- 1Department of Earth Sciences, University of Oxford, Oxford, United Kingdom
- 2The Scottish Association for Marine Science, Oban, United Kingdom
- 3School of GeoSciences, University of Edinburgh, Edinburgh, United Kingdom
Phytoplankton community composition, and its dependency on environmental variation, are key to understanding marine primary production, processes of trophic transfer and the role of marine phytoplankton in global biogeochemical cycles. Understanding changes in phytoplankton community composition on Arctic shelves is important, because these productive environments are experiencing rapid change. Many different methods have been employed by researchers to quantify phytoplankton community composition. Previous studies have demonstrated that the way in which community composition is quantified can influence the interpretation of environmental dependencies. Researchers must consider both the suitability of the data they collect for monitoring marine ecosystems, as well as the research effort required to collect representative datasets. We therefore seek to understand how the representation of phytoplankton community structure in the western Barents Sea, a rapidly changing Arctic shelf sea, influences the interpretation of environmental dependencies. We compare datasets of cell counts, phytoplankton pigments and bio-optics (absorption spectra), relating them to a suite of environmental conditions with multivariate exploratory analyses. We show that, while cell counts reveal the greatest insight into environmental dependencies, pigment and absorption spectral datasets still provide useful information about seasonal succession and the influence of Atlantic water masses– two key subjects of great research interest in this region. As pigments and optical properties influence remotely-sensed ocean-colour, these findings hold implications for remote detection of phytoplankton community composition.
1 Introduction
There are many thousands of phytoplankton species in the global ocean, adapted to different marine environments. For example, some Arctic specialists can survive through polar night (Berge et al., 2020), and others practice sessile lifestyles underneath or within sea ice (Syvertsen, 1991). The different environmental tolerances and physiologies of different phytoplankton groups mean that they make different contributions to global biogeochemical cycles and marine food webs. Calcite secretion by coccolithophores may moderate the dissolution of carbon dioxide in the oceans (Shutler et al., 2013). Diatoms, by contrast, secrete silicified frustules, resulting in rapid sinking, the nourishment of deep-sea animals (Falkowski et al., 1998; Jiang et al., 2005) and a key role in marine silica cycles (Egge and Aksnes, 1992). Phaeocystis spp., (hereafter referred to as Phaeocystis) constitutes an important component of the global sulphur cycle (Wang et al., 2015).
Thus, understanding and monitoring the relationship between phytoplankton community composition and environmental conditions is important, because environmental change may restructure the functional contributions that ecosystems make to biogeochemical cycles. Exploring how these dynamics may evolve in a changing environment is of great research interest, because phytoplankton contribute half of global net primary production (Field et al., 1998), sustain most marine animals and transfer atmospheric carbon to long-term reservoirs (Buesseler et al., 2020).
Nowhere are these questions more urgent than the Arctic Ocean, which is undergoing rapid environmental changes (Overland et al., 2018), including receding sea ice (Kwok, 2018) and increasing inflows of temperate waters (Polyakov et al., 2017; Ingvaldsen et al., 2021). The composition of phytoplankton communities on productive Arctic shelves is shifting in response to a warmer, more Atlantic-influenced hydrography. Small haptophyte species are increasingly dominant in the Fram Strait region (Nöthig et al., 2015), coccolithophores are expanding further into historically Arctic-influenced waters (Neukermans et al., 2018; Oziel et al., 2020) and there may be an increasing occurrence of Phaeocystis (Orkney et al., 2020; Galí et al., 2021). These changes may substantially alter fluxes of climatologically-relevant aerosols (Galí et al., 2019), and modify carbon cycling (Dybwad et al., 2020).
The Barents Sea is a productive Arctic shelf region (Wassmann et al., 2006), supporting a significant fishery (Drinkwater, 2006) and there is hence great interest in understanding its response to climate change. Changes in ecosystem function in the Barents Sea may influence pan-Arctic biogeochemical cycling and have significant economic consequences. The Barents Sea is relatively accessible and therefore has a long history of study, fostering a mature understanding of the region’s phytoplankton community composition and the common modes of seasonal succession it experiences [e.g. Degerlund and Eilertsen (2010)]. Microscopic cell counts, often resolved to genera or species, have revealed ‘indicator’ species that dominate seasonal succession and compositional variety across different water masses in the Barents Sea [see Rat’kova and Wassmann (2002); Wassmann et al. (2006); Degerlund and Eilertsen (2010) for detailed reviews].
Continued collection of microscopic observations will, no doubt, form an indispensable component of research effort to monitor and understand Arctic change. However, large-scale studies are limited because producing cell count data is labor intensive and requires expert personnel. Furthermore, nanophytoplankton cannot be enumerated at genera or species level under optical microscopes, requiring less taxonomically-resolved methods such as flow-cytometry (Zubkov et al., 2007). Hence, micro- and nanophytoplankton components of community structure are intrinsically treated differently in cell count analyses. As it is not possible to process large volumes of microscopic observations, alternative measures of community structure are deployed at scale. These include studies of the ratios of taxon-indicative pigments or bio-optical properties. These methods do not require the separation of micro- and nanophytoplankton, which may mean these datasets are inherently different to cell count data. Ratios of taxon-indicative pigments measured by High Performance Liquid Chromatography (HPLC) provide a coarser impression of community composition than microscopic observations, because they are limited to the resolution of broad taxonomic groups, rather than key species or genera (Mackey et al., 1996). Furthermore, few pigment biomarkers are specific to single taxonomic groups of phytoplankton (Jeffrey et al., 2011), meaning de-convolution methods such as ‘CHEMTAX’ are often employed to produce estimates of contributions from broad taxonomic groups [e.g. Fragoso et al. (2017)]. These methods assume prior knowledge of pigment complements for specific phytoplankton groups in particular regions. Photoacclimation mechanisms can change the ratios of pigments within phytoplankton cells (Kauko et al., 2019), which can undermine de-convolution. We elect not to attempt de-convolution here.
Phytoplankton pigments interact with visible light, and research has demonstrated that ratios of taxon-indicative pigments can be inferred from phytoplankton absorption spectra (Johnsen et al., 1994; Chase et al., 2013). Recent investigations in the western Barents Sea have demonstrated absorption spectral properties can also provide information about spatial distribution of pigments within cells (pigment packaging), which can proxy the distribution of cell-sizes within phytoplankton communities (Liu et al., 2019). We can therefore see that researchers monitoring Barents Sea ecosystem function, and its response to climate change, exploit a variety of different methods to quantify phytoplankton community composition.
It is important to explore how these different types of data may affect capacity to measure and monitor phytoplankton community composition and the way it changes in response to environmental change. We might expect that less taxonomically resolved datasets, such as pigment ratios, should provide us with a less detailed interpretation of environmental dependencies, compared to the insight offered by detailed microscopic cell counts resolved to the level of genus or species. However, a study in the English Channel shows this may not always be the case; ecological equivalence between many phytoplankton species meant that broad phylogenetic groupings were more informative than individual species counts (Mutshinda et al., 2016). The influence of data type on the interpretation of environmental dependencies can hence not be concluded a priori, and must result from exploratory analysis. Previous studies have undertaken such analysis [e.g. Oliveira et al. (2021)], and made qualitative statements about the utility of different data types to foster the interpretation of environmental dependencies and ecosystem dynamics in other parts of the global ocean.
We apply multivariate statistical analyses to explore how variation in phytoplankton community composition depends on environmental conditions in the western Barents Sea. Cell counts, pigment ratios and absorption spectra are employed to quantify phytoplankton community composition. Differences in the environmental dependencies, that control variance in these different datasets, may reveal how research methods affect the interpretation of variance in phytoplankton community composition. Such differences may be important for interpreting the significance of ecosystem change for wider biogeochemical cycles and marine food web function. We also explore whether overall biomass variation and photoacclimation influence indices of phytoplankton community composition and investigate the relevance of diatom dominance to overall patterns of seasonal succession. We perform tests to determine whether phytoplankton communities from Atlantic waters have distinctive community compositions and environmental dependencies. Seeking answers to these questions is important because it will help inform the collection of future ocean observations and provide researchers with a better understanding of sources of marine environmental change, such as increasing Atlantic influence in the Eurasian Arctic.
2 Methods
2.1 Data Collection
Phytoplankton samples were collected aboard three research cruises conducted as part of the UK-led Changing Arctic Ocean research programme, throughout the western Barents Sea, with a special focus on sampling the seasonal ice zone along the 30th eastern meridian. The locations of hydrographic and biological sampling are presented in Figure 1.
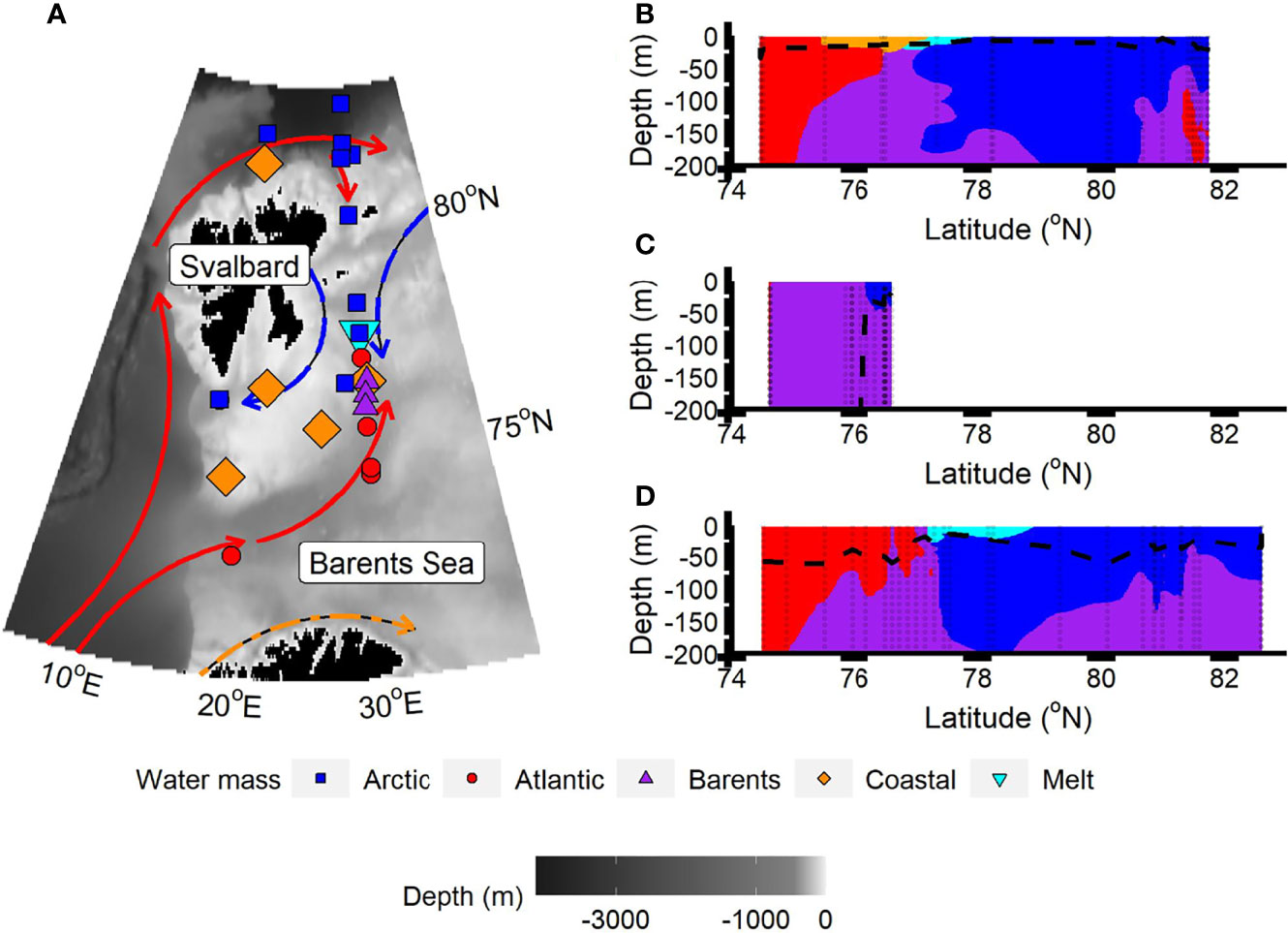
Figure 1 (A) Map of phytoplankton samples considered in this study. Inflowing Atlantic, Arctic and coastal water masses are represented with solid red, dashed blue and dot-dashed orange arrows. Right; from top to bottom: (B) JR16006 (summer 2017), (C) HH180423 (spring 2018), (D) JR17006 (summer 2018). Water masses: Arctic Water (blue), Atlantic Water (red), Barents Sea Water (purple), Coastal Water (orange) and Melt Water (cyan). The mixed layer depth, defined in accordance with Peralta-Ferriz and Woodgate (2015), is represented by a dashed black line. Vertical dotted lines represent CTD profiles.
The three cruises were distributed across late summer 2017 (JR16006; 30th June– 8th August 2017) spring 2018 (HH180423; 23rd April– 5th May, 2018), and early summer 2018 (JR17006; 11th June– 6th July 2018) in order to capture the seasonal evolution of hydrographic conditions in the western Barents Sea and commensurate succession of phytoplankton communities. Some of the variety in environmental conditions is caused by inter-annual variability. This is evident in sea ice conditions, which varied widely between the two summer cruises. The northern limit of sampling was restricted on the spring cruise by heavy sea ice conditions, and the southern limit of the sea ice was determined by the extent of the Arctic water mass (Barton et al., 2020).
A Conductivity-Temperature-Depth (CTD) rosette equipped with a Seabird SBE911Plus CTD unit, including a LICOR PAR sensor and Chelsea AQUAtracka III fluorometer, was used to produce downward profiles of in-vivo chlorophyll-a fluorescence and hydrography, which informed the selection of depths for the collection of waters for biological sampling on the upward cast. Typically the surface, chlorophyll-maximum, and 2-3 other depths bracketing these features, were targeted for sampling with remotely-triggered Niskin bottles. The sample bottles were then subsampled for measurements of phytoplankton absorption spectral properties, HPLC (High Performance Liquid Chromatography) pigments, nutrient concentrations and phytoplankton cell abundances.
2.2 Environmental Data
2.2.1 Hydrography
The sensor array on the CTD package recorded profiles of temperature, practical salinity, depth, oxygen saturation and Photosynthetically Available Radiation (PAR) at a 1 m vertical resolution on all deployments. Oxygen saturation was determined using a Seabird polargraphic oxygen probe, calibrated with Winkler titrations (Carpenter, 1965). Temperature was measured with SBE3plus sensors and salinity inferred from conductivity readings derived from SBE 4C sensors. Further detail on the preparation of hydrographic data is available in the cruise reports (see data availability statement for further details). The depth of the maximum Brunt-Väisälä (B-V)/‘buoyancy’ frequency at each station was computed from temperature, pressure and salinity, using pre-built functions of the oce R package version 1.2-0 (Kelley and Richards, 2020). This provides an indication of water column physical structure. We refer to this property as ‘Stratification’. σθ was calculated automatically on deployments from CTD profiles of temperature, salinity and pressure.
The seasonality of hydrographic conditions is illustrated in Figure 1, and water masses are adapted from Oziel et al. (2016) and Våge et al. (2016), defined by salinity and temperature (Atlantic >34.8‰, > 3°C; Arctic < 34.8‰, < 0°C; melt < 34.4‰, > 0°C, < 3°C; coastal < 34.8‰, > 3°C; Barents < 34.8‰, > 2°C). We assigned those waters which did not meet any of these criteria to the ‘Barents’ water mass. A potential-temperature–salinity plot for the phytoplankton samples presented in this study, with water mass definitions, is presented in the supplement (Figure S1). We note that our definition of ‘coastal’ water includes waters that form around the Svalbard archipelago and Spitsbergen bank (Figure 1). Mixed layer depth, which is illustrated in Figure 1 to provide insight into the density structure of the upper water column, is defined as the depth at which the potential density anomaly (σθ) increases to 0.1 kg m-3 above the surface reference (Peralta-Ferriz and Woodgate, 2015). An example dataset of CTD-derived hydrographic parameters on cruise JR16006 is available online (Dumont et al. 2019).
2.2.2 Nutrients
Triplicate 50 ml subsamples were taken from Niskin bottles for assays of nitrate plus nitrite (hereafter referred to as ‘nitrate’ as contributions of nitrite were negligible), silicic acid and ammonium, with acid-washed polythene vials. Samples were filtered through clean 200 μm nylon mesh to remove zooplankton. Samples were brought to room temperature before measurement with a Lachat Quikchem 8500 flow injection auto-analyser within 12 hours of collection. The following manufacturer-recommended methods were used to quantify nutrient concentrations: ammonium, 31-107-06-1-B; silicic acid, 31-114-27-1-A and nitrate/nitrite, 31-107-04-1-A. The analyser was standardized using international certified reference materials for nutrients in seawater (KANSO Limited, Japan), and samples were analysed against standard solutions made up in a low-nutrient seawater (OSIL batch 26) standard matrix. Five calibration standards and blank seawater were analysed before each batch process and a linear correction was applied to correct for drift over each analytical run. There was a detection limit of 0.1 μM. No nitrate samples measured below this value. No alteration was made to silicic acid or ammonium samples below this concentration, because any absolute error is small compared to overall gradients across the dataset. Nutrient data were matched to phytoplankton community composition samples under the criteria that they must originate from the same location and date. If there is no exact depth match, then a linear interpolation is computed among the two samples with the closest two depths. If there are no samples within a 20 m interval then a match is not found. Of the 40 phytoplankton samples considered in this manuscript, 19 had matching measurements of nutrient concentration and 21 were assigned interpolated nutrient concentrations (Brand et al., 2019a; Brand et al., 2019b).
2.3 Phytoplankton Community Structure
2.3.1 Cell Counts
50 ml subsamples, preserved with 1% Lugol’s solution, were prepared for optical microscopy following the Utermöhl technique (Utermöhl, 1958). Chamber counts were made with a Zeiss Axio S100 inverted microscope with x20 magnification and phase-2 contrast conditions, following established practice (Davidson et al., 2013). Counts of recognisable genera and species were produced in consultation with Tomas (1997), Throndsen et al. (2007), and Hoppenrath et al. (2009). Colonial forms such as Phaeocystis and Dinobryon spp. were excluded, reflecting routine practice in the literature (Degerlund and Eilertsen, 2010). We supplemented the microphytoplankton counts with flow-cytometric measurements of nanophytoplankton, following the example of Ardyna et al. (2011), and the method of Zubkov and Burkill (2006) to stain and process samples. Generally, a size cut-off of 10 μm divided micro- and nanophytoplankton counts. We distinguished phototrophic (PNAN) and heterotrophic (HNAN) nanoflagellates by their red fluorescence (Zubkov et al., 2007) and further divided them into small (< 6μm) and large (> 6μm) categories. As cell counts did not always originate from the same Niskin bottles as pigment and absorption spectra samples, we matched them to one another under the criterion they must originate from the same location, within 2 metres of depth. The sample with the smallest number of microphytoplankton cells was represented by 450 cells, whereas 166195 cells were observed in the sample with the most microphytoplankton cells. The dimensions of a maximum of 30 cells of each recognisable genus or species were measured with a calibrated microscope eye-piece graticule at the chlorophyll-a maximum of each sampling site. Shape-dependent geometric relationships (Sun and Liu, 2003) were then used to calculate cellular volumes across each recognisable genus or species. Calculated volumes were converted to biomass with the aid of correction factors for preservational effects, for diatoms (Menden-Deuer et al., 2001; Montagnes and Franklin, 2001), dinoflagellates (Menden-Deuer and Lessard, 2000; Menden-Deuer et al., 2001) and ciliates (Leakey et al., 1994), to arrive at final carbon biomass estimates. These inferred biomasses may be biased if cells in chlorophyll-maximum communities differ significantly from other depth horizons. The average cellular carbon biomasses were then extended across the full dataset to produce an estimate of biomass composition. Phototrophic and heterotrophic nanoflagellate average biomass values per cell were assumed to be identical to samples from ICE CHASER II cruise JR219. Original cell count datasets are available online (Mitchell et al., 2019a; itchell et al., 2019b).
2.3.2 Pigment Analysis
Subsamples, typically between 1 and 2 L, were collected from sample bottles and filtered through 0.7 μm pore GF/F filter pads. Samples were frozen in liquid nitrogen and transferred to -80◦C storage. Samples were shipped to the Dansk Hydrualisk Institut (DHI; Hørsholm, Danmark) for HPLC analysis. The samples were prepared in a vortex, sonicated and extracted at 4◦C before further mixing. The samples were filtered through a 0.2 μm Teflon syringe filter and the filtrate transferred to vials for analysis. Buffer solution and filtrate were mixed in a 5:2 ratio, and analysed following the HPLC method of Van Heukelem and Thomas (2001). Concentrations of the following pigments were quantified: chlorophyll-a, chlorophillide-a, chlorophyll-b, chlorophyll-c3, chlorophyll-c2, chlorophyll-c2-monogalactosyldiacylglyceride, chlorophyll-c1, pheophorbide-a, phaeophytin-a, peridinin, 19′-butanoyloxyfucoxanthin, fucoxanthin, neoxanthin, prasinoxanthin, 4-keto-19′-hexanoyloxyfucoxanthin, violaxanthin, 19′-hexanoyloxyfucoxanthin, diadinoxanthin, dinoxanthin, myxoxanthophyll, antheraxanthin, alloxanthin, diatoxanthin, zeaxanthin, lutein, gyroxanthin-diester, α- and β-carotene. All pigments were considered in the analyses presented herein.
2.3.3 Phytoplankton Absorption Spectra
Subsamples of up to 1 L were collected from Niskin bottles and filtered through 0.7 μm pore size GF/F filters under a pressure not exceeding one half atmosphere. Samples were frozen in liquid nitrogen and transferred to -80°C storage. In the laboratory, samples were thawed to -20°C in a dark environment and moistened with a drop of filtered deep Atlantic water. The absorption spectra of the moistened filter pads were recorded with a Shimadzu UV-Vis spectrophotometer equipped with an integrating sphere at a 1 nm resolution, following the method of Kishino et al. (1985). This included the extraction of pigments with methanol to determine absorption by detritus. Filter pads were rotated by a quarter revolution 4 times, collecting replicates that were subsequently averaged, to control for uneven distribution of cells on the filter pads. We used the binomial path length correction equation of Hoepffner and Sathyendranath (1992) to convert the optical densities into absorption coefficients. We subtracted exponential fits to the detrital absorption spectra from the total particulate absorption spectra to attain the absorption spectra of the phytoplankton. Original datasets of phytoplankton absorption spectra are available online (Orkney and Bouman., 2019a; Orkney and Bouman., 2019b; Orkney and Bouman., 2019c).
2.4 Data Analysis
A total of 40 phytoplankton samples were found across the three cruises, for which complete cell count, pigment, bio-optical, hydrographic and nutrient data were available.
Prior to analysis, cell counts were log-plus-one-transformed, as in Peperzak (2010), and normalized to the sum of all transformed counts in each sample. Pigment ratios were normalized to the sum of pigments in each sample and absorption spectra were similarly prepared by normalization to the integral between 400 and 750 nm. This normalization procedure was performed in order to render variation in phytoplankton community composition independent from overall gradients of biomass.
Redundancy Analyses (RDA), a multivariate exploratory analytical method (Legendre et al., 2011; Legendre and Legendre, 2012), were implemented in R version 3.6.3 (R Core Team, 2020), using the vegan package version 2.5-6 (Oksanen et al., 2019), to explore the relationships between phytoplankton community composition and environmental variation. This resembles the methodology employed in previous studies to probe environmental dependencies across biological communities (e.g. Janišová et al., 2007; Ardyna et al., 2011 and Fragoso et al., 2017). In each RDA analysis, a phytoplankton community compositional dataset (cell counts, pigments, absorption spectra), assumed the role of the dependent variable matrix ‘P’ and the environmental variables were employed as the explanatory variable matrix ‘X’. RDA produces a multivariate ordination space that is constrained by the explanatory variables. RDA is conducted by performing multiple linear regressions of columns in P depending on columns in X , to find the fitted portion of P that is explained by X; '':
Where X′ represents the transpose of X. See Legendre and Legendre (2012) Chapter 11 ‘Redundancy Analysis’ section for an involved description. Thereafter, singular value decomposition can be performed on '':
where WT is a rotation matrix that aligns onto a new set of mutually orthonormal axes. U represents the distribution of individual samples (rows) in along those new axes which describe the greatest variance in . Σ is a rectangular matrix, whose diagonal elements describe the relative contribution of explained variance by pairs of vectors in U and WT . Rows of P can be projected into this space, as can gradients in the constituent variables that make up X. The angles between the vectors, representing rows of P and constituent variables of X, describe the environmental dependencies across P. These angles can be summarised as a matrix of dot-products (also known as the cosθ index). The cosθ index is a scaled similarity index employed by biologists and ecologists to represent pair-wise structure between different replicates in multivariate space. The order of rows and columns in this matrix is arbitrary, therefore we have elected to order them by the contribution of diatoms to microphytoplankton biomass. We made this decision because the fractional dominance of diatoms is of interest to many marine scientists [e.g. Sathyendranath et al. (2004); Jackson et al. (2010); Mutshinda et al. (2016)]. We also present a version ordered by the month of sampling, to facilitate interpretation of seasonal patterns in environmental dependencies. The angles between variation in community composition and environmental variables represent the relative affinity of different community compositions to different environmental conditions. Therefore, if a gradient in community composition is accompanied by a gradient in cosθ index for a certain environmental variable, this provides evidence that an environmental dependency upon that variable could explain some structure in phytoplankton community composition. Desirable properties of the cosθ index include its agnosticism to magnitude and its capacity to represent inverse similarity, with a possible range varying between -1 and 1.
We computed further RDAs in which the environmental variables have been scaled by their variance and decomposed by Principal Component Analysis. While this may make interrogation of patterns more taxing, transformation of the explanatory variables to eliminate co-linearity may make the resultant analysis output more amenable to significance testing. Pure, term-wise and marginal effect tests (Legendre et al., 2011) were applied to each RDA, to provide an indication of the combinations of environmental variables that significantly explain structure in phytoplankton community composition.
We produced biplots of the PC-axes that were recurrently identified as significantly driving structure in phytoplankton community composition, in order to explore their intersection with water mass properties and seasonal succession.
Finally, we produced ‘triplots’, by plotting the leading axes of decomposed derived from cell counts, pigments and absorption spectra. This produced spaces which describe the environmental dependencies across the different phytoplankton community compositional datasets. We projected salient environmental variables and phytoplankton community compositional indices on to these spaces, to provide an intuitive summary of our findings.
3 Results
3.1 Overview of Datasets
Examples of vertical sampling profiles are presented in Figure 2 to provide an impression of the range of data we collected.
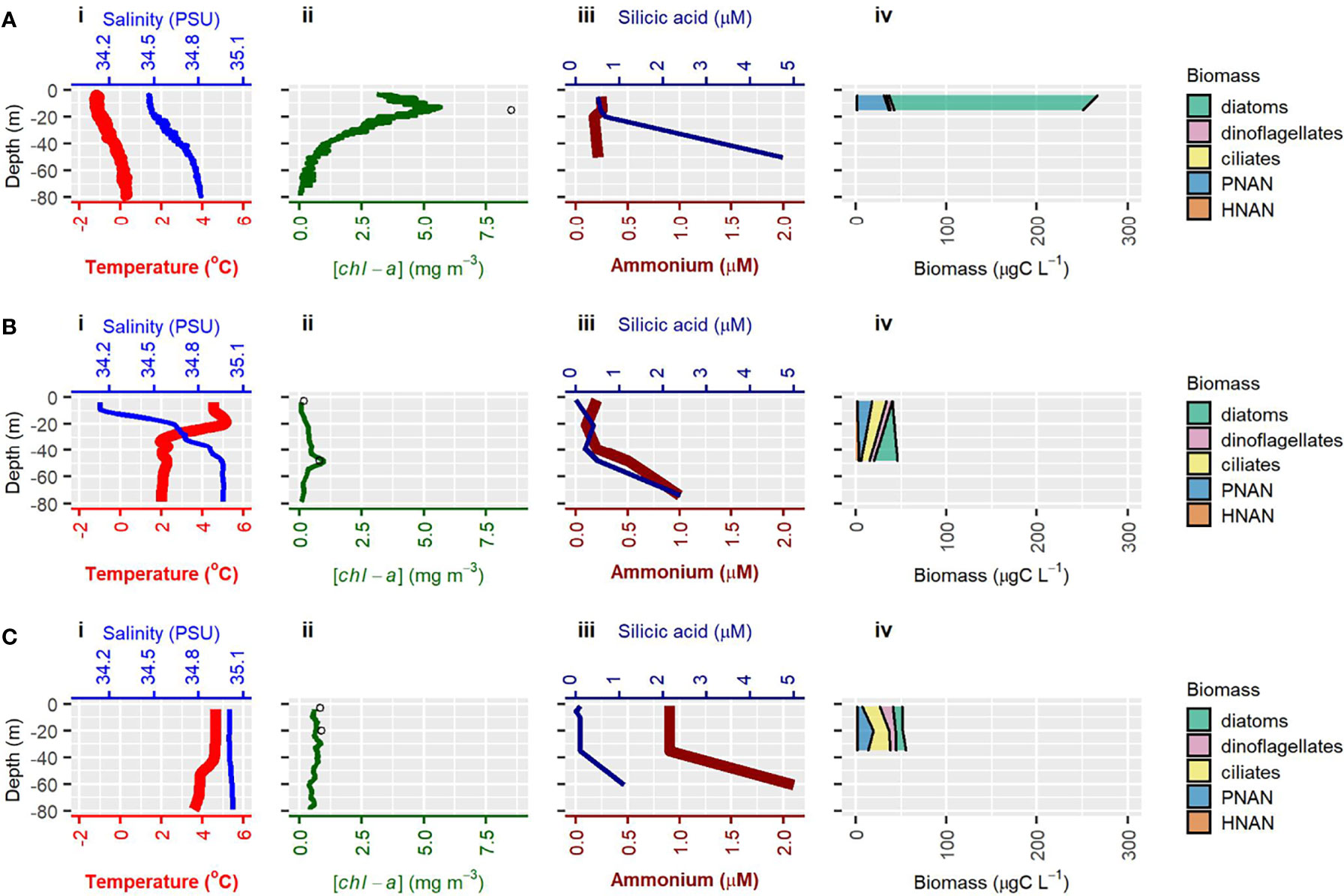
Figure 2 Profiles of vertical properties: (A) 29th April, 28.3∘ east 76.5∘ north (HH180423), (B) 30th July, 30.3∘ east, 76.5∘ north (JR16006), (C) 2nd July, 30∘ east, 75.5∘ north (JR17006). (i) Hydrography, (ii) Chlorophyll-a fluorescence (line) and HPLC validation (points), (iii) Nutrient, (iv) Biomass estimates of community composition derived from cell counts. PNAN indicates phototrophic nanoflagellates. HNAN indicates heterotrophic nanoflagellates.
The fraction of variance in the environmental datasets explained by the scaled principal component decomposition axes is as follows: PC1: 0.34 PC2: 0.22 PC3: 0.15 PC4: 0.11 PC5: 0.07 PC6: 0.06 PC7: 0.04 PC8: 0.01 PC9: <0.01 PC10: <0.01. Principal components beyond PC7 explain only ≈ 6% of variance in the Environmental dataset. A truncated rotation matrix, outlining the contribution of variables (loadings) that constitute each PC-axis, is provided in Table 1 and a full version is provided in Table S1. Table 1 shows that increasing PC1 is associated with declining σθ, lower salinity and temperature, higher oxygen saturation and declining nutrients. This axis’ hydrographic associations indicate it is a proxy for Arctic influence; lower stratification depths and nutrient concentrations could indicate that this axis is associated with strong haline stratification. PC2, by contrast, describes a mode of correlation in which temperature, salinity, and PAR are inversely correlated with nitrate concentrations. This is indicative of high PC2 representing Atlantic influence and being associated with samples taken later in the productive season, after heterotrophs have liberated ammonium. Higher PC3 scores are associated with enhanced silicic acid and nitrate concentrations, higher PAR and shallow sampling depth. An affiliation of PC3 to a specific water mass is not clear. Higher PC4 scores are associated with deeper stratification depths, high ammonium concentrations, low σθ, and low salinity. High scores in PC5 and PC6 are both associated with warmer temperatures, deeper sampling depths and lower σθ, but have antithetical relationships to the evolution of oxygen and ammonium concentrations. The meaning of higher axes, such as PC5-6, for which water mass affiliations are not immediately clear, are discussed and clarified in more detail later in the results section.
Figure 3 shows that the balance of major phytoplankton groups changes as a function of both geography and seasonality. Diatoms dominate in the spring, before phototrophic nanoflagellates rise to dominance, and eventually are themselves succeeded by heterotrophs. Tables, representing the rank abundance of the most common microphytoplankton by counts and biomass, are presented in the supplementary material (Tables S2, S3). By late summer, diatoms are only a substantial component in under-ice blooms, that are otherwise dominated by phototrophic nanoflagellates. Gelatinous mucus, ship-board microscopic observations and a strong smell of dimethyl sulphide, lead us to believe these under-ice blooms could be Phaeocystis pouchetii blooms, vis à vis Pavlov et al. (2017); Dybwad et al. (2020). The mean contribution of chlorophyll-c3 to pigment biomass in samples suspected of Phaeocystis pouchetii dominance was 20%, compared to 6% in other samples, while 19′-hexanoyloxyfucoxanthin contributed 10% of pigment biomass, compared to 4% in remaining samples. Previous published research has shown that these samples possess bio-optical properties similar to Phaeocystis bloom samples from the Labrador Sea [see Supplementary Materials in Orkney et al. (2020)].
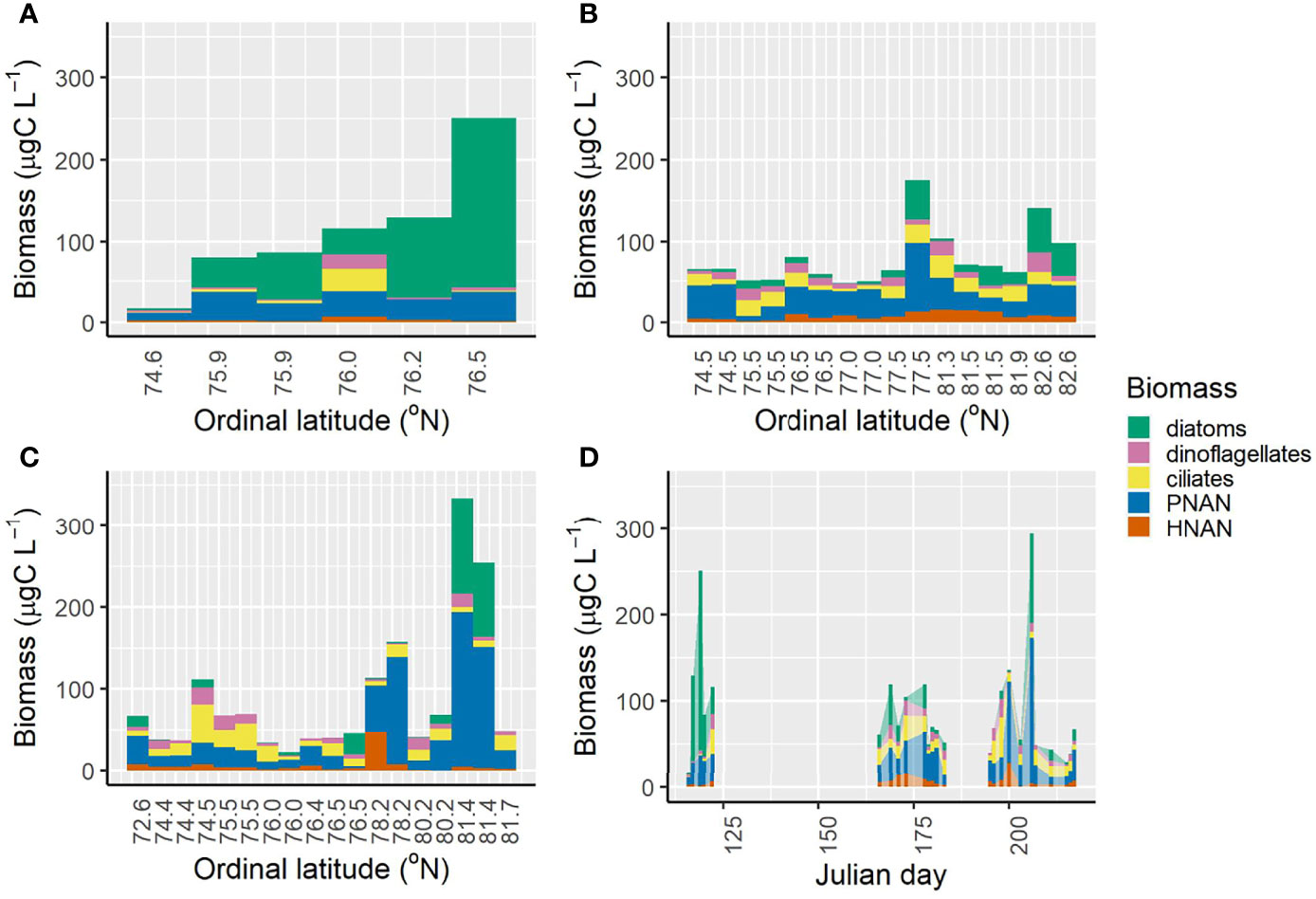
Figure 3 Biomasses derived from cell count data for (A) HH180423 (spring 2018) (B) JR17006 (summer 2018) (C) JR16006 (summer 2017), organised by latitude and (D) all samples, aggregated by Julian day, with linear interpolation between samples separated by fewer than 10 Julian days. PNAN indicates phototrophic nanoflagellates. HNAN indicates heterotrophic nanoflagellates.
Redundancy Analyses are not a comprehensive summary of all variance in phytoplankton community composition, only the portion of fitted variance that can be explained by the explanatory dataset of environmental variables. However, it is this portion of variance in phytoplankton community structure that is of special interest to researchers exploring the intersection of environmental change and phytoplankton community structure. 42%, 45% and 42% of total variance is explained by environmental variables in the cell count, pigment ratio and absorption spectra datasets respectively. Therefore, while a majority of variance is unconstrained, a substantial portion of variance does respond to environmental variation. The unexplained portion might result from stochastic variability in phytoplankton community structure [e.g. Mutshinda et al. (2016)], variability resulting from internal biological variables such as inter-specific interactions, or environmental variables that are not represented in our dataset, such as the advection of inoculating phytoplankton communities from temperate water masses in Atlantic inflow currents [e.g. Oziel et al. (2020)], or variable concentrations of micro-nutrients.
3.2 Gradients in Diatom Dominance
The cosθ matrices, displayed in Figures 4, S2, S3, demonstrate that different environmental controls of phytoplankton community composition are emphasized by cell counts, pigment ratios and absorption spectra. For example, fractional diatom contribution to microphytoplankton biomass is associated with changing values of cosθ for silicic acid and nitrate concentration across the cell counts and absorption spectral datasets. However, this pattern is not manifest within the pigment data. Additionally, RDA derived from pigments reveals that diatom-rich samples are associated with the evolution of dissolved O2, a feature less evident in analyses derived from cell counts and absorption spectra. Despite these differences, all analyses identify a broad swath of similar environmental dependencies.
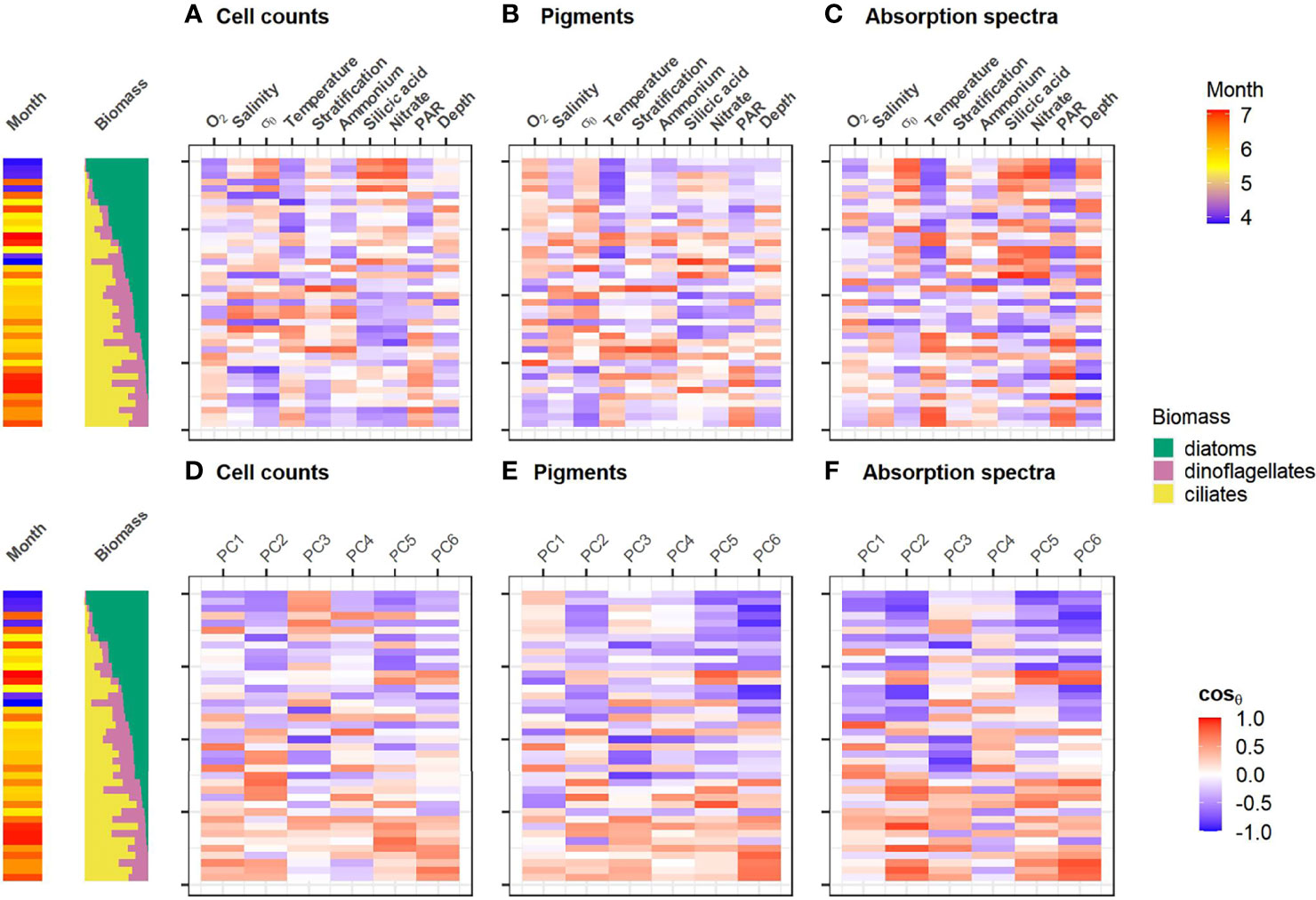
Figure 4 Cosθ matrices computed from Redundancy Analysis (RDA), relating phytoplankton cell counts, pigments and absorption spectra to explanatory variables– (A–C), and by decomposed axes– (D–F) (higher axes that do not significantly explain variance in community composition are omitted; see Figure S2 for full Figure). Samples are ordered by diatom contribution to microphytoplankton biomass to provide an intuitive impression of environmental dependencies across the samples. Differing gradients, for example over silicic acid concentration between cell counts and pigments, demonstrate that salient environmental dependencies across the phytoplankton samples depend on data type. PAR, ‘Photosynthetically Available Radiation’. Depth: depth of sample. σθ: potential density. Stratification: depth of maximum buoyancy frequency.
All analyses indicate gradients in cosθ, aligned with diatom dominance, for temperature, PAR, depth and σθ. Taken together, these patterns indicate a succession of diatom blooms beginning in cold spring conditions, associated with the upwelling of deep, nutrient-rich waters. Depth and PAR likely exhibit a co-linearity, and it is possible this relationship is exaggerated by self-shading when phytoplankton blooms are intense (Barros et al., 2003).
In order to explore this possibility, we contrived a proxy of pigment packaging by dividing the absorption coefficient at 676 nm by chlorophyll-a concentration. The logarithm of this ratio has a strong linear negative relationship to the logarithm of chlorophyll-a concentration, consistent with the power-law model fit of Bricaud et al. (1995) (see Figure S4). This suggests higher pigment packaging in more pigment-rich samples. We hypothesized that this is because high biomass concentrations were usually associated with more intense blooms of diatoms, that tended to have larger cells and more intense pigmentation. We used the reciprocal of the logarithm of specific absorption at 676 nm as a proxy of pigment packaging in our samples (so that higher values connoted higher packaging). We constructed a linear model in samples shallower than 20 m (and therefore likely to experience intense PAR) of pigment packaging depending additively on PAR and diatom biomass. This model had a moderate goodness of fit. Variation in pigment packaging was explained significantly by diatom biomass but not PAR (p for PAR =0.27, p for diatom biomass <0.01, adjusted R2 = 0.58 for the overall additive model). This lead us to conclude that variation in the packaging index is most likely driven by variation in diatom and microphytoplankton dominance, rather than photoacclimation or self-shading mechanisms.
Inspection of cosθ matrices, for which principal component decomposition has been performed on the environmental variables (Figure 4), implies that gradients in diatom-dominance are associated with varying scores along PC1, 2, 5 and 6, although patterns along PC1 and 2 are less clearly organized in the analyses derived from pigments. Therefore, there is strong evidence that gradients in diatom-dominance are structured by water mass properties.
3.3 Seasonal Succession
Re-ordering the rows of the cosθ matrices with respect to time (Figure S3) reveals modes of seasonal succession. Due to a trend of decreasing diatom dominance over the productive season (Figure 3), these modes of succession are similar to those revealed by diatom biomass. RDA derived from cell counts and absorption spectra both indicate a similar progression of declining cosθ for nitrate and silicic acid concentrations with time, associated with early dominance of diatoms. cosθ values for temperature and PAR generally increase with time across analyses derived from cell counts, pigments and absorption spectra. This may indicate that increasing insolation, and its consequent effects on hydrographic properties, play an increasingly important role structuring phytoplankton community composition as the season progresses from spring to summer.
RDA derived from cell counts and pigments both indicated an abrupt and transient increase in cosθ in early summer across salinity, σθ, temperature, stratification depth and ammonium concentration, but this signal is not clearly evident in the analysis derived from absorption spectra. The increase in cosθ coincides with sampling in the Atlantic water mass. A decrease in cosθ for salinity and σθ towards the end of the time series, in RDA derived from cell counts, is associated with diatom-rich samples derived from the Arctic water mass.
Organization of cosθ across time for the decomposed environmental variables follows a similar pattern to that evident in Figure 4. cosθ values tend to increase across PC5 and 6 across all datasets, as diatom dominance declines over time. This interaction between PC5, PC6 and time-of-sampling implies that PC5 and 6 capture a signal of seasonal succession in environmental conditions. Organization of PC1 and 2, which proxy hydrography, is less evident when samples are organized by time. This is consistent with the fact that different water masses were sampled multiple times across the different research cruises.
3.4 Significance of Environmental Drivers
Significance testing finds inconsistent results between RDA derived from cell counts, pigments and absorption spectra (Table S4). Furthermore, different methods for significance detection produce contradictory results. This could make it difficult to discern which environmental variables significantly control variation in phytoplankton community composition. It is likely that this results from co-linearities that exist between environmental variables. Multiple co-linearity is known to disrupt significance testing (Legendre et al., 2011). Despite this, it can be stated that some hydrographic variables, such as temperature and σθ, are repeatedly identified as significantly explaining variance. Salinity and silicic acid concentration significantly explain structure in cell count and pigment datasets, while the evolution of ammonium is only revealed as a significant explanatory variable in cell count data.
Inspection of Tables 2 and S5, for which the environmental variables have been rotated by principal component decomposition to eliminate multiple co-linearity, reveals a much more consistent picture. PC2, 5 and 6 significantly explain variance across almost all datasets, while PC1 and PC3 are more likely to explain variance in cell counts. In general, a greater number of PC-axes significantly explain structure in cell counts compared to absorption spectra or pigments. This suite of results shows that all phytoplankton community compositional datasets provide some level of insight into processes of seasonal succession (PC5-6) and Atlantic influence (PC2) upon phytoplankton community structure.
3.5 Visualization of Phytoplankton Community Structure and Environmental Dependencies
Biplots of leading PC-axes, that significantly explain phytoplankton community composition, are presented in Figure 5. PC1-2 clearly distinguish water masses (Figure 5A); environmental properties become increasingly ‘Arctic’ along PC1 and increasingly ‘Atlantic’ along PC2, with intermediary Barents Sea Water typified by low scores on both axes. Hence, Table 2 demonstrates that RDA derived from cell counts, pigments and absorption spectra all successfully identify varying community composition along the ‘Atlantic’ axis. Community compositional change that is structured along the ‘Arctic’ axis is more evident when cell counts are employed to represent community composition. A linear model of time-of-sampling, depending additively on PC5 and PC6 (of the form Y = aX1 + bX2 +c, where X1 and X2 are PC5 and PC6-score), is highly significant (p < 0.01, adjusted R2 = 0.34). PC5 and PC6 significantly explain change in community composition across all phytoplankton community compositional datasets (Table 2), demonstrating that seasonal succession is an important feature that structures community composition in the western Barents Sea. A biplot of PC1-3 space (Figure 5B) does not show any strong association of PC3 with water mass. Given higher PC3 scores are associated with higher silicic acid and nitrate concentrations, PC3 may perhaps be considered a ‘eutrophy’ axis. However, we comment that it is difficult to reconcile this observation with the fact that diatoms often prosper in eutrophic conditions, because PC3-score does not significantly covary with time-of-sampling (p = 0.07), or contributions of diatoms, dinoflagellates or ciliates to microphytoplankton biomass (p = 0.50, 0.15, 0.38). A significant negative linear correlation is found between PC3 score and fucoxanthin contribution to pigmentation (p = 0.04, adjusted R2 = 0.09). The inconsistency of the linear relationships of PC3-score with diatom biomass contribution and fucoxanthin contribution suggests that fucoxanthin may occur in phytoplankton groups other than diatoms. Alternatively, different populations of diatoms may have variable fucoxanthin content.
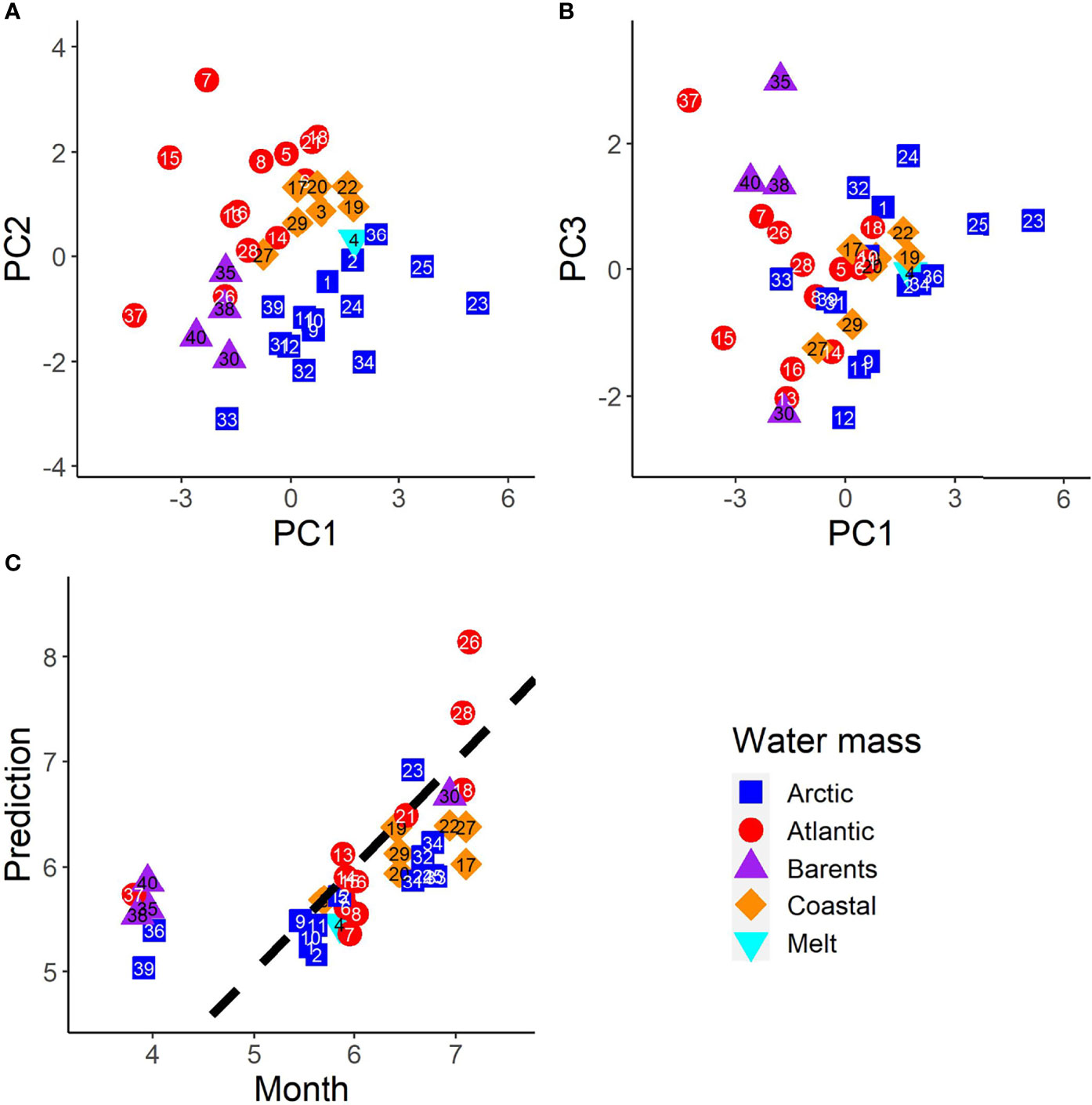
Figure 5 (A) PC1-2 space of environmental variables, showing clear evidence of clustering by water mass. (B) PC1-3 space of environmental variables. (C) Month of sampling, compared to prediction derived from linear additive model of PC5-6. A dashed line marks a 1:1 slope. Samples are identified by unique numbers.
RDA triplots are presented in Figure 6. It can be seen that, for all community compositional datasets, PC2, 5 and 6 are aligned along RDA1. This reflects the important role of seasonal succession in structuring phytoplankton community composition. The alignment of PC2 with RDA1 indicates that overall water mass properties are a key variable structuring phytoplankton community composition. It may also reflect diachronous sampling of water masses across our dataset (see Figure 5C), with Atlantic and coastally-influenced waters better represented later in the productive season, while Barents Sea Water was sampled almost exclusively in spring.
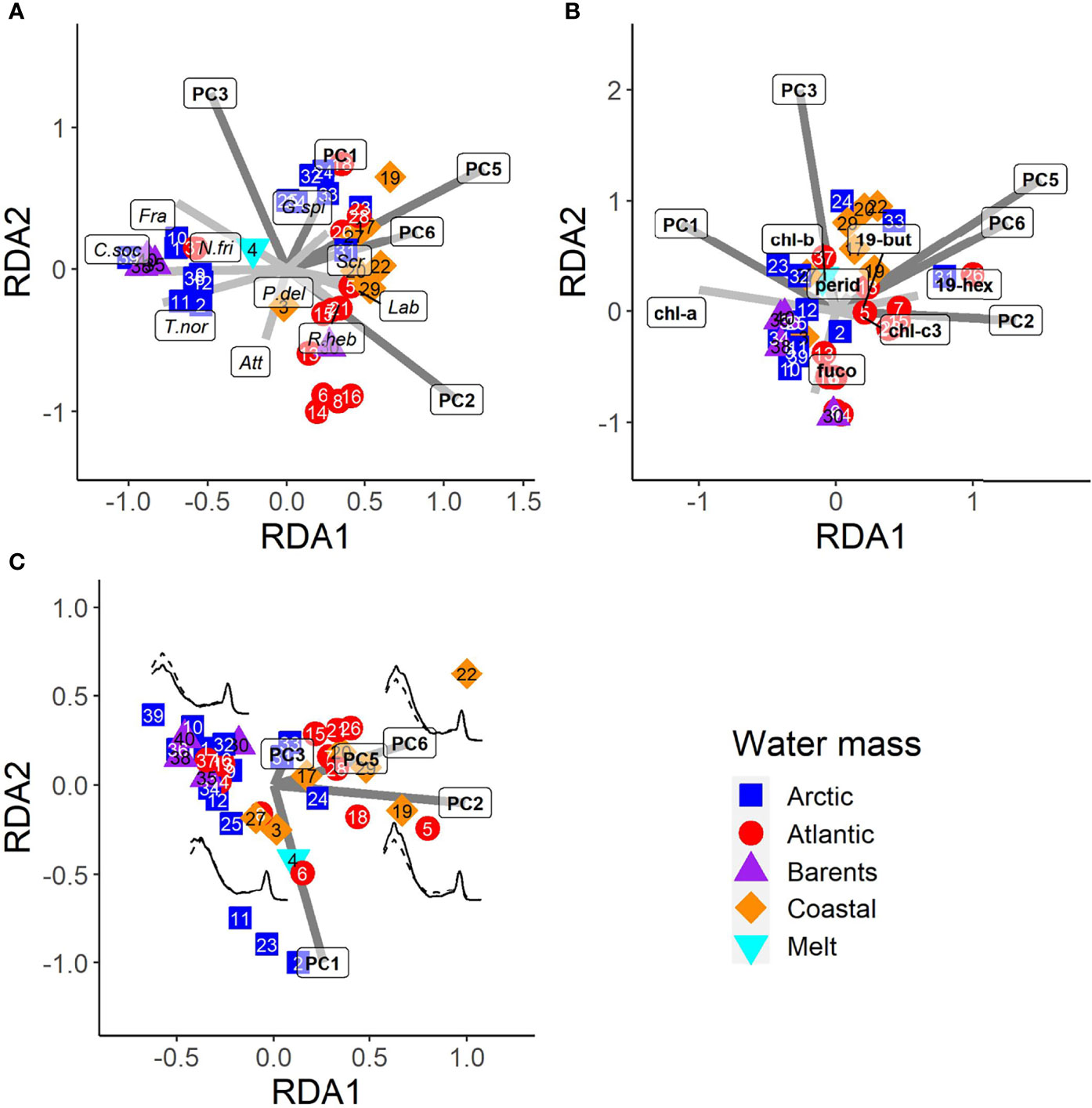
Figure 6 (A) RDA1-2 space for cell count structure, with salient environmental variables (PC1,2,3,5,6) and representative species vectors. ‘C.soc’, Chaetoceros socialis; ‘T.nor’, Thalassiosira nordenskioeldii; ‘N.fri’, Nitzschia frigida; ‘Fra’, Fragilariopsis; ‘Lab’, Laboea; ‘Att’, Attheya; ‘P.del’, Pseudo-nitzschia delicatissima; ‘Scr’, Scrippsiella; ‘G.spi’, Gyrodinium spirale; ‘R.heb’, Rhizosolenia hebetata. (B) RDA1-2 space for pigment structure, with salient environmental variables and pigment contribution vectors. ‘chl-a’, chlorophyll-a; ‘chl-b’, chlorophyll-b; ‘chl-c3’, chlorophyll-c3; ‘fuco’, fucoxanthin; ‘19-hex’, 19′-hexanoyloxyfucoxanthin; ‘19-but’, 19′-butanoyloxyfucoxanthin; ‘perid’, peridinin. (C) RDA1-2 space for absorption spectra, with salient environmental variables and representative absorption spectra (400-750 nm), normalised to absorption at 676 nm. A dashed line represents the mean absorption spectrum across all samples. Samples are identified by unique numbers.
The RDA triplot for cell counts (Figure 6A) shows that Arctic and Atlantic-influenced water masses are separated along RDA2. PC1 and 3, which represent Arctic influence and potentially trophic status, increase along RDA2. Hence, structure in the cell count dataset reveals that Arctic and Atlantic influences both coincide to determine overall community composition, in the context of seasonal succession. Example genera and species, with known environmental associations, are projected onto this space. Their distribution in the plot-space is broadly compatible with the network of relationships reported in the wider literature. Sympagic specialists, such as Nitzschia frigida and Fragilariopsis spp. [described as sympagic-neritic by Rat’kova and Wassmann (2002)], are clearly associated with Arctic and melt waters. The spring-bloom (samples 35,36,38,37,40), represented in our dataset pre-eminently by Arctic and Barents Sea water masses, is dominated by cosmopolitan and Arctic-boreal species such as Chaetoceros socialis and Thalassiosira nordenskioeldii, also consistent with Rat’kova and Wassmann (2002) and Degerlund and Eilertsen (2010). Atlantic waters are, in general, typified by nanophytoplankton. Sympagic-neritic diatoms such as Attheya spp. are abundant in the earlier Atlantic samples (samples 6,8,13,14,16), but are rapidly replaced by pelagic specialists such as Pseudo-nitzschia delicatissima and Rhizosolenia hebetata. These marine specialists are aligned with PC2, indicating that they are especially indicative of Atlantic influence. Atlantic samples from later in summer (samples 18,26,28) are associated with dinoflagellates. Waters classed as coastally-influenced have shallow stratification depths, and tend to be depleted in nutrients. These waters (samples 20, 22, 29) are associated with higher abundances of mixotrophic ciliates and dinoflagellates, such as Laboea spp., and Scrippsiella spp. (Jeong et al., 2005). Neritic dinoflagellate species of the genus Gyrodinium spp. [capable of heterotrophy (Tillmann, 2004)] tend to be more typical of late summer samples, in coastally-influenced, Atlantic and Arctic samples.
We note that many of the species that appear to drive structure in the cell counts dataset are diatoms, and that diatom-dominance is a major factor underlying dependency of cell count structure on environmental variables (Figure 4). A sizeable portion of the dependency of phytoplankton community structure on environmental variation could therefore be driven by abundant and ecologically-specialist diatom species. In order to test this, we prepared a version of the cell count dataset in which diatom taxa are omitted, and re-performed analyses to explore whether the environmental dependencies that are revealed are significantly changed. 36% of variance in the cell count dataset are constrained by environmental variance when diatoms are removed, which is lower than the 42% constraint when diatoms are present. Pure effect tests reveal that PC1, PC2, PC3, and PC5 significantly explain structure in the data (at p < 0.05). Term-wise effect statistics produce a coherent result, with PC1, PC2, PC3 and PC5 reported as significant, with PC6 sub-significant (p = 0.09). An RDA triplot for cell count structure, once diatoms are removed, is presented in the supplement (Figure S5). Compared to Figure 6A, the spring-bloom samples are not clearly distinguished from other Arctic water mass samples, and there is no longer a clear separation of samples originating from Atlantic and coastally-influenced water masses. Diatom taxa may therefore convey useful information that distinguishes different water masses.
An RDA triplot derived from pigment ratios (Figure 6B) provides a similar synopsis; RDA1 separates Atlantic and Arctic-influenced samples, and is aligned with PC2, 5 and 6. PC1 possesses a smaller component along RDA2, compared to the cell counts triplot. Hence, separation of samples by water mass is not as clear in this space as it is for cell counts. In general, spring samples tend to be associated with high contributions of chlorophyll-a and low contributions of accessory pigments, with the exception of fucoxanthin. This is consistent with early dominance by diatoms, especially in Arctic-influenced waters, reproducing a previous observation by Fragoso et al. (2017) in the Labrador Sea. Some Atlantic and coastal samples, occupying their own Attheya-rich space in the cell counts triplot, plot much more closely to the spring-bloom samples in the pigment triplot (samples 3, 6, 8, 13, 14, 30). Low contributions of fucoxanthin distinguish most coastally-influenced samples and some later summer Arctic samples (samples 24 and 33). Coastally-influenced samples appear to be associated with higher PC3 scores, which is surprising given that these waters are frequently oligotrophic. Rather than varying in response to PC3, these samples’ projection may be controlled by their low fucoxanthin content. This interpretation is consistent with the observation that PC3 does not significantly structure pigment inventories (Table 2). Variation in fucoxanthin is almost orthogonal to PC2, but is well-aligned with PC5 and 6, indicating that this proxy of diatom presence is more strongly related to seasonal succession than water mass. Accessory pigments such as chlorophyll-b, chlorophyll-c3, 19′-butanoyloxyfucoxanthin, 19′-hexanoyloxyfucoxanthin and peridinin, tend to plot near the origin and are hence only weakly indicative of different water masses or community compositions by themselves. The total sum of these accessory pigments appears to be a faithful proxy of Atlantic influence, although this is potentially a simple reflection of low inventories of accessory pigmentation in Arctic waters; indeed, vectors for PC1 and 2 are almost diametrically opposed. This co-linearity explains why PC1 is not identified as a significant explanatory vector in Table 2. In order to account for the effects of small inventories of accessory pigmentation (which could result in these pigments explaining only a small portion of variance even if they have strong environmental dependencies) we perform RDA on a pigment dataset normalize by variance, instead of expressed as ratios (see Figure S6). In this ordination, 19′-hexanoyloxyfucoxanthin, peridinin and chlorophyll-c3 are much more salient vectors, aligned with PC5, while chlorophyll-b is opposed to PC2 and more aligned with PC1. The alignment of peridinin with PC5 is consistent with a more substantial population of dinoflagellates emerging later in the productive season. 19′-hexanoyloxyfucoxanthin and chlorophyll-c3 are likely both indicative of haptophytes. Chlorophyll-c3 is not strongly aligned with any specific axis of environmental variation (see Figures 6B and S6). This could result from the occurrence of Phaeocystis blooms, which are rich in chlorophyll-c3, in multiple hydrographic settings. We hypothesise that chlorophyll-b may potentially be associated with prasinophytes (Fragoso et al., 2017).
The RDA triplot for absorption spectra (Figure 6C) is also dominated by an alignment of PC2, 5 and 6 along RDA1, separating Arctic and Atlantic-influenced samples. Fucoxanthin and Attheya-rich Atlantic samples typically cluster with Arctic-influenced spring-bloom samples, similarly to the pigment triplot. This overall structure is dominated by a spectral gradient in the packaging effect– a feature known to strongly structure variety in phytoplankton absorption spectra in the western Barents Sea (Liu et al., 2019). Representative absorption spectra from the spring-bloom are relatively flat, especially at blue wavelengths, even though they are rich in chlorophyll-a and fucoxanthin, while samples with high RDA1 scores have strong blue absorption. This is consistent with relatively large diatom cells, colonies and other microphytoplankton dominating the spring-bloom and Arctic waters, while nanophytoplankton or marine diatoms that are less likely to form colonial structures are more typical of Atlantic and coastally-influenced waters. Coastally-influenced samples do not clearly cluster in their own space, as in cell count and pigment triplots, perhaps because some distinctive pigment species (e.g. fucoxanthin, peridinin) possess similar absorption spectra (Chase et al., 2013). RDA2 appears to distinguish a spectrally distinct subset of Arctic samples (samples 2, 11, 23), with heightened absorption in the very far blue, and a reduced shoulder in absorption between 450 and 500 nm. Samples 2 and 23 possess unusually high antheraxanthin and zeaxanthin, but there is no single measured pigment species that appears to adequately explain their spectral signature. Samples 2, 11 and 23 are all distinguished by unusually high counts of Gyrodinium spp. It is known some dinoflagellates, including species of Gyrodinium, produce mycosporine-like amino acids that absorb strongly in the near-UV (Vernet and Whitehead, 1996; Klisch et al., 2001, Klisch and Häder, 2002), often as a protective response to UV-irradiation. While these compounds are water-soluble when exuded from cells (Vernet and Whitehead, 1996), particle-associated mycosporine-like amino acids retained inside cells could explain the strong near-UV absorption measured in samples 2, 11 and 23. We note that Klisch et al. (2001) was able to extract mycosporine-like amino acids from cell culture in 100% methanol, which means that the presence of mycosporine-like amino acids should result in a clear signal in our absorption spectra measurements. Mycosporine-like amino acids were not measured in our HPLC analysis, explaining why samples 2, 11 and 23 are not distinctive in the pigment triplot (Figure 6B). The Arctic nature of these samples means that PC1 is strongly aligned with RDA2, but does not significantly explain structure in the absorption spectral dataset as a whole (Table 2).
3.6 Relationship of Environmental Dependencies to Overall Biomass Gradients, Seasonal Succession and Atlantic Influence
It is important to explore the influence of total biomass variation, which may be accompanied by changes in phytoplankton community structure. We calculated total biomass by summing estimated biomasses of enumerated cells associated with each sample, and have regressed RDA1 and RDA2 site scores against this vector. RDA1 for the cell counts analysis is significantly related to biomass, but the goodness of the fit is low (p = 0.03 and adjusted R2 = 0.09). RDA2 for the cell counts analysis is not significantly related to biomass (p = 0.32 and adjusted R2 < 0.01). This is consistent with a general progression of time-of-sampling along RDA1, with higher biomasses generally but not exclusively sampled in the spring-bloom (which is clearly distinguished in the triplot for this analysis: Figure 6A). RDA1 for the pigment ratios analysis is also significantly related to biomass (p = 0.03 and adjusted R2 = 0.09). RDA2 for the pigment ratios analysis is not significantly related to biomass (p = 0.19 and adjusted R2 = 0.02). RDA1 for the absorption spectra analysis is the most strongly related to biomass (p < 0.01 and adjusted R2 = 0.16), and is clearly associated with a gradient of declining diatom dominance and increasing ciliates. We note that an adjusted R2 of 0.16 is still not indicative of a tight fit. RDA2 for the absorption spectra analysis is unrelated to biomass (p ≈ 1 and adjusted R2 = −0.03). Overall, this pattern is consistent with biomass possessing a significant relationship to gross dynamics of phytoplankton succession. We hypothesize that this may be controlled by available nutrients to fuel phytoplankton growth. However, overall biomass is not the sole variable structuring the relationships between phytoplankton community structure and environmental variability.
In order to probe the influence of seasonal succession further, we constructed linear models of the form Y = aX1 + bX2 + c, where Y is the date of sample collection and X1 and X2 are the RDA1 and RDA2 scores for redundancy analyses derived from cell counts, pigments and absorption spectra. If seasonal succession underpins variation in phytoplankton community compositions we should expect time-of-sampling to depend strongly on the RDA site scores. The results for the cell counts dataset confirm this (p < 0.01 for the dependency on RDA1, and p < 0.01 for RDA2, with an adjusted R2 = 0.72). The results for the pigment dataset also show a significant relationship with time-of-sampling, but it is only significant for RDA1 and the overall goodness of fit is lower, which suggests that there are modes of variance in the cell count data that capture seasonal succession more fully than pigment data. (p < 0.01 for the dependency on RDA1, 0.34 for RDA2, and adjusted R2 = 0.24). A similar result is found for the redundancy analysis derived from absorption spectra. (p < 0.01 for the dependency on RDA1, 0.68 for RDA2, and adjusted R2 = 0.32). This shows that, while cell count data possess the clearest relationship with the time-of-sampling, pigment and absorption spectra datasets still retrieve a significant relationship. Seasonal succession is one of the most important features of these data’s relationship to environmental variability.
In order to explore the role of Atlantic influence, and whether its effects in structuring the explained variance in the phytoplankton community compositional datasets is significant, we performed MANOVA analyses. We divided samples into ‘Atlantic’ and ‘Not Atlantic’ groups, in adherence with their water mass properties, and sought to discover whether this categorize was accompanied by significantly different scores along the RDA axes in redundancy analyses. Atlantic samples had significantly different means along RDA1 and RDA2 in redundancy analysis derived from cell count data (p = 0.03, < 0.01). Atlantic samples had significantly different means along RDA1 and sub-significant different means along RDA2 in redundancy analysis derived from pigment ratio data (p < 0.01, 0.07).
There was not a significant difference in means along RDA1 and RDA2 in redundancy analysis derived from absorption spectra (p = 0.13, 0.60). However, treatment of Coastal Water as an independent group, or grouping it with Atlantic Water, resulted in a significant difference in means across RDA1 (p < 0.01).
It is possible distinct Atlantic influence results in part from a diachronous collection of samples across water masses. Atlantic samples are more likely to have been procured later in the productive season. We attempted to separate seasonality and Atlantic influence by fitting linear models between RDA axes, that depend significantly on time-of-sampling, and extracted their residuals. We then re-performed MANOVA, using these residuals axes, to check whether Atlantic samples continue to possess distinct means. The MANOVA on residual cell count RDA axes revealed significant differences in means between Atlantic and Not Atlantic samples along residual RDA1 and residual RDA2 (p < 0.01 and p < 0.01). MANOVA performed on residual pigment ratio RDA1 and the original RDA2 axis reveals that there is still a significant separation of Atlantic and Not Atlantic samples across RDA1 (p < 0.01 and p = 0.07). For absorption spectra, Atlantic samples were only significantly different across residual RDA1 and only when Coastal samples are categorized as their own group (p < 0.01) or when Coastal samples are grouped with Atlantic samples (p < 0.01). This indicates that Atlantic influence significantly drives the relationship between phytoplankton community structure and environmental conditions, even when an attempt is made to remove effects attributable to seasonality.
3.7 Influence of Photoacclimation on Pigment Ratio Data Structure
There is good evidence that photoacclimation results in significant variations in phytoplankton pigment complements in the Barents Sea (Johnsen et al., 2018; Kauko et al., 2019) and that photoacclimation processes may significantly influence phytoplankton absorption spectra.
We tested whether variance in pigment ratios explains variance in cell count structure by performing RDA in which cell counts were the dependent variable matrix and pigment ratios the explanatory variable matrix. Pigment ratios explained 81% of variance in cell count structure, which suggests that pigment ratios are a faithful representation of at least some major portion of variance in the cell count data. We also performed variance-partitioned RDA with the ‘varpart’ function of the vegan R package (Oksanen et al., 2019), to determine the portions of cell count variance explained by photoprotective [α-carotene, β-carotene, zeaxanthin, alloxanthin and diadinoxanthin (Chase et al., 2013)] and other pigment species. Photoprotective pigments explain 22% of variance, of which 9% is variance that is uniquely explained (cannot be explained by other pigment species). The remaining pigment species explain 69% of variance, of which 32% is uniquely explained. This shows that photoprotective and other pigments have substantial overlap in their explained variance. This is consistent with photoprotective pigments co-varying with other pigment species, such as those that are taxonomically indicative. This may result from different taxonomic groups of phytoplankton having different baseline complements of photoprotective pigment, and suggests that photoacclimation responses do not substantially undermine correspondence of pigment ratios to overall phytoplankton community structure.
4 Discussion
RDA results, derived from cell counts and absorption spectra, demonstrate that cosθ values for silicic acid and nitrate concentrations correlate strongly with diatom dominance. Diatom blooms routinely occur as r-strategists in eutrophic spring conditions in the Barents Sea (Båmstedt et al., 1991) and may be found in close association with the marginal ice zone into summer (Strass and Nöthig, 1996), an observation consistent with the geographic and seasonal distribution of diatom biomass across our samples (Figure 3). However, the absence of this pattern in RDA derived from pigments (Figure 4B) requires explanation. This could have resulted if variation in pigment concentrations was not indicative of the fractional mass dominance of diatoms. There is widespread attestation in the literature to the inference of diatoms from taxonomic markers such as fucoxanthin [e.g. Vidussi et al. (2001); Fragoso et al. (2017)]. However, this diagnostic pigment is not exclusive to diatoms [e.g. Roy et al. (2011); Latasa et al. (2017)], which may weaken capacity to infer their contribution to community composition. We also note that fucoxanthin occurred in high concentrations in some Atlantic-influenced samples that possessed marine specialist diatom genera, such as Pseudo-nitzschia, spp outside of the spring-bloom. Pigment ratios that indicated diatom presence therefore occurred in multiple different environmental settings, which resulted in a less resolved picture of the environmental controls on community structure than that which is provided by cell count data.
This discussion helps explain why a larger number of axes of environmental variance significantly explain structure in the cell counts dataset, compared to pigments or absorption spectra. Ecological equivalence, vis à vis Mutshinda et al. Mutshinda et al. (2016), does not substantially undermine the capacity of the cell counts dataset to reveal environmental dependencies, and our triplot for cell counts (Figure 6A) indicates that a number of highly abundant species, with strong environmental preferences [e.g. sympagic-neritic species (Syvertsen, 1991)], structure variance in this dataset (Rat’kova and Wassmann, 2002). However, such ‘indicator’ species do not always possess unique pigment complements or absorptive spectral properties– as demonstrated by the clustering of distinctive Atlantic diatom communities with the Arctic spring-bloom in Figures 6B, C. Hence, there are axes of variance in phytoplankton community composition that simply cannot be captured in pigment or absorptive spectral data. This hypothesis is further reinforced by a subsetted RDA of cell counts that excludes diatom taxa (see Figure S5). While the overall variance structure and environmental dependencies are similar to Figure 6A, specificity is lost that distinguishes spring-bloom communities from Arctic Water samples, and diatom-dominated Atlantic Water communities from other Atlantic or Coastal Water samples.
Despite differences in the environmental dependencies revealed by cell counts, pigments and absorption spectra, all datasets coincide to reveal a seasonal succession from diatom-dominated spring-blooms, towards communities dominated by nanophytoplankton, and in which microphytoplankton are increasingly represented by mixotrophic dinoflagellates and ciliates. This succession conforms well with established descriptions of phytoplankton phenology and trophic cascades in this region (Wassmann et al., 2006). This interpretation is further reinforced by significant linear relationships between time-of-sampling and major axes of variation in RDA across all datasets. This demonstrates that seasonal succession underpins major modes of variation in phytoplankton community composition. In particular, gradients in the relationship between community composition and the cosθ index for σθ [which we interpret as indicative of deeper-sourced waters that form as a result of turbulent mixing and convective upwelling in winter (Loeng, 1991)] decline over the productive season. This is consistent with established hypotheses that relate modes of phytoplankton succession in seasonal seas with ocean turbulence and the provision of new nutrients (Margalef, 1978). This gradient is also compatible with increasing cosθ index values for ammonium concentrations in the cell count dataset over time (Figure S3). This may reflect an increase in smaller phytoplankton species more likely to exploit regenerated forms of nitrogen (Kristiansen and Farbrot, 1991). We note that abrupt changes in the cosθ index for ammonium concentration (Figure S3) reflect changes in water mass. Atlantic waters are much more likely to exhibit the emergence of summer phytoplankton communities that interact with this resource. Concomitant with this, MANOVA analyses generally support distinct patterns of community composition in Atlantic Water samples, and absorption spectral properties are indicative of smaller cell sizes (see Figure S4). This interpretation is compatible with literature reports that show there are different summer nutrient cycling pathways in Atlantic and Arctic waters. There is weak surface retention of particulate organic carbon in Arctic water masses in the western Barents Sea (Reigstad et al., 2008). In Atlantic waters, by contrast, coprophagous copepods remineralise faecal pellets, retaining 90% of particulate organic carbon within the top 50 m of the water column (Riser et al., 2002; Riser et al., 2007), making available a pool of regenerated nitrogen. This is sympathetic with previous research that suggests grazing by calanoid copepods is a significant control on phytoplankton dynamics in the Norwegian Sea, which is adjacent to our field area (Bathmann et al., 1990). Hence, while they were not enumerated directly, the effects of mesozooplankton populations may have left a clear fingerprint on our phytoplankton community composition datasets.
Rat’kova and Wassmann (2002) notes that the spring-bloom develops independently in Arctic and Atlantic water masses, with distinct species successions. This description differs from that of Degerlund and Eilertsen (2010), which notes a ‘striking similarity’ in community composition across spring-bloom communities in different water masses. The difference between these studies is perhaps accommodated by their different definitions of spring-bloom. While Rat’kova and Wassmann (2002) describes the Atlantic water mass as blooming later than Arctic waters, Degerlund and Eilertsen (2010) strictly defines the spring-bloom as the phytoplankton communities sampled between March and May. Our analysis of cell counts is most coherent with the description of Rat’kova and Wassmann (2002), but distinct successions in different water masses are less apparent in the pigment and absorption spectral datasets. We note that our diachronous sampling of the Atlantic water mass means we cannot confidently comment on the definition of spring-bloom employed by Degerlund and Eilertsen (2010). We found that Atlantic Water samples formed distinct clusters in RDA output, even after we attempted to remove the influence of seasonal succession, which plays a strong role structuring the RDA results. This could imply that phytoplankton communities in Atlantic Water follow an independent trajectory of seasonal succession, compared to other phytoplankton communities. However, we caution that we cannot comment confidently without a greater number of spring samples from the Atlantic water mass. We observe that a single spring Atlantic sample (sample 37) clusters with the Arctic samples in the cell count triplot (Figure 6A). However, a single sample is insufficient to draw conclusions. We are also aware that our analytical method (Redundancy Analysis) is designed to explore variance explained by environmental differences, unlike the method of Degerlund and Eilertsen (2010) (Principal Component Analysis), which considers variance within the community compositional dataset itself. Our analytical method could therefore prioritize small sources of variance in the cell counts dataset, if they are well-explained by environmental difference, whereas this structure would not be salient in the analysis of Degerlund and Eilertsen (2010).
Rat’kova and Wassmann (2002) noted that species diversity reached its maximum after mid-summer, that species which had previously been associated with specific water masses began to be distributed more widely, and that dinoflagellates increased in frequency. These observations are directly supported by the cell counts triplot in Figure 6A, which shows that later Arctic, coastal and Atlantic samples converge in a space strongly associated with dinoflagellate genera such as Gyrodinium spp. An RDA that excluded diatom taxa (Figure S5) provides additional nuance, because ecological differences between different dinoflagellate species become clearer in this analysis. Specific ecological associations of Mesodinium spp., Gyrodinium fusiforme and Peridinium spp. to ice-associated or neritic environments structure the leading axes of this ordination. Ordering matrices of environmental associations by time (Figures 3, S3) shows that overall dinoflagellate biomass never becomes dominant in any sample and does not follow a consorted trajectory across the productive season. Therefore, there is considerable variety in environmental preferences within dinoflagellates.
Overall, pigment and absorption spectral data, despite providing less insight into environmental dependencies than cell counts, still illuminate processes of seasonal succession and Atlantic influence.
However, while differences caused by variety in community composition are the dominant driver of variance in pigmentation and bio-optical patterns in our samples, we caution that photoacclimation may still alter pigment packaging or pigment ratios. The effects of photoacclimation must therefore be carefully considered. We also note that distinct Atlantic influence was most readily detected in cell count data, owing to the occurrence of specialist diatom taxa. Atlantic assemblages can be difficult to distinguish from Coastal Water assemblages in absorption spectra (Figure 6C). Coastal Water was typically encountered near the Svalbard archipelago in our dataset (Figure 1). These waters were typified by species such as Scrippsiella spp. and Pseudo-nitzschia delicitassima. Atlantic assemblages, by contrast, were distinguished by Attheya spp. and Rhizosolenia hebetata. This suggests a limited collection of cell count data could be used to clarify ambiguities in bio-optical structure.
Changing phenology, in response to a changing Arctic climate, is a major subject of contemporary research (Ardyna et al., 2014; Crawford et al., 2020; Dalpadado et al., 2020; Dong et al., 2020), as is the significance of increasing Atlantic influence in the Barents Sea (Rat’kova and Wassmann, 2002; Lalande et al., 2013; Nöthig et al., 2015; Engel et al., 2017; Oziel et al., 2020). Pigment and absorption spectral datasets therefore have the capacity to provide researchers with useful information studying some of the most prescient research questions in the Eurasian Arctic. In particular, recent demonstrations that absorption spectral measurements can be produced at scale in underway systems (Liu et al., 2019), demonstrate that bio-optical observations will form an integral component of future research. Where signals of Atlantification and seasonal succession are not easily separated, for example when marine-specialist diatom species are abundant, researchers may find it useful to determine the relative abundance of a small number of indicator species.
Our findings have implications for researchers exploiting remotely-sensed ocean-colour to monitor phytoplankton environmental dependencies. Pigments and absorption spectra have clear relationships to ocean-colour (Sathyendranath et al., 2004; Jackson et al., 2010; Sathyendranath et al., 2014). Researchers should therefore anticipate that structure in ocean-colour data should more closely map onto environmental dependencies evident from pigment or bio-optical data than microscopic cell counts. This may influence the selection of appropriate data types for the definition of simplified schemes of phytoplankton functional types derived from community composition, and the datasets used for in-situ validation.
5 Conclusion
The network of relationships between dominant phytoplankton taxa and environmental conditions in the Barents Sea is pronounced and its major features can be retrieved with a variety of different observational methods. The dependencies we find, of phytoplankton community composition upon hydrography and seasonality, are consistent with broad patterns of water mass structure and distribution previously reported to control phytoplankton succession in the Barents Sea. Detailed cell counts, resolved to genus or species level, reveal a greater number of significant environmental dependencies than other datasets, as a result of a small number of highly abundant indicator species with strong environmental preferences. Researchers can therefore identify sentinels of ecosystem change by monitoring shifts in cell count data for a small number of key species with strong environmental dependencies. While they provide a less nuanced summary of environmental dependencies in comparison to cell counts, pigment and absorption spectral data provide useful insight into seasonal succession and Atlantic influence, two subjects of major research interest in the Barents Sea. This is because both pigments and absorption spectra capture broad compositional changes in the fractional contribution of different phytoplankton assemblages (e.g. diatom or nanophytoplankton dominance) to community biomass. We suggest that, as the collection of pigment and absorption spectral data require less labor than the production of comprehensive microscopic cell counts, researchers can employ these techniques to produce large-scale records of phytoplankton community compositional change in the western Barents Sea and probe its functional significance. Specifically, these datasets are appropriate choices to study possible regime-shifts in gross phytoplankton community structure, and to compare observational datasets with model simulations, in which phytoplankton assemblages such as diatoms and nanophytoplankton are routinely represented. Furthermore, we suggest that pigment and absorption spectral datasets might be augmented by combining them with microscopic observation of key indicator species for specific applications, depending on research goals.
Data Availability Statement
Minimal datasets and code required to reproduce our study are available in the following GitHub repository: https://github.com/aorkney/Western-Barents-Sea. Bio-optical datasets are available via the British Oceanographic Data Centre: (Orkney and Bouman, 2019b). Phytoplankton absorption spectra, derived from measurements of absorption from phytoplankton samples taken in the Barents Sea during cruise JR16006: British Oceanographic Data Centre, National Oceanography Centre, NERC, UK: https://doi.org/10.5285/97daa7ea-8792-6cff-e053-6c86abc0dd46 (Orkney and Bouman, 2019a). Phytoplankton absorption spectra, derived from measurements of absorption from phytoplankton samples taken in the Barents Sea during cruise HH180423: British Oceanographic Data Centre, National Oceanography Centre, NERC, UK: https://doi.org/10.5285/982b6da2-7e11-060a-e053-6c86abc09389 (Orkney and Bouman, 2019c). Phytoplankton absorption spectra, derived from measurements of absorption from phytoplankton samples taken in the Barents Sea during cruise JR17006: British Oceanographic Data Centre, National Oceanography Centre, NERC, UK: https://doi.org/10.5285/982b6da2-7e12-060a-e053-6c86abc09389. Cell count datasets are available at: (Mitchell et al., 2021b). Phytoplankton enumeration and biomass calculations from samples collected in the Barents Sea during 2017-2018 (Version 1.0) [Data set]: UK Polar Data Centre, Natural Environment Research Council, UK Research & Innovation, https://doi.org/10.5285/69ae1fa2-cf1d-44da-8415-d9cc512c0256. Flow-cytometry datasets are available at: (Mitchell et al., 2021a). Flow cytometry analysis of water samples for bacterial and Pico-plankton enumeration, samples collected in the Barents Sea during 2017-2018 (Version 1.0) [Data set]: UK Polar Data Centre, Natural Environment Research Council, UK Research & Innovation: https://doi.org/10.5285/2182fd8d-0a0d-4f56-9bf9-4dffaa67ff4b. Hydrographic data are available via the British Oceanographic Data Centre: (Dumont et al., 2019). CTD data from NERC Changing Arctic Ocean Cruise JR16006 on the RRS James Clark Ross, June-August 2017: British oceanogtaphic Data Centre, National Oceanography Centre, NERC, UK: https://doi.org/10.5285/89a3a6b8-7223-0b9c-e053-6c86abc0f15d. Nutrient assay data are available via the British Oceanographic Data Centre: (Brand et al., 2019b). Dissolved nutrient samples collected in the Barents Sea as part of the Changing Arctic Ocean programme for the Arctic PRIZE and ARISE projects during RRS James Clark Ross cruise JR16006: British Oceanographic Data Centre, National Oceanography Centre, NERC, UK: https://doi.org/10.5285/b4c1537e-c729-6463-e053-6c86abc0c7de (Brand et al., 2019a). Dissolved nutrient samples collected in the Barents Sea as part of the Changing Arctic Ocean programme during cruise JR17006, June-July 2018: British Oceanographic Data Centre, National Oceanography Centre, NERC, UK: https://doi.org/10.5285/b62f2d5d-1f3f-0c2c-e053-6c86abc0265d.
Author Contributions
AO acquired bio-optical and pigment samples, drafted the manuscript, performed analysis and was instrumental in the study’s conception. HAB supervised in the study’s conception and undertaking, providing key conceptual input in analysis, and assisted in acquisition of bio-optical and pigment datasets. EM acquired cell counts and flow-cytometric data. KD provided expert conceptual input on cell counts and flow-cytometric datasets and analytical methods used in the existing literature to relate phytoplankton community structure to environmental variation. SFH provided critical input on nutrient data and biogeochemical processes and contributed substantially to drafting the manuscript. All authors contributed to the text of the manuscript. All authors have read and approved the final manuscript.
Funding
HAB and AO’s work was funded by the UK Natural Environment Research Council (NERC) Arctic PRIZE project, grant number NE/P006507/1. EM and KD’s work was funded by Arctic PRIZE, grant number NE/P006302/1. SFH’s work was funded by the UK Natural Environment Research Council (NERC) Arctic PRIZE project, grant number NE/P006086/1.
Conflict of Interest
The authors declare that the research was conducted in the absence of any commercial or financial relationships that could be construed as a potential conflict of interest.
Publisher’s Note
All claims expressed in this article are solely those of the authors and do not necessarily represent those of their affiliated organizations, or those of the publisher, the editors and the reviewers. Any product that may be evaluated in this article, or claim that may be made by its manufacturer, is not guaranteed or endorsed by the publisher.
Acknowledgments
We acknowledge the dutiful assistance and congeniality of the crews of the RRS James Clark Ross and FF Helmer Hanssen, without which this research would not have been possible.
Supplementary Material
The Supplementary Material for this article can be found online at: https://www.frontiersin.org/articles/10.3389/fmars.2022.860773/full#supplementary-material
References
Ardyna M., Babin M., Gosselin M., Devred E., Rainville L., Tremblay J.-É. (2014). Recent Arctic Ocean Sea Ice Loss Triggers Novel Fall Phytoplankton Blooms. Geophys. Res. Lett. 41, 6207–6212. doi: 10.1002/2014GL061047
Ardyna M., Gosselin M., Michel C., Poulin M., Tremblay J.-É. (2011). Environmental Forcing of Phytoplankton Community Structure and Function in the Canadian High Arctic: Contrasting Oligotrophic and Eutrophic Regions. Mar. Ecol. Prog. Ser. 442, 37–57. doi: 10.3354/meps09378
Båmstedt U., Eilertsen H. C., Tande K. S., Slagstad D., Skjoldal H. R. (1991). Copepod Grazing and its Potential Impact on the Phytoplankton Development in the Barents Sea. Pol. Res. 10, 339–354. doi: 10.3402/polar.v10i2.6751
Barros M. P., Pedersén M., Colepicolo P., Snoeijs P. (2003). Self-Shading Protects Phytoplankton Communities Against H2O2-Induced Oxidative Damage. Aquat. Microbial. Ecol. 30 (3), 275–282. doi: 10.3354/ame030275
Barton B. I., Lenn Y.-D., Lique C. (2020). Observed Atlantification of the Barents Sea Causes the Polar Front to Limit the Expansion of Winter Sea Ice. J. Phys. Oceanog. 500, 18–0003. doi: 10.1175/JPO-D-18-0003.1
Bathmann U., Noji T., Von Bodungen B. (1990). Copepod Grazing Potential in Late Winter in the Norwegian Sea– A Factor in the Control of Spring Phytoplankton Growth? Mar. Ecol. Prog. Ser., 60, 225–233. doi: 10.3354/meps060225
Berge J., Johnsen G., Cohen J. H. (2020). Polar Night Marine Ecology: Life and Light in the Dead of Night Vol. 4 (Springer Nature). doi: 10.1007/978-3-030-33208-2
Brand T., Henley S., Mahaffey C., Crocket K., Norman L., Tuerena R. (2019a). Dissolved Nutrient Samples Collected in the Barents Sea as Part of the Changing Arctic Ocean Programme During Cruise JR17006 (Liverpool: British Oceanographic Data Centre). doi: 10.5285/b62f2d5d-1f3f-0c2c-e053-6c86abc0265d
Brand T., Norman L., Henley S., Mahaffey C., Tuerena R., Crocket K. (2019b). Dissolved Nutrient Samples Collected in the Barents Sea as Part of the Changing Arctic Ocean Programme for the Arctic PRIZE and ARISE Projects During RRS James Clark Ross Cruise JR16006 (Liverpool: British Oceanographic Data Centre). doi: 10.5285/b4c1537e-c729-6463-e053-6c86abc0c7de
Bricaud A., Babin M., Morel A., Claustre H. (1995). Variability in the Chlorophyll-Specific Absorption Coefficients of Natural Phytoplankton: Analysis and Parameterization. J. Geophys. Res.: Ocean. 100 (C7), 13321–13332. doi: 0.1029/95JC00463
Buesseler K. O., Boyd P. W., Black E. E., Siegel D. A. (2020). Metrics That Matter for Assessing the Ocean Biological Carbon Pump. Proc. Natl. Acad. Sci. 117, 9679–9687. doi: 10.1073/pnas.1918114117
Carpenter J. H. (1965). The Chesapeake Bay Institute Technique for the Winkler Dissolved Oxygen Method. Limnol. Oceanog. 10, 141–143. doi: 10.4319/lo.1965.10.1.0141
Chase A., Boss E., Zaneveld R., Bricaud A., Claustre H., Ras J., et al. (2013). Decomposition of In Situ Particulate Absorption Spectra. Methods Oceanog. 7, 110–124. doi: 10.1016/j.mio.2014.02.002
Crawford A. D., Krumhardt K. M., Lovenduski N. S., van Dijken G. L., Arrigo K. R. (2020). Summer High-Wind Events and Phytoplankton Productivity in the Arctic Ocean. J. Geophys. Res.: Ocean. 125, e2020JC016565. doi: 10.1029/2020JC016565
Dalpadado P., Arrigo K. R., van Dijken G. L., Skjoldal H. R., Bagøien E., Dolgov A. V., et al. (2020). Climate Effects on Temporal and Spatial Dynamics of Phytoplankton and Zooplankton in the Barents Sea. Prog. Oceanog. 185, 102320. doi: 10.1016/j.pocean.2020.102320
Davidson K., Gilpin L. C., Pete R., Brennan D., McNeill S., Moschonas G., et al. (2013). Phytoplankton and Bacterial Distribution and Productivity on and Around Jones Bank in the Celtic Sea. Prog. Oceanog. 117, 48–63. doi: 10.1016/j.pocean.2013.04.001
Degerlund M., Eilertsen H. C. (2010). Main Species Characteristics of Phytoplankton Spring Blooms in NE Atlantic and Arctic Waters (68–80 N). Estua. Coast. 33, 242–269. doi: 10.1007/s12237-009-9167-7
Dong K., Kvile K.Ø., Stenseth N. C., Stige L. C. (2020). Associations Among Temperature, Sea Ice and Phytoplankton Bloom Dynamics in the Barents Sea. Mar. Ecol. Prog. Ser. 635, 25–36. doi: 10.3354/meps13218
Drinkwater K. F. (2006). The Regime Shift of the 1920s and 1930s in the North Atlantic. Prog. Oceanog. 68, 134–151. doi: 10.1016/j.pocean.2006.02.011
Dumont E., Brand T., Hopkins J. (2019). CTD Data From NERC Changing Arctic Ocean Cruise JR16006 on the RRS James Clark Ross (Liverpool: British Oceanographic Data Centre). doi: 10.5285/89a3a6b8-7223-0b9c-e053-6c86abc0f15d
Dybwad C., Assmy P., Olsen L. M., Peeken I., Nikolopoulos A., Krumpen T., et al. (2020). Carbon Export in the Seasonal Sea Ice Zone North of Svalbard From Winter to Late-Summer. Front. Mar. Sci. 7. doi: 10.3389/fmars.2020.525800
Egge J., Aksnes D. (1992). Silicate as Regulating Nutrient in Phytoplankton Competition. Mar. Ecol. Prog. Series. 83, 281–289. doi: 10.3354/meps083281
Engel A., Piontek J., Metfies K., Endres S., Sprong P., Peeken I., et al. (2017). Inter-Annual Variability of Transparent Exopolymer Particles in the Arctic Ocean Reveals High Sensitivity to Ecosystem Changes. Sci. Rep. 7, 4129. doi: 10.1038/s41598-017-04106-9
Falkowski P. G., Barber R. T., Smetacek V. (1998). Biogeochemical Controls and Feedbacks on Ocean Primary Production. Science 281, 200–206. doi: 10.1126/science.281.5374.200
Field C. B., Behrenfeld M. J., Randerson J. T., Falkowski P. (1998). Primary Production of the Biosphere: Integrating Terrestrial and Oceanic Components. Science 281, 237–240. doi: 10.1126/science.281.5374.200
Fragoso G. M., Poulton A. J., Yashayaev I. M., Head E. J., Purdie D. A. (2017). Spring Phytoplankton Communities of the Labrador Se–2014): Pigment Signatures, Photophysiology and Elemental Ratios. Biogeosciences 14, 1235–1259. doi: 10.5194/bg-14-1235-2017
Galí M., Devred E., Babin M., Levasseur M. (2019). Decadal Increase in Arctic Dimethylsulfide Emission. Proc. Natl. Acad. Sci. 116, 19311–19317. doi: 10.1073/pnas.1904378116
Galí M., Lizotte M., Kieber D. J., Randelhoff A., Hussherr R., Xue L., et al. (2021). DMS Emissions From the Arctic Marginal Ice Zone. Elementa.: Sci. Anthropo. 9, 113. doi: 10.1525/elementa.2020.00113
Hoepffner N., Sathyendranath S. (1992). Bio-Optical Characteristics of Coastal Waters: Absorption Spectra of Phytoplankton and Pigment Distribution in the Western North Atlantic. Limnol. Oceanog. 37, 1660–1679. doi: 10.4319/lo.1992.37.8.1660
Hoppenrath M., Elbrächter M., Drebes G. (2009). Marine Phytoplankton (Stuttgart: Schweizerbart Science Publishers). Available at: https://www.schweizerbart.de/publications/detail/isbn/9783510613922/Marine.
Ingvaldsen R., Assman K. M., Primicerio R., Fossheim M., Polyakov I. V., Dolgov A. V. (2021). Physical Manifestations and Ecological Implications of Arctic Atlantification. Nat. Rev. Earth Environ., 2, 1–16. doi: 10.1038/s43017-021-00228-x
Jackson T., Bouman H. A., Sathyendranath S., Devred E. (2010). Regional-Scale Changes in Diatom Distribution in the Humboldt Upwelling System as Revealed by Remote Sensing: Implications for Fisheries. ICES. J. Mar. Sci. 68, 729–736. doi: 10.1093/icesjms/fsq181
Janišová M., Hrivnák R., Gömöry D., Ujházy K., Valachovič M., Gömöryová E., et al. (2007). “Changes in Understorey Vegetation After Norway Spruce Colonization of an Abandoned Grassland,” in Annales Botanici Fennici, Helsinki: Finnish Zoological and Botanical Publishing Board 44, 256–266.
Jeffrey S. W., Wright S. W., Zapata M. (2011). Microalgal Classes and Their Signature Pigments (Cambridge: Cambridge University Press). doi: 10.1017/CBO9780511732263.004
Jeong H. J., Du Yoo Y., Park J. Y., Song J. Y., Kim S. T., Lee S. H., et al. (2005). Feeding by Phototrophic Red-Tide Dinoflagellates: Five Species Newly Revealed and Six Species Previously Known to be Mixotrophic. Aquat. Microbial. Ecol. 40, 133–150. doi: 10.3354/ame040133
Jiang L., Schofield O. M., Falkowski P. G. (2005). Adaptive Evolution of Phytoplankton Cell Size. Am. Nat. 166, 496–505. doi: 10.1086/444442
Johnsen G., Norli M., Moline M., Robbins I., Von Quillfeldt C., Sørensen K., et al. (2018). The Advective Origin of an Under-Ice Spring Bloom in the Arctic Ocean Using Multiple Observational Platforms. Pol. Biol. 41 (6), 1197–1216. doi: 10.1007/s00300-018-2278-5
Johnsen G., Samset O., Granskog L., Sakshaug E. (1994). In Vivo Absorption Characteristics in 10 Classes of Bloom-Forming Phytoplankton: Taxonomic Characteristics and Responses to Photoadaptation by Means of Discriminant and HPLC Analysis. Mar. Ecol. Prog. Ser., 105, 149–157. doi: 10.3354/meps105149
Kauko H. M., Pavolv A. K., Johnsen G., Granskog M. A., Peeken I., Assmy P. (2019). Photoacclimation State of an Arctic Underice Phytoplankton Bloom. J. Geophys. Res.: Ocean. 124 (3), 1750–1762. doi: 10.1029/2018JC014777
Kelley D., Richards C. (2020). Oce: Analysis of Oceanographic Data, R package version 1.2-0. Available at: https://CRAN.R-project.org/package=oce
Kishino M., Takahashi M., Okami N., Ichimura S. (1985). Estimation of the Spectral Absorption Coefficients of Phytoplankton in the Sea. Bull. Mar. Sci. 37, 634–642.
Klisch M., Häder D.-P. (2002). Wavelength Dependence of Mycosporine-Like Amino Acid Synthesis in Gyrodinium Dorsum. J. Photochem. Photobio. B.: Biol. 66 (1), 60–66 . doi: 10.1016/S1011-1344(01)00276-7
Klisch M., Sinha R. P., Richter P. R., Häder D.-P. (2001). Mycosporine-Like Amino Acids (MAAs) Protect Against UV-B-Induced Damage in Gyrodinium Dorsum Kofoid. J. Plant Physiol. 158 (11), 1449–1454. doi: 10.1078/0176-1617-00552
Kristiansen S., Farbrot T. (1991). Nitrogen Uptake Rates in Phytoplankton and Ice Algae in the Barents Sea. Pol. Res. 10, 187–192. doi: 10.1111/j.1751-8369.1991.tb00644.x
Kwok R. (2018). Arctic Sea Ice Thickness, Volume, and Multiyear Ice Coverage: Losses and Coupled Variability, (1958–2018). Environ. Res. Lett. 13, 105005. doi: 10.1088/1748-9326/aae3ec
Lalande C., Bauerfeind E., Nöthig E.-M., Beszczynska-Möller A. (2013). Impact of a Warm Anomaly on Export Fluxes of Biogenic Matter in the Eastern Fram Strait. Prog. Oceanog. 109, 70–77. doi: 10.1016/j.pocean.2012.09.006lalande2013impact
Latasa M., Cabello A. M., Morán X. A. G., Massana R., Scharek R. (2017). Distribution of Phytoplankton Groups Within the Deep Chlorophyll Maximum. Limnol. Oceanog. 62, 665–685. doi: 10.1002/lno.10452
Leakey R., Burkill P., Sleigh M. (1994). A Comparison of Fixatives for the Estimation of Abundance and Biovolume of Marine Planktonic Ciliate Populations. J. Plankt. Res. 16, 375–389. doi: 10.1093/plankt/16.4.375
Legendre P., Oksanen J., ter Braak C. J. (2011). Testing the Significance of Canonical Axes in Redundancy Analysis. Methods Ecol. Evol. 2, 269–277. doi: 10.1111/j.2041-210X.2010.00078.x
Liu Y., Boss E., Chase A., Xi H., Zhang X., Röttgers R., et al. (2019). Retrieval of Phytoplankton Pigments From Underway Spectrophotometry in the Fram Strait. Remote Sens. 11, 318. doi: 10.3390/rs11030318
Loeng H. (1991). Features of the Physical Oceanographic Conditions of the Barents Sea. Pol. Res. 10, 5–18. doi: 10.3402/polar.v10i1.6723
Mackey M., Mackey D., Higgins H., Wright S. (1996). CHEMTAX- a Program for Estimating Class Abundances From Chemical Markers: Application to HPLC Measurements of Phytoplankton. Mar. Ecol. Prog. Ser. 144, 265–283. doi: 10.3354/meps144265
Margalef R. (1978). Life Forms of Phytoplankton as Survival Alternatives in an Unstable Environment. Oceanol. Acta 1, 4–493.
Menden-Deuer S., Lessard E. J. (2000). Carbon to Volume Relationships for Dinoflagellates, Diatoms, and Other Protist Plankton. Limnol. Oceanog. 45, 569–579. doi: 10.4319/lo.2000.45.3.0569
Menden-Deuer S., Lessard E. J., Satterberg J. (2001). Effect of Preservation on Dinoflagellate and Diatom Cell Volume and Consequences for Carbon Biomass Predictions. Mar. Ecol. Prog. Ser. 222, 41–50. doi: 10.3354/meps222041
Mitchell E., McNeil S., Whyte C., Cottier F., Hopkins J., Davidson K. (2021a). Flow Cytometry Analysis of Water Samples for Bacterial and Pico-Plankton Enumeration, Samples Collected in the Barents Sea During 2017-2018 (V1) (Cambridge: UK Polar Data Centre). doi: 10.5285/2182fd8d-0a0d-4f56-9bf9-4dffaa67ff4b
Mitchell E., McNeil S., Whyte C., Cottier F., Hopkins J., Davidson K. (2021b). Phytoplankton Enumeration and Biomass Calculations From Samples Collected in the Barents Sea During 2017-2018 (V1) (Cambridge: UK Polar Data Centre). doi: 10.5285/69ae1fa2-cf1d-44da-8415-d9cc512c0256
Montagnes D. J., Franklin M. (2001). Effect of Temperature on Diatom Volume, Growth Rate, and Carbon and Nitrogen Content: Reconsidering Some Paradigms. Limnol. Oceanog. 46, 2008–2018. doi: 10.4319/lo.2001.46.8.2008
Mutshinda C. M., Finkel Z. V., Widdicombe C. E., Irwin A. J. (2016). Ecological Equivalence of Species Within Phytoplankton Functional Groups. Funct. Ecol. 30, 1714–1722. doi: 10.1111/1365-2435.12641
Neukermans G., Oziel L., Babin M. (2018). Increased Intrusion of Warming Atlantic Water Leads to Rapid Expansion of Temperate Phytoplankton in the Arctic. Global Change Biol. 24, 2545–2553. doi: 10.1111/gcb.14075
Nöthig E.-M., Bracher A., Engel A., Metfies K., Niehoff B., Peeken I., et al. (2015). Summertime Plankton Ecology in Fram Strait- a Compilation of Long and Short-Term Observations. Pol. Res. 34, 23349. doi: 10.3402/polar.v34.23349
Oksanen J., Blanchet F. G., Friendly M., Kindt R., Legendre P., McGlinn D., et al. (2019). Vegan: Community Ecology Package, R package version 2.5-6. Availlable at: https://CRAN.R-project.org/package=vegan
Oliveira A. L., Rudorff N., Kampel M., Sathyendranath S., Pompeu M., Detoni A. M., et al. (2021). Phytoplankton Assemblages and Optical Properties in a Coastal Region of the South Brazil Bight. Continent. Shelf. Res. 227, 104509. doi: 10.1016/j.csr.2021.104509
Orkney A., Bouman H. A. (2019a). Phytoplankton Absorption Spectra, Derived From Measurements of Absorption From Phytoplankton Samples Taken in the Barents Sea During Cruise HH180423 (V1) (Liverpool: British Oceanographic Data Centre). doi: 10.5285/982b6da2-7e11-060a-e053-6c86abc09389orkney2019HH180423
Orkney A., Bouman H. A. (2019b). Phytoplankton Absorption Spectra, Derived From Measurements of Absorption From Phytoplankton Samples Taken in the Barents Sea During Cruise JR16006 (V1) (Liverpool: British Oceanographic Data Centre). doi: 10.5285/97daa7ea-8792-6cff-e053-6c86abc0dd46orkney2019jr16006
Orkney A., Bouman H. A. (2019c). Data From: Phytoplankton Absorption Spectra, Derived From Measurements of Absorption From Phytoplankton Samples Taken in the Barents Sea During Cruise JR17006 (V1) (Liverpool: British Oceanographic Data Centre). doi: 10.5285/982b6da2-7e12-060a-e053-6c86abc09389
Orkney A., Platt T., Narayanaswamy B. E., Kostakis I., Bouman H. A. (2020). Bio-Optical Evidence for Increasing Phaeocystis Dominance in the Barents Sea. Philos. Trans. R. Soc. A. 378, 20190357. doi: 10.1098/rsta.2019.0357
Overland J., Dunlea E., Box J. E., Corell R., Forsius M., Kattsov V., et al. (2018). The Urgency of Arctic Change. Pol. Sci. 28, 6–13. doi: 10.1016/j.polar.2018.11.008
Oziel L., Baudena A., Ardyna M., Massicotte P., Randelhoff A., Sallée J.-B., et al. (2020). Faster Atlantic Currents Drive Poleward Expansion of Temperate Phytoplankton in the Arctic Ocean. Nat. Commun. 11, 1–8. doi: 10.1038/s41467-020-15485-5
Oziel L., Sirven J., Gascard J.-C. (2016). The Barents Sea Frontal Zones and Water Masses Variability, (1980–2011). Ocean. Sci. 12 (1), 169–184. doi: 10.5194/os-12-169-2016
Pavlov A. K., Taskjelle T., Kauko H. M., Hamre B., Hudson S. R., Assmy P., et al. (2017). Altered Inherent Optical Properties and Estimates of the Underwater Light Field During an Arctic Under-Ice Bloom of Phaeocystis Pouchetii. J. Geophys. Res.: Ocean. 122, 4939–4961. doi: 10.1002/2016JC012187
Peperzak L. (2010). An Objective Procedure to Remove Observer-Bias From Phytoplankton Time-Series. J. Sea. Res. 63, 152–156. doi: 10.1016/j.seares.2009.11.004
Peralta-Ferriz C., Woodgate R. A. (2015). Seasonal and Interannual Variability of Pan-Arctic Surface Mixed Layer Properties From 1979 to 2012 From Hydrographic Data, and the Dominance of Stratification for Multiyear Mixed Layer Depth Shoaling. Prog. Oceanog. 134, 19–53. doi: 10.1016/j.pocean.2014.12.005
Polyakov I. V., Pnyushkov A. V., Alkire M. B., Ashik I. M., Baumann T. M., Carmack E. C., et al. (2017). Greater Role for Atlantic Inflows on Sea-Ice Loss in the Eurasian Basin of the Arctic Ocean. Science 356, 285–291. doi: 10.1126/science.aai8204
Rat’kova T. N., Wassmann P. (2002). Seasonal Variation and Spatial Distribution of Phyto- and Protozooplankton in the Central Barents Sea. J. Mar. Syst. 38, 47–75. doi: 10.1016/S0924-7963(02)00169-0
R Core Team (2020). R: A Language and Environment for Statistical Computing (Vienna, Austria: R Foundation for Statistical Computing).
Reigstad M., Riser C. W., Wassmann P., Ratkova T. (2008). Vertical Export of Particulate Organic Carbon: Attenuation, Composition and Loss Rates in the Northern Barents Sea. Deep. Sea. Res. Part II.: Top. Stud. Oceanog. 55, 2308–2319. doi: 10.1016/j.dsr2.2008.05.007
Riser C. W., Reigstad M., Wassmann P., Arashkevich E., Falk-Petersen S. (2007). Export or Retention? Copepod Abundance, Faecal Pellet Production and Vertical Flux in the Marginal Ice Zone Through Snap Shots From the Northern Barents Sea. Pol. Biol. 30, 719–730. doi: 10.1007/s00300-006-0229-z
Riser C. W., Wassmann P., Olli K., Pasternak A., Arashkevich E. (2002). Seasonal Variation in Production, Retention and Export of Zooplankton Faecal Pellets in the Marginal Ice Zone and Central Barents Sea. J. Mar. Syst. 38, 175–188. doi: 10.1016/S0924-7963(02)00176-8
Roy S., Llewellyn C. A., Egeland E. S., Johnsen G. (2011). Phytoplankton Pigments: Characterization, Chemotaxonomy and Applications in Oceanography (Cambridge: Cambridge University Press).
Sathyendranath S., Aiken J., Alvain S., Barlow R., Bouman H., Bracher H., et al. (2014). Reports of the International Ocean-Colour Coordinating Group (IOCCG) (Dartmouth, Canada: International Ocean-Colour Coordinating Group), 1–156. Available at: https://ioccg.org/wp-content/uploads/2018/09/ioccg_report_15_2014.pdf.
Sathyendranath S., Watts L., Devred E., Platt T., Caverhill C., Maass H. (2004). Discrimination of Diatoms From Other Phytoplankton Using Ocean-Colour Data. Mar. Ecol. Prog. Ser. 272, 59–68. doi: 10.3354/meps272059
Shutler J., Land P., Brown C., Findlay H., Donlon C., Medland M., et al. (2013). Coccolithophore Surface Distributions in the North Atlantic and Their Modulation of the Air-Sea Flux of CO2 From 10 Years of Satellite Earth Observation Data. Biogeosciences 10, 2699–2709. doi: 10.5194/bg-10-2699-2013
Strass V. H., Nöthig E.-M. (1996). Seasonal Shifts in Ice Edge Phytoplankton Blooms in the Barents Sea Related to the Water Column Stability. Pol. Biol. 16, 409–422. doi: 10.1007/BF02390423
Sun J., Liu D. (2003). Geometric Models for Calculating Cell Biovolume and Surface Area for Phytoplankton. J. Plankt. Res. 25, 1331–1346. doi: 10.1111/j.1751-8369.1991.tb00653.x
Syvertsen E. E. (1991). Ice Algae in the Barents Sea: Types of Assemblages, Origin, Fate and Role in theIce-Edge Phytoplankton Bloom. Polar Res 10, 277–288. doi: 10.1111/j.1751-8369.1991.tb00653.x
Throndsen J., Hasle G. R., Tangen K. (2007). Phytoplankton of Norwegian Coastal Waters (Almater Forlag AS).
Tillmann U. (2004). Interactions Between Planktonic Microalgae and Protozoan Grazers 1. J. Euk. Microbiol. 51, 156–168. doi: 10.1111/j.1550-7408.2004.tb00540.x
Utermöhl H. (1958). Zur Vervollkommnung Der Quantitativen Phytoplankton-Methodik: Mit 1 Tabelle Und 15 Abbildungen Im Text Und Auf 1 Tafel. Internat. Vereinigung. für. Theoret. Und. Angewandte. Limnol.: Mitt. 9, 1–38. doi: 10.1080/05384680.1958.11904091
Våge K., Pickart R. S., Pavlov V., Lin P., Torres D. J., Ingvaldsen R., et al. (2016). The Atlantic Water Boundary Current in the Nansen Basin: Transport and Mechanisms of Lateral Exchange. J. Geophys. Res.: Ocean. 121, 6946–6960. doi: 10.1002/2016JC011715
Van Heukelem L., Thomas C. S. (2001). Computer-Assisted High-Performance Liquid Chromatography Method Development With Applications to the Isolation and Analysis of Phytoplankton Pigments. J. Chromatog. J. Chromatogr. 910 (1), 31–49. doi: 10.1016/S0378-4347(00)00603-4
Vernet M., Whitehead K. (1996). Release of Ultraviolet-Absorbing Compounds by the Red-Tide Dinoflagellate Lingulodinium Polyedra. Mar. Biol. 127, 35–44. doi: 10.1007/BF00993641
Vidussi F., Claustre H., Manca B. B., Luchetta A., Marty J.-C. (2001). Phytoplankton Pigment Distribution in Relation to Upper Thermocline Circulation in the Eastern Mediterranean Sea During Winter. J. Geophys. Res.: Ocean. 106, 19939–19956. doi: 10.1029/1999JC000308
Wang S., Elliott S., Maltrud M., Cameron-Smith P. (2015). Influence of Explicit Phaeocystis Parameterizations on the Global Distribution of Marine Dimethyl Sulfide. J. Geophys. Res.: Biogeosci. 120, 2158–2177. doi: 10.1002/2015JG003017
Wassmann P., Reigstad M., Haug T., Rudels B., Carroll M. L., Hop H., et al. (2006). Food Webs and Carbon Flux in the Barents Sea. Prog. Oceanog. 71, 232–287. doi: 10.1016/j.pocean.2006.10.003
Zubkov M. V., Burkill P. H. (2006). Syringe Pumped High Speed Flow Cytometry of Oceanic Phytoplankton. Cytomet. Part A.: J. Int. Soc. Analyt. Cytol. 69, 1010–1019. doi: 10.1002/cyto.a.20332
Keywords: Arctic, Barents Sea, marine ecosystems, bio-optics, pigments, ocean monitoring, atlantification, phytoplankton diversity
Citation: Orkney A, Davidson K, Mitchell E, Henley SF and Bouman HA (2022) Different Observational Methods and the Detection of Seasonal and Atlantic Influence Upon Phytoplankton Communities in the Western Barents Sea. Front. Mar. Sci. 9:860773. doi: 10.3389/fmars.2022.860773
Received: 23 January 2022; Accepted: 04 April 2022;
Published: 04 May 2022.
Edited by:
Johnna M. Holding, Aarhus University, DenmarkReviewed by:
Dylan Catlett, Woods Hole Oceanographic Institution, United StatesTimothy James Smyth, Plymouth Marine Laboratory, United Kingdom
Copyright © 2022 Orkney, Davidson, Mitchell, Henley and Bouman. This is an open-access article distributed under the terms of the Creative Commons Attribution License (CC BY). The use, distribution or reproduction in other forums is permitted, provided the original author(s) and the copyright owner(s) are credited and that the original publication in this journal is cited, in accordance with accepted academic practice. No use, distribution or reproduction is permitted which does not comply with these terms.
*Correspondence: Andrew Orkney, c2F4b24ud2Vzc2V4Lm9ya25leUBnbWFpbC5jb20=
†ORCID: Andrew Orkney, orcid.org/0000-0003-4972-2541
Keith Davidson, orcid.org/0000-0001-9269-3227
Elaine Mitchell, orcid.org/0000-0001-7274-1009
Sian F. Henley, orcid.org/0000-0003-1221-1983
Heather A. Bouman, orcid.org/0000-0002-7407-9431