- 1Marine Electromechanical Department, Marine electromechanical Department, Xiamen Ocean Vocational College, Xiamen, China
- 2Key Laboratory of Underwater Acoustic Communication and Marine Information Technology, Xiamen University, Xiamen, China
Underwater signal processing based on passive acoustic technology has carried out a lot of research on the behavioral sound of underwater creatures and the protection of marine resources, which proves the feasibility of passive acoustic technology for long-term and non-destructive monitoring of underwater biological sound production. However, at present, most relevant research focuses on fish but little research on shrimp. At the same time, as the main economic breeding industry, Penaeus vannamei has a backward industrial structure, in which the level of intelligence needs to be improved. In this paper, the acoustic signals generated by different physiological behaviors of P. vannamei are collected based on passive acoustic technology. Their different behaviors are finally classified and identified through feature extraction and analysis. Meanwhile, the characteristic non-parametric ANOVA is carried out to explore the relationship between the acoustic signals and the behavior state of P. vannamei to achieve the purpose of real-time monitoring of the behavior state of P. vannamei. The experimental results show that linear prediction cepstrum coefficient (LPCC) and Mel-frequency cepstrum coefficient (MFCC) characteristic coefficients are effective in the classification and recognition of different behavioral acoustic signals with interspecific acoustic signals of P. vannamei. Meanwhile, the SVM classifier based on OvR classification strategy can model the acoustic signal characteristics of different underwater biological behaviors more efficiently and has classification accuracy as high as 93%.
Introduction
For underwater organisms, specific psychological and physiological behaviors are difficult to observe visually. However, they can transmit information within and between species by making sound, which means that they could produce different signals according to specific behavior (Thomsen et al., 2020). Since the 1970s, acoustic methods have been used by fishery developed countries to investigate and evaluate fish resources. Among them, passive acoustic technology is the key technology of target detection, positioning, tracking, and recognition according to the radiated noise of the underwater target, which is gradually applied in the research of underwater organisms because of its low harmfulness and destructiveness to the research object. Meanwhile, the collected signal contains the characteristics of the sounding target itself to characterize the target behavior (Mann et al., 2016). Most of the organisms that can emit and rely on sound waves recorded in the study are fish. Now, it is known that there are 109 families, and more than 800 kinds of fish can make sound (Rountree et al., 2006), most of which are large mammals, such as whales and dolphins, and important economic fish, such as cod, grouper, and yellow croaker. Some invertebrates in important fisheries can also send out sound signals by behavioral movement, such as white shrimp (Berk, 1998), American lobster (Henninger and Watson, 2005), and squid (Iversen et al., 1963). Because of this, the difference between passive acoustic technology and other types of biological acoustics is using the underwater signal acquisition tools such as hydrophones, which are used to collect the acoustic signals of underwater marine organisms instead of the artificially generated sound. It can be used to find and monitor the organisms that emit the acoustic signals. As a non-invasive and non-destructive observation tool, it provides the ability of continuous long-term and remote monitoring. This long-term monitoring can provide important information about the daily and seasonal activity patterns of fish and other marine organisms. It can not only provide an effective acoustic signal database of aquatic organisms but also be of great significance for the development of marine resources and the protection of marine ecology (Noda et al., 2016).
Back in the 1950s, many scholars first studied the different vocal behaviors of aquatic species based on passive acoustic technology. In the field of fish biology and fishery, fishing (Fish et al., 1952) began to apply passive acoustic technology in the study of underwater sound signals generated by fish along the North Atlantic coast in 1952. Nordeide and Kjellsby (1999) recorded and collected the sound generated by the northeast Arctic cod (Gadus morhua L.) near Norway and suggested that passive acoustics can be used to study the spawning behavior. Scharer et al. (Schärer et al., 2014) conducted passive acoustic and synchronous video recording at two spawning gathering points in a spawning season on Mona Island, Puerto Rico, to study the sound related to the reproductive behavior of black grouper and quantify the correlation between the sound signal and the temporal and spatial distribution of reproductive behavior. Parks et al. (2011) recorded the vocal behavior of North Atlantic right whales (Eubalaena glacialis) in the Bay of Fundy, Canada, explored the differences in vocal call types and calling rates of North Atlantic right whales under different behavioral states, and proved that the behavioral state is the main factor affecting the calling rates. Soldevilla et al. (2010) used the passive acoustic records of Risso’s dolphin at six locations in the Southern California Bight from 2005 to 2007 to explore the spatial and temporal trends of its echolocation behavior and motion patterns. The final results showed that Risso dolphins foraged at night, and the southern end of Santa Catalina Island was an important habitat for Risso dolphins throughout the year. In addition to other underwater organisms of fish, Au et al. (Au and Banks, 1998) studied the environmental noise in Kaneoh Bay, which generated when the claws of snapping shrimp are closed, so as to reduce its impact on other offshore organisms and underwater acoustic using for human. Silva et al. (2019) studied the vocal mechanism and main related acoustic variables of feeding signals produced by Litopenaeus vannamei with different body sizes under artificial feeding conditions and explored the relationship between feeding consumption rate and feeding sound signals. Daniel et al. (Smith and Tabrett, 2013) analyzed the feeding sound characteristics of tiger shrimp according to the feeding signal of tiger shrimp in a commercial pond with complex acoustics, which provided a reliable means to detect feeding activities.
Related works
At present, there are few studies on the acoustic signals produced by shrimp culture, especially the life behavior of Penaeus vannamei. Therefore, based on the passive acoustic technology, this paper collects the acoustic signals generated by different physiological behaviors of P. vannamei and analyzes the effective characteristics of the collected signals. Meantime, it cooperates with the efficient classifier and recognizes different behavior states to study the relationship between different behaviors and acoustic signal characteristics of P. vannamei. After receiving a large number of underwater acoustic signals by signal acquisition equipment such as hydrophone, underwater acoustic signal classification and recognition technology analyzes and transforms the acoustic signals accordingly to the automatic classification and recognition through various classification methods. In the practical application of underwater acoustic signal processing, due to the complexity of underwater environment and targets, underwater acoustic signal classification and recognition have always been a hot and difficult point. However, extracting effective signal features are important premises to improve the classification and recognition accuracy and the design of classification method. The difference between the combination method and features will also affect the final classification results.
So far, in the research on the classification and recognition of underwater biological vocal signals, the mainstream research tends to extract the characteristics of time, frequency, and time-frequency domains and classify them by using classical statistical methods, machine learning, and deep learning. The previous research work of this paper mainly focuses on the characteristics of acoustic variables in time and frequency domains (Wei et al., 2020). Mellinger et al. (Mellinger and Clark, 2000), based on the spectral correlation method, constructed a two-dimensional synthetic kernel according to the segmented sound signal of bowhead whale to include the shape of the spectrum diagram called by the target, cross-correlated with the spectrum diagram of the signal to be classified, so as to generate a recognition function and apply the threshold to this function to distinguish the sound signal of bowhead whale. Compared with the classification results of other classifiers such as matched filter, neural network, and hidden Markov model, it is the best method of classification and recognition. Gillespie et al. (2013) described the fully automatic detection and classification method of odontocete whistles. This method finds out that the connected data areas are higher than different pre-determined thresholds based on the spectrum of noise-eliminated sound data and then classifies different species. Finally, the correct classification rate of the four odontocete species has reached more than 94%. However, the classification rate depends largely on the number of species categories entered. When 12 species are included, the average correct classification rate decreases to 58.5%. Relatively, with the deepening of interdisciplinary research in recent years, more and more researchers use signal recognition methods in the field of speech signal processing based on human auditory perception mechanism to recognize underwater target acoustic signals, which opens up a new direction. Ibrahim et al. (2016) proposed a feature extraction method composed of discrete wavelet transform (DWT) and Mel-frequency cepstrum coefficient (MFCC), which is classified by support vector machine (SVM). Experimental studies show that the proposed detection scheme outperforms the spectrogram-based techniques in both detection accuracy and speed. Vieira et al. (2015)extracted cepstrum, MFCC, perceptual linear predictive (PLP), and other characteristic coefficients from different acoustic signals emitted by male toadfish, and classified them by hidden Markov model (HMM) classifier. The final cepstrum coefficient has the best classification effect. Pace et al. (2010) extracted MFCC feature coefficients from humpback whale vocal signals collected in Madagascar in August 2008 and 2009 and used K-means clustering algorithm for automatic classification, which has higher accuracy than manual classification. Taking the underwater acoustic signals of different behaviors of P. vannamei collected based on passive acoustic technology as the research object, and improving the passive recognition ability of P. vannamei underwater behavior as the research goal, this paper studies the feature extraction algorithm and classification recognition model of underwater acoustic signals of different behaviors of P. vannamei. The research results are expected to be applied to the field of underwater biological classification and recognition, including the analysis of underwater acoustic signals of P. vannamei, feature extraction, classification and recognition, and other related application fields.
The main work accomplished in this paper is as follows:
1. Extracting the MFCC and linear prediction cepstrum coefficient (LPCC) features of different behavioral acoustic signals of P. vannamei.
2. Performing non-parametric ANOVA on the extracted features to reduce feature dimension.
3. Using SVM classifier to classify and identify different behavioral signals of P. vannamei according to the characteristics.
The rest of the paper is organized as follows: in s III, the feature extraction algorithm of acoustic signals from different behaviors of P. vannamei is presented. In Section IV, the characteristic non-parametric ANOVA is done. In Section V, the experimental results are carried out. Finally, conclusion is provided in Section VI.
Acoustic signal feature extraction of P. VANNAMEI
Linear prediction cepstrum coefficient
As shown in Figure 1, the LPC coefficient is homomorphically processed to obtain the LPCC. Since the LPCC mainly reflects the channel frequency response, that is, the spectral envelope information of the signal, T can be approximately used as the short-time cepstrum of the original acoustic signal, which can improve the stability of the characteristic parameters (Gupta and Gupta, 2016).
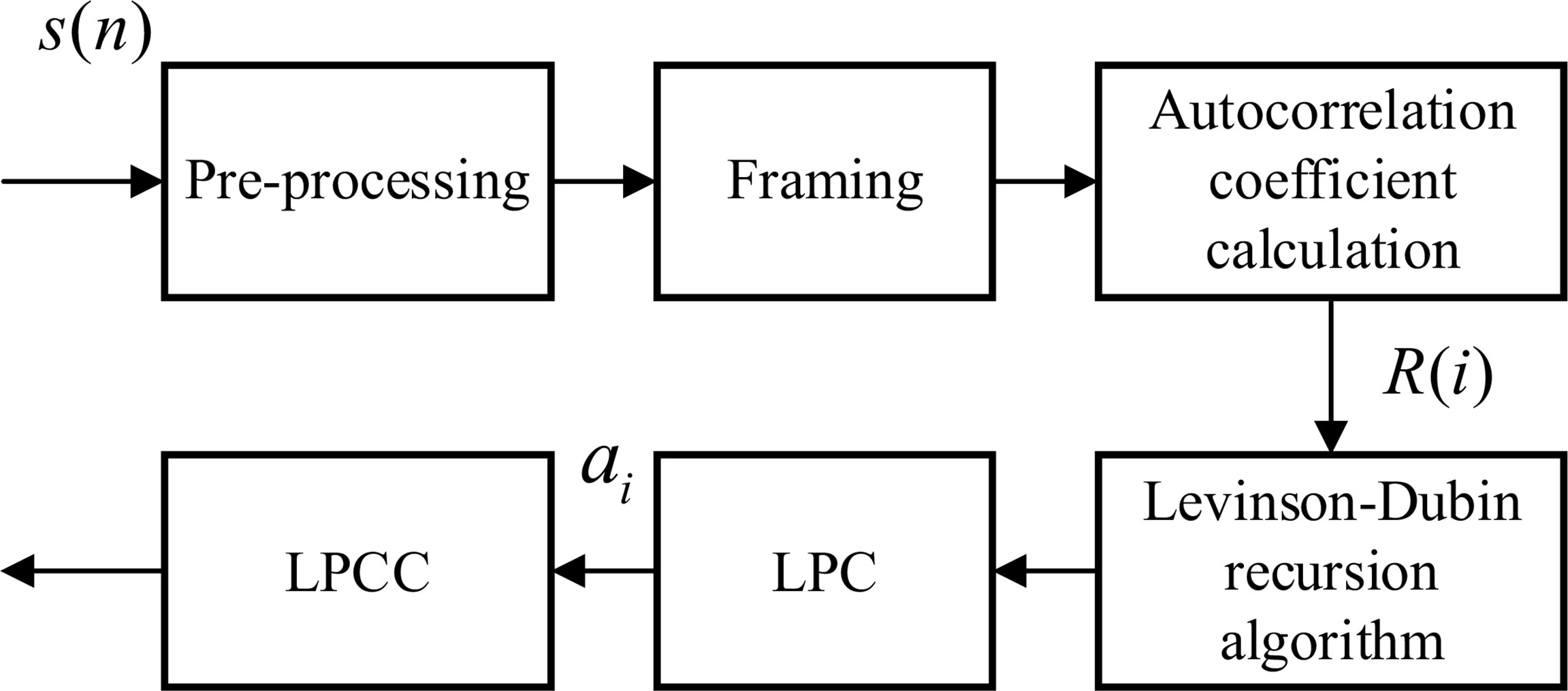
Figure 1 Flow chart of linear prediction cepstrum coefficient (LPCC) characteristic coefficient calculation.
The channel transfer function H(z) is obtained by linear prediction analysis, and its impulse response is h(n). The cepstrum of is obtained.
p is the order, and ai is the coefficient. Since H(z) is resolved in the unit circle, take logarithms on both sides of the above formula at the same time to obtain:
If , the derivation of both sides of the above formula is obtained at the same time z-1:
After sorting, we get:
Namely:
Make the coefficients of z on both sides of Equation (5) equal to each power and obtain the recurrence relationship between and ai:
From the above formula, the cepstrum can be recursively obtained from the LPC prediction coefficient ai. Finally, the LPCC characteristic coefficient ai of behavioral acoustic signal can be obtained for subsequent behavior state classification. Two different behavioral acoustic signals with a time length of 5 s are intercepted, respectively, for 12-dimensional LPCC feature coefficient extraction. Setting the signal frame length to 20 ms, the frame shifts to 10% of the frame length, and the LPCC feature coefficient size to 2,487 frames in 12 dimensions, as shown in Figure 2.
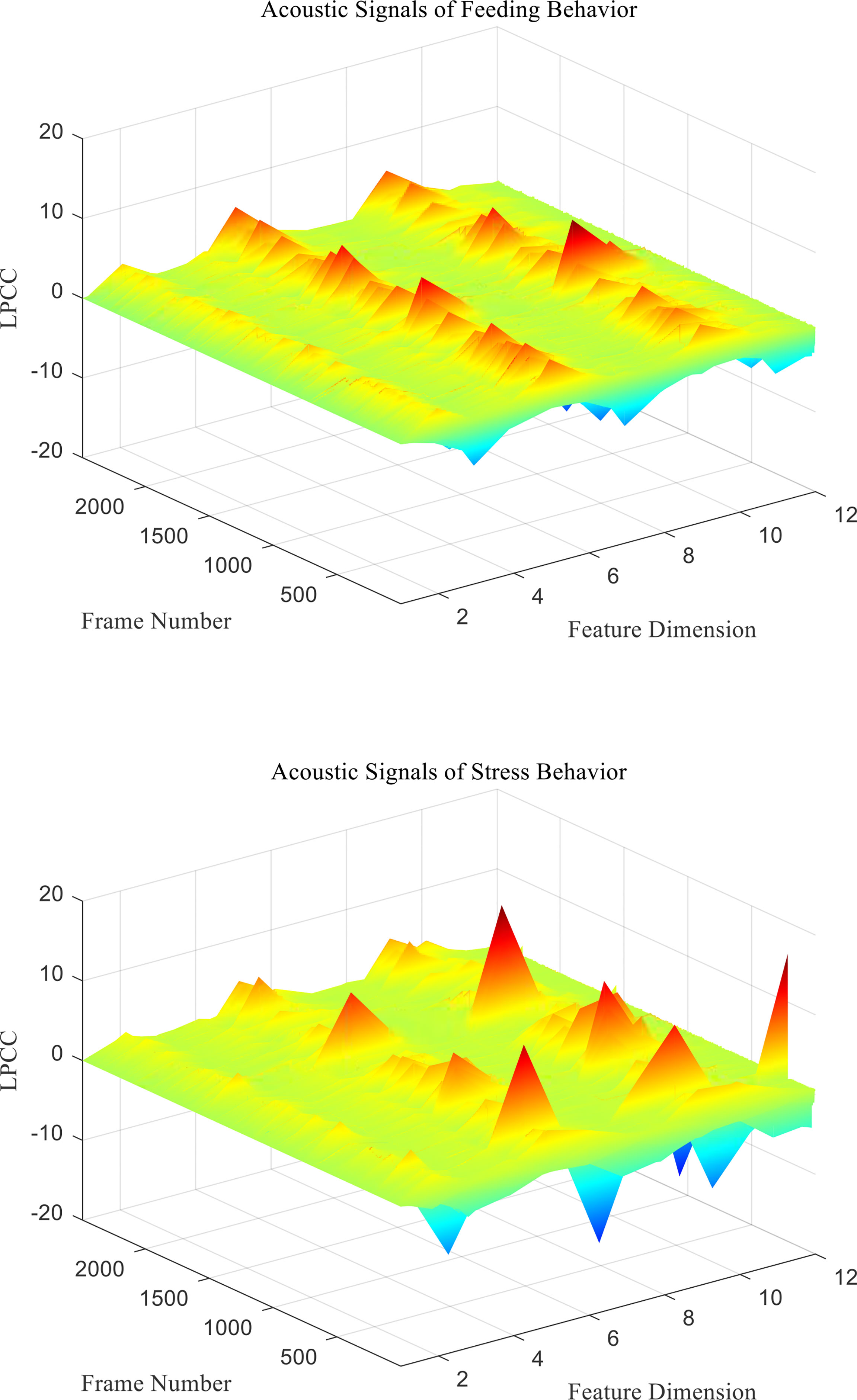
Figure 2 LPCC characteristic coefficients of acoustic signals from different behaviors of P. vannamei.
Mel-frequency cepstrum coefficient
A large number of studies have shown that due to the non-linear auditory sensing mechanism of human ears, they have different auditory perception abilities for sounds with different frequencies. The specific performance is as follows: the perception ability for sounds below 1 kHz follows a linear relationship, while the perception ability for sounds above 1 kHz follows a logarithmic relationship. Therefore, the researchers introduced Mel frequency to describe the non-linear characteristics of human auditory system (Tong et al., 2020): high-frequency resolution at low frequency and low-frequency resolution at high frequency. Mel frequency is defined as follows:
where fMel is Mel frequency, and the unit is Mel. f is the actual frequency, and the unit is Hz. The corresponding relationship curve between them is shown in Figure 3.
Therefore, according to the division method of critical bandwidth, the critical band-pass filter bank, namely, Mel filter bank, is set within the actual spectrum range of the signal, which can simulate the frequency perception characteristics of human ears, as shown in Figure 4.
As can be seen from the Figure 4, Mel filters are formed by superposition of several triangular band-pass filters, and the transfer function of each band-pass filter is:
In the Equation (8), 0 ≤ m < M, and M is the number of filters.f (m) is the center frequency. The spacing between the center frequencies increases with the increase m. f (m) can be defined as:
In Equation (9), the lowest frequency of the filter range is fl. The highest frequency is fh. N is the DFT length, and fs is the sampling frequency. The inverse function of fMel is:
The key point of MFCC feature extraction is to transform the signal linear power spectrum into Mel scale power spectrum. The actual spectrum of the signal can be transformed into Mel scale spectrum through Mel filter bank, and then, the cepstrum coefficient can be calculated, which has good robustness. The main process of MFCC feature parameter extraction of behavioral acoustic signal of P. vannamei is shown in Figure 5.
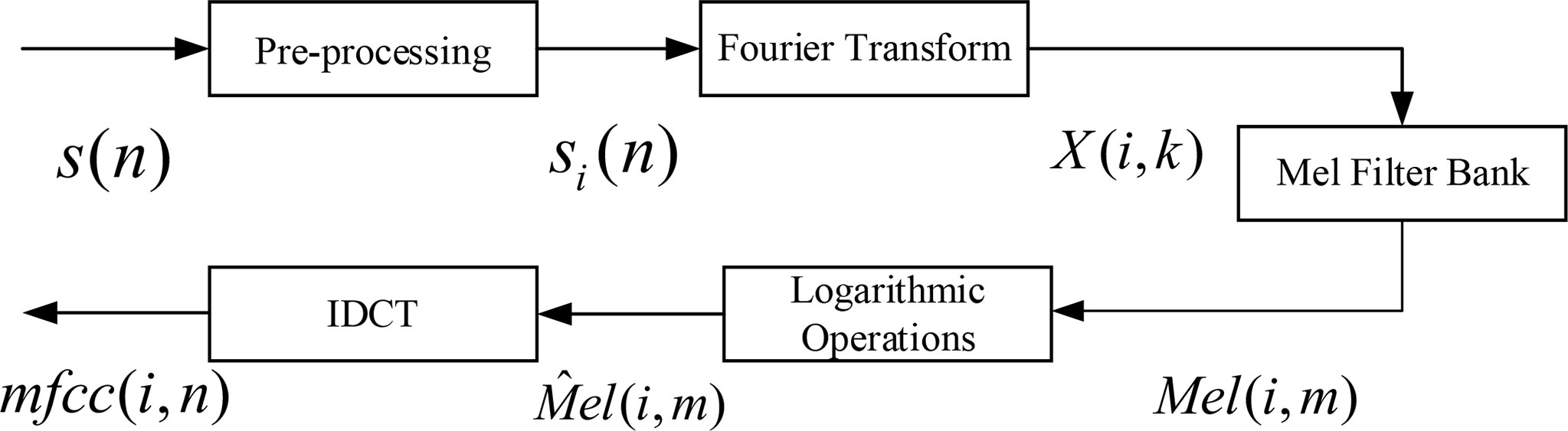
Figure 5 Mel-frequency cepstrum coefficient (MFCC) feature coefficient extraction principle block diagram.
The specific process is as follows:
1) Pre-processing
Pre-processing includes pre-denoising, framing, and windowing with Hamming window. The behavior signal after pre-processing is si (n), and the subscript i represents the ith frame after framing.
2) Fast Fourier Transform
The behavior signal of each frame after framing is transformed by FFT, and the signal is converted from time domain to frequency domain.
The above formula k is the kth spectral line in the frequency domain, and the spectral energy is calculated for the spectrum X (i,k) to obtain the energy spectrum:
3) Calculate the Mel spectral energy of the signal
The calculated signal energy spectrum E(i,k) of each frame is passed through M Mel filter banks to calculate Mel spectrum energy:
4) Mel cepstrum coefficient is calculated by DCT inverse transformation
Calculate the logarithmic energy of Mel spectrum energy:
Calculate the inverse discrete cosine transform (DCT) of to obtain the n-order MFCC of each frame signal:
The above formula M is the number of Mel filters, and m refers to the mth Mel filter. n is the order of MFCC.
In contrast, the standard MFCC only represents the static characteristics of the signal, while the dynamic characteristics can be characterized by the differential MFCC, which can further improve the classification and recognition rate. The calculation process of first- and second-order difference MFCCs is as follows:
mfcc (i) is the MFCC standard coefficient of the ith frame signal. D (i) is the first-order differential MFCC of the ith frame signal. D′ ( i ) is the second-order differential MFCC of the ith frame signal, and k is the number of differential signal frames, usually taken as 2. Two different behavioral acoustic signals with a time length of 5 s are intercepted, respectively, and the 12-dimensional standard MFCC characteristic coefficients are shown in Figure 6. Moreover, the first- and second-order differential MFCCs are extracted, as shown in Figures 7, 8. The signal frame length is set to 20 ms. The frame shift is 10% of the frame length, and the size of the three types of characteristic coefficients is 2,487 frames in 12 dimensions.
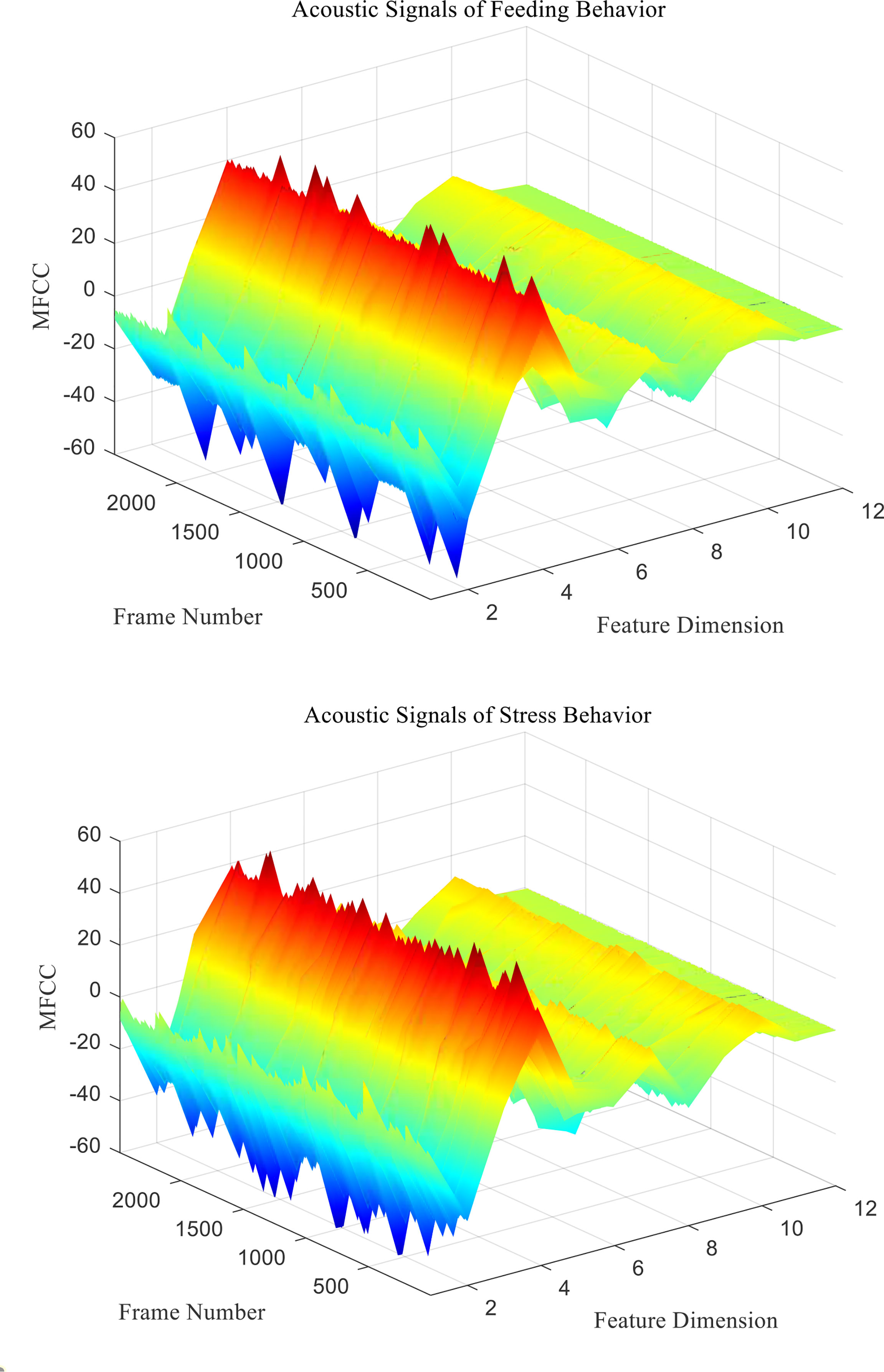
Figure 6 Standard MFCC characteristic coefficients of acoustic signals from different behaviors of Penaeus vannamei.
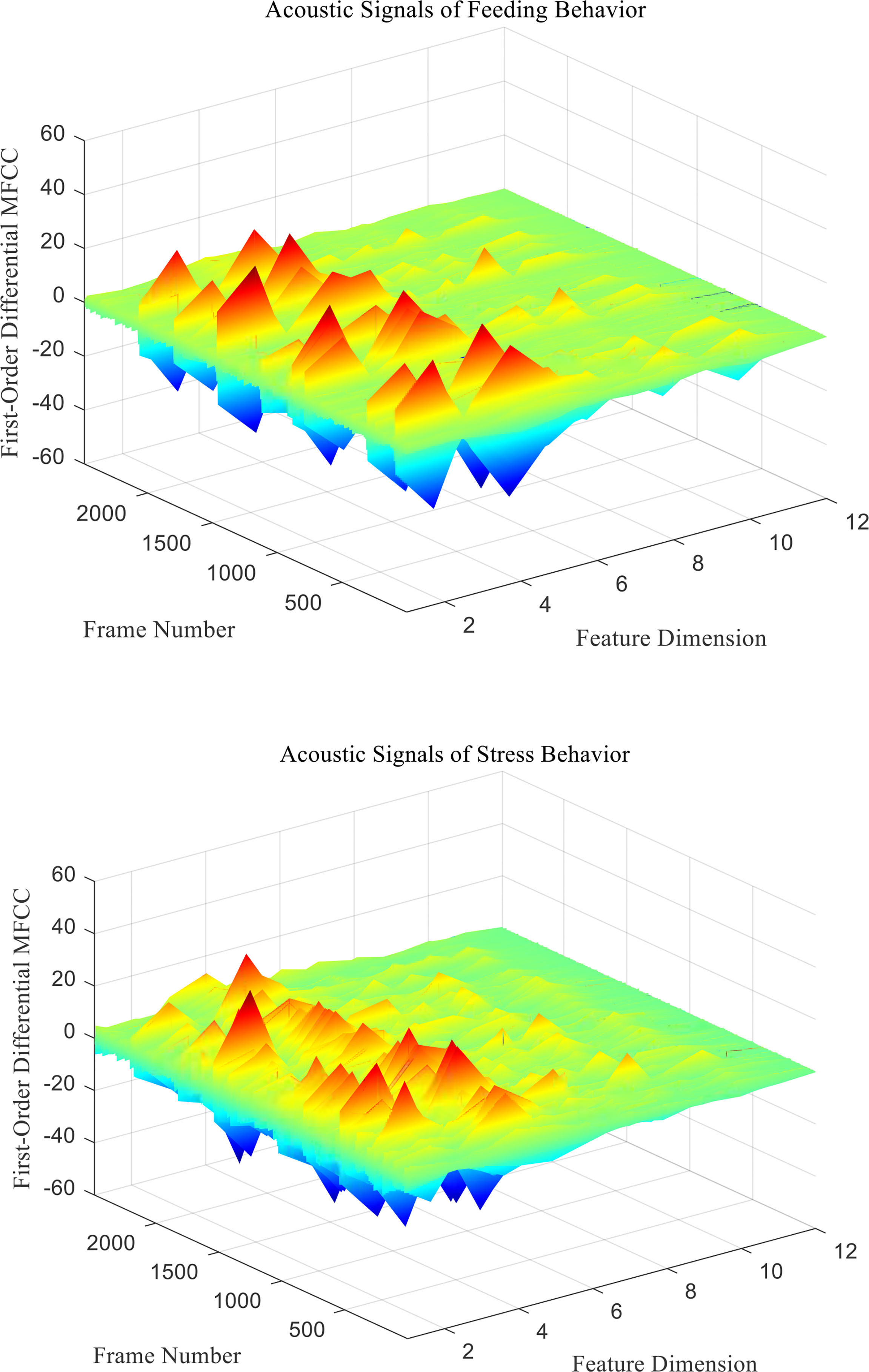
Figure 7 First-Order differential MFCC characteristic coefficients of acoustic signals from different behaviors of Penaeus vannamei.
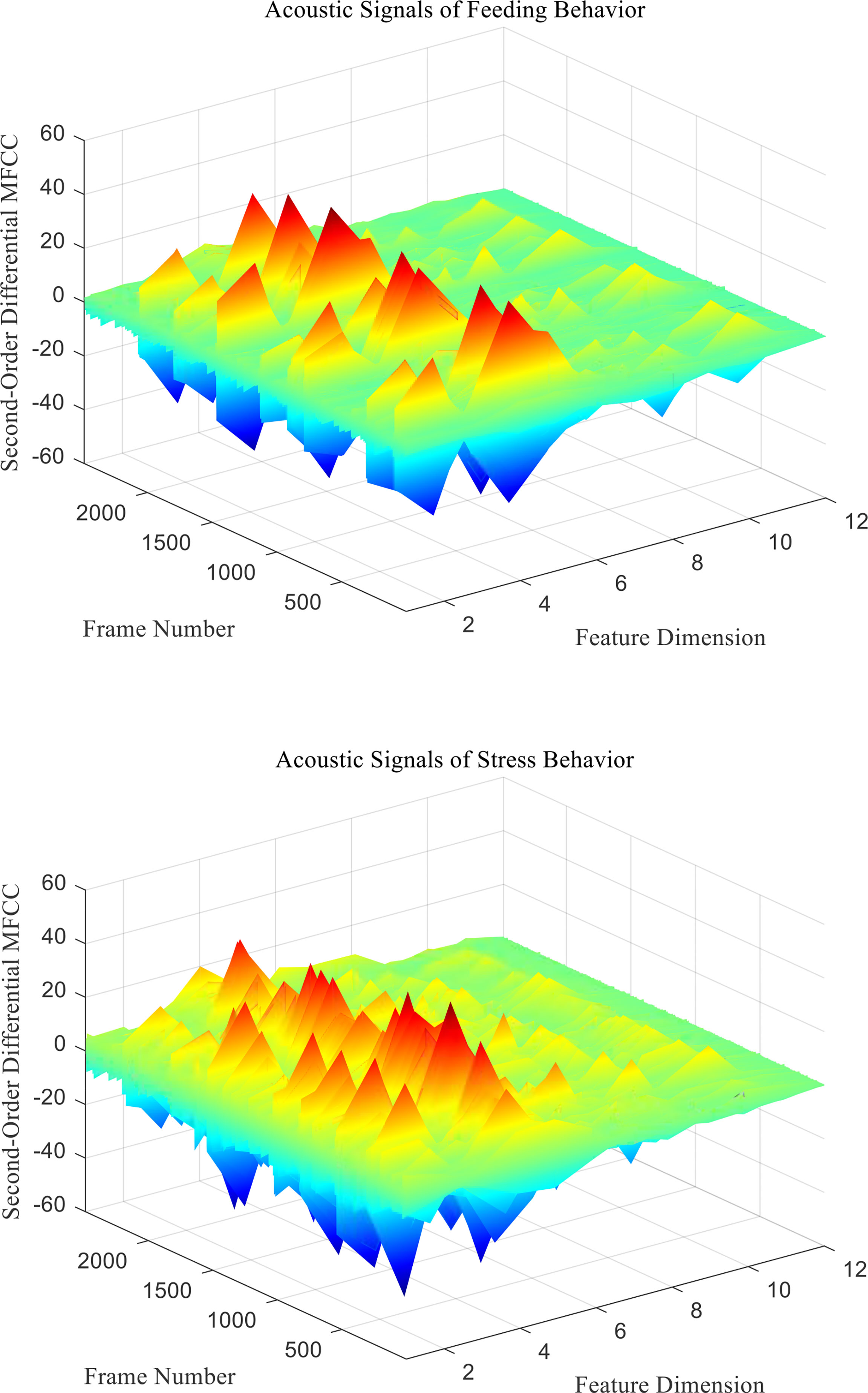
Figure 8 Second-Order differential MFCC characteristic coefficients of acoustic signals from different behaviors of Penaeus vannamei.
Since the channel impulse response in the low cepstrum period can more effectively characterize the signal itself, the main information of the acoustic signal is concentrated in the low dimension. By observing the MFCC characteristic amplitude and changing trend of each dimension of the acoustic signal in Figures 6–8, it can be found that the MFCC just emphasized the low order part.
There are differences in the low-order characteristic dimension. At the same time, for different behavioral acoustic signals, the first- and second-order differential MFCCs can reflect more differences between coefficients in different orders than the standard MFCCs, as shown in Figure 9.
Characteristic non-parametric ANOVA
All kinds of features of different behavioral acoustic signals obey the non-homogeneous distribution of normal variance. If the variance analysis is used, the analysis results will be wrong. Therefore, rank-based non-parametric ANOVA can be used for the data of such distribution. Non-parametric ANOVA does not require the data to obey the normal variance homogeneous distribution, which has wider applicability than variance analysis (Pardo-Fernandez et al., 2015).
In this paper, 1,000 acoustic signal samples of different behaviors were selected, and the sample duration was 100 ms. LPCC and MFCC features were extracted from feeding behavior and stress behavior signals. When the environment changes suddenly, the shrimps will be in a state of stress, such as hypoxia, salinity with different gradients, and water temperature. According to Kruskal–Wallis significance difference analysis, for a given significance level = 0.05, the original assumption is that there is no significant difference between the same type of characteristics of different behavioral acoustic signals. Under this hypothesis, if p < 0.05, the original hypothesis is rejected, and there are significant differences between the same characteristics of different behavioral acoustic signals. If p > 0.05, the original hypothesis is accepted, and there is no significant difference between the same characteristics of different behavioral acoustic signals.
According to the LPCC features, the 12th order LPCC feature coefficients are extracted and analyzed. Through the analysis, it can be found that there were significant differences in the LPCC characteristic coefficient values of each order of sound signals of feeding behavior and stress behavior. However, the L2 coefficient value is p>0.05, and there is no significant difference. Similarly, it can be discarded in the subsequent classification and recognition, as shown in Table 1.

Table 1 Kruskal–Wallis non-parametric ANOVA for LPCC characteristics of acoustic signals with different behaviors.
For MFCC features, the total 35 orders of MFCC feature coefficients M2 to M36 are extracted for significant difference analysis. Among them, M2to M12 are the standard MFCCs, M13 to M24 are the first-order differential MFCCs, and M25 to M36 are the second-order differential MFCCs. Through analysis, it can be found that there are significant differences in the MFCC characteristic coefficient values of feeding and stress behavior signals, but the coefficient values of M13, M14, M17, M18, M20 to M23, and M28 to M30 are p>0.05, so there was no significant difference in these coefficients, and it was difficult to distinguish different behavioral acoustic signals of P. vannamei. Therefore, in the subsequent classification and recognition, it can be discarded to achieve the effect of feature dimensional reduction, as shown in Table 2.
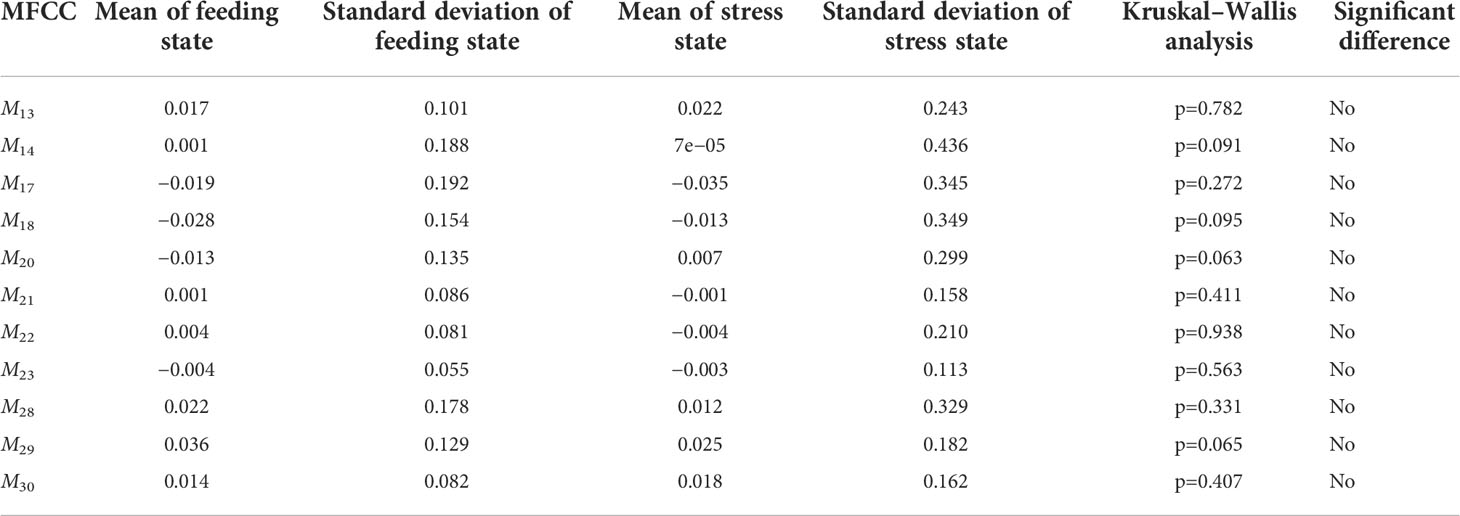
Table 2 Kruskal–Wallis Non-parametric ANOVA of MFCC order characteristics of acoustic signals with different behaviors.
Therefore, based on non-parametric ANOVA of their significant differences, some features with redundant information are eliminated, and the dimension of the features is further reduced. It verifies the effectiveness of acoustic signal features of different behaviors and provides a certain implementation basis for subsequent classification and recognition research.
Experimental analysis
Based on the two kinds of characteristic coefficients of different behavioral acoustic signals of P. vannamei, SVM with different classification strategies is selected to classify and identify different behavioral states within the species. While exploring the classification effect of intraspecific behavior state of P. vannamei, this paper adds the vocal signals of other species of marine organisms to study the differences in behavioral acoustic signals between P. vannamei and other biological species, further verify the effectiveness of feature extraction and classification model, and improve the generalization ability of classification system. This section will compare and analyze the acoustic signals of different behaviors within P. vannamei species and the classification and recognition results of interspecific signals.
Data set processing
The data set of the classification and recognition experiment in this paper consists of five categories of underwater biological signals. The shrimp sound signal experiment uses a WBT22-1107 hydrophone. The frequency range is 1–22 kHz. The receiving sensitivity response of the hydrophone is −193 ± 3dB @ 22 kHz (re 1 V / μPa @1 m, 20 m cable), and the experimental sampling rate is 100 kHz. After feeding, the shrimps mainly eat at the bottom of tank. Therefore, during the experiment, the hydrophone was placed at the center of the water tank down close to the shrimps, as shown in Figure 10 (Wei et al., 2020). In addition to the sound signals of feeding and stress behavior of P. vannamei collected according to the experimental methods introduced in Section II, the other three types of biological sound signals are humpback song signal (Mark), sperm whales “clicks” call signal, and bottlenose dolphin “clicks” call signal (Heimlich et al.).
First, the five types of acoustic signals are pre-processed in frames. A total of 1,500 signals are selected for each type of signal, including 1,000 acoustic signals as the training set, 250 signals as the test set, and 250 signals as the verification set. The length of all signals is 0.1 s. The frame length is 0.02 s. The frame shift is 10%. According to the input form of the classifier and the previous feature significance analysis, the signal feature coefficient extraction of different classifiers is introduced:
1) MFCC characteristics
Calculating the 36-dimensional MFCC of each frame signal for each sample after framing includes the 12-dimensional standard and first- and second-order difference MFCCs. At the same time, after summing and averaging the coefficients corresponding to each order of each frame, the 12-dimensional coefficients of dimensions 1, 13, 14, 17, 18, 20–23, and 29–30 with small significant difference are discarded. The dimension of each sample signal characteristic matrix is 1 × 24.
2) LPCC characteristics
Calculating the 12-dimensional LPCC of each frame signal for each sample after framing, then summing and averaging the corresponding order coefficients of each frame, and discarding the first and second dimensional coefficients with small significant difference, then the dimension of each sample signal characteristic matrix is 1 × 10.
SVM classifier design
In the design and selection of SVM classifiers, based on the two classification strategies of OvR and OvO, this paper designs six SVM classifiers by using ECOC coding principle by selecting three kernel functions: linear, Gaussian, and polynomial kernel functions, as shown in Table 3.
Among them, the penalty factor of all SVM classifiers is C = 1. For the classification and recognition of five kinds of acoustic signals within and between P. vannamei species in this paper, different features based on SMO algorithm are used to quickly optimize the training SVM classifier. Finally, the test set is used to test the training classifier and analyze the results, as shown in Table 4.
In conclusion, LPCC and MFCC are effective in the classification of different behavioral acoustic signals and interspecific acoustic signals of P. vannamei. Meantime, classifiers based on different types and structures have different classification effects. Through experimental comparison and analysis, SVM classifier based on OvR classification strategy according to MFCC features has more stable and excellent classification results. It can model the acoustic signal characteristics of different underwater biological behaviors more efficiently and has the classification accuracy as high as 93%.
Conclusion
Based on passive acoustic technology, this paper collects different behavioral acoustic signals produced by feeding and stress state of P. vannamei and establishes a behavioral acoustic signal database of P. vannamei. At the same time, the characteristics of acoustic signals are studied. After obtaining effective features, SVM classifiers with different structures are designed. According to different features, the classification of intra- and interspecific behavior states of P. vannamei is finally completed. Finally, the SVM classifier based on the OvR classification strategy based on MFCC features can roughly solve the five types of underwater biological acoustic signal classification problems proposed in this paper with the best performance and have a classification accuracy of 93%. Generally, underwater target behavior classification based on underwater acoustic signal is an important research direction of underwater acoustic signal processing, which involves many disciplines such as marine science, sonar technology, signal processing, feature engineering, pattern recognition, and computer technology. Therefore, as a comprehensive subject, the classification and recognition of different behavior states of P. vannamei based on passive acoustic technology not only overcomes the limitations of underwater visual observation research and application but also helps the intelligent development of related industries. It has important theoretical research and engineering practical application value.
Data availability statement
The original contributions presented in the study are included in the article/supplementary material. Further inquiries can be directed to the corresponding author.
Author contributions
MW performed the experiment. KC contributed to the conception of the study. YL performed the data analyses and wrote the manuscript. EC helped perform the analysis with constructive discussions. All authors contributed to the article and approved the submitted version.
Funding
This work was supported by the Xiamen marine and fishery development special fund project “Key technology research and industrialization demonstration of aquaculture automation equipment” (19CZB015HJ02); Fujian marine economic development subsidy fund project (ZHHY-2019-4); Fujian marine economic development special fund project (FJHJF-L-2021-12); Fujian Provincial Department of Education “Smart fishery Application Technology Collaborative Innovation Center” (XTZX-ZHYY-1914).
Conflict of interest
The authors declare that the research was conducted in the absence of any commercial or financial relationships that could be construed as a potential conflict of interest.
Publisher’s note
All claims expressed in this article are solely those of the authors and do not necessarily represent those of their affiliated organizations, or those of the publisher, the editors and the reviewers. Any product that may be evaluated in this article, or claim that may be made by its manufacturer, is not guaranteed or endorsed by the publisher.
References
Au W. W. L., Banks K. (1998). The acoustics of the snapping shrimp synalpheus parneomeris in kaneohe bay. J. Acoustical Soc. America 103 (1), 41–47. doi: 10.1121/1.423234
Berk I. M. (1998). Sound production by white shrimp (penaeus setiferus), analysis of another crustacean-like sound from the gulf of Mexico, and applications for passive sonar in the shrimping industry. J. Shellfish Res. 17 (5), 1497–1500.
Fish M. P., Kelsey A. S., Mowbray W. H. (1952). Studies on the production of underwater sound by north Atlantic coastal fishes (University of Rhode Island: Narragansett Marine Laboratory).
Gillespie D., Caillat M., Gordon J., White P.. (2013). Automatic detection and classification of odontocete whistles. J. Acoustical Soc. America 134 (3), 2427–2437. doi: 10.1121/1.4816555
Gupta H., Gupta D. (2016). “LPC and LPCC method of feature extraction in speech recognition system,” in 2016 6th International Conference-Cloud System and Big Data Engineering (Confluence). IEEE. Noida, India, 498–502.
Heimlich S., Mellinger D., Klinck H. MobySound.org [EB/OL]. Available at: http://www.mobysound.org/mobysound.html.
Henninger H. P., Watson III W H. (2005). Mechanisms underlying the production of carapace vibrations and associated waterborne sounds in the American lobster, homarus americanus. J. Exp. Biol. 208 (17), 3421–3429. doi: 10.1242/jeb.01771
Ibrahim A. K., Zhuang H., Erdol N., Ali AM. (2016). “A new approach for north atlantic right whale upcall detection,” in 2016 International Symposium on Computer, Consumer and Control (IS3C). IEEE. Xi'an, China 260–263.
Iversen R. T. S., Perkins P. J., Dionne R. D. (1963). An indication of underwater sound production by squid. Nature 199 (4890), 250–251. doi: 10.1038/199250a0
Mann D., Locascio J., Wall C. (2016). Listening in the ocean: new discoveries and insights on marine life from autonomous passive acoustic recorders. Listening Ocean 2016, 309–324. doi: 10.1007/978-1-4939-3176-7_12
Mark A. M. Whale acoustics [EB/OL]. Available at: http://www.whaleacoustics.com.
Mellinger D. K., Clark C. W. (2000). Recognizing transient low-frequency whale sounds by spectrogram correlation. J. Acoustical Soc. America 107 (6), 3518–3529. doi: 10.1121/1.429434
Noda J. J., Travieso C. M., Sánchez-Rodríguez D. (2016). Automatic taxonomic classification of fish based on their acoustic signals. Appl. Sci. 6 (12), 443. doi: 10.3390/app6120443
Nordeide J. T., Kjellsby E. (1999). Sound from spawning cod at their spawning grounds. ICES J. Mar. Sci. 56 (3), 326–332. doi: 10.1006/jmsc.1999.0473
Pace F., Benard F., Glotin H., Adam O., White P. (2010). Subunit definition and analysis for humpback whale call classification. Appl. Acoustics 71 (11), 1107–1112. doi: 10.1016/j.apacoust.2010.05.016
Pardo-Fernandez J. C., Jimenez-Gamero M. D., Ghouch A. E. (2015). A non-parametric ANOVA-type test for regression curves based on characteristic functions. Scandinavian J. Stat 42 (1), 197–213. doi: 10.1111/sjos.12102
Parks S. E., Searby A., Celerier A., Johnson M. P., Nowacek D. P., Tyack P. L., et al. (2011). Sound production behavior of individual north Atlantic right whales: implications for passive acoustic monitoring. Endangered Species Res. 15 (1), 63–76. doi: 10.3354/esr00368
Rountree R. A., Gilmore R. G., Goudey C. A., Hawkins AD., Luczkovich JJ., Mann DA., et al. (2006). Listening to fish: applications of passive acoustics to fisheries science. Fisheries 31 (9), 433–446. doi: 10.1577/1548-8446(2006)31[433:LTF]2.0.CO;2
Schärer M. T., Nemeth M. I., Rowell T. J., Appeldoorn RS. (2014). Sounds associated with the reproductive behavior of the black grouper (Mycteroperca bonaci)[J]. Mar. Biol. 161 (1), 141–147. doi: 10.1007/s00227-013-2324-3
Silva J. F., Hamilton S., Rocha J. V., Borie A., Travassos P., Soares R, et al. (2019). Acoustic characterization of feeding activity of litopenaeus vannamei in captivity. Aquaculture 501, 76–81. doi: 10.1016/j.aquaculture.2018.11.013
Smith D. V., Tabrett S. (2013). The use of passive acoustics to measure feed consumption by penaeus monodon (giant tiger prawn) in cultured systems. Aquac. Eng. 57, 38–47. doi: 10.1016/j.aquaeng.2013.06.003
Soldevilla M. S., Wiggins S. M., Hildebrand J. A. (2010). Spatial and temporal patterns of risso’s dolphin echolocation in the southern California bight. J. Acoustical Soc. America 127 (1), 124–132. doi: 10.1121/1.3257586
Thomsen F., Erbe C., Hawkins A., Lepper P., Popper AN., Scholik-Schlomer A., et al. (2020). Introduction to the special issue on the effects of sound on aquatic life. J. Acoustical Soc. America 148 (2), 934–938. doi: 10.1121/10.0001725
Tong Y., Zhang X., Ge Y. (2020). “Classification and recognition of underwater target based on MFCC feature extraction,” in 2020 IEEE International Conference on Signal Processing, Communications and Computing (ICSPCC). IEEE Macau, China. 1–4.
Vieira M., Fonseca P. J., Amorim M. C.P., Teixeira CJC. (2015). Call recognition and individual identification of fish vocalizations based on automatic speech recognition: An example with the lusitanian toadfish. J. Acoustical Soc. America 138 (6), 3941–3950. doi: 10.1121/1.4936858
Keywords: passive acoustic technology, acoustic signal of Penaeus vannamei, feature extraction, SVM, underwater
Citation: Wei M, Chen K, Lin Y and Cheng E (2022) Recognition of behavior state of Penaeus vannamei based on passive acoustic technology. Front. Mar. Sci. 9:973284. doi: 10.3389/fmars.2022.973284
Received: 20 June 2022; Accepted: 29 June 2022;
Published: 27 July 2022.
Edited by:
Xuebo Zhang, Northwest Normal University, ChinaReviewed by:
Qingjian Liang, South China Normal University, ChinaPan Huang, Weifang University, China
Copyright © 2022 Wei, Chen, Lin and Cheng. This is an open-access article distributed under the terms of the Creative Commons Attribution License (CC BY). The use, distribution or reproduction in other forums is permitted, provided the original author(s) and the copyright owner(s) are credited and that the original publication in this journal is cited, in accordance with accepted academic practice. No use, distribution or reproduction is permitted which does not comply with these terms.
*Correspondence: Keyu Chen, chenkeyu@xmu.edu.cn