Advancing fishery-independent stock assessments for the Norway lobster (Nephrops norvegicus) with new monitoring technologies
- 1Functioning and Vulnerability of Marine Ecosystems Group, Department of Renewable Marine Resources, Institute of Marine Sciences (ICM-CSIC), Spanish National Research Council, Barcelona, Spain
- 2Anton Dohrn Zoological Station, Naples, Italy
- 3Marine Institute, Fisheries and Ecosystem Advisory Services, Oranmore, Ireland
- 4Computer Vision and Robotics Institute, University of Girona, Girona, Spain
- 5Research Center for Engineering and Agro-Food Processing, Council for Agricultural Research and Economics, Rome, Italy
- 6Remote Acquisition and Data Processing Systems in Marine Environment (SARTI-MAR) Research Group, Electronic Engineering Department, Polytechnic University of Catalunya (UPC), Vilanova i la Geltrú, Spain
- 7Fisheries Biology Laboratory, Fisheries Sciences and Technologies Research Unit, Department of Biological Resources and Environment, French Research Institute for Exploitation of the Sea (IFREMER), Plouzané, France
- 8GEOMAR Helmholtz Centre for Ocean Research Kiel, Kiel, Germany
- 9Department of Ocean System Sciences, NIOZ Royal Netherlands Institute for Sea Research and Utrecht University, Den Burg, Netherlands
- 10Demersal Division, Marine and Freshwater Research Institute, Hafnarfjörður, Iceland
- 11Department of Aquatic Resources (SLU Aqua), Swedish University of Agricultural Sciences, Lysekil, Sweden
- 12Agri-Food and Biosciences Institute (AFBI), Belfast, Northern Ireland, United Kingdom
- 13Institute of Marine Sciences (CNR-ISMAR), National Research Council, La Spezia, Italy
- 14Institute of Marine Biological Resources and Biotechnologies (CNR-IRBIM), National Research Council, Ancona, Italy
- 15Department of Natural Resources and the Environment, Marine and Freshwater Research Centre, School of Science and Computing, Galway-Mayo Institute of Technology, Galway, Ireland
- 16Alantic Technological University, Galway, Ireland
- 17Science and Technology Unit, Umm al Qura Univeristy, Makkah, Saudi Arabia
- 18Institute of Ocean Engineering (IIO), University of Málaga, Málaga, Spain
- 19Department of Excellence in Robotics and AI, Sant’Anna School of Advanced Studies (SSSA), The BioRobotics Institute, Pisa, Italy
- 20Division of Modelling and Management of Fisheries Resources (DivRP), Portuguese Institute for Sea and Atmosphere (IPMA), Lisbon, Portugal
- 21Cádiz Oceanographic Center, Spanish Institute of Oceanography (IEO), Cádiz, Spain
- 22Marine Scotland Science, Aberdeen, Scotland, United Kingdom
The Norway lobster, Nephrops norvegicus, supports a key European fishery. Stock assessments for this species are mostly based on trawling and UnderWater TeleVision (UWTV) surveys. However, N. norvegicus are burrowing organisms and these survey methods are unable to sample or observe individuals in their burrows. To account for this, UWTV surveys generally assume that “1 burrow system = 1 animal”, due to the territorial behavior of N. norvegicus. Nevertheless, this assumption still requires in-situ validation. Here, we outline how to improve the accuracy of current stock assessments for N. norvegicus with novel ecological monitoring technologies, including: robotic fixed and mobile camera-platforms, telemetry, environmental DNA (eDNA), and Artificial Intelligence (AI). First, we outline the present status and threat for overexploitation in N. norvegicus stocks. Then, we discuss how the burrowing behavior of N. norvegicus biases current stock assessment methods. We propose that state-of-the-art stationary and mobile robotic platforms endowed with innovative sensors and complemented with AI tools could be used to count both animals and burrows systems in-situ, as well as to provide key insights into burrowing behavior. Next, we illustrate how multiparametric monitoring can be incorporated into assessments of physiology and burrowing behavior. Finally, we develop a flowchart for the appropriate treatment of multiparametric biological and environmental data required to improve current stock assessment methods.
Introduction
The Norway lobster, Nephrops norvegicus, supports one of the most important fisheries in Europe, with landings of almost 60,000 t (FAO, 2021a), and a first sale income of approximately 300 million € per year1 (Issifu et al., 2022). Most of N. norvegicus global landings are accounted for in the Northeast Atlantic fisheries (FAO zone 27), and the United Kingdom contributes over 56% of them. The Mediterranean Sea (FAO zone 37) in turn, contributes around 7% of total landings (FAO, 2020b). These fisheries are currently showing signs of decline in various regions (Ungfors et al., 2013; FAO, 2021b; Letschert et al., 2021). As such, there is considerable value in developing sustainable harvesting guidelines for accurately quantifying the distribution, abundance, and biomass of N. norvegicus populations over large geographic areas (ICES, 2016). Conventional stock assessment methods for N. norvegicus are based on animal sampling via fishery-dependent trawls or underwater video cameras; however, capture-rates are strongly influenced by this species’ burrowing behavior and its temporal modulation (Aguzzi et al., 2021). There is therefore an opportunity to improve current stock assessment methods through the use of novel tools such as optoacoustic imaging, telemetry, environmental DNA (eDNA) metabarcoding, and Artificial Intelligence (AI) (Aguzzi et al., 2020b). Moreover, with recent development in ecological monitoring technologies (e.g., Aguzzi et al., 2019; Rountree et al., 2020) these tools could be implemented using fishery independent, autonomous and remote robotic platforms. We consider that many of the proposed technologies are expected to work in tandem, as their operational ranges and monitoring targets make them complementary rather than directly competing/comparable. Thus, we believe that ranking these technological solutions is not something within the scope of this manuscript.
This review explores how novel monitoring techniques could improve current stock assessment methodologies for N. norvegicus. Specifically: i. We outline present status and threat for overexploitation in N. norvegicus stocks; ii. We discuss how the burrowing behavior of N. norvegicus biases current stock assessment methods; iii. We propose that state-of-the-art stationary and mobile robotic platforms endowed with innovative sensors and complemented with AI tools could be used to count both animals and burrows systems in-situ, as well as to provide key insights into burrowing behavior; iv. We indicate how multiparametric monitoring can identify the role of the environment in conditioning the physiology and burrowing behavior; and v. We develop a flowchart for data treatment with multiparametric biological and environmental data to improve current stock assessment methods.
Stock exploitation and management strategies for N. norvegicus
N. norvegicus stocks are typically assessed using a combination of fishery dependent data from landings (i.e., biological sampling of catch composition) and fishery independent data from video surveys (see next section). Both biological sampling and surveys are carried out under the Data Collection Framework (DCF; EU, 2008) in various EU countries. Northeast Atlantic stocks of N. norvegicus are managed through Total Allowable Catches (TACs) and quotas set at an ICES sub-area level (EU, 2020). The sub-areas are comprised of ICES statistical rectangles and spatially explicit and uniquely numbered regions referred to as Functional Units (FUs; Figure 1). The FU status (except for Iceland) is assessed by the International Council for the Exploration of the Sea (ICES) through their expert Working Groups (Leocádio et al., 2018). In most FUs, commercial fishery sampling data are combined with UnderWater TeleVision (UWTV) surveys (Figure 2). The UWTV methodology uses seabed video footage or high definition images captured by a camera mounted on a towed sledge to identify N. norvegicus burrow systems within a known surface area (e.g., Morello et al., 2007). Burrow systems are visually counted by trained experts (Dobby et al., 2021). Footage is streamed in real time on board research vessels through an armored cable and recorded so that it can be reviewed in detail whilst aboard (or back on land) by at least two independent experts. A third review is occasionally required when a significant discrepancy between the first pair of counts is highlighted by the Lin’s Concordance Correlation Coefficient (CCC; Lin, 1989), which is applied to quality check data for outliers before final inclusion in assessments. Over the last decades, especially in the context of the ICES Working Group on Nephrops Surveys (WGNEPS), technical protocols for both camera system set-up, training procedures and data analysis have been agreed internationally to establish a consistent, efficient and effective UWTV approach throughout Europe (ICES, 2017; Dobby et al., 2021). The number of stocks routinely assessed by UWTV surveys has increased over time to twenty FUs in 2022. Stock assessments using relative abundance or biomass indices from trawl surveys in production models are also used in some FUs, where results indicate they had been overfished but overfishing was not currently occurring (Figure 3) (Abad et al., 2021). Based on data from Iceland, Scotland and Ireland we have estimated that the cost of UWTV monitoring is approximately 1 to 2% of the landings value for FU1 (Iceland); FU7 to 13 inclusive and FU34 (Scotland); FU16 to 17 and FU19 to 22 (Ireland). This is in line with monitoring costs reported for other OECD countries which range from 1 to 11% with an average of 2.6% (Kelleher, 2002).
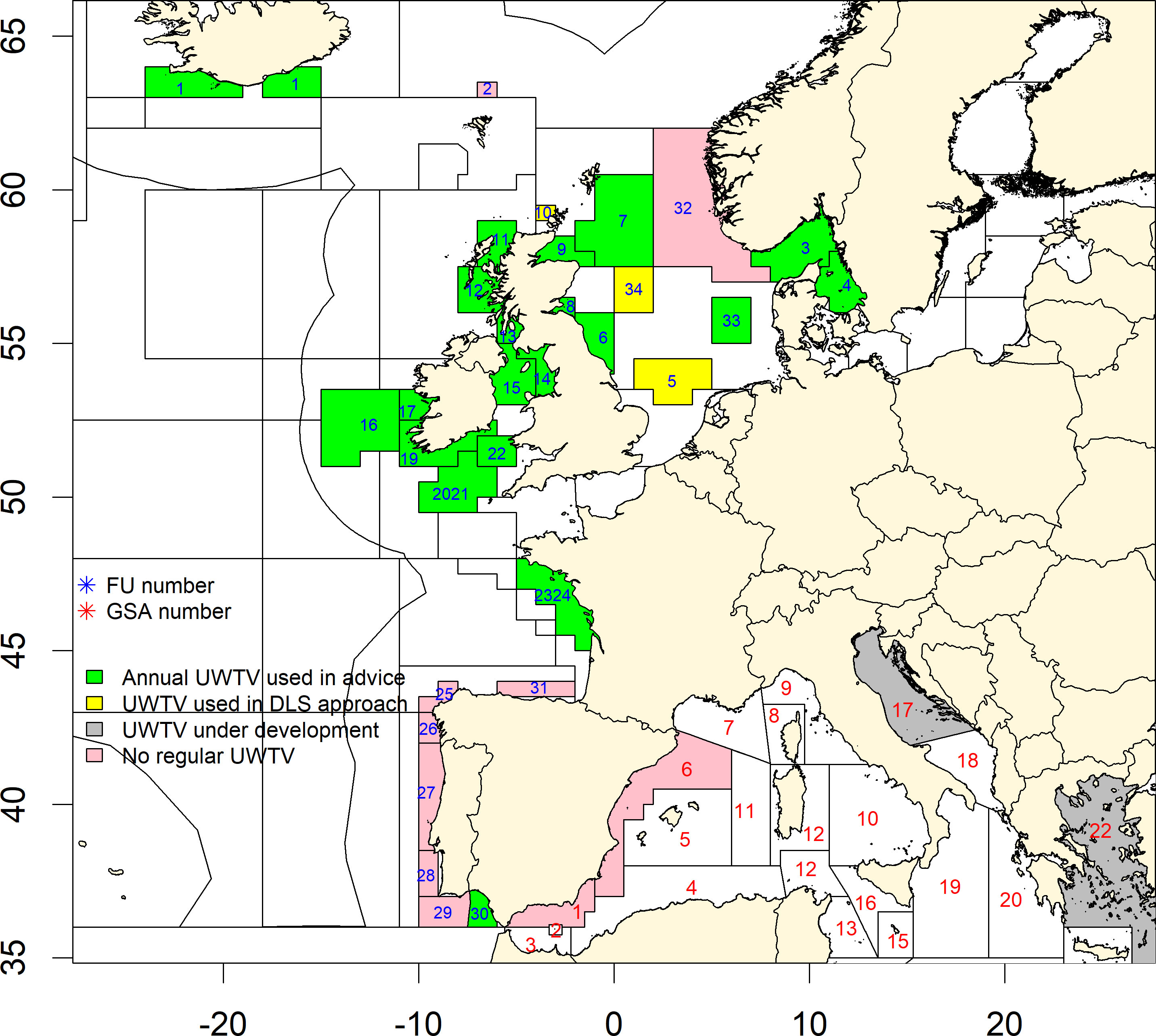
Figure 1 Fishery Units (FUs, from ICES) in the Atlantic and Geographical Subareas in the Mediterranean (GSAs, from FAO) as of 2022. FUs and GSAs are used for the fishery assessment of Norway lobster performed by UWTV surveys or by trawling, including areas (for the Mediterranean) where no surveys occur yet.
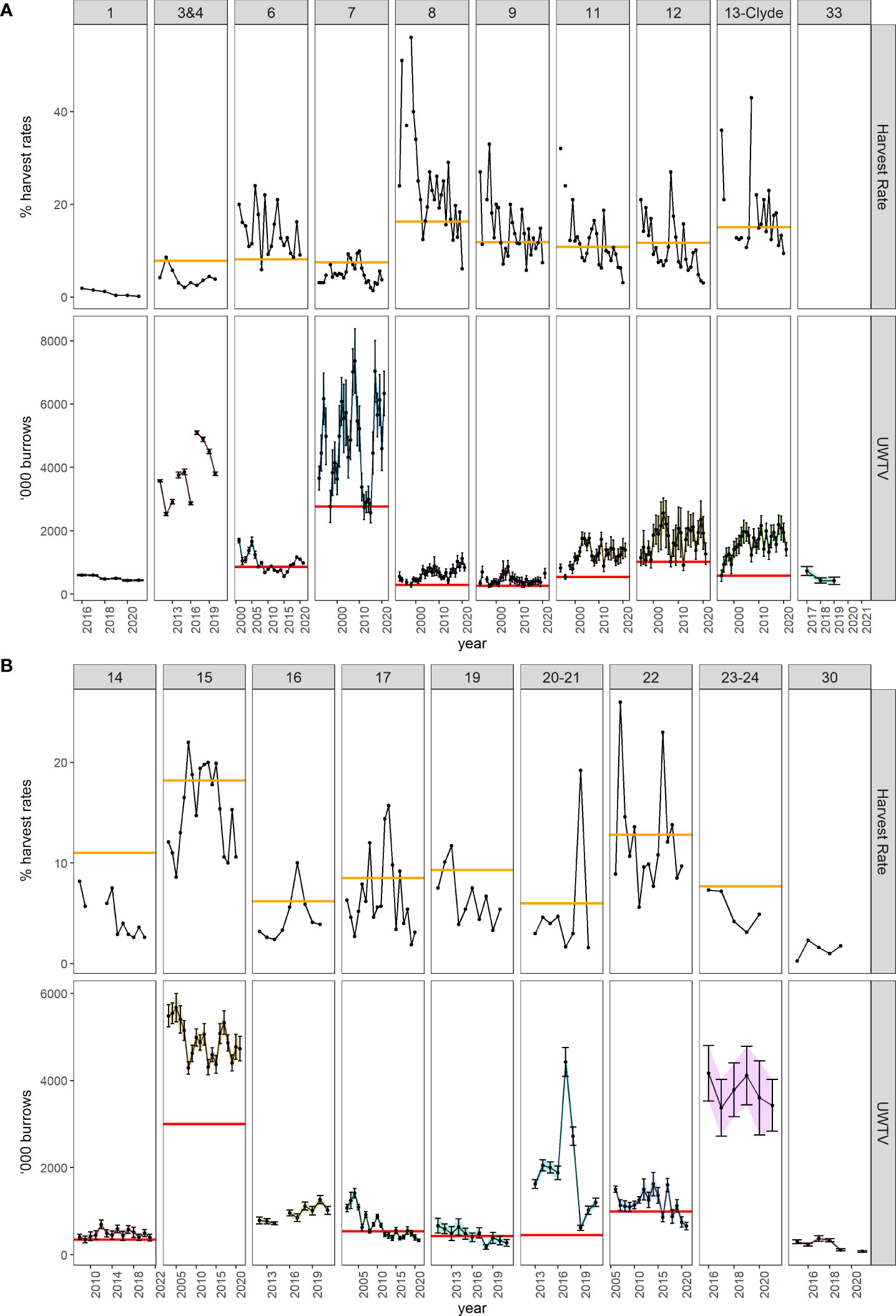
Figure 2 Harvest rate and UWTV time series for N. norvegicus stocks by FU in the “northern” (A) and “southern” (B) grounds. Top panels: % harvest rates (solid line), the FMSY harvest rate reference point is shown as the orange horizontal line. Bottom panels: UWTV burrows’ abundance estimate (number of burrow systems, solid line, with ±2 standard deviation bars for FUs using stratified models, and ±95% confidence intervals for FUs using kriging models; Dobby et al., 2021) presented as ‘000 burrows, the Btrigger reference point is shown as the horizontal red line. Some stocks have no defined reference points. Source, ICES Stock Information Database, Copenhagen, Denmark2.
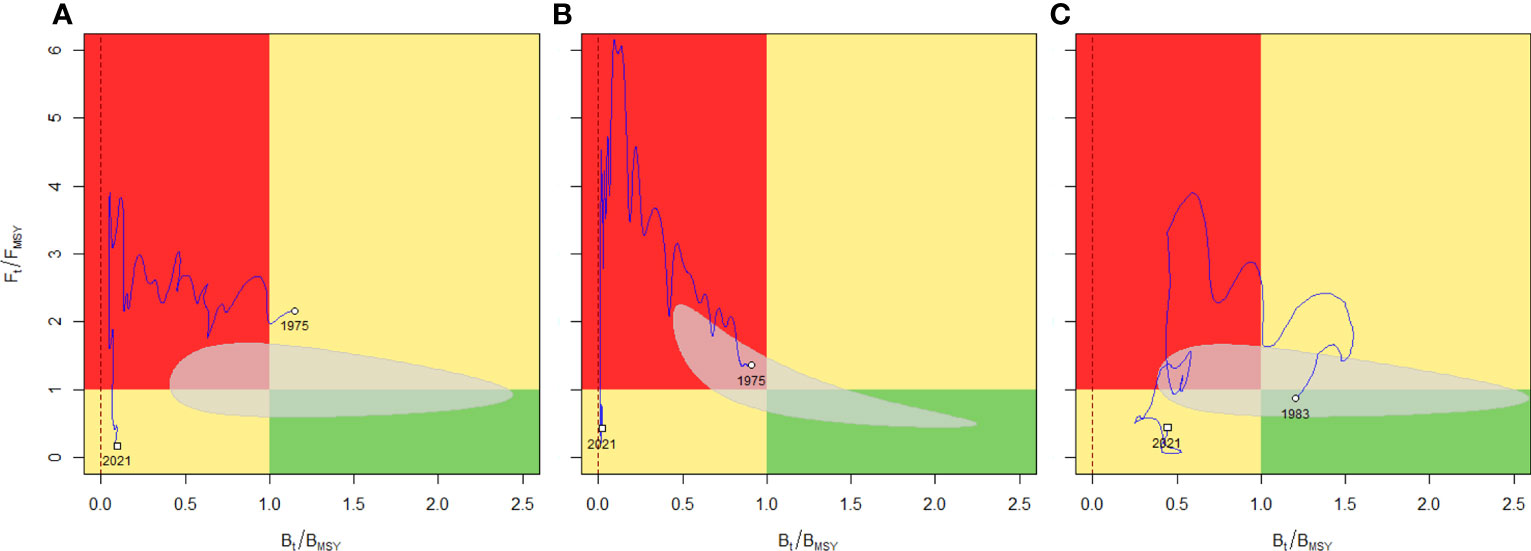
Figure 3 Kobe plot representing the fishing mortality as a function of biomass (relative to MSY) for the N. norvegicus fishery in FU 25 (A), FU 26-27 (B) and FU 31 (C), according to stock assessment carried out by ICES expert groups using trawl survey data. Red panel corresponds to the “overfishing phase”, with biomass below BMSY and fishing mortality F > FMSY. The green panel is the “no risk” area where F < FMSY and B > BMSY. The two yellow panels characterize intermediate situations (upper right “overfishing but not overfished”; lower left “overfished but not overfishing”). A vertical dashed red line at B/BMSY = 0 indicates the biomass level below which the stock has crashed. The grey-shaded region represents the uncertainty of estimated reference points pairs (95% CI) in relative terms (Pedersen and Berg, 2017). Source, ICES Stock Information Database, Copenhagen, Denmark.
UWTV estimates are used by ICES to propose fishing quotas for each Nephrops stock based on the theory of Maximum Sustainable Yield (MSY) (ICES, 2021a). These stock assessments are classified into categories, where “Category 1” is a data rich stock and “Category 3” is a data limited stock (ICES, 2021b). Recent assessments indicate that the harvest rate of stocks in most FUs is below the maximum sustainable yield level of fishing (i.e., is less than the fishing pressure reference point, FMSY). Indeed, many of the “northern” FUs stocks (see Figure 2A) are above their biomass trigger (Btrigger) reference points (thus they are considered sustainably harvested) compared to “southern” FUs stocks (see Figure 2B). Nevertheless, as several stocks (i.e., data limited stocks, “Category 3”) do not have yet defined reference points due to a lack of fishery data or short survey time series, UWTV based approaches have also been used for precautionary catch advice. Overall, while most stocks appear sustainably exploited, the assumptions and caveats within the trawl and UWTV surveys (ICES, 2009) mean there is need to improve current stock assessment methods. The precision of UWTV surveys is high; however, there are underlying concerns such as burrow occupancy. This unknown uncertainty could be investigated through studies using novel technologies to provide insights as to whether this should be monitored routinely (ICES, 2007).
Stocks of N. norvegicus in the Mediterranean Sea (FAO zone 37) are divided spatially into Geographical Subareas (GSAs; see Figure 1), and stock assessments are conducted by the General Fisheries Commission for the Mediterranean (GFCM) Scientific Advisory Committee on Fisheries (SAC) (Cardinale et al., 2021). The evaluation is typically carried out through stock assessment models (Carpi et al., 2017; GFCM, 2021) fed with biological parameters, including: indices of abundance derived from scientific trawl surveys not specifically targeting Norway lobster (e.g., MEDiterranean International Trawl Survey; Bertrand et al., 2002; Sbrana et al., 2019), size composition of commercial catches and official landings from DCF (EU, 2008). Recent assessments revealed stocks under different conditions (e.g., GSA 6 Northern Spain is overfished, GSA 5 Balearic Islands and 9 Ligurian Sea and Northern Tyrrhenian Sea are being sustainably exploited; GFCM, 2021; GFCM, 2022). Furthermore, in some areas of the Mediterranean some exploratory UWTV or Remotely Operated Vehicle (ROV) investigations have been implemented thanks to the support of other sources (ICES, 2018; ICES 2022). Noteworthy is the case of the GSA 17 (Northern and Central Adriatic) stock, exploited by the Italian and Croatian fleets, whose assessment is considered a priority, but difficult to achieve and under ongoing methodological discussion (Carpi et al., 2017; GFCM, 2021). Investigations on various modeling approaches have been recently carried out in GSA17 (Martinelli pers. comm.; GFCM, 2022) taking into account, in addition to traditional data: the available UWTV data (Martinelli et al., 2013; Martinelli et al., 2022), new assumptions on the availability of the species to trawl fishery (Aguzzi et al., 2021), the presence in the area of various subpopulations with limited connectivity (Melaku Canu et al., 2021) and different biological characteristics (Angelini et al., 2020), the spatial origin of the commercial catches (Russo et al., 2018) and standardized trawl data from a survey specifically targeting N. norvegicus and not covered by DCF (Chiarini et al., 2022).
Besides the fishery advice on the status of N. norvegicus stocks, published on a yearly basis by ICES and The General Fisheries Commission for the Mediterranean (CGPM), the EU also participates and provides scientific advice and management recommendations through other Regional Fisheries Management Organizations (RFMOs) and Regional Fisheries Bodies (RFBs), such as the Scientific, Technical and Economic Committee for Fisheries (STECF), the International Commission for the Conservation of Atlantic Tunas (ICCAT) or the Northwest Atlantic Fisheries Organization (NAFO). N. norvegicus stocks are characterized globally as overexploited (European Commission, Joint Research Centre, Scientific, Technical and Economic Committee for Fisheries, 2022)
Burrowing behavior of N. norvegicus and its impacts on trawl-based stock assessments
N. norvegicus require silt and clay habitat with a low current profile to construct stable burrow systems (Bailey et al., 1995; Tully and Hillis, 1995; Maynou and Sardà, 1997). A burrow system has a distinct U-shape with at least two entrances and a connecting tunnel approximately 20-30 cm below the seabed (Farmer, 1974). Several smaller shafts branch off from the main burrow system and extend to the surface to improve ventilation (Rice and Chapman, 1971; Tuck et al., 1994; Tuck et al., 1997; Froglia et al., 1997; Hugens and Atkinson, 1997). Over time, additional entrances and connective tunnels may be created. The location of these entrances initially follows a predictable pattern but becomes more random with additional entrances, although the tunnels always converge at a central point. The development of the burrow system is influenced by both biotic (e.g., the capability of the animal to both maintain and defend a complex) and abiotic factors (e.g., sediment type, burrow density/available space, hydrographic and benthic morphology, benthic disturbance by trawling) (ICES, 2017).
N. norvegicus actively maintain their burrows, which provide protection from predators and developmental habitats for juveniles. Adult N. norvegicus are highly territorial of their burrows and have never been reported to co-occupy a single shelter. Nevertheless, cohabitation between adults and juveniles has been reported (Bell et al., 2006; Aguzzi and Sardà, 2008; Sardà and Aguzzi, 2012). Juveniles co-occupying tunnels with adults will eventually excavate their own tunnels that branch off from the initial adult tunnel. Eventually, the juvenile will collapse the connection between the original burrow system and the new section to create their own isolated burrow (Tuck et al., 1994). Juveniles may even have some capability to dig their own burrow (Powell and Eriksson, 2013).
The burrowing behavior of N. norvegicus has been thoroughly researched over several decades (e.g., Chapman et al., 1975; Moller and Naylor, 1980; Bell et al., 2006; Sardà and Aguzzi, 2012; Katho et al., 2013), yet many aspects related to this life-trait remain poorly understood. For example, little is known about some dynamics of early life history stages, such as whether N. norvegicus larvae settle out of the water column onto sediment randomly (e.g., passive drift) or due to active searching for suitable substrates and how they transition to juveniles and young adults, stepping from burrow co-occupation to digging their own.
Trawling for stock assessment
Traditional stock assessments of N. norvegicus are based on fishery-dependent data mainly obtained via trawling (e.g., Bertrand et al., 2002), but individuals are protected from capture while they remain in their burrows (Chapman and Howard, 1979; Main and Sangster, 1985; Newland et al., 1992). In addition to the bimodal description of N. norvegicus behavior, being inside or outside their burrows, Aguzzi et al. (2007) added a “door-keeping” activity. Door-keeping is when an animal waits at a burrow entrance. Animals exhibiting this behavior are likely more susceptible to capture via trawl than those deeply positioned into the burrow; however, animals engaging in door-keeping can suddenly retreat into the burrow as a trawl approaches. Thus, the probability to capture door-keeping individuals likely remains low unless using electrical nets (Chapman and Howard, 1979). Indeed, the use of electrified nets increases capture by 35% (Stewart, 1975).
Burrow emergence is strongly influenced by ambient light, and massive emergence frequently occurs at night in shallow shelf areas. Furthermore, as light penetration decreases with depth, individuals emerge from their burrow earlier in the evening and return later in the morning (reviewed by Aguzzi and Sardà, 2008). Accordingly, emergence is fully nocturnal and elicited by illumination achieved at clear full moon phases in the upper shelf (5-50 m; Naylor, 1988), being crepuscular on the lower shelf (50-200 m; e.g., Farmer, 1974; Chapman et al., 1975; Moller and Naylor, 1980), and becoming fully diurnal on the slope (200-450; Aguzzi et al., 2003). Recently, this diurnal burrow emergence pattern has been confirmed for the slope (350 m) at the level of the individuals, by acoustic tracking (Vigo et al., 2021).
Comparing data from trawls to visual surveys conducted by scuba divers suggests that only 10-30% of animals emerge daily on the shallow shelf (Chapman, 1980). This observation is likely valid throughout the bathymetric range of the species. As only emerged animals may be captured by trawling, burrowing behavior should serve as inherent protection from over-exploitation by fisheries. It also means that swept area based assessments using trawling data will underestimate the true population abundance. Where relative abundance from trawl surveys is used to calibrate assessments, the index may be inherently variable or biased due to emergence patterns.
In laboratory settings, the monitoring of locomotor rhythms has been carried out under controlled light-dark cycles (Chiesa et al., 2010), tidal flow (Sbragaglia et al., 2015) and various social settings (Sbragaglia et al., 2018). These experiments demonstrated a control of the burrow emergence in response to light, water flows, and territorial behavior (fighting for burrow eviction). Furthermore, the daily burrow emergence patterns of N. norvegicus differ over a range of spatio-temporal scales in relation to environmental factors, including:
i. Emergence inhibitors (e.g., some animals may not emerge due to predator presence) with berried (i.e., ovigerous) females remaining concealed for longer periods than males (i.e., given their lower catchability rates; possibly showing a preference to the different pH conditions in the burrow in relation to the overlying water; Zhu et al., 2006).
ii. Emergence social-related enhancers (e.g., social interactions related to territorial control and to fight against intruders or for mating; Sbragaglia et al., 2013; Sbragaglia et al., 2018).
iii. Photic-tidal combined effects (e.g., some animals may emerge for the presence of food odor plumes at temporal windows with inhibitory environmental light intensity thresholds, since feeding is reported as an out of burrow activity; Oakley, 1979; Parslow-Williams et al., 2002).
These factors influencing burrow emergence will inherently also affect how many N. norvegicus are caught via trawls or observed on UWTV surveys. In turn, this will impact the accuracy of these methods for conducting accurate estimates of population abundance. There are also issues with the use of trawling for stock-assessments as it can be too habitat-invasive for Marine Protected Areas or areas hosting vulnerable marine ecosystems (e.g., Funiculina quadrangularis and Isidella elongata assemblages; Grinyó et al., 2020) and will incur unnecessary mortality.
UWTV for fishery-independent stock assessments
Over the last 25 years, UWTV surveys have become the primary assessment method used by ICES for estimating N. norvegicus stocks (Leocádio et al., 2018). The video-inspection method focuses on burrows as they are a static and relatively constant element through constant animal maintenance (Sardà and Aguzzi, 2012). This method assumes that “1 burrow system = 1 animal” due to the reported territoriality of adults. Alongside fishery dependent data, the status of the stocks is evaluated by annual UWTV surveys, to assess increases in the numbers of burrow systems as a positive indicator of population growth, whereas a decreasing number of systems would indicate population decline.
Although the UWTV methodology has gained widespread acceptance, it has been criticized in the literature and within some parts of the fishing industry (e.g., Sardà and Aguzzi, 2012). Despite advancements in technology, including the use of High Definition cameras in some surveys (Friard and Gamba, 2016; Aristegui, 2020), the UWTV approach is still extremely time-consuming. Recounts increase labour hours and approximately 200 hours of video from 1450 UWTV stations are recorded each year across all FUs. Also, there is evidence that counts can drift and vary significantly between years and regions. The issue of reproducibility and consistency in counts is thus a key concern. The key uncertainties related to counting burrow systems visually are:
i. Burrow systems morphological identification and edge computing effects on counting.
ii. Persistence of empty burrows (because the animal has been predated or fished, or occupied by other opportunistic species), according to contextually different hydrographic conditions (i.e., structures persistence may vary according to local currents).
iii. Exclusion from tunnel occupation by other fish and crustacean species that maintain tunnel integrity.
iv. Multiple-occupancy (adults and juveniles).
v. Eviction and conquest of neighboring tunnels by dominant individuals, which consequently occupy more than one burrow system.
As one burrow system does not necessarily equate to one animal, it is clear that some form of correction factor is needed. In an optimal scenario, such a correction factor should be based on direct calculations from data collected on how the number of burrow systems compares to the number of N. norvegicus in a given area. Yet, as burrowed individuals cannot be seen from the surface, innovative non-visual methods should be used as an alternative. Furthermore, as burrowing behavior in N. norvegicus is influenced by light-levels and other environmental factors, such correction factors must be informed by in-situ environmental data. Comparable correction factors have already been developed for improving population assessment of other marine species that are not always visible during conventional surveys. For example, aerial surveys for large, air-breathing vertebrates such as manatees and sea turtles are often adjusted based on knowledge of these animals’ diving behavior to account for those individuals that are below the surface and thus not visible during the survey (Langtimm et al., 2011; Borchers et al., 2013; Fuentes et al., 2015).
Developing novel sensors and data collection strategies for stationary and mobile robotic platforms
Optimizing ecological monitoring efforts requires knowledge of the sampling scale (e.g., populations, geographic regions, or individuals). As such, efforts should aim to maximize benefits and minimize costs associated with sampling redundancy. This will ultimately define which platforms are most suitable to collect the necessary data at the appropriate scale (Danovaro et al., 2020). All sensors and platform technologies that we will discuss are being developed or are already well commercialized and can be readily deployed for ecological studies over varying levels of ecological complexity (Supplementary Table S1).
Image-based technologies
Typically, UWTV surveys count burrow systems, not animals. In contrast, trawls collect animals outside their burrow but do not convey any information about the number of burrow systems or the animals inside them. A simple step to improve the data collected by UWTV surveys would therefore be to not only count burrow systems, but to also count how many animals are outside their burrows, as well as how many animals are engaged in door-keeping. Their cumulative number could be compared with the total counted burrow systems (Aguzzi et al., 2021). In particular, animals reported numbers during phases of massive population emergence (see previous section on activity rhythms) could be related to counted tunnel systems, deriving a minimum correction factor for the UWTV assumption “1 burrow system = 1 animal” (Aguzzi et al., 2021). The repetition of that monitoring procedure, over the 24-h cycle, would allow to count animals emerging with a timing other than that of the massive population peak. Those asynchronous demographic components could be added to population numbers to improve population estimates above those generated from exclusively counting burrow systems.
Stationary cameras can improve estimates on the proportion of counted burrow systems in relation to resident animals, providing additional information to UWTV surveys. A similar application context can be found in (Marini et al., 2022) where the dynamics of the coastal Antarctic benthic fauna have been estimated for a whole year through a specifically designed autonomous and stand-alone camera (Corgnati et al., 2016; Marini et al., 2018a). The consistent monitoring of specific burrow systems (replicated over the seabed space by a network of cameras) can be used to fine-tune the demographic estimation of targeted populations with data on the temporal use of burrows by individuals (see Supplementary Table S1). Similar information could also be achieved by mobile cameras by using robotic platforms that are capable of conducting repeat surveys of consistent geographic areas over consecutive 24-h cycles.
Improvements in technology can reduce the potential impact of the UWTV surveys. For example, there is evidence that the white light illuminators for the UWTV can cause retinal damage to surveyed animals (Shelton et al., 1985). Thus, the current white light illuminators can be replaced by low-light (Barbier et al., 2012) and multibeam optoacoustic imaging assets (Adaptive Resolution Imaging Sonar; ARIS; see below) or 3D laser-scanning technologies (Aguzzi et al., 2019), to reduce the potential for disturbing the behavior of N. norvegicus or other low-light habituated species (Davies and Smyth, 2017; Robinson et al., 2021). Infrared imaging could also be used but only if cameras are located close to the seabed surface (i.e., for the attenuation of this wavelength into the water; e.g., Widder et al., 2005).
Conventional cameras can be modified to include multibeam acoustic, stereo, and 3D laser-scanning technologies that may also provide size estimates for any animal within the field of view. Similarly, geo-sonars producing acoustic or microwave images of the first 20-30 cm of the seabed surface could disclose burrow systems’ architectural features, possibly also portraying sheltering adults and the often non-visible animals such as juveniles.
Non image-based technologies
While considerable research has previously focused on the factors influencing the burrowing behavior and activity-levels of N. norvegicus in laboratory settings (see previous section), technological developments enable future studies to be conducted in-situ in the field.
In the past decade, image-based methods for studying the behavior of individual decapods have increasingly been complemented with telemetry (Florko et al., 2021). Specifically for N. norvegicus, acoustic telemetry has been used to study burrow emergence rhythms over several months (Vigo et al., 2021). To achieve this, animals were captured and equipped with acoustic tags before being released by canister within a hydrophone array (Masmitja et al., 2020; Vigo et al., 2021). As long as animals remained within range of the hydrophones, the hydrophones would receive the signals from the acoustic tags when the individual had emerged from the burrow. In this manner, daily patterns in signal reception could be used to infer emergence patterns (see Supplementary Table S1).
Hydrophones can track a tagged animal’s spatial movements (Vigo et al., 2021). From these positions, taken at consecutive times, a total traveled distance (or covered seabed surface) can be computed. The comparative analysis of animals’ presence into the different monitoring area quadrants (e.g., by heat-map analysis approaches) allows the estimation of individuals’ home range. That can be further analyzed in terms of site fidelity, trajectories, relations with co-specifics evaluating interactions (also present in the same quadrants at the same time), and territoriality (home ranges overlap with co-specifics).
Another promising non-imaging technique for the study of N. norvegicus stocks is using environmental DNA (eDNA). All animals continually leave behind traces of organic material with trace amounts of DNA that can be amplified and identified from seawater or sediment samples (Huver et al., 2015). When identified, this can provide evidence that the species of interest has previously been in the vicinity of the sampled area (Beng and Corlett, 2020). This could be particularly useful in the case of N. norvegicus, as eDNA samples can be collected in proximity to the burrow system. Such samples could be used to assess whether the burrow system is currently (but also in the recent past) occupied by a single or even multiple individuals. Nevertheless, such techniques would require validation via in-situ and laboratory-condition controlled studies, as many questions still remain. For example, it is not yet known how long eDNA persists in marine sediment and what the dispersal capability of eDNA is in benthic environments occupied by N. norvegicus, among other uncertainties (Zaiko et al., 2018). If eDNA persists for substantial periods of time (> months), then this method could be complemented by the co-analysis of eRNA that has a faster degradation rate and hence is expected to be representative of recent occurrence (Marshall et al., 2021). Additionally, the use of eDNA/eRNA analysis could not only reveal whether a burrow system is currently occupied or not, but also if it is occupied by other non-N. norvegicus opportunistic burrowing species (Sardà and Aguzzi, 2012). Additional information on burrow-fidelity generated by eDNA/eRNA analysis would however be extremely beneficial to further refine UWTV-based stock assessment.
Using robotic platforms to complement UWTV and trawl-based stock assessments
Stationary cameras could observe specific burrow systems over time and hence track the behavioral performance of a group of individuals. In contrast, mobile cameras can be used to focus on both individuals and the surrounding ecosystem over larger geographic areas but over shorter periods of time. Using these methods simultaneously would allow for scaling the knowledge on the temporal patterns in individual locomotion and burrow emergence to the population level. In this scenario, stand-alone imaging systems mounted on landers, cabled observatories and their docked mobile platforms such as Autonomous Underwater Vehicles (AUV), tethered crawlers or biomimicking robots; e.g., legged-robots, such as Silver 2 (Picardi et al., 2020; Figure 4), could be used for local observation for burrow systems and individual counting.
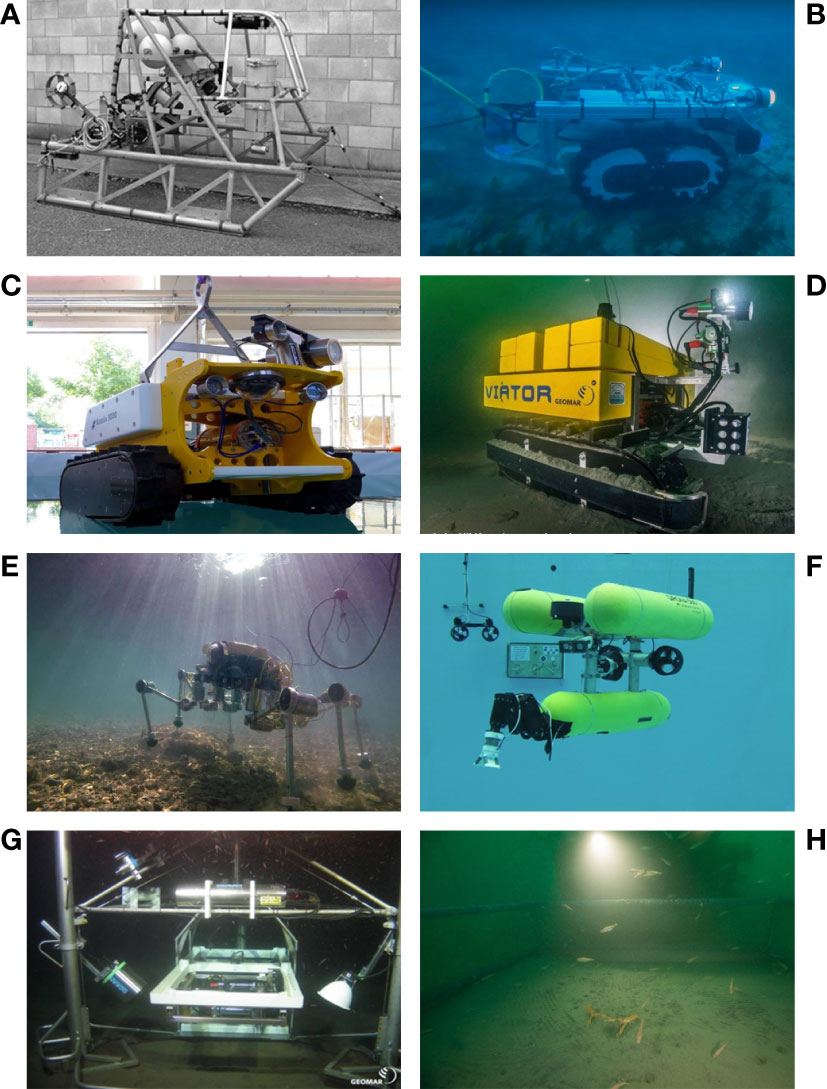
Figure 4 Different types of image-recording mobile vessel assisted platforms (A) towed sledges; adapted from Campbell et al., 2009) and autonomous mechanic and biomimetic robots (B) Teleoperated Ecological Monitoring Crawler at the cabled seafloor observatory, OBSEA3; (C) deep-sea commercial crawler Rossia4; (D) untethered rover Mansio-VIATOR5; and (E) Silver 2 arthropod robot6), along with AUVs (F) Girona 10007), biogeochemical landers (G)8 and cabled observatories (SmartBay, with the SmartLobster experiment; (H)9 One should notice the possibility to tune the monitoring capability with variable sensor payload for a comprehensive image and synchronous oceanographic-geochemical data acquisition.
Another tool for the study of N. norvegicus are cabled observatories (see Supplementary Table S1). Observatories receive constant power to feed a complex and adaptable payload of sensors and relay data in real-time, allowing prolonged insight into the animals and their habitat at a specific location (Aguzzi et al., 2018). When cabled observatories are coupled with mobile and dockable platforms such as crawlers and AUVs, then their monitoring area could be greatly expanded (i.e., from few to hundreds of meter squares). When considering a lander or a cabled observatory as the epicenter for stock monitoring, scenarios for data collection with advanced mobile and fixed platforms could be for example as follows (Figure 5).
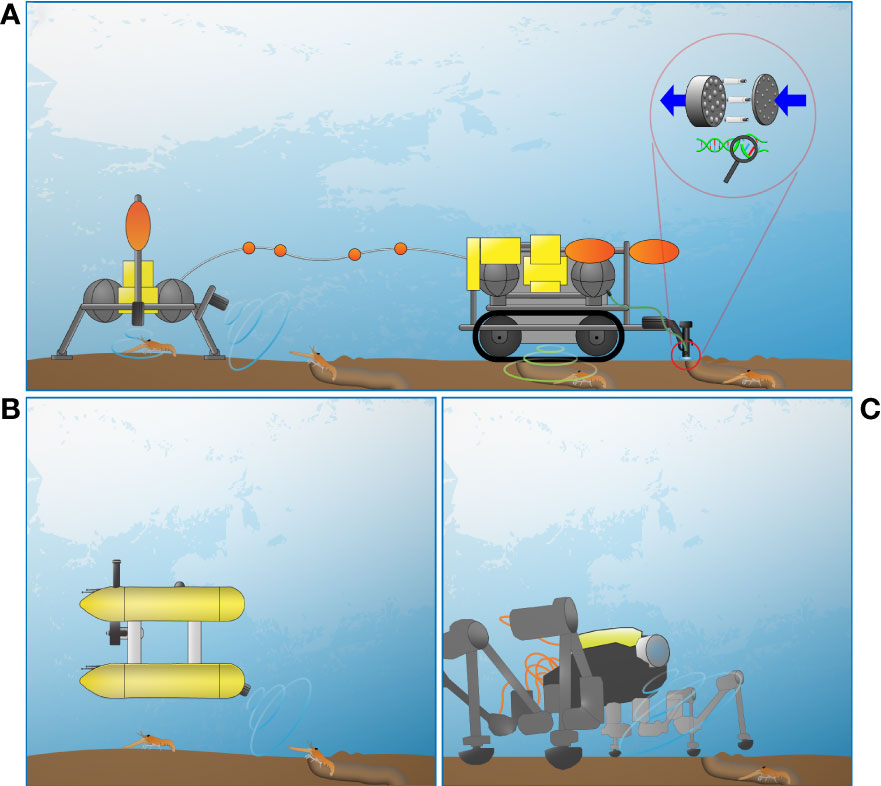
Figure 5 Different monitoring scenarios where a lander or a cabled observatory is the epicenter for stock monitoring by other mobile platforms (see Supplementary Table S1): (A) a satellite stand-alone lander (or cabled observatory) with a tethered crawler with an eDNA collector for genetic screening (in-situ or in the laboratory) which could be installed on benthic robots The lander would monitor the few m2 at high frequency for long-term deployments (e.g., year-long), with construction and operations costing approximately 54,000 €. A crawler’s cost can range between 20,000 € (small-sized, approximately 0.25 m3) and 150,000 € (medium-sized, approximately 1 m3), with the vehicle able to cover surfaces of several hundreds of m2 in long-term deployments (multiannual); (B) docked AUV conducting pre-programmed surveys during 2-3 months, covering 1 to 10 km2 around the fixed stations (see plate A). The cost of production and operations is estimated to be approximately 500,000 €; (C) a tethered biomimicking legged-robot (“crabster”) could cover hundreds of m2 (less than the crawler, but able to approach and enter hard to access or sensible terrains) in long-term deployments (multiannual), at an approximate cost of 450,000 €.
Easy to move satellite landers that can dock with mobile small-range benthic platforms (crawlers, rovers and biomimicking legged-robots) can be used for spatially referenced monitoring by repeating transects over specific pathways with stepping-stone stations at identified burrow systems (Figure 5A). Their seabed imaging could be chiefly performed by multibeam and 3D laser-scanning plus geo-sonars. Monitoring operations in such a setting (e.g., SmartBay observatory) should follow a strict protocol based on previous knowledge on the use of crawlers in Ocean Networks Canada NEPTUNE observatory (e.g., Chatzievangelou et al., 2020; Chatzievangelou et al., 2022 in review) to reduce sediment suspension that can impact burrow systems. Specifically, the crawlers patrol the perimeter without entering areas of high burrow systems’ density and remain stationary near spots of interest (e.g., a specific burrow of an active individual) for longer-term monitoring.
Benthic robots could be equipped with robotic arms to sample sediment for eDNA at burrow entrances or to introduce micro-cameras within the sedimentary structure of the burrow systems (see Figure 5A). Wide-range pelagic platforms (AUVs) with multibeam and 3D laser-scanning image acquisition capabilities (Figure 5B) would further expand the monitoring radius capability by adding visual pre-programmed surveys along consistent pathways. Simultaneously, legged-robots could cross the field, overcoming potential obstacles (i.e., operating monitoring in those areas where trawling of UWTV is not carried out for the presence of rock outcrops or biogenic reefs; Aguzzi et al., 2022) (Figure 5C).
AUVs are often mobile and suitable for long range surveys. In fact, monitoring systems consisting of Unmanned Surface Vehicles (USV) combined with deep AUV, equipped with cameras and Multi-Beam Echo-Sounders (MBES), like those proposed in the ENDURUNS project (Marini et al., 2020), can be used for autonomous multi-resolution deep surveys extended in time. The MBES can be operated at different altitudes acquiring coarse-to-fine and geo-referenced data of the seabed, with resolutions from decimeters to centimeters, depending on the sensor resolution and distance from the seabed. Additionally, AUVs can be equipped with Forward Looking Sonar (FLS) systems or acoustic cameras that complement classical optical vision systems, without being affected by poor water visibility and without affecting species by not requiring optical illumination (Figure 6). Within the ENDURUNS system, a hybrid AUV (capable to navigate either with propellers or as a sea glider) periodically reaches the sea surface and transfers the acquired data to the USV through its WiFi communication system (Sanchez et al., 2021). Thereafter, the USV can autonomously process the data onboard for selecting relevant content-based information to transmit via satellite to a land-station for validation by experts. Then the operation room at the land station can send back new navigation instructions to the AUV for a higher resolution survey of the seabed if needed, or to navigate on sea glider mode to a new site. However, the AUV propellers can produce considerable noise and can suspend large amounts of sediment when operating near the seafloor. In contrast, benthic platforms can remain stationary passively and thus can be silent and cause minimal sediment disturbance when not in motion.
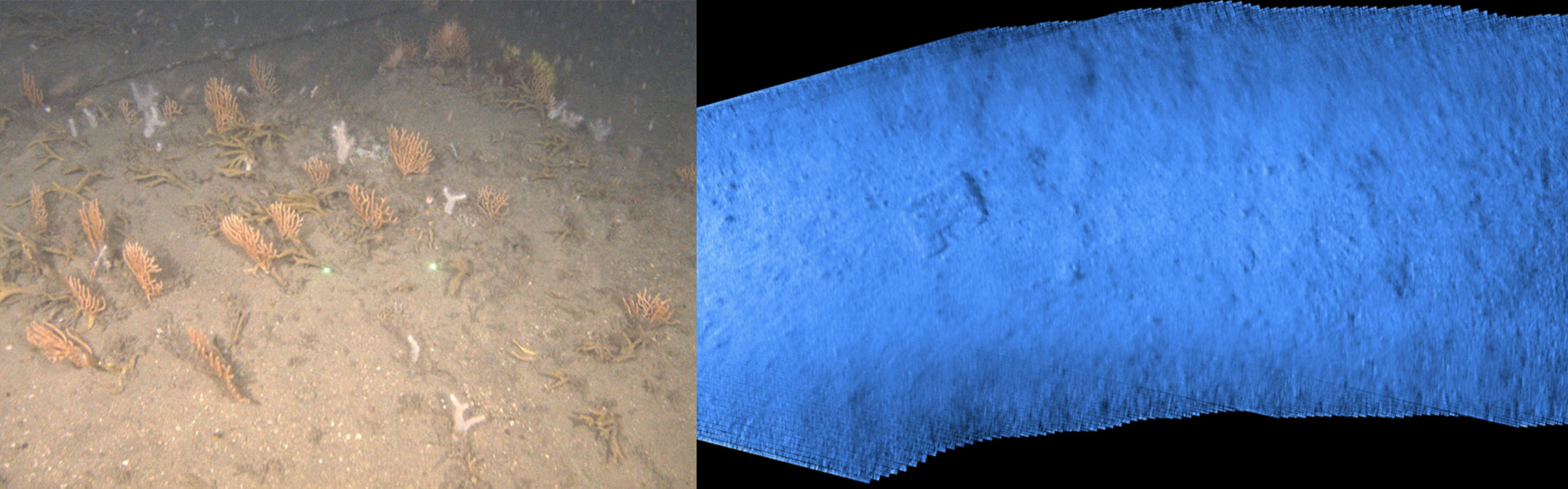
Figure 6 Optical and acoustic mapping using AUVs. The Girona 1000 AUV, equipped with an optical camera and an illumination system, can hover close to the seafloor and obtain high quality images of the habitat (left image, taken at 100m depth at Cap de Creus, Mediterranean Sea). Alternatively, the same AUV can be equipped with a FLS and cover the seabed without affecting the species with light emission. After processing the acoustic images, a detailed mosaic can be generated (right image, taken at 15m depth in a harbor in the Mediterranean coast).
Among benthic robots, crawlers can carry heavy payloads, acting as mobile stations within similar monitoring capacities as cabled-observatories. Nevertheless, they can resuspend considerable amounts of sediment when moving. At best this can obscure viewing of the burrows, while in a worst case scenario it could lead to the burrows’ destruction. One way of addressing this solution is to use platforms such as biomimicking (arthropod-like) legged-robots. Those platforms can have minimal impact on the seabed (no footprint left and minimal sediment resuspension) and individual feet can be specifically positioned to avoid accidental damage to burrow systems and tunnels. Legged-robots can also traverse greater variation of terrains than wheeled or tracked counterparts.
The technologically advanced monitoring should be complemented by that of vessels (UWTV surveys) and their deployed technologies such as drifting cameras (Dominguez-Carrió et al., 2021), ROVs or hybrid ROVs and AUVs platforms (HROVs; e.g., Ariane from Ifremer10). Additionally, a large volumetric sediment grab could be obtained from a chosen area that has been intensively monitored by cameras for several months. The sediment removal would allow counting all individuals of N. norvegicus and associated fauna within its volume, to relate the observations on the occupation and burrow emergence of animals in different burrow systems with their density. This sampling procedure would allow quantifying the putative presence of a typically hidden demographic component (i.e., animals with reduced or erratic emergence temporal patterns).
Counting burrow systems and animals using machine learning
Machine Learning (ML) tools could overcome the bottleneck of manually-extracting data on species identification and quantification from terabytes of images11 (MacLeod et al., 2010; Moniruzzaman et al., 2017; Malde et al., 2019). Developing such tools is of strategic importance as they would allow for real-time processing of footage from UWTV surveys as well as on cabled observatories and other robotic platforms (Aguzzi et al., 2011). In turn, this would allow for the in-situ quantification of other ecological indicators (see next section) such as population density, demographics, biomass and cohabiting species (Aguzzi et al., 2020b). Indeed, the ICES WGNEPS recognized that the development of an internationally coordinated ML tool for identifying and counting burrow systems would help improve standardization among assessments carried out in different regions (Aguzzi et al., 2022).
The automated detection of N. norvegicus burrows and individuals is a relatively challenging problem for ML technology. There is notable variation in burrow shape and the clarity of the video image may differ based on light-levels (which are influenced by depth and time of day) as well as turbidity (Marini et al., 2018b; Corrigan et al, 2019). Nevertheless, the evolution of Deep Learning (DL) has consistently increased the accuracy rates of marine object detection (Girshick et al., 2016), classification (Shima et al., 2017; Soltan et al., 2020) and segmentation (Haque and Neubert, 2020; Masubuchi et al., 2020).
The development of ML tools for burrow and animal classification from UWTV surveys will likely follow four key phases. These are: i. Defining appropriate image collection methods and quality standards; ii. Identify reference imagery based on expert judgment to train the ML model; iii. Outlining an ML classification process; and, iv. Integrating the automated burrow system identification tool into data processing channels within the various platforms (e.g., UWTV surveys, crawler surveys).
The earliest use of ML tools to identify and count burrows was conducted under laboratory conditions. Firstly, Sooknanan et al. (2014) used mosaics for detecting the animals by a K-Nearest Neighbor classifier. Next, Sokolova et al. (2021) presented an automated ML-based observation system called NepCon to analyze N. norvegicus detection and quantification. The data set was prepared by using four different background colors and cropped the images with N. norvegicus.
More recent tools have been using DL techniques for automatic detection and classification of N. norvegicus burrows (Figure 7) (Naseer et al., 2022a; Naseer et al., 2022b). UWTV footage was converted to frames using the OpenCV12. The collected dataset was annotated using the Microsoft VOTT image annotation tool (CSE, 2020) and validated manually. To detect burrows, a transfer learning approach (Abadi et al., 2016) was adopted, and fine-tuned the Faster R-CNN Inceptionv2 (Sandler et al., 2018) and MobileNetv2 (Szegedy et al., 2016) models in TensorFlow (Abadi et al., 2016). The models were trained using three different dataset combinations. Two models were trained separately using either the FU22 or FU30 datasets, while a third, hybrid model was trained using both datasets in conjunction. Multiple sets of experiments were performed to evaluate the mean Average Precision (mAP) of each model, resulting into an 80% average performance.
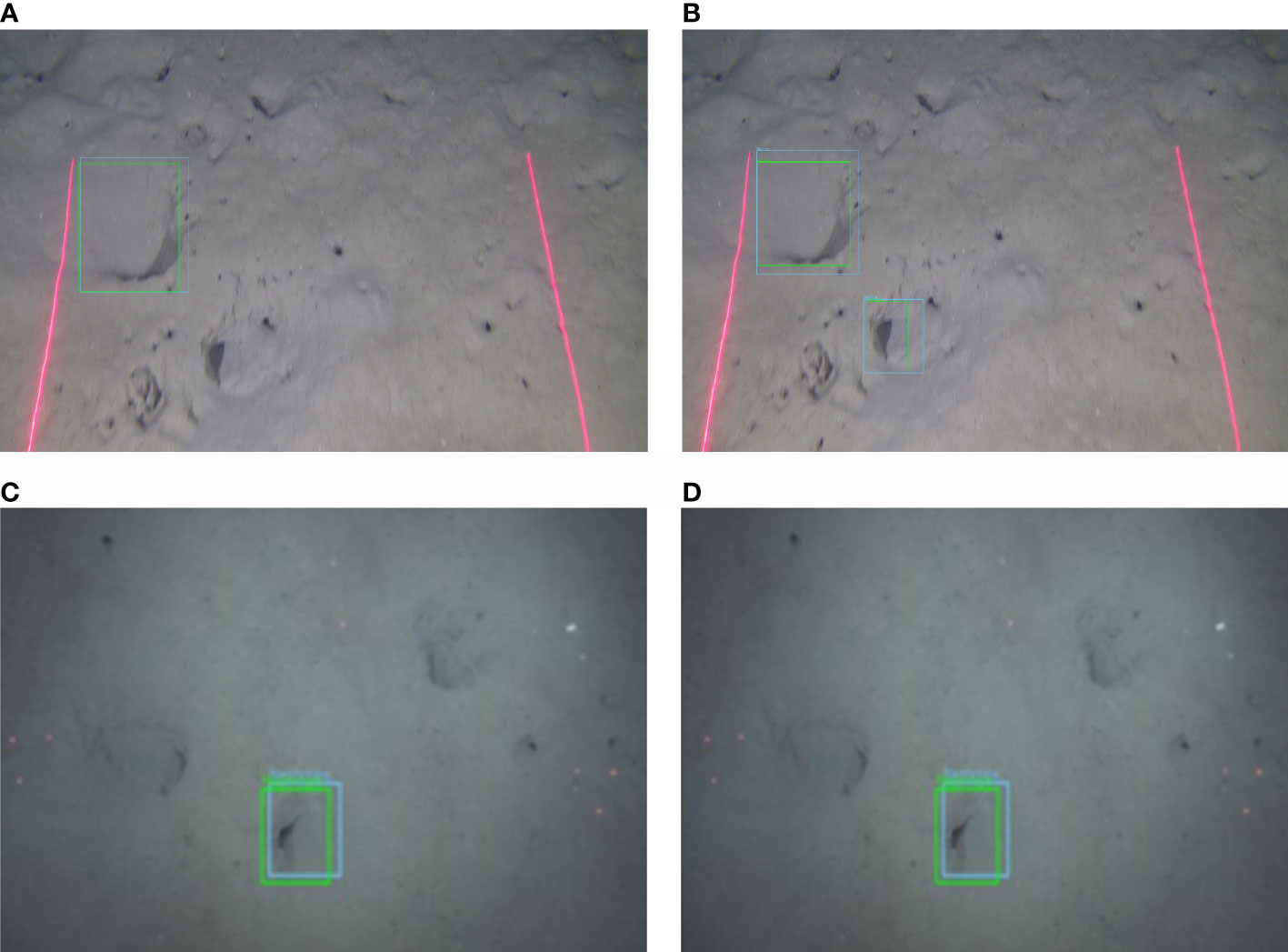
Figure 7 N. norvegicus burrows detections by FU30-MobileNet (plate A) vs. by FU30-Inception (plate B) and by FU22-MobileNet (plate C) vs. by FU22-Inception (plate D).
While current methods utilizing ML tools for stock assessment of N. norvegicus are exclusively based on identifying burrows, it should be noted that this method could also be used to identify and count all visible individuals. Furthermore, it may eventually be possible to not only identify where an individual is onscreen, but also its behavior (e.g., door-keeping) and size. Identifying individuals may even be possible at higher accuracies than burrow-identification due to the distinct color and morphological differences between N. norvegicus and the surrounding sediment. Simultaneous identification of burrows as well as individuals and their behavior could allow for the rapid assessment of the accuracy of the “1 burrow system = 1 individual” assumption and allow for correction factors (such as those described in the section “UWTV for fishery-independent stock assessments”), to be estimated in-situ.
Biotic interactions, such as predator-prey, play an essential role in population dynamics and species distribution (Ritchie et al., 2012). ML tools could also automatically identify and count the main predators of N. norvegicus, to evaluate population fluctuations due to predation. Some studies based on stomach content analyses, identified the Atlantic cod (Gadus morhua) as the main predator in the Atlantic waters (Chapman, 1980; Dombaxe, 2002). However, this predator is not present all over the species’ area of distribution, being absent in the Mediterranean Sea. A recent study from the NW Mediterranean, combining genetic and isotope stable analyses on stomach contents, has identified the most relevant predators (i.e., possibly feeding on N. norvegicus juveniles, the bobtail squid Sepietta sp. and the squid Abralia veranyi; other major predators identified were the small-spotted catshark Scyliorhinus canicula and the European hake Merluccius merluccius), providing also a Predation Index (Vigo et al., 2022). Their influence on N. norvegicus population was assigned according to their density (via trawling) in the targeted area.
Multiparametric environmental monitoring
Complementing data collection on the behavior and distribution of N. norvegicus with ancillary data on the local physical environment allows for further investigation into the environmental drivers of burrow emergence behavior (sensu Aguzzi et al., 2020a). In fact, more field research is needed on the identification of oceanographic and geochemical variables modulating burrow emergence at the level of individuals and populations (see Supplementary Table S1). Additionally, multiparametric monitoring may also be of strategic value for eDNA/eRNA sampling (see previous section). In fact, the persistence in water or sediment of this molecular tracer depends upon the contingent seascape status (Stefanni et al., 2022).
Sensors providing new information on animal behavioral response to different oceanographic and geo-chemical variables may be used to widen our knowledge on the species ecological niche (Supplementary Table S2). For example, ocean acidification, expressed as increased seawater CO2 and inorganic carbon levels, pH reductions, and alterations in acid-base chemistry of estuarine, coastal and open ocean water surfaces (Scott, 2020), may broadly alter ecosystems by acting at the level of organisms’ physiology, biomineralization, growth, reproduction, sensory perception, behavior, and survival (Cooley et al., 2009; Scott, 2020). This may even lead to ecological shifts in marine ecosystems (Cattano et al., 2018). To improve the field knowledge of biogeochemical processes, technical effort has recently increased in the development of pH sensors for high resolution monitoring of coastal ocean acidification (e.g., Saba et al., 2018). This latter improvement could also be used for the monitoring of the health status of N. norvegicus stocks, although crustaceans generally show a higher level of tolerance to ocean acidification (Kroeker et al., 2010).
Water temperature and salinity are key variables influencing the physiology and hence the survival of marine species (Smyth and Elliott, 2016; Bruno et al., 2018). In some geographical areas, but most notably on the west coast of Scotland and in the Clyde (FU 11 through 13), N. norvegicus are often exposed to the parasitic dinoflagellate Hematodinium. Studies showed temperature influences seasonal patterns of infection (Stentiford et al., 1999) with a significant impact on animal behavior (Stentiford et al., 2001). Infected animals appear paler and lack motor skills. In heavily infected individuals, a milky white haemolymph is visible on the ventral side of the abdomen. Infection also increases metabolic rate, which in return applies a greater nutritional load on the infected animal. This forces the animal out of the protection of the burrow system for longer periods of time as individuals require more food. This exposes the animal to predation or capture and reduces time available for burrow maintenance. Environmental temperature has an important influence on developmental rate and physiology of marine ectotherms such as Nephrops. McGeady et al. (2021) observed a notable shift in larval release in the Irish Sea which was attributed to warming temperatures. This highlights the need to collect long-term Essential Ocean Variables (EOVs) data when carrying out monitoring of marine ecosystems.
Current speed may affect the persistence of empty burrows, favoring their collapsing, varying at the same time the overall level of anoxia in relation to eutrophic contamination. This latter phenomenon may cause animals to have longer emergence phases as a reaction to stressing low oxygen levels (Baden et al., 1990). Broadly, current measurements and associated dissolved oxygen concentration measurements are required to evaluate the effects of extending Oxygen Minimum Zones (OMZs) on shelf and slope ecosystems (Breitburg et al., 2018). Also, current flow variations could influence the burrow emergence rhythms at tidal and inertial periodicity, hence acting as conflicting zeitgeber with light cycles of different intensity (according to depth; Aguzzi et al., 2009; Aguzzi and Company, 2010).
Variations of local phytoplankton biomass (measured as fluorescence; see Supplementary Table S2) can directly influence food input into benthic environments at variable time lags (Thomsen et al., 2017). Santana et al. (2020) highlighted the importance of suspended particulate organic matter in the diet of N. norvegicus finding that significantly more suspension feeding was observed in small or medium-sized individuals than large ones in both sexes. Water clarity and depth impact the level of light reaching the seabed, with river run-off, trawling activity (Martín et al., 2014) and storms resuspending sediment, and therefore reducing water clarity and available light.
Flowchart for data treatment, using environmental and biological data
We have illustrated various methods by which novel technologies can augment stock assessments for N. norvegicus. Incorporating these new data streams into current stock assessment will provide a complex challenge, but to facilitate this process, we have developed a flowchart for data treatment (Figure 8). Moreover, when combining unique datasets, several considerations must be taken into account. For example, environmental data may require pre-processing to match the frequency of the biological (i.e., optoacoustic) data acquisition. In addition, several processing steps for counting, measuring and sizing must be applied to the biological data to extract the relevant information on the structure of burrows, the abundances of burrow systems, door-keeping/fully emerged individuals, and the size-class frequency distribution of local populations. As mentioned in the previous sections, it is necessary to apply a correction factor to obtain an accurate density of N. norvegicus individuals from the different abundances. By combining this adjusted density with the size-class frequency distribution, an estimation of biomass can be achieved (Morello et al., 2007; Aydin and Aydin, 2011). Behavioral aspects such as activity rhythms and habitat use can be provided by acoustic tracking of individuals and eDNA methods. Finally, biodiversity data can complement the steps that focus on N. norvegicus, bringing the monitoring protocol towards a more holistic, ecosystem-based approach. In ecosystem-based fisheries’ management, a lack of knowledge of biotic interactions, such as predation or competition, could lead to a misinterpretation of the consequences of overfishing and therefore restraining stocks viability (Shackell et al., 2010).
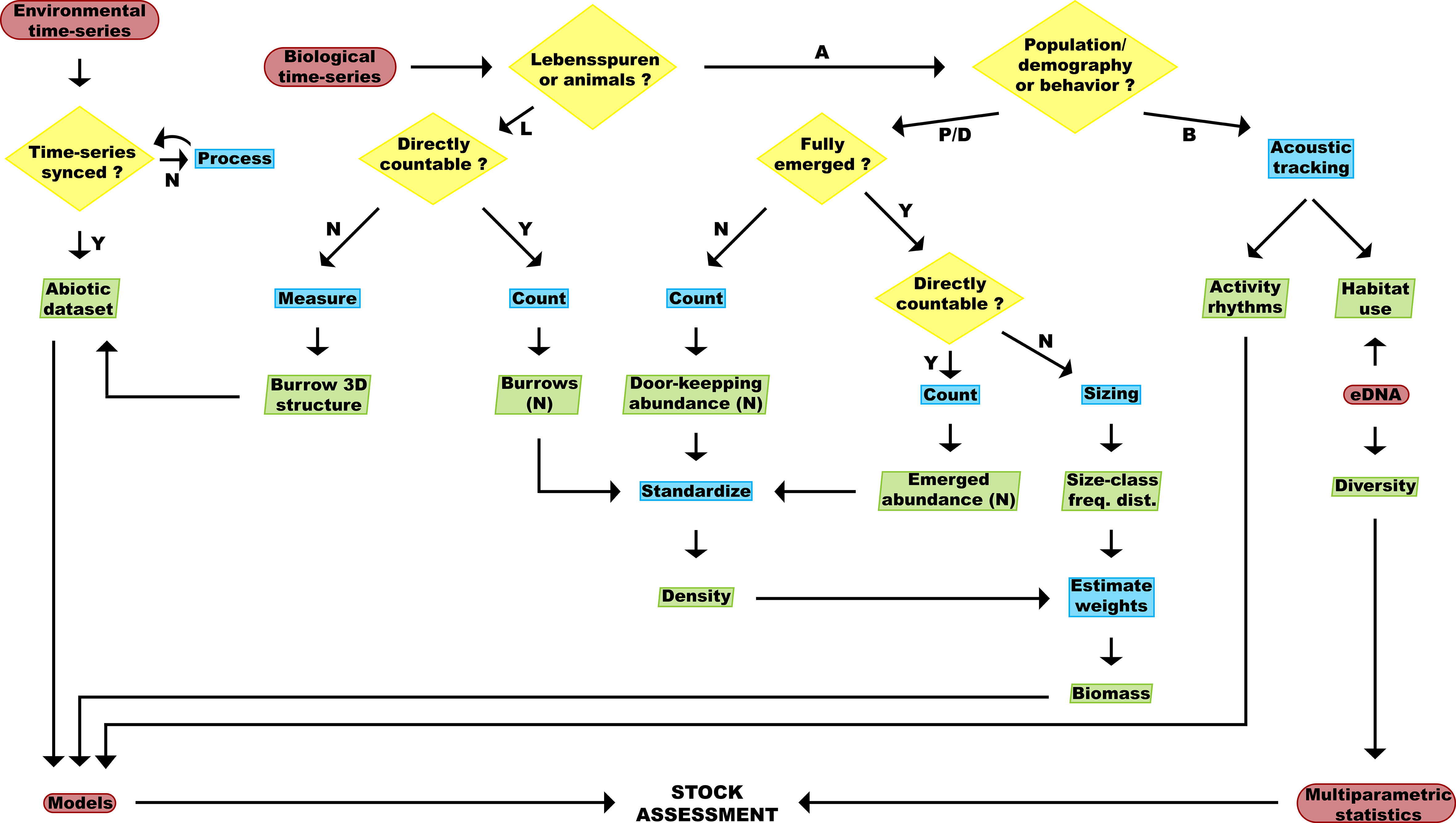
Figure 8 Flowchart of environmental and biological data treatment steps which comprise the proposed protocol for the assessment of N. norvegicus stocks. Red round-edged boxes, yellow diamond-shaped boxes, blue rectangular boxes and green tilted boxes represent input/output points, binary decision knots, processes and datasets, respectively. In the binary decision nodes: “Lebensspuren or animals?” L stands for “Lebensspuren” and (A) for “Animals”; “Population/Demography or Behavior?” P/D stands for “Population/Demography” and (B) for “Behavior”. Y/N stand for “Yes” or “No”, respectively.
Applying an index such as the Predation Index (Vigo et al., 2022), based on predator’s densities (as identified by stable isotope and DNA analyses on predators’ stomachs contents (see previous Section), could help to better understand the fluctuations on N. norvegicus population. Ecosystem modelling is widely used for ecosystem-based fishery management and the assessment of Marine Protected Areas (MPAs; Buchary et al., 2002). Ecosystem modelling considers consumer-prey interaction, approaching the magnitude of these interactions, densities and biomasses of each trophic level (Fogarty, 2014). Predator densities are the main factor altering prey biomasses (Angelini et al., 2016), regulating the restoration of overexploited species (Clements and Hay, 2017) and whose lack of knowledge could even lead to regime habitat shifts (Daskalov et al., 2007).
The different datasets can either be used to feed modelling approaches or in conventional multivariate analyses, in order to reach the final objective (i.e., stock assessment). A simplified visualization of the entire process is presented in Figure 8.
Author contributions
JA: Conceptualization, Software, Investigation, Resources, Data Curation, Writing – Original Draft, Writing – Review and Editing, Visualization, Supervision. DC: Methodology, Writing – Original Draft, Writing – Review and Editing, Visualization. NR: Writing – Review and Editing. NB: Methodology, Software. AB: Methodology, Resources. MC: Methodology, Resources, Data Curation. JC: Conceptualization, Investigation, Resources. CC: Methodology, Software, Formal Analysis, Resources, Data Curation, Writing – Original Draft, Visualization. JR: Methodology, Resources. AF: Methodology. SFi: Methodology. SFl: Methodology, Writing – Review and Editing. JG: Methodology. JJ: Methodology. PJ: Methodology. CL: Conceptualization, Investigation, Resources. ML: Methodology, Visualization. SM: Methodology. MM: Conceptualization, Investigation, Resources, Data Curation, Writing – Review and Editing, Visualization. IM: Methodology, Visualization. LM: Methodology, Resources. AN: Methodology, Visualization. JN: Methodology. NP: Methodology, Data Curation. GP: Methodology. CS: Methodology. SS: Methodology, Resources. MV: Methodology. YV: Writing – Review and Editing. AW: Methodology, Writing – Review and Editing. JD: Conceptualization, Investigation, Resources, Data Curation, Writing – Original Draft, Writing – Review and Editing, Visualization, Supervision. All authors contributed to the article and approved the submitted version.
Funding
The present research was carried out within the framework of the activities of the Spanish Government through the “Severo Ochoa Centre Excellence” accreditation to ICM-CSIC (CEX2019-000928-S) and the Research Unit Tecnoterra (ICM-CSIC/UPC). Other projects that supported the work were those of the Plan Estatal de Investigación Científica y Técnica y de Innovación 2017–2020 of the Spanish government: BITER-LANDER (PID2020-114732RB-C32); BITER-ECO (PID2020-114732RB-C31); BITER-AUV (PID2020-114732RB-C33); PLOME (PLEC2021-007525/AEI/10.13039/501100011033). Moreover, part of the conceptual development, falls within the framework of EU LIFE Project ECOREST (LIFE20 NAT/ES/001270), which also funded DC. SaF acknowledges the HGF-project (Helmholtz-Future Topic Project) ARCHES (Autonomous Robotic Networks to Help Modern Societies) and HGF POF IV funding for conducting this research. NR was funded by the Spanish Government (Agencia Española de Investigación; AEI) through the ‘Severo Ochoa Centre of Excellence’ accreditation (CEX2019-000928-S).
Conflict of interest
The authors declare that the research was conducted in the absence of any commercial or financial relationships that could be construed as a potential conflict of interest.
Publisher’s note
All claims expressed in this article are solely those of the authors and do not necessarily represent those of their affiliated organizations, or those of the publisher, the editors and the reviewers. Any product that may be evaluated in this article, or claim that may be made by its manufacturer, is not guaranteed or endorsed by the publisher.
Supplementary material
The Supplementary Material for this article can be found online at: https://www.frontiersin.org/articles/10.3389/fmars.2022.969071/full#supplementary-material
Footnotes
- ^ https://ec.europa.eu/commission/presscorner/detail/en/ip_21_5660.
- ^ https://standardgraphs.ices.dk.
- ^ https://resbio.obsea.es/galeria.php.
- ^ https://www.iseamc.com/crawler-systems/the-rossia-series-crawler/.
- ^ https://www.robex-allianz.de/en/deep-sea-crawler/viator/.
- ^ https://www.santannapisa.it/it/news/passo-avanti-nella-salvaguardia-e-nella-conoscenza-dellecosistema-marino-presentati-i-primi.
- ^ https://cirs.udg.edu/auvs-technology/auvs/.
- ^ https://www.geomar.de/en/research/fb2/fb2-mg/benthic-biogeochemistry/in-situ-englisch-titel/lander-systems.
- ^ emso.eu/wp-content/uploads/2020/06/SmartLobster-project_final_26June2020.pdf.
- ^ https://www.flotteoceanographique.fr/en/Facilities/Vessels-Deep-water-submersible-vehicles-and-Mobile-equipments/Deep-water-submersible-vehicles/Ariane.
- ^ https://www.marineboard.eu/publications/big-data-marine-science.
- ^ https://opencv.org/.
References
Abad E., Cerviño-López S., Drogou M., Fifas S., Garcia D., Gerritsen H., et al. (2021). ICES report of the working group for the bay of Biscay and the Iberian waters ecoregion (WGBIE). ICES Sci. Rep. 3 (48), 1101. doi: 10.17895/ices.pub.8212
Abadi M., Barham P., Chen J., Chen Z., Davis A., Dean J., et al. (2016). “Tensorflow: large-scale machine learning on heterogeneous distributed systems,” in Proceedings of the 12th USENIX conference on operating systems design and implementation (Savannah, GA, USA: USENIX Association), 265–283.
Aguzzi J., Aristegui-Ezquibela M., Burgos C., Doyle J., Fifas S., Firmin C., et al. (2022). ICES report of the working group on Nephrops surveys (WGNEPS). ICES Sci. Rep. 4 (29), 183. doi: 10.17895/ices.pub.19438472
Aguzzi J., Bahamon N., Doyle J., Lordan C., Tuck I. D., Chiarini M., et al. (2021). Burrow emergence rhythms of Nephrops norvegicus by UWTV and surveying biases. Sci. Rep. 11, 5797. doi: 10.1038/s41598-021-85240-3
Aguzzi J., Bahamon N., Marotta L. (2009). The influence of light availability and predatory behavior of the decapod crustacean Nephrops norvegicus on the activity rhythms of continental margin prey decapods. Mar. Ecol. 30, 366–375. doi: 10.1111/j.1439-0485.2008.00276.x
Aguzzi J., Chatzievangelou D., Company J. B., Thomsen L., Marini S., Bonofiglio F., et al. (2020a). The potential of video imagery from worldwide cabled observatory networks to provide information supporting fish-stock and biodiversity assessment. ICES J. Mar. Sci. 77, 2396–2410. doi: 10.1093/icesjms/fsaa169
Aguzzi J., Chatzievangelou D., Francescangeli M., Marini S., Bonofiglio F., del Río J., et al. (2020b). The hierarchic treatment of marine ecological information from spatial networks of benthic platforms. Sensors 20, 1751. doi: 10.3390/s20061751
Aguzzi J., Chatzievangelou D., Marini S., Fanelli E., Danovaro R., Flögel S., et al. (2019). New high-tech interactive and flexible networks for the future monitoring of deep-sea ecosystems. Environ. Sci. Technol. 53, 6616–6631. doi: 10.1021/acs.est.9b00409
Aguzzi J., Company J. B. (2010). Chronobiology of deep-water decapod crustaceans on continental margins. Adv. Mar. Biol. 58, 155–225. doi: 10.1016/B978-0-12-381015-1.00003-4
Aguzzi J., Company J. B., Navarro J., Bahamon N., Rotllant G., García J. A., et al. (2018). “New monitoring technologies assisting deep-water and deep-sea crustacean decapods stock assessment,” in ICES report of the working group on Nephrops surveys (WGNEPS). ICES CM 2018/EOSG, vol. :18. (Lorient, France: ICES), 226.
Aguzzi J., Company J. B., Sardà F. (2007). The activity rhythm of berried and unberried females of Nephrops norvegicus (Crustacea, decapoda). Crustaceana 80, 1121–1134. doi: 10.1163/156854007782008577
Aguzzi J., Costa C., Robert K., Matabos M., Antonucci F., Juniper K., et al. (2011). Automated image analysis for the detection of benthic crustaceans and bacterial mat coverage using the VENUS undersea cabled network. Sensors 11, 10534–10556. doi: 10.3390/s111110534
Aguzzi J., Sardà F. (2008). A history of recent advancements on Nephrops norvegicus behavioral and physiological rhythms. Rev. Fish Biol. Fish. 18, 235–248. doi: 10.1007/s11160-007-9071-9
Aguzzi J., Sardà F., Abelló P., Company J. B., Rotllant G. (2003). Diel and seasonal patterns of Nephrops norvegicus (Decapoda: Nephropidae) catchability in the western Mediterranean. Mar. Ecol. Prog. Ser. 258, 201–211. doi: 10.3354/meps258201
Angelini S., Hillary R., Morello E. B., Plagányi E. E., Martinelli M., Manfredi C., et al. (2016). An ecosystem model of intermediate complexity to test management options for fisheries: A case study. Ecol. Model. 319, 218–232. doi: 10.1016/j.ecolmodel.2015.07.031
Angelini S., Martinelli M., Santojanni A., Colella S. (2020). Biological evidence of the presence of different subpopulations of Norway lobster (Nephrops norvegicus) in the Adriatic Sea (Central Mediterranean Sea). Fish. Res. 221, 105365. doi: 10.1016/j.fishres.2019.105365
Aydin I., Aydin C. (2011). Length-length and length-weight relationships in Nephrops norvegicus from the Aegean Sea (Linnaeus, 1758). Mediterr. Mar. Sci. 12, 121–128. doi: 10.12681/mms.56
Baden S., Pihl L., Rosenberg R. (1990). Effects of oxygen depletion on the ecology, blood physiology and fishery of the Norway lobster Nephrops norvegicus. Mar. Ecol. Prog. Ser. 67, 141–155. doi: 10.3354/meps067141
Bailey N., Chapman C. J., Alfonso-Dias M., Turrell W. (1995). The influence of hydrographic factors on Nephrops distribution and biology. ICES CM/Q :17, 13.
Barbier R., Cajgfinger T., Dominjon A., Doan Q. T. (2012). “An ebCMOS camera system for extreme low light imaging,” in Proceedings of the imaging and applied optics technical papers: ITu4C.6 (Washington, DC, USA: Optical Society of America OSA). doi: 10.1364/ISA.2012.ITu4C.6
Bell M. C., Redant F., Tuck I. (2006). “Nephrops species,” in Lobsters: biology, management, aquaculture and fisheries. Ed. Phillips B. F. (Oxford, UK: Blackwell Publishing), 412–461. doi: 10.1002/9780470995969.ch13
Beng K. C., Corlett R. T. (2020). Applications of environmental DNA (eDNA) in ecology and conservation: opportunities, challenges and prospects. Biodivers. Conserv. 29, 2089–2121. doi: 10.1007/s10531-020-01980-0
Bertrand J. A., de Sola L. G., Papaconstantinou C., Relini G., Souplet A. (2002). The general specifications of the MEDITS surveys. Sci. Mar. 66 (S2), 9–17. doi: 10.3989/scimar.2002.66s29
Borchers D. L., Zucchini W., Heide-Jørgensen M. P., Canadas A., Langrock R. (2013). Using hidden Markov models to deal with availability bias on line transect surveys. Biometrics 69, 703–713. doi: 10.1111/biom.12049
Breitburg D., Levin L. A., Oschlies A., Grégoire M., Chavez F. P., Conley D. J., et al. (2018). Declining oxygen in the global ocean and coastal waters. Science 359, eaam7240. doi: 10.1126/science.aam7240
Bruno J. F., Bates A. E., Cacciapaglia C., Pike E. P., Amstrup S. C., van Hooidonk R., et al. (2018). Climate change threatens the world’s marine protected areas. Nat. Clim. Change 8, 499–503. doi: 10.1038/s41558-018-0149-2
Buchary E. A., Alder J., Nurhakim S., Wagey T. (2002). “The use of ecosystem-based modelling to investigate multi-species managemetn strategies for capture fisheries in the Bali strait, indonesia,” in In the use of ecosystem models to investigate multispecies management strategies for capture fisheries. Eds. Pitcher T., Cochrane K. (Vancouver, BC, Canada: The Fisheries Centre, University of British Columbia), 24–32. doi: 10.14288/1.0348151
Campbell N., Allan L., Weetman A., Dobby H. (2009). Investigating the link between Nephrops norvegicus burrow density and sediment composition in Scottish waters. ICES J. Mar. Sci. 66, 2052–2059. doi: 10.1093/icesjms/fsp176
Cardinale M., Colloca F., Bonanno A., Scarcella G., Arneri E., Jadaud A., et al. (2021). The Mediterranean fishery management: A call for shifting the current paradigm from duplication to synergy. Mar. Policy 131, 104612. doi: 10.1016/j.marpol.2021.104612
Carpi P., Scarcella G., Cardinale M. (2017). The saga of the management of fisheries in the Adriatic Sea: History, flaws, difficulties, and successes toward the application of the common fisheries policy in the Mediterranean. Front. Mar. Sci. 4. doi: 10.3389/fmars.2017.00423
Cattano C., Claudet J., Domenici P., Milazzo M. (2018). Living in a high CO2 world: A global meta-analysis shows multiple trait-mediated fish responses to ocean acidification. Ecol. Monogr. 88, 320–335. doi: 10.1002/ecm.1297
Chapman C. J. (1980). “Ecology of juvenile and adult Nephrops,” in The biology and management of lobsters II. Eds. Cobb. J. S., Phillips B. F. (New York, USA: New York Academic Press), 143–178. doi: 10.1016/B978-0-08-091734-4.50011-1
Chapman C. J., Howard F. G. (1979). Field observations on the emergence rhythm of the Norway lobster Nephrops norvegicus using different methods. Mar. Biol. 51, 157–165. doi: 10.1007/BF00555195
Chapman C. J., Johnstone A. D. F., Rice A. L. (1975). “The behaviour and ecology of the Norway lobster Nephrops norvegicus (L),” in Proceedings of the 9th European marine biology symposium. Ed. Barnes H. B. (Aberdeen, UK: Aberdeen University Press), 59–74.
Chatzievangelou D., Aguzzi J., Ogston A., Suárez A., Thomsen L. (2020). Spatio-temporal monitoring of key deep-sea megafauna with Internet operated crawlers as a tool for ecological status assessment. Prog. Oceanogr. 184, 102321. doi: 10.1016/j.pocean.2020.102321
Chatzievangelou D., Thomsen L., Doya C., Purser A., Aguzzi J. (2022). Transects in the deep: opportunities with tele-operated resident seafloor robots. Front. Mar. Sci. 9:833617. doi: 10.3389/fmars.2022.833617
Chiarini M., Guicciardi S., Angelini S., Tuck I. D., Grilli F., Penna P., et al. (2022). Accounting for environmental and fishery management factors when standardizing CPUE data from a scientific survey: a case study for Nephrops norvegicus in the pomo pits area (Central Adriatic Sea). PloS One 17, e0270703. doi: 10.1371/journal.pone.0270703
Chiesa J. J., Aguzzi J., García J. A., Sardà F., de la Iglesia H. (2010). Light intensity determines temporal niche switching of behavioral activity in deep-water Nephrops norvegicus (Crustacea: Decapoda). J. Biol. Rhythms 25, 277–287. doi: 10.1177/0748730410376159
Clements C. S., Hay M. E. (2017). Size matters: Predator outbreaks threaten foundation species in small marine protected areas. PloS One 12, e0171569. doi: 10.1371/journal.pone.0171569
Cooley S. R., Kite-Powell H. L., Doney S. C. (2009). Ocean acidification's potential to alter global marine ecosystem services. Oceanography 22, 172–181. doi: 10.5670/oceanog.2009.106
Corgnati L., Marini S., Mazzei L., Ottaviani E., Aliani S., Conversi A., et al. (2016). Looking inside the ocean: Toward an autonomous imaging system for monitoring gelatinous zooplankton. Sensors 16, 2124. doi: 10.3390/s16122124
Corrigan D., Sooknanan K., Doyle J., Lordan C., Kokaram A. (2019). A low-complexity mosaicking algorithm for stock assessment of seabed-burrowing species. IEEE J. Ocean. Eng. 44, 386–400. doi: 10.1109/JOE.2018.2808973
CSE (2020) Visual object tagging tool (VOTT). Available at: https://github.com/microsoft/VoTT (Accessed June 02, 2020).
Danovaro R., Fanelli E., Aguzzi J., Billett D., Carugati L., Corinaldesi C., et al. (2020). Ecological variables for developing a global deep-ocean monitoring and conservation strategy. Nat. Ecol. Evol. 4, 181–192. doi: 10.1038/s41559-019-1091-z
Daskalov G. M., Grishin A. N., Rodionov S., Mihneva V. (2007). Trophic cascades triggered by overfishing reveal possible mechanisms of ecosystem regime shifts. Proc. Natl. Acad. Sci. U.S.A. 104, 10518–10523. doi: 10.1073/pnas.0701100104
Davies T. W., Smyth T. (2017). Why artificial light at night should be a focus for global change research in the 21st century. Glob. Change Biol. 24, 872–882. doi: 10.1111/gcb.13927
Dobby H., Doyle J., Jónasson J., Jonsson P., Leocádio A., Lordan C., et al. (2021). ICES survey protocols – manual for Nephrops underwater TV surveys, coordinated under ICES working group on Nephrops surveys (WGNEPS). ICES Techniques Mar. Environ. Sci. 65, 44. doi: 10.17895/ices.pub.8014
Dombaxe M.Á.D. (2002). “Predation intensity of Norway lobster (Nephrops norvegicus) by cod (Gadus morhua),” in Háfadjúp, southwest Iceland and the relative prey preference of cod fed on capelin (Mallotus vissosus) and Norway lobster. [MSc thesis] ([Reykjavík (Icealand)]: University of Iceland).
Dominguez-Carrió C., Fontes J., Morato T. (2021). A cost-effective video system for a rapid appraisal of deep-sea benthic habitats: The azor drift-cam. Methods Ecol. Evol. 12, 1379–1388. doi: 10.1111/2041-210X.13617
EU (2008). Council regulation (EC) no. 199/2008 concerning the establishment of a community framework for the collection, management and use of data in the fisheries sector and support for scientific advice regarding the common fisheries policy. Off. J. Eur. Union L 60, 1–12.
EU (2020) Council regulation (EU) no. 123/2020 of 27 January 2020 fixing for 2020 the fishing opportunities for certain fish stocks and groups of fish stocks, applicable in union waters and, for union fishing vessels, in certain non-union waters. Available at: https://eur-lex.europa.eu/eli/reg/2020/123/oj (Accessed July 29, 2022).
European Commission, Joint Research Centre, Scientific, Technical and Economic Committee for Fisheries (2022). STECF 69th plenary report (PLEN-22-01). Eds. Ulrich C., Doerner H. (Luxemburg: Publications of the European Union). doi: 10.2760/192738
FAO (2020). “The state of Mediterranean and black Sea fisheries 2020,” in General fisheries commission for the Mediterranean (Rome: FAO). doi: 10.4060/cb2429en
FAO (2021a). “FAO yearbook. fishery and aquaculture statistics 2019/FAO annuaire,” in Statistiques des pêches et de l'aquaculture 2019/FAO anuario. estadísticas de pesca y acuicultura 2019 (Rome/Roma: FAO).
FAO (2021b) Fishery and aquaculture statistics. global capture production 1950-2019 (FishstatJ). Available at: www.fao.org/fishery/statistics/software/fishstatj/en (Accessed 2021).
Farmer A. S. D. (1974). Field assessments of diurnal activity in Irish Sea populations of the Norway lobster Nephrops norvegicus (L.) (Decapoda: Nephropidae). Estuar. Coast. Mar. Sci. 2, 37–47. doi: 10.1016/0302-3524(74)90026-7
Florko K. R., Davidson E. R., Lees K. J., Hammer L. J., Lavoie M. F., Lennox R. J., et al. (2021). Tracking movements of decapod crustaceans: a review of a half-century of telemetry-based studies. Mar. Ecol. Prog. Ser. 679, 219–239. doi: 10.3354/meps13904
Fogarty M. J. (2014). The art of ecosystem-based fishery management. Can. J. Fish. Aquat. Sci. 71, 79–490. doi: 10.1139/cjfas-2013-0203
Friard O., Gamba M. (2016). BORIS: a free, versatile open-source event-logging software for video/audio coding and live observations. Methods Ecol. Evol. 7, 1325–1330. doi: 10.1111/2041-210X.12584
Froglia C., Atkinson J. R., Tuck I., Arneri E. (1997). “Underwater television survey a tool to estimate Nephrops stock biomass on the Adriatic trawling grounds,” in Tisucu godina prvoga spomena ribarstva u hrvata. Ed. Finka B. (Zagreb, Croatia: Hrvatska Akademija Znanosti I Umjetnosti), 657–667.
Fuentes M. M. P. B., Bell I., Hagihara R., Hamann M., Hazel J., Huth A., et al. (2015). Improving in-water estimates of marine turtle abundance by adjusting aerial survey counts for perception and availability biases. J. Exp. Mar. Biol. Ecol. 471, 77–83. doi: 10.1016/j.jembe.2015.05.003
GFCM (2021) Report of the working group on stock assessment of demersal species (WGSAD). Available at: https://www.fao.org/gfcm/technical-meetings/detail/en/c/1412431/ (Accessed June 02, 2022).
GFCM (2022) Report of the scientific advisory committee on fisheries (SAC) working group on stock assessment of demersal species (WGSAD). Available at: https://www.fao.org/gfcm/technical-meetings/detail/en/c/1506058/ (Accessed June 02, 2022).
Girshick R., Donahue J., Darrell T., Malik J. (2016). Region-based convolutional networks for accurate object detection and segmentation. IEEE Trans. Pattern Anal. Mach. Intell. 38, 142–158. doi: 10.1109/TPAMI.2015.2437384
Grinyó J., Iacono C. L., Pierdomenico M., Conlon S., Corbera G., Gràcia E. (2020). Evidences of human impact on megabenthic assemblages of bathyal sediments in the alboran Sea (western Mediterranean). Deep Sea Res. I 165, 103369. doi: 10.1016/j.dsr.2020.103369
Haque I. R. I., Neubert J. (2020). Deep learning approaches to biomedical image segmentation. Inform. Med. Unlocked 18, 100297. doi: 10.1016/j.imu.2020.100297
Hugens D. J., Atkinson R. J. A. (1997). A towed video survey of megafaunal bioturbation in the north-eastern Irish Sea. J. Mar. Biol. Ass. U.K. 77, 635–653. doi: 10.1017/S0025315400036122
Huver J. R., Koprivnikar J., Johnson P. T. J., Whyard S. (2015). Development and application of an eDNA method to detect and quantify a pathogenic parasite in aquatic ecosystems. Ecol. Appl. 25, 991–1002. doi: 10.1890/14-1530.1
ICES (2007). “Workshop on the use of UWTV surveys for determining abundance in Nephrops stocks throughout European waters,” in ICES CM 2007/ACFM, vol. 14. (Heraklion, Greece: ICES), 198.
ICES (2009). “Report of the study group on Nephrops surveys (SGNEPS),” in ICES CM: 2009/LRC, vol. 15. (Aberdeen, UK: ICES), 52.
ICES (2016). “Report of the workshop to consider FMSY ranges for stocks in ICES categories 1 and 2 in Western waters (WKMSYREF4),” in ICES CM 2015/ACOM, vol. 58. (Brest, France: ICES), 187.
ICES (2017). “Report of the workshop on Nephrops burrow counting,” in WKNEPS 2016 report. ICES CM 2016/SSGIEOM, vol. 34. (Reykjavík, Iceland: ICES), 62.
ICES (2018). “Interim report of the working group on Nephrops surveys (WGNEPS),” in ICES CM: 2017/SSGIEOM, vol. 19. (Heraklion, Greece: ICES), 78.
ICES (2021a). “Advice on fishing opportunities. report of the ICES advisory committe,” in ICES advice 2021, section 1.1.1 (Copenhagen, Denmark: ICES). doi: 10.17895/ices.advice.7720
ICES (2021b). “ICES fisheries management reference points for category 1 and 2 stocks; technical guidelines,” in Report of the ICES advisory committee 2021. ICES advice 2021, section 16.4.3.1. doi: 10.17895/ices.advice.7891
ICES (2022). ICES Report of the Working Group on Nephrops Surveys (WGNEPS). ICES Sci. Rep. 4 (29), 183 doi: 10.17895/ices.pub.19438472
Issifu I., Alava J. J., Lam V. W., Sumaila U. R. (2022). Impact of ocean warming, overfishing and mercury on European fisheries: A risk assessment and policy solution framework. Front. Mar. Sci. 8. doi: 10.3389/fmars.2021.770805
Katho E., Sbragaglia V., Aguzzi J., Breithaupt T. (2013). Sensory biology and behaviour of Nephrops norvegicus. Adv. Mar. Biol. 64, 66–106. doi: 10.1016/B978-0-12-410466-2.00003-0
Kelleher K. (2002). “The costs of monitoring, control and surveillance of fisheries in developing countries,” in FAO fisheries circular 976 (Rome: FAO).
Kroeker K. J., Kordas R. L., Crim R. N., Singh G. G. (2010). Meta-analysis reveals negative yet variable effects of ocean acidification on marine organisms. Ecol. Lett. 13, 1419–1434. doi: 10.1111/j.1461-0248.2010.01518.x
Langtimm C. A., Dorazio R. M., Stith B. M., Doyle T. J. (2011). New aerial survey and hierarchical model to estimate manatee abundance. J. Wildl. Manage. 75, 399–412. doi: 10.1002/jwmg.41
Leocádio A., Weetman A., Wieland K. (2018). Using UWTV surveys to assess and advise on Nephrops stocks. ICES Cooperative Res. Rep. 340, 49. doi: 10.17895/ices.pub.4370
Letschert J., Stollberg N., Rambo H., Kempf A., Berkenhagen J., Stelzenmüller V. (2021). The uncertain future of the Norway lobster fisheries in the north Sea calls for new management strategies. ICES J. Mar. Sci. 78, 3639–3649. doi: 10.1093/icesjms/fsab204
Lin L. I.-K. (1989). A concordance correlation coefficient to evaluate reproducibility. Biometrics 45, 255–268. doi: 10.2307/2532051
MacLeod N., Benfield M., Culverhouse P. (2010). Time to automate identification. Nature 467, 154–155. doi: 10.1038/467154a
Main J., Sangster G. I. (1985). The behaviour of the Norway lobster Nephrops norvegicus (L) during trawling. Department Agric. Fisheries Scotland Rep. 34, 1–23.
Malde K., Handegard N. O., Eikvil L., Salberg A. B. (2019). Machine intelligence and the data-driven future of marine science. ICES J. Mar. Sci. 77, 1274–1285. doi: 10.1093/icesjms/fsz057
Marini S., Bonofiglio F., Corgnati L. P., Bordone A., Schiaparelli S., Peirano A. (2022). Long-term automated visual monitoring of Antarctic benthic fauna. Methods Ecol. Evol. 13, 1746–1764. doi: 10.1111/2041-210X.13898
Marini S., Corgnati L., Mantovani C., Bastianini M., Ottaviani E., Fanelli E., et al. (2018a). Automated estimate of fish abundance through the autonomous imaging device GUARD1. Measurement 126, 72–75. doi: 10.1016/j.measurement.2018.05.035
Marini S., Fanelli E., Sbragaglia V., Azzurro E., Del Rio J., Aguzzi J. (2018b). Tracking fish abundance by underwater image recognition. Sci. Rep. 8, 13748. doi: 10.1038/s41598-018-32089-8
Marini S., Gjeci N., Govindaraj S., But A., Sportich B., Ottaviani E., et al. (2020). Enduruns: An integrated and flexible approach for seabed survey through autonomous mobile vehicles. J. Mar. Sci. Eng. 8, 633. doi: 10.3390/JMSE8090633
Marshall N. T., Vanderploeg H. A., Chaganti S. R. (2021). Environmental (e)RNA advances the reliability of eDNA by predicting its age. Sci. Rep. 11, 2769. doi: 10.1038/s41598-021-82205-4
Martinelli M., Medvešek D., Chiarini M., Domenichetti F., Canduci G., Zacchetti L., et al. (2022). Italy And Croatia pomo pits, central Adriatic Sea (GSA 17) ADRIATIC UWTV SURVEYS and pomo monitoring activity. ICES Sci. Rep. 4, 176–180. doi: 10.17895/ices.pub.19438472
Martinelli M., Morello E. B., Isajlović I., Belardinelli A., Lucchetti A., Santojanni A., et al. (2013). Towed underwater television towards the quantification of Norway lobster, squat lobsters and sea pens in the Adriatic Sea. Acta Adriat. 154, 3–12. doi: 10.32582/aa.54.1.369
Martín M., Puig P., Palanques A., Giamportone A. (2014). Commercial bottom trawling as a driver of sediment dynamics and deep seascape evolution in the anthropocene. Anthropocene 7, 1–15. doi: 10.1016/j.ancene.2015.01.002
Masmitja I., Navarro J., Gomariz S., Aguzzi J., Kieft B., O'Reilly T., et al. (2020). Mobile robotic platforms for the acoustic tracking of deep-sea demersal fishery resources. Sci. Robot. 5, eabc3701. doi: 10.1126/scirobotics.abc3701
Masubuchi S., Watanabe E., Seo Y., Okazaki S., Sasagawa T., Watanabe K., et al. (2020). Deep-learning-based image segmentation integrated with optical microscopy for automatically searching for two-dimensional materials. NPJ 2D Mater. Appl. 4, 3. doi: 10.1038/s41699-020-0137-z
Maynou F. X., Sardà F. (1997). Nephrops norvegicus population and morphometrical characteristic in relation to substrate heterogeneity. Fish. Res. 30, 139–149. doi: 10.1016/S0165-7836(96)00549-8
McGeady R., Lordan C., Power A. M. (2021). Shift in the larval phenology of a marine ectotherm due to ocean warming with consequences for larval transport. Limnol. Oceanogr. 66, 543–557. doi: 10.1002/lno.11622
Melaku Canu D., Laurent C., Morello E. B., Querin S., Scarcella G., Vrgoc N., et al. (2021). Nephrops norvegicus in the Adriatic Sea: Connectivity modeling, essential fish habitats, and management area network. Fish. Oceanogr. 30, 349–365. doi: 10.1111/fog.12522
Moller T. H., Naylor E. (1980). Environmental influence on locomotor activity in Nephrops norvegicus (Crustacea:Decapoda). J. Mar. Biol. Ass. U.K. 60, 103–113. doi: 10.1017/S0025315400024152
Moniruzzaman M., Islam S. M. S., Bennamoun M., Lavery P. (2017). “Deep learning on underwater marine object detection: A survey,” in Advanced concepts for intelligent vision systems, vol. 10617 . Eds. Blanc-Talon J., Penne R., Philips W., Popescu D., Scheunders P. (Cham, Switzerland: Springer), 150–160. doi: 10.1007/978-3-319-70353-4_13
Morello E. B., Froglia C., Atkinson R. J. A. (2007). Underwater television as a fishery-independent method for stock assessment of Norway lobster (Nephrops norvegicus) in the central Adriatic Sea (Italy). ICES J. Mar. Sci. 64, 1116–1123. doi: 10.1093/icesjms/fsm082
Naseer A., Nava Baro E., Khan S. D., Vila Y. (2022a). A novel detection refinement technique for accurate identification of Nephrops norvegicus burrows in underwater imagery. Sensors 22, 4441. doi: 10.3390/s22124441
Naseer A., Nava Baro E., Khan S. D., Vila Y., Doyle J. (2022b). Automatic detection of Nephrops norvegicus burrows from underwater imagery using deep learning. Comput. Mater. Contin. 70, 5321–5344. doi: 10.32604/cmc.2022.020886
Newland P. L., Neil D. M., Chapman C. J. (1992). Escape swimming in the Norway lobster. J. Crust. Biol. 12, 342–353. doi: 10.2307/1549027
Oakley S. G. (1979). “Diurnal and seasonal changes in the timing of peak catches of Nephrops norvegicus reflecting changes in behavior,” in Cyclical phenomena in marine plants and animals. Eds. Naylor E., Hartnoll R. G. (Oxford, UK: Pergamon Press), 367–373. doi: 10.1016/B978-0-08-023217-1.50054-0
Parslow-Williams P., Goodheir C., Atkinson R. J. A., Taylor A. C. (2002). Feeding of the Norway lobster, Nephrops norvegicus in the firth of Clyde, Scotland. Ophelia 56, 101–120. doi: 10.1080/00785236.2002.10409493
Pedersen M. W., Berg C. W. (2011). A stochastic surplus production model in continuous time. Fish Fish 18, 226–243. doi: 10.1111/faf.12174
Picardi G., Chellapurath M., Iacoponi S., Stefanni S., Laschi C., Calisti M. (2020). Bioinspired underwater legged robot for seabed exploration with low environmental disturbance. Sci. Robot. 5, eaaz1012. doi: 10.1126/scirobotics.aaz1012
Powell A., Eriksson S. P. (2013). Reproduction: life cycle, larvae and larviculture. Adv. Mar. Biol. 64, 201–245. doi: 10.1016/B978-0-12-410466-2.00006-6
Rice A. L., Chapman C. J. (1971). Observations on the burrows and burrowing behaviour of two mud-dwelling decapod crustaceans, Nephrops norvegicus and Goneplax rhomboides. Mar. Biol. 10, 330–342. doi: 10.1007/BF00368093
Ritchie E. G., Elmhagen B., Glen A. S., Letnic M., Ludwig G., McDonald R. A. (2012). Ecosystem restoration with teeth: What role for predators? Trends Ecol. Evol. 27, 265–271. doi: 10.1016/j.tree.2012.01.001
Robinson N. J., Johnsen S., Brooks A., Frey L., Judkins H., Vecchione M., et al. (2021). Studying the swift, smart, and shy: Unobtrusive camera-platforms for observing large deep-sea squid. Deep Sea Res. I 172, 103538. doi: 10.1016/j.dsr.2021.103538
Rountree R., Aguzzi J., Marini S., Fanelli E., De Leo F., Del Rio J., et al. (2020). Towards an optimal design for ecosystem-level ocean observatories. Oceanogr. Mar. Biol. Annu. Rev. 58, 79–106. doi: 10.1201/9780429351495-2
Russo T., Morello E. B., Parisi A., Scarcella G., Angelini S., Labanchi L., et al. (2018). A model combining landings and VMS data to estimate landings by fishing ground and harbor. Fish. Res. 199, 218–230. doi: 10.1016/j.fishres.2017.11.002
Saba G. K., Wright-Fairbanks E., Miles T. N., Chen B., Cai W. J., Wang K., et al. (2018). “Developing a profiling glider pH sensor for high resolution coastal ocean acidification monitoring,” in OCEANS 2018 MTS/IEEE (Charleston, SC, USA: IEEE). doi: 10.1109/OCEANS.2018.8604918
Sanchez P. J. B., Marquez F. P. G., Govindara S., But A., Sportich B., Marini S., et al. (2021). Use of UIoT for offshore surveys through autonomous vehicles. Pol. Marit. Res. 28, 175–189. doi: 10.2478/pomr-2021-0044
Sandler M., Howard A., Zhu M., Zhmoginov A., Chen L. (2018). “Mobilenetv2: inverted residuals and linear bottlenecks,” in Proceedings of the IEEE conference on computer vision and pattern recognition (Salt Lake City, UT, USA: IEEE), 4510–4520. doi: 10.1109/CVPR.2018.00474
Santana C., Wieczorek A. M., Browne P., Graham C. T., Power A. M. (2020). Importance of suspended particulate organic matter in the diet of Nephrops norvegicus (Linnaeus 1758). Sci. Rep. 10, 3387. doi: 10.1038/s41598-020-60367-x
Sardà F., Aguzzi J. (2012). A review of burrow counting as an alternative to other typical methods of assessment of Norway lobster populations. Rev. Fish Biol. Fish. 22, 409–422. doi: 10.1007/s11160-011-9242-6
Sbragaglia V., Aguzzi J., García J. A., Sarriá D., Gommariz S., Costa C., et al. (2013). An automated multi-flume actograph for the study of behavioral rhythms of burrowing organisms. J. Exp. Mar. Biol. Ecol. 446, 177–185. doi: 10.1016/j.jembe.2013.05.018
Sbragaglia V., García J. A., Chiesa J. J., Aguzzi J. (2015). Effect of simulated tidal currents on the burrow emergence rhythms of the Norway lobster (Nephrops norvegicus). Mar. Biol. 162, 2007–2016. doi: 10.1007/s00227-015-2726-5
Sbragaglia V., Leiva D., Arias A., García J. A., Aguzzi J., Breithaupt T. (2018). Fighting over burrows: Rank related benefits during emergence of dominance hierarchies in the Norway lobster (Nephrops norvegicus). J. Exp. Biol. 220, 4624–4633. doi: 10.1242/jeb.165969
Sbrana M., Zupa W., Ligas A., Capezzuto F., Chatzispyrou A., Follesa M. C., et al. (2019). Spatiotemporal abundance pattern of deep-water rose shrimp, Parapenaeus longirostris, and Norway lobster, Nephrops norvegicus, in European Mediterranean waters. Sci. Mar. 83 (S1), 71–80. doi: 10.3989/scimar.04858.27A
Scott K. N. (2020). Ocean acidification: A due diligence obligation under. Int. J. Mar. Coast. Law 35, 382–408. doi: 10.1163/15718085-BJA10005
Shackell N. L., Frank K. T., Fisher J. A. D., Petrie B., Leggett W. C. (2010). Decline in top predator body size and changing climate alter trophic structure in an oceanic ecosystem. Proc. R. Soc Lond. B. 277, 1353–1360. doi: 10.1098/rspb.2009.1020
Shelton P. M. J., Gaten E., Chapman C. J. (1985). Light and retinal damage in Nephrops norvegicus (L.) (Crustacea). Proc. R. Soc Lond. B. 226, 236. doi: 10.1098/rspb.1985.0092217
Shima R., Yunan H., Fukuda O., Okumura H., Arai K., Bu N. (2017). “Object classification with deep convolutional neural network using spatial information,” in Proceedings of the international conference on intelligent informatics and biomedical sciences (ICIIBMS) (Okinawa, Japan: IEEE), 135–139. doi: 10.1109/ICIIBMS.2017.8279704
Smyth K., Elliott M. (2016). “Effects of changing salinity on the ecology of the marine environment” in Stressors in the Marine Environment: Physiological and Ecological Responses; Societal Implications, eds. Solan M., Whiteley N. (Oxford, UK: Oxford University Press), 161–174. doi: 10.1093/acprof:oso/9780198718826.003.0009
Sokolova M., Thompson F., Mariani P., Krag L. A. (2021). Towards sustainable demersal fisheries: NepCon image acquisition system for automatic Nephrops norvegicus detection. PloS One 16, e0252824. doi: 10.1371/journal.pone.0252824
Soltan S., Oleinikov A., Demirci M. F., Shintemirov A. (2020). Deep learning-based object classification and position estimation pipeline for potential use in robotized pick-and-place operations. Robotics 9, 63. doi: 10.3390/robotics9030063
Sooknanan K., Doyle J., Lordan C., Wilson J., Kokaram A., Corrigan D. (2014). “Mosaics for Nephrops detection in underwater survey videos,” in OCEANS 2014 (St. John's, NL, Canada: IEEE). doi: 10.1109/OCEANS.2014.7003142
Stefanni S., Mirimin L., Stanković D., Chatzievangelou D., Bongiorni L., Marini S., et al. (2022). Framing cutting-edge integrative deep-sea biodiversity monitoring via environmental DNA and optoacoustic augmented infrastructures. Front. Mar. Sci. 8. doi: 10.3389/fmars.2021.797140
Stentiford G. D., Douglas N., Atkinson R. J. A. (2001). Alteration of burrow-related behaviour of the Norway lobster Nephrops norvegicus during infection by the parasitic dinoflagellate Hematodinium. Mar. Fresh. Behav. Physiol. 34, 139–156. doi: 10.1080/10236240109379068
Stentiford G. D., Neil D. M., Atkinson R. J. A. (1999). “Infection by the dinoflagellate hematodinium in the Norway lobster (Nephrops norvegicus l.) on the west coast of Scotland, united kingdom. j. shellfish res. 18, 334,” in Abstract from the 1999 annual meeting of the national shellfisheries association (Canada: Halifax, NS).
Stewart P. A. M. (1975). Comparative fishing for Nephrops norvegicus (Linnaeus) using a beam trawl fitted with electric ticklers. Scot. Dep. Agric. Fish. Mar. Res. 1, 1–10.
Szegedy C., Vanhoucke V., Ioffe S., Shlens J., Wojna Z. (2016). “Rethinking the inception architecture for computer vision,” in Roceedings of the IEEE conference on computer vision and pattern recognition (Las Vegas, NV, USA: IEEE), 2818–2826. doi: 10.1109/CVPR.2016.308
Thomsen L., Aguzzi J., Costa C., De Leo F., Ogston A., Purser A. (2017). The oceanic biological pump: rapid carbon transfer to depth at continental margins during winter. Sci. Rep. 7, 10763. doi: 10.1038/s41598-017-11075-6
Tuck I. D., Atkinson R. J. A., Chapman C. J. (1994). The structure and seasonal variability in the spatial distribution of Nephrops norvegicus burrows. Ophelia 40, 13–25. doi: 10.1080/00785326.1994.10429547
Tuck I. D., Chapman C. J., Atkinson R. J. A. (1997). Population biology of the Norway lobster, Nephrops norvegicus (L.) in the firth of Clyde, Scotland-I: Growth and density. ICES J. Mar. Sci. 54, 125–135. doi: 10.1006/jmsc.1996.0179
Tully O., Hillis J. P. (1995). Causes and spatial scales of variability in population structure of Nephrops norvegicus (L.) in the Irish Sea. Fish. Res. 21, 329–347. doi: 10.1016/0165-7836(94)00303-E
Ungfors A., Bell E., Johnson M. L., Cowing D., Dobson N. C., Bublitz R., et al. (2013). Nephrops fisheries in European waters. Adv. Mar. Biol. 64, 247–314. doi: 10.1016/B978-0-12-410466-2.00007-8
Vigo M., Navarro J., Giménez J., Andón N., Martínez-Lage A., Company J. B., et al. (2022). Using molecular and stable isotope markers to identify the main predators of Nephrops norvegicus in Mediterranean deep-water ecosystems. Mar. Ecol. Prog. Ser. doi: 10.3354/meps14110
Vigo M., Navarro J., Masmitja I., Aguzzi J., García J. A., Rotllant G., et al. (2021). Spatial ecology of the Norway lobster (Nephrops norvegicus) in Mediterranean deep-water environments: implications for designing no-take marine reserves. Mar. Ecol. Prog. Ser. 674, 173–188. doi: 10.3354/meps13799
Widder E. A., Robison B. H., Reisenbichler K. R., Haddock S. H. D. (2005). Using red light for in situ observations of deep-sea fishes. Deep Sea Res. I 52, 2077–2085. doi: 10.1016/j.dsr.2005.06.007
Zaiko A., Pochon X., Garcia-Vazquez E., Olenin S., Wood S. A. (2018). Advantages and limitations of environmental DNA/RNA tools for marine biosecurity: management and surveillance of non-indigenous species. Front. Mar. Sci. 5. doi: 10.3389/fmars.2018.00322
Keywords: Nephrops norvegicus, UWTV, stocks monitoring, autonomous networks, biomimicking platforms, optoacoustic imaging, geo-sonars, eDNA
Citation: Aguzzi J, Chatzievangelou D, Robinson NJ, Bahamon N, Berry A, Carreras M, Company JB, Costa C, del Rio Fernandez J, Falahzadeh A, Fifas S, Flögel S, Grinyó J, Jónasson JP, Jonsson P, Lordan C, Lundy M, Marini S, Martinelli M, Masmitja I, Mirimin L, Naseer A, Navarro J, Palomeras N, Picardi G, Silva C, Stefanni S, Vigo M, Vila Y, Weetman A and Doyle J (2022) Advancing fishery-independent stock assessments for the Norway lobster (Nephrops norvegicus) with new monitoring technologies. Front. Mar. Sci. 9:969071. doi: 10.3389/fmars.2022.969071
Received: 14 June 2022; Accepted: 11 August 2022;
Published: 09 September 2022.
Edited by:
Daphne Cuvelier, University of the Azores, PortugalReviewed by:
Simon De Lestang, Department of Primary Industries and Regional Development of Western Australia (DPIRD), AustraliaIan David Tuck, Ministry for Primary Industries, New Zealand
Nick Caputi, Department of Primary Industries and Regional Development of Western Australia (DPIRD), Australia
Copyright © 2022 Aguzzi, Chatzievangelou, Robinson, Bahamon, Berry, Carreras, Company, Costa, del Rio Fernandez, Falahzadeh, Fifas, Flögel, Grinyó, Jónasson, Jonsson, Lordan, Lundy, Marini, Martinelli, Masmitja, Mirimin, Naseer, Navarro, Palomeras, Picardi, Silva, Stefanni, Vigo, Vila, Weetman and Doyle. This is an open-access article distributed under the terms of the Creative Commons Attribution License (CC BY). The use, distribution or reproduction in other forums is permitted, provided the original author(s) and the copyright owner(s) are credited and that the original publication in this journal is cited, in accordance with accepted academic practice. No use, distribution or reproduction is permitted which does not comply with these terms.
*Correspondence: Jacopo Aguzzi, jaguzzi@icm.csic.es; Jennifer Doyle, jennifer.Doyle@marine.ie