- 1College of Oceanic and Atmospheric Sciences, Ocean University of China, Qingdao, China
- 2School of Mathematical Sciences, Ocean University of China, Qingdao, China
- 3Frontiers Science Center for Deep Ocean Multispheres and Earth System and Key Laboratory of Physical Oceanography, Ocean University of China, Qingdao, China
- 4Pilot National Laboratory for Marine Science and Technology, Qingdao, China
The surface turbulent heat flux feedback αT plays an important role in the atmosphere–ocean coupling. However, spatio-temporal variability of αT for sea surface temperature anomaly (SSTA) at oceanic mesoscales in the global ocean remains poorly assessed. In this study, we tackle this issue using an advanced statistical model, i.e., the geographically and temporally weighted regression model. The estimated time-mean αT for mesoscale SSTA generally ranges from 10 to 50 W/(m2 K) within 70°S–70°N, except in the Antarctic coastal region where its value drops to zero. The αT is larger in the tropics than in off-tropical regions and locally enhanced in the equatorial cold tongues, western boundary currents, and their extensions. The spatial structure αT is primarily attributed to the non-linearity in the Clausius–Clapeyron relation and inhomogeneity in the background wind speed, whereas adjustment of surface wind speed, air temperature, or moisture to mesoscale SSTA plays an important role in the regional variability. There is an evident seasonal cycle of αT in the tropics and under the northern hemisphere’s storm tracks. The former is due to the seasonally varying response of surface wind speed to mesoscale SSTA, and the latter results from the seasonality of atmospheric and oceanic background states. Our analysis reveals prominent spatio-temporal variability of αT for mesoscale SSTA governed by complicated dynamics.
1 Introduction
Response of turbulent heat flux at the air–sea interface to the sea surface temperature anomaly (SSTA), also known as the surface turbulent heat flux feedback, is a crucial element in the coupled atmosphere–ocean system (Frankignoul and Hasselmann, 1977; Frankignoul, 1985; Barsugli and Battisti, 1998; Bishop et al., 2020). It causes damping of SSTA by air–sea interactions. The intensity of the feedback αT , defined as the surface turbulent heat flux change in response to 1 K SSTA change, is controlled by both the background atmospheric and oceanic states and the adjustment of the marine atmospheric boundary layer (MABL) to the underlying SSTA, varying evidently with location, time, and spatial scale (Frankignoul and Kestenare, 2002; Park et al., 2005; Hausmann et al., 2016; Hausmann et al., 2017; Li et al., 2017).
A large fraction of SSTA variance resides in the oceanic mesoscales of O(100 km), especially in the major frontal regions such as western boundary currents (WBCs) and their extensions (e.g., Hausmann and Czaja, 2012). It has been well recognized that the air–sea interaction at mesoscales differs fundamentally from that at the broader basin scales (Bryan et al., 2010; Ma et al., 2015; Hausmann et al., 2017; Small et al., 2019; Bishop et al., 2020). At mesoscales, SSTA is primarily generated by oceanic intrinsic variability via anomalous heat advection (Yang et al., 2019; Shan et al., 2020a; Guo et al., 2022). Once generated, it is strongly damped via the surface turbulent heat flux feedback (Ma et al., 2016; Yang et al., 2019; Guo et al., 2022). Such damping is found to be a major pathway of mesoscale eddy potential energy dissipation and could further regulate the intensity of WBC extensions and stratification via changing the horizontal and vertical buoyancy fluxes induced by mesoscale eddies, respectively (Ma et al., 2016; Shan et al., 2020a and Shan et al., 2020b). Therefore, it is essential to have an in-depth knowledge of αT for mesoscale SSTA and its governing factors.
Early studies on αT and its underlying dynamics were mostly based on coarse-resolution observational products and climate simulations that do not resolve mesoscale SSTA (Frankignoul et al., 1998; Frankignoul and Kestenare, 2002; Park et al., 2005). More recent analyses by Hausmann et al. (2016); Hausmann et al. (2017) and Li et al. (2017) revealed that αT depends on the spatial scale, being much greater at the mesoscales than at the broader basin scales. Moreton et al. (2021) made a composite of surface turbulent heat flux anomaly over mesoscale coherent eddies in the global ocean and reported a mean αT between 35 and 45 W/(m2 K). By regressing the surface total (turbulent plus radiative) heat flux anomaly onto mesoscale SSTA, Yang et al. (2018) estimated the spatial distribution of surface total heat flux feedback for mesoscale SSTA and found its value varying from 20 to 65 W/(m2 K) within 60°S–60°N. Their findings can be used to infer the spatial variability of αT for mesoscale SSTA, as the surface total heat flux feedback is dominated by αT . However, the underlying dynamics governing such spatial variability have not been thoroughly understood.
So far, the temporal variability of αT for mesoscale SSTA remains poorly assessed. This is partially due to the limitation of statistical models used by the oceanography community for estimation. Currently, αT or surface total heat flux feedback for mesoscale SSTA is typically estimated from the classical regression analysis assuming a constant regression coefficient (Ma et al., 2015; Ma et al., 2016; Yang et al., 2018; Moreton et al., 2021). However, in the past several decades, advanced regression models such as the geographically weighted regression (GWR) model (Brunsdon et al., 1996; Fotheringham et al., 1996; Fotheringham et al., 2002) and their extensions have been developed to handle the varying regression coefficient. In this study, we use one extension of the GWR model, i.e., the geographically and temporally weighted regression (GTWR) model (Huang et al., 2010; Fotheringham et al., 2015), to estimate the spatio-temporal variability of αT for mesoscale SSTA in the global ocean and to uncover the underlying dynamics for such variability.
The rest of the paper is structured as follows. In Section 2, we describe the GTWR model as well as the surface turbulent heat flux and SST dataset used for analysis. The spatio-temporal variability of αT for mesoscale SSTA estimated from the GTWR model and its governing factors are presented in Section 3. Section 4 discusses some notable features of MABL adjustment to the underlying mesoscale SSTA revealed by the GTWR model. Conclusions are listed in Section 5. For neatness, αT refers specifically to αT for mesoscale SSTA hereinafter unless noted otherwise.
2 Data and methods
2.1 The ERA5 data
The surface sensible, latent heat flux, and SST are obtained from the ERA5 reanalysis produced by the European Centre for Medium-Range Weather Forecasts (ECMWF). The data cover the period from 1950 to the present with a spatial resolution of 31 km and a temporal resolution of 1 h (Hersbach et al., 2020). Two different SST products are used as SST input to the ERA5 reanalysis during different periods, i.e., Hadley Centre Sea Ice and Sea Surface Temperature dataset (HadISST2) on the 1° × 1° grids before September 2007 and Operational Sea Surface Temperature and Ice Analysis (OSTIA) on the 0.05° × 0.05° grids after then. In the following analysis, we only use the ERA5 reanalysis data during 2008–2020, as the HadISST2 is too coarse to resolve the mesoscale SSTA. To analyze the factors governing the spatio-temporal variability of αT , quantities to compute the sensible and latent heat fluxes through the bulk formula (Large and Yeager, 2004) are also obtained from the ERA5 reanalysis. It should be noted that the ERA5 reanalysis does not output the surface air-specific humidity. We compute this quantity based on the surface air temperature, dew point temperature, and surface pressure. Finally, all the quantities are daily averaged to reduce the computation burden.
2.2 Isolation of mesoscale signals
Following Yang et al. (2018), mesoscale signals are first computed as the spatially high-pass-filtered field achieved by removing a 4° × 4° running mean. Then, a 5-day running mean is applied to the spatially high-pass-filtered field. This low-pass filtering in the time domain has nearly no influence on the variance of mesoscale SSTA but causes an evident reduction in the variance of mesoscale surface turbulent heat flux anomaly (Supplementary Figure S1). The removed variance of mesoscale surface turbulent heat flux anomaly is likely to be generated by atmospheric intrinsic variability rather than underlying mesoscale SSTA. Therefore, the application of the 5-day running mean enhances the signal-to-noise ratio for the following regression analysis, providing a more robust estimate for αT . Nevertheless, this neglects the possible dependence of αT on the frequency, which is beyond the scope of this study. Finally, we remove the seasonal cycle from the mesoscale SSTA.
2.3 The geographically and temporally weighted regression model
Let xi,j and yi,j represent the explanatory and response variables at the ith location, jth time, respectively. The GTWR model extends the classical regression model by allowing the regression coefficient to vary in the spatio-temporal domain, i.e.,
(Huang et al., 2010; Fotheringham et al., 2015) where βi,j is the regression coefficient at the ith location and jth time and ϵi,j is an independent and identically distributed white noise process with mean zero and constant variance. The βi,j corresponds to αT if xi,j is the mesoscale SSTA and yi,j is the mesoscale surface turbulent heat flux anomaly (Li et al., 2017; Yang et al., 2018).
The core assumption underpinning the GTWR model as well as other varying regression coefficient models is the closeness between βi,j at proximate locations and times, which seems physically reasonable for αT . As confirmed by previous studies (e.g., Yang et al., 2018), the value of αT generally varies smoothly in space. Although there are no existing estimates for the temporal variation of αT , it is unlikely that the value of αT should changes abruptly or randomly with time. In this case, βi,j can be estimated based on the observations not only at the ith location and jth time but also at its surrounding points in the spatio-temporal domain. Specifically, its value can be estimated by minimizing the following weighted residual sum of squares:
where is a weight that reflects the different importance of individual observations used to estimate βi,j and is greater for closer observations.
The value of is usually derived from a prescribed spatio-temporal kernel function. One of the widely used kernel functions is the exponential function,
where Δ x (Δ y ) is the zonal (meridional) distance between the ith and i′th locations, Δ t is the temporal distance between the jth and j′th times, and θs=(θx,θy) and θt are the bandwidths determining the decay rate of in the spatial and temporal domains, respectively.
The θs and θt serve as tuning parameters and control the bias–variance trade-off of the GTWR model. On the one hand, an overly large θs or θt will lead to excessive smoothing of βi,j , causing a larger bias of the GTWR model. On the other hand, a too-small θs or θt will result in overfitting, increasing the variance of the GTWR model. The values of θs and θt can be optimized using cross-validation if there is no prior knowledge. Alternatively, their choices can be guided by the domain knowledge for a specific application. In this study, the value of θs is set as (4°,2°) . Such a choice is small enough to resolve the fine structure of αT along the WBCs and their extensions (Figure 1A). The value of θt is set as 60 days, enabling to resolve the prominent seasonal cycle of αT . Although using smaller values of θs and θt could better resolve small-scale variability of αT in the spatio-temporal domain, we find that the estimates become less robust in some parts of the global ocean.
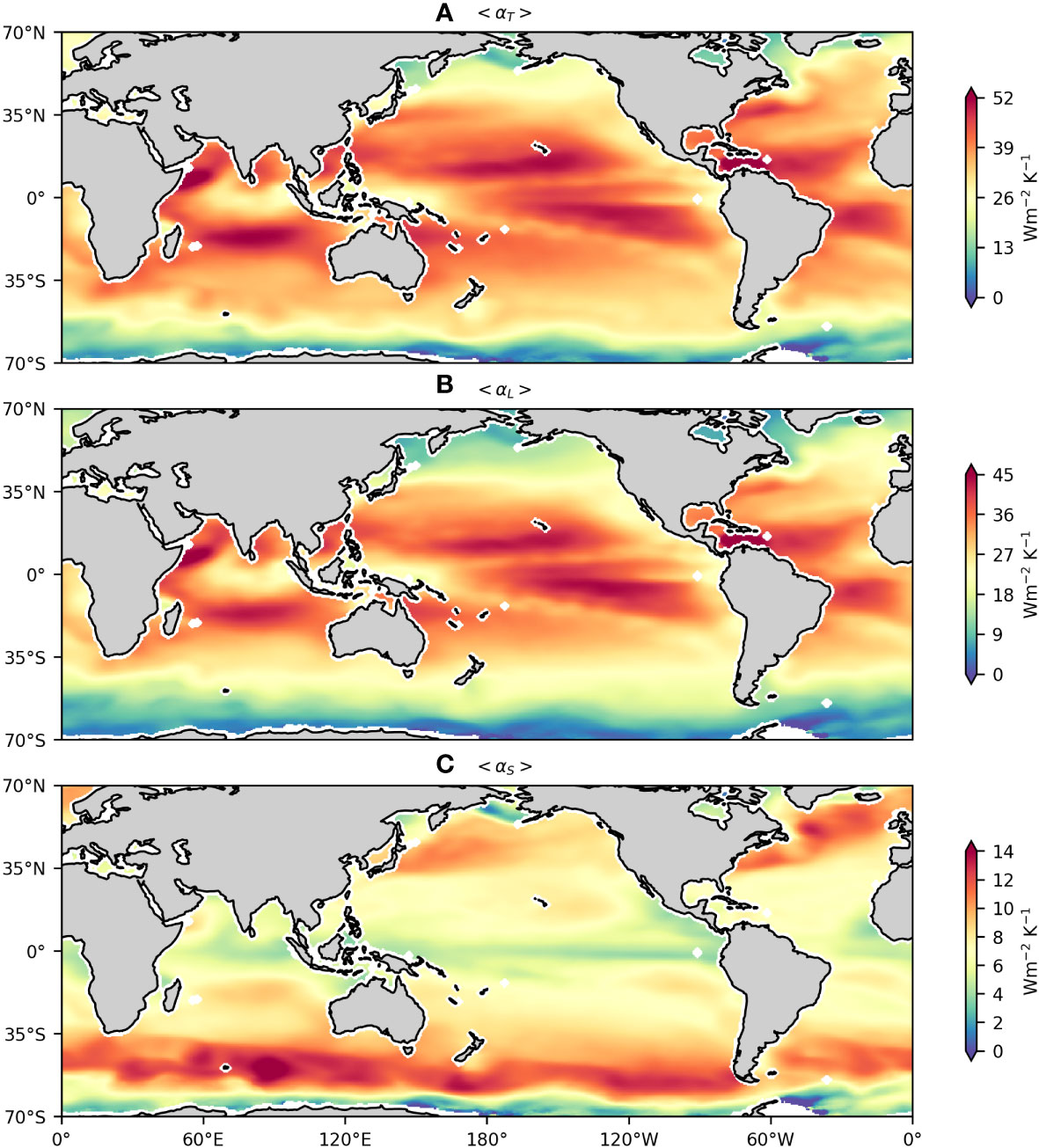
Figure 1 Time-mean (A) αT , (B) αL , and (C) αS in the global ocean during 2008–2020. Note that the colorbars are different for panels (A–C)..
For the computation of Equation. (2), values of on the land and sea-ice grids are set as zero. To avoid problematic estimation, we confine the regression analysis to 70°S–70°N and discard the estimates of βi,j within 1.5° from the coastlines.
3 Results
3.1 Spatio-temporal variability of αT
Figure 1A displays the spatial distribution of the time-mean αT (denoted as <αT> henceforth) within 70°S–70°N. There is pronounced spatial variability in <αT> , with its value ranging from 0 to 52 W/(m2 K). The value of <αT> depends on the latitude, being larger in the tropics than in off-tropical regions and dropping to zero in the Antarctic coastal region. Moreover, <αT> exhibits evident zonal asymmetry. For instance, in the tropics, <αT> is more than 40 W/(m2 K) in the cold tongues, but the value is reduced to 20 W/(m2 K) or so in the Indo-Pacific warm pool. Finally, there is a local enhancement of <αT> along the major WBCs and their extensions. These spatial features of <αT> are qualitatively consistent with those of surface total heat flux feedback for mesoscale SSTA reported by Yang et al. (2018), indicating that <αT> makes a dominant contribution to the surface total heat flux feedback for mesoscale SSTA.
We decompose αT into the surface latent and sensible heat flux feedbacks, denoted as αL and αS , respectively (Figures 1B, C). The magnitude of <αL> is generally larger than that of <αS> . The spatial distributions of <αL> and <αS> differ substantially from each other. The <αL> is generally larger in the tropics than in off-tropical regions. The opposite is true for <αS> . The spatial variability of <αT> is primarily attributed to <αL> , with their pattern correlation coefficient reaching up to 0.97. In contrast, the pattern correlation coefficient decreases to 0.11 for <αS> and <αT> .
We next examine the temporal variability of αT measured as the standard deviation of αT time series (denoted as σ(αT) henceforth) (Figure 2). The σ(αT) exhibits banded enhancement in the tropics that is mainly ascribed to σ(αL) . As to σ(αT) in the off-tropical regions, there is an evident asymmetry between the two hemispheres: large values of σ(αT) occurring mainly in the northern hemisphere, particularly under the storm tracks. Both σ(αL) and σ(αS) contribute importantly to σ(αT) there.
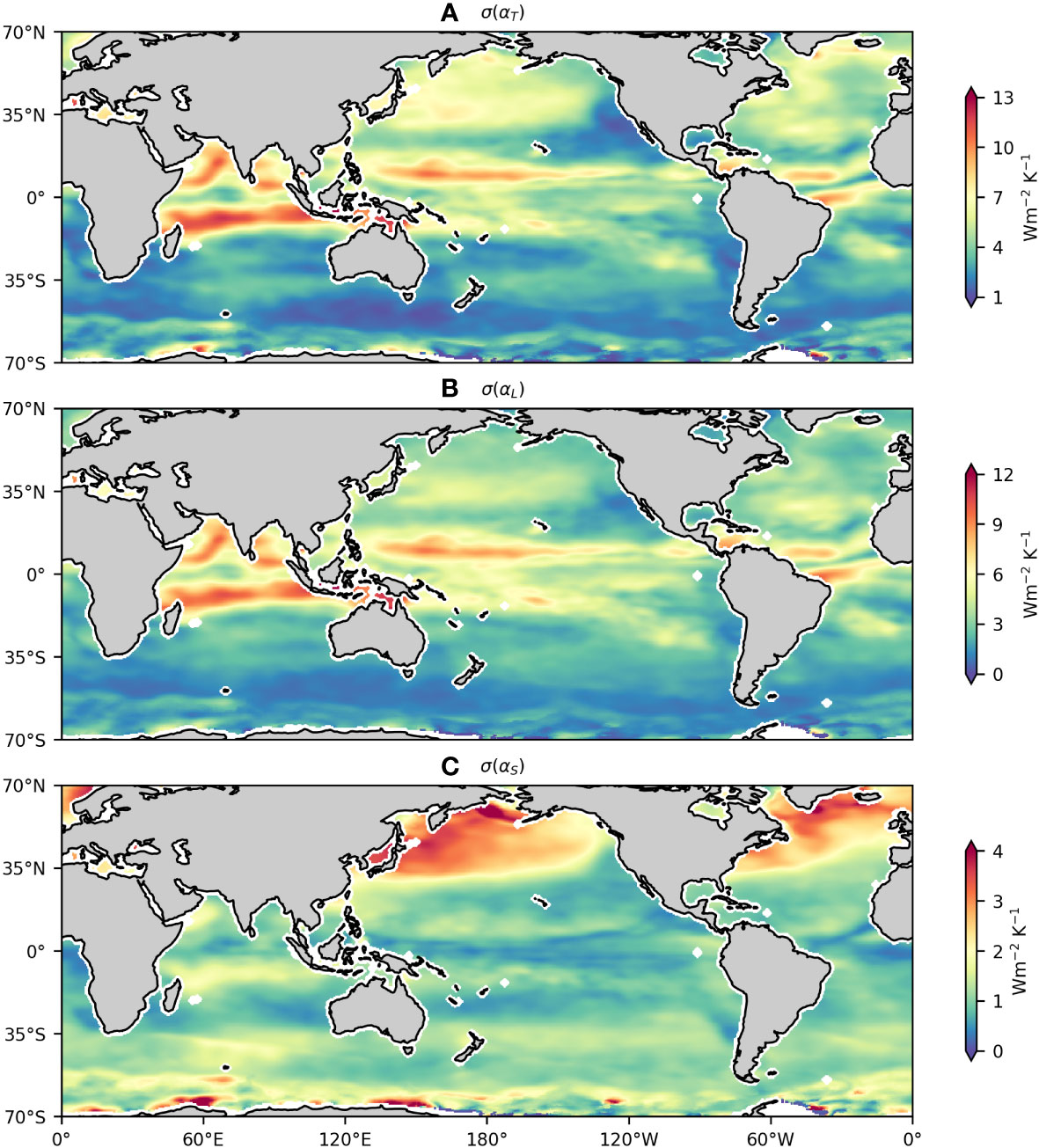
Figure 2 Standard deviation of (A) αT , (B) αL , and (C) αS in the global ocean during 2008–2020. Note that the colorbars are different for panels (A–C).
The temporal variability of αT is primarily attributed to its prominent seasonal cycle (Figure 3). The global mean σ(αT) is about 4.0 W/(m2 K), about 80% of which is contributed by the seasonal cycle of αT . Similar is the case for σ(αS) and σ(αL) . The peaking season of αT varies in space. The αT mostly peaks in autumn or winter off the tropics, whereas it generally peaks in boreal summer or autumn in the tropical region. The peaking seasons of αL and αS are the same in many parts of the tropics. However, they differ from each other off the tropics. Specifically, αL generally reaches its maximum in autumn, whereas αS peaks in winter.
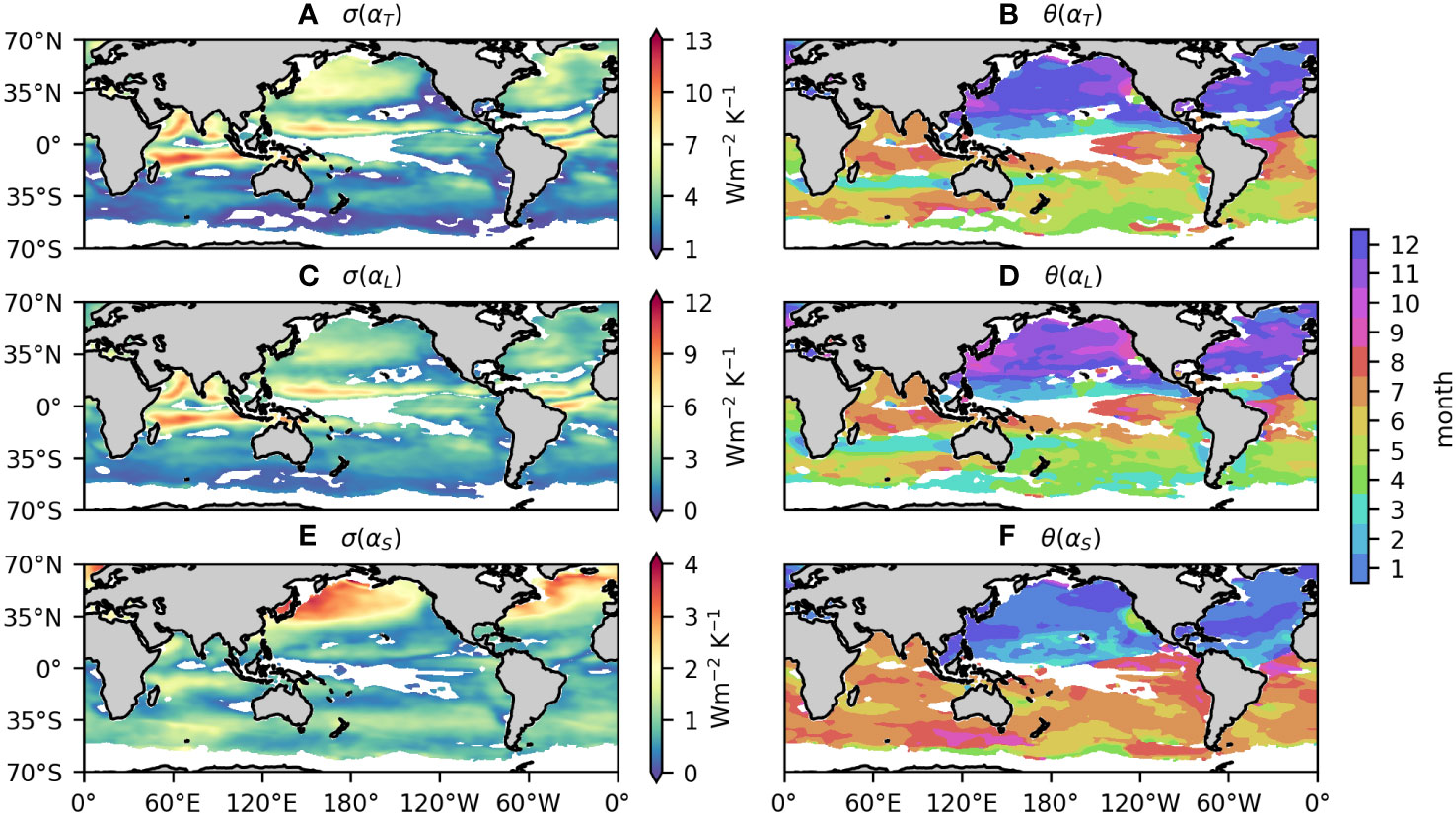
Figure 3 (A) Standard deviation and (B) peaking time of seasonal cycle of αT in the global ocean during 2008–2020. Here the peaking time of seasonal cycle is defined as the month when αT reaches its maximum. Regions with statistically insignificant seasonal cycles at the 95% confidence level or seasonally covered by the sea ice are masked by white. (C, D) and (E, F) Same as panels (A, B) but for αL and αS , respectively. Note that the colorbars are different for panels (A, C, E).
3.2 Underlying dynamics
In this section, we attempt to uncover the underlying dynamics for the spatio-temporal variability of αT . The αL and αS are controlled by the background atmospheric and oceanic states and different kinds of MABL adjustment to the underlying mesoscale SSTA. Following Hausmann et al. (2017) and Yang et al. (2018), their respective contributions under the assumption of a small magnitude of mesoscale SSTA can be estimated as follows:
(see Appendix A for derivation details) where ρa is the surface (2 m) air density, ΛV is the latent heat of vaporization, q is the surface (2 m) air-specific humidity, qsat is its saturated value at SST (denoted as T ), Tair is the surface (2 m) air temperature, U10 is the surface (10 m) wind speed, cp is the surface (2 m) air-specific heat capacity, and Ce and Ch are the transfer coefficients for evaporation and sensible heat. The overbar and prime denote the large-scale background value and mesoscale anomaly, respectively. The first terms ( and ) on the right-hand side of Equations. (4) and (5) are determined entirely by the background atmospheric and oceanic states. The second terms including the minus signs ( and ) are known as the thermodynamic adjustment and depend on the change of q′ and T′air induced by T′ . The third terms ( and ), i.e., the dynamic adjustment, originate from the response of U′10 to T′. The second and third terms can be estimated by regressing q′ (T′air) and U′10 onto T′ based on the GTWR model. It should be noted that the spatio-temporal variability of the second and third terms is not only determined by the MABL adjustment to underlying mesoscale SSTA but also affected by the background atmospheric and oceanic states. The values of αL,A and αS,A are found to agree well with αL and αS (Figures 4, 5), respectively, lending support to the validity of Equations. (4) and (5).
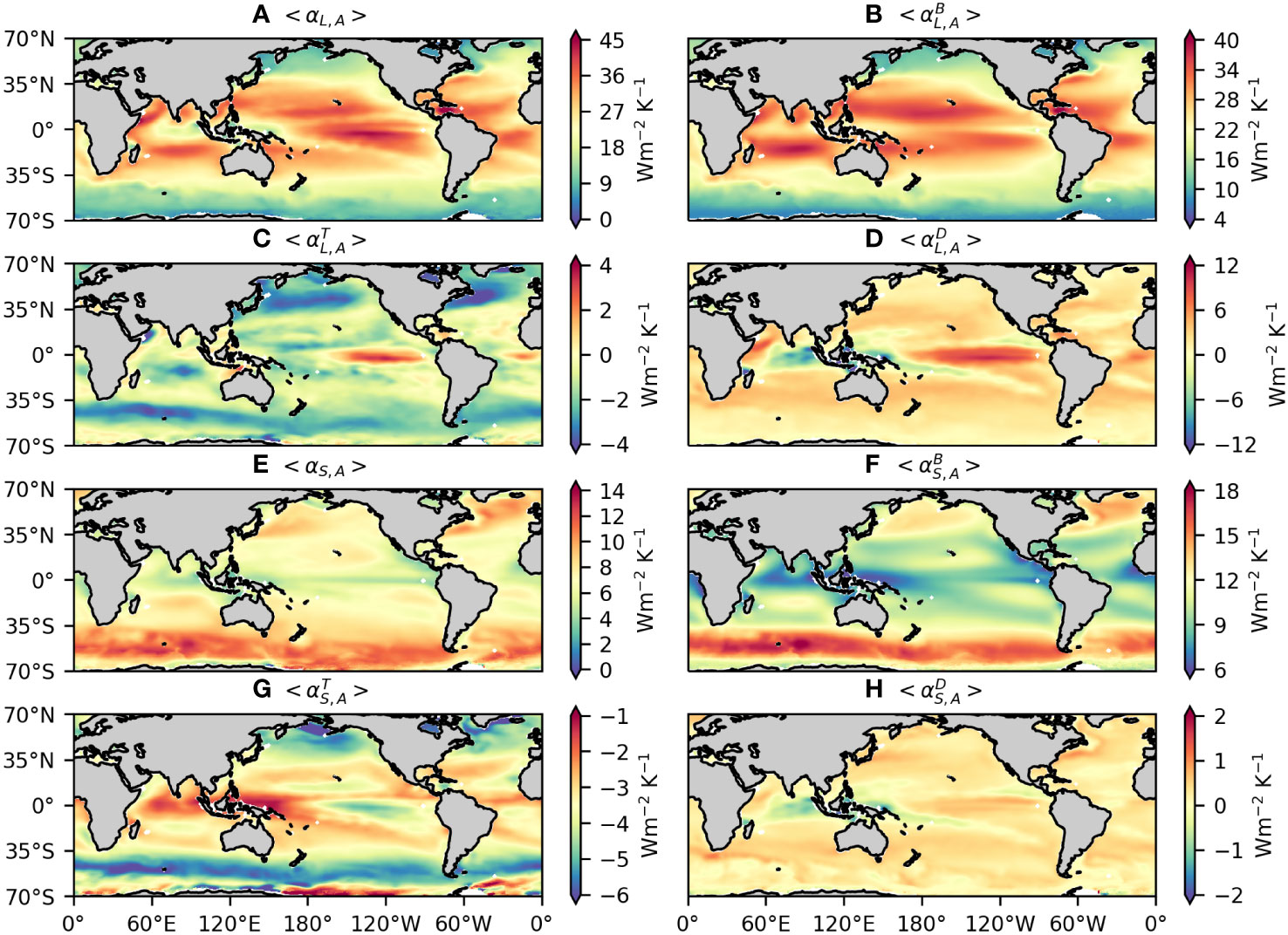
Figure 4 Decomposition of <αL> into components related to the (B) background atmospheric and oceanic states , (C) thermodynamic adjustment , (D) dynamic adjustment , and (A) the sum of the above three components <αL,A> . (E–H) Same as panels (A–D) but for <αS> . Note that the colorbars are different for different panels.
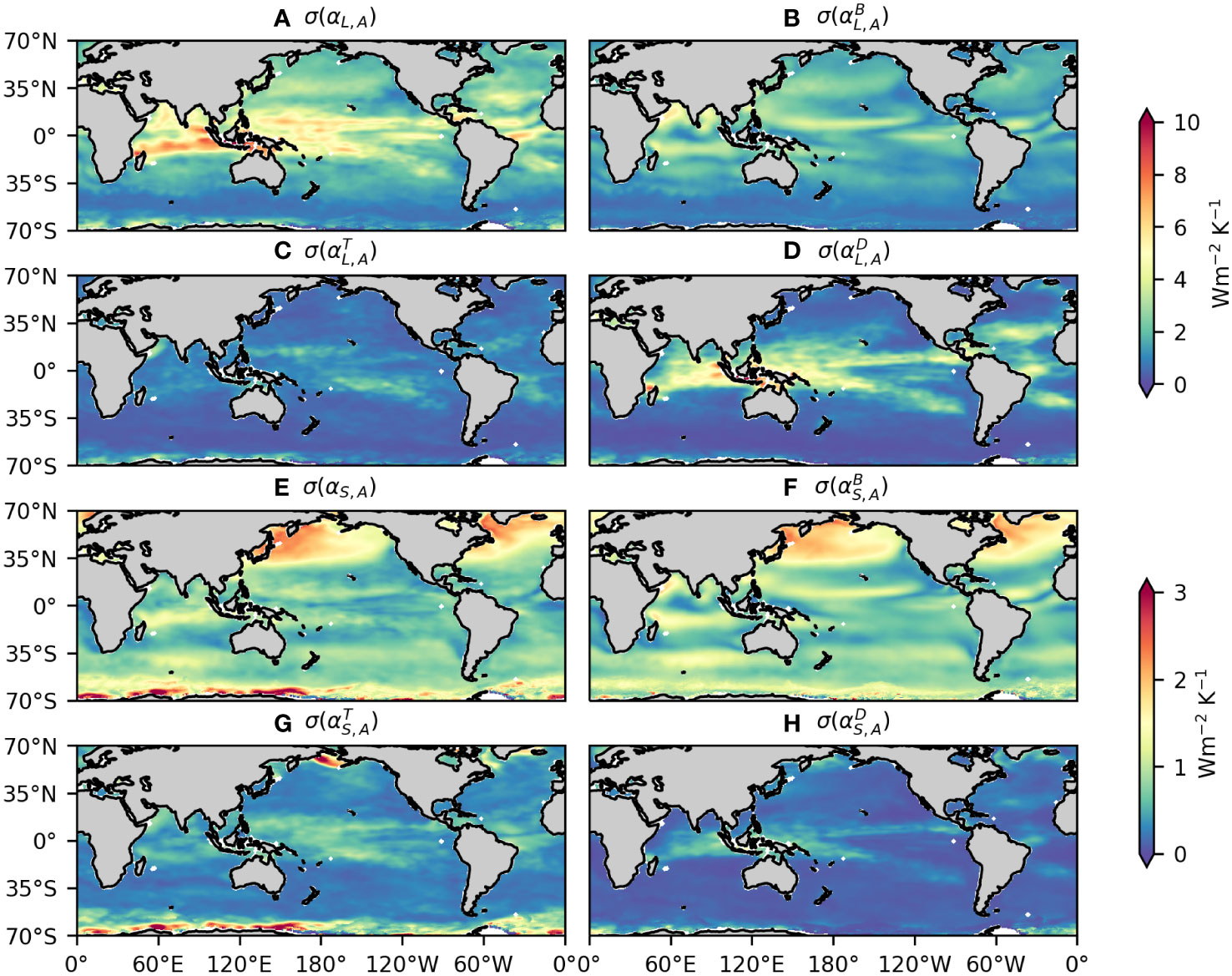
Figure 5 Same as Figure 4 but for σ(αL) and σ(αS) .
The spatial variability of <αL> is largely attributed to (Figures 4A, B). Their pattern correlation coefficient is 0.97, much larger than 0.31 (0.44) between <αL> and (). The spatial pattern of results primarily from the non-linearity in the Clausius–Clapeyron relation; i.e., is an increasing function of (Figure 6A). Such a feature of accounts for the larger in the tropics than off-tropical regions and the minimal in the Southern Ocean. The spatial inhomogeneity in also plays a role (Figures 6C, E). On the one hand, is enhanced under the storm tracks, partially compensating for the reduced off the tropics especially in the Southern Ocean due to . On the other hand, is weakened in the Indo-Pacific warm pool, accounting for the local minimum of in this region.
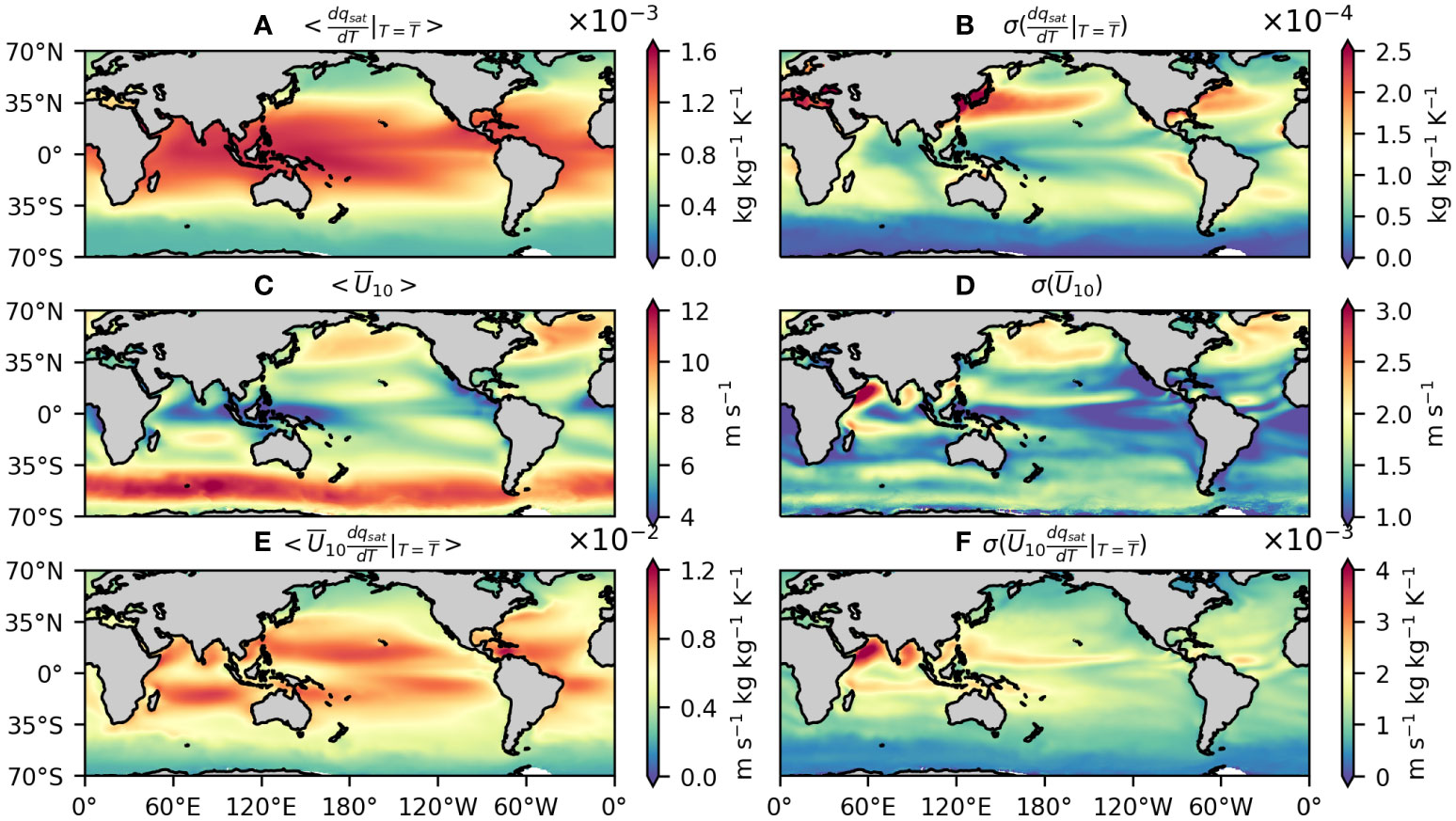
Figure 6 Time-mean (A) , (C) , and (E) their product in the global ocean during 2008–2020. (B, D, F) Same as panels (A, C, E) but for the standard deviation of , , and .
Although determines the overall spatial structure of <αL> , there is an evident discrepancy between these two quantities in some parts of the global ocean, suggesting that and may be locally important (Figures 4C, D). In particular, <αL> reaches a local maximum in the equatorial cold tongues, whereas the opposite is true for . This discrepancy is due to the strong adjustment of surface air-specific humidity and wind speed to mesoscale SSTA in these regions. Both and contribute positively to <αL> in the equatorial cold tongues, with their sum comparable to . Moreover, makes a non-negligible contribution to the enhanced <αL> along the WBCs and their extensions.
As to <αS> , its spatial variability is also dominated by that of with a pattern correlation coefficient of 0.78 (Figures 4E, F). However, is systematically larger than <αS> , especially in the off-tropical regions. This bias is mainly attributed to that is always negative and becomes larger in magnitude as the latitude increases (Figure 4G). The effect of on <αS> is generally weaker than that of but could be locally important in some parts of the global ocean (Figure 4H).
We next examine the underlying dynamics for the temporal variability of αL and αS . Both and make important contributions to σ(αL) but in different regions (Figures 5A–D). The off-tropical σ(αL) is mainly attributed to , whereas becomes dominant in the tropics. The temporal variability of in the off-tropical region is mainly caused by the combined effects of and (Figures 6B, D, F). Recomputing by setting and as their time-mean values largely suppresses the temporal variability of (Figure 7A). As and are largest in summer and winter, respectively, their effects on the temporal variability of counteract each other. Indeed, taking either or alone as its time-mean value will make unchanged or even larger (Supplementary Figure S2). This to some extent explains why αL peaks in autumn off the tropics. As to , its temporal variability in the tropics is primarily due to dU′10/dT′ (Figure 7B), suggesting that the response of surface wind speed to mesoscale SSTA varies evidently with time in this region.
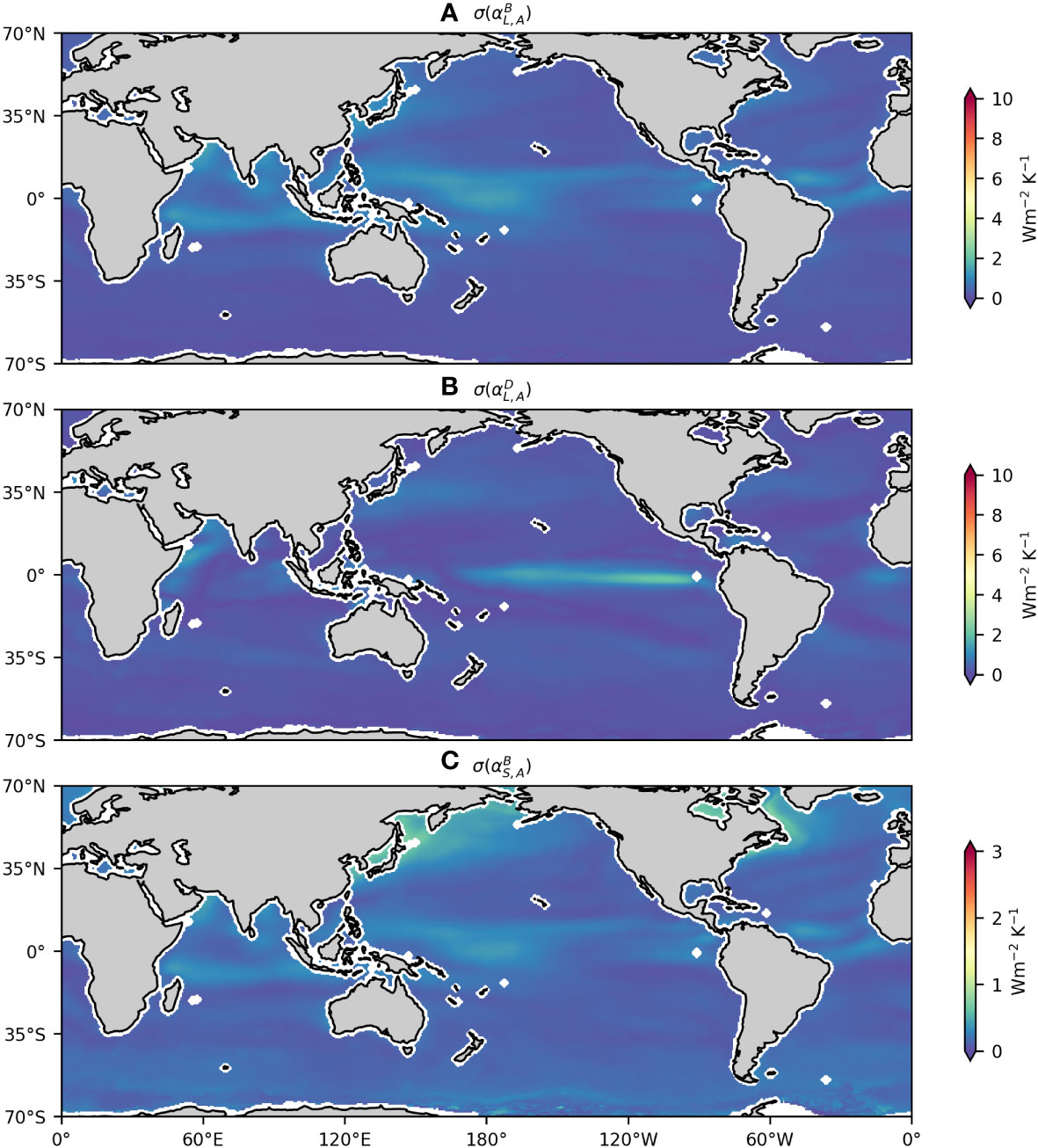
Figure 7 Standard deviation of (A) recomputed by using time-mean and , (B) recomputed by using time-mean dU′10/dT′ , and (C) recomputed by using time-mean . These quantities should be compared to their counterparts in Figure 5.
The temporal variability of αS is mainly attributed to , with and playing a minor or negligible role (Figures 5E–H). The temporal variability of off the tropics results from (Figures 6D, 7C), accounting for the peak of αS in winter. Finally, it should be noted that the off-tropical temporal variability of , , and their product in the northern hemisphere is more evident than their counterpart in the southern hemisphere (Figures 6B, D, F). This contributes to the asymmetry of σ(αL) and σ(αS) off the tropics between the two hemispheres.
4 Discussion
The above analysis suggests that the thermodynamic and dynamic adjustments have important effects on the spatio-temporal variability of αT . In this section, we discuss several notable features of these adjustments that are unexpected from the prevailing thoughts (Barsugli and Battisti, 1998; Xie, 2004; Chelton et al., 2004; Hausmann et al., 2017; Yang et al., 2018). First, classical theories (Barsugli and Battisti, 1998; Hausmann et al., 2017) suggest that the thermodynamic adjustment should reduce αT because a warm (cold) mesoscale SSTA induces a surface heat and moisture flux anomaly into (out of) the atmosphere, increasing (decreasing) the surface air temperature and specific humidity. However, our results reveal that is not always negative. In particular, it reinforces <αL> in the equatorial cold tongues (Figure 4C). This apparently odd feature is not an artifact caused by the GTWR model, as it is also consistently reproduced by the classical constant regression model (Supplementary Figure S3). Instead, it may be explained by the “vertical mixing mechanism” (Wallace et al., 1989; Seo et al., 2007; Chelton and Xie, 2010; Frenger et al., 2013; Putrasahan et al., 2013; Laurindo et al., 2019). A warm mesoscale SSTA heats the MABL from the bottom, reducing the stability and enhancing the vertical mixing in the MABL. The opposite is true for a cold mesoscale SSTA. Due to the large negative vertical gradient of air-specific humidity within the MABL over the equatorial cold tongues (Bond, 1992), the enhanced (weakened) vertical mixing may decrease (increase) the surface air-specific humidity over the warm (cold) mesoscale SSTA, leading to positive . Indeed, the efficacy of the vertical mixing mechanism, measured as the regression coefficient of mesoscale MABL height anomaly onto SSTA, is largest in the equatorial cold tongues (Figure 8).
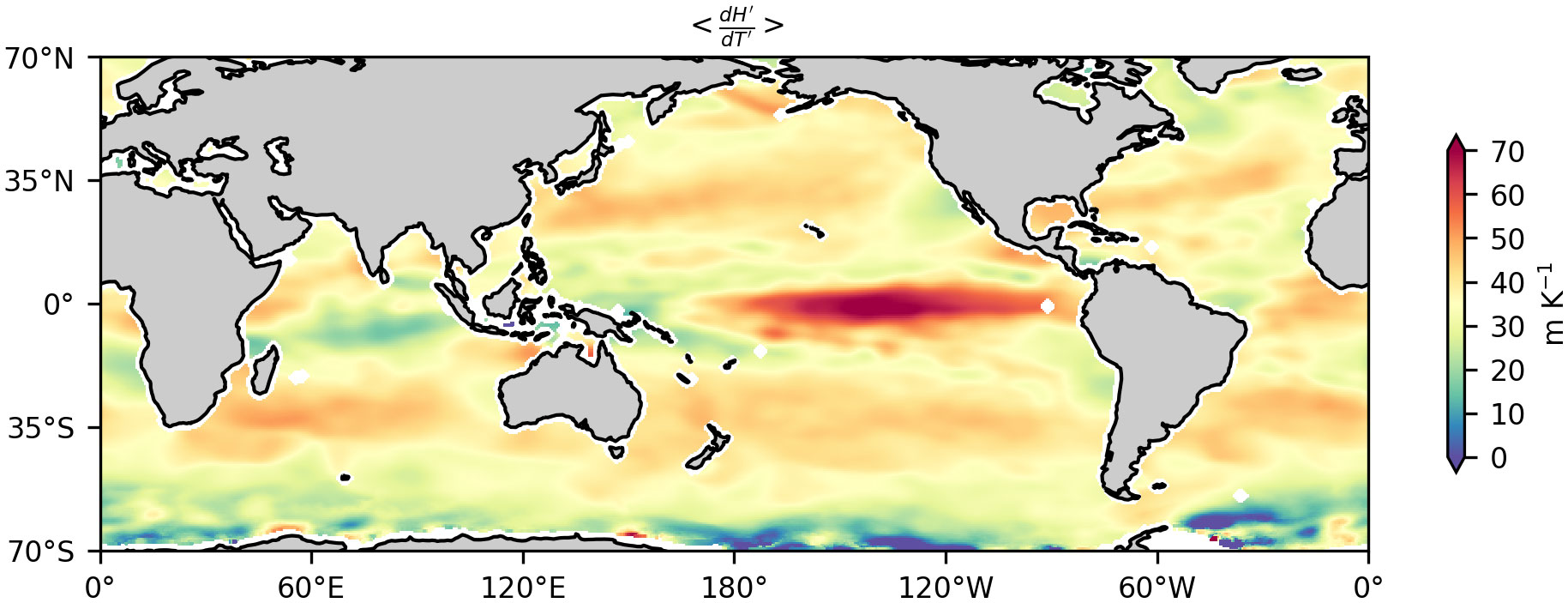
Figure 8 Time-mean regression coefficient of mesoscale MABL height (H) anomaly onto SSTA in the global ocean. MABL, marine atmospheric boundary layer; SSTA, sea surface temperature anomaly.
Second, it is generally thought that the mesoscale air–sea interactions could cause a positive correlation between U′10 and T′ via the vertical momentum mixing or pressure gradient effects (Lindzen and Nigam, 1987; Chelton et al., 2004; Xie, 2004; Small et al., 2008; Chelton and Xie, 2010; Frenger et al., 2013; Ma et al., 2015; Laurindo et al., 2019) so that the dynamic adjustment increases αT . Such a thought is consistent with the positive and over the majority of the global ocean. However, there are some regions where and are significantly negative, especially in the Indo-Pacific warm pool. These negative values are not an artifact of the GTWR model (Supplementary Figure S4). As the regression analysis cannot distinguish the cause and effect, one possibility might be that the negative and there reflect the forced response of T′ by U′10 . A positive U′10 could generate a negative T′ by increasing the heat flux out of the ocean and entrainment of cooler thermocline water into the surface layer. At this stage, the underlying dynamics for the negative correlation between U′10 and T′ remains unknown and deserves further analysis in future studies.
5 Conclusions
In this study, we quantify the spatio-temporal variability of surface turbulent heat flux feedback for mesoscale SSTA over the global ocean, based on the GTWR model. The major conclusions are summarized as follows.
First, there is pronounced spatial variability in <αT> with its value generally ranging from 0 to 50 W/(m2 K) within 70°S–70°N. It is larger in the tropics than off-tropical regions and shows local enhancement in the equatorial cold tongues and WBCs as well as their extensions. The overall spatial pattern of <αT> is primarily attributed to the non-linear Clausius–Clapeyron relation and inhomogeneous background wind speed. The thermodynamic and dynamic adjustments play an important role in the regional variability, accounting for the large <αT> in the equatorial cold tongues and contributing to the enhanced <αT> along the WBCs as well as their extensions.
Second, the temporal variability of αT is mainly ascribed to its seasonal cycle. The amplitude and peaking time of αT seasonal cycle vary in space. The strong seasonal cycle occurs in the tropics and under the storm tracks in the northern hemisphere. The former is primarily caused by the seasonally varying response of surface wind speed to mesoscale SSTA, whereas the latter is due to the seasonality of background atmospheric and oceanic states.
The superiority of the GTWR model over the classical constant regression model enables it to uncover more features of αT that have not been reported by previous studies. Nevertheless, the GTWR model is incapable of analyzing any potential non-linearity in αT , e.g., the dependence of αT on the magnitude of mesoscale SSTA (e.g., Moreton et al., 2021) and the asymmetry of αT between warm and cold mesoscale SSTA. These issues deserve analysis in future studies but require more advanced regression models.
Data availability statement
The original contributions presented in the study are included in the article/Supplementary Material. Further inquiries can be directed to the corresponding author.
Author contributions
All authors contributed to the manuscript and approved the submitted version.
Funding
This research is supported by the National Science Foundation of China (41906011).
Acknowledgments
We thank Dr. Fengfei Song for his insightful advice. The transfer coefficients for evaporation and sensible heat in the bulk formula are calculated by AeroBulk (https://github.com/brodeau/aerobulk) (Brodeau et al., 2017).
Conflict of interest
The authors declare that the research was conducted in the absence of any commercial or financial relationships that could be construed as a potential conflict of interest.
Publisher’s note
All claims expressed in this article are solely those of the authors and do not necessarily represent those of their affiliated organizations, or those of the publisher, the editors and the reviewers. Any product that may be evaluated in this article, or claim that may be made by its manufacturer, is not guaranteed or endorsed by the publisher.
Supplementary material
The Supplementary Material for this article can be found online at: https://www.frontiersin.org/articles/10.3389/fmars.2022.957796/full#supplementary-material
References
Barsugli J. J., Battisti ,. D. S. (1998). The basic effects of atmosphere–ocean thermal coupling on midlatitude variability*. J. Atmos. Sci. 55, 477–493. doi: 10.1175/1520-0469(1998)055<0477:TBEOAO>2.0.CO;2
Bishop S. P., Small R. J., Bryan F. O. (2020). The global sink of available potential energy by mesoscale air-Sea interaction. J. Adv. Model. Earth Syst. 12, e2020MS002118. doi: 10.1029/2020MS002118
Bond N. A. (1992). Observations of planetary boundary-layer structure in the Eastern equatorial pacific. J. Clim. 5, 699–706. doi: 10.1175/1520-0442(1992)005<0699:OOPBLS>2.0.CO;2
Brodeau L., Barnier B., Gulev S. K., Woods C. (2017). Climatologically significant effects of some approximations in the bulk parameterizations of turbulent air–Sea fluxes. J. Phys. Oceanogr. 47, 5–28. doi: 10.1175/JPO-D-16-0169.1
Brunsdon C., Fotheringham A. S., Charlton M. E. (1996). Geographically weighted regression: A method for exploring spatial nonstationarity. Geogr. Anal. 28, 281–298. doi: 10.1111/J.1538-4632.1996.TB00936.X
Bryan F. O., Tomas R., Dennis J. M., Chelton D. B., Loeb N. G., Mcclean J. L. (2010). Frontal scale air-sea interaction in high-resolution coupled climate models. J. Clim. 23, 6277–6291. doi: 10.1175/2010JCLI3665.1
Chelton D. B., Schlax M. G., Freilich M. H., Milliff R. F. (2004). Satellite measurements reveal persistent small-scale features in ocean winds. Sci. (80-. ) 303(5660), 978–983. doi: 10.1126/science.1091901
Chelton D., Xie S.-P. (2010). Coupled ocean-atmosphere interaction at oceanic mesoscales. Oceanography 23, 52–69. doi: 10.5670/oceanog.2010.05
Fotheringham A. S., Brunsdon C., Charlton M. (2002). Geographically weighted regression: The analysis of spatially varying relationships. 269. The Atrium, Southern Gate, Chichester, West Sussex PO19 8SQ, England: John Wiley & Sons Ltd.
Fotheringham A., Charlton M., Brunsdon C. (1996). The geography of parameter space: An investigation of spatial non-stationarity. Int. J. Geogr. Inf. Syst. 10, 605–627. doi: 10.1080/02693799608902100
Fotheringham A. S., Crespo R., Yao J. (2015). Geographical and temporal weighted regression (GTWR). Geogr. Anal. 47, 431–452. doi: 10.1111/gean.12071
Frankignoul C. (1985). Sea Surface temperature anomalies, planetary waves, and air-sea feedback in the middle latitudes. Rev. Geophys. 23, 357. doi: 10.1029/RG023i004p00357
Frankignoul C., Czaja A., L’Heveder B. (1998). Air–Sea feedback in the north Atlantic and surface boundary conditions for ocean models. J. Clim. 11, 2310–2324. doi: 10.1175/1520-0442(1998)011<2310:ASFITN>2.0.CO;2
Frankignoul C., Hasselmann K. (1977). Stochastic climate models, part II application to sea-surface temperature anomalies and thermocline variability. Tellus 29, 289–305. doi: 10.3402/tellusa.v29i4.11362
Frankignoul C., Kestenare E. (2002). The surface heat flux feedback. part I: Estimates from observations in the Atlantic and the north pacific. Clim. Dyn. 19, 633–648. doi: 10.1007/s00382-002-0252-x
Frenger I., Gruber N., Knutti R., Münnich M. (2013). Imprint of southern ocean eddies on winds, clouds and rainfall. Nat. Geosci. 6, 608–612. doi: 10.1038/ngeo1863
Guo Y., Bishop S., Bryan F., Bachman S. (2022). A global diagnosis of eddy potential energy budget in an eddy permitting ocean model. J. Phys. Oceanogr. 52(8), 1731–1748. doi: 10.1175/JPO-D-22-0029.1
Hausmann U., Czaja A. (2012). The observed signature of mesoscale eddies in sea surface temperature and the associated heat transport. Deep. Res. Part I Oceanogr. Res. Pap. 70, 60–72. doi: 10.1016/j.dsr.2012.08.005
Hausmann U., Czaja A., Marshall J. (2016). Estimates of air-sea feedbacks on sea surface temperature anomalies in the southern ocean. J. Clim. 29, 439–454. doi: 10.1175/JCLI-D-15-0015.1
Hausmann U., Czaja A., Marshall J. (2017). Mechanisms controlling the SST air-sea heat flux feedback and its dependence on spatial scale. Clim. Dyn. 48, 1297–1307. doi: 10.1007/s00382-016-3142-3
Hersbach H., Bell B., Berrisford P., Hirahara S., Horányi A., Muñoz-Sabater J., et al. (2020). The ERA5 global reanalysis. Q. J. R. Meteorol. Soc 146, 1999–2049. doi: 10.1002/qj.3803
Huang B., Wu B., Barry M. (2010). Geographically and temporally weighted regression for modeling spatio-temporal variation in house prices. Int. J. Geogr. Inf. Sci. 24, 383–401. doi: 10.1080/13658810802672469
Large W. G., Yeager S. G. (2004). Diurnal to decadal global forcing for ocean and sea-ice models: The data sets and flux climatologies. doi: 10.5065/D6KK98Q6
Laurindo L. C., Siqueira L., Mariano A. J., Kirtman B. P. (2019). Cross-spectral analysis of the SST/10-m wind speed coupling resolved by satellite products and climate model simulations. Clim. Dyn. 52, 5071–5098. doi: 10.1007/s00382-018-4434-6
Lindzen R. S., Nigam S. (1987). On the role of Sea surface temperature gradients in forcing low-level winds and convergence in the tropics. J. Atmos. Sci. 44, 2418–2436. doi: 10.1175/1520-0469(1987)044<2418:OTROSS>2.0.CO;2
Li F., Sang H., Jing Z. (2017). Quantify the continuous dependence of SST-turbulent heat flux relationship on spatial scales. Geophys. Res. Lett. 44, 6326–6333. doi: 10.1002/2017GL073695
Ma X., Jing Z., Chang P., Liu X., Montuoro R., Small R. J., et al. (2016). Western Boundary currents regulated by interaction between ocean eddies and the atmosphere. Nature 535, 533–537. doi: 10.1038/nature18640
Ma J., Xu H., Dong C., Lin P., Liu Y. (2015). Atmospheric responses to oceanic eddies in the kuroshio extension region. J. Geophys. Res. Atmos. 120, 6313–6330. doi: 10.1002/2014JD022930
Moreton S., Ferreira D., Roberts M., Hewitt H. (2021). Air-Sea turbulent heat flux feedback over mesoscale eddies. Geophys. Res. Lett. 48, e2021GL095407. doi: 10.1029/2021GL095407
Park S., Deser C., Alexander M. A. (2005). Estimation of the surface heat flux response to Sea surface temperature anomalies over the global oceans. J. Clim. 18, 4582–4599. doi: 10.1175/JCLI3521.1
Putrasahan D. A., Miller A. J., Seo H. (2013). Isolating mesoscale coupled ocean–atmosphere interactions in the kuroshio extension region. Dyn. Atmos. Ocean. 63, 60–78. doi: 10.1016/j.dynatmoce.2013.04.001
Seo H., Miller A. J., Roads J. O. (2007). The Scripps coupled ocean–atmosphere regional (SCOAR) model, with applications in the Eastern pacific sector. J. Clim. 20, 381–402. doi: 10.1175/JCLI4016.1
Shan X., Jing Z., Gan B., Wu L., Chang P., Ma X., et al. (2020a). Surface heat flux induced by mesoscale eddies cools the kuroshio-oyashio extension region. Geophys. Res. Lett. 47, e2019GL086050. doi: 10.1029/2019GL086050
Shan X., Jing Z., Sun B., Chang P., Wu L., Ma X. (2020b). Influence of the ocean mesoscale eddy–atmosphere thermal feedback on the upper-ocean haline stratification. J. Phys. Oceanogr. 50, 2475–2490. doi: 10.1175/JPO-D-19-0193.1
Small R. J., Bryan F. O., Bishop S. P., Tomas R. A. (2019). ). air–Sea turbulent heat fluxes in climate models and observational analyses: What drives their variability? J. Clim. 32, 2397–2421. doi: 10.1175/JCLI-D-18-0576.1
Small R. J., deSzoeke S. P., Xie S. P., O’Neill L., Seo H., Song Q., et al. (2008). Air–sea interaction over ocean fronts and eddies. Dyn. Atmos. Ocean. 45, 274–319. doi: 10.1016/j.dynatmoce.2008.01.001
Wallace J. M., Mitchell T. P., Deser C. (1989). The influence of Sea-surface temperature on surface wind in the Eastern equatorial pacific: Seasonal and interannual variability. J. Clim. 2, 1492–1499. doi: 10.1175/1520-0442(1989)002<1492:TIOSST>2.0.CO;2
Xie S.-P. (2004). Satellite observations of cool ocean–atmosphere interaction. Bull. Am. Meteorol. Soc 85, 195–208. doi: 10.1175/BAMS-85-2-195
Yang H., Chang P., Qiu B., Zhang Q., Wu L., Chen Z., et al. (2019). Mesoscale air–Sea interaction and its role in eddy energy dissipation in the kuroshio extension. J. Clim. 32, 8659–8676. doi: 10.1175/JCLI-D-19-0155.1
Yang P., Jing Z., Wu L. (2018). An assessment of representation of oceanic mesoscale eddy-atmosphere interaction in the current generation of general circulation models and reanalyses. Geophys. Res. Lett. 45, 11,856–11,865. doi: 10.1029/2018GL080678
Appendix A derivations of equations (4) and (5)
According to the bulk formula (Large and Yeager, 2004), latent (QL ) and sensible heat fluxes (QS ) can be formulated as follows:
where QL and QS are defined positive upwards.
Decompose the quantities in Equations. (A1) and (A2) into the large-scale background values and mesoscale anomalies. Linearizing Equations. (A1) and (A2) with respect to the mesoscale anomalies yields the following:
where we have dropped the mesoscale anomalies for ρa , ΛV , cp , Ce , and Ch , as these terms are negligible. Differentiating Equations. (A3) and (A4) with respect to T′ yields Equations. (4) and (5).
Keywords: surface turbulent heat flux feedback, mesoscale, spatio-temporal variability, geographically and temporally weighted regression, marine atmospheric boundary layer adjustment
Citation: Yuan M, Li F, Ma X and Yang P (2022) Spatio-temporal variability of surface turbulent heat flux feedback for mesoscale sea surface temperature anomaly in the global ocean. Front. Mar. Sci. 9:957796. doi: 10.3389/fmars.2022.957796
Received: 31 May 2022; Accepted: 16 September 2022;
Published: 05 October 2022.
Edited by:
Chunyan Li, Louisiana State University, United StatesReviewed by:
Dian Putrasahan, Max Planck Society, GermanyJing Ma, Nanjing University of Information Science and Technology, China
Copyright © 2022 Yuan, Li, Ma and Yang. This is an open-access article distributed under the terms of the Creative Commons Attribution License (CC BY). The use, distribution or reproduction in other forums is permitted, provided the original author(s) and the copyright owner(s) are credited and that the original publication in this journal is cited, in accordance with accepted academic practice. No use, distribution or reproduction is permitted which does not comply with these terms.
*Correspondence: Furong Li, bGlmdXJvbmdAb3VjLmVkdS5jbg==