Mangroves Cover Change Trajectories 1984-2020: The Gradual Decrease of Mangroves in Colombia
- 1Biospheric Sciences Laboratory, The National Aeronautics and Space Administration ( NASA) Goddard Space Flight Center, Greenbelt, MD, United States
- 2Departamento de Topografía, Facultad de Tecnología, Universidad del Tolima, Ibagué, Colombia
- 3Jet Propulsion Laboratory, California Institute of Technology, Pasadena, CA, United States
Awareness of the significant benefits of mangroves to human lives and their role in regulating environmental processes has increased during the recent decades. Yet there remains significant uncertainty about the mangrove change trajectories and the drivers of change at national scales. In Colombia, the absence of historical satellite imagery and persistent cloud cover have impeded the accurate mapping of mangrove extent and change over time. We create a temporally consistent Landsat-derived dataset using the LandTrendr algorithm to track the historical land cover and mangrove conversion from 1984-2020 across Colombia. Over this period, mangrove extent decreased by ~48.000ha (14% of total mangrove area). We find a gradual reduction of mangrove extent along the Pacific coast since 2004, whereas, in the Caribbean, mangrove cover declined around during 1984-1988 and also after 2012. Our time-series analysis matches with drivers of mangrove change at three local sites. For instance, hydroclimatic events, dredging activities, and high sediment loads transported by the rivers have collectively improved mangrove recovery in some sites. In contrast, human activities pressure linked to agricultural expansion and road construction have degraded mangroves. The transition from dense mangrove to other vegetation types is the most significant conversion affecting mangrove cover in Colombia, impacting an area of 38,469 ± 2,829 ha. We anticipate increased mangrove loss, especially along the Pacific coast, resulting from intensified human activity. Prioritization of conservation areas is needed to support local institutions, maintain currently protected areas, and develop strategies (e.g. payment for ecosystem services) to preserve one of the most pristine mangrove regions in the Western Hemisphere.
1 Introduction
Mangroves are complex ecosystems with various benefits to humans and the environment (de Jong et al., 2021). They are well-recognized for their ecosystem services, especially for communities living in tropical and subtropical regions (Guo et al., 2021). While mangroves are known to provide services such as coastal protection (Yang et al., 2008; Shahbudin et al., 2012), carbon storage (Fatoyinbo et al., 2018; Tang et al., 2018), and biodiversity conservation (Polidoro et al., 2010), they continue to be impacted by human activities. Common land cover change trajectories result from mangrove conversion to aquaculture, agriculture, and urbanization (Goldberg et al, 2020). Other change trajectories include subtle changes ranging from selective logging and forest degradation as well as mangrove gain or recovery (Bhargava et al., 2021; Vélez-Castaño et al., 2021).
Tracking land change trajectories in mangrove is needed to identify their human and natural drivers and evaluate their impacts. Initial efforts to produce consistent global mangrove extent maps focused on finite epochs (Giri et al., 2011; Hamilton and Casey, 2016). Applications of the Landsat archive is commonly used to track local mangrove gain (Gilani et al., 2021), loss (Thampanya et al., 2006; Bhargava et al., 2021), and degradation (Lee et al., 2021). While research utilizing the extensive record of Landsat imagery for monitoring mangroves remains less exploited (Pasquarella et al., 2016), these approaches and publicly available datasets already provide a much better understanding of the location of coarse drivers (Thomas et al., 2017) of mangroves change (Goldberg et al., 2020) and specific periods of loss and gain (Luijendijk et al., 2018; Mentaschi et al., 2018). The increased availability of complementary remote sensing datasets from radar has also been successfully used to produce global change maps, albeit with a coarser and more limited time-span (Bunting et al., 2018; Thomas et al., 2018).
Earth Observation technologies provide an objective means of monitoring the status of mangrove forests. However, tracking mangrove dynamics is difficult for many reasons. For instance, cloud cover and the restricted access to historical optical satellite imagery in tropical regions limit our ability to accurately evaluate mangrove extent and change. Additionally, most current mangroves datasets fail to recognize their historical dynamism because they primarily focus on bi-temporal or decadal analysis aggregating the outcomes at coarse spatial scales. Finally, there is often a discrepancy in mangrove extent area, loss, and gain at national levels (Ruiz-Luna et al., 2008; Mejía-Rentería et al., 2018; Suyadi Gao et al., 2018; Alban et al., 2020) which can potentially affect the design of policies to improve conservation outcomes (Hamilton et al., 2018).To our knowledge, there is no country-specific continuous assessment of the change in the extent of mangroves in Colombia (Bernal et al., 2017; Mejía-Rentería et al., 2018), and large uncertainties about historical mangrove change trajectories remain unknown (Mejía-Rentería et al., 2018). Previous studies have estimated mangrove extent in Colombia ranging from ~500,000 ha in 1966 (IGAC, 1966) to 214,000 ha in 2000 (Giri et al., 2011), suggesting a mangrove loss of 57% in 45 years (López-Angarita et al., 2016). However, these estimates are highly uncertain because of the diverse methodologies employed and the lack of reference validation (Zambrano Escamilla and Rubiano-Rubiano, 1996). Most previous mangrove research in Colombia has focused on localized studies. National assessments are scarce due to the limited availability of cloud-free optical imagery and the lack of a consistent method to exploit remote sensing archives. The Caribbean coast has more research given its accessibility since colonial times whereas accessibility has limited the number of studies along the Pacific coast (Castellanos-Galindo et al., 2021b).
Recent efforts by different Colombian agencies have contributed to a better assessments of mangrove extent (IDEAM, 2010; INVEMAR, 2014). Projects such as Sístema de Información para la gestión de los manglares en Colombia – (SIGMA) by the Colombian government seek to establish a platform for research and conservation strategies for these ecosystems (http://sigma.invemar.org.co/geovisor). Given the increasing global demand from commodities such as rice, shrimp, and oil palm cultivation and the current post-conflict opportunities in Colombia (Murillo-Sandoval et al., 2020), it is imperative to identify the past and current mangrove change trajectories. While large areas in Colombia are profitable for establishing Blue Carbon projects based on carbon prices (Zeng et al., 2021), the ability to facilitate management and conservation priorities depends on the capacity to monitor the condition of mangroves quantitatively. Thus, understanding historical changes in extent is the initial step for developing carbon accounting and resource management systems.
This paper combines Landsat imagery with the LandTrendr algorithm (Kennedy et al., 2010) to develop consistent temporal land cover maps at annual steps between 1984 and 2020. We quantify land change trajectories associated with mangrove gain, changes from mangrove to other vegetation types, mangrove to open water, as well as urban and agricultural expansion. We highlight sites where these changes are evident and describe potential drivers using previous research studies. Different sites highlight diverse mangrove change trajectories, and social and environmental conditions that contribute to test our remote sensing method at the National level. The contributions of this study are threefold: we initially reduce the uncertainty about the current extent and distribution of mangroves in Colombia using 36 years of satellite data. Additionally, our methodology produces consistent land change maps and is compatible with national agencies’ requirements to monitor mangroves regularly (i.e., SIGMA). Finally, we identify the location of mangrove change and qualitatively identify specific natural and human drivers. We specifically ask:
1. What is the current and historical extent of mangroves in Colombia?
2. What were the mangrove change trajectories and specific transitions of gain and loss?
3. How can the most dynamic locations of mangroves change be attributed to specific human-induced activities and natural drivers?
2 Study Area
Approximately 70% of Colombia’s mangroves are found along the Pacific coast, with 30% on the Caribbean coast (Bernal et al., 2017). Colombia hosts 1.5% of global mangrove carbon stock (Simard et al., 2019a). Low alluvial plains characterize the Pacific coast fed by many large rivers from the Western Andes. The coastline is about 1600 km long and considered one of the rainiest places on the Earth (Mejía et al., 2021). With an average precipitation of ~8000 mm, frequent cloud cover seriously hampers optical remote sensing studies (Fagua and Ramsey, 2019). The environmental conditions and poor accessibility have contributed to keeping the remaining mangroves mostly undisturbed across large swaths of the Pacific coast. This condition has allowed the development of some of the tallest mangroves forests in South America, with canopies reaching 54 meters (Simard et al., 2019a) and trees measured in situ reaching 57 meters (Castellanos-Galindo et al., 2021a).
In contrast, the Caribbean coast is drier than the Pacific, with annual precipitation reaching ~2500 mm along the ~1300 km coastline. Under microtidal conditions in the Caribbean, mangroves are mainly located on small deltas and coastal lagoons. The Caribbean is more developed than the Pacific coast, with many small cities and five large commercial ports (Riohacha, Santa Marta, Barranquilla, Cartagena, and Turbo) (Correa and Morton, 2010). Human pressure on mangrove forests has been more extensive on the Caribbean than on the Pacific coast. With more consistent cloud-free satellite observations, the availability of studies in the Caribbean is more extensive (Simard et al., 2008; Villate Daza et al., 2020; Vélez-Castaño et al., 2021).The geomorphological setting of mangroves in Colombia varies greatly with small and large deltas, estuaries, lagoons, open coasts, and carbonate islands. The environmental conditions between the Caribbean and the Pacific coasts also contribute to diverse mangrove structure (Blanco-Libreros et al., 2022). The meso- and macro-tidal Pacific coast is dominated by alluvial plains and large river deltas where mangrove have developed under high rainfall conditions (Castellanos-Galindo et al., 2021b). In contrast, the microtidal Caribbean coast is mainly a dry environment in which mangroves occur in medium to small deltas, coastal lagoons, and some marginal areas along the coast (Correa and Morton, 2010).
In Colombia, the protected mangrove areas cover 67,000 ha, corresponding to 23% of the total mangrove area. There are seven mangrove species (Palacios and Cantera, 2017): Avicennia germinans (black mangrove), Rhizophora mangle (red mangrove), Laguncularia racemosa (white mangrove), Conocarpus erectus, (button mangrove) andPelliciera rhizophorae (piñuelo) are the five found in the Caribbean. Along the Pacific, we find these five species as well as Rhizophora racemosa and Mora oleifera.
2.1 Case Study Sites
We selected three sites with diverse human activities and natural processes that have affected mangrove cover. The selected sites are important to test our method and link them to specific drivers of mangrove change. First, in the Caribbean, Cienaga Grande de Santa Marta (CGSM) is the largest lagoon–delta complex in Colombia (1280 km2) (Botero and Salzwedel, 1999). Mangrove extent here has dramatically decreased due to the construction of roads (Botero and Salzwedel, 1999). These roads resulted in the diversion, diking, and drainage of freshwater courses and subsequent die-off of 60 to 90% of the mangrove areas (Bernal et al., 2017). Dredging activities increased freshwater input into the CGSM that temporally improved mangrove recovery. Second, the Gulf of Urabá, also known as the Darien region, is the most extensive sea inlet on the Colombian coasts (Blanco et al., 2012). The Western part is mainly affected by natural conditions, while its Eastern part has suffered the impact of anthropogenic activities such as large agricultural expansion linked to land grabbing processes (Ballvé, 2013). Finally, Sanquianga is the largest protected area along the Pacific coast of South America and is located on the new Patía-Sanquianga delta plain. In 1972 water diversion and channelization changed this northern estuarine system into an active delta plain mainly for easier extraction of logged trees (Restrepo, 2012; Bernal et al., 2017). This diversion shifted more than 90% of the Patia River discharged through the Sanquianga River with diverging impacts on mangrove cover (Restrepo, 2012).
3 Data and Methods
We divide the methods into four parts (see Figure 1). First, we combine Sentinel 1 (S1) and Sentinel 2 (S2) to create a 10m-resolution land cover base map for 2020 (Section 3.1) trained with official map sources. This base map allows the collection of enough training data for time-series analysis. Second, we apply the LandTrendr algorithm to remove year-to-year variability, interpolate missing data and enhance spectral separability between land cover classes using temporally consistent and multivariate descriptions of the landscape (Section 3.2). Third, we identify sites where mangrove loss and gain are evident and explain these changes using available reports and previous research (Section 3.3). Finally, an accuracy assessment and area estimation are provided (Section 3.4).
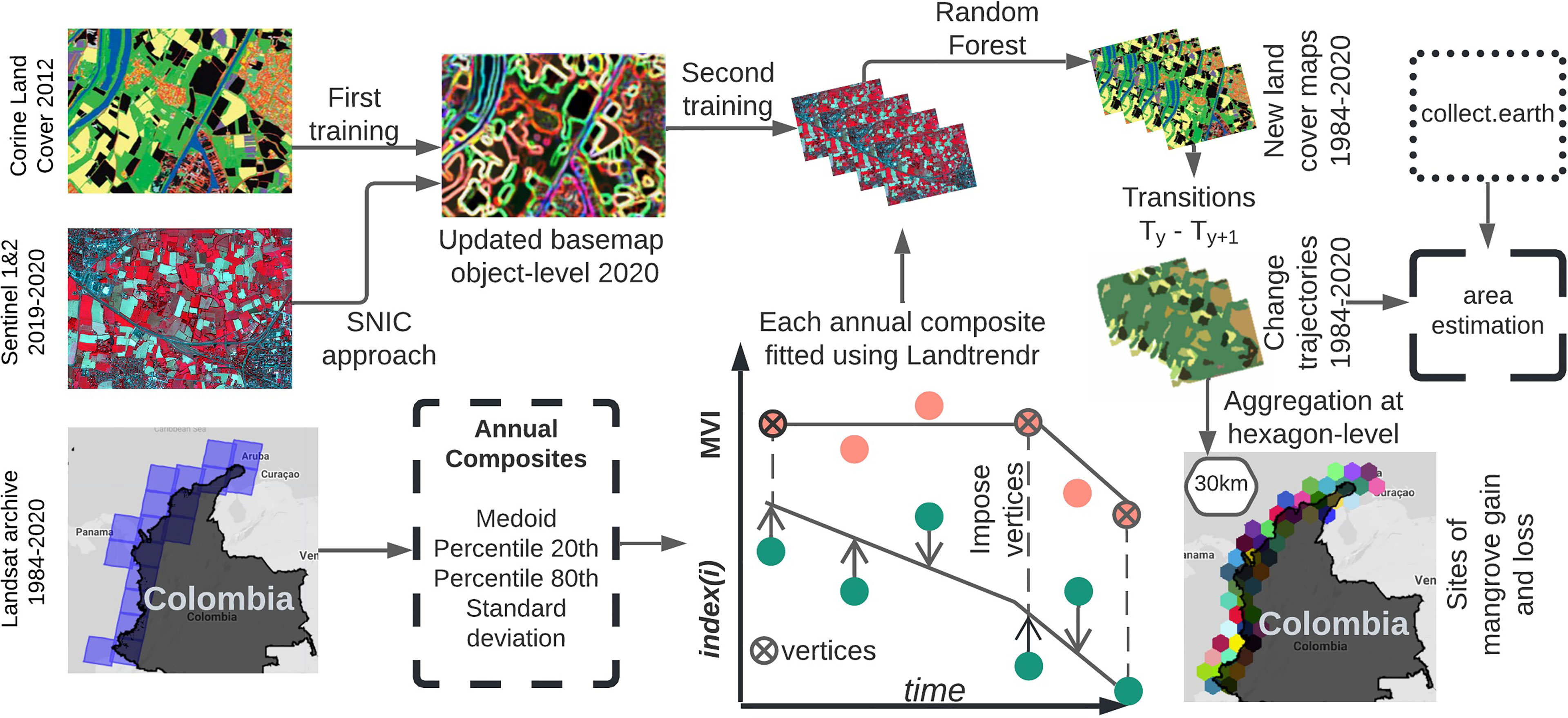
Figure 1 Flowchart methodology. Mangrove Vegetation Index (MVI) is the main index employed for temporal segmentation. Vertices from MVI are imposed over each spectral predictor (index (i), see Supplementary) to create smoothed profiles.
3.1 Base Map 2020
We employ Synthetic Aperture Radar (SAR) data from Sentinel 1 A and B Sensors and optical data from Sentinel 2 to create an new land cover map with a spatial resolution of 10m across Colombia. First, we build a cloud-free Sentinel-2 image composite including data collected in 2019-2020. Our predictors based on the image composites include the mean, percentile 20th, 80th, and the standard deviation for each spectral optical band in Sentinel-2 and VV and VH polarizations for Sentinel-1A/B. Additionally, we include several vegetation indices and Gray-Level Co-occurrence Matrix (GLCM) indicators (see Supplementary). Second, we collect training data (first training, see Figure 1). The training data comes from the official Colombian Corine Land Cover map 2012 (CLC) (IDEAM, 2010). The CLC map identifies the five most common land cover classes along the Colombian coasts as agriculture, dense forest, mangroves, water, and scrub-shrub wetland associations.
We created a mask of 2012 to 2020 class persistence using the Global Forest Cover disturbance, tree canopy cover datasets (Hansen et al., 2013), and JRC Global Surface Water (Pekel et al., 2016). This allowed the selection of training samples on S1 and S2 image composite for year 2020 based on classes identified in 2012 Corine Land cover map. We applied a Simple Non-Iterative Clustering (SNIC) algorithm available in Google Earth Engine to S1 and S2 image composite and spectral indices (see Supplementary). SNIC is an object-based image segmentation approach useful to group neighboring pixels with similar characteristics into clusters (Tassi and Vizzari, 2020). We randomly sampled 1000 SNIC clusters per class and confirmed class labels through visual inspection of Planet data in the Collect Earth application. This process removed 549 samples that could not be assigned (e.g., cloud cover or not available imagery). A total of 4451 samples were divided into training (70%) and validation (30%). We found the Mangrove Vegetation Index (MVI) as the dominant metric for spatial segmentation to delineate mangroves from non-mangroves (Baloloy et al., 2020). After image segmentation, mean values of each predictor were assigned to each cluster and classified with the RandomForest algorithm to produce a new base land cover for 2020 (Breiman, 2001). Our method is highly automated using the Google Earth Engine platform (Gorelick et al., 2017).
3.2 Landsat Preprocessing and LandTrendr Setup
In this section, we created a new spectral dataset after a linear fit using LandTrendr algorithm (Kennedy et al., 2010). This process creates a temporally consistent dataset that provides a better temporal stabilization of spectral metrics to build consistent land cover maps. We analyzed 7853 Landsat Tier 1 images in the GEE Catalog between 1984 and 2020. For each image, we applied a series of steps. First, after using the standard cloud and shadow masking procedure, we visually removed 238 images with artifacts that would affect the creation of annual composites. Second, we produced homogenous spectral values for Landsat 4 through 8 using Roy’s parameters (Roy et al., 2016). Third, topographic effects were reduced using the Dymond-Shepherd physical correction (Shepherd and Dymond, 2003). Fourth, a negative 2 km buffer around each image was applied to remove scene edge artifacts. Finally, we clumped the spectral data to eliminate undesirable outliers.
After preprocessing, we created annual image composites using four methods: medoid, percentile 20th, 80th, and standard deviation. Medoid is a well-recognized method for building radiometrically consistent Landsat composites with the smallest average heterogeneity in tropical regions (Van doninck and Tuomisto, 2018). Instead of minimum and maximum values, we use the 20th and 80th percentiles to reduce sensitivity to shadows and atmospheric contamination effects (Zhang and Roy, 2017). Additionally, the 20th percentile is helpful in differential vegetated areas that are persistent green throughout the year (Lymburner et al., 2020). Standard deviation highlights dynamic and stable surfaces. While the scarcity of enough observations in the early years (e.g., 80 and 90) can affect the performance of percentiles (Xie et al., 2020), missing Landsat observations mostly occur in mountainous regions and few locations on the coast. These are spatially filled using an average window (8 x 8 pixels) procedure.We applied the LandTrendr (LT) algorithm on each of these composite datasets. While extensively used for tracking forest disturbances and agents of change (Kennedy et al., 2010; Zhu, 2017; Kennedy et al., 2018), its usability for building consistent landcover maps is less common (Murillo-Sandoval et al., 2021). LT identifies breakpoints (vertices) where the temporal progression of spectral values is approximated to be linear to reduce noise. We simplify chronosequences into representative temporal trajectories that improve land cover maps creation through linear fit smoothing. LT needs different parameters to identify breaks; here, we use previous parametrization available for Colombian forest (see Supplementary) (Murillo-Sandoval et al., 2021). We increased the spectral separability among land cover classes by imposing the timing of the vertices detected using MVI onto a set of spectral predictors (see Supplementary Figure 1). This process provides temporal stabilization of spectral metrics removing ephemeral changes across years for each composite method. The algorithm also interpolates temporal data gaps due to clouds, cloud shadows, and Landsat-7 SLC errors.We randomly sampled our new base land cover map in 2020 (section 3.1) for the five landcover classes incorporating the predictors from different Landsat composite methods (second training, see Figure 2). Additionally, we added more training data using purposive sampling in 1990, 2000, and 2010 to have a complete temporal description of land changes. The additional training data was verified using Landsat images and high-resolution images in Google Earth. A set of ten individual landcover maps per year were created employing a RandomForest. This iterative process decreases salt-pepper effects; the mode from these maps produced the final map per year.
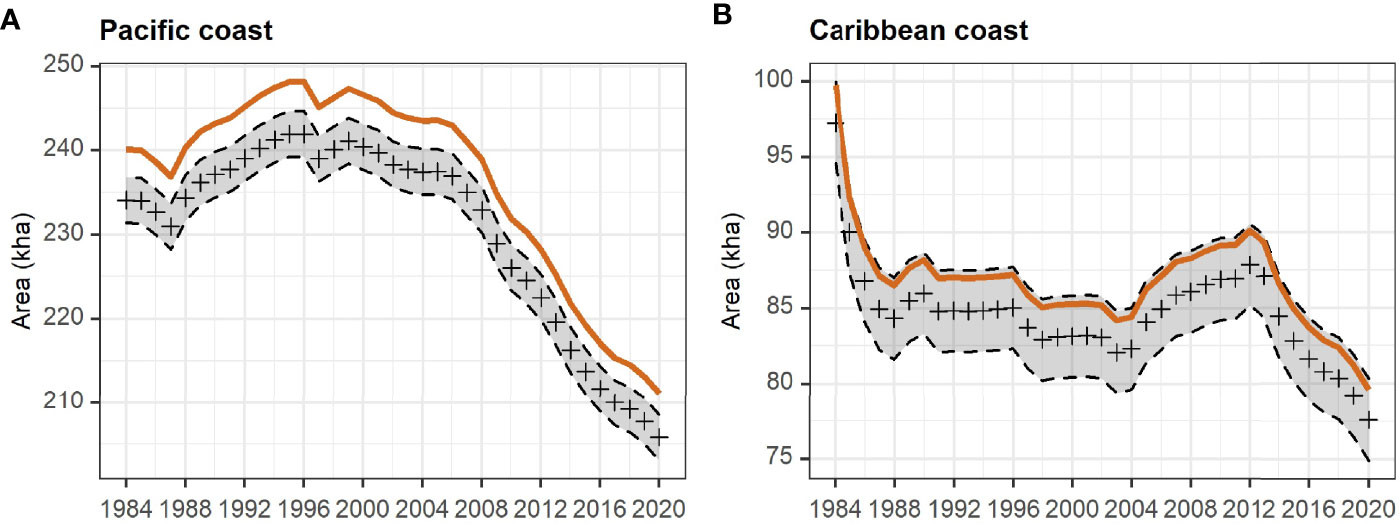
Figure 2 Mangroves area for the Pacific and Caribbean coasts. Area estimates with 95% confidence intervals (dashed lines). Brown line represents the area mapped (pixel counts), and cross symbols represent the area estimated.
3.3 Quantifying Land Change Trajectories
Land cover change maps are a proxy for detecting mangrove loss and gain processes and infer types of land use conversion. We derived five land change trajectories based on our land cover maps. The first conversion is from dense mangrove to scrub-shrub vegetation. The Instituto Geografico Agustin Codazzi (IGAC) defines dense mangroves in Colombia as tree height>15m that follows a continuous woody pattern with tree cover >70%. The scrub-shrub vegetation is also defined as tree height<4m that has been human or naturally intervened (IGAC, 1999). This conversion of mangrove to other vegetation type indicates mangrove loss. Loss and mangrove gain can be natural or anthropogenic processes that evolve in shorelines (Bhargava et al., 2021). The transition from mangroves to open water (Mentaschi et al., 2018) is considered mangrove loss. Mangrove gain is related to new mangroves expanding into the coastal ocean or inland. To determine mangrove gain, we consider the conversions from water, agriculture, and scrub-shrub vegetation to mangrove. Mangrove loss includes conversion of mangrove to open water, other vegetation types, and urban and agriculture areas. The latter two are human-driven conversions that affects the development of other land cover types (IDEAM, 2010). To reduce misclassification with tiny settlements, we employ the new ESA 2020 (Zanaga et al., 2021) urban layer to separate settlements from agriculture. Finally, classes such as permanent water and other non-mangrove-related transitions were calculated for the mapping process but not included in the area estimation assessment.
We create a difference map between 1984 and 2020 to identify the locations of mangroves gain and mangrove loss. We use spatial aggregation based on hexagons of 30 km diameter to visualize mangrove gain and loss, and identify three locations that experience mangrove gain and loss: Ciénaga Grande de Santa Marta, the Gulf of Urabá, and the Sanquianga Protected Area. We identify potential drivers of mangrove change in these regions using expert knowledge and available research.
3.4 Accuracy and Area Estimation Assessment
Good practices in remote sensing tasks recommend using unbiased estimators on stratified data using reference observations (Olofsson et al., 2020). Using an established procedure (Olofsson et al., 2014), the accuracy assessment was derived from the change map between 1984 and 2020. A random stratification sampling of 1,230 points was distributed among the five types of change trajectory and stable mangroves classes. The allocation follows 800 samples for mangroves (65% proportion area), 150 to mangrove to other vegetation types and mangrove gain, 50 to mangrove to open water and agriculture, and 30 to urban areas. While using a multi-sample approach can highlight more detail into the area estimation, developing an annual assessment would increase the number of needed samples to ~1230x35 = 43,050. Therefore, the choice of 1,230 points is merely practical and conservative, involving available human resources and making a careful effort to keep precision in the reference process.
We use a sampling unit of 30 m pixel size of the Landsat image for the reference process. We utilized CollectEarth (https://collect.earth/) which links the samples with the reference observation through a visual process. CollectEarth offers a set of tools for connecting each sampled point with its reference using Landsat images subsets, Landsat time-series plots, and high-resolution images from OpenStreetMap and Planet. Additionally, CollectEarth offers a degradation tool widget that tracks forest disturbances and helps in assigning the reference class to each sampled point. After reference data collection, we constructed error matrices and reported the estimated areas for each class. The bias in the mapped area (pixel counts) is assumed to be uniformly distributed in time and space. Consequently, the coefficient between the mapped area and the estimated area (unbiased) for the full stratification is multiplicated by the mapped area in a given year for each class to obtain the estimated area (Olofsson et al., 2016).
4 Results
4.1 Colombian Mangroves Extent and Change Trajectories
In this paper, we provide a consistent method and historical examination of mangrove extent in Colombia. Our Landsat-based analysis reveals that mangroves had declined throughout the entire study area from 331,356 ± 2,708ha in 1984 to 283,419 ± 2,708ha in 2020. In other words, Colombia lost 14% (~47,937ha) of its mangrove in 36 years (0.38% per annual change). The base map produced with Sentinel-1 and Sentinel-2 supports the current extent in 2020, with a mangrove cover of 287,678 ± 34,107ha. The Pacific coast held 234,345 ± 2,644ha, with mangroves decreasing by about ~32,000ha since 2004 (Figure 2A). The map bias, the difference between the mapped area and estimated area, is 5898ha, an overestimation that falls outside confidence intervals (Figure 2A). In contrast, the Caribbean coast has 75,689 ± 853ha, with two notable periods of decline of ~15,000ha between 1984-1988 and~10,000ha between 2012-2020 (Figure 2B). Our mapped area is within the limits of confidence intervals (Figure 2B). Producer’s accuracy was greater than 90% for all classes except for urban that reaches 40%. User’s accuracy was also greater than 90% for all classes, excluding agriculture, with a value of 79%.
The decrease in mangrove extent (Figure 3E) is mainly associated with mangrove to other vegetation types followed by mangrove to open water, adding a loss of 56439 ± 4747ha (Figures 3A, B). These two conversions gradually rise on the Pacific coast, whereas, in the Caribbean, they remain relatively constant from 1988 to 2004 (see Supplementary Figures). Other change trajectories such as agricultural and urban expansion into mangroves have a minor contribution in mangroves loss from 1984 to 2020; however, they have a higher area uncertainty: 11,285 ± 10,68ha for agriculture (Figure 3C) and 2,824 ± 1,478ha for urban areas (Figure 3D). In contrast to mangroves loss, mangrove gain reaches 46,541 ± 1,068ha (Figure 3F).
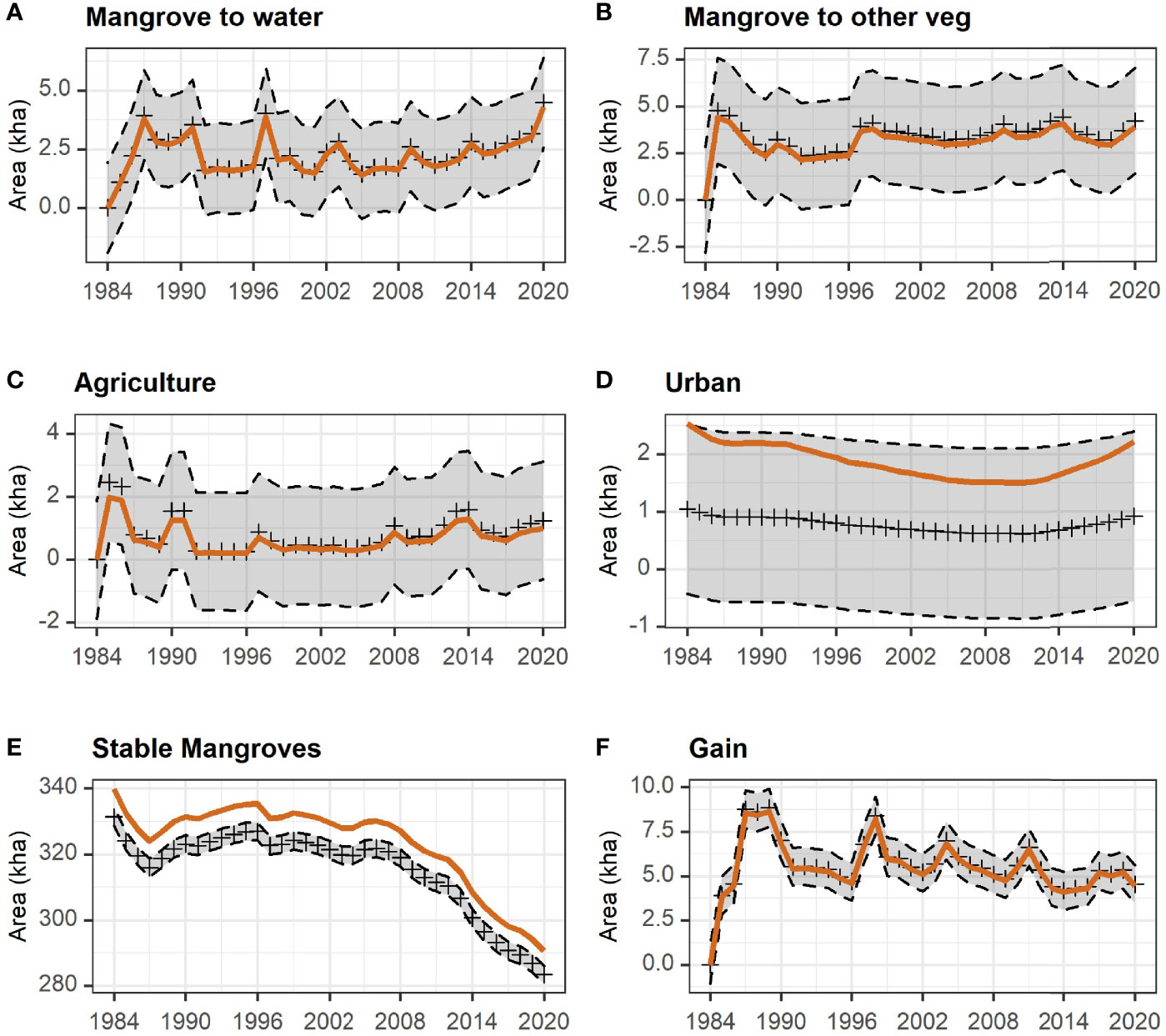
Figure 3 National level estimated and mapped areas for five trajectories of change and stable mangroves. Area estimates with 95% confidence intervals (dashed lines). Brown line represents the area mapped (pixel counts) and cross symbols represent the area estimated.
4.2 Locations of Mangroves Loss and Gain
The Landsat-based difference map between 1984 and 2020 shows the long-term mangrove gain and loss changes (Figure 4). Mangrove gain indicates new mangroves expanding into the coastal ocean or inland. Mangroves loss results from mangrove conversion to other vegetation types, open water, agricultural and urban. We found changes in mangrove extent in all coastal departments over the 36 years of analysis, except in the department of Guajira in the Caribbean and Chocó in the Pacific (Figure 4). While the Department of Guajira has little mangrove areas to start with, the Department of Chocó has a significant portion of Colombia’s mangroves. Diverse changes are presenting in our three local sites: CGMS, the gulf of Urabá and Sanquianga.
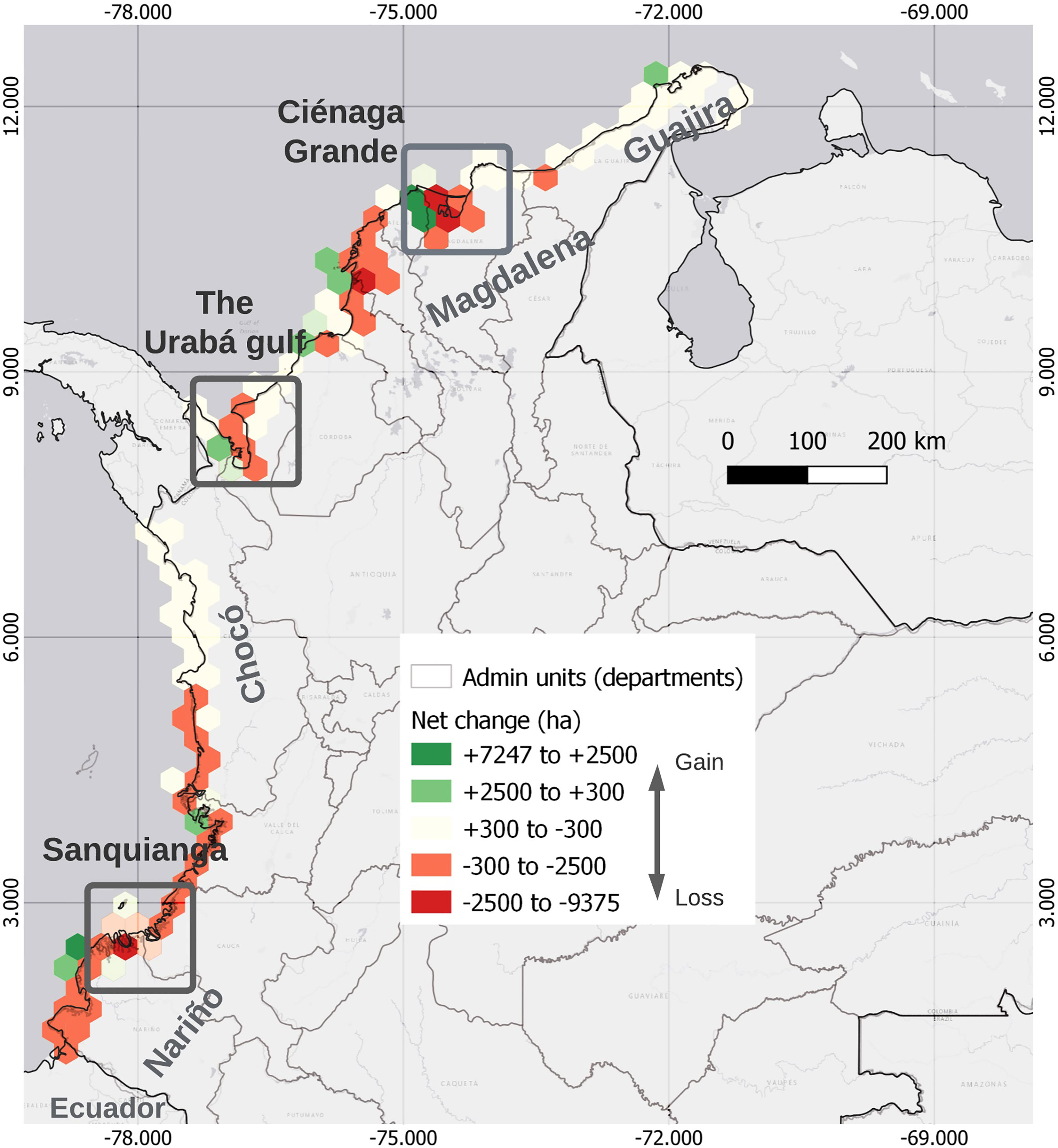
Figure 4 Study area and distribution of net mangrove extent change between 1984 and 2020. The selected study sites present diverse trends in mangrove loss and gain.
4.2.1 Ciénaga Grande de Santa Marta
The construction of roads around 1950 and consequent water diversion caused the destruction of more than 60% of mangrove cover in CGSM due to an increase in salinity (Botero and Salzwedel, 1999). However, during our analyzed period 1984-2020, the main trajectory is linked to mangrove gain (Figure 5). By 1984 mangrove cover was steady with episodes of loss and gains until 2004 and increased between 2004 and 2012 by 6,614 ± 74ha (Figure 5C). From 2012 onward, mangrove extent decreased linked to the transition from mangrove to other vegetation and mangrove to water, by 2020 mangrove extent reaches 39,996 ± 451ha. The CGSM shows prominent locations with mangrove to open water mainly located near the coast, while mangrove gain is widely distributed across the lagoon, and mangrove to other vegetation is situated in the periphery. A complete table with areas of annual change for each transition is available in Supplementary Material (Table SP7).
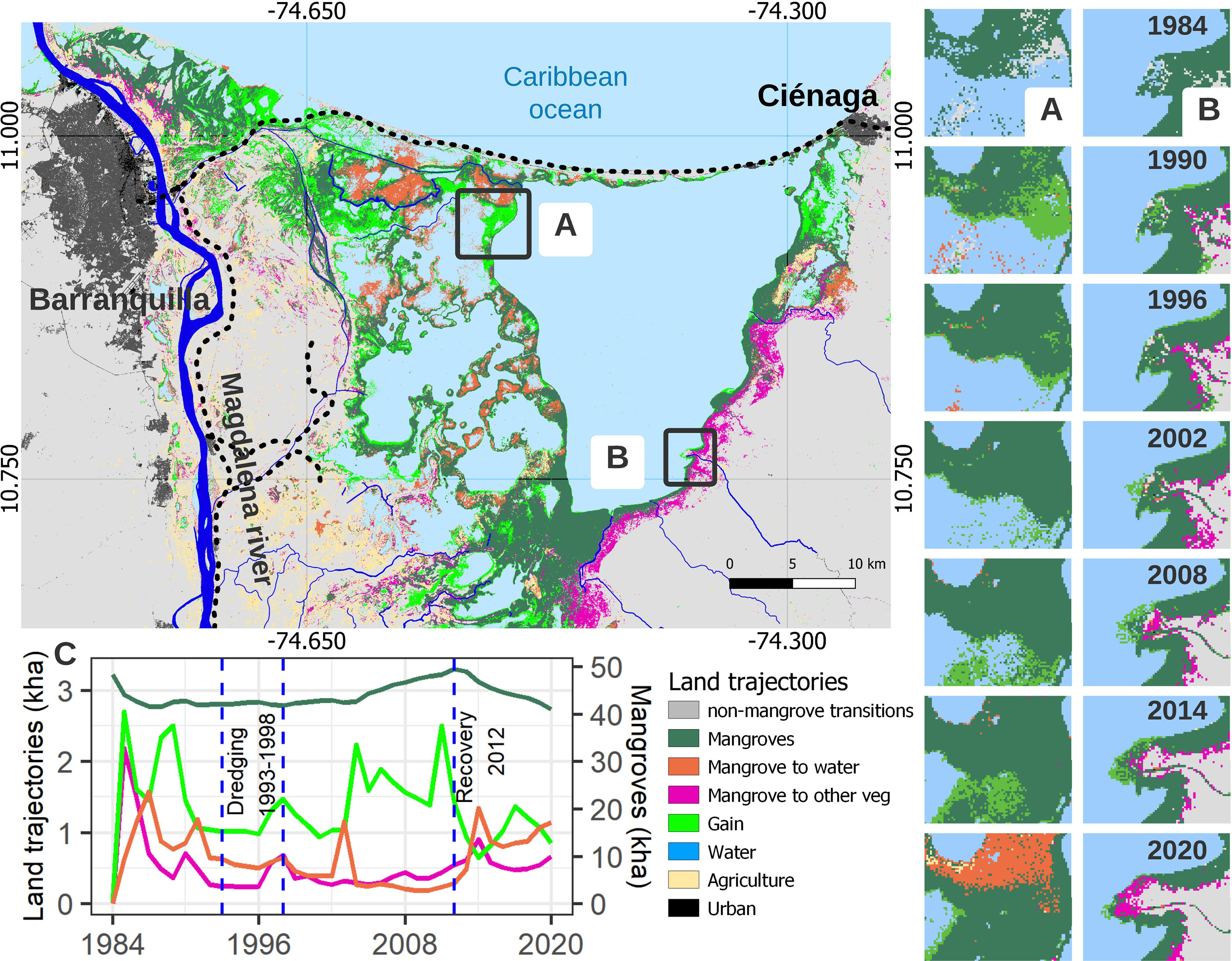
Figure 5 Map difference between 1984 and 2020 in Ciénaga Grande de Santa Marta. Black dotted lines represent the roads constructed to join Barranquilla and Ciénaga cities between 1956 to 1960 and the road parallel to Magdalena River constructed in 1970. (A) Image subsets represented mangrove gain with mangrove to open water detected in 2020. (B) Image subsets show mangrove to other vegetation inland and gain since 2008. (C) The time series plot shows the land trajectories of change and dredging period between 1993-1998 and mangrove recovery from 2004 to maximum in 2012.
4.2.2 The Gulf of Urabá
In total, our Landsat analysis indicates a mangrove area of 6,342 ± 71 ha in the Gulf of Uraba in 2020. Mangrove to other vegetation in 1999 is the main trajectory of change with a large value of 261 ± 19 ha in 1999, followed by mangrove to open water of 78 ha ± 8 ha (Figure 6C). On the Western coast, extensive mangrove gain is mapped in the mouth of the Atrato delta. While many mouths in the Atrato delta show mangroves gain, the mouth called El Roto experienced the most significant expansion of mangroves in the last 36 years with 735 ± 16 ha of mangrove gain with an annual increase rate of 32 ha per year (Figure 6). On the other hand, mangrove to open water is visible in the Northwest coast, with a yearly retreat of -14 meters per year (Figure 6A). Contrarily to the natural processes presented on the Western coast of Urabá, mangrove loss due to urbanization is presented along the eastern coast is significant around Turbo city and across the whole East Coast, putting pressure over smaller mangrove areas. In addition, some location shows mangroves gain with areas where mangroves have migrated in small river deltas. The development of large channels to drain the excess water from large banana plantations led to a large load of sediment deposits contributing to mangrove expansion (Figure 6B). More detail about areas of change is available in Supplementary Material(Table SP8).
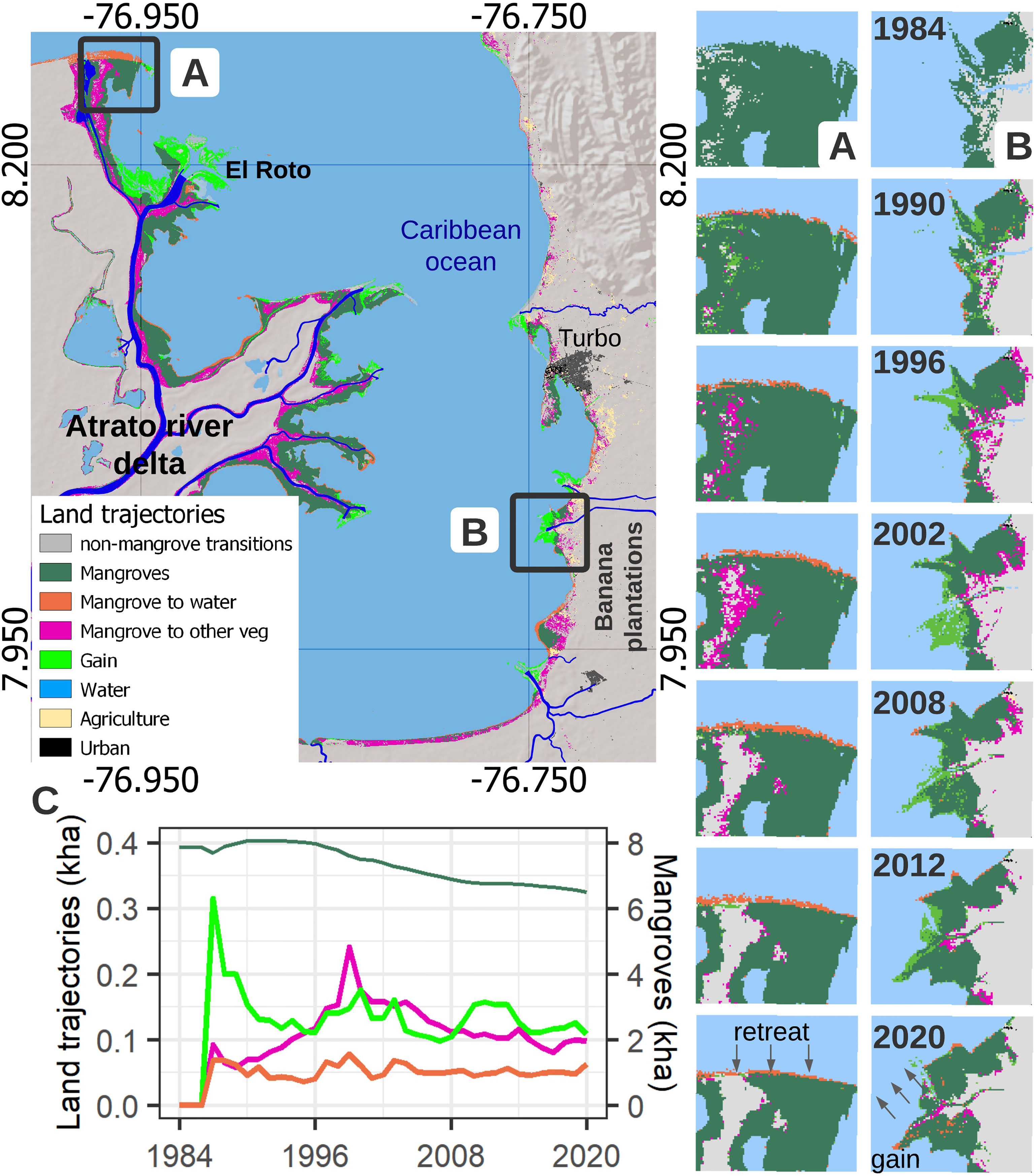
Figure 6 Map difference between 1984 and 2020 in the Gulf of Urabá. (A) Image subsets show the retreat of mangroves from in 36-years due to solid swell from the Caribbean. (B) Image subsets present gradual mangrove expansion primarily associated with sediments load from large-scale banana plantations. (C) The time series plot shows mapped areas presented in the map extent.
4.2.3 Sanquianga Protected Area
Mangrove change trajectories indicate more mangrove gain than mangrove to open water and mangrove to other vegetation. Mangrove cover in Sanquianga slightly increased from 2000 to 2014 (see change values in SP Table SP9), currently holding 41,138 ± 464ha of pristine mangroves in 2020. Additionally, two peaks of mangrove gain occurred in 1987-1988 and 1997-1998, coinciding with intense El Niño events. The warmer circumstances during El Niño favored mangrove growth, reproduction, and respiration (Riascos et al., 2018). The combined impact of these two climatic events increased mangrove gain by about 3,000ha. However, abrupt La Niña events followed these El Niño events in 1988-1989 and 1998-1999, reducing these overall gains (Figure 7B). Mangrove to open water and mangrove to other vegetation within the official limits of Sanquianga park are located on the shorelines and dispersed in small areas across the protected area. Both processes account for an annual loss of 500ha during the analysis period. Interestingly, mangrove to other vegetation is prone to happen inland outside the protected area, meaning pressure over the remaining mangrove.
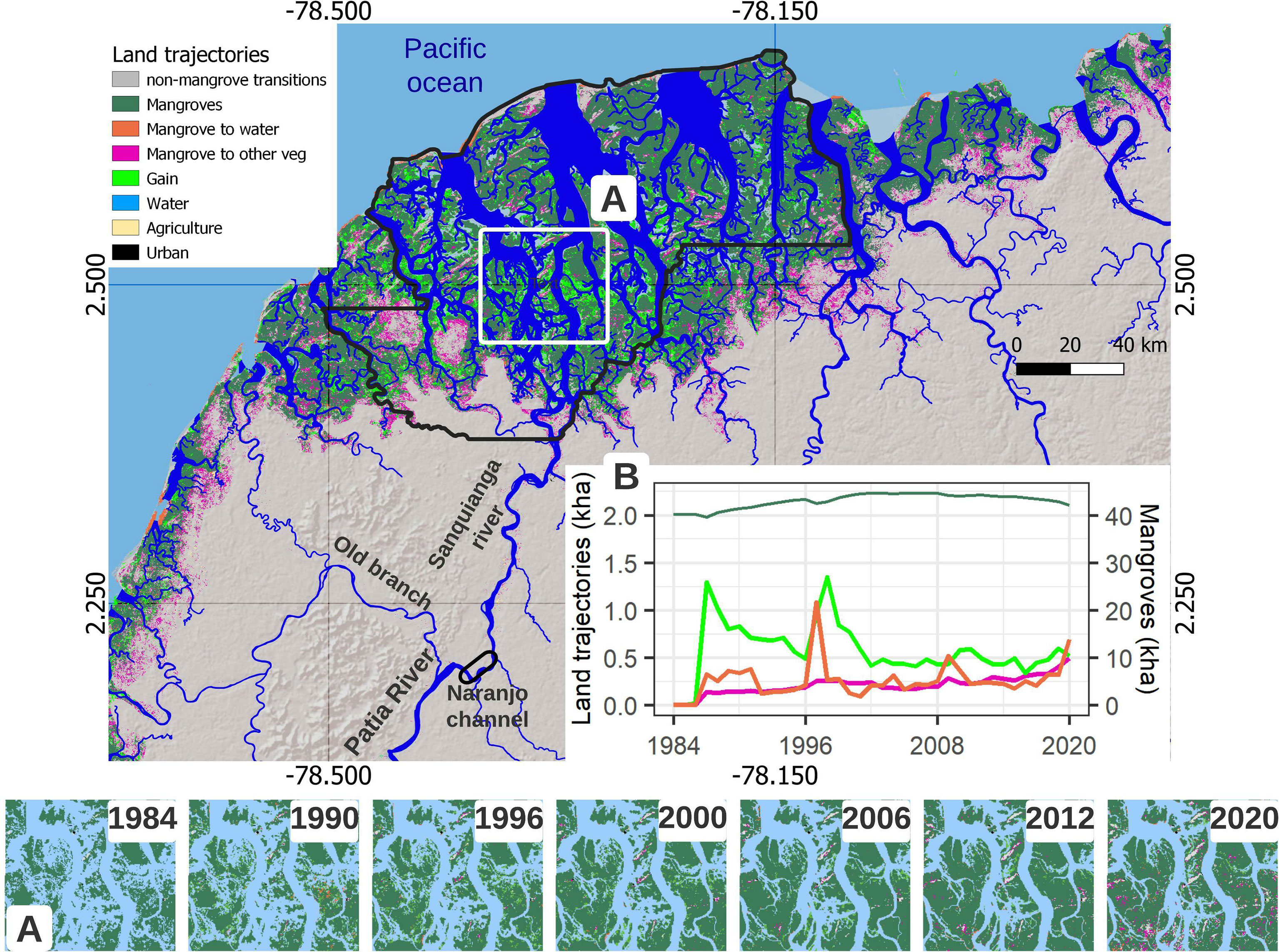
Figure 7 Difference map between 1984 and 2020 in Sanquianga Protected Area. The black line defines the official limits of Sanquianga. (A) Image subsets show the gradual channel fill with progressive mangrove formations. (B) The time series plot shows mapped areas for the official limit of Sanquianga (black line).
5 Discussion
5.1 Mangrove Dynamics at National Scale
We produce historical maps of mangrove extent in Colombia using the temporal smoothing profiles derived from LandTrendr (LT) onto Landsat imagery. Using LT fitted spectral information significantly reduced noise from phenological changes, atmospheric conditions, and illumination conditions, leading to a better representation of changes on mangrove cover. Our methodology provides historical trajectories of mangrove to open water and other vegetation types, and mangrove gain, improving previous national and global datasets efforts. Our estimate of mangrove cover for 2020 is 283419 ± 2708ha is 26%, 42%, and 20% higher than global datasets by Giri et al. (2011), Hamilton & Casey (2016), and Global Mangroves Watch in 2016 (Bunting et al., 2018) respectively. We identify mangrove patches often missed by global datasets, especially on the Pacific coast. Global datasets are very conservative and with few available imagery they often miss large portions of the mangroves distribution, this aspect has been well-documented in previous studies (Mejía-Rentería et al., 2018). However, our current Landsat mangrove cover map in 2020 is 1% smaller than the available dataset produced by national agencies (IDEAM, 2010; INVEMAR, 2014). Our method offers a quick and effective way to monitor local and regional mangrove change and provide time-effective information about mangrove status. The method can be incorporated within the official Sístema de Información para la gestión de los manglares en Colombia (SIGMA).
While Colombia has large persistent areas of mangroves, the net 14% decline in both coasts and the more recent increase in mangrove losses on the Pacific is a big concern. The conversion from mangrove to other vegetation is by far the most common trajectory of mangrove loss, and it is generally located inland in the landward mangrove zones. The Pacific coast shows a gradual decline mostly from the middle of Choco to the Southern of the country since 2014 to the present. In contrast, in the Caribbean coast, two periods of mangrove loss indicate that changes are widely distributed along the entire coastline. While we observed both mangrove gain and losses in Colombia, there has been a net decrease in mangrove area since 1984 due to human activities associated with the gradual expansion of agricultural activities, selective logging and mining (Mejía-Rentería et al., 2018; INVEMAR, 2020).
5.2 Potential Drivers of Mangrove Change at Local Scale
Our empirical contribution investigates mangrove change trajectories based on previous studies in three mangrove sites. The construction of roads surrounding the Cienaga Grande de Santa Marta region started in 1950. The consequent reduction in hydrologic connectivity, combined with warmer periods, resulted in hypersaline conditions that caused conversion of mangrove to other vegetation types and prevented mangrove recovery (Jaramillo et al., 2018). To re-establish the necessary hydrological conditions for mangroves recovery, five of the pre-existing natural tributaries were dredged between 1993 and 1998 to improve freshwater and reduce salinity (Botero and Salzwedel, 1999). Our analysis shows that mangrove extent increased in 2004, suggesting that dredging activities were successful. In addition, the persistent La Niña event between 1999 and 2001 also enhanced freshwater into the CGSM and boosted mangrove gain. The positive increase in mangroves after 2012 was altered by the intense El Niño events in 2015-2016 and 2018-2019 that reduced the mangrove recovery trend (Blanco et al., 2006). In CGSM, the increased streamflow in the Magdalena River combined with dredging operations reduce salinity that contribute to mangrove growth during a short timeframe (Blanco et al., 2006; Jaramillo et al., 2018).
In the Gulf of Urabá, diverging mangrove processes have occurred along its Eastern and Western coasts. First, on the Western side, mangrove gain dominates trajectory in Atrato river delta mouth. Here mangrove gain is driven by high sediment load discharge of the Atrato River fed by high precipitation rates Chocó Region (Blanco et al., 2012). Some authors also linked the expansion of mangroves in the Atrato delta with legal gold mining peaks and periods of heavy rain associated with La Niña events (Vélez-Castaño et al., 2021). Erosion only occurs in limited areas mainly due to the low sinuosity of the Atrato River, which is a highly vegetated floodplain with resilient riverbanks. A larger area of erosion led to a retreat of the mangroves due to north-to-south coastal drift and extreme swell events that constantly hit the coast in a perpendicular orientation (Vélez-Castaño et al., 2021). On the Eastern coast, the role of armed conflict has shaped land distribution and consequently the expansion of agriculture affecting mangroves extent (Muñoz-mora et al., 2015). Through different strategies such as paper falsification, direct threats, and killing, illegal actors have taken land from small farmers and established large-scale agricultural projects (Grajales, 2011; Ballvé, 2013). The increase in bananas, plantain crops, and the expansion of pasture lands are the leading cause of transition of mangrove to other vegetation and deforestation (Figure 6). The struggles between small and large landholders remain in Urabá (Truth Commission - Forensic Architecture, 2021). However, disentangling these drivers and their relative impact would require field monitoring, local testimonies, and participative research that was beyond the scope of this study.In Sanquianga, water diversion in 1972 converted this estuarine system into a new active delta. The higher discharged contributed to a widening of the Sanquianga River, leading to active sedimentation and channel filling (Restrepo, 2012). Consequently, pioneer mangroves gradually colonized the fine sediments, and morphological changes associated with channel fill are detected in the Landsat time-series (Figure 7A). While an evident mangrove gain is observed through the 36-years analysis, the conversion of mangrove to open water and to other vegetation types are minimal along Sanquiaga’s shoreline. Episodes of sea-level rise associated with the El Niño events (Restrepo, 2012) may have increased shoreline loss. Sanquianga shows a persistent mangrove extent, indicating the system is currently balanced in terms of mangrove gain and loss. In addition, the growth of coca crops surrounding Sanquianga affects mangrove cover, given the contamination of water by wasted chemicals (Parra and Restrepo-Angel, 2014). The presence of illegal groups also limits the livelihoods of communities. As losing access to gathering cockles, coca’s economy is the only farming alternative putting more pressure over mangroves, increasing communities vulnerability, and shifting social and economic outcomes (UNODC, 2017; Treviño and Murillo-Sandoval, 2021).
5.3 Limitations and Future Work
Our remote sensing analysis capture commonly absent mangrove patches in previous global datasets, however some uncertainties remain. For instance, we do not use pixel-based tidal masking to remove effects from extreme climate events. The temporal variability in mangrove to open water and mangrove gain presented in (Figures 3A, F) may be potentially minimized after modeling tidal effects. The tidal masking helps delineate better shorelines (Bishop-Taylor et al., 2021) to update mangrove change trajectories. Our accuracy assessment shows that urban, and agriculture (primarily pasturelands) shows high uncertainty and low accuracy (see Supplementary). Although these classes have a small area proportion, adding urban more training data and other higher spatial resolution dataset might help track these minor transitions.
Future research in Colombia to track mangrove status from aerial and satellite imagery should incorporate the role of natural processes and the participation of communities and institutions (i.e., institutional framework) (Ostrom and Nagendra, 2006). Multidisciplinary approaches that consider social sciences are often missed, even if the role of local testimonies is known to be invaluable (e.g., Truth Commission - Forensic Architecture, 2021). Local community involvement highlight otherwise overlooked problems and provides insight into complex and unintended environmental and social aspects (Treviño and Murillo-Sandoval, 2021). Another relevant aspect is the role of causality. Future studies require regular ground validation and sensors to monitor river discharge, tracking sediments, weather stations, and sea-level rises. Although expensive, causality analysis disentangles specific processes that lead to better policy-making decisions. Additionally, forest structure is an important factor that can be detected from space to provide a more accurate estimate of carbon stocks (Simard et al., 2019b) and support assessment of mangrove vulnerability to certain drivers of mangrove degradation and loss. However, in situ ground data collection is needed to reduce uncertainty and provide better estimates to build blue carbon initiatives.The Landsat archive and the temporal smoothing profiles derived from LandTrendr are practical to track historical trends and inform mangrove gain and loss. However, discrimination of mangrove forests from subsistence agriculture, coca farming and small settlements could potentially be improved with better spatial, spectral and temporal resolutions offers by new local and global datasets such as UAVSAR and NISAR respectively. Finally, identifying governance opportunities through public-private partnerships is essential, given the need to keep carbon safe and reduce deforestation (Furumo and Lambin, 2020). Consequently, reviews highlighting the synergy between stakeholders provide crucial elements to extrapolate into other locationssuch as the efforts in Cispatá, the first long-term sustainable financing strategy in the Colombian Caribbean (Verra, 2021; Kuwae et al., 2022).
6 Conclusions
We produced a consistent high-resolution (30m) dataset to track annual mangrove extent change in Colombia from 1984 to 2020. Analysis of potential natural and human-induced drivers of change in three sites shows the response of the mangrove landscape. While many large mangroves remain unchanged, a 14% net decline in mangrove extent poses concern about their future status. Human activities such as gold mining, pasturelands expansion, and coca farming expansion can seriously affect mangrove cover. Colombia’s mangrove ecosystems include some of the largest and relatively undisturbed mangrove extensions in the Western Hemisphere and require consistent monitoring to support management strategies that consider hydrological, climatic, and socioeconomic factors.
The three sites selected in this study Cienaga Grande de Santa Marta, Gulf of Urabá, and Sanquianga, have been found to be profitable for blue carbon projects, and they could be financially sustainable based on current carbon prices over a 30-year time frame (Zeng et al., 2021). Despite the high carbon density in these locations, realistic conservation strategies are needed. For instance, the role of armed conflict processes, narcotrafficking, and local community participation is commonly ignored in these estimations. Moreover, the potential extent of mangrove blue carbon financing depends on a suite of conservation approaches and community participation that minimize current and future threats (Zeng et al., 2021).
Data Availability Statement
The datasets presented in this article are not readily available. However, all methods details are included in the article and Supplementary Material. Additional inquiries can be asked to the corresponding author. A GitHub repository with all code and examples to be tested at local and regional levels is available in https://paulomur.github.io/mangroves/. Requests to access the datasets should be directed to pjmurillos@ut.edu.co.
Author Contributions
PM-S conceptualization, methodology, writing-original draft; and LF and MS contributed to the writing, review, and supervision of the paper. All authors contributed to the article and approved the submitted version.
Funding
The United States Silva Carbon program provided funding. LF and MS were funded by SilvaCarbon and NASA’s LCLUC programs.
Conflict of Interest
The authors declare that the research was conducted in the absence of any commercial or financial relationships that could be construed as a potential conflict of interest.
Publisher’s Note
All claims expressed in this article are solely those of the authors and do not necessarily represent those of their affiliated organizations, or those of the publisher, the editors and the reviewers. Any product that may be evaluated in this article, or claim that may be made by its manufacturer, is not guaranteed or endorsed by the publisher.
Acknowledgments
We thank members of IDEAM (Gustavo Galindo and Andres Zuluaga) for early discussions about mapping changes strategy. We also thank Cheryl Doughty for her review and useful comments in the manuscript. Part of this work was performed at the Jet Propulsion Laboratory, California Institute of Technology, under contract with the National Aeronautics and Space Administration (NASA).
Supplementary Material
The Supplementary Material for this article can be found online at: https://www.frontiersin.org/articles/10.3389/fmars.2022.892946/full#supplementary-material
References
Alban J.D.T.De, Jamaludin J, de Wen D. W., Than M. M., Webb E. L.(2020). Improved Estimates of Mangrove Cover and Change Reveal Catastrophic Deforestation in Myanmar. Environ. Res. Lett. 15 (3), 34034. doi: 10.1088/1748-9326/ab666d
Ballvé T. (2013). Grassroots Masquerades: Development, Paramilitaries, and Land Laundering in Colombia. Geoforum 50, 62–75. doi: 10.1016/j.geoforum.2013.08.001
Baloloy A. B., Blanco A. C., Sta. Ana R. R. C., Nadaoka K(2020). Development and Application of a New Mangrove Vegetation Index (MVI) for Rapid and Accurate Mangrove Mapping. ISPRS J. Photogrammetry Remote Sens. 166, 95–117. doi: 10.1016/j.isprsjprs.2020.06.001
Bernal B., Sidman G., Pearson T. (2017). Assessment of Mangrove Ecosystems in Colombia and Their Potential for Emissions Reductions and Restoration. Arlington. Winrock International.
Bhargava R., Sarkar D., Friess D. A. (2021). A Cloud Computing-Based Approach to Mapping Mangrove Erosion and Progradation: Case Studies From the Sundarbans and French Guiana. Estuar. Coast. Shelf Sci. 248, 106798. doi: 10.1016/j.ecss.2020.106798
Bishop-Taylor R., Nanson R, Sagar S, Lymburner L (2021). Mapping Australia’s Dynamic Coastline at Mean Sea Level Using Three Decades of Landsat Imagery. Remote Sens. Environ. 267, 112734. doi: 10.1016/j.rse.2021.112734
Blanco J. F., Estrada E. A., Ortiz L. F., Urrego L. E.(2012). Ecosystem-Wide Impacts of Deforestation in Mangroves: The Urabá Gulf (Colombian Caribbean) Case Study. ISRN Ecol., 2012:1–14. doi: 10.5402/2012/958709
Blanco-Libreros J. F., López-Rodríguez S. R., Valencia-Palacios A. M., Perez-Vega G. F., Álvarez-León R(2022). Mangroves From Rainy to Desert Climates: Baseline Data to Assess Future Changes and Drivers in Colombia. Front. Forests Global Change 5. doi: 10.3389/ffgc.2022.772271
Blanco J. A., Viloria E. A., Narváez B J. C. (2006). ‘ENSO and Salinity Changes in the Ciénaga Grande De Santa Marta Coastal Lagoon System, Colombian Caribbean’. Estuarine Coast. Shelf Sci. 66 (1–2), 157–167. doi: 10.1016/j.ecss.2005.08.001
Botero L., Salzwedel H. (1999). Rehabilitation of the Cienaga Grande De Santa Marta, a Mangrove-Estuarine System in the Caribbean Coast of Colombia. Ocean Coast. Manage. 42 (2–4), 243–256. doi: 10.1016/S0964-5691(98)00056-8
Bunting P., Rosenqvist A, Lucas R. M., Rebelo L-M, Hilarides L, Thomas N, et al. (2018). The Global Mangrove Watch—A New 2010 Global Baseline of Mangrove Extent. Remote Sens. 10,1–19. doi: 10.3390/rs10101669
Castellanos-Galindo G. A., Casella E., Tavera H, Zapata Padilla L. A., Simard M (2021a). Structural Characteristics of the Tallest Mangrove Forests of the American Continent: A Comparison of Ground-Based, Drone and Radar Measurements. Front. Forests Global Change 4, 1–11. doi: 10.3389/ffgc.2021.732468
Castellanos-Galindo G. A., Kluger L. C., Camargo M. A., Cantera J, Mancera Pineda J. E., Blanco-Libreros J. F., et al. (2021b). Mangrove Research in Colombia: Temporal Trends, Geographical Coverage and Research Gaps. Estuarine Coast. Shelf Sci. 248. doi: 10.1016/j.ecss.2020.106799
Correa I., Morton R. (2010). Caribbean Coast of Colombia. Encyclopedia World’s Coast. Landforms 2010, 259–264. doi: 10.1007/978-1-4020-8639-7_41
de Jong S. M., Shen Y, de Vries J, Bijnaar G, van Maanen B, Augustinus P, et al. (2021). Mapping Mangrove Dynamics and Colonization Patterns at the Suriname Coast Using Historic Satellite Data and the LandTrendr Algorithm. Int. J. Appl. Earth Observat. Geoinform. 97, 102293. doi: 10.1016/j.jag.2020.102293
Fagua J. C., Ramsey R. D. (2019). Geospatial Modeling of Land Cover Change in the Chocó-Darien Global Ecoregion of South America; One of Most Biodiverse and Rainy Areas in the World. PLoS One 14 (2), e0211324. doi: 10.1371/journal.pone.0211324
Fatoyinbo T., Feliciano E. A., Lagomasino D, Lee S. K., Trettin C(2018). Estimating Mangrove Aboveground Biomass From Airborne {LiDAR} Data: A Case Study From the Zambezi River Delta. Environ. Res. Lett. 13 (2), 25012. doi: 10.1088/1748-9326/aa9f03
Furumo P. R., Lambin E. F. (2020). Scaling Up Zero-Deforestation Initiatives Through Public-Private Partnerships : A Look Inside Post-Con Fl Ict Colombia. Global Environ. Change 62, 13. doi: 10.1016/j.gloenvcha.2020.102055
Gilani H., Naz H. I., Arshad M, Nazim K, Akram U, Abrar A, et al. (2021). Evaluating Mangrove Conservation and Sustainability Through Spatiotemporal, (1990–2020) Mangrove Cover Change Analysis in Pakistan. Estuarine Coast. Shelf Sci. 249, 107128. doi: 10.1016/j.ecss.2020.107128
Giri C., Ochieng E, Tieszen L. L., Zhu Z, Singh A, Loveland T, et al. (2011). Status and Distribution of Mangrove Forests of the World Using Earth Observation Satellite Data. Global Ecol. Biogeogr. 20 (1), 154–159. doi: 10.1111/j.1466-8238.2010.00584.x
Goldberg L., Lagomasino D, Thomas N, Fatoyinbo T (2020). Global Declines in Human-Driven Mangrove Loss. Global Change Biol. 26 (10), 5844–5855. doi: 10.1111/gcb.15275
Gorelick N., Hancher M, Dixon M, Ilyushchenko S, Thau D, Moore R (2017). Google Earth Engine: Planetary-Scale Geospatial Analysis for Everyone. Remote Sens. Environ. 202, 18–27. doi: 10.1016/j.rse.2017.06.031
Grajales J. (2011). The Rifle and the Title: Paramilitary Violence, Land Grab and Land Control in Colombia. J. Peasant Stud. 38 (4), 771–792. doi: 10.1080/03066150.2011.607701
Guo Y., Liao J., Shen G. (2021). Mapping Large-Scale Mangroves Along the Maritime Silk Road From 1990 to 2015 Using a Novel Deep Learning Model and Landsat Data. Remote Sens. 13 (2), 1–20. doi: 10.3390/rs13020245
Hamilton S. E., Casey D. (2016). Creation of a High Spatio-Temporal Resolution Global Database of Continuous Mangrove Forest Cover for the 21st Century (CGMFC-21). Global Ecol. Biogeogr. 25 (6), 729–738. doi: 10.1111/geb.12449
Hamilton S. E., Castellanos-Galindo G.A ., Millones-Mayer M, Chen M (2018). “‘Remote Sensing of Mangrove Forests: Current Techniques and Existing Databases’,” in Threats to Mangrove Forests: Hazards, Vulnerability, and Management. Eds. Makowski C., Finkl C. W. (Cham: Springer International Publishing), 497–520. doi: 10.1007/978-3-319-73016-5_22
Hansen M. C., Potapov P. V., Moore R, Hancher M, Turubanova S. A., Tyukavina A. (2013). High-Resolution Global Maps of Forest Cover Change. Science 342 (6160), 850–853. doi: 10.1126/science.1244693
IDEAM (2010). Leyenda Nacional De Coberturas De La Tierra. Metodologia CORINE Land Cover Adaptada Para Colombia Escala 1:100000 (Bogota, Colombia: IDEAM). I. de H. M. y E. A. de C.
INVEMAR (2014). Informe Del Estado De Los Ambientes Y Recursos Marinos Costeros En Colombia Año 2014Instituto de Investigaciones Marinas y Costeras. (Santa Marta, Colombia). Available at: www.invemar.org.co.
INVEMAR (2020). Informe Del Estado De Los Ambientes Y Recursos Marinos Y Costeros En Colombia 2020, INVEMAR (Santa Marta: Serie de Publicaciones Periódicas No.3).
Jaramillo F., Licero L, Åhlen I, Manzoni S, Rodríguez-Rodríguez J. A., Guittard A, et al. (2018). Effects of Hydroclimatic Change and Rehabilitation Activities on Salinity and Mangroves in the Ciénaga Grande De Santa Marta, Colombia. Wetlands 38 (4), 755–767. doi: 10.1007/s13157-018-1024-7
Kennedy R. E., Ohmann J, Gregory M, Roberts H, Yang Z, Bell D. M., et al. (2018). An Empirical, Integrated Forest Biomass Monitoring System. Environ. Res. Lett. 13, 1–10. doi: 10.1088/1748-9326/aa9d9e
Kennedy R. E., Yang Z., Cohen W. B. (2010). Detecting Trends in Forest Disturbance and Recovery Using Yearly Landsat Time Series: 1. LandTrendr - Temporal Segmentation Algorithms. Remote Sens. Environ. 114 (12), 2897–2910. doi: 10.1016/j.rse.2010.07.008
Kuwae T., Watanabe A, Yoshihara S, Suehiro F, Sugimura Y (2022). Implementation of Blue Carbon Offset Crediting for Seagrass Meadows, Macroalgal Beds, and Macroalgae Farming in Japan. Mar. Policy 138, 104996. doi: 10.1016/j.marpol.2022.104996
Lee C. K. F., Duncan C, Nicholson E, Fatoyinbo T. E., Lagomasino D, Thomas N, et al. (2021). Mapping the Extent of Mangrove Ecosystem Degradation by Integrating an Ecological Conceptual Model With Satellite Data. Remote Sens. 13 (11), 1–19. doi: 10.3390/rs13112047
López-Angarita J., Roberts C. M., Tilley A, Hawkins J. P., Cooke R. G. (2016). Mangroves and People: Lessons From a History of Use and Abuse in Four Latin American Countries. For. Ecol. Manage. 368, 151–162. doi: 10.1016/j.foreco.2016.03.020
Luijendijk A., Hagenaars G, Ranasinghe R, Baart F, Donchyts G, Aarninkhof S (2018). The State of the World’s Beaches. Sci. Rep. 8 (1), 1–11. doi: 10.1038/s41598-018-24630-6
Lymburner L., Bunting P, Lucas R, Scarth P, Alam I, Phillips C, et al. (2020). Mapping the Multi-Decadal Mangrove Dynamics of the Australian Coastline. Remote Sens. Environ. 238, 111185. doi: 10.1016/j.rse.2019.05.004
Mejía J. F., Yepes J, Henao J. J., Poveda G, Zuluaga M. D., Raymond D. J., et al. (2021). Towards a Mechanistic Understanding of Precipitation Over the Far Eastern Tropical Pacific and Western Colombia, One of the Rainiest Spots on Earth. J. Geophysical Res.: Atmosph. 126 (5), e2020JD033415. doi: 10.1029/2020JD033415
Mejía-Rentería J. C., Castellanos-Galindo G. A., Cantera-Kintz J. R., Hamilton S. E. (2018). A Comparison of Colombian Pacific Mangrove Extent Estimations: Implications for the Conservation of a Unique Neotropical Tidal Forest. Estuarine Coast. Shelf Sci. 212, 233–240. doi: 10.1016/j.ecss.2018.07.020
Mentaschi L., Vousdoukas M. I., Pekel J, Voukouvalas E, Feyen L(2018). Global Long-Term Observations of Coastal Erosion and Accretion. Sci. Rep. 8 (1), 1–11. doi: 10.1038/s41598-018-30904-w
Muñoz-mora J. C., Johansson S. L., Giraldo-ramírez J, Fortou J. A.(2015). This Land is My Land : Understanding the Relationship Between Armed Conflict This Land Is My Land : Understanding the Relationship Between Armed Con. Work. Pap. ECARES ECARES 2015-17, ULB -- Univ. Libr. Bruxelles.
Murillo-Sandoval P. J., Van Dexter K, Van Den Hoek J, Wrathall D, Kennedy R. E., et al. (2020). The End of Gunpoint Conservation: Forest Disturbance After the Colombian Peace Agreement. Environ. Res. Lett. 15,1–12 (3). doi: 10.1088/1748-9326/ab6ae3
Murillo-Sandoval P. J., Gjerdseth E, Correa-ayram C, Wrathall D, Hoek J, Van Den, Davalos L. M., et al. (2021). No Peace for the Forest : Rapid , Widespread Land Changes in the Andes-Amazon Region Following the Colombian Civil War. Global Environ. Change 69, 1–12. doi: 10.1016/j.gloenvcha.2021.102283
Olofsson P., Foody G. M., Herold M, Stehman S. V., Woodcock C. E., Wulder M. A.(2014). Good Practices for Estimating Area and Assessing Accuracy of Land Change. Remote Sens. Environ. 148, 42–57. doi: 10.1016/j.rse.2014.02.015
Olofsson P., Holden C. E., Bullock E. L., Woodcock C. E.(2016). ‘Time Series Analysis of Satellite Data Reveals Continuous Deforestation of New England Since the 1980s’. Environ. Res. Lett. 6), 1–8. doi: 10.1088/1748-9326/11/6/064002
Olofsson P., Arévalo P, Espejo A. B., Green C, Lindquist E, McRoberts R. E., et al. (2020). Mitigating the Effects of Omission Errors on Area and Area Change Estimates. Remote Sens. Environ. 236, 111492. doi: 10.1016/j.rse.2019.111492
Ostrom E., Nagendra H. (2006). Insights on Linking Forests, Trees, and People From the Air, on the Ground, and in the Laboratory. Proc. Natl. Acad. Sci. 103 (51), 19224–19231. doi: 10.1073/pnas.0607962103
Palacios M. L., Cantera J. R. (2017). Mangrove Timber Use as an Ecosystem Service in the Colombian Pacific. Hydrobiologia 803 (1), 345–358. doi: 10.1007/s10750-017-3309-x
Parra A. S., Restrepo-Angel J. D. (2014). El Colapso Ambiental En El Rio Patia, Colombia: Variaciones Morfologicas Y Alteraciones En Los Ecosistemas De Manglar. Latin Am. J. Aquat. Res. 42 (1), 40–60. doi: 10.3856/vol42-issue1-fulltext-4
Pasquarella V. J., Holden C. E., Kaufman L, Woodcock C. E. (2016). From Imagery to Ecology: Leveraging Time Series of All Available Landsat Observations to Map and Monitor Ecosystem State and Dynamics. Remote Sens. Ecol. Conserv., 2, 1–19. doi: 10.1002/rse2.24
Pekel J.-F., Cottam A, Gorelick N, Belward A. S.(2016). High-Resolution Mapping of Global Surface Water and its Long-Term Changes. Nature 540 (7633), 418–422. doi: 10.1038/nature20584
Polidoro B. A., Carpenter K. E., Collins L, Duke N. C., Ellison A. M., Ellison J. C., et al. (2010). The Loss of Species: Mangrove Extinction Risk and Geographic Areas of Global Concern. PLoS One 5 (4), 1–10. doi: 10.1371/journal.pone.0010095
Restrepo A. J. D. (2012). Assessing the Effect of Sea-Level Change and Human Activities on a Major Delta on the Pacific Coast of Northern South America: The Patía River. Geomorphology 151–152, 207–223. doi: 10.1016/j.geomorph.2012.02.004
Riascos J. M., Cantera J. R., Blanco-Libreros J. F. (2018). Growth and Mortality of Mangrove Seedlings in the Wettest Neotropical Mangrove Forests During ENSO: Implications for Vulnerability to Climate Change. Aquat. Bot. 147, 34–42. doi: 10.1016/j.aquabot.2018.03.002
Roy D. P., Kovalskyy V, Zhang H. K., Vermote E. F., Yan L, Kumar S. S., et al 2016. Characterization of Landsat-7 to Landsat-8 Reflective Wavelength and Normalized Difference Vegetation Index Continuity. Remote Sens. Environ., 185, 57–70. doi: 10.1016/j.rse.2015.12.024
Ruiz-Luna A., Acosta-Velázquez J., Berlanga-Robles C. A. (2008). On the Reliability of the Data of the Extent of Mangroves: A Case Study in Mexico. Ocean Coast. Manage. 51 (4), 342–351. doi: 10.1016/j.ocecoaman.2007.08.004
Shahbudin S., Zuhairi A., Kamaruzzaman B. Y. (2012). Impact of Coastal Development on Mangrove Cover in Kilim River, Langkawi Island, Malaysia. J. Forest. Res. 23 (2), 185–190. doi: 10.1007/s11676-011-0218-0
Shepherd J. D., Dymond J. R. (2003). Correcting Satellite Imagery for the Variance of Reflectance and Illumination With Topography. Int. J. Remote Sens. 24 (17), 3503–3514. doi: 10.1080/01431160210154029
Simard M., Rivera-Monroy V. H., Mancera-Pineda J. E., Castañeda-Moya E, Twilley R. R.(2008). A Systematic Method for 3D Mapping of Mangrove Forests Based on Shuttle Radar Topography Mission Elevation Data, ICEsat/GLAS Waveforms and Field Data: Application to Ciénaga Grande De Santa Marta, Colombia. Remote Sens. Environ. 112 (5), 2131–2144. doi: 10.1016/j.rse.2007.10.012
Simard M., Fatoyinbo T, Smetanka C, Rivera-Monroy V, Castaneda-Mova E., Thomas N., et al. (2019a). ‘Global Mangrove Distribution, Aboveground Biomass, and Canopy Height’ ( ORNL Distributed Active Archive Center). doi: 10.3334/ORNLDAAC/1665
Simard M., Fatoyinbo T, Smetanka C, Rivera-Monroy V. H., Castaneda-Mova E., Thomas N., et al. (2019b). Mangrove Canopy Height Globally Related to Precipitation, Temperature and Cyclone Frequency. Nat. Geosci. 12 (1), 40–45. doi: 10.1038/s41561-018-0279-1
Suyadi Gao J., Lundquist C. J., Schwendenmann L. (2018). Sources of Uncertainty in Mapping Temperate Mangroves and Their Minimization Using Innovative Methods. Int. J. Remote Sens. 39 (1), 17–36. doi: 10.1080/01431161.2017.1378455
Tang W., Zheng M, Zhao X, Shi J, Yang J, Trettin C. C.(2018). Big Geospatial Data Analytics for Global Mangrove Biomass and Carbon Estimation. Sustainability. 10,1–17. doi: 10.3390/su10020472
Tassi A., Vizzari M. (2020). Object-Oriented LULC Classification in Google Earth Engine Combining SNIC, GLCM, and Machine Learning Algorithms. Remote Sens. 12,1–17. doi: 10.3390/rs12223776
Thampanya U., Vermaat J. E., Sinsakul S, Panapitukkul N (2006). Coastal Erosion and Mangrove Progradation of Southern Thailand. Estuarine Coast. Shelf Sci. 68 (1), 75–85. doi: 10.1016/j.ecss.2006.01.011
Thomas N., Lucas R, Bunting P, Hardy A, Rosenqvist A, Simard M (2017). Distribution and Drivers of Global Mangrove Forest Change 1996–2010. PLoS One 12 (6), 1–14. doi: 10.1371/journal.pone.0179302
Thomas N., et al. (2018). Mapping Mangrove Extent and Change: A Globally Applicable Approach. Remote Sens. 10 (9), 1–20. doi: 10.3390/rs10091466
Treviño M., Murillo-Sandoval P. J. (2021). Uneven Consequences: Gendered Impacts of Shrimp Aquaculture Development on Mangrove Dependent Communities. Ocean Coast. Manage. 210, 1–13. doi: 10.1016/j.ocecoaman.2021.105688
Truth Commission - Forensic Architecture (2021). in Dispossession and the Memory of the Earth: Land Dispossession in Nueva Colonia. Bogota, Colombia. Available at: https://forensic-architecture.org/investigation/land-dispossession-in-nueva-colonia.
UNODC (2017) Colombia Survey of Territories Affected by Illicit Crops – 2016’. Available at: https://www.unodc.org/documents/crop-monitoring/Colombia/Colombia_Coca_survey_2016_English_web.pdf.
Van doninck J., Tuomisto H. (2018). A Landsat Composite Covering All Amazonia for Applications in Ecology and Conservation. Remote Sens. Ecol. Conserv. 4 (3), 197–210. doi: 10.1002/rse2.77
Vélez-Castaño J. D., Betancurth-Montes G. L., Cañón-Barriga J. E. (2021). Erosion and Progradation in the Atrato River Delta: A Spatiotemporal Analysis With Google Earth Engine. Rev. Facultad Ingenieria 99), 83–98. doi: 10.17533/udea.redin.20200688
Verra (2021) Verra Has Registered Its First Blue Carbon Conservation Project. Available at: https://verra.org/.
Villate Daza D. A., Sánchez Moreno H, Portz L, Portantiolo Manzolli R, Bolívar-Anillo H. J., Anfuso G (2020). Mangrove Forests Evolution and Threats in the Caribbean Sea of Colombia. Water, 12,1–20. doi: 10.3390/w12041113
Xie S., Liu L., Yang J. (2020). Time-Series Model-Adjusted Percentile Features: Improved Percentile Features for Land-Cover Classification Based on Landsat Data. Remote Sens. 12(18):1–24. doi: 10.3390/rs12183091
Yang S. L., Li H, Ysebaert T, Bouma T. J., Zhang W. X., Wang Y. Y., et al. (2008). Spatial and Temporal Variations in Sediment Grain Size in Tidal Wetlands, Yangtze Delta: On the Role of Physical and Biotic Controls. Estuarine Coast. Shelf Sci. 77 (4), 657–671. doi: 10.1016/j.ecss.2007.10.024
Zambrano Escamilla C. H., Rubiano-Rubiano D. J. (1996). Memoria De Los Mapas De Los Bosques De Manglar Del Caribe Colombiano: 1996. Proy. PD 171/91 Rev. 2 (F) Fase I ‘Conservación Y Manejo Para El Usos Múltiple Y El Desarrollo De Los Manglares En Colombia’, MMA/OIMT. Tech. Rep. 8, 1–41 + 81 Cartas (1 :100000) (SantaFé de Bogotá: MINAMBIENTE).
Zanaga D., Van De Kerchove R, De Keersmaecker W, Souverijns N, Brockmann C, Quast R, et al. (2021). ESA WorldCover 10 M 2020 V100. doi: 10.5281/zenodo.5571936
Zeng Y., Friess D. A., Sarira T. V., Siman K, Koh L. P. (2021). Global Potential and Limits of Mangrove Blue Carbon for Climate Change Mitigation. Curr. Biol. 31 (8), 1737–1743.e3. doi: 10.1016/j.cub.2021.01.070
Zhang H. K., Roy D. P. (2017). Using the 500 M MODIS Land Cover Product to Derive a Consistent Continental Scale 30 M Landsat Land Cover Classification. Remote Sens. Environ. 197, 15–34. doi: 10.1016/j.rse.2017.05.024
Keywords: Landsat, Colombia, mangrove, Landtrendr, land cover change (LCC)
Citation: Murillo-Sandoval PJ, Fatoyinbo L and Simard M (2022) Mangroves Cover Change Trajectories 1984-2020: The Gradual Decrease of Mangroves in Colombia. Front. Mar. Sci. 9:892946. doi: 10.3389/fmars.2022.892946
Received: 09 March 2022; Accepted: 22 June 2022;
Published: 20 July 2022.
Edited by:
Catherine Lovelock, The University of Queensland, AustraliaReviewed by:
Rodolfo Rioja-Nieto, Rodolfo Rioja Nieto, MexicoAnusha Rajkaran, University of the Western Cape, South Africa
Copyright © 2022 Murillo-Sandoval, Fatoyinbo and Simard. This is an open-access article distributed under the terms of the Creative Commons Attribution License (CC BY). The use, distribution or reproduction in other forums is permitted, provided the original author(s) and the copyright owner(s) are credited and that the original publication in this journal is cited, in accordance with accepted academic practice. No use, distribution or reproduction is permitted which does not comply with these terms.
*Correspondence: Paulo J. Murillo-Sandoval, pjmurillos@ut.edu.co; Lola Fatoyinbo, lola.fatoyinbo@nasa.gov