et al., 2019a; Gillespie et al., 2020; Polagye et al., 2020).
Small cetacean species are often highly vocal, using echolocation clicks to navigate and forage, and many species also produce tonal sounds (whistles) for communication. All of these can be readily detected using Passive Acoustic Monitoring (PAM) and, where arrays of hydrophones are used to detect the sounds, can be localized in three dimensions by measuring time of arrival differences on multiple receivers (Gillespie et al., 2020). Sounds can often be classified to species, and where that is not possible they can be more easily classified to species group, e.g., porpoise or dolphin. The detection, classification, and localization (DCL) processes can also be largely automated using a variety of signal processing algorithms (e.g., Gillespie et al., 2013).
Seal species in UK waters (gray seals and harbor seals) produce vocalizations infrequently or only in specific behavioral contexts such that PAM is not a reliable option for detecting and tracking seals underwater in high resolution. Onoufriou et al. (2021) showed that harbor seals (Phoca vitulina) tagged with GPS telemetry tags avoided a turbine array when it was operational, leading to reduced collision risk. Importantly however, data from the telemetry tags do not provide information on the underwater movements of seals at a sufficient resolution to measure their close-range interactions with the turbine. However, seals can be detected and localized using multibeam sonars, or Active Acoustic Monitoring (AAM) (Hastie et al., 2019a,b). Unlike PAM systems, which can detect in all directions from the receiver, sonars only allow data collection within the ensonification limits of the particular sonar system (e.g., a typical horizontally oriented multibeam sonar suitable for animal detection will detect over a 120° horizontal arc out to a range of around 60 m Hastie et al., 2019a,b). Further, individual sonars generally only provide location and tracking information in two dimensions; however, Hastie et al. (2019a) showed that rudimentary 3D information can be extracted using multiple sonars collecting data concurrently by using the relative amplitude of received signals on two horizontally mounted sonars with offset vertical angles.
For many tidal turbine monitoring applications, it will be important to differentiate marine mammal species. While differences in vocalizations detected using PAM can often be used to identify cetacean species, it is currently likely to be challenging to reliably differentiate similar sized marine mammals (e.g., gray and harbor seals, and harbor porpoises) in AAM data. However, by combining both AAM and PAM data it is possible to distinguish between seals and cetaceans based on the absence or presence of vocalizations at times when an animal is detected in AAM data, and then to distinguish between cetacean species based on more detailed analysis of the PAM data (Gillespie et al., 2020). The species information which can be derived from each type of detection is summarized in Figure 1.
Here we describe a monitoring system which combines both AAM and PAM detection into a single sensor platform to detect and track multiple species of marine mammals. We describe the sensors, how they are integrated into a stable platform and connected to shore. We also report on performance tests of the system components and a dockside test of the complete platform. The current design is optimized for monitoring an Atlantis AR1500 tidal turbine off the coast of Scotland (58°39′N 3°08′W). The turbine sits on a gravity mounted base, with a hub height of 14 m from the seabed and has three 9 m blades rotating at nominal speeds of 14 rpm (see Gillespie et al., 2021 for further information).
Materials and Methods
Design
The broad design of the monitoring system is a single seabed mounted platform, containing PAM and AAM sensors and their associated support electronics. Based on existing telemetry data (Onoufriou et al., 2021) and earlier studies using passive acoustics (Gillespie et al., 2021; Palmer et al., 2021) in the study area, close interactions are likely to be relatively rare events. To have a high chance of successfully monitoring these interactions, data collection must continue around the clock for many months. Our broad goal was therefore to develop a system which could operate continuously for at least a year and contained combinations of sensors capable of tracking both seals and small cetaceans. To overcome the limitations of power and data storage inherent in battery powered systems we have developed a cabled system which is both powered from shore and also streams data to shore, meaning that there are no fundamental limits to the system lifetime once it’s deployed. Data from the sensors can be viewed in real time on a computer at the turbine sub-station and adjustments made to sensors and real time detection algorithms.
Sensors
The active acoustic component consists of two Tritech Gemini 720 multibeam sonars (Hastie et al., 2019b). To maximize detection probability, cover the full height of the turbine blades (18 m), and to be able to track animals approaching the turbine on both the flood and ebb tides, these need to be mounted approximately 30 m to the side of the turbine which, with a 120° horizontal beam, allows tracking of animals 26 m upstream and downstream of the turbine center. The -3 dB vertical beam height is approximately 20° and the vertical angle offset between the two sonars is 15° giving adequate coverage of the turbine rotors, although it would be possible for animals to pass by undetected close to the surface above the turbine. For optimal performance, the two sonar heads must be accurately aligned to minimize reflections from the surface and the sea floor, and to ensure that animal detections can be accurately geo-located with respect to the turbine. They are therefore mounted on a custom-designed pitch-roll mechanism which can be controlled from the sub-station computer once the platform is deployed. The pitch-roll mechanism (see Figure 2) is based on two LA35 linear actuators made by Servo Components and Systems.1 The vertical alignment (pitch and roll) of the sonars is determined using a Hillcrest Labs FSM300 Inertial Measurement Sensor (IMU). Ultra violet (UV-C) LED’s are mounted close to the sonar heads in order to reduce biofouling (Patil et al., 2007).
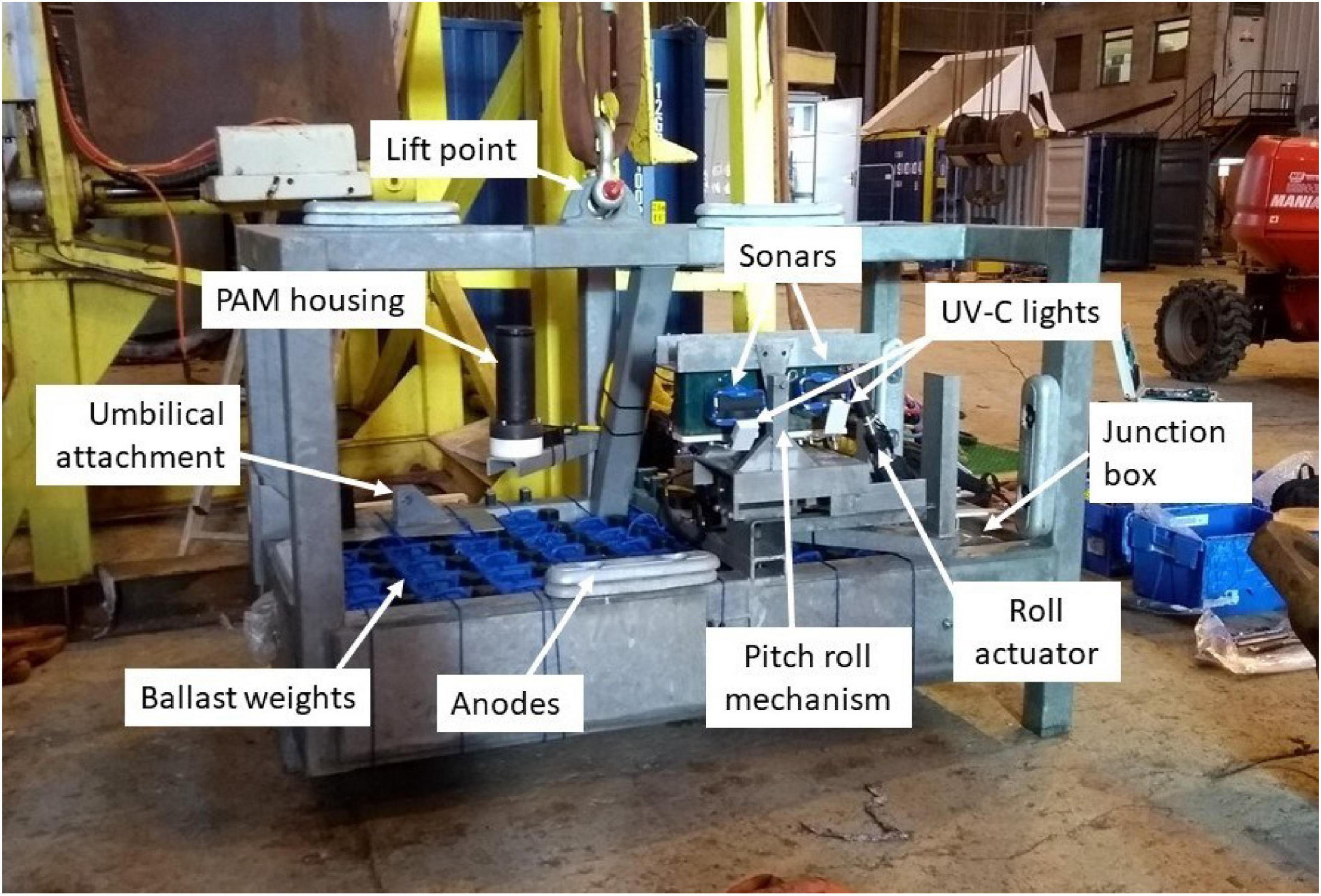
Figure 2. Photograph of the platform ready for deployment showing the principal components of the system.
A single tetrahedral cluster of hydrophones is mounted close to the sonars, which can detect cetacean vocalizations and measure horizontal and vertical angles to the sounds but provides no range information. Importantly, with hydrophones mounted close together, 30 m from the turbine, it would be unrealistic to implement a 3D PAM tracking array of the type previously installed on the turbine foundation (see Gillespie et al., 2020). However, by combining the angular data from the hydrophones with the AAM data it is possible to track cetaceans in three dimensions within the area covered by the sonar obtaining vertical angle from the hydrophones, horizontal angle from both the hydrophones and from the sonars, and range information from the sonars (Table 1). The hydrophone cluster design is broadly similar to that of a single hydrophone cluster described in Gillespie et al. (2020); however for this sensor platform, Reson TC4015 hydrophone elements were used which have a flat frequency response up to 190 kHz, and a smaller (5 cm) inter-hydrophone spacing. Modified Etec preamplifiers were used which allow for remote control of amplifier gain and high pass filter settings. Data acquisition is also the same as that described in Gillespie et al. (2020), with raw audio data being digitized using a National Instruments cRIO-9063 Controller chassis equipped with a single NI-9222 4 channel ADC. The cRIO Controller was programmed to acquire data on the four channels at 500,000 samples/s per channel and transfer the data directly into the PAMGuard software (Gillespie et al., 2008) for real time processing, display, and storage. Unlike the sonars, the absolute orientation of the hydrophone cluster is unimportant so long as it is known. This can be determined using a second IMU mounted within the junction box (see below). As with the sonars, a UV-C LED is mounted close to the hydrophones to reduce biofouling.
Peripherals and Integration
All peripheral electronics are housed in a stainless steel pressure vessel which is connected to the sonars and hydrophones using under water dry-mate connectors (Hydrobond, HMD series), as well as the controls for the positioning actuators, orientation sensors, and UV lights. All components are connected via Ethernet, ensuring the integration, control, and data transfer from multiple instruments used standard networking components. The actuators for the pitch-roll mechanism, the PAM preamplifier gain and filter settings, and the data from the IMU sensors are all controlled from an Arduino Mega 2560 micro controller with a KeyStudio Ethernet adapter. This receives commands for each component over Ethernet from a bespoke module written for PAMGuard. This allows data on the system status, pitch-roll reading, preamplifier settings, etc. to be logged in the PAMGuard database together with other collected data.
All components are powered from a low voltage 24 V power supply, with additional DC-DC converters providing 12 and 5 V power to the UV-C LED’s and the Arduino, respectively. The total power consumption is approximately 60 W (Table 2). For some deployment scenarios, it would be possible to run the system directly from a DC power supply; however most tidal turbine deployment scenarios will require cable lengths of several 100’s–1,000’s of meters, such that the system is currently powered using 240 V AC voltage converted internally to 24 V using a Phoenix Quint4-PS 24 V power supply.
Standard copper Ethernet cable can only work reliably over cable lengths less than 100 m. Initial dock tests, requiring cable lengths of 400 m, were therefore conducted using Perle eX-1S110 Ethernet extenders, which can transfer data using Ethernet protocols over greater distances using a single copper twisted pair. Deployment of the system alongside a tidal turbine will use optical fiber. The compatibility of these different Ethernet based components, and the availability of converters between different types of fiber and cable, makes changing between data transfer systems extremely straightforward, with the only requirement being a change in the junction box face plate connector to one that supports optical fibers.
Construction
The platform design was largely governed by the need for the system to be stable in high currents, which can reach speeds of up to 5 m/s at the intended deployment location in Scotland. The platform consists of a relatively simple 210 × 110 × 140 cm galvanized mild steel frame welded from 100 mm box section. The base contains a “ballast bay” designed to fit 48 25 kg “handy weights,” (Metalast Ltd., 1,200 kg total). All electronic components are mounted within a 30.5 cm diameter, 57 cm long stainless steel junction box, which also sits within the ballast bay, providing protection to the dry mate connectors on the end plate of the junction box. Zinc anodes (50 kg) are bolted to the frame to protect against corrosion. A photograph of the frame is shown in Figure 2 and a technical drawing of the assembly is available in Supplementary Material A.
Software, Data Displays, and Offline Processing
The high resolution sensors generate considerable amounts of data. The two sonars operate at a combined frame rate of ∼12 fps. Each frame contains 512 beams and typically 770 range measurements sampled with 8 bit resolution, generating 4.7 Mbytes of raw data per second (400 Gbytes per day). Although the proprietary sonar software (Tritech SeaTec) does use some data compression, this only has a modest effect on data volumes which are typically around 320Gbyte per day. Raw data from the PAM system (4 channels of 16 bit data sampled at 500 kHz) are 4Mbyte/second or 321Gbytes per day.
The sensor system is controlled from a single PC housed on shore (HP Z2 workstation with an Intel® Core i7-1,900 CPU @ 3 GHz, 16Gbyte RAM running Windows 10). The sonars are controlled and data recorded using the proprietary SeaTec software, and the PAM system uses the open source PAMGuard software (Gillespie et al., 2008).2 Both SeaTec and PAMGuard have dedicated watchdog software which will restart them in the event of a software malfunction or after a computer restart (for example, Windows updates or power outages).
The SeaTec software includes the target/track detection algorithm described in Hastie et al. (2019b), but not the target classification algorithm which is currently run offline in the R language (R Core Team, 2018). Previous versions of SeaTec wrote track and target information to text files, but the software was modified so that these data are broadcast as UDP datagram packets. A new bespoke module for PAMGuard receives and decodes these packets, writes the data within them to the PAMGuard database and allows the detected targets to be displayed on a PAMGuard map alongside bearings from the PAM system to detected vocalizations.
When viewing data post hoc, it is also possible to display the image data from both sonars as an additional overlay on the PAMGuard map, with both the sonar targets and the PAM detections. Standard PAMGuard functionality for annotating acoustic data, as has been used in previous studies (Palmer et al., 2021) can then be applied to the AAM data.
Position and Time
Accurate time alignment of the AAM and PAM data streams is important both for combining the two data sets for accurate 3D localization and also for direct comparison with turbine blade positions. With current speeds of up to 5 m/s, our aim is to be able to time align our data to within about 0.1 s, corresponding to a passive movement of around 0.5 m at the highest flow rates. Within the PAM system, time is taken from the PC’s internal clock whenever the system starts or resets, and then calculated on the number of samples acquired by the acquisition system from that moment. The PC clock automatically sets from the Windows time server, so is likely to be accurate to within a few seconds. The clock drift on the NI acquisition system is approximately 2–3 ppm, though can vary with temperature. This equates to around 0.25 s per day, so after several weeks of uninterrupted operation, it is possible that times will have errors of several seconds. Similarly, times within the sonar data are based on the PC time and records of ping numbers, which again, are subject to clock drift.
To align the times of the AAM and PAM data, the Gemini SeaTec software was modified to output status data (SeaTec file name and frame number) to PAMGuard, which is logged every 60 s. In addition, all targets detected by SeaTec are sent to PAMGuard, time stamped, and stored in the PAMGuard database. This allows accurate time alignment of the PAM and AAM data. PAMGuard also regularly (every minute) takes an accurate time from a time server and writes all data to a database. This makes it possible to align the SeaTec data to the PAM data and to align all data to the server (absolute) time reference.
Measurements of the speed of sound at the sonars is also available from the sonars, which are logged and can be used in the PAM data angle measurements.
Performance Testing
Two sets of performance tests were conducted during the design and construction of the platform. PAM and AAM system components were initially tested from a small (7 m) research vessel on the west coast of Scotland in August 2018 (57°15′N,05°36′W). Porpoises were located visually at the surface and the sonar and PAM system were deployed on a rigid mount over the side of the vessel, which then drifted in the vicinity of the animals, with occasional small maneuvers to attempt to keep the animals in the sonar swath. This work was approved by the University of St Andrews School of Biology Ethics Committee (Ref number: SEC18017).
The second trial was of the fully constructed platform and sensor system, and was designed to test overall system integrity, integrated detection capabilities, sensor interference, and data collection and storage capabilities, and took place at the end of a disused ferry pier in the river Tay (56°26.3′N,02°56.6′W) for 12 days in November 2020. This second trial took place during the COVID Pandemic at a time when it was not possible to travel to a location where animals were likely to be encountered. Instead a 80 × 32 cm metal cylinder as a sonar target and an electronic pinger playing synthetic porpoise like clicks, were deployed from a small vessel that maneuvered slowly in the area in front of the platform. The vessel position was recorded using a GlobalSat BU-353-S4 WAAS enabled Differential GPS with an error of less than 3 m 95% of the time. GPS data were compared with both the AAM and PAM data; however, it became apparent that the sonar signal was dominated by the vessel wake, meaning that only the PAM data were useful. PAM derived bearings were compared with the expected bearing to the vessel position; however, at ranges of a few 10’s of meters, the 3 m GPS uncertainty can lead to uncertainties in expected bearings of several degrees, which is greater than the anticipated accuracy of the bearing measurements. Therefore, each click bearing was also compared to the bearing to the preceding click, which if we can assume that the errors on each bearing are independent, and that the vessel has not moved significantly between clicks (which were 50 ms apart in time), then the difference in adjacent bearings should be:
Where △θ is the difference in bearing between adjacent clicks and δθ is the uncertainty on the bearing of each individual clicks.
Results
Both trials successfully collected data, and derived tracks of animals and targets using both the AAM and PAM systems. Importantly, during the 12 day test of the full system in 2020, all components worked throughout that period, and data were acquired continuously without interruption, demonstrating that the system is suitable for long term data collection once deployed at a turbine site. Some interference from the sonars could be detected on the PAM system, though this did not affect our ability to detect porpoise clicks.
Harbor porpoises were encountered on 1 day during the 2018 trials and only swam through the region covered by the sonar on a single occasion. Figure 3 shows bearings to two harbor porpoises tracked by the system over a 2-min period. For the first minute, the animals can be clearly seen on both the AAM and PAM systems. After 10:35, detections are only visible on the PAM system. This is largely due to the animals moving beyond the horizontal swath limits of the sonar (60°) during this period, though there was also considerable “clutter” on one of the sonars from waves at the surface, making detection on this sonar difficult. The track of the porpoise was detected automatically in the sonar data and an operator was able to manually pick out additional components of the track, which were not detected automatically. A video file containing the AAM data with an overlay of the PAM bearings is available in Supplementary Material B.
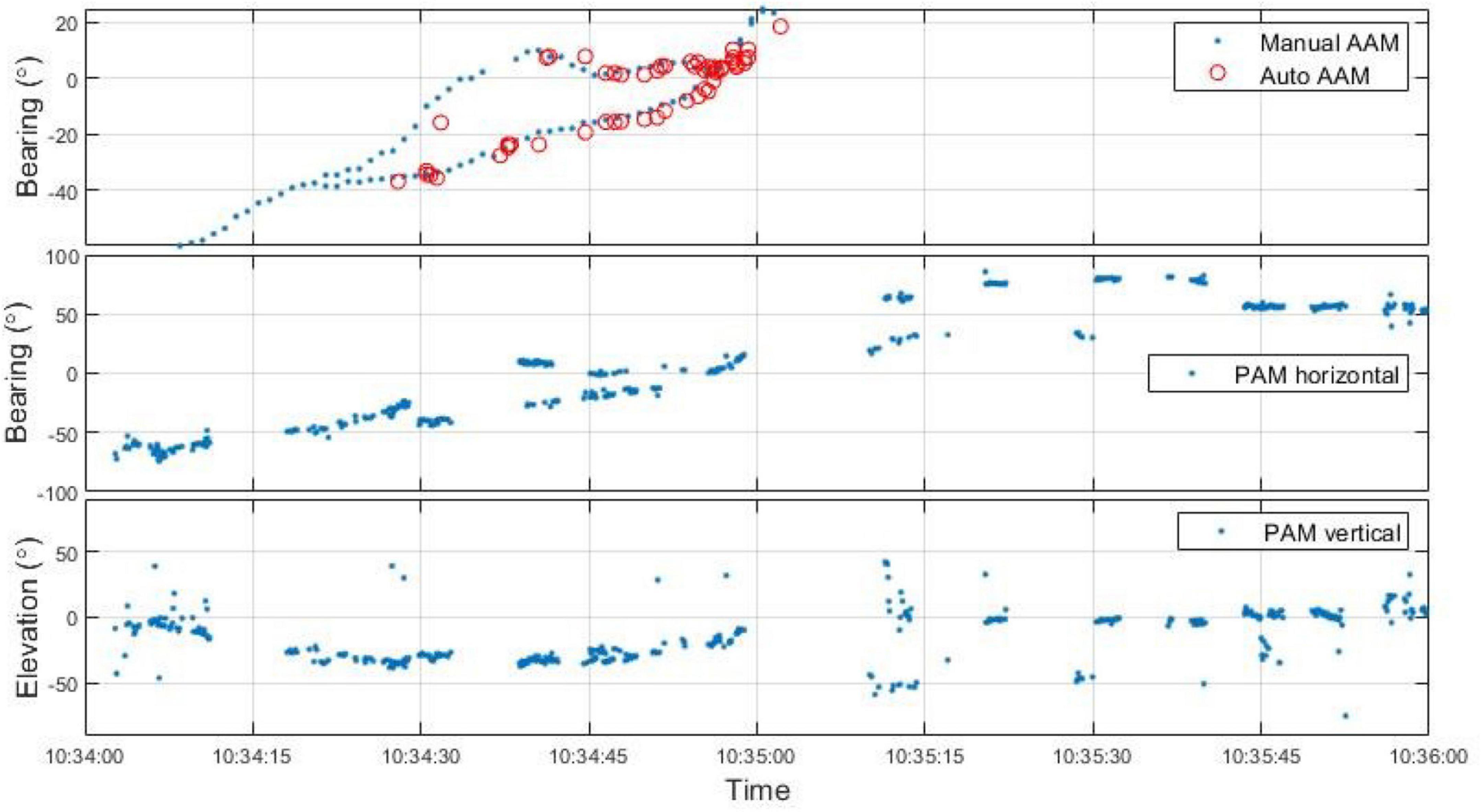
Figure 3. Horizontal and vertical angles to AAM and PAM detections of two porpoise swimming close to the detection system over a 2-min period.
Figure 4 shows the offset angle between the PAM horizontal angle and the expected angle from the vessels GPS location as a function of range and Figure 5 shows errors as a function of signal to noise ratio (SNR) based on angle differences between adjacent clicks. Absolute errors are larger than the relative differences between adjacent clicks. This is most likely due to uncertainties in the GPS position of the vessel and possibly in the position of the platform itself. These contribute to larger errors at short distances where small differences in position will create large differences in angle. As a function of SNR, there is a marked improvement in bearing accuracy as SNR increases. This result is broadly in line with the improved timing accuracy with SNR reported in Gillespie and Macaulay (2019) where it was shown that below an SNR of around 12 dB it becomes increasingly difficult to measure accurate Time Difference Of Arrival (TDOAs) between porpoise like signals. However, bearing errors are slightly higher than those estimated from simulations in Gillespie and Macaulay (2019). This would appear to be due to increased signal distortion in real data, in part caused by echoes in the waveforms appearing about 1 ms after the initial pules, indicating reflection off nearby (< 1 m distant) objects.
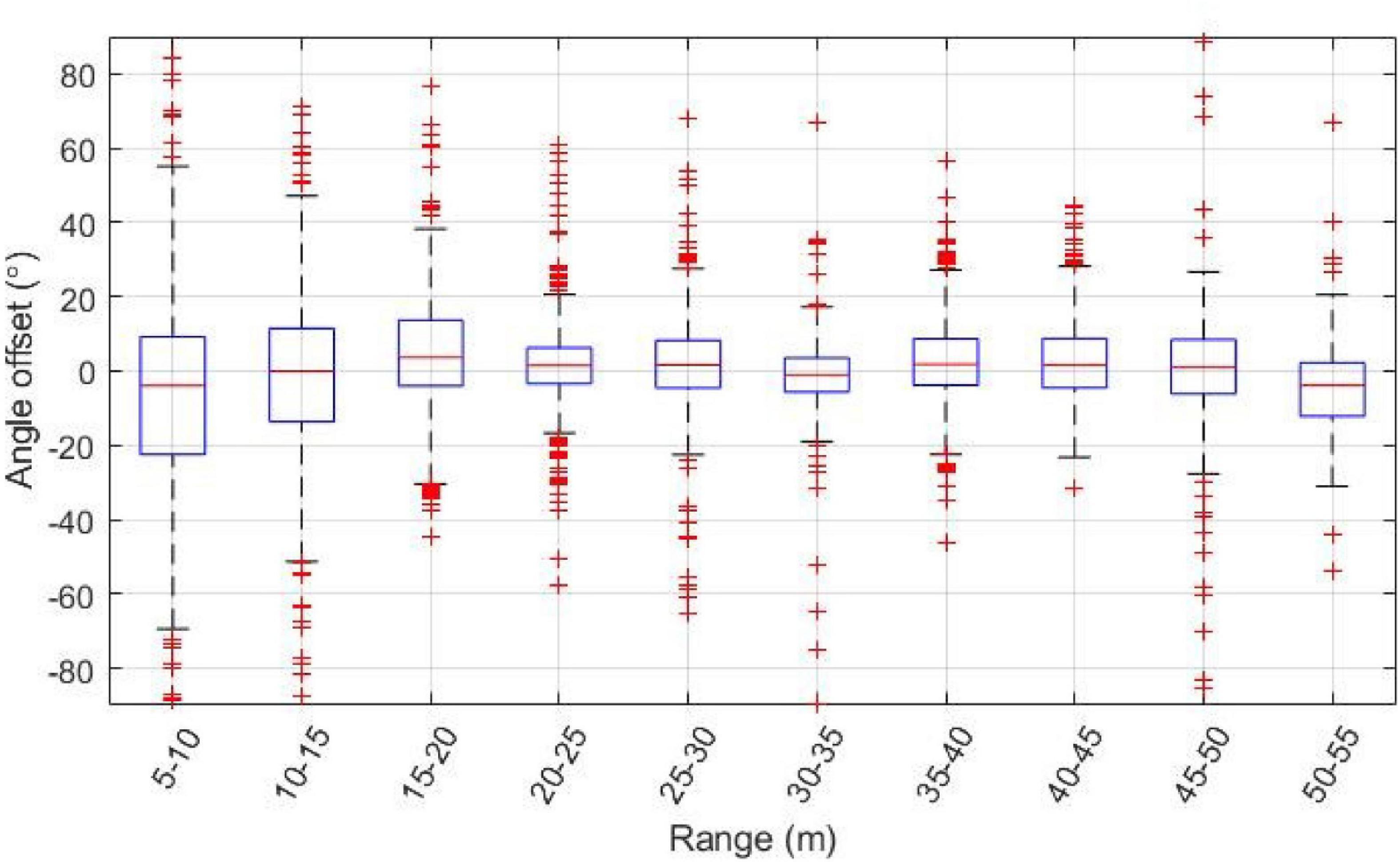
Figure 4. Horizontal angle offsets for bearings to generated porpoise clicks based on GPS position as a function of range. On each box, the central mark indicates the median, and the bottom and top edges of the box indicate the 25th and 75th percentiles, respectively. The whiskers extend to the most extreme data points not considered outliers, and the outliers are plotted individually using the “+” symbol.
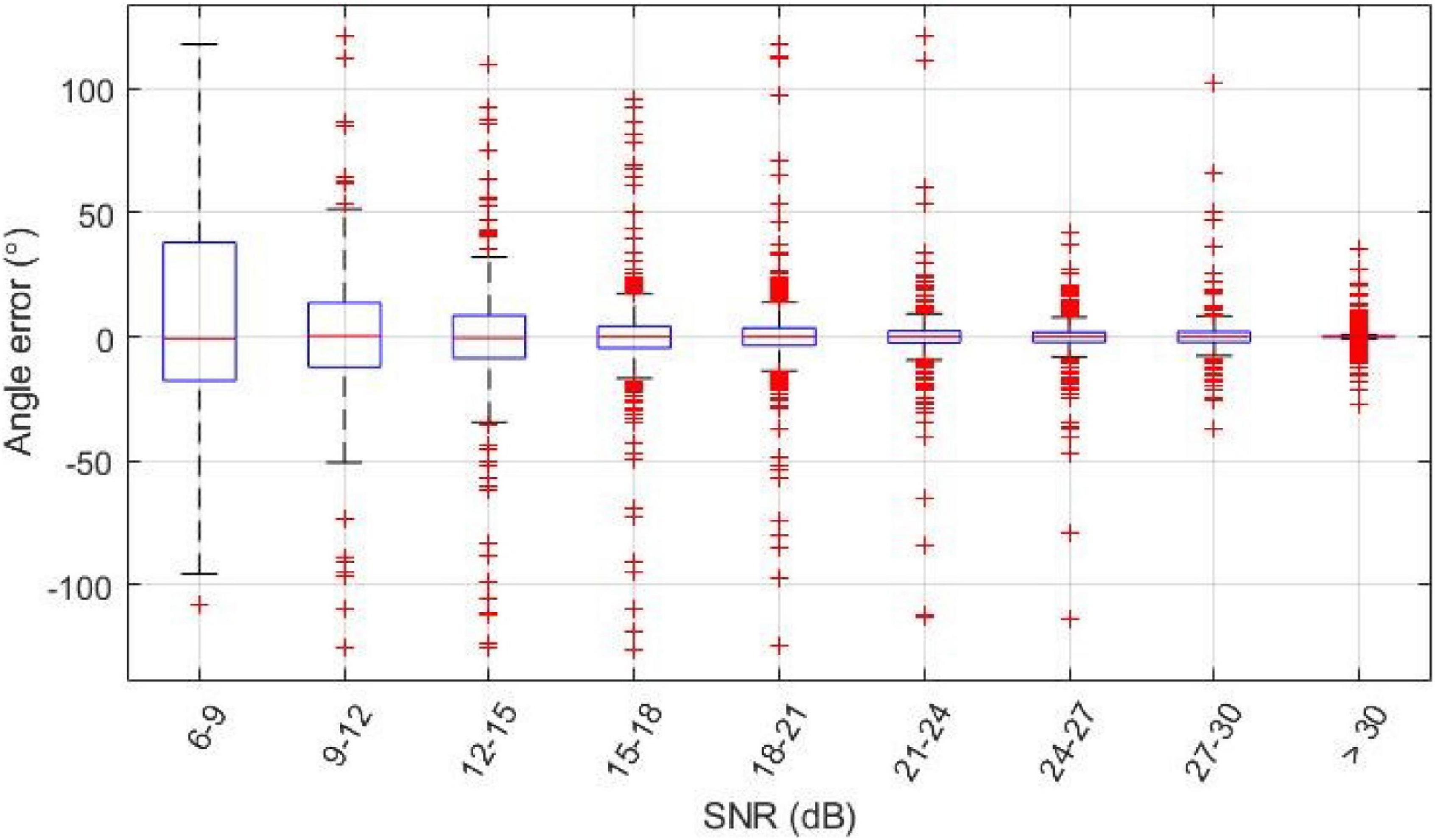
Figure 5. Horizontal angle errors based on the angle difference between adjacent clicks, where the error is the difference in angle to adjacent clicks divided by .
Discussion
We have developed a sensor platform capable of detecting and tracking both seals and small cetaceans using a combination of active multibeam sonar and PAM. The individual components of the system have been well tested in previous tracking studies of seals (using active sonar) and small cetaceans (using PAM), and these have now been integrated into a single platform suitable for long-term deployment close to a tidal turbine. Raw data volumes from the platform sensors are ∼640 Gbytes per day and the total power requirement is 61 W.
Previously, a number of other platform-based monitoring systems have been developed for monitoring wildlife interactions with tidal turbines. For example, Williamson et al. (2017) developed an autonomous (battery powered) platform (FLOWBEC) that combined several sonar systems [upward-facing multifrequency Simrad EK60 echosounder and Imagenex Delta T multibeam sonar, and an acoustic Doppler velocimeter (ADV)] to record information on a range of physical and multitrophic levels for durations of 2 weeks. This has provided information on the behavior and movements of diving seabirds, fish, and fish schools around a tidal turbine structure (Williamson et al., 2017). The clear benefits of a system like FLOWBEC are that the data collection and storage are fully autonomous, and deployment and retrieval logistics are independent of the tidal turbine. Importantly however, encounters between tidal turbines and marine mammals are expected to be infrequent, for instance Gillespie et al. (2021) report a single porpoise passing through the area swept by turbine rotors in 451 days of monitoring, so long time series of data (years) would likely be required to meaningfully analyze risk for these species; this likely makes short duration deployment systems financially prohibitive due to the requirement for repeated deployments and recoveries. As a solution to this, Cotter et al. (2016) developed a monitoring system that combined a multibeam sonar, acoustic camera, current profiler, optical cameras, and hydrophones, that was connected to a PC onshore via a subsea cable (∼150 m). This successfully collected data on diving birds, fish, and seals over a period of 76 days. In the current study, we further developed this cabled approach and designed the system for a cabled connection to shore via the turbine infrastructure. While the integration into the turbine systems can be costly and logistically complex, this approach means that the logistics and financial costs associated with providing a dedicated subsea cable between the monitoring system and shore are effectively removed.
From a monitoring perspective, the PAM system is sensitive to sound arriving from any direction, while the sonars have a limited field of view (120° horizontally and ∼20° vertically for each device) and a detection range of ∼50 m. This provides adequate coverage of a single fixed turbine. However, monitoring other turbine designs, such as tidal kites or moored turbines, which do not have a fixed location, may require a different solution. The current sensor system on the platform has also been configured for the most abundant marine mammal species at a site off the north coast of Scotland and those that are of conservation concern to regulatory authorities (harbor seals). Other regions proposed for tidal development are likely to contain other species of interest, such that the optimal configuration of the platform or sensors may be different to the one in the current study. For example, at sites where small cetaceans are the primary species of interest, it may be more effective to reconfigure the platform to omit the active sonars and increase the numbers of hydrophones. However, for effective 3D tracking with hydrophones in isolation, the additional hydrophones would need to be positioned on platforms closer to, or ideally all around, the tidal turbine which may introduce significant logistical challenges for deployment.
The platform here has been designed to monitor behavior within a few 10’s of meters around a single turbine. Although this will provide data on the fine scale movements of animals in close proximity to the turbine, it will not provide information on larger scale avoidance (from 100’s m to km) of the turbine as has been observed for seals using GPS tags (Onoufriou et al., 2021). Such information is important for understanding potential chronic impacts of tidal turbine developments (Scottish Natural Heritage, 2016). Satellite tag attachment to small cetaceans has been conducted on a small scale (e.g., Sveegaard et al., 2011) but is logistically challenging and relatively invasive. More importantly, there would be no guarantee that tagged animals would visit the site of interest, so tagging is unlikely to be employed close to tidal turbine sites. The broader scale distribution of cetaceans about a tidal array could most easily be measured using wider spaced arrays of hydrophones (e.g., Tollit et al., 2019) which would not necessarily require the localization abilities of the platform described in this paper and archival autonomous recorders may provide the most cost effective solution.
While our current studies are designed only to understand fine scale interactions between marine mammals and tidal energy devices, there is increasing interest from some regulators in the implementation of real time mitigation measures which could be triggered when animals were detected approaching a turbine, e.g., the production of an aversive sound signal (Acoustic Deterrent Device) to deter the animal from the immediate vicinity of the turbine. Although the broad design of the platform here lends itself to real time monitoring and mitigation, the logistical challenges of implementing such mitigation procedures at other sites may be considerable. In practice, for such real-time monitoring and mitigation, the effective integration of the sonar and hydrophone data, processing PC, and mitigation systems would be required, together with a series of software developments such that the detection and classification software could be run in real time with a high degree of accuracy and the results used to trigger the mitigation measure when an animal was detected.
In summary, this study describes the development and testing of a seabed mounted sensor platform to monitor marine mammal movements in close vicinity to operational tidal turbines. It incorporates a combination of PAM and AAM sensors to detect, classify, and track seals and small cetaceans in high spatial and temporal resolution. Field tests showed that the system is effective at producing tracks of animals using the sensor combination, and that the full system can operate and collect data continuously for periods of several weeks without interruption, demonstrating that the system is suitable for long term data collection once deployed at a turbine site. This provides an effective monitoring system for the tidal industry and regulators to measure interactions between operational tidal turbines and marine mammals, provide data to parameterize collision risk models, and inform the environmental risks associated with the developing tidal industry. At present the system is due for deployment alongside an operational tidal turbine at the Meygen site in Scotland in early 2022.
Data Availability Statement
The raw data supporting the conclusions of this article will be made available by the authors, without undue reservation.
Ethics Statement
The animal study was reviewed and approved by the University of St Andrews Animal Welfare and Ethics Review Body (Ref number: SEC18017).
Author Contributions
MO contributed to engineering design. MO and DG contributed to construction. All authors contributed to the system conception and it’s overall design, participated in field tests, and contributed to this manuscript.
Funding
Funding was provided by the Natural Environment Research Council (Grant Nos. NE/R015007/1 and NE/R014639/1) with additional resources from the Scottish Government as part of the Marine Mammal Scientific Support Program (Grant No. MMSS/002/15).
Conflict of Interest
The authors declare that the research was conducted in the absence of any commercial or financial relationships that could be construed as a potential conflict of interest.
Publisher’s Note
All claims expressed in this article are solely those of the authors and do not necessarily represent those of their affiliated organizations, or those of the publisher, the editors and the reviewers. Any product that may be evaluated in this article, or claim that may be made by its manufacturer, is not guaranteed or endorsed by the publisher.
Acknowledgments
We are grateful to members of the project steering group for advice and guidance on the development of the platform. Further, the project was only possible with the input and advice from Fraser Johnson at Simec Atlantis who advised on design for installation in the high current environment and oversaw the integration into the turbine cabling system.
Supplementary Material
The Supplementary Material for this article can be found online at: https://www.frontiersin.org/articles/10.3389/fmars.2022.850446/full#supplementary-material
Footnotes
References
Benjamins, S., van Geel, N., Hastie, G., Elliott, J., and Wilson, B. (2017). Harbour porpoise distribution can vary at small spatiotemporal scales in energetic habitats. Deep Sea Res. Part II. 141, 191–202.
Coles, D., Angeloudis, A., Greaves, D., Hastie, G., Lewis, M., Mackie, L., et al. (2021). A review of the UK and British Channel Islands practical tidal stream energy resource. Proc. R. Soc. A 477:20210469. doi: 10.1098/rspa.2021.0469
Copping, A. E. (2020). “Marine Renewable Energy and Ocean Energy System Pacific Northwest National Lab.(PNNL),” in 2020 State of the Science Report, (Richland:PNNL).
Cotter, E., Matzner, S., Horne, J., Murphy, P., and Polagye, B. (2016). “Benchmarking sensor fusion capabilities of an integrated monitoring package,” in 4th Marine Energy Technology Symposium (METS).(New York: METS)
Gillespie, D., Caillat, M., Gordon, J., and White, P. (2013). Automatic detection and classification of odontocete whistles. J. Acoust. Soc. Am. 134, 2427–2437. doi: 10.1121/1.4816555
Gillespie, D., Gordon, J., Mchugh, R., Mclaren, D., Mellinger, D., Redmond, P., et al. (2008). PAMGUARD: Semiautomated, open source software for real-time acoustic detection and localisation of cetaceans. J. Acoust. Soc. Am. 125:2547. doi: 10.1121/1.4808713
Gillespie, D., and Macaulay, J. (2019). Time of arrival difference estimation for narrow band high frequency echolocation clicks. J. Acoust. Soc. Am. 146:EL387. doi: 10.1121/1.5129678
Gillespie, D., Palmer, L., Macaulay, J., Sparling, C., and Hastie, G. (2020). Passive acoustic methods for tracking the 3D movements of small cetaceans around marine structures. PLoS One 15:e0229058. doi: 10.1371/journal.pone.0229058
Gillespie, D., Palmer, L., Macaulay, J., Sparling, C., and Hastie, G. (2021). Harbour porpoises exhibit localized evasion of a tidal turbine. Aquat. Conserv. 31, 2459-2468. doi: 10.1002/aqc.3660
Hastie, G. D., Bivins, M., Coram, A., Gordon, J., Jepp, P., MacAulay, J., et al. (2019a). Three-dimensional movements of harbour seals in a tidally energetic channel: Application of a novel sonar tracking system. Aquat. Conserv. 26, 564-575
Hastie, G. D., Wu, G.-M., Moss, S., Jepp, P., MacAulay, J., Lee, A., et al. (2019b). Automated detection and tracking of marine mammals: A novel sonar tool for monitoring effects of marine industry. Aquat. Conserv. 29, 119–130. doi: 10.1002/aqc.3103
Malinka, C., Gillespie, D., Macaulay, J., Joy, R., and Sparling, C. (2018). First in-situ passive acoustic monitoring for marine mammals during operation of a tidal turbine in Ramsey Sound, Wales. Mar. Ecol. Prog. Ser. 590, 247–266. doi: 10.3354/meps12467
Onoufriou, J., Brownlow, A., Moss, S., Hastie, G., and Thompson, D. (2019). Empirical determination of severe trauma in seals from collisions with tidal turbine blades. J. Appl. Ecol. 56, 1712–1724. doi: 10.1111/1365-2664.13388
Onoufriou, J., Russell, D. J., Thompson, D., Moss, S. E., and Hastie, G. D. (2021). Quantifying the effects of tidal turbine array operations on the distribution of marine mammals: Implications for collision risk. Renew. Energy 180, 157–165. doi: 10.1016/j.renene.2021.08.052
Palmer, L., Gillespie, D., MacAulay, J. D., Sparling, C. E., Russell, D. J., and Hastie, G. D. (2021). Harbour porpoise (Phocoena phocoena) presence is reduced during tidal turbine operation. Aquat. Conserv. 31, 3543-3553
Patil, J. S., Kimoto, H., Kimoto, T., and Saino, T. (2007). Ultraviolet radiation (UV-C): a potential tool for the control of biofouling on marine optical instruments. Biofouling 23, 215–230. doi: 10.1080/08927010701275598
Polagye, B., Joslin, J., Murphy, P., Cotter, E., Scott, M., Gibbs, P., et al. (2020). Adaptable monitoring package development and deployment: Lessons learned for integrated instrumentation at marine energy sites. J. Mar. Sci. Eng. 8:553. doi: 10.3390/jmse8080553
R Core Team. (2018). R: A Language and Environment for Statistical Computing. (Vienna, AUS: R Foundation for Statistical Computing)
Scottish Natural Heritage (2016). Assessing collision risk between underwater turbines and marine wildlife.(Scotland: Scottish Natural Heritage)
Sveegaard, S., Teilmann, J., Tougaard, J., Dietz, R., Mouritsen, K. N., Desportes, G., et al. (2011). High-density areas for harbor porpoises (Phocoena phocoena) identified by satellite tracking. Mar. Mammal Sci. 27, 230–246.
Ocean Energy Systems (2020). Annual Report: An Overview of Ocean Energy Activities in 2020. Available online at: https://www.ocean-energy-systems.org/publications/oes-annual-reports/, p. 188 (accessed January, 2020).
Tollit, D., Joy, R., Wood, J., Redden, A. M., Booth, C., Boucher, T., et al. (2019). Baseline presence of and effects of tidal turbine installation and operations on harbour porpoise in Minas Passage, Bay of Fundy, Canada. J. Ocean Technol.14:24
Tróndheim, H. M., Niclasen, B. A., Nielsen, T., Da Silva, F. F., and Bak, C. L. (2021). 100% Sustainable Electricity in the Faroe Islands: Expansion Planning Through Economic Optimization. IEEE Open Access J. Power Energy 8, 23–34.
Williamson, B. J., Blondel, P., Armstrong, E., Bell, P. S., Hall, C., Waggitt, J. J., et al. (2015). A self-contained subsea platform for acoustic monitoring of the environment around Marine Renewable Energy Devices–Field deployments at wave and tidal energy sites in Orkney, Scotland. IEEE J. Oceanic Eng. 41, 67–81. doi: 10.1109/joe.2015.2410851
Williamson, B. J., Fraser, S., Blondel, P., Bell, P. S., Waggitt, J. J., and Scott, B. E. (2017). Multisensor acoustic tracking of fish and seabird behavior around tidal turbine structures in Scotland. IEEE J. Oceanic Eng. 42, 948–965.
Keywords: marine mammal, seal (Pinnipedia), porpoise, tidal energy, passive acoustic monitoring, active acoustic monitoring
Citation: Gillespie D, Oswald M, Hastie G and Sparling C (2022) Marine Mammal HiCUP: A High Current Underwater Platform for the Long-Term Monitoring of Fine-Scale Marine Mammal Behavior Around Tidal Turbines. Front. Mar. Sci. 9:850446. doi: 10.3389/fmars.2022.850446
Received: 07 January 2022; Accepted: 11 February 2022;
Published: 02 March 2022.
Edited by:
Huidong Li, Pacific Northwest National Laboratory (DOE), United StatesReviewed by:
Robert McCauley, Curtin University, AustraliaChi-Fang Chen, National Taiwan University, Taiwan
Copyright © 2022 Gillespie, Oswald, Hastie and Sparling. This is an open-access article distributed under the terms of the Creative Commons Attribution License (CC BY). The use, distribution or reproduction in other forums is permitted, provided the original author(s) and the copyright owner(s) are credited and that the original publication in this journal is cited, in accordance with accepted academic practice. No use, distribution or reproduction is permitted which does not comply with these terms.
*Correspondence: Douglas Gillespie, ZGc1MEBzdC1hbmRyZXdzLmFjLnVr