Delineation of Eastern Beaufort Sea Sub-regions Using Self-Organizing Maps Applied to 17 Years of MODIS-Aqua Data
- Bedford Institute of Oceanography, Fisheries and Oceans Canada, Dartmouth, NS, Canada
Satellite observations are an integral component of long-term Arctic Ocean monitoring and help identifying changes resulting from climate warming. A Self-Organizing Maps (SOM) approach was applied to four-day composite satellite images of the Eastern Beaufort Sea (EBS) acquired by the MODerate resolution Imaging Spectroradiometer over the period 2003–2019. Using sea-surface temperature (SST), suspended particulate matter concentration (SPM) and chlorophyll-a concentration (Chl-a) as input the EBS was partitioned into six biogeochemical regions. The SOM approach revealed region-specific mean conditions and seasonal cycles for all properties, particularly for SPM and Chl-a. Three of the six regions, located on the continental shelf, had the highest SST, SPM and Chl-a with earlier maxima compared to the remaining three regions which comprised the shelf edge, Canada Basin and Amundsen Gulf. While mean and maximum SST did not exhibit significant trends over the 17 years of observations, the annual maximum SST in Amundsen Gulf was reached significantly earlier in recent years compared to the start of the time series. With the exception of Amundsen Gulf, sea-ice concentration (SIC) derived from microwave satellites declined throughout the study area; monthly trends showed dramatic SIC declines in regions on the shelf during May and June, and in Canada Basin during August. Correlation analysis of properties within and between regions showed that SST and SIC were driven by large scale processes while SPM and Chl-a showed regional features. SST and Chl-a in the regions nearest the Mackenzie River showed a strong relationship during seasonal warming. The SOM approach, applied to 17 years of satellite data, revealed spatially distinct marine units with unique characteristics, emphasizing the need for regional considerations when assessing the impact of climate warming in the Arctic Ocean.
1 Introduction
The Arctic is undergoing rapid changes as a result of climate forcing, including increasing air temperature [three to four times the rate of the Northern Hemisphere average; Serreze et al. (2009); Miller et al. (2010)], thawing of permafrost (Payette et al., 2004; Jorgenson et al., 2006), increasing cloudiness (Bélanger et al., 2013) and fall storminess (Ardyna et al., 2014). The Arctic Ocean, in particular, is experiencing major shifts to its sea ice regime, including a lengthening open-water season, reduction in sea ice thickness and extent, and disappearance of multi-year ice that propagate cascading effects on the entire marine ecosystem (Maslanik et al., 2011; Stroeve et al., 2012; Kwok and Cunningham, 2015). Due to the reduction in sea ice, the light field in the euphotic zone and conditions for phytoplankton growth have been impacted, with Arctic “spring” blooms starting earlier and ending later (Kahru et al., 2011; Kahru et al., 2016) at increasing latitudes (Renaut et al., 2018). As a result, an annual increase in net primary productivity of up to 30% over the period 1998 to 2012 in the Arctic has been documented (Arrigo and van Dijken, 2015). Furthermore, focusing on the Eastern Beaufort Sea (EBS) located in the western Canadian Arctic, the flux of SPM of terrestrial origin delivered by the Mackenzie River into the coastal ocean has dramatically increased in recent decades (Doxaran et al., 2015) driven by permafrost thaw, positive precipitation anomalies and increasing river discharge. These changes may also be delivering more contaminants into the EBS, which is concerning as polar environments, in particular, are very sensitive to changes (Macdonald et al., 1995; Macdonald et al., 2005).
Ocean colour satellite remote sensing is integral for monitoring climate change and its large-scale impacts occurring on the surface of the Arctic Ocean. Sensors mounted on satellites image a large spatial extent and allow long-term monitoring of particular biogeochemical and physical properties of the ocean surface. However, many studies are performed at the pan-Arctic scale (i.e., Ardyna and Arrigo (2020); Kahru et al. (2011); Kahru et al. (2016)) and may miss regional responses to local forcing, which lead to departures from trends recorded at large spatial scales. For instance, Screen (2017) discussed region-specific sea ice loss propagating errors when estimating loss of sea ice at large scales. Some studies have addressed this issue by partitioning the ocean into spatially discrete areas, also referred to as “ecological provinces” (Longhurst et al., 1995), using oceanographic data collected in situ or from satellite platforms. Ecological provinces were defined in the fundamental work of Longhurst et al. (1995), and in recent decades methods using satellite remote sensing of ocean colour and biogeochemical data have included delineation with dynamic boundaries (Devred et al., 2007; McIver et al., 2018; Reygondeau et al., 2018; Reygondeau et al., 2020), as well as objective and feature-based classifications (Martin Traykovski and Sosik, 2003; Oliver et al., 2004; Oliver and Irwin, 2008), principal component analysis (i.e., Hardman-Mountford et al., 2008), and neural gas methods (Taşdemir, 2011) to cite a few (see IOCCG, 2009).
The EBS and other areas of the Arctic Ocean have been neglected in previous ocean colour remote sensing studies delineating ecological provinces due to the challenges associated with remote sensing at high latitudes (IOCCG, 2015), such as sea ice cover, polar night, data loss from low sun angle, and poor performance of traditional ocean colour algorithms. In the current study, we focused on the EBS located in the Canadian Arctic to examine ecosystem characteristics and trends at a regional scale. Large geographic areas of the EBS have been designated Marine Protected Areas (MPAs) in recent years, with efforts to further classify hotspots and areas of ecological and biological significance using physical and biological data sources (DFO, 2015; Hodgson et al., 2015; Cobb et al., 2020). In the current study, we delineated biogeochemical regions using Self-Organizing Maps [SOMs; Kohonen (1984); Kohonen (1997)], a form of artificial neural network (ANN) well suited to non-linear datasets with missing data. SOMs have been applied in an extensive variety of studies from the medical field to biology and economics (Kaski et al., 1998) and in particular to study the ocean surface using satellite data to identify spatio-temporal patterns and relationships between variables (Ainsworth, 1999; Ainsworth and Jones, 1999; Hewitson and Crane, 2002; Richardson et al., 2003; El Hourany et al., 2019; Park et al., 2019; Xu et al., 2019), and estimate spatial fields (i.e., pCO2 in Xu et al. (2019) and net community production in Park et al. (2019)). We applied the SOM methodology to a remote sensing dataset of three essential climate variables (WMO, 2016) spanning 17 years (2003–2019) acquired by the MODerate resolution Imaging Spectroradiometer (MODISA): sea-surface temperature (SST), suspended particulate matter concentration (SPM) and chlorophyll-a concentration (Chl-a, proxy for phytoplankton biomass). In addition, we used sea ice concentration (SIC) from the Advanced Microwave Scanning Radiometer - Earth Observing System sensors (AMSRE and AMSR2) to help interpret our findings. By constructing regions in this manner, using satellite data and finding “natural” spatio-temporal groupings, we demonstrate an effective and unsupervised methodology that can be further integrated into marine spatial planning and investigating biogeochemical regions in the Arctic Ocean. The long time series of properties, provided a means to derive climatologies, seasonal cycles and trends in each region, as well as allow investigation of connectivity between the regions.
2 Materials and Methods
2.1 Study Area
The Beaufort Sea is a marginal sea of the Arctic Ocean spanning the coasts of the Yukon and Northwest Territories westward to northern Alaska (Figure 1). Our region of interest bounds the eastern (Canadian) portion of the Beaufort Sea, from 122° to 142° West and 68.5° to 72.5° North. There are two Marine Protected Areas (MPAs) in the area, namely the Tarium Niryutait MPA and Anguniaqvia Niqiqyuam MPA, along with terrestrial and coastal protected areas including the Kendall Island, Anderson River Delta and Banks Island No. 1 Migratory Bird Sanctuaries. At high latitudes during the summer the sun remains above the horizon from approximately May 19 to July 24 at the latitude of the Mackenzie Delta, and from May 9 to August 4 at the latitude of Banks Island.
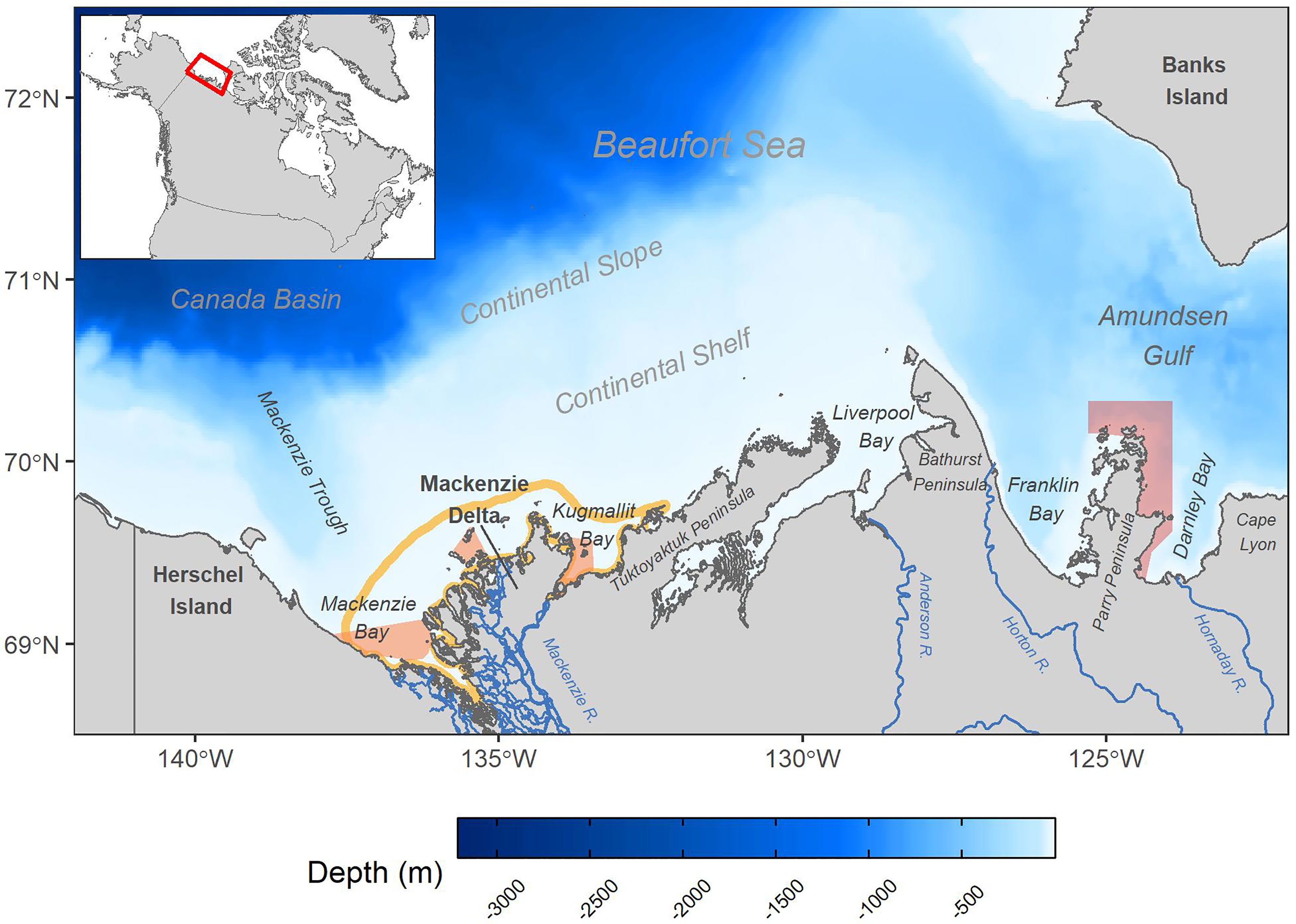
Figure 1 Study area in the Eastern Beaufort Sea shown with bathymetry, notable physical features and freshwater inputs, Marine Protected Areas (MPAs) and location on inset map of Canada. The area of raised cloud albedo processing is shown as an orange outline around the Mackenzie Delta (see section 2.2.1). Tarium Niryutait MPA is indicated in orange, and Anguniaqvia Niqiqyuam MPA in red.
The region encompasses a large bathymetric range and variety of oceanographic conditions, and includes the Mackenzie Delta, the continental shelf and slope, the southern portion of the Canada Basin, and Amundsen Gulf. The Mackenzie River, the fourth largest Arctic river by discharge [mean annual discharge ~ 9,299 m3 s–1, ECCC (2021)], enters the ocean via the Mackenzie Delta on the western side of the shallow and expansive shelf. The river significantly impacts the physical and optical properties of the EBS, delivering suspended particles, nutrients and coloured-dissolved organic matter (CDOM) from its large watershed (drainage area of ~ 1.787 million km2, Peucker-Ehrenbrink (2009)). The large freshwater input to the shelf (also referred to as “Mackenzie Shelf” due to its influence from and proximity to the Mackenzie River) makes it the most estuarine shelf in the Arctic (Macdonald et al., 1995). North of Mackenzie Bay the seafloor deepens into Mackenzie Trough, which extends north into Canada Basin, exceeding a depth of 3000 m, where the movement of water and ice are heavily influenced by the anti-cyclonic surface circulation of the Beaufort Gyre (Galley et al., 2013). The Bathurst Peninsula divides the eastern side of the continental shelf from Amundsen Gulf and physically impedes the eastward movement of the fresh, buoyant and sediment-laden plume from the Mackenzie River (Fissel et al., 2013).
The sea ice regime varies through the region, with perennial sea ice present in Canada Basin, while Amundsen Gulf has a variable inter-annual open water area (Arrigo and van Dijken, 2004). To the northeast of Cape Bathurst, as sea ice retreats in early June, a large open water area (flaw lead) expands and forms the Cape Bathurst polynya (Barber and Hanesiak, 2004). A landfast ice barrier forms in autumn on the Mackenzie Shelf within the 20-m isobath and persists through the winter. Leads form between this landfast ice and mobile offshore pack ice as ice movement is driven by winds, and ice ridges and rubble accumulate at the landfast interface (“stamukhi”) physically trapping fluvial discharge to the coastal ocean (Giovando and Herlinveaux, 1981; Macdonald et al., 1995; Fissel et al., 2013). The barrier melts then breaks up typically in June as the temperature warms and the Mackenzie River discharge peaks, and the river plume is then free to expand up to hundreds of kilometers from shore (Macdonald et al., 1995).
2.2 Satellite Data and Processing
2.2.1 MODIS-Aqua SST, SPM and Chl-a
The MODerate Resolution Imaging Spectroradiometer is an optical sensor on the Aqua platform (referred to hereafter as MODISA) which has been collecting data at 36 wavebands since late 2002; nine bands image in the visible through near infrared wavelengths for ocean colour observation (i.e. signal-to-noise ratio of >400; Qi et al. (2017)). All but seven bands collect data at 1–km spatial resolution, with two collecting data at 250-m resolution (bands 1–2) and five collecting data at 500-m resolution (bands 3–7). Its polar orbit increases the number of times high latitude regions are imaged in a given day compared to temperate regions; as solar elevation peaks during summer the Mackenzie Delta and EBS are imaged by MODISA up to seven times daily.
MODISA images intersecting the study region during the years 2003 through 2019 were acquired from the NASA Ocean Biology Processing Group website (OBPG; https://oceancolor.gsfc.nasa.gov) at Level-1A (L1A, unprocessed full-resolution instrument data). Due to low sun angles and the lack of daylight from late fall through boreal winter to early spring, combined with sea ice cover, images used in this study were confined from March 14th to October 1st of each year (year-day 73 to 274, 202 days per year), corresponding to dates with a noon solar zenith angle of <70° at 68.5°N (IOCCG, 2015). Images acquired prior to 16:30 UTC were removed to restrict the sun angle and the descending node. L1A images were processed to Level-2 (L2, derived geophysical variables) at 250-m spatial resolution to retrieve remote sensing reflectance (Rrs) and SST using the SeaWiFS Data Analysis System (SeaDAS v7.5.2.1, https://seadas.gsfc.nasa.gov, l2gen processor) with the Wang and Shi (2007) NIR-SWIR switching algorithm to correct for atmospheric signal. The 250-m resolution was used to ensure maximum spatial coverage near the Mackenzie Delta and to decrease the influence of sub-pixel ice floes on bio-optical retrievals.
A similar processing methodology to Doxaran et al. (2015) was used for retrieving additional pixels near the outlet of the Mackenzie River, where high sediment concentrations resulted in data loss when using standard atmospheric correction methods due to pixel brightness at infrared wavelengths which trigger ice and cloud masks. In brief, the area adjacent to the Mackenzie Delta was re-processed using a relaxed cloud albedo threshold (standard threshold of 0.027 replaced with 0.4; see orange contour on Figure 1), provided that a processed scene had sufficient valid ocean pixels (minimum 5000 pixels at 250-m resolution). We updated the area used in Doxaran et al. (2015), limiting this “high albedo region” to the 20 g m–3 contour identified from a climatology layer constructed with the SPM product from the standard albedo processing (see Figure S1 for an example of improved spatial coverage).
The Rrs obtained from the standard and re-processed images were combined to calculate SPM and Chl-a, while daytime long-wave SST (11 μm) was calculated with l2gen. The SPM and Chl-a algorithms were implemented using python version 3.7.4 (Van Rossum and Drake, 2009).
2.2.2 Algorithms Used for SPM and Chl-a
Given the large dynamic range of SPM in the study area (i.e., < 1.0 g m-3 to > 100.0 g m–3) we used the semi-analytical algorithm of Han et al. (2016), an algorithm parameterized with in situ data from the global ocean including 38 samples collected in the Beaufort Sea. The method relies on Rrs (667) where SPM is calculated as the weighted average of two algorithms (one for low-medium SPM and one for high SPM waters):
where low (SPML) and high (SPMH) SPM were calculated following:
and
When Rrs are between 0.03 and 0.04 sr-1 the following equations are used for the weights WL and WH:
and
If a pixel Rrs≤0.03 sr−1, WL is set to 1 and WH is set to 0. If Rrs≥0.04 sr−1, WL is set to 0 and WH is set to 1. Note that ρw=π×Rrs.
Chlorophyll-a concentration (Chl-a, mg m–3) was computed using the Arctic Ocean empirical band-ratio algorithm (AOEmp; Lewis and Arrigo (2020)), which relies on Rrs at 443, 488 and 547 nm. The algorithm was developed using the largest in situ dataset yet assembled for the Arctic Ocean from 34 expeditions that occurred between 1994 and 2016. Like the global OC3M Chl-a algorithm (O’Reilly et al., 1998), AOEmp is a band-ratio algorithm with updated coefficients [see Lewis and Arrigo (2020)]. In summary, Chl-a was calculated as the following:
and
Where a = 0.0957, b = -2.7973, c = 0.6581 and R is the log10-transformed blue-green maximum band ratio. In areas with high SPM, i.e., near the Mackenzie Delta, Chl-a may be over-estimated due to the large sediment load and high concentration of yellow substances.
Following calculation of SPM and Chl-a, additional steps were taken to ensure data quality and create temporal composites. Pixels with negative Rrs at 555 and 667 nm or with SST < -1.89°C were discarded. SPM range of variation was limited from 0.05 – 1000.00 g m–3 and Chl-a pixels were restricted to the range 0.05 – 40.00 mg m-3. All SST, SPM and Chl-a images were projected on a 300-m regular grid using Generic Mapping Tools software (GMT, Wessel et al. (2013); https://www.generic-mapping-tools.org/). All individual scenes from a given day (including standard and raised cloud albedo processing) were combined into daily composite images by calculating the median value at each pixel. Finally, daily composite images were visually inspected to ensure that abnormal values were removed, then aggregated into four-day median composites (grouping by day of year, i.e., days 1 – 4, days 5 – 8, etc.) to reduce the impact of cloud cover while retaining an acceptable temporal resolution to resolve short term events.
A total of 13,325 L1A scenes from 2003 to 2019 were downloaded and processed among which 5006 were re-processed with a raised cloud albedo threshold near the outlet of the Mackenzie River. The resulting 2565 daily composites were aggregated into 740 four-day composites for each parameter. All analyses were performed using R software version 4.0.2 (R Core Team, 2019). ETOPO1 global relief data was used for bathymetry (Amante and Eakins, 2009), and hydrometric data from the Water Survey of Canada station at Arctic Red River [Station ID 10LC014; ECCC (2021)] during the study period were accessed using the weathercan R package LaZerte and Albers, 2018).
2.2.3 AMSRE and AMSR2 Sea Ice Concentration
SIC data from 2003 to 2019 were downloaded from the Universität Bremen website (https://seaice.uni-bremen.de/data; see Table S1) for two sensors: the Advanced Microwave Scanning Radiometer - Earth Observing System (AMSRE, 3.125-km resolution), which operated late 2002 to late 2011, and it’s successor, the AMSR2 sensor, which began operations in late 2012.
The AMSRE and AMSR2 images were re-gridded using GMT to the 300-m grid corresponding to the SST, SPM and Chl-a data, and aggregated into four-day composites using the mean SIC at each grid cell. Pixels with SIC of <10% were discarded as in Perrette et al. (2011). We investigated bridging the gap in coverage between AMSRE and AMSRE2 (2011-10-03 to 2012-07-04) with data from the Special Sensor Microwave Imager Sounder (SSMIS, data at https://www.nsidc.org), but its coarse resolution (i.e., 25-km) prevented access to reliable data along the coast, so 2011 and 2012 were excluded from our SIC analysis.
2.3 Delineating Regions With Self-Organizing Maps
The kohonen R package (version 3.0.10, Wehrens and Buydens, 2007; Wehrens and Kruisselbrink, 2018), based on the original SOM methodology developed by Kohonen (1997), was used to partition the ESB into areas with similar temporal dynamics of SST, SPM and Chl-a using the four-day composites from all three parameters simultaneously as input. SOMs have been applied to a large variety of studies, and are well suited for pattern identification in large satellite datasets as they perform effectively even with a high percentage of missing data [i.e., Richardson et al. (2003)]. We implemented SOM processing using the supersom() function in the R kohonen package, resulting in a map showing pixels with high temporal similarity of concurrent SST, SPM and Chl-a assigned to the same groups.
Before running the SOM some pre-processing steps were taken. SPM and Chl-a were log10-transformed to obtain a normal distribution (Campbell, 1995), and the three input datasets were each centered and scaled (i.e. each value of each column had the column mean removed and were divided by the column standard deviation) to have a consistent range of values. Four-day composites with low spatial coverage (<5%) and individual pixels with valid data in less than 5% of the images were removed (see Figure S2 for spatial and temporal coverage). Finally, to improve speed, reduce small gaps between pixels (i.e., Moiré pattern) and reduce ice-edge contamination of the SPM data due to straylight at the NIR wavelengths, we reduced the spatial resolution to 1.5-km, where each reduced pixel represented the median of at least five high resolution (i.e., 250-m) pixels. The SST, SPM and Chl-a datasets were arranged into three spatio-temporal matrices, which were concatenated together for SOM processing in order to consider all three parameters simultaneously (providing a final dataset of 98,844 rows corresponding to each pixel in the area of interest, and 635 columns corresponding to the time-series time step for all three parameters; Figure 2).
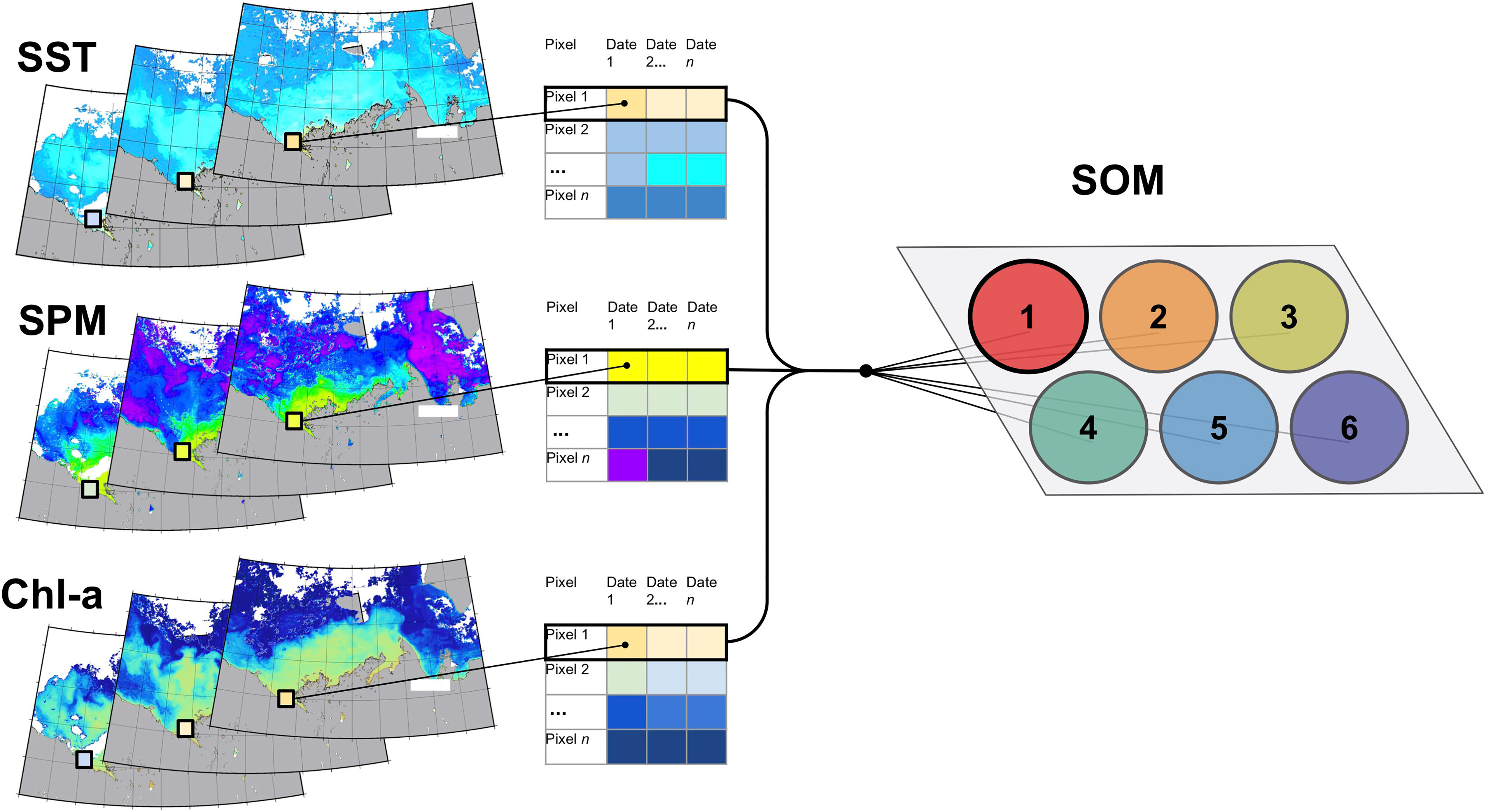
Figure 2 Illustration of the SOM method clustering satellite-derived SST, SPM and Chl-a pixels. The input parameters were concatenated, and spatial pixels were individually presented to SOM nodes, which includes all values of that pixel over time for the three parameters. Each pixel is examined for similarity with the other pixels assigned to that node. This recurs over a set number of iterations with the final characteristics of the node consisting of a weighted average of the assigned pixels and variables over time. Here the example pixel is assigned to Node 1.
The first stage of processing was defining an appropriate number of groups to partition the study area into. SOM processing requires an x, y layout of nodes (see Figure 2) with data assigned to each node (i.e., SST, SPM and Chl-a pixels assignedto a 2 x 3 node array results in six groups). We tested a variety of node array sizes in hexagonal layouts. During processing, each node is initially assigned a random subset of the input dataset (i.e., each node starts with a “prototype pattern” of SST, SPM and Chl-a), then pixels are iteratively presented to the node layout and assigned to the node with greatest similarity. Once a pixel is assigned to a node, the node’s “prototype pattern” is updated by computing a weighted average of all assigned pixels. The weight declines as processing progresses with an increasing number of iterations (i.e., times the pixel is presented to the node layout) so that the mean node distance converges. SOM processing maintains similarity between nodes, such that the nodes adjacent to a “winning node” (in the original x, y layout - see Figure 2) are also updated during the beginning of the processing as the map learns which pixels fit into which groups most effectively (see Kohonen, 1997; Wehrens and Buydens, 2007, for details). Each pixel retains a measure of fit to its assigned node called node “distance” (unitless) which can be used to assess the fit of individual pixels to each node. As the process is iterative, with each pixel repeatedly presented to the node array, the mean node distance declines and eventually stabilizes. To assess the optimal layout for the SOM, we processed node layouts of differing sizes (ranging from 3 nodes to 36 nodes) and examined the mean node distance for each region and parameter; in all layouts it stabilized after 400 iterations. Overall, the mean node distance was lowest using a 2 x 3 SOM layout (six nodes), which was analyzed further. Once the spatial information of each pixel were reassigned (i.e., latitude and longitude), the SOM classification revealed six distinct regions arranged in spatially consistent and quasi-contiguous areas. The regional boundaries were converted to shapefiles and used to extract the corresponding four-day composite SIC, SST, SPM and Chl-a pixels.
2.4 Regional Statistics of SST, SPM, Chl-a and SIC
2.4.1 Regional Time Series, Climatology and Trends
For all parameters (i.e., SST, SPM, Chl-a and SIC), climatology and standard deviation (SD) maps were computed for the entire time series (i.e. summarizing 2003-2019 per pixel). The arithmetic mean and SD were used for SST and SIC, and the geometric mean (Equation S1) and geometric standard deviation (GSD; Equation S2) for SPM and Chl-a (Kirkwood, 1979) due to the lognormal distribution of these data [i.e., Campbell (1995)]. As the GSD is a unitless multiplicative factor, unlike the SD which is added or subtracted from the mean when describing uncertainty, the lower and upper bounds of non-transformed data were obtained by dividing (Equation S3) or multiplying (Equation S4) the geometric mean by the GSD (See Supplementary Material).
Time series and climatologies of the parameters in the six regions defined by the SOM were calculated in a similar manner (i.e. arithmetic mean for SST and SIC, and geometric mean for SPM and Chl-a). First, the time series were calculated by averaging the SST, SPM, Chl-a and SIC pixels in individual regions for each four-day time step. Second, we averaged the four-day composites across all years to provide an average climatology of 2003-2019 for each four-day time step. When calculating the four-day time series and climatologies for a given parameter and region, the upper and lower percentiles of pixels were removed prior to calculating mean and SD to reduce the impact of possible outliers. The number of valid pixels in a four-day composite image changed in time and space due to the spatial variability of open-water area (i.e., ice-free), as well as data missing from cloud cover and low sun angle; here composites with less than 5% spatial coverage in a given region were excluded from the analysis (see Figure S2B).
In order to calculate the temporal trends of the four parameters in the SOM-defined regions, the SST, SPM, Chl-a and SIC data were aggregated further into monthly and annual mean values (again using arithmetic mean for SST and SIC, and geometric mean for SPM and Chl-a, removing the upper and lower 1% of data). Trends of SST, SPM, Chl-a and SIC for each region from 2003–2019 were calculated for these time resolutions using linear least-squares regressions of a given variable against time (function lm() in R). Only trends (i.e., slopes of the linear regression) with p-value less than 0.05 are discussed further. In addition to annual and monthly SST trends, we examined the trends of annual maximum SST and the trends of the dates at which it was reached.
2.4.2 Intra- and Inter-Regional Correlations of Satellite-Derived Parameters
To examine the relationships between all four parameters within and between all six regions, a correlation matrix of the four-day time series was computed for all possible combinations of the parameters. The Spearman rank correlation coefficient (p) showed the strength of correlation between parameters and regions, with significance indicated by accompanying p-value calculated from a two-tailed t-test of each correlation (function corr.test() in R package psych). Given that we analysed four parameters in six regions, we ended up with a total of 276 correlation coefficients.
The response of phytoplankton to physical forcing as expressed by SST was further investigated in terms of regional annual warming and cooling. The date of maximum SST in a given year (section 2.2.1) was used to divide the year into a warming period (i.e., first day of satellite data to day of warmest SST) and a cooling period (i.e., day of warmest SST to last day of satellite data recorded in the year). For both warming and cooling periods, the time series of Chl-a geometric mean were linearly regressed against SST using a Type-2 major axis regressions (R package lmodel2), retaining the slope, intercept, R2 and p-value for each region.
3 Results
3.1 Spatial Climatology of SOM Input Parameters: SST, SPM and Chl-a
Over the period 2003–2019, the SST climatology was warmest near the mouth of the Mackenzie River with a mean temperature of approximately 10°C (Figure 3A). It varied between 6 and 8°C in the nearby bays and coastal shelf waters from Cape Bathurst to the west of the Mackenzie River. A plume with SST ranging from 4 to 6°C extended northeast from the Mackenzie River toward the Canada Basin. The cold Alaskan Current was evident to the west of Herschel Island where the waters were cooler (<4°C) compared to its eastern side. The nearshore waters of Banks Island on the eastern side of the study region, lacking a shallow shelf and inputs from large rivers, had a similar SST climatology to open water areas with values lower than 5°C. The climatological SST was lowest in the surface waters of Canada Basin. SST showed the highest SD near the Mackenzie Delta with values ranging between 4 and 5°C (Figure 3B). The continental shelf and the Canadian Basin showed also a large SD with values between 2 and 4°C while Amundsen Gulf exhibited the least variation.
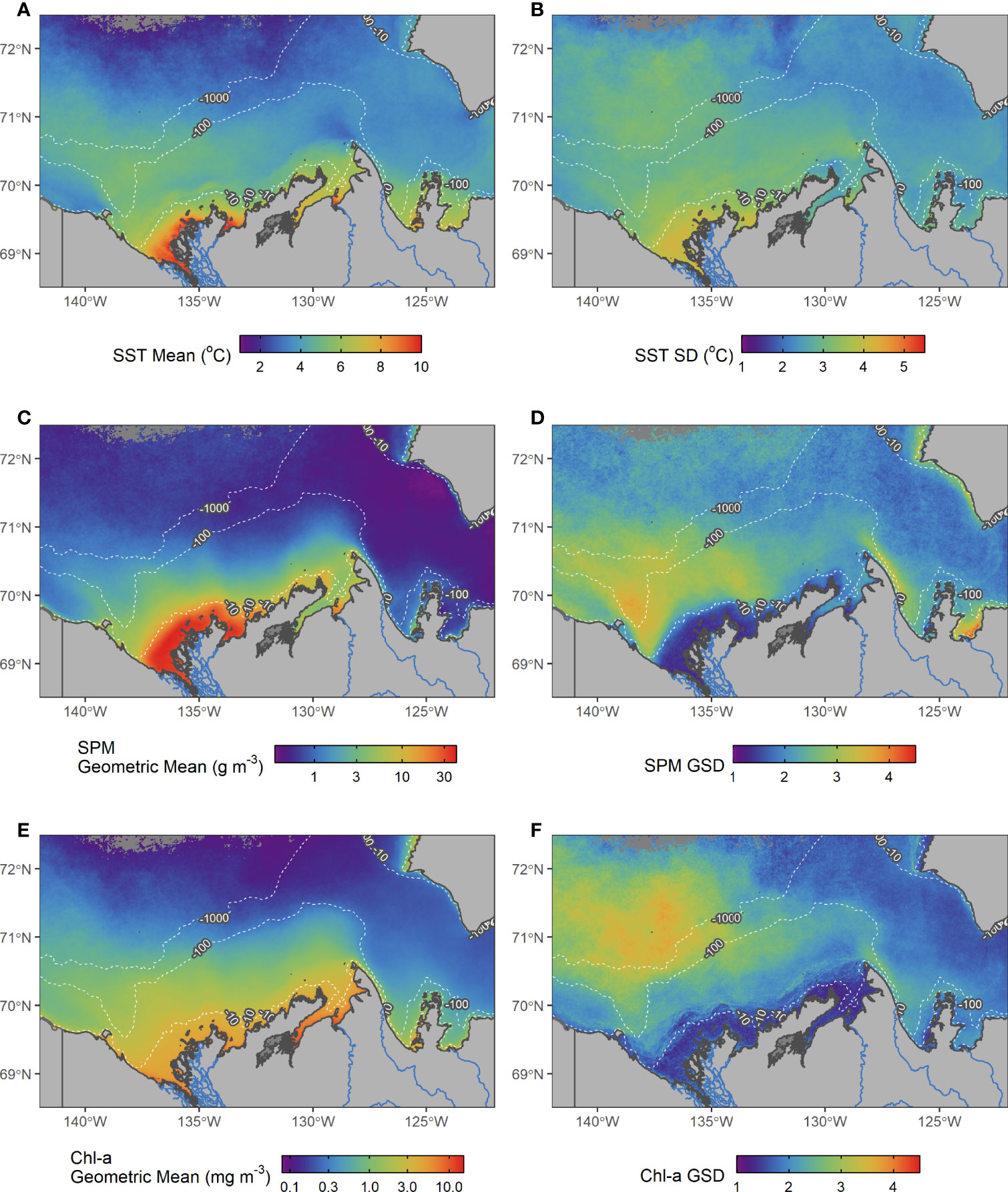
Figure 3 Mean and SD maps of SST (A, B), SPM (C, D) and Chl-a (E, F) for the time period 2003–2019 (note different colour scales). The GSD used for SPM and Chl-a is unitless. The 10, 100 and 1000 meter bathymetric contours are shown as dashed white lines. Dark grey ocean pixels indicate areas with inadequate data.
Similar to SST, the climatology of SPM was highest at the direct outlet of the Mackenzie River, exceeding 30 g m–3 within the 10-m bathymetric contour, and was greater than 20 g m–3 at the Anderson River outlet in Liverpool Bay (Figure 3C). SPM decreased moving north from the Mackenzie Delta and shallow coastal waters. Overall, SPM remained very low in Amundsen Gulf (<2 g m–3) and northwest off the shelf extending into the surface waters of the Canada Basin. The SPM GSD near the Mackenzie River was lower than that of Amundsen Gulf and Canada Basin. A “wedge” near the outlet of the Mackenzie River on the western side of Mackenzie Bay demonstrated high variability (highest GSD), likely caused by spatially constrained landfast ice that breaks up during spring and releases a plume with high particle concentration (Figure 3D). Further east, the SPM GSD was also elevated on the eastern side of Cape Bathurst, north of the outlet of the Horton River, in Darnley Bay near the Hornaday River, and along the southwest coast of Banks Island.
On average, Chl-a over the shelf waters was greater than Chl-a on the continental slope and Canada Basin, remaining on average above 1 mg m–3 for depths <100 m. In waters off the shelf (>1000 m), surface waters showed both latitudinal and longitudinal gradients; extending from the shelf west of approximately 135°W, Chl-a remained greater than 0.5 mg m–3, while east of 135°W, spreading into Amundsen Gulf, Chl-a remained lower than 0.5 mg m–3, and continued decreasing moving northward to deeper waters (Figure 3E). The GSD of Chl-a was highest at the shelf edge north of the Mackenzie River, extending over the Canada Basin (more than three times the mean, Figure 3F). The GSD was also elevated along the shelf edge (i.e., 100-m isobath) and eastern side of Cape Bathurst continuing southward to Franklin Bay. The GSD was low (i.e., less than two times the mean) off the shelf east of Canada Basin and into Amundsen Gulf. The GSD was also very low on the shelf where bathymetry was less than 20 m.
3.2 Mean Properties of the SOM-Delineated Regions
The six regions identified with the SOM approach (Figure 4) retained similar features to the ones revealed by the SST, SPM and Chl-a climatology and SD maps (Figure 3). The SOM-delineated regions successfully distinguishing the outlets of large rivers (mainly the Mackenzie and Anderson; Region 1), shallow inner shelf, mid-shelf and shelf-edge waters (Regions 2 to 4), Amundsen Gulf (Region 5) and the Canada Basin (Region 6). The six regions had a large range of size and extent, though they remained congruent with bathymetric features; Region 1, closest the Mackenzie River was smallest and shallowest, and Region 6 which overlapped much of Canada Basin was largest and deepest and covered a large depth range (Table 1).
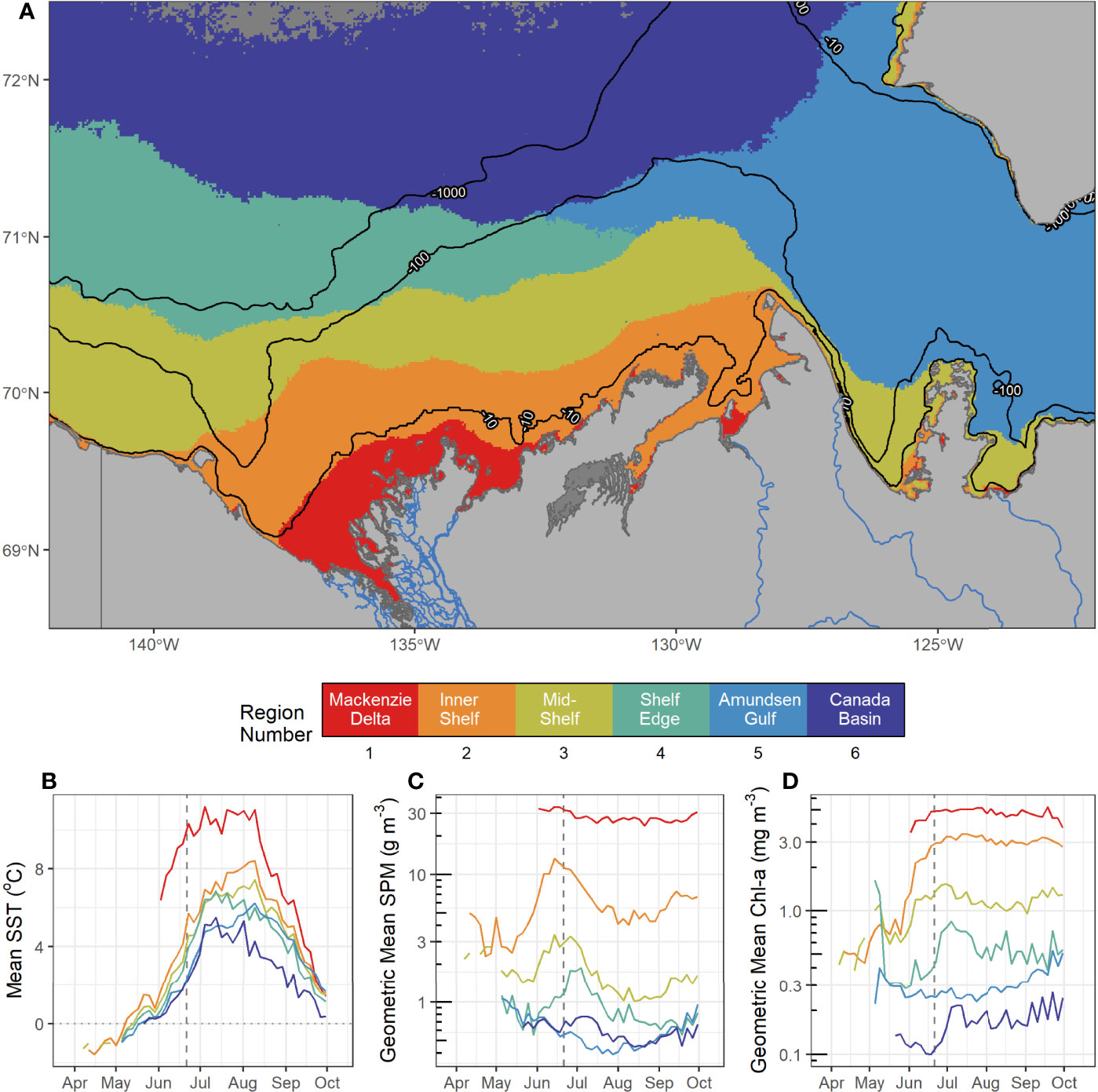
Figure 4 Six biogeochemical regions defined by the SOM analysis of satellite SST, SPM and Chl-a for the period 2003–2019 (A). The solid black lines correspond to the 10-m, 100-m and 1000-m bathymetric contours. The middle inset indicates the biogeochemical region and its name. The four-day climatologies of SST (B), SPM (C) and Chl-a (D) are presented for each region with the grey dashed line indicating the date of the summer solstice (i.e., June 21st). Ocean pixels indicated in grey did not contain enough data and were not included in the analysis.
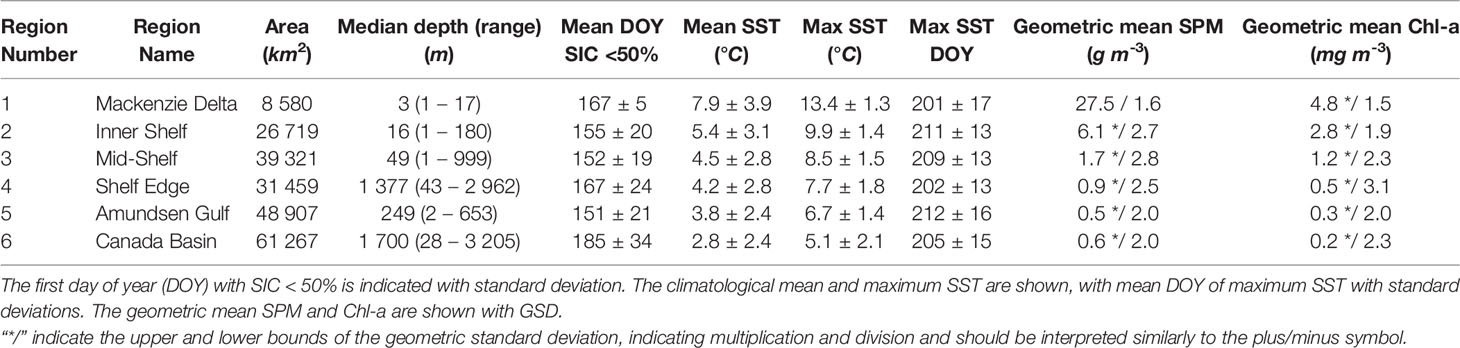
Table 1 Descriptive statistics from 2003–2019 of the six SOM regions, including the area, median depth and depth range of each region.
Region 1, referred to hereafter as the Mackenzie Delta or R1, was the warmest biogeochemical region with a mean SST value of 7.9°C (Table 1) and SST remaining above 8°C in Summer (Figure 4B) with a peak at 13.4°C in late summer (day of year 201 ± 17). SPM was also highest in this region with values greater than 20 g m–3 all year long and the annual maximum occuring soon after ice breakup (i.e, >30 g m–3, Figure 4C). In spring the phytoplankton biomass increased rapidly, but with a delayed timing compared to that of SPM (Figure 4D). Chl-a reached values above 3 mg m–3 in late June and remained high through the year (geometric mean of 4.5 mg m–3). This area exhibited persistent sea ice cover until mid-June after which it rapidly declined to entirely open water (Figure 5).
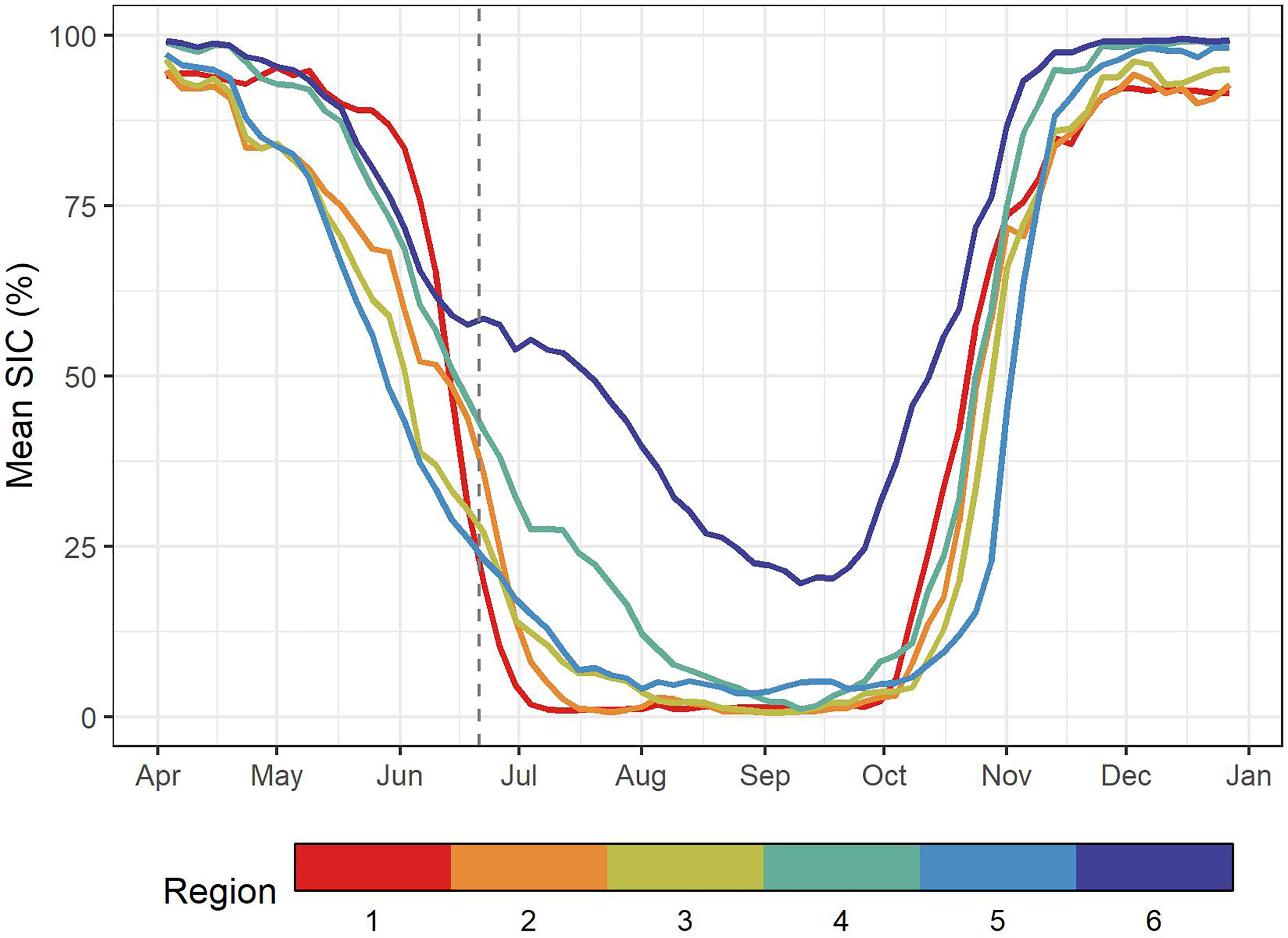
Figure 5 The mean SIC variation from April to January averaged over the period 2003 to 2019 (excluding 2011 and 2012). The vertical dashed line indicates the summer solstice.
Regions 2 and 3, referred to as the inner shelf (R2) and mid-shelf (R3) regions respectively, were mostly located within the 100-m bathymetric contour (Figure 4A). The inner shelf region spanned between Herschel Island on its west side and Cape Bathurst at the east (see Figure 1 for place names). The mid-shelf region (R3), and a small part of the inner shelf (R2), extended over the deep waters (i.e. deeper than 100-m) of the Mackenzie Trough, Franklin and Darnley Bays as well as on the west coast of Banks Island (Figure 4A). Region 4, corresponding to the shelf edge (R4), had its eastern boundary on the Mackenzie Shelf north of the Tuktoyaktuk Peninsula, and it extended west over deep waters of Canada Basin. It was bordered by Amundsen Gulf (R5) on its eastern side and the Canadian Basin (R6) on its northern side.
In agreement with the climatology, SST within the three shelf regions north of the Mackenzie River (R2 through R4) exhibited a northward gradient with the inner shelf being the warmest (R2: 5.4° ± 3.1°C) and shelf-edge the coldest (R4: 4.2° ± 2.8°C; Table 1, Figure 4B). The SST maxima in these regions were reached later in the year compared to the Mackenzie Delta (R1), with peak temperatures reached in July for the shelf-edge (R4: day of year 202 ± 13), and in August for the mid-shelf (R3: day of year 209 ± 13) then inner shelf (R2: day of year 211 ± 13). The SPM annual cycle in these three regions followed a similar pattern with latitudinal gradient (Figure 4C), with a rapid increase in SPM early in the year that peaked between mid-June in the inner (R2 maximum: 13.3 g m–3 on June 14, DOY 165) and mid- shelf regions (R3 maximum: 3.4 g m–3 on DOY 165), to early July in the shelf edge region (R4 maximum: 1.9 g m–3 on June 26, DOY 177), after which SPM declined through the summer and exhibited moderate increases in concentration again in September in all three regions (Table 1). Mean SPM on the inner shelf (R2 mean: 6.05 g m–3) was 3.7 times higher than the mean SPM on the mid-shelf (R3: 1.65 g m–3), and 6.7 times higher than the shelf edge (R4: 0.90 g m–3; Figure 4). Chl-a reached its peak in these regions at the beginning of July a few weeks later than the SPM peaks, with the inner shelf exceeding 3.0 mg m–3 most of the summer (R2 mean of 2.80 mg m–3; Figure 4D), while the mid-shelf remained above 1 mg m–3 following its peak (R3 mean of 1.16 mg m–3), and the shelf edge remained below 1.0 mg m–3 (R4 mean of 0.53 mg m–3). On the mid-shelf (R3) and shelf edge (R4) regions (Figure 4D), high phytoplankton biomass immediately occurred following sea ice retreat in agreement with the known sea ice dynamics of the region and early open water season (Figure 5). These events were also evident in the four-day composite images (Figure 6), which revealed the open water area and associated high phytoplankton biomass located between offshore pack ice and landfast ice. SIC declined earlier (i.e., April) on the shelf, mid-shelf and shelf-edge regions (R2 to R4) compared to the Mackenzie Delta (R1; Figure 5).
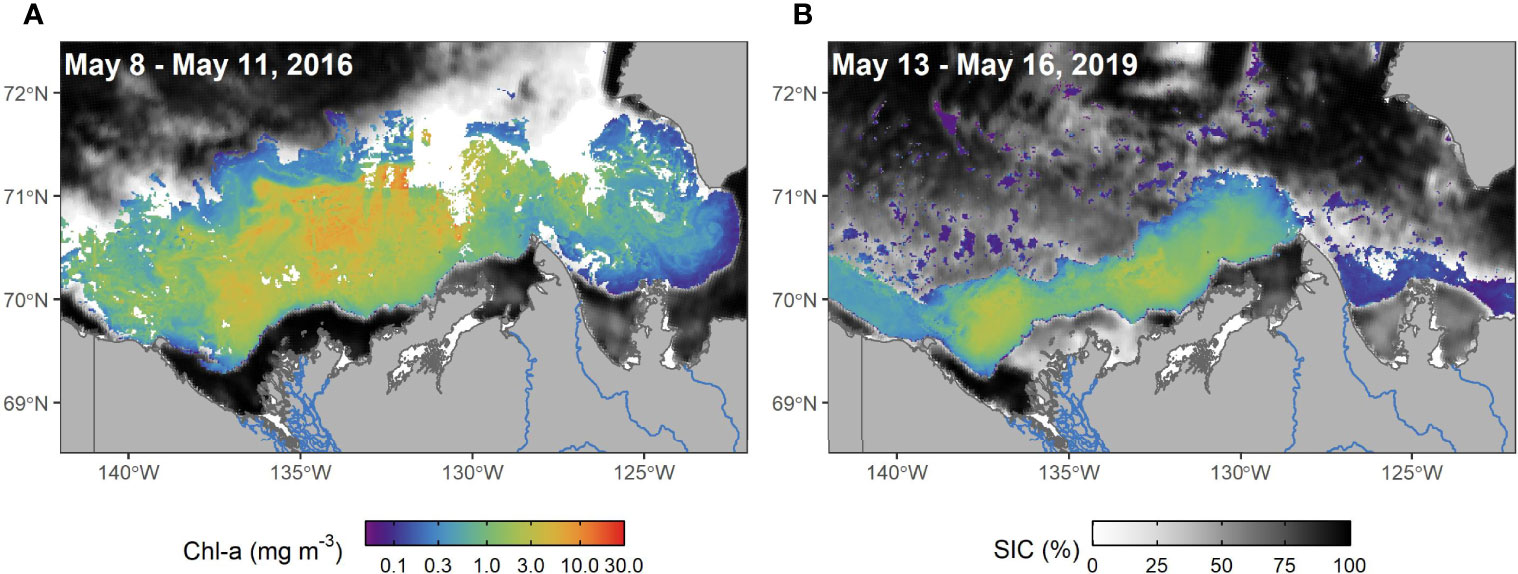
Figure 6 Four-day composite images of SIC and Chl-a showing flaw lead phytoplankton blooms during May. The geometric mean of Chl-a is shown from May 8-11, 2016 (A) and May 13-16, 2019 (B).
Amundsen Gulf (R5) and Canada Basin (R6) regions exhibited properties typical of offshore oceanic waters [i.e., Case 1, as opposed to coastal, Morel and Prieur (1977)]. Amundsen Gulf (R5) contained the eastern side of the study area and protruded onto the continental shelf between the Canada Basin (R6) and the continental shelf edge (R4, Figure 4A). This narrow protrusion of R5 west onto the shelf was consistently delineated in all SOM layouts (i.e., any number of nodes, results not shown). The four-day SST cycles of both regions were characterized by rapid warming from May through early July (approximately two weeks following the summer solstice). Canada Basin (R6) reached a maximum mean SST of 5.1°C on July 24 (DOY 205; Table 1). Amundsen Gulf (R5) continued warming until July 31 (maximum SST of 6.7°C, DOY 212), but at a slower rate compared to early summer, while the Canada Basin SST declined following its peak (Figure 4B). The SPM seasonal cycles were similar for the two regions, with a slight decrease following the SIC decline in early May, followed by an increase in SPM at the end of the summer (Figure 4C). The Canada Basin (R6) exhibited a peak SPM in July that was absent in Amundsen Gulf (R5), though both regions had the lowest geometric mean SPM of all six regions (R5: 0.54 and R6: 0.59 g m–3, Table 1). Both regions also exhibited the lowest Chl-a (R5: 0.29 and R6: 0.16 mg m–3 respectively), but with differing phenology; two Chl-a peaks occurred in Amundsen Gulf, the first at the beginning of the open-water season (i.e., May) and the second one in the fall (September), while Chl-a remained fairly low and stable through the summer. On the other hand, Chl-a in the Canada Basin showed relatively low concentration from late spring to early summer (< 0.15 mg m–3), followed by a slight increase to about 0.22 mg m–3 at the beginning of July and remained > 0.15 mg m–3 for the rest of the open-water season (Figure 4D).
Differences in mean SIC between regions were evident (Figure 5). The Mackenzie Delta region (R1), subject to landfast ice, showed a rapid decline in SIC from about 90% in May to no sea ice by early July as the ice barrier melted and was exported by fluvial discharge. Flaw leads, open-water formation between landfast and sea-ice, generally occurred early in the year (May-June) between Herschel Island and Cape Bathurst and were evident in the inner (R2) and mid- shelf (R3) regions after which SIC dropped below 10% by mid-July. Compared to R2 and R3, SIC on the shelf edge (R4) declined for a longer period of time, with little to no sea ice present by August. Amundsen Gulf (R5) showed a similar pattern to the shelf regions (R2 – R4) in terms of SIC seasonal cycle but with a delay in freeze-up timing. Finally, the Canada Basin (R6) exhibited persistent sea ice through the year, though high inter-annual variability (see Figure S6) reaching a climatological minimum of 20% in mid-September.
The years 2006, 2012 and 2013 were notable in the time series. In 2012 record high SST was present in the adjacent outer shelf (R4) and Canada Basin (R6) regions (See Figure S3). SST was most pronounced in the Canada Basin where SST reached 10.4°C on July 31st. The years 2006 and 2013 exhibited anomalously high SPM in R2, R3, R4 and R6, and high Chl-a in R4 and R6 (see Figures S4 and S5) and corresponded to years with highest level (2006) and discharge (2013) measured at the Water Survey of Canada hydrometric station at Arctic Red River [ID: 10LC014; ECCC (2021)] over the same time period.
3.3 Regional Annual and Monthly Trends
We examined annual trends from 2003 to 2019 for SST, SPM, Chl-a and SIC across the six regions. Interestingly, only one significant annual SPM trend was found, namely, SPM in the Canada Basin with a decreasing trend of 0.31 ± 0.13 g m–3 decade–1, with declines not observed in SST or Chl-a (Table 2). On the other hand, most annual SIC trends were significant and negative, with the most dramatic decline occurring on the shelf edge (R4, -8.4 ± 3.5% decade–1) followed by the mid-shelf (R3, -7.7 ± 2.2% decade–1). The exceptions were Amundsen Gulf (R5) and Canada Basin (R6), where no significant annual trends in SIC were found.
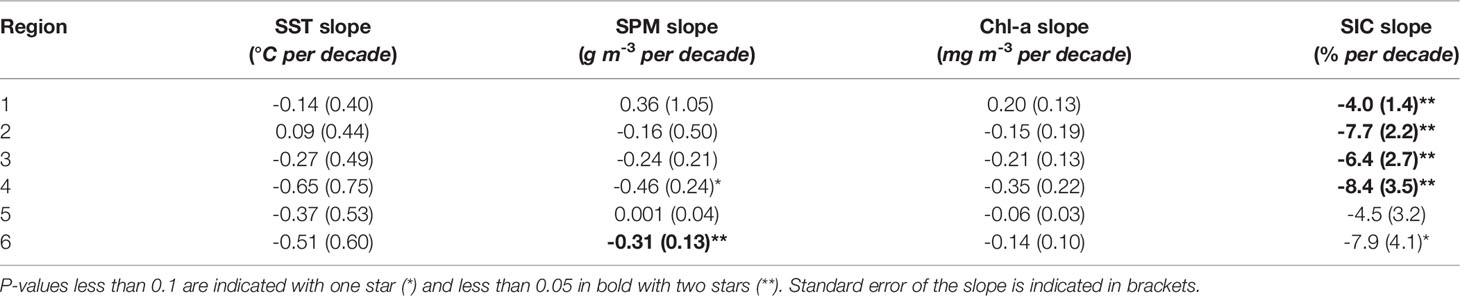
Table 2 Annual mean trends of SST, SPM, Chl-a and SIC for the six regions over the period 2003-2019, with slope expressed as change per decade. (°C per decade).
Monthly mean trends provided insights to seasonal changes over the period of observation (Table 3). We examined monthly trends for May through September in SIC, SST, SPM and Chl-a across all six regions. As the month of April had low data coverage due to sea ice cover, regions with fewer than 10 years of data were removed. Out of the 135 possible trends only 12 were significant at the 0.05 level. This included a cooling on the inner shelf in April (SST trend of -0.61 ± 0.21°C decade–1), a decrease in SPM in the Mackenzie Delta in June (-2.88 ± 1.15 g m–3 decade–1), and SPM declines both on the shelf edge (-0.88 ± 0.40 g m–3 decade–1) and Canada Basin (-0.44 ± 0.15 g m–3 decade–1) in August. Two significant Chl-a trends were observed in May on the inner (0.43 ± 0.18 mg m–3 decade–1) and mid- shelf (0.34 ± 0.13 mg m–3 decade–1). SIC trends were generally negative, with a decline in May on the entire shelf (R1 – R3): Mackenzie Delta: -5.7 ± 1.8% decade–1, inner shelf: -23.7 ± 7.4% decade–1 and mid-shelf: -26.8 ± 9.5% decade–1. The declined observed in May for the inner shelf continued into June with a negative trend of -20.1 ± 7.6% decade–1. Finally, SIC in the Canada Basin declined later in the year in August (-19.8 ± 8.0% decade–1).
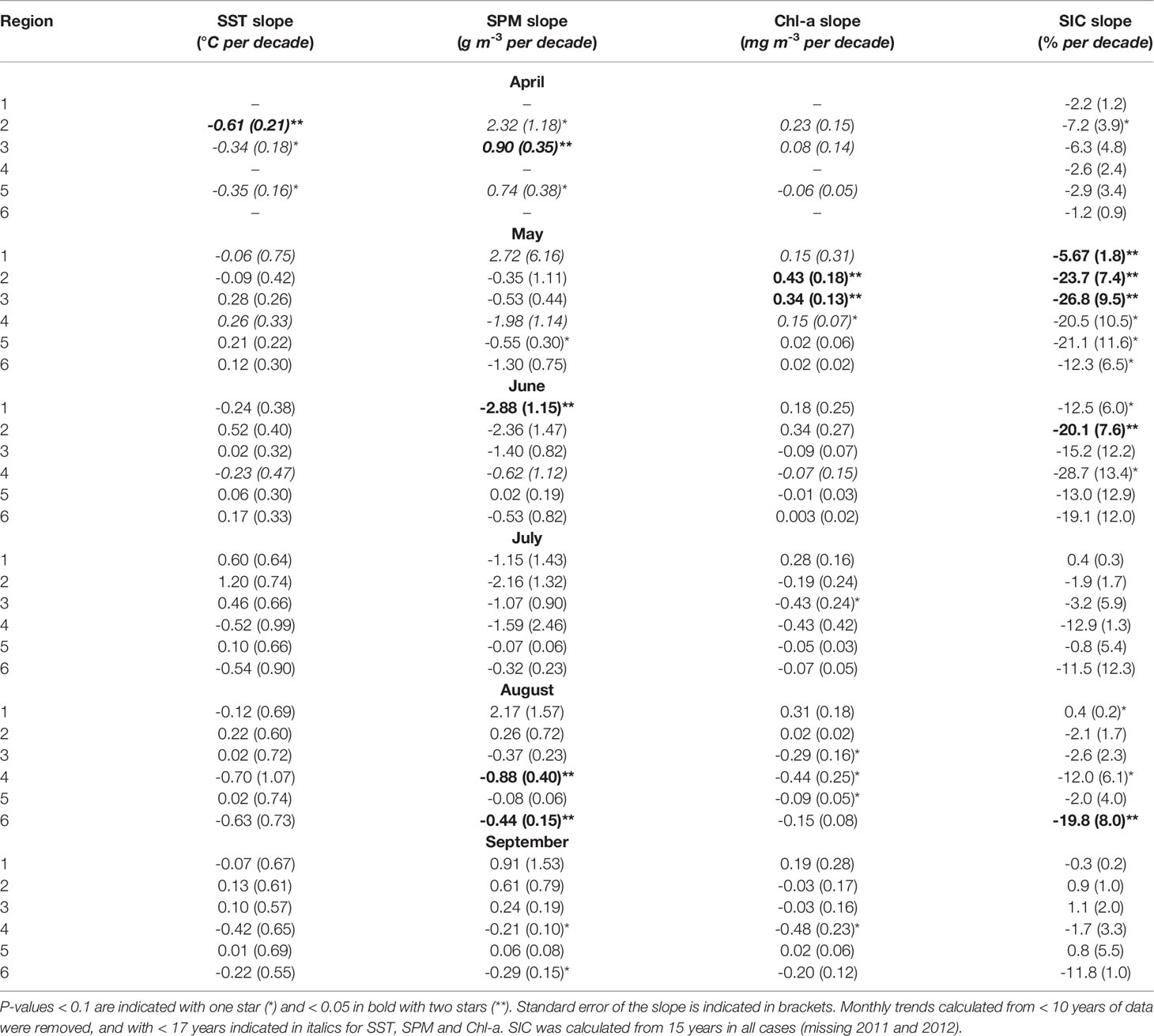
Table 3 Monthly mean trends of SST, SPM, Chl-a and SIC for the six regions over 2003–2019, with slope expressed per decade.
3.4 Time Series Correlations Between Properties and Regions
3.4.1 Correlation Analysis of the Same Property Between Regions
The correlation matrix of the 24 four-day time series (i.e., four properties for six regions) provided information on underlying processes and the connectivity between regions (Figure 7). In general, when considering properties individually, SST and SIC showed the highest correlation between regions, with ρ values ranging from 0.49 to 0.94 for SST and from 0.26 to 0.83 for SIC. The SST ρ remained high between spatially adjacent regions (>0.75) and decreased as regions were more physically separated, reaching a minimum of ρ = 0.49 between the Mackenzie Delta (R1) and Amundsen Gulf (R5) regions. The SIC ρ values followed the same pattern as SST but with lower magnitude.
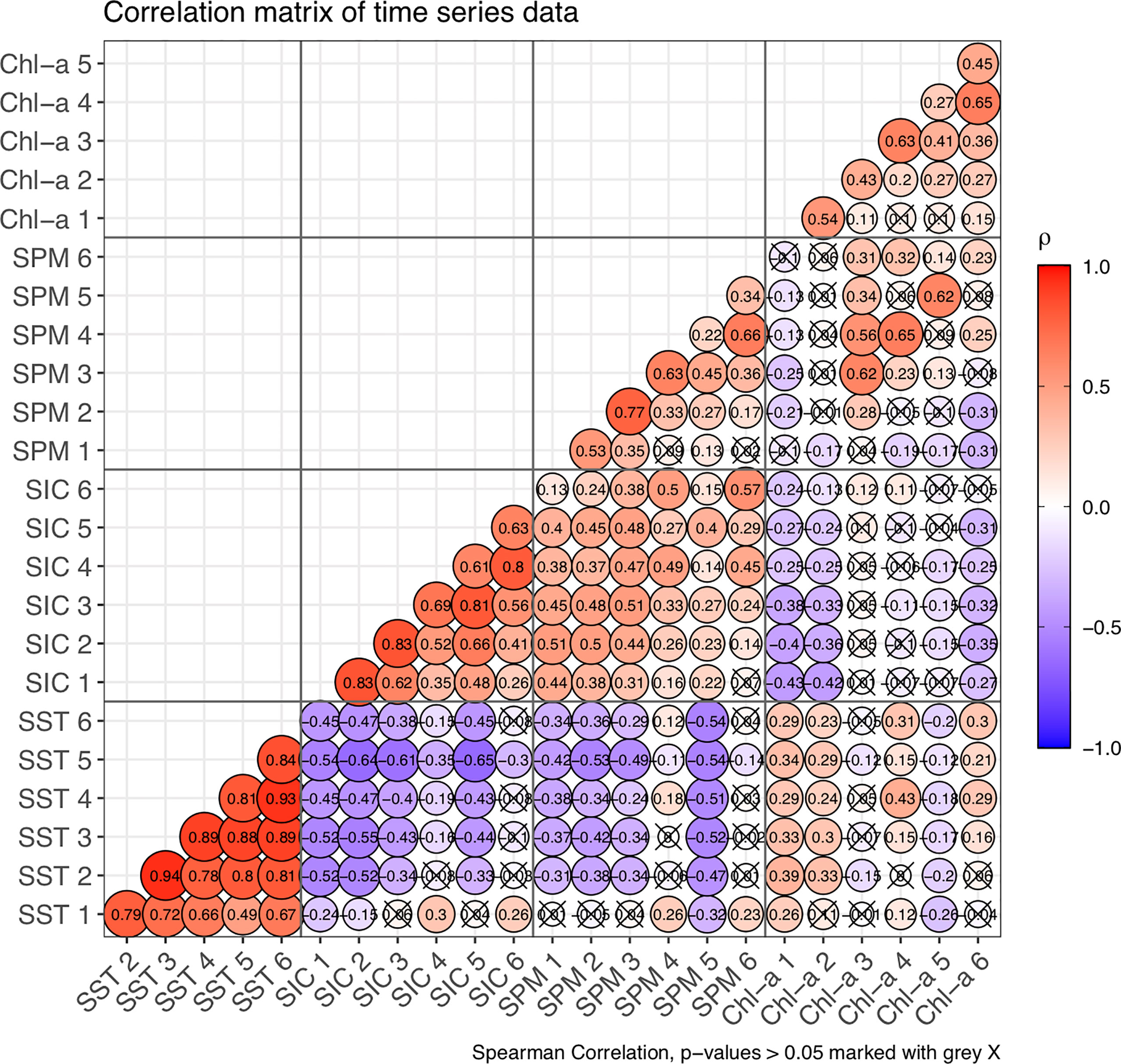
Figure 7 Correlation matrix of time series between parameters, with parameter and region number indicated (i.e., SST 1 is SST of Region 1). The size of the bubble indicates the significance (low p-value corresponds to large bubble; p-value > 0.05 covered by a grey “X”) and colour indicates the Spearman correlation coefficient (ρ).
The SPM and Chl-a ρ values between regions were overall lower and fewer were significant than for SST and SIC. The SPM time series had moderate to high p values between the four regions on the continental shelf (R1 – R4, ρ > 0.53) when directly adjacent, and between the Canada Basin and shelf edge (R6 and R4: ρ = 0.66). The Amundsen Gulf SPM time series was correlated to the SPM time series on the mid-shelf region (p = 0.45) and in a lesser extent to the Canada Basin (ρ = 0.34). Finally, Chl-a time series showed moderate correlation (ρ > 0.4) only between physically connected regions, the correlation coefficient decreasing to < 0.3 when regions did not share common borders. No correlations between regions were found for the SPM and Chl-a time series when the regions were furthest apart (i.e., the Mackenzie Delta and Canada Basin).
3.4.2 Correlation Analysis Between Differing Properties
The correlation analysis showed more complex patterns when comparing differing properties across the SOM regions (Figure 7). Since SST was highly correlated between regions (section 3.4.1), and Chl-a or SPM were correlated to SST within the same region, both SST and Chl-a had a moderate to high degree of correlation with the SST time series in all the other regions. For instance, SPM and SST were negatively correlated within Amundsen Gulf (R5, ρ = 0.45); given the high SST correlation in R5 with the SST in all the other regions, SPM in Amundsen Gulf was negatively correlated with the SST in all regions.
SST and SIC were negatively correlated across the majority of regions, with some exceptions. The time series of SST in the Mackenzie Delta (R1) was positively correlated with SIC on the shelf edge (R4) and Canada Basin (R6) regions, with a weak negative correlation to the SIC on the inner shelf (R2), and no correlation to SIC on the mid-shelf (R3) and Amundsen Gulf (R5). The Canada Basin SIC was only weakly positively correlated with SST in the Mackenzie Delta, and slightly negatively correlated with Amundsen Gulf SST.
In general, SST (SIC) and SPM were negatively (positively) correlated with ρ varying between -0.25 (0.24) and -0.54 (0.57). The absence of correlation between SST and SPM occurred in the same regions where no correlations were found between SST and SIC (for instance, the SST time series in the Mackenzie Delta (R1) against the SPM time series on the inner and mid- self regions).
The SST and Chl-a time series showed a slight to moderate degree of correlation in the Mackenzie Delta (ρ = 0.26), the inner shelf (i.e., ρ = 0.33), the shelf edge (i.e., ρ = 0.43) and the Canada Basin (ρ = 0.30) but none on the mid-shelf and Amundsen Gulf regions (ρ = -0.12). The time series of SPM and Chl-a were moderately correlated in the mid-shelf region (R3, ρ = 0.62), shelf edge (R4, ρ = 0.65) and Amundsen Gulf (R5, ρ = 0.62).
The relationships between SST and Chl-a during the warming and cooling periods were also analysed for each region as a Type-II linear regression, in an attempt to assess the impact of physical forcing on primary producers (Figure 8). In general, Chl-a increased with SST during the warming period (Figure 8), but no relationship between SST and Chl-a was found during seasonal cooling (see Figure S7). During warming, SST explained 76, 61, 28 and 33% of variance in Chl-a in the Mackenzie Delta (R1), inner and mid-shelf (R2 and R3), and the shelf edge (R4) regions respectively. The Canada Basin (R6) region also exhibited a positive significant relationship between SST and Chl-a (R2= 0.30) but no significant relationship was found in Amundsen Gulf.
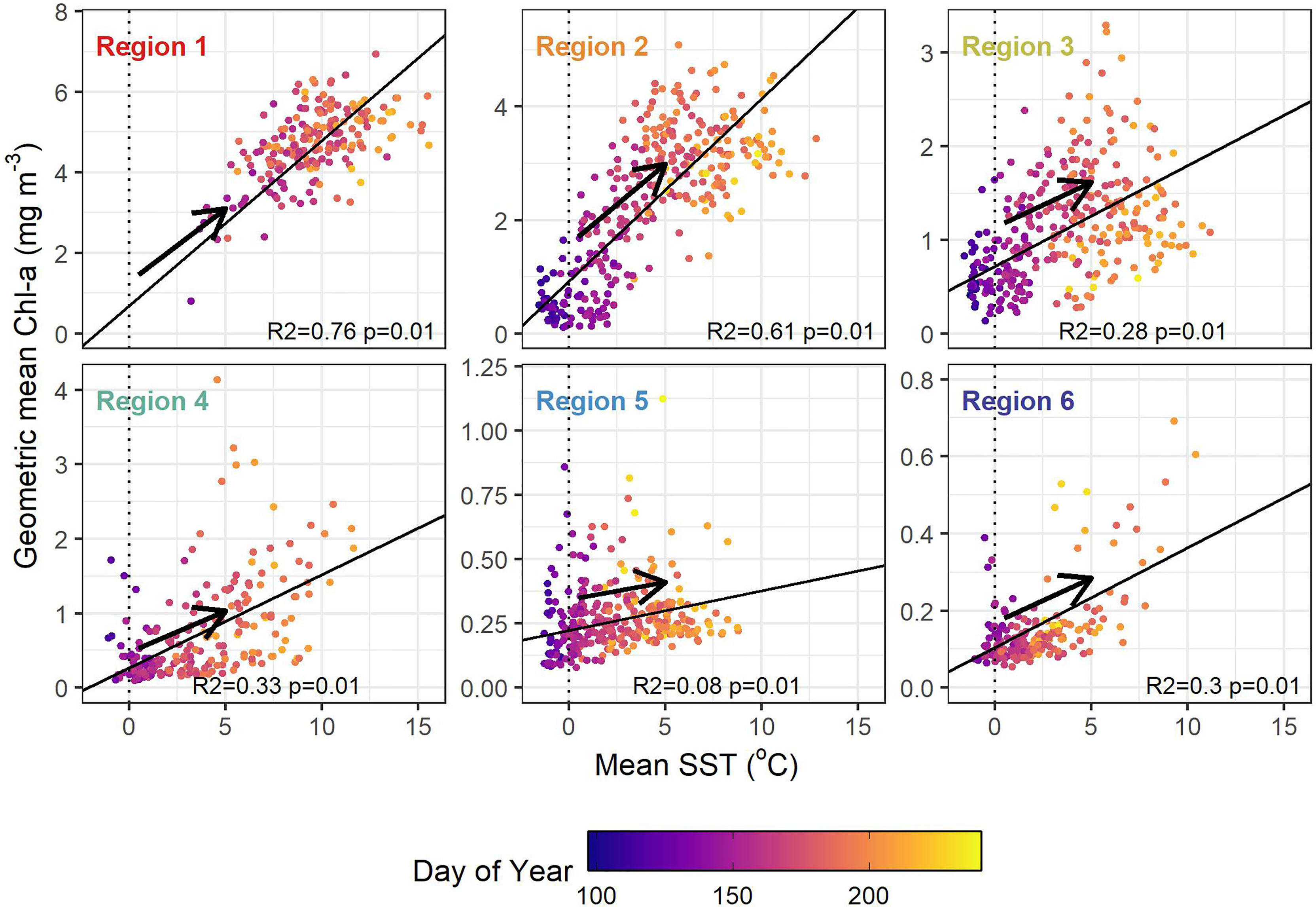
Figure 8 Chl-a and SST regression for time series values from annual warming (dates preceding the maximum annual SST value for all years). Points are coloured by the day of year, with arrows indicating the general temporal progression.
4 Discussion
The SOM approach to identify spatio-temporal patterns of SST, SPM and Chl-a over 17-years, combined with the climatology maps (Figure 3), statistics (Table 1, Figure 4), trends (Table 2) and correlation matrices (Figure 7), provided detailed information on the biogeochemical regimes of the surface EBS (Figure 4). By dividing the EBS into six biogeochemical regions, perhaps losing the fine spatial scale at which to examine trends (i.e. pixel-by-pixel changes), we reduced the negative impacts of missing data on our metrics (Cole et al., 2012) and used a robust method to group pixels with similiar temporal characteristics of SST, SPM and Chl-a.
The SOM-delineated regions were generally spatially continuous and congruent with bathymetry and sea ice regimes, though SIC and pixel information (i.e., lat, lon, bathymetry) were not input during the classification procedure. To some extent, information on sea ice presence was inherently included in the MODIS-Aqua imagery (i.e., SST, SPM and Chl-a), as no data from the sea surface were acquired by optical satellite when sea ice was present. However, the presence of clouds, which also prevented data acquisition, would mitigate some bias caused by ice cover on the results, as each pixel contained a differing number of observations over time. The regions were also arranged roughly by bathymetry, i.e. the Mackenzie Delta region (R1) closely followed the 10-m isobath while the eastern side of the inner shelf (R2) coincided with the 1000-m isobath, and the relationship between shallow bathymetry and SIC presence in these regions was evident as a reduced number of valid data available in the time series.
Most regions identified using the SOM approach were generally in agreement with other comprehensive classifications from the area, including Ecologically and Biologically Significant Areas (EBSAs) in the Western Arctic and bioregional Eco-units (DFO, 2015; Hodgson et al., 2015), constructed using physical datasets of multiple trophic levels of the ecosystem including marine mammal and benthos species distributions. For example, the northern boundary of the inner shelf region (R2; see Figure 4A), which corresponded with the transition of moderate to low SPM in the climatology (Figure 3C), closely matched the Mackenzie Estuary Eco-unit of the Western Arctic Bioregion [WAB; Hodgson et al. (2015)], and the Mackenzie Estuary and Nearshore continental shelf EBSAs (DFO, 2011; DFO, 2015). East of Cape Bathurst, the mid-shelf region (R3) had significant overlap with the Mackenzie Inner-shelf, Outer-shelf and Amundsen Gulf Bays WAB Eco-units (Hodgson et al., 2015). The agreement of the SOM regions with existing EBSAs and Eco-units demonstrated that biogeochemical processes at the ocean surface have some consistency with ecological communities. Our study demonstrates that the inclusion of discrete and categorical data may complement the satellite parameters in this SOM approach to find spatio-temporal patterns for marine spatial planning and conservation.
The regions identified in this study were in agreement with physical characteristics of the EBS observed in other studies. Lower SPM and SST further from the warm, sediment-laden waters delivered by the Mackenzie River (Mulligan and Perrie, 2019) was well reflected in the seasonal climatology (Figure 4). The high SST near the river mouth can also be attributed to high concentrations of optical constituents absorbing solar radiation (Soppa et al., 2019). The stratification and nutrients from the Mackenzie River discharge can sustain high biomass on the shelf (Forest et al., 2013) as shown in the climatology of phytoplankton biomass in R1 through R3. These relationships were also evident in the correlation matrix which showed moderate to strong correlations of SPM and Chl-a between these three regions (Figure 7). The Canada Basin (R6) and Amundsen Gulf (R5) exhibited properties of offshore oceanic waters, with low SPM and Chl-a, and temperature and open-water area strongly influenced by the presence of sea ice. R5 and R6 also exhibited the lowest SST due to latent heat from melting sea-ice preventing greater warming (Stroh et al., 2015).
The impact of low SIC on SST was demonstrated in Canada Basin particularly in 2012, a year with record low SIC throughout the Arctic Ocean (Perovich et al., 2013) causing greater surface heating. Unfortunately, due to the gap between AMSRE and AMSR2 data, we did not include SIC during 2012, but the highest maximum SST in Canada Basin (10.4°C on July 31) was observed that year, which corresponded to a value 2.5 standard deviations higher than the maximum average SST for this region over 2003–2019 (Table 1, Figure S3). The correlation matrices showed a relationship of SIC and SST, which varied in opposite phase for the shelf regions (R1 through R4) and Amundsen Gulf (R5), consistent with previous studies that evidenced the role of large scale atmospheric and solar forcing (i.e., Lukovich et al., 2009; Liu et al., 2021; Henderson et al., 2021) and ocean circulation (i.e., Morison et al., 2021) on the temperature and sea-ice of the Beaufort Sea. The sea surface circulation impacts these regions differently, with the Alaskan Coastal Current flowing eastward along the coast into Amundsen Gulf (Pickart, 2004). The impact of this current was evident in the climatology of SST, which was overall cooler west of Herschel Island and similar to the spatial pattern observed in Mulligan and Perrie (2019) (Figure 3). The shelf edge, Canada Basin and Amundsen Gulf (R4, R5 and R6) were most impacted by the anti-cyclonic Beaufort Gyre defining the boundary between the shelf and open waters. By identifying the seasonal cycles and trends in regions of similar SST, SPM and Chl-a, in addition to dealineating biogeophysical regions, we were able to demonstrate that this method is effective at quantifying some surface processes occuring in the EBS.
A key difference between the shelf edge and Canada Basin (R4 and R6) to Amundsen Gulf (R5) in our satellite dataset, the three regions furthest removed from the optical influence of the Mackenzie River, was the higher variability of phytoplankton biomass (Chl-a GSD; see Figure 3). Factors that contributed to the high Chl-a GSD intersecting the shelf edge and basin (R4 and R6) may be i) local topographically induced upwelling events delivering nutrients to the surface and stimulating phytoplankton growth (Williams and Carmack, 2008), ii) ice-edge blooms that take place under favourable light conditions when nutrients are released as ice recedes (Perrette et al., 2011; Renaut et al., 2018), or iii) wind-driven advection of the Mackenzie River plume toward the central Beaufort Sea (Mulligan and Perrie, 2019). For example, high Chl-a was observed in 2006 and 2013 (> 1.3 mg m–3) as detailed in Babb et al. (2019) and Doxaran et al. (2015), when intense northward advection of stratified nutrient-loaded plume waters with high SPM concentration followed an unusual spring with high SIC that constrained river disharge and limited the plume expansion. For both of these years high Chl-a and SPM were observed in R4 and R6 (see Figure S4 and S5). Amundsen Gulf is physically separated from the shelf regions by the Bathurst Peninsula (Fissel et al., 2013) and experiences spring conditions unfavourable for phytoplankton growth (i.e., storms and ice-creation, Arrigo and van Dijken (2004)). With the Canada Basin region, Amundsen Gulf (R5) showed the least variability in temperature and remained cool most of the year. This region overlaps with the Cape Bathurst flaw polynya (Williams and Carmack, 2008), which is subject to early summer vertical mixing driven by wind and convection and the low Chl-a in Amundsen Gulf in spring is consistent with Arrigo and van Dijken (2004) who demonstrated the importance of stratification to phytoplankton growth in the polynya.
When compared to SST and SIC, the SPM and Chl-a seasonal cycles and time series showed markedly regional differences. The correlation matrix emphasized the thermal characteristics of SST and SIC between regions, while the lack of correlations in SPM and Chl-a between regions suggested that these properties responded to regional forcing identified by the SOM (Figure 7). In spring, the mid-shelf (R3) and Amundsen Gulf (R5) were the first regions free of sea-ice in May (Figure 5), followed by the inner shelf (R2) and the shelf-edge (R4) in early June. These regions showed a peak in particle concentration following sea-ice melt with the highest values occurring on the shelf (Figure 4) following the breakup of the ice barrier at the mouth of the Mackenzie Delta in late June. This emphasizes the impact of the Mackenzie River on the bio-optical conditions and temperature of the coastal EBS surface waters (Figure 3), which is also supported by the magnitude and timing of the SPM peak (Figure 4C) and high correlation between SPM time series on the shelf regions (Figure 7). The phytoplankton biomass peaked a few weeks following the SPM peaks on these shelf regions, potentially corresponding to the settling of mineral particles that let more light enter the water column, when nutrients released by sea-ice melt support phytoplankton growth (Hill et al., 1991). However, these results remain valid for surface Chl-a only, as Chl-a maxima occur at depths not recorded by satellites, particularly further from shore as nutrients delivered by the river plume may be increasingly limited (Retamal et al., 2008) or following ice-edge blooms when the nitracline deepens (Ardyna et al., 2013).
The relationship between SST and Chl-a showed weak relationships throughout the study area. This was in agreement with the work of Blais et al. (2017) who found a correlation coefficient between temperature and phytoplankton biomass using in situ measurements in the EBS in a similar range (i.e., 0.23) to the values found here using a relatively long time series of satellite observations (range of no correlation to 0.43 in our study depending on the region). However, when dividing the time series into warming and cooling periods, we found relationships between SST and Chl-a near the outlet of the Mackenzie River (R1; Figure 8) and on the inner Shelf (R2, i.e., R2 ≥ 0.61). These relationships emphasized the role of freshwater discharge on primary production, as warm, buoyant and nutrient-rich water keep phytoplankton in the surface well-lit water favouring their growth; for example Emmerton et al. (2008) found that the Mackenzie River had a positive impact on phytoplankton production as far as 100 km from the river mouth. The correlation between SST and Chl-a during the warming period decreased as regions were more distant from the Mackenzie Delta region, but remained significant with about 30% of variation in Chl-a explained by SST. We did not find comparable correlations between SST and Chl-a during the cooling period in any of the six regions, with all R2 ≤ 0.17. However, as mentioned previously, it is likely that Chl-a maxima occurred at depths not recorded by satellite, suggesting that past the peak of temperature phytoplankton biomass is driven by other processes such as depletion of nutrients at the surface and light limitation later in the year (Tremblay et al., 2009; Ardyna et al., 2013; Ardyna and Arrigo, 2020).
In this study we did not find temporal trends at the annual scale for SST, SPM and Chl-a, while SIC declined throughout most of the study region on annual and monthly time scales. We did not observe significant monthly changes in Amundsen Gulf (R5) for the four parameters including SIC, however, we observed a shift of the date at which the region reached maximum SST, which has been occurring earlier in recent years. This observation suggests that shifts are occurring to the thermal conditions (i.e., changing ice structure) which may impact temperature-dependent species like Arctic Cod (Michaud et al., 1996). For the other regions, it is noteworthy that the only significant positive SPM trend occurred in spring (April) and trends were negative later in the year, which suggests shifts in the seasonal cycle, though the magnitude of the trends corresponded to small change overall on the decadal scale. We did not find positive trends in SST, nor did we find positive trends in the magnitude of maximum annual SST. However, this result is corroborated by Carvalho and Wang (2020) who found no warming over the period 1982-2018 in the Beaufort Sea, with even cooling trends in some areas. The Chl-a trends, with moderate increases in the inner (R2) and mid-shelf (R3) regions during May, corresponded to dramatic declines in SIC. This result is consistent with projections from Forest et al. (2013) who estimated production increases near the Mackenzie River are most likely to occur on the inner and mid- continental shelf. Additionally, Tolosa et al. (2013) discussed prolonged absences of sea ice over shallow shelves driving resuspension which can enhance production. However, in this study we calculated trends from monthly and annual mean values and did not quantify the increase in open water surface area like other studies reporting on primary production, so further analysis is needed.
5 Conclusion
We partitioned 17 years of satellite-measured sea-surface temperature, suspended particulate matter and chlorophyll-a concentration of the Eastern Beaufort Sea into six regions using Self-Organizing Maps. Combined with sea-ice concentration, the regional climatologies, trends and time series correlations emphasized differing processes dominating the surface ocean characteristics of the EBS. The connectivity of regions and parameters were analyzed using a correlation matrix which showed that regions on or adjacent to the continental shelf had greater similarities when compared to the offshore regions. The continental shelf regions were under greatest influence from the Mackenzie River, which influenced the optical properties of the Mackenzie Shelf waters by delivering large amounts of SPM and other optical constituents, and increasing the temperature, freshwater content and stratification of the surface waters. The continental shelf edge region represented a transitional area, with similarities to the continental shelf regions in terms of the temperature and climatology of SPM and Chl-a, but correlated with the processes of offshore Canada Basin. Canada Basin and Amundsen Gulf had characteristics of open ocean, Case 1 waters, having very low concentrations of optical constituents, with sea-ice dominated processes as shown by the low temperature and lack of warm riverine input, and in Canada Basin, the persistent presence of ice throughout the year. Amundsen Gulf was most distinct from the other five regions, having lowest phytoplankton biomass, lowest concentration of suspended particles, and the absence of annual or monthly temporal trends of any of the parameters. However, the day of maximum annual SST has significantly declined, suggesting changes in its thermal characteristics, which may further impact species susceptible to changes in temperature seasonality. Future work can also include SOM analysis with climatological fields in order to train and articulate changing spatial extent of regions over time.
Overall, the annual and monthly trends showed that phytoplankton concentration of the inner and mid-shelf regions has increased in May concurrent with dramatic declines in SIC. Changing phytoplankton seasonality has already been observed in the EBS (Ardyna and Arrigo, 2020), but this study quantifies the changes in spatially discrete regions. Combined with the other trends observed in this study, namely the inter-annual and monthly declines in SIC on the continental shelf, paired with the lack of SST trends observed, we showed that areas within the EBS are experiencing differing responses when compared to large-scale oceanographic shifts occurring at the Arctic Ocean surface caused by climate warming.
Our results emphasize the need to consider regional scales (similarly mentioned in Screen, 2017) when studying the impacts of climate change and the functioning of the surface Arctic Ocean ecosystems. For example, while SIC is declining throughout the Arctic Ocean and changes in the timing and northward expansion of phytoplankton blooms have been observed, we showed that in the EBS these shifts may currently be more spatially constrained than previously considered. This is further emphasized when specifically considering SST and SIC trends; SST is demonstrably increasing at the pan-Arctic scale, while SIC continues to dwindle, with dire implications for the ecosystems of the Arctic Ocean. However, we did not find SST to be significantly increasing in the time period and area of this study, though SIC declines were demonstrated throughout the area. These results will aid future work investigating and prioritizing protection for vulnerable species and ecosystems, and help with identification of marine refugia that may be currently less impacted by climate warming.
Data Availability Statement
The raw data supporting the conclusions of this article will be made available by the authors, without undue reservation.
Author Contributions
ED designed the initial study, obtained funding and edited the manuscript. AH performed the data processing and statistical analysis, produced the figures and the initial draft of the manuscript. AH and ED realized the study, including method development and interpretation of results, and developed and revised the manuscript. All authors contributed to the article and approved the submitted version.
Funding
This project was funded by the Fisheries and Oceans Canada Arctic Science Fund program.
Conflict of Interest
The authors declare that the research was conducted in the absence of any commercial or financial relationships that could be construed as a potential conflict of interest.
Publisher’s Note
All claims expressed in this article are solely those of the authors and do not necessarily represent those of their affiliated organizations, or those of the publisher, the editors and the reviewers. Any product that may be evaluated in this article, or claim that may be made by its manufacturer, is not guaranteed or endorsed by the publisher.
Acknowledgments
We want to thank Kristen Wilson, Ryan Galley and Stephanie Clay for valuable revisions of the manuscript and Charles Hannah for helpful comments during the development of this study. We thank the DFO Arctic Science Fund program for financial support for this project, which was also conducted under Work Package 4 of the Nunataryuk project, led by M. Babin (Université Laval-CNRS) and funded by the European Union Horizon 2020 framework programme. We also want to acknowledge the NASA Ocean Biology and Processing Group and Universität Bremen for making the satellite data used in this study freely and openly available.
Supplementary Material
The Supplementary Material for this article can be found online at: https://www.frontiersin.org/articles/10.3389/fmars.2022.912865/full#supplementary-material
References
Ainsworth E. J. (1999). Visualization of Ocean Colour and Temperature From Multi-Spectral Imagery Captured by the Japanese Adeos Satellite. J. Vis. 2, 195–204. doi: 10.1007/BF03181523
Ainsworth E. J., Jones S. F. (1999). Radiance Spectra Classification From the Ocean Color and Temperature Scanner on Adeos. IEEE Trans. Geosci. Remote Sens. 37, 1645–1656. doi: 10.1109/36.763281
Amante C., Eakins B. W. (2009). Etopo1 1 Arc-Minute Global Relief Model: Procedures, Data Sources and Analysis. NOAA Technical Memorandum NESDIS, NGDC-24,, 19 pp. doi: 10.1594/PANGAEA.769615
Ardyna M., Arrigo K. (2020). Phytoplankton Dynamics in a Changing Arctic Ocean. Nat. Clim. Change 10, 892–903. doi: 10.1038/s41558-020-0905-y
Ardyna M., Babin M., Gosselin M., Devred E., Bélanger S., Matsuoka A., et al. (2013). Parameterization of Vertical Chlorophyll a in the Arctic Ocean: Impact of the Subsurface Chlorophyll Maximum on Regional, Seasonal, and Annual Primary Production Estimates. Biogeosciences 10, 4383–4404. doi: 10.5194/bg-10-4383-2013
Ardyna M., Babin M., Gosselin M., Devred E., Rainville E., Tremblay J.-E. (2014). Recent Arctic Ocean Sea Ice Loss Triggers Novel Fall Phytoplankton Blooms. Geophys. Res. Lett. 41, 6207–6212. doi: 10.1002/2014GL061047
Arrigo K., van Dijken G. (2004). Annual Cycles of Sea Ice and Phytoplankton in Cape Bathurst Polynya, Southeastern Beaufort Sea, Canadian Arctic. Geophys. Res. Lett. 31, 60–70. doi: 10.1029/2003GL018978
Arrigo K., van Dijken G. (2015). Continued Increases in Arctic Ocean Primary Production. Prog. Oceanogr. 136, 60–70. doi: 10.1016/j.pocean.2015.05.002
Babb D., Landy J., Barber D., Galley R. (2019). Winter Sea Ice Export From the Beaufort Sea as a Preconditioning Mechanism for Enhanced Summer Melt: A Case Study of 2016. J. Geophys. Res. Ocean. 124, 6575–6600. doi: 10.1029/2019JC015053
Barber D. G., Hanesiak J. M. (2004). Meteorological Forcing of Sea Ice Concentrations in the Southern Beaufort Sea Over the Period 1979 to 2000. J. Geophys. Res. 109, 1–16. doi: 10.1029/2003JC002027
Bélanger S., Babin M., Tremblay J. (2013). Increasing Cloudiness in Arctic Damps the Increase in Phytoplankton Primary Production Due to Sea Ice Receding. Biogeosciences 10, 4087–4101. doi: 10.5194/bg-10-4087-2013
Blais M., Ardyna M., Gosselin M., Dumont D., Bélanger S., Tremblay J.-E., et al. (2017). Contrasting Interannual Changes in Phytoplankton Productivity and Community Structure in the Coastal Canadian Arctic Ocean. Limnol. Oceanogr. 62, 2480–2497. doi: 10.1002/lno.10581
Campbell J. W. (1995). The Lognormal Distribution as a Model for Bio-Optical Variability in the Sea. J. Geophys. Res. 100, 13237–13254. doi: 10.1029/95JC00458
Carvalho K., Wang S. (2020). Sea Surface Temperature Variability in the Arctic Ocean and its Marginal Seas in a Changing Climate: Patterns and Mechanisms. Global Planet. Change 193, 103265. doi: 10.1016/j.gloplacha.2020.103265
Cobb D. G., MacPhee S., Paulic J., Martin K., Roy V., Reist J., et al. (2020). Information in Support of the Identification of Ecologically Significant Species, Functional Groups and Community Properties (ESSCP) in the Western Arctic Biogeographic Region. Tech. Rep. 2018/027, iv + 66. 2018/027DFO Can. Sci. Advis. Sec. Res. Doc.
Cole H., Henson S., Martin A., Yool A. (2012). Mind the Gap: The Impact of Missing Data on the Calculation of Phytoplankton Phenology Metrics. J. Geophys. Res. Ocean. 117, 1–8. doi: 10.1029/2012JC008249
Devred E., Sathyendranath S., Platt T. (2007). Delineation of Ecological Provinces Using Ocean Colour Radiometry. Mar. Ecol. Prog. Ser. 346, 1–13. doi: 10.3354/meps07149
DFO (2011). Identification of Ecologically and Biologically Significant Areas (EBSA) in the Canadian Arctic. Tech. Rep. DFO Can. Sci. Advis. Sec. Sci. Advis. Rep. 2011/055, 1–40
DFO (2015). Eco-Units and Potential Priority Conservation Areas in the Western Arctic Bioregion. Tech. Rep. DFO Can. Sci. Advis. Sec. Sci. Advis. Rep. 2014/021, 1–19.
Doxaran D., Devred E., Babin M. (2015). A 50% Increase in the Mass of Terrestrial Particles Delivered by the Mackenzie River Into the Beaufort Sea (Canadian Arctic Ocean) Over the Last 10 Years. Biogeosciences 12, 3551–3565. doi: 10.5194/bg-12-3551-2015
ECCC (2021). Real-Time Hydrometric Data. (Gatineau, Quebec: Environment and Climate Change Canada) https://wateroffice.ec.gc.ca/contactus/contact_us_e.html.
El Hourany R., Abboud-Abi Saab M., Faour G., Mejia C., Crépon M., Thiria S. (2019). Phytoplankton Diversity in the Mediterranean Sea From Satellite Data Using Self-Organizing Maps. J. Geophys. Res. Ocean. 124, 5827–5843. doi: 10.1029/2019JC015131
Emmerton C., Lesack L., Warwick V. (2008). Nutrient and Organic Matter Patterns Across the Mackenzie River, Estuary and Shelf During the Seasonal Recession of Sea-Ice. J. Mar. Syst. 74, 741–755. doi: 10.1016/j.jmarsys.2007.10.001
Fissel D., Cross W., Howland K. (2013). An Ecological and Oceanographic Assessment of the Beaufort Sea Region: Evaluation of the Risks Associated With Ballast Water Exchange. Tech. Rep. 2012/149, vi + 64.DFO Can. Sci. Advis. Sec. Res. Doc.
Forest A., Babin M., Stemmann L., Picheral M., Sampei M., Fortier L., et al. (2013). Ecosystem Function and Particle Flux Dynamics Across the Mackenzie Shelf (Beaufort Sea, Arctic Ocean): An Integrative Analysis of Spatial Variability and Biophysical Forcings. Biogeosciences 10, 2833–2866. doi: 10.5194/bg-10-2833-2013
Galley R. J., Else B. G. T., Prinsenberg S. J., Babb D., Barber D. G. (2013). Summer Sea Ice Concentration, Motion, and Thickness Near Areas of Proposed Offshore Oil and Gas Development in the Canadian Beaufort Sea – 2009. Arctic 66(1), 105–16. doi: 10.14430/arctic4270
Giovando L. F., Herlinveaux R. H. (1981). A Discussion of Factors Influencing Dispersion of Pollutants in the Beaufort Sea. Tech. Rep. 81-4, 198. DFO Institute of Ocean Sciences, Sidney, British Columbia.
Han B., Loisel H., Vantrepotte V., Mériaux X., Bryère P., Ouillon S., et al. (2016). Development of a Semi-Analytical Algorithm for the Retrieval of Suspended Particulate Matter From Remote Sensing Over Clear to Very Turbid Waters. Remote Sens. 8, 211. doi: 10.3390/rs8030211
Hardman-Mountford N., Hirata T., Richardson K., Aiken J. (2008). An Objective Methodology for the Classification of Ecological Pattern Into Biomes and Provinces for the Pelagic Ocean. Remote Sens. Environ. 112, 3341–3352. doi: 10.1016/j.rse.2008.02.016
Henderson G. R., Barrett B. S., Wachowicz L. J., Mattingly K. S., Preece J. R., Mote T. L. (2021). Local and Remote Atmospheric Circulation Drivers of Arctic Change: A Review. Front. Earth Sci. 9. doi: 10.3389/feart.2021.709896
Hewitson B. C., Crane R. G. (2002). Self-Organizing Maps: Applications to Synoptic Climatology. Clim 22, 13–26. doi: 10.3354/cr022013
Hill P. R., Blasco S. M., Harper J. R., Fissel D. B. (1991). Sedimentation on the Canadian Beaufort Shelf. Continent. Shelf. Res. 11, 821–842. doi: 10.1016/0278-4343(91)90081-G. Proceedings of the Canadian Continental Shelf Seabed Symposium (C2S3).
Hodgson R., Martin K., Melling H. (2015). Marine Protected Area Network Planning in the Western Arctic Bioregion: Development and Use of a Classification System to Identify Ecological Units as Required Planning Components. Tech. Rep. 2015/020, v + 41. DFO Can. Sci. Advis. Sec. Res. Doc.
IOCCG (2009). Partition of the Ocean Into Ecological Provinces: Role of Ocean-Colour Radiometry, Vol. No. 9 of Reports of the International Ocean Colour Coordinating Group (Dartmouth, Canada: IOCCG). doi: 10.25607/OBP-99
IOCCG (2015). Ocean Colour Remote Sensing in Polar Seas, Vol. No. 16 of Reports of the International Ocean Colour Coordinating Group (Dartmouth, Canada: IOCCG). doi: 10.25607/OBP-107
Jorgenson M. T., Shur Y., Pullman E. (2006). Abrupt Increase in Permafrost Degradation in Arctic Alaska. Geophys. Res. Lett. 33, 1–4. doi: 10.1029/2005GL024960
Kahru M., Brotas V., Manzano.-Sarabia M., Mitchel B. (2011). Are Phytoplankton Blooms Occurring Earlier in the Arctic? Global Change Biol. 17, 1733–1739. doi: 10.1111/j.1365-2486.2010.02312.x
Kahru M., Lee Z., Mitchell B. G., Nevison C. D. (2016). Effects of Sea Ice Cover on Satellite-Detected Primary Production in the Arctic Ocean. Biol. Lett. 12, 20160223. doi: 10.1098/rsbl.2016.0223
Kaski S., Kangas J., Kohonen T. (1998). Bibliography of Self-Organizing Map (Som) Papers: 1981-1997. Neural Comput. Survey. 1, 1–176. doi: 10.1007/978-1-4471-0513-8_6
Kwok R., Cunningham G. F. (2015). Variability of Arctic Sea Ice Thickness and Volume From Cryosat-2. Phil. Trans. R. Soc A. 373, 1–20. doi: 10.1098/rsta.2014.0157
LaZerte S. E., Albers S. (2018). Weathercan: Download and Format Weather Data From Environment and Climate Change Canada. J. Open Source Softw. 3, 571. doi: 10.21105/joss.00571
Lewis K., Arrigo K. (2020). Ocean Color Algorithms for Estimating Chlorophyll a, Cdom Absorption, and Particle Backscattering in the Arctic Ocean. J. Geophys.Res. Ocean. 125, 1–23. doi: 10.1029/2019JC015706
Liu Z., Risi C., Codron F., He X., Poulsen C., Wei Z., et al. (2021). Acceleration of Western Arctic Sea Ice Loss Linked to the Pacific North American Pattern. Nat. Comm. 12, 1–9. doi: 10.1038/s41467-021-21830-z
Longhurst A., Sathyendranath S., Platt T., Caverhill C. (1995). An Estimate of Global Primary Production in the Ocean From Satellite Radiometer Data. J. Plankt. Res. 17, 1245–1271. doi: 10.1093/plankt/17.6.1245
Lukovich J. V., Asplin M. G., Barber D. G. (2009). Atmospheric Forcing of the Beaufort Sea Ice Gyre: Surface-Stratosphere Coupling. J. Geophys. Res.: Ocean. 114, 1–14. doi: 10.1029/2008JC004849
Macdonald R. W., Harner T., Fyfe J. (2005). Recent Climate Change in the Arctic and its Impact on Contaminant Pathways and Interpretation of Temporal Trend Data. Sci. Tot. Environ. 342, 5–86. doi: 10.1016/j.scitotenv.2004.12.059
Macdonald R. W., Paton D. W., Carmack E. C. (1995). The Freshwater Budget and Under-Ice Spreading of the Mackenzie River Water in the Canadian Beaufort Sea Based on Salinity and 18o/16o Measurements in Water and Ice. J. Geophys. Res. Ocean. 100, 895–919. doi: 10.1029/94JC02700
Martin Traykovski L. V., Sosik H. M. (2003). Feature-Based Classification of Optical Water Types in the Northwest Atlantic Based on Satellite Ocean Color Data. J. Geophys. Res. Ocean. 108, 19-1–19-18. doi: 10.1029/2001JC001172
Maslanik J., Stroeve J., Fowler C., Emery W. (2011). Distribution and Trends in Arctic Sea Ice Age Through Spring 2011. Geophys. Res. Lett. 38, 1–6. doi: 10.1029/2011GL047735
McIver R., Breeze H., Devred E. (2018). Satellite Remote-Sensing Observations for Definitions of Areas for Marine Conservation: Case Study of the Scotian Slope, Eastern Canada. Remote Sens. Environ. 214, 23–47. doi: 10.1016/j.rse.2018.05.017
Michaud J. L., Fortier P., Ramseier R. (1996). Feeding Success and Survivorship of Arctic Cod Larvae, Boreogadus Saida, in the Northeast Water Polynya (Greenland Sea). Fish. Oceanogr. 5, 120–135. doi: 10.1111/j.1365-2419.1996.tb00111.x
Miller G. H., Alley R. B., Brigham-Grette J., Fitzpatrick J. J., Polyak L., Serreze M. C., et al. (2010). Arctic Amplification: Can the Past Constrain the Future? Quaternar. Sci. Rev. 29, 1779–1790. doi: 10.1016/j.quascirev.2010.02.008
Morel A., Prieur L. (1977). Analysis of Variation in Ocean Color. Limnol. Oceanogr. 22, 709–722. doi: 10.4319/lo.1977.22.4.0709
Morison J., Kwok R., Dickinson S., Andersen R., Peralta-Ferriz C., Morison D., et al. (2021). The Cyclonic Mode of Arctic Ocean Circulation. J. Phys. Oceanogr. 51, 1053–1075. doi: 10.1175/JPO-D-20-0190.1
Mulligan R., Perrie W. (2019). Circulation and Structure of the Mackenzie River Plume in the Coastal Arctic Ocean. Continent. Shelf. Res. 177, 59–68. doi: 10.1016/j.csr.2019.03.006
Oliver M., Glenn S., Kohut J., Irwin A., Schofield O., Moline M., et al. (2004). Bioinformatic Approaches for Objective Detection of Water Masses on Continental Shelves. J. Geophys. Res. Ocean. 109, 1–12. doi: 10.1029/2003JC002072
Oliver M., Irwin A. (2008). Objective Global Ocean Biogeographic Provinces. Geophys. Res. Lett. 35, 1–6. doi: 10.1029/2008GL034238
O’Reilly J. E., Maritorena S., Mitchell B. G., Siegel D. A., Carder K. L., Garver S. A., et al. (1998). Ocean Color Chlorophyll Algorithm for SeaWiFS. J. Geophys. Res. 103, 24,937–24,953. doi: 10.1029/98JC02160
Park K., Hahm D., Choi J. O., Xu S., Kim H.-C., Lee S. (2019). Spatiotemporal Variation in Summer Net Community Production in the Amundsen Sea Polynya: A Self-Organizing Map Analysis Approach. Continent. Shelf. Res. 184, 21–29. doi: 10.1016/j.csr.2019.07.001
Payette S., Delwaide S., Caccianiga M., Beauchemin M. (2004). Accelerated Thawing of Subarctic Peatland Permafrost Over the Last 50 Years. Geophys. Res. Lett. 31, 1–4. doi: 10.1029/2004GL020358
Perovich D., Meier W., Tschudi M., Gerland S., Richter-Menge J. (2013). [the Arctic] Sea Ice Cover [in “State of the Climate in 2012”]. Bull. Am. Meteor. Soc 94, S1–S258. doi: 10.1175/2013BAMSStateoftheClimate.1
Perrette M., Yool A., Quartly G. D., Popova E. E. (2011). Near-Ubiquity of Ice-Edge Blooms in the Arctic. Biogeosciences 8, 515–524. doi: 10.5194/bg-8-515-2011
Peucker-Ehrenbrink B. (2009). Land2sea Database of River Drainage Basin Sizes, Annual Water Discharges, and Suspended Sediment Fluxes. Geochem. Geophys. Geosyst. 10, 1–10. doi: 10.1029/2008GC002356
Pickart R. S. (2004). Shelfbreak Circulation in the Alaskan Beaufort Sea: Mean Structure and Variability. J. Geophys. Res. 109, 1–14. doi: 10.1029/2003JC001912
Qi L., Lee Z., Hu C., Wang M. (2017). Requirement of Minimal Signal-to-Noise Ratios of Ocean Color Sensors and Uncertainties of Ocean Color Products. J. Geophys. Res. Ocean. 122, 2595–2611. doi: 10.1002/2016JC012558
R Core Team (2019). R: A Language and Environment for Statistical Computing (Vienna, Austria: R foundation for Statistical Computing).
Renaut S., Devred E., Babin M. (2018). Northward Expansion and Intensification of Phytoplankton Growth During the Early Ice-Free Season in Arctic. Geophys. Res. Lett. 45, 10,590–10,598. doi: 10.1029/2018GL078995
Retamal L., Bonilla S., Vincent W. F. (2008). Optical Gradients and Phytoplankton Production in the Mackenzie River and the Coastal Beaufort Sea. Polar. Biol. 31, 363–379. doi: 10.1007/s00300-007-0365-0
Reygondeau G., Cheung W. W. L., Wabnitz C. C. C., Lam V. W. Y., Frölicher T., Maury O. (2020). Climate Change-Induced Emergence of Novel Biogeochemical Provinces. Front. Mar. Sci. 7. doi: 10.3389/fmars.2020.00657
Reygondeau G., Guidi L., Beaugrand G., Henson S. A., Koubbi P., MacKenzie B. R., et al. (2018). Global Biogeochemical Provinces of the Mesopelagic Zone. J. Biogeogr. 45, 500–514. doi: 10.1111/jbi.13149
Richardson A. J., Risien C., Shillington F. A. (2003). Using Self-Organizing Maps to Identify Patterns in Satellite Imagery. Prog. Oceanogr. 59, 223–239. doi: 10.1016/j.pocean.2003.07.006
Screen J. A. (2017). Simulated Atmospheric Response to Regional and Pan-Arctic Sea Ice Loss. J. Climate 30, 3945–3962. doi: 10.1175/JCLI-D-16-0197.1
Serreze M., Barrett A., Stroeve J., Kindig D., Holland M. (2009). The Emergence of Surface-Based Arctic Amplification. Cryosphere 3, 11–19. doi: 10.5194/tc-3-11-2009
Soppa M. A., Pefanis V., Hellmann S., Losa S. N., H’ólemann J., Martynov F., et al. (2019). Assessing the Influence of Water Constituents on the Radiative Heating of Laptev Sea Shelf Waters. Front. Mar. Sci. 6. doi: 10.3389/fmars.2019.00221
Stroeve J. C., Serreze M. C., Holland M. M., Kay J. E., Malanik J., Barrett A. P. (2012). The Arctic’s Rapidly Shrinking Sea Ice Cover: A Research Synthesis. Clim. Change 110, 1005–1027. doi: 10.1007/s10584-011-0101-1
Stroh J. N., Panteleev G., Kirillov S., Makhotin M., Shakhova N. (2015). Sea-Surface Temperature and Salinity Product Comparison Against External in Situ Data in the Arctic Ocean. J. Geophys. Res. 120, 7223–7236. doi: 10.1002/2015JC011005
Taşdemir K. (2011). “Neural Network Based Approximate Spectral Clustering for Remote Sensing Images,” in 2011 IEEE International Geoscience and Remote Sensing Symposium, 2884–2887 doi: 10.1109/IGARSS.2011.6049817. (Institute of Electrical and Electronics Engineers).
Tolosa I., Fiorini S., Gasser B., Martín J., Miquel J. C. (2013). Carbon Sources in Suspended Particles and Surface Sediments From the Beaufort Sea Revealed by Molecular Lipid Biomarkers and Compound-Specific Isotope Analysis. Biogeosciences 10, 2061–2087. doi: 10.5194/bg-10-2061-2013
Tremblay G., Belzile C., Gosselin M., Poulin M., Roy S., Tremblay J.-E. (2009). Late Summer Phytoplankton Distribution Along a 3500 Km Transect in Canadian Arctic Waters: Strong Numerical Dominance by Picoeukaryotes. Aquat. Microb. Ecol. 54, 55–70. doi: 10.3354/ame01257
Wang M., Shi W. (2007). The Nir-Swir Combined Atmospheric Correction Approach for Modis Ocean Color Data Processing. Opt. Exp. 15, 15722–15733. doi: 10.1364/OE.15.015722
Wehrens R., Buydens L. M. C. (2007). Self- and Super-Organizing Maps in R: The Kohonen Package. J. Stat. Softw. 21, 1–19. doi: 10.18637/jss.v021.i05
Wehrens R., Kruisselbrink J. (2018). Flexible Self-Organizing Maps in Kohonen 3.0. J. Stat. Softw. 87, 1–18. doi: 10.18637/jss.v087.i07
Wessel P., Smith W. H. F., Scharroo R., Luis J., Wobbe F. (2013). Generic Mapping Tools: Improved Version Released. Eos. Trans. Am. Geophys. Union. 94, 409–410. doi: 10.1002/2013EO450001
Williams W., Carmack E. (2008). Combined Effect of Wind-Forcing and Isobath Divergence on Upwelling at Cape Bathurst, Beaufort Sea. J. Mar. Res. 66, 645–663(19). doi: 10.1357/002224008787536808
WMO (2016). The Global Observing System for Climate: Implementation Needs. Tech. Rep GCOS, World Meteorological Organization.200, 1–315
Keywords: ocean colour, Beaufort Sea, chlorophyll-a concentration, suspended particulate matter, sea surface temperature, biogeochemical regions, self-organizing maps
Citation: Hilborn A and Devred E (2022) Delineation of Eastern Beaufort Sea Sub-regions Using Self-Organizing Maps Applied to 17 Years of MODIS-Aqua Data. Front. Mar. Sci. 9:912865. doi: 10.3389/fmars.2022.912865
Received: 05 April 2022; Accepted: 26 May 2022;
Published: 01 July 2022.
Edited by:
William Savidge, University of Georgia, United StatesReviewed by:
Frano Matić, Institute of Oceanography and Fisheries (IZOR), CroatiaRoy El Hourany, UMR8187 Laboratoire d’océanologie et de géosciences (LOG), France
Keyhong Park, Korea Polar Research Institute, South Korea
Copyright © 2022 Hilborn and Devred. This is an open-access article distributed under the terms of the Creative Commons Attribution License (CC BY). The use, distribution or reproduction in other forums is permitted, provided the original author(s) and the copyright owner(s) are credited and that the original publication in this journal is cited, in accordance with accepted academic practice. No use, distribution or reproduction is permitted which does not comply with these terms.
*Correspondence: Andrea Hilborn, Andrea.Hilborn@dfo-mpo.gc.ca