Introduction
Coastal waters are inherently dynamic due to river discharge, industrial effluents, shipping, dredging, waste dumping, and sewage disposal. Population growth in urban cities, climate change and variability, and changes in land-use practices all contribute to pressure on coastal water quality (Sekovski et al., 2012; Miller and Hutchins, 2017; Kumar et al., 2020; Vijay Prakash et al., 2021). Anthropogenic activity is evident around these estuaries and coastal and open ocean environments. Hence, it is important to assess the water quality on a regular basis and provide mitigation measures for coastal pollution (Yuvaraj et al., 2018). Improving water quality and variability in coastal waters is necessary and should be prioritized. Observational programs, which are more expensive and time-consuming, aid in understanding the status of water quality and its trends. Many countries have coastal programs that use predictive systems to inform the public and stakeholders about coastal health. Hydrodynamic processes are an integral part of complex surface water systems. The main factor that determines the concentration of pollutants is hydrodynamic transport, which includes advection, dispersion, vertical mixing, and convection (James, 2002). The flow and circulation patterns have a great influence not only on the distribution of temperature, nutrients, and dissolved oxygen (DO) but also on the aggregation and distribution of sediments and pollutants. When a load of pollutants is discharged into coastal waters, it is affected by the fate and transportation processes that change its concentration.
Several studies have been conducted to evaluate the coastal water quality spatiotemporally along the east coast of Indian coastal waters using site-specific data and model configuration (Panda et al., 2006; Bharathi et al., 2017; Naik et al., 2020; Mohanty et al., 2021). Through numerical modeling and remote sensing, estimation is user-friendly and low-cost in evaluating any water quality (Fallconer, 1993; Kageyama and Nishida, 2000; Brando and Dekker, 2003; El-Din et al., 2013); still, the field data are essential to validate the model and correct of ground value to the remote data. In situIn-situ sampling and laboratory analysis are more accurate than modeling and remote sensing data, while the remote sensing data provide better spatiotemporal resolution than the data obtained from in situ samplings (Miller and McKee, 2004; Wu et al., 2014), and numerical modeling infers spatiotemporal and water column information. However, satellite image data cannot consistently obtain the information due to the limitations of weather conditions, date of the pass, and its swath (Song et al., 2018); similarly, numerical modeling required accurate time series boundary information. Water temperature and salinity play a substantial role in regulating the processes in the aquatic system (Mogaddam et al., 2019). The physical and biological processes are under influence by little changes in salinity and temperature in the water column through land runoff (van Beusekom et al., 2009; Deininger et al., 2016), precipitation (Ackerman and Weisberg, 2003), and storm (Noble et al., 2003), which resulted in the coastal water quality and biomass such as diversity, local species composition, and bloom.
Knowing that the coastal environment is highly dynamic and ecologically complex, therefore, an integrated modeling system is required to address the coastal water quality issues. Currently, there is no real-time water quality information system for beach goers, tourists, and coastal stakeholders. Unless a site-specific model is set up to generate as per the coastal user’s requirement, there will be a scientific–societal gap in information. As a result, spatiotemporal collection of time series information is easy to evaluate with model-based water quality prediction. The predictive approach is basically followed by a three-step procedure that includes a) monitoring of various environmental conditions, b) simulation and observational validation of the model, and c) dissemination of the predictive information in the public domain. Therefore, the present study aimed to set up the coastal water quality model at the beach level, which simulates and forecasts the water quality as a public information system.
Study area
Chennai city, spreading over an area of 176 km2, is located on the western seaboard of the Bay of Bengal (BoB) and covers three major tourist beaches, viz., Marina, Elliot, and Thiruvanmiyur, along the east coast of India (Figure 1A). Two rivers, viz., Cooum and Adyar, flow crisscrossing the city, carrying a major share of domestic sewage, a part of this industrial, port, and harbor effluents into the coast are highly significant. To develop a model to achieve forecasting of coastal water quality, a comprehensive program was designed, which includes in situ measurements, the development of a model, and an information dissemination system.
Data and methods
The in situ monitoring stations were carefully located keeping in view of discharge points, off-tourist beaches, transition zones, and the surf zone dynamics. A total of 30 sampling stations were fixed (Figure 1A), among which three are at the river inlets, which act as point sources discharging urban runoff, 21 stations in nearshore waters, i.e., 1 km from the coast wherein the concentration of land inputs are apparent, and nine stations are at a distance of 2 km from the beaches where concentrations are significantly reduced (Mishra et al., 2015).
The in situ monitoring stations were strategically fixed to include discharge points, off-tourist beaches, transition zones, and the dynamics of the surf zone. The observations were made for the period 2013–2016, at above 30 locations and further up to 2019 at seven stations right on the beaches (Figure 1A). In each sampling, 11 water quality variables were collected such as water temperature, salinity, pH, DO, biological oxygen demand (BOD), nitrate, nitrite, total nitrogen, phosphate, total phosphorus, and chlorophyll-a, following standard protocols of seawater quality analysis (Strickland and Parsons, 1972). The discharges of all inlets were calculated using the change in water level, current speed and direction, and geomorphology of inlets. Hourly meteorology data from an automated weather station at Ennore (a coastal observatory) were used for model validation, while National Centre for Medium-Range Weather Forecast (NCMRWF) data were used to forecast water quality for the next 3 days. The model required coastal oceanography data such as tide and current for different seasons (2013 to 2015). The primary goals of oceanographic data collection have traditionally been to understand and describe the state of the coastal ocean and its variability, whereas the current study is primarily concerned with model forcing (input) and validation. The tide gauge (Valeport make) was used to collect sea-level changes (Tide), while the current meter was used to collect current data (RCM, Aanderaa make). A water quality buoy (WQB) mounted with YSI Exo-2 water quality sensors and MaxiMet meteorological sensors provide a 10-min interval of uninterrupted time series data. The data are transmitted on GSM mode and received through FTP. These time series (60-min interval) data have been used to understand the variability and validation of the model.
Model description
Water quality models have evolved into an important tool for identifying water environmental pollution, as well as the fate and transport of pollutants in the coastal water environments. With the advancement of model theory and the fast-updating computer technique, an increasing number of water quality models with various model algorithms have been developed. In the present study, a cell-centered finite volume method was adapted to compute the hydrodynamics with a very high resolution near the coast. As the river inlets are very fragile and more dynamic with siltation processes along this coast, hence continuous inlet monitoring (width) has been carried out for a couple of years, and an average width of river inlet is considered for model domain preparation (Figure 1B). An open equation solver water quality module (Ecolab) has been developed to model the fate of coastal waters at different spatiotemporal scales. Formulation of coastal water quality models requires fine-resolution bathymetry, proper initial conditions, boundary forcing, point discharge quantities, and water quality information (Supplementary Figure S1). The real-time observational data are assimilated into the models for better forecast results.
Three boundaries, viz., a) open ocean, b) point sources at the land interface, and c) atmospheric inputs, were configured in the model. The time series boundary condition for the open ocean is given from the regional ocean model reanalysis provided by Indian National Center for Ocean Information Services (INCOIS). The fluxes at point sources were computed from the observations, and the atmospheric forcing on forecast mode is given by the National Centre for Medium Range Weather Forecast (NCMRWF). A spatial varying bed resistance in terms of Chezy coefficient computed with respect to the depth profiles and coefficient for viscosity is 0.5 by Smagorinsky formulation (Supplementary Table 1). Various sensitivity analyses were carried out to develop an optimized model for the coastal waters off the Chennai coast.
Model skill and forecast accuracy
Proper model validation is highly necessary, while the output of the model is used as input for other models or real applications. The establishment of the model should be confirmed by agreement between observation and model output. In the present study, the correlation coefficient and root mean absolute error (RMAE) are used for the model skill test and the forecasting accuracy estimation, respectively. The correlation coefficient (r = Sxy/SxSy, where Sxand Syare the sample standard deviations and Sxyis the sample covariance) describes the degree of colinearity, and their range is 1 to −1, which is an index of the degree of learner relationship between observed and predicted values. When r = 1 or r = −1, a perfect positive and perfect negative relationship exists, while r = 0 indicates no linear relationship. The relative means absolute error (RMAE = ||P| − |O||/|P|; where P is the simulated value and O is the observed value) gives the relative error between the observed and predicted values and is extensively used to evaluate the numerical model performance. The accuracy of daily water quality forecasts was estimated through correlation coefficient by using the 10-min interval time series data from an automated sensor-based water quality buoy platform.
Results and discussion
Hydrodynamic
The hydrodynamic plays a key role in the variation of water quality in the nearshore region. The nearshore circulation along the east coast of India is mainly season-dependent with reversal wind, varying wave regimes, and river runoff (Shetye and Shenoi, 1988). The advection and dispersion along the coastal water are mainly subject to tidal action, wind, and river discharge. The advection and dispersion modules (particle tracking and Ecolab) coupled with hydrodynamic modeling were carried out for 15 days in each season, i.e., northeast monsoon (NEM: December 2013) and southwest monsoon (SWM: September 2015), and validated with observed data of sea-level changes (tide) and depth-averaged current (speed and direction).
The tidal analysis of the measured water level that clears the tide is micro (<2 m) and semi-diurnal. During December (NEM), the tidal range is ~1.25 m on a spring tide and 0.5 m on the neap phase, while it is ~1 m during spring and 0.5 m in the neap phase in September (SWM). The tide is classified as micro-tidal (Rao et al., 2009; Kankara et al., 2013). Though the rivers are small and semi-perennial, there is no significant variation of tidal amplitude throughout the domain. The harmonic analysis of measure tides generated 18 tidal constituents, out of which five important constituents are used in the prediction and validation (Supplementary Table S2). The dominant tidal constituents are principal lunar semidiurnal (M2), principal solar semidiurnal (S2), lunisolar declinational diurnal (K1), and principal lunar declinational diurnal (O1), which are used to calculate the tidal form number (F = O1 + K1/M2 + S2). The form number is <0.25, which describes the tide as mostly semi-diurnal. Figures 2A1 and B1 depict the validation of water levels for both seasons. The simulated amplitude and phase of tide are in agreement with the observations. The simulated current patterns (Supplementary Figure S2A, B) are in line with the East India Coastal Current (EICC), which is dominated by seasonal reversing. EICC is northward during SWM, while it is southward during NEM (Shetye et al., 1996; Babu et al., 2003; Gangopadhyay et al., 2013; Kankara et al., 2013). The validation of the current speed and direction for the NEM and SWM is shown in Supplementary Figure S2 (A2, B2, A3, B3), respectively. The observed current speed ranged from 0 to 0.38 and from 0 to 0.55 m/s during September and December, respectively, whereas the simulated speed ranged from 0 to 0.35 m/s in September and from 0 to 0.65 m/s in December. The current direction during December is varied from 200 to 280° and 200 to 218° for observation and modeling, respectively. The hydrodynamic model result indicates that the coastal current is primarily driven by wind, with no evidence of the tide’s sinusoidal nature. The combined action of northerly longshore current and micro tide triggers the inlet siltation and blocking along the coasts. The model has the potential to simulate hydrodynamics precisely.
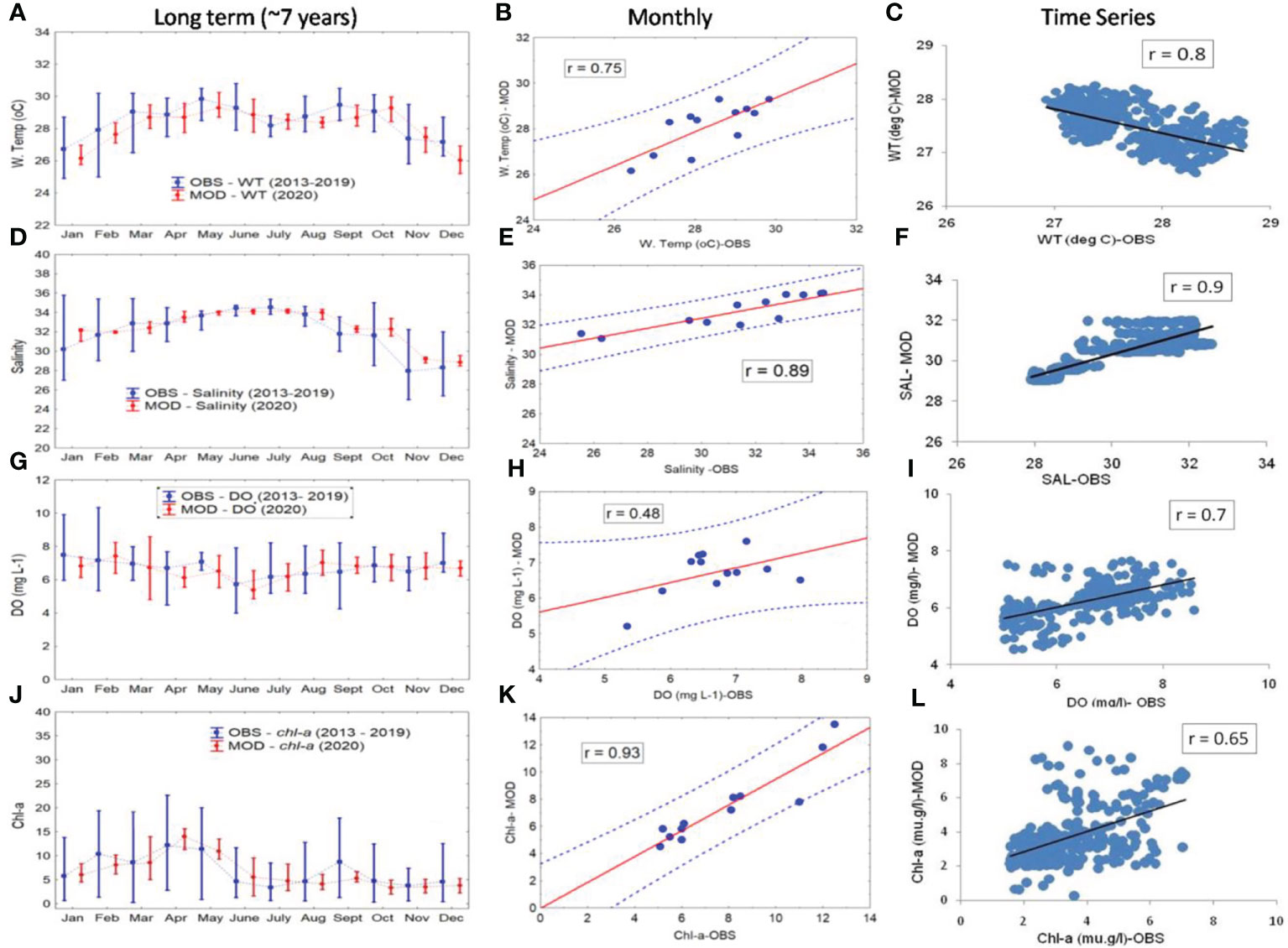
Figure 2 Validation of water temperature: (A) long term, (B) monthly, and (C) time series. Salinity: (D) long term, (E) monthly, and (F) time series. Dissolved oxygen: (G) long term, (H) monthly, and (I) time series. Chl-a: (J) long term, (K) monthly, and (L) time series.
Discharge
The accurate quantification of discharge at the point source, viz., Adyar and Cooum river inlets, is critical information because it directly affects coastal water quality and prediction. A current meter is placed in the mid of the inlet for 15 days. The cross-section and depth of the active inlet opening and water levels were considered to calculate the discharge values. For continuous-time series discharge information, the same data have been used in the model to develop probabilistic input of discharges calculated for a different season, tidal condition, and variable geomorphology of the inlets. The probabilistic inputs were calculated as d = a * d * v, where d is the discharge (m3/s), a is the cross-sectional area (m2), and v is the speed (m/s), while −ve discharge indicates discharge from sea to river during the flooding phase of the tide, and +ve discharge indicates discharge from the river to sea during the ebb phase of the tide. The coastal orientation (16°) is taken as considered in the discharge calculation. Supplementary Figure S3 shows the estimated discharge for Adyar and Cooum rivers. The higher discharged values from October to February are due to the major precipitations received during NEM over Chennai, which increase due to the increase in the land runoffs.
Water quality
The simulated water quality parameters at different spatiotemporal scales were generated for different seasons (Supplementary Figure S4) for water temperature, salinity, DO and Chl-a, and validated with the in situ measurements. The seasonal dispersion pattern of concentrations for distinct wind regimes, viz., NEM (northward) and SWM (southward), for the year 2020 agree with the wind pattern. This implies the coastal current is the driving factor for the fate and transport of pollutants along this coast. The higher concentration of all the parameters is nearly parallel to the coast and is intense near the river inlets (Supplementary Figure S4). Further, the dispersion depends on the local circulation pattern, which subsequently affects the adjacent coast.
Spatial variation of the Water Temperature (WT) is higher in coastal water compared to the deeper during SWM, while it reverses during NEM, because of the influence of variation in atmospheric temperature, land runoff, and land–sea interaction. The observed water temperature at the Marina nearshore region varied from 25.4°C to 30.9°C during December and April, respectively. The higher order of WT variation (~3.3°C) was recorded during the NEM compared to other seasons (Figure 2A). The maximum average WT (29.3°C to 29.8°C) was observed from the end of May to early September, while it is minimum (27°C to 28.6°C) from November to February. During the survey, the standard deviation of WT is 0.4°C to 1.1°C during March and December, respectively. The maximum diurnal variation in air temperature, rainfall, and a cloudy sky is the triggering factor in reducing the surface WT during the NEM period. The northeast monsoonal rain over Chennai brought huge fresh cold water to the nearshore region. The validation of WT was assessed by comparing observed (2013 to 2019) and model results (2020) on a monthly basis (Figure 2A) and found a very good agreement in trend. The WT trend is better fitting, while the average WT variation between the observed and model results is ±0.5°C. The quality of model fit was evaluated by calculating the correlation coefficient (r) by using the monthly average of the analyzed month. The correlation coefficient (r) is 0.75, and the model performance is very good (Figure 2B). Similarly, the correlation coefficient (r) between observed time series water quality buoy and simulated data of water temperature is 0.8 (Figure 2C).
The salinity off Marina beach ranges between 25 and 35.4. The highest salinity was recorded in July, while the lowest was recorded in December. The salinity structure of this coastal water increased from January to July and then decreased till December. Early research indicated that the EICC transports freshwater southward along India’s east coast beginning in September and that this freshwater plume reaches the extreme southern part of India in November (Sengupta et al., 2006; Akhil et al., 2014). The freshwater from the riverine system to the coastal water along India’s east coast is freshening up to 100 km from the coast, causing the salinity to decrease (Vinayachandran and Kurian, 2007). From June to September, monsoonal rain-derived freshwater is predominant in the extreme northern part of BoB, and the southern current pattern (November, December, and January) begins transporting freshwater southward along the shore (Shetye et al., 1996; Jensen, 2001; Rao, 2003). The southward freshwater plume through EICC and rainfall during the NEM are the most noticeable features of salinity variation along Chennai coastal water. As the vertical stratification began in early January along the east coast of the Indian nearshore waters, the average salinity increased from January to July (Shetye and Shenoi, 1988). The observed salinity trends are easily captured by regional modeling through a proper validation of coastal current. Figure 2D depicts that the model salinity trend (2020) is matching with the observed salinity trend (2013–2019). The correlation coefficient (r) of salinity (monthly average) is 0.9 (Figure 2E), and for time series, it is 0.85 (Figure 2F), and the performance of validation is excellent.
DO concentrations ranged from 4.1 to 10.5 mg/L during the annual cycle. There were no seasonal variations in water temperature or salinity. During the investigation, the average DO value ranged from 5.5 to 7.5 mg/L. High winds blow during the southwest monsoon, but DO is lower than in other seasons. The high DO concentration was recorded in January, which could be due to the higher photosynthetic rate and air–sea interaction (Carignan et al., 2000; Naik et al., 2020). From January onwards, the DO decreases steadily until June, when it slowly increases again until January. During the NEM period, the deviation of the DO value is high (January and February). A minimum variation in DO was observed (0.6 mg/L) during the validation plot between observed DO (2013 to 2019) and model DO (2020) (Figure 2G). Figure 2H shows a positive correlation between model and monthly average DO (r = 0.5), while the model DO versus time series observation (r = 0.7) was recorded (Figure 2I). The model results for DO show within the range. The variation of DO between model and observation is subjected to photosynthesis, local wind patterns, and urban discharge.
The Chl-a concentration in Chennai coastal water varied from 0.5 to 23.8 mg/m3. The maximum concentration was observed during April, while the minimum was in November. The Chl-a concentration is found to be higher during April, which might be due to the pre-monsoon convection process that brings nutrient-rich bottom water to the surface and or regenerated resources, which is responsible for this enhancement of the biological production (Jha et al., 2013; Naik et al., 2020). It follows an increasing trend from January to May and then started decreasing till November with an abrupt increase in September. A similar trend also reported for the Chl-a concentration along Puducherry coastal water is reported by Bharathi et al. (2017). The seasonal rainfall and cloudy days during November and December bring nutrient-rich freshwater to the coastal waters and reduced sunlight at the sea surface, which makes it more turbid and less productive and causes less Chl-a concentrations. Light conditions also play an important role in influencing the seasonal development of Chl-a (Yang and Ye, 2022). From January onwards, the existence of high water nutrients in coastal water will favor increasing the Chl-a concentration, and a similar study was also reported for Brahmani estuarine region by Palleyi et al. (2010). The observed average Chl-a value ranged from 3.5 to 13.4 mg/m3, while the model value ranged from 3.5 to 14.0 mg/m3 (Figure 2J). The validation for Chl-a between observed and model follows the same trend with an excellent correlation coefficient (r = 0.93) and a maximum deviation of ±0.75 mg/m3 (Figure 2K), while the correlation (r) between model and observed data in the time series is 0.63 (Figure 2L).The exercise confirms, with long-term validation, that the seasonal trends are well captured by the model and in short-term comparison agree with in situ observations.
Prediction performance
Predictive models of coastal water quality supplement traditional monitoring by providing timely and adequate information for public health protection and coastal ecosystem management. It is critical to choose an appropriate model type and metrics to reduce errors and ensure that the predicted outcome is reliable. The development of an optimized model is the result of intensive sampling to collect a sufficient amount of data, analysis and processing, sensitivity analyses, and model calibration. However, as prediction duration increases, the uncertainty in the forcing parameters increases, resulting in a wide variation in the forecast range (Counillon et al., 2021; Džal et al., 2021). The model in this study simulates up to 8 days in advance, but the RMSE skill scores are acceptable for up to 3 days after which the ranges widen (Supplementary Figures S5A–D). The forecast skills are varied when considering individual parameters. RMSE skill scores for water temperature, salinity, DO, and Chl-a follow similar trends. The bias error is significantly increasing from the fifth day onwards; therefore, the forecast is considered for up to 3 days.
Conclusion
Coastal water quality modeling is of great environmental interest and scientific value and is an efficient tool for developing water quality information for beachgoers, stakeholders, and coastal managers. A coupled hydrodynamic-water quality model was setup to simulate and forecast coastal water quality parameters. The in situ measured data and sensor-based time series data were used to calibrate and validate the model. The skill test confirms that the model performance for hydrodynamics and water quality are in the acceptable ranges up to 3 days of forecast. Sensor-based data assimilation, high frequency of point source quantities, estimation of non-point source components, and data assimilation in the model will improve forecast accuracy. This paper attempted to predict the coastal water quality, which is highly dynamic and complex and paves the way for future improvements. The data generated assist beachgoers and coastal stakeholders during any abnormalities in water quality. This is an imperative study to achieve the targets under United Nations’ Sustainable Development Goal (SDG)-14 and will assist coastal managers in the sustainable management of coastal regions.
Data availability statement
The original contributions presented in the study are included in the article/Supplementary Material. Further inquiries can be directed to the corresponding author.
Ethics statement
All applicable international, national, and/or institutional guidelines for the care and use of animals were followed by the authors.
Author contributions
UKP: fieldwork, data analysis, modeling, and writing original draft. USP: review, modeling, and manuscript writing. SN: fieldwork, literature review, data analysis, and interpretation. PM: final draft and supervision. DK: model data analysis. MB: review and data interpretation. MVR: review and project administration. All authors revised and edited the manuscript. All authors contributed to the article and approved the submitted version.
Acknowledgments
The authors are grateful to The Secretary, Ministry of Earth Sciences (MoES), Government of India, for his continuous support and encouragement. This manuscript has an NCCR contribution no. NCCR/MS 388.
Conflict of interest
The authors declare that the research was conducted in the absence of any commercial or financial relationships that could be construed as a potential conflict of interest.
Publisher’s note
All claims expressed in this article are solely those of the authors and do not necessarily represent those of their affiliated organizations, or those of the publisher, the editors and the reviewers. Any product that may be evaluated in this article, or claim that may be made by its manufacturer, is not guaranteed or endorsed by the publisher.
Supplementary material
The Supplementary Material for this article can be found online at: https://www.frontiersin.org/articles/10.3389/fmars.2022.987067/full#supplementary-material
References
Ackerman D., Weisberg S. B. (2003). Relationship between rainfall and beach bacterial concentrations on Santa Monica bay beaches. J. Water Health 1, 85–89. doi: 10.2166/wh.2003.0010
Akhil V. P., Durand F., Lengaigne M., Vialard J., Keerthi M. G., Gopalakrishna V. V., et al. (2014). A modeling study of the processes of surface salinity seasonal cycle in the bay of Bengal. J. Geophys. Res. Ocean. 119, 3926–3947. doi: 10.1002/2013jc009632
Babu M. T., Sarma Y. V. B., Murty V. S. N., Vethamony P. (2003). On the circulation in the bay of Bengal during northern spring inter-monsoon. Deep Sea Res. Part {II} Top. Stud. Oceanogr. 50, 855–865. doi: 10.1016/s0967-0645(02)00609-4
Bharathi M. D., Patra S., Sundaramoorthy S., Madeswaran P., Sundaramanickam A. (2017). Elucidation of seasonal variations of physicochemical and biological parameters with statistical analysis methods in puducherry coastal waters. Mar. pollut. Bull. 122, 432–440. doi: 10.1016/j.marpolbul.2017.05.048
Brando V. E., Dekker A. G. (2003). Satellite hyperspectral remote sensing for estimating estuarine and coastal water quality. IEEE Trans. Geosci. Remote Sens. 41, 1378–1387. doi: 10.1109/tgrs.2003.812907
Carignan R., Planas D., Vis C. (2000). Planktonic production and respiration in oligotrophic Shield lakes. Limnol. Oceanogr. 45, 189–199. doi: 10.4319/lo.2000.45.1.0189
Counillon F., Keenlyside N., Toniazzo T., Koseki S., Demissie T., Bethke I., et al. (2021). Relating model bias and prediction skill in the equatorial Atlantic. Clim. Dyn. 56, 2617–2630. doi: 10.1007/s00382-020-05605-8
Deininger A., Faithfull C. L., Lange K., Bayer T., Vidussi F., Liess A. (2016). Simulated terrestrial runoff triggered a phytoplankton succession and changed seston stoichiometry in coastal lagoon mesocosms. Mar. Environ. Res. 119, 40–50. doi: 10.1016/j.marenvres.2016.05.001
Džal D., Kosović I. N., Mastelić T., Ivanković D., Puljak T., Jozić S. (2021). Modelling bathing water quality using official monitoring data. Water 13, 3005. doi: 10.3390/w13213005
El-Din M. S., Gaber h., Koch M., Ahmed R. S., Bahgat I. (2013). Remote sensing application for water quality assessment in lake timsah, Suez canal, Egypt. J. Remote Sens. Technol. 1(3), 61–74. doi: 10.18005/jrst0103002
Fallconer R. A. (1993). Application of numerical models for water quality studies. Proc. Inst. Civ. Eng. - Civ. Eng. 97, 163–170. doi: 10.1680/icien.1993.25304
Gangopadhyay A., Raj G. N. B., Chaudhuri A. H., Babu M. T., Sengupta D. (2013). On the nature of meandering of the springtime western boundary current in the bay of Bengal. Geophys. Res. Lett. 40, 2188–2193. doi: 10.1002/grl.50412
James I. D. (2002). Modelling pollution dispersion, the ecosystem and water quality in coastal waters: A review. Environ. Model. Software 17, 363–385. doi: 10.1016/S1364-8152(01)00080-9
Jensen T. G. (2001). Arabian Sea And bay of Bengal exchange of salt and tracers in an ocean model. Geophys. Res. Lett. 28, 3967–3970. doi: 10.1029/2001gl013422
Jha D. K., Prashanthi Devi M., Vinithkumar N. V., Das A. K., Dheenan P. S., Venkateshwaran P., et al. (2013). Comparative investigation of water quality parameters of aerial & rangat bay, Andaman islands using in-situ measurements and spatial modelling techniques. Water Qual. Expo. Heal. 5, 57–67. doi: 10.1007/s12403-013-0086-2
Kageyama Y., Nishida M. (2000). Water quality analysis based on remote sensing data and numerical model. J. Geogr. (Chigaku Zasshi) 109, 27–36. doi: 10.5026/jgeography.109.27
Kankara R. S., Mohan R., Venkatachalapathy R. (2013). Hydrodynamic modelling of chennai coast from a coastal zone management perspective. J. Coast. Res. 29, 347–357. doi: 10.2307/23353632
Kumar S. S., Panda U. S., Pradhan U. K., Mishra P., Murthy M. V. R. (2020). Web-based decision support system for coastal water quality. J. Coast. Res. 89, 139–144. doi: 10.2112/SI89-023.1
Miller J. D., Hutchins M. (2017). The impacts of urbanisation and climate change on urban flooding and urban water quality: A review of the evidence concerning the united kingdom. J. Hydrol. Reg. Stud. 12, 345–362. doi: 10.1016/j.ejrh.2017.06.006
Miller R. L., McKee B. A. (2004). Using MODIS Terra 250 m imagery to map concentrations of total suspended matter in coastal waters. Remote Sens. Environ. 93, 259–266. doi: 10.1016/j.rse.2004.07.012
Mishra P., Panda U. S., Pradhan U., Kumar C. S., Naik S., Begum M., et al. (2015). Coastal water quality monitoring and modelling off chennai city. Proc. Eng. 116, 955–962. doi: 10.1016/j.proeng.2015.08.386
Mogaddam S. F., Bidokhti A. A., Givi F. A., Ezam M. (2019). Evaluation of physical changes (temperature and salinity) in the Persian gulf waters due to climate change using field data and numerical modeling. Int. J. Environ. Sci. Technol. 17, 2141–2152. doi: 10.1007/s13762-019-02532-y
Mohanty A. K., Sathishkumar R. S., Sahu G., Suriyaprakash R., Arunachalam K. D., Venkatesan R. (2021). Spatial and seasonal variations in coastal water characteristics at kalpakkam, western bay of Bengal, southeast India: a multivariate statistical approach. Environ. Monit. Assess. 193, 366. doi: 10.1007/s10661-021-09115-w
Naik S., Mishra R. K., Sahu K. C., Lotliker A. A., Panda U. S., Mishra P. (2020). Monsoonal influence and variability of water quality, phytoplankton biomass in the tropical coastal waters – a multivariate statistical approach. Front. Mar. Sci. 7. doi: 10.3389/fmars.2020.00648
Noble R. T., Weisberg S. B., Leecaster M. K., McGee C. D., Dorsey J. H., Vainik P., et al. (2003). Storm effects on regional beach water quality along the southern California shoreline. J. Water Health 1, 23–31. doi: 10.2166/wh.2003.0004
Palleyi S., Kar R. N., Panda C. R. (2010). Seasonal variability of phytoplankton population in the brahmani estuary of orissa, India. J. Appl. Sci. Environ. Manage. 12, 19–23. doi: 10.4314/jasem.v12i3.55474
Panda U., Sundaray S., Rath P., Nayak B., Bhatta D. (2006). Application of factor and cluster analysis for characterization of river and estuarine water systems – a case study: Mahanadi river (India). J. Hydrol. 331, 434–445. doi: 10.1016/j.jhydrol.2006.05.029
Rao R. R. (2003). Seasonal variability of sea surface salinity and salt budget of the mixed layer of the north Indian ocean. J. Geophys. Res. 108, 3009. doi: 10.1029/2001jc000907
Rao V. R., Murthy M. V. R., Bhat M., Reddy N. T. (2009). Littoral sediment transport and shoreline changes along ennore on the southeast coast of India: Field observations and numerical modeling. Geomorphology 112, 158–166. doi: 10.1016/j.geomorph.2009.05.015
Sekovski I., Newton A., Dennison W. C. (2012). Megacities in the coastal zone: Using a driver-pressure-state-impact-response framework to address complex environmental problems. Estuar. Coast. Shelf Sci. 96, 48–59. doi: 10.1016/j.ecss.2011.07.011
Sengupta D., Raj G. N. B., Shenoi S. S. C. (2006). Surface freshwater from bay of Bengal runoff and Indonesian throughflow in the tropical Indian ocean. Geophys. Res. Lett. 33, L22609. doi: 10.1029/2006gl027573
Shetye S. R., Gouveia A. D., Shankar D., Shenoi S. S. C., Vinayachandran P. N., Sundar D., et al. (1996). Hydrography and circulation in the western bay of Bengal during the northeast monsoon. J. Geophys. Res. Ocean. 101, 14011–14025. doi: 10.1029/95jc03307
Shetye S. R., Shenoi S. S. C. (1988). Seasonal cycle of surface circulation in the coastal north Indian ocean. Proc. Indian Acad. Sci. - Earth Planet. Sci. 97, 53–62. doi: 10.1007/BF02861627
Song N., Wang N., Lin W., Wu N. (2018). Using satellite remote sensing and numerical modelling for the monitoring of suspended particulate matter concentration during reclamation construction at dalian offshore airport in China. Eur. J. Remote Sens. 51, 878–888. doi: 10.1080/22797254.2018.1498301
Strickland J. D. H., Parsons T. R. (1972). A practical handbook of seawater analysis, issue 167; issue 1977 (Fisheries Research Board of Canada, Ottawa).
van Beusekom J. E. E., Loebl M., Martens P. (2009). Distant riverine nutrient supply and local temperature drive the long-term phytoplankton development in a temperate coastal basin. J. Sea Res. 61, 26–33. doi: 10.1016/j.seares.2008.06.005
Vijay Prakash K., Geetha Vimala C. S., Preethi Latha T., Jayaram C., Nagamani P. V., Laxmi C. N. V. (2021). Assessment of water quality along the southeast coast of India during COVID-19 lockdown. Front. Mar. Sci. 8. doi: 10.3389/fmars.2021.659686
Vinayachandran P. N., Kurian J. (2007). Hydrographic observations and model simulation of the bay of Bengal freshwater plume. Deep Sea Res. Part I Oceanogr. Res. Pap. 54, 471–486. doi: 10.1016/j.dsr.2007.01.007
Wu G., Liu L., Chen F., Fei T. (2014). Developing {MODIS}-based retrieval models of suspended particulate matter concentration in dongting lake, China. Int. J. Appl. Earth Obs. Geoinf. 32, 46–53. doi: 10.1016/j.jag.2014.03.025
Yang C., Ye H. (2014). Enhanced Chlorophyll-a in the coastal waters near the Eastern Guangdong during the Downwelling Favorable Wind Period. Remote Sens. 14, 1138. doi: 10.3390/rs14051138
Keywords: coastal water quality, hydrodynamics, modelling, prediction, chennai
Citation: Pradhan U, Panda US, Naik S, Mishra P, Kumar DS, Begum M and Murthy MVR (2022) Coupled hydrodynamic and water quality modeling in the coastal waters off Chennai, East Coast of India. Front. Mar. Sci. 9:987067. doi: 10.3389/fmars.2022.987067
Received: 05 July 2022; Accepted: 01 August 2022;
Published: 26 August 2022.
Edited by:
Dilip Kumar Jha, National Institute of Ocean Technology, IndiaReviewed by:
Thilagam Harikrishnan, Pachaiyappa’s College for Men, IndiaRajamanickam Krishnamurthy, Arignar Anna Government Arts and Science College Chennai, India
Copyright © 2022 Pradhan, Panda, Naik, Mishra, Kumar, Begum and Murthy. This is an open-access article distributed under the terms of the Creative Commons Attribution License (CC BY). The use, distribution or reproduction in other forums is permitted, provided the original author(s) and the copyright owner(s) are credited and that the original publication in this journal is cited, in accordance with accepted academic practice. No use, distribution or reproduction is permitted which does not comply with these terms.
*Correspondence: Uma Sankar Panda, dXNwYW5kYUBuY2NyLmdvdi5pbg==