- 1Faculty of Science and Engineering, Swansea University, Swansea, United Kingdom
- 2Project Seagrass, Bridgend, United Kingdom
Coastal ecosystems, including coral reefs, mangroves, and seagrass, are in global decline. Mitigation approaches include restoration and other managed recovery interventions. To maximise success, these should be guided by an understanding of the environmental niche and geographic limits of foundational species. However, the choices of data, variables, and modelling approaches can be bewildering when embarking on such an exercise, and the biases associated with such choices are often unknown. We reviewed the current available knowledge on methodological approaches and environmental variables used to model and map habitat suitability for coastal ecosystems. While our focus is on seagrass, we draw on information from all marine macrophyte studies for greater coverage of approaches at different scales around the world. We collated 75 publications, of which 35 included seagrasses. Out of all the publications, we found the most commonly used predictor variables were temperature (64%), bathymetry (61%), light availability (49%), and salinity (49%), respectively. The same predictor variables were also commonly used in the 35 seagrass Habitat Suitability Models (HSM) but in the following order: bathymetry (74%), salinity (57%), light availability (51%), and temperature (51%). The most popular method used in marine macrophyte HSMs was an ensemble of models (29%) followed by MaxEnt (17%). Cross-validation was the most commonly used selection procedure (24%), and threshold probability was the favoured model validation (33%). Most studies (87%) did not calculate or report uncertainty measures. The approach used to create an HSM was found to vary by location and scale of the study. Based upon previous studies, it can be suggested that the best approach for seagrass HSM would be to use an ensemble of models, including MaxEnt along with a selection procedure (Cross-validation) and threshold probability to validate the model with the use of uncertainty measures in the model process.
Introduction
To manage environmental changes and aid conservation of biodiversity, it is vital that we increase our understanding of the relationships between species distribution and their surrounding environment (Bellamy et al., 2013; Matthiopoulos et al., 2020). However, there is often a lack of systematic biological survey data available (Elith et al., 2011) making it impossible to account for all individuals of a species at all times. Recent economic crises have seen major cuts in government spending with environmental protection agencies one of the sectors to suffer, exacerbating this lack of systematic biological data collection (Borja & Elliott, 2013). Habitat suitability models (HSMs) and species distribution models (SDMs) can be used to predict the likelihood of species occurrence based on an understanding of the environmental variables that determine species distribution (Hirzel & Le Lay, 2008; Elith et al., 2011), so that understanding of species-habitat associations can be developed even when biological datasets are limited. HSMs are relied upon for species reintroduction (Adhikari et al., 2012), species management (Vinagre et al., 2006) and restoration projects (Barnes et al., 2007) and can be developed without the need for comprehensive biological datasets. More recently these models have been used to forecast the likely responses to future disturbances and climate change (Jueterbock et al., 2016; Davis et al., 2021), including changes in temperature, ocean acidification and sea level (Convertino et al., 2012; Valle et al., 2014; Wesselmann et al., 2021).
Global decline, resulting from multiple, often synergistic pressures is particularly acute in coastal ecosystems, including coral reefs, mangroves, salt marshes, and seagrass (Waycott et al., 2009; Silliman, 2014; Spivak et al., 2019; Stafford et al., 2021). Typical of these, seagrasses are distributed globally and are among the most ecologically valuable habitats, providing numerous ecosystem services, including carbon sequestration, sediment stabilisation, support for biodiversity, nursery grounds for commercial species, and water purification (Fourqurean et al., 2012; Nordlund et al., 2016; Kerr, 2017; Röhr et al., 2018, Unsworth et al., 2019b) yet these ecosystems are in global decline and face threats from numerous natural and anthropogenic factors (Waycott et al., 2009).
To combat the impacts of coastal ecosystem decline, restoration efforts are increasing in the marine environment, especially of coral reef, mangrove, but also seagrass habitats (Kairo et al., 2009; Guarnieri et al., 2019; Gamble et al., 2021). Seagrass restoration projects have been attempted globally but with varying degrees of success (Marion & Orth, 2010; van Katwijk et al., 2016; Unsworth et al., 2019a; Tan et al., 2020), partly due to the dynamic environment seagrasses are often found to grow (McDonald et al., 2016). Large-scale restoration efforts lead to more effective results with a higher proportion of seagrass survival and increases in population growth rate (van Katwijk et al., 2016). Furthermore, ecosystem services have been seen to return following seagrass restoration (Reynolds et al., 2016), in particular where this has been achieved at scale (Orth et al., 2020). However, the use of HSM for informing the restoration of habitats is relatively unexplored, with the majority of marine studies until recently focused on theoretical ecology, movement of non-native species, conservation planning and climate change (Robinson et al., 2017). There is, therefore, the need to understand the environmental niche required for successful seagrass restoration, in order to map that onto suitable habitat across multiple locations which is the motivation of this review of wider marine macrophyte habitat suitability modelling approaches.
The most important variables in predicting habitat suitability can be influenced by the scale of the study, for example, Valle et al. (2013) found that wave exposure and current velocity were the key variables predicting Zostera marina distribution when using broadly distributed data, whereas slope and depth were important at predicting species distribution of conservative presence areas. This will have implications for the creation of HSMs for informing restoration and may require the need for different models and variables dependent on the scale, local topography, and location in question.
For the context of seagrass restoration, it should be considered that the environmental requirements for seeds to germinate and seedlings to be able to establish may differ from those of established mature plants that are able to reduce water-flow rates, sediment resuspension and have considerable anchorage within sediments (Maxwell et al., 2016). This difference in environmental requirements may also be the case for transplanted mature shoots. The feedback mechanism that occurs within an established meadow can improve localized conditions for plant growth and for new seedlings to establish. This will be dependent upon meadow characteristics such as shoot density, with sparse and patchy areas having little effect on stabilizing conditions (Maxwell et al., 2016). These factors could have significant effects on successful seagrass restoration. The use of presence data over the range of environmental gradients seagrasses are found is therefore likely to give broader suitability outputs in HSMs. It may also be beneficial to integrate experimental (flume) knowledge into HSM on the physical stability of individual seedlings and plants in varied environments. The availability of fine-scale hydrodynamic data would prove extremely useful in focusing planting efforts.
The aim of this paper was to review the development and use of HSMs in marine macrophyte ecosystems in order to optimize the use of HSM on seagrasses for facilitating restoration. Our specific objectives were to i) interrogate the current body of research using HSM for marine macrophytes, ii) review the selection of appropriate environmental predictor variables, and iii) review the statistical modelling approach used to draw the species-habitat association (including choice of algorithm for model parameterization, model selection (relative goodness-of-fit), and model validation (absolute goodness-of-fit)).
Methods - Literature review of macrophyte HSM studies
We collated data on studies using HSMs to determine the distribution of marine macrophytes. A recent systematic review by Robinson et al. (2017) assembled papers of marine HSM studies published between 1950 and 26 July 2016. Publications from Robinson et al. (2017) that contained marine macrophytes were included in our review and a further search for publications with keywords ‘marine macrophyte’+’macroalgae’+’ seagrass’+’HSM’+’SDM’ was performed using ISI Web of Science (http://apps.webofknowledge.com/) and Google Scholar (https://scholar.google.co.uk/) for papers published between 2016 and 20 Jan 2022. We identified 75 publications, 35 of which were on or included seagrass.
For each of the publications identified, the main factors of interest were extracted into a database, based upon the methods outlined by Robinson et al. (2017). These factors included geographical area and scale of study, taxa, species, approach to modelling, model method (algorithm, selection and validation) and environmental variables used (Supplementary Data, Table S1).
This database was used to cross-examine trends in studies and to elucidate methods currently in use for carrying out HSM.
Results
HSM studies of marine macrophytes dated back to 2007, with seagrass studies beginning in 2009. Although initially there were more macroalgae HSM studies than seagrass there has been a marked increase in seagrass HSM publications since 2016 (77% of published in 2016 and since) so that seagrass studies are more equal (Figure 1). Just over half of macrophyte HSM papers studied multiple species (51%), whereas seagrass studies appear to be more species focused, with 31% covering multiple species. Seagrass species targeted for studies so far, include, Z. marina (Canal-Vergés et al., 2016; Beca-Carretero et al., 2020), Z. noltei (Valle et al., 2011; Valle et al., 2014), Z. japonica (Shafer et al., 2016), Posidonia oceanica (Bakirman & Gumusay, 2020; Catucci & Scardi, 2020), Cymodocea nodosa (Chefaoui et al., 2016), Enhalus acoroides (Lanuru et al., 2018) and Halophila stipulacea (Wesselmann et al., 2021).
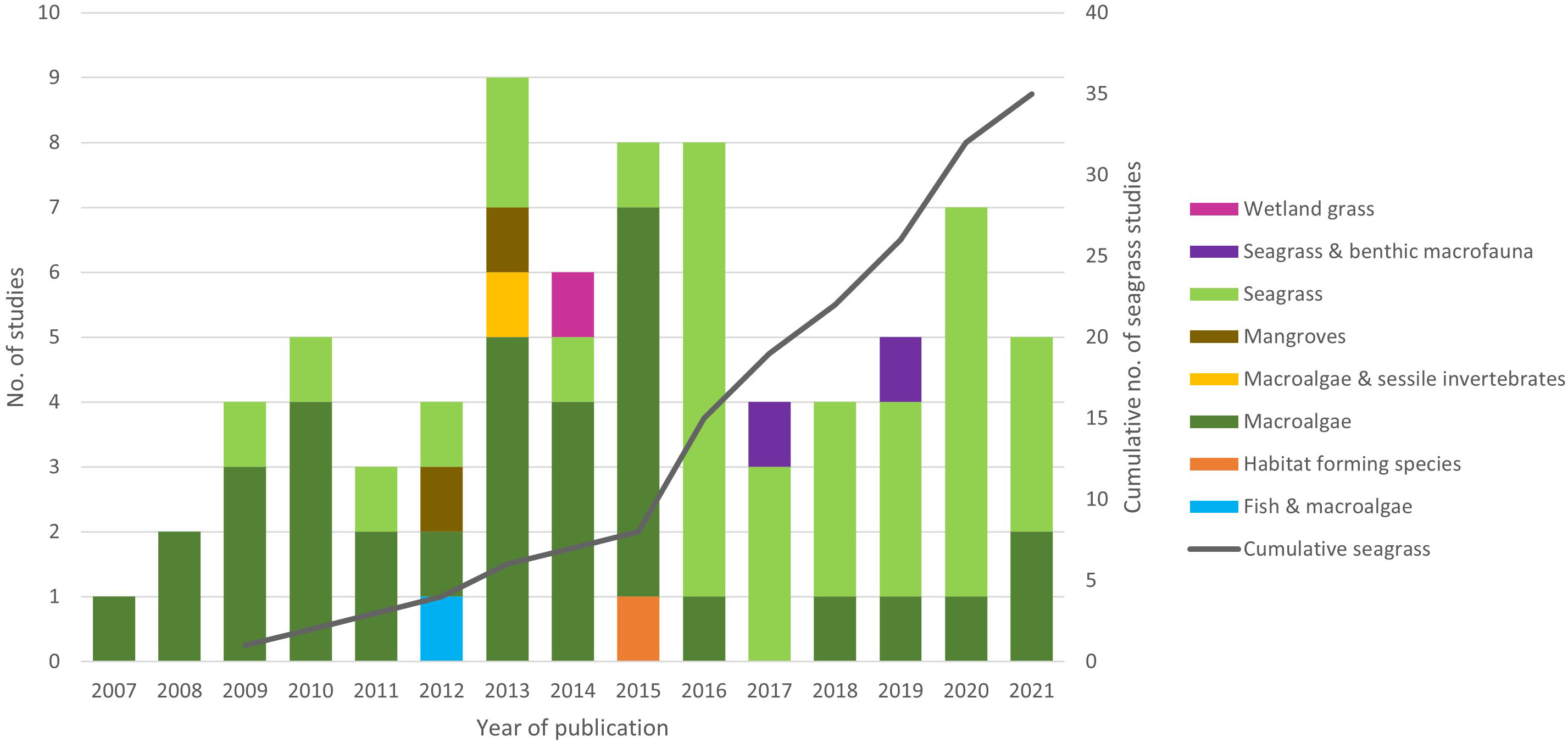
Figure 1 Trends in taxa used as focus within marine macrophyte HSM studies with year of publication and cumulative number of seagrass studies on secondary y axis.
The vast majority of HSM studies on macrophytes have been carried out in the temperate northern Atlantic region (48%), the majority of which on macroalgae then seagrasses. This is followed by temperate Australasia (12%) where macroalgae are also dominant above seagrass studies, and central Indo-Pacific (11%) where seagrass is the dominant taxa followed by mangroves (Figure 2).
From the 75 marine macrophyte studies, temperature (usually sea surface, SST) was the most frequently used predictor variable (64%), followed by bathymetry (61%), light availability (4 9%), and salinity (49%). When filtered to only seagrass studies (n=35), bathymetry was most frequently included (74%), followed by salinity (57%), light availability (51%), and temperature (51%). Substrate, wave energy and slope were the next most utilized variables in seagrass HSMs (Figure 3).
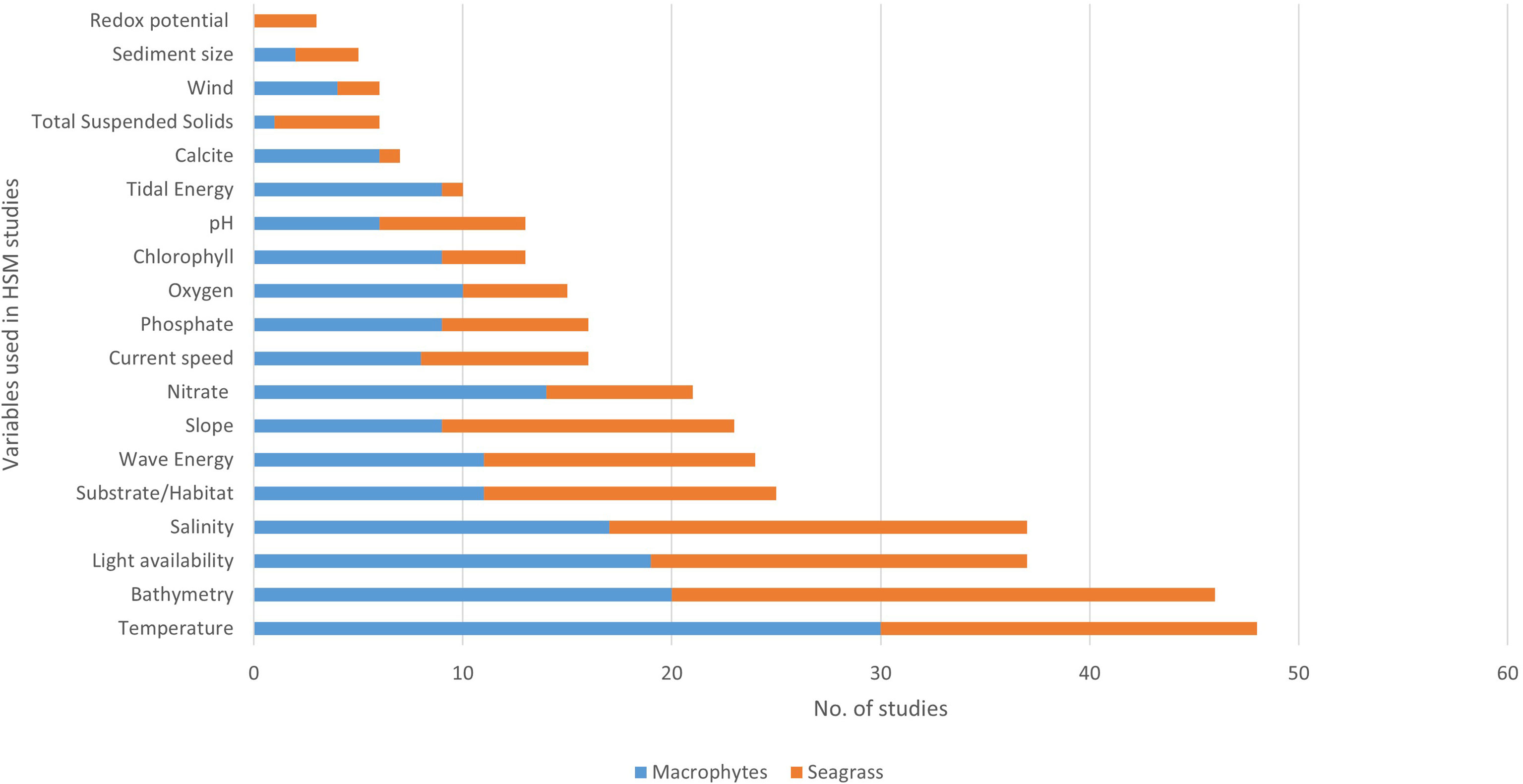
Figure 3 Most common environmental variables used in macrophyte HSM based on literature review. Data are divided into number from seagrass HSM (orange) and other macrophyte HSM (blue).
In our review, we found a total of 18 algorithms had been used. An ensemble of algorithms was the most dominant method and was used in 22 publications (29%), followed by Maximum entropy (MaxEnt) (n = 15; 20%), and Generalized Additive Models (GAMs), Generalized Linear Models (GLMs) and GIS based algorithms, which were each used in 7-8 publications (9-11%) (see Supplementary Data; Table S1). Alternatively, 27% of the algorithms were used just one time (e.g. Welch’s test, Cellular automata, and Production-environmental suitability model). An ensemble was also the most popular in seagrass HSM publications, with 5 publications using two or more models.
Of the studies found within the review, the majority (40%) were focused on a local scale, covering a relatively small area such as an individual bay or estuary, followed by regional scale studies covering larger areas, such as a whole country or sea (30.7%). 16% of studies were over an intermediate area or scale (>100km coastline), and only 8% at a global scale. Ensemble was found to be used at a most scales; local, intermediate and regional, but not in any of the global studies. MaxEnt was more popular for larger scale studies including majority of global studies (66.7%), and regional (26%), but only 3 (9.7%) at a local scale. Local studies utilized the same environmental variables overall, with temperature, salinity (both 23%) and bathymetry (22.7%) the most commonly used followed by light (20%), substrate (16%) and wave energy (13%).
There are a total of 11 selection procedures present from the 75 publications. The most popular procedures were cross-validation, variable contribution and stepwise (n = 11, 9, and 8 publications respectively). Cross-validation was also the selection procedure most used in the seagrass HSM publications. When an ensemble of algorithms was used the most popular selection procedure was Cross-validation (29%), yet when the MaxEnt algorithm was used, variable contribution was the most commonly used selection procedure (86%).
Seven model validations were identified in the review of marine macrophyte HSM papers (Chi-squared, Cross-validation, Independent dataset, Markov Chain Monte Carlo, Multimodel inference, Regression methods and Threshold probability); the most popular was Threshold probability (33%). Just 9% of all marine macrophyte HSM publications calculated uncertainty measures, mainly through predictive power.
Discussion
This review shows the increase in trend for using HSM for marine macrophytes, particularly seagrasses in more recent years (since 2016). As a tool HSM is recognized as a viable option for interpolating spatial data for predicting where environmental conditions are conducive to habitat forming species such as seagrasses, macrophytes and mangroves. This information clearly has uses for informing restoration work, although this is still new to the field and as of yet, little evidence can be found to show its actual efficacy. Nonetheless, HSM is a useful tool for focusing efforts of restoration which can be a labor intensive and expensive to carry out.
Predictor (environmental) variables and selection
The majority of studies used over 5 predictor variables, although this ranged from only 1 to over 16 factors (Fabbrizzi et al., 2020). However, if too many environmental parameters are added to an HSM it can lead to over or underfitting a model, therefore, it has been suggested that species presences in a model should be 10 X greater than the number of environmental predictors (Singer et al., 2017). The variables that are used most are not necessarily the most important for determining species presence but could be more commonly used due to data availability. For example, years of climate modelling has refined models used to forecast factors such as sea surface, seabed temperature and salinities at a global scale. The most popular variable used in macrophyte HSM was temperature, but when only seagrass studies were looked at, bathymetry became the most popular. The majority of seagrass studies focused on a single species, with Z. marina the most common. This species has wide geographical range and can tolerate a range of temperatures and salinities (Blok et al., 2018). At a regional scale, temperature is not likely to fluctuate greatly and so is arguably not as important as other parameters such as light availability (Abe et al., 2003; Bertelli & Unsworth, 2018). However, localized studies utilized similar environmental variables as the overall average of studies, with few using fine-scale hydrodynamic data for example. The importance of this data is highlighted by Valle et al. (2013), which found current velocity to be the most important variable in determining Z. marina distributions within the Ems estuary (Netherlands) in the Wadden Sea, followed by wave exposure and depth. Good environmental data availability is key to providing the data needed for HSMs to run successfully.
It is suggested that including environmental variables that correlate may impede the performance and interpretation of the study (Georgian et al., 2019). Therefore, the removal of correlated variables is a valid method for assisting the selection of model parameters. For MaxEnt, there is a selection criteria called ‘MaxEntVariableSelection’ which could be used to increase the performance of the model by detecting the most important environmental variables that are not correlated (Jueterbock et al., 2016). The removal of correlated variables is always recommended for variable selection to improve model functionality which can easily be achieved using VIF (Variance Inflation Factor) tests (vifstep and vifcor) in R, (Guisan et al., 2017).
Model algorithm
HSM approaches are varied across studies and models have been designed for different types of data. For example, there are specific models in place for presence-only data such as Ecological Niche Factor Analysis (ENFA) (Valle et al., 2011), MaxEnt (Yesson et al., 2015), and Relative Environmental Suitability (RES) (Davis et al., 2016); and for presence-absence data, including Generalized Linear Models (GLMs) (Adams et al., 2016), and Generalized Additive Models (GAMs) (Nyström Sandman et al., 2013). However, if reliable absence data is not available for a species some studies and methods allow the creation of pseudo-absences or background points to use in their models (Chefaoui et al., 2016).
Various algorithms have been tested to model seagrass (Z. marina) distribution at a local scale, including Machine learning methods; Boosted Regression Trees (BRT), MaxEnt, Artificial Neural Networks (ANN), Random Forest (RF), and Regression-based models; GAMs, GLMs and Multivariate Adaptive Regression Spline (MARS) (Valle et al., 2013). The models used were a mix of presence-absence and presence-only models. Valle et al. (2013) compared these algorithms with presence-only data and pseudo-absences which were created in areas never occupied to avoid overlap with presence data. In this study machine learning methods were found to perform better than regression-based models. However, according to the main findings from comparative studies (listed in chapter 11, Guisan et al., 2017), it is suggested that machine learning methods do not provide better results than regression based models, but can be represented in a more informative format and reveal other properties not always available from other approaches (Guisan et al., 2017). It must be taken into account that when using machine learning approaches, that they are calculated numerous times as outcomes will differ each time, unlike GLM, ENFA or BIOCLIM that will give the same result for the same species presence datasets (Sillero & Barbosa, 2021).
Ensemble models have been shown to perform better than individual models and are a way of avoiding dependence on a single type of model (Georgian et al., 2019). Chefaoui et al., 2016 used pseudo-absence data and an ensemble of six models for presence-absence data. The six presence-absence models were GLM, GAM, GBM (Generalized Boosting Model), RF, MARS, and FDA (Flexible Discriminant Analysis). GAM was most accurate at predicting presences compared to the presence-only MD (Mahalanobis Distance) model used for comparison. MaxEnt was the second most popular algorithm to be used in the reviewed marine macrophyte HSMs (Verbruggen et al., 2009; Tyberghein et al., 2012; Jueterbock et al., 2013; Verbruggen et al., 2013; Gormley et al., 2015; Yesson et al., 2015; Jayathilake & Costello, 2018). MaxEnt, based on maximum entropy, has been found to outperform many other models (Valle et al., 2014). The principle of maximum entropy is that, allowing for constraints, the best probability representation of species distribution that best represents the data is the one with the greatest entropy, i.e. the one which best reproduces the data (Guisan et al., 2017). There is a standalone MaxEnt package available on Java platform which is freely accessible for download with a user-friendly interface (Paquit et al., 2017). This program models habitat suitability from presence-only data (Elith et al., 2011). MaxEnt has also been written into a number of SDM packages in R including ‘sdm’, enabling it to be run from R.
Many marine HSM papers do not report uncertainty measures but it has been suggested that all HSMs should be accounting for uncertainty levels or estimates of errors as part of the process (Robinson et al., 2017).
Conclusions
There is no set approach used to model habitat suitability of marine macrophytes. Various sources are available to download marine species presence data and it is recommended to use distribution data with at least four years of monitoring, if possible. The number of presences of a species in a model should be 10 x more than the number of environmental predictors. Bathymetry and light availability were popular parameters and are also recommended as essential for seagrass HSM. MaxEnt and ensemble approaches were the most popular methods used in the review. Model choice will be dependent upon presence data available (presence-absence or presence only) but we suggest creating an ensemble approach to combine predictions from several high-performance models to decrease dependence on a single type of model (Table 1). Cross validation was the most used selection procedure and threshold probability the most common validation approach. Best practice measures and a checklist of important model features that need to be reported in marine HSMs are explained in Robinson et al. (2017). Using HSMs to predict the distribution of seagrass depending on environmental variables around the UK will help identify areas that could be suitable for seagrass growth and survival but do not currently show signs of presence. The output of planned HSMs will give a good indication of the most suitable locations with the highest chance of survival and help provide guidance for the future restoration of seagrass habitats around the UK.
Author contributions
RU and JB contributed to study design. CB and HS completed the literature review and analysis of the metadata. HS wrote the initial draft and CB completed the draft and updated the review. All authors contributed to the article and approved the submitted version.
Funding
This study was part of the ReSOW (Restoring Seagrass for Ocean Wealth) project funded by NERC (Natural Environment Research Council) NE/V01711X/1.
Acknowledgments
This research has been published online as a preprint (Bertelli et al., 2022).
Conflict of interest
The authors declare that the research was conducted in the absence of any commercial or financial relationships that could be construed as a potential conflict of interest.
Publisher’s note
All claims expressed in this article are solely those of the authors and do not necessarily represent those of their affiliated organizations, or those of the publisher, the editors and the reviewers. Any product that may be evaluated in this article, or claim that may be made by its manufacturer, is not guaranteed or endorsed by the publisher.
Supplementary material
The Supplementary Material for this article can be found online at: https://www.frontiersin.org/articles/10.3389/fmars.2022.997831/full#supplementary-material
References
Abe M., Hahimoto N., Kurashima A., Maegwa M. (2003). Estimation of light requirement for the growth of zostera marina in central Japan. Fish Sci. 69, 890–895. doi: 10.1046/j.1444-2906.2003.00704.x
Adams M. P., Saunders M. I., Maxwell P. S., Tuazon D., Roelfsema C. M., Callaghan D. P., et al. (2016). Prioritizing localized management actions for seagrass conservation and restoration using a species distribution model. Aquat Conserv. Mar. Freshw. Ecosyst. 26, 639–659. doi: 10.1002/aqc.2573
Adhikari D., Barik S. K., Upadhaya K. (2012). Habitat distribution modelling for reintroduction of ilex khasiana purk., a critically endangered tree species of northeastern India. Ecol. Eng. 40, 37–43. doi: 10.1016/j.ecoleng.2011.12.004
Bakirman T., Gumusay M. U. (2020). A novel GIS-MCDA-based spatial habitat suitability model for Posidonia oceanica in the Mediterranean. Environ. Monit. Assess 192. doi: 10.1007/s10661-020-8198-1
Barnes T. K., Volety A. K., Chartier K., Mazzoti F. J., Pearlstine L. (2007). A habitat suitability index model for the Eastern oyster (Crassostrea virginica), a tool for restoration of the caloosahatchee estuary, Florida. J. Shellfish Res. 26, 949–959. doi: 10.2983/0730-8000(2007)26[949:AHSIMF]2.0.CO;2
Beca-Carretero P., Varela S., Stengel D. B. (2020). A novel method combining species distribution models, remote sensing, and field surveys for detecting and mapping subtidal seagrass meadows. Aquat. Conserv. Mar. Freshw. Ecosyst. 30, 1098–1110. doi: 10.1002/aqc.3312
Bellamy C., Scott C., Altringham J. (2013). Multiscale, presence-only habitat suitability models: Fine-resolution maps for eight bat species. J. Appl. Ecol. 50, 892–901. doi: 10.1111/1365-2664.12117
Bertelli C., Stokes H., Bull J., Unsworth R. K. F. (2022). The use of habitat suitability modelling for seagrass: A review. Figshare. doi: 10.6084/m9.figshare.20310429.v2
Bertelli C. M., Unsworth R. K. F. (2018). Light stress responses by the eelgrass, zostera marina (L). Front. Environ. Sci. 6, 1–13. doi: 10.3389/fenvs.2018.00039
Blok S. E., Olesen B., Krause-Jensen D. (2018). Life history events of eelgrass zostera marina l. populations across gradients latitude temperature. Mar. Ecol. Prog. Ser. 590, 79–93. doi: 10.3354/meps12479
Borja Á, Elliott M. (2013). Marine monitoring during an economic crisis: The cure is worse than the disease. Mar. Pollut. Bull. 68, 1–3. doi: 10.1016/j.marpolbul.2013.01.041
Canal-Vergés P., Petersen J. K., Rasmussen E. K., Erichsen A., Flindt M. R. (2016). Validating GIS tool to assess eelgrass potential recovery in the Limfjorden (Denmark). Ecol. Modell. 338, 135–148. doi: 10.1016/j.ecolmodel.2016.04.023
Catucci E., Scardi M. (2020). A Machine Learning approach to the assessment of the vulnerability of Posidonia oceanica meadows. Ecol. Indic. 108, 105744. doi: 10.1016/j.ecolind.2019.105744
Chefaoui R. M., Assis J., Duarte C. M., Serrão E. A. (2016). Large-Scale prediction of seagrass distribution integrating landscape metrics and environmental factors: The case of cymodocea nodosa (Mediterranean–Atlantic). Estuaries Coasts 39, 123–137. doi: 10.1007/s12237-015-9966-y
Convertino M., Welle P., Muñoz-Carpena R., Kiker G. A., Chu-Agor M. L., Fischer R. A., et al. (2012). Epistemic uncertainty in predicting shorebird biogeography affected by sea-level rise. Ecol. Modell 240, 1–15. doi: 10.1016/j.ecolmodel.2012.04.012
Davis T. R., Champion C., Coleman M. A. (2021). Climate refugia for kelp within an ocean warming hotspot revealed by stacked species distribution modelling. Mar. Environ. Res. 166, 105267. doi: 10.1016/j.marenvres.2021.105267
Davis T. R., Harasti D., Smith S. D. A., Kelaher B. P. (2016). Using modelling to predict impacts of sea level rise and increased turbidity on seagrass distributions in estuarine embayments. Estuar. Coast. Shelf Sci. 181, 294–301. doi: 10.1016/j.ecss.2016.09.005
Elith J., Phillips S. J., Hastie T., Dudík M., Chee Y. E., Yates C. J. (2011). A statistical explanation of MaxEnt for ecologists. Divers. Distrib 17, 43–57. doi: 10.1111/j.1472-4642.2010.00725.x
Fabbrizzi E., Scardi M., Ballesteros E., Benedetti-Cecchi L., Cebrian E., Ceccherelli G., et al. (2020). Modeling macroalgal forest distribution at mediterranean scale: Present status, drivers of changes and insights for conservation and management. Front. Mar. Sci. 7. doi: 10.3389/fmars.2020.00020
Folmer E. O., van Beusekom J. E. E., Dolch T., Gräwe U., van Katwijk M. M., Kolbe K. P., et al (2016). Consensus forecasting of intertidal seagrass habitat in the Wadden Sea. J. Appl. Ecol. 53, 1800–1813. doi: 10.1111/1365-2664.12681
Fourqurean J. W., Duarte C. M., Kennedy H., Marba N., Holmer M., Mateo M. A., et al. (2012). Seagrass ecosystems as a globally significant carbon stock. Nat. Geosci 5, 505–509. doi: 10.1038/ngeo1477
Gamble C., Debney A., Glover A., Bertelli C., Green B., Hendy I., et al. (2021). Seagrass restoration handbook UK & Ireland. London, UK: Zoological society of London, UK.
Georgian S. E., Anderson O. F., Rowden A. A. (2019). Ensemble habitat suitability modeling of vulnerable marine ecosystem indicator taxa to inform deep-sea fisheries management in the south pacific ocean. Fish Res. 211, 256–274. doi: 10.1016/j.fishres.2018.11.020
Gormley K. S. G., Hull A. D., Porter J. S., Bell M. C., Sanderson W. G. (2015). Adaptive management, international co-operation and planning for marine conservation hotspots in a changing climate. Mar. Policy 53, 54–66. doi: 10.1016/j.marpol.2014.11.017
Guarnieri G., McOwen C., Papa L., Papadopoulou N., Bilan M., Boström C., et al. (2019). “Active restoration across marine coastal habitats: A focus on the Mediterranean Sea,” in 3rd Mediterranean symposium on the conservation of coralligenous & other calcareous bio-concretions. Springer, Cham: Coral Reefs of the World, 9
Guisan A., Thuiller W., Zimmermann N. E. (2017). Habitat suitability and distribution models with applications in r (Cambridge: Cambridge University Press). doi: 10.1017/9781139028271
Hirzel A. H., Le Lay G. (2008). Habitat suitability modelling and niche theory. J. Appl. Ecol. 45, 1372–1381. doi: 10.1111/j.1365-2664.2008.01524.x
Jayathilake D. R. M., Costello M. J. (2018). A modelled global distribution of the seagrass biome. Biol. Conserv. 226, 120–126. doi: 10.1016/j.biocon.2018.07.009
Jueterbock A., Smolina I., Coyer J. A., Hoarau G. (2016). The fate of the Arctic seaweed fucus distichus under climate change: An ecological niche modeling approach. Ecol. Evol. 6, 1712–1724. doi: 10.1002/ece3.2001
Jueterbock A., Tyberghein L., Verbruggen H., Coyer J. A., Olsen J. L., Hoarau G. (2013). Climate change impact on seaweed meadow distribution in the north Atlantic rocky intertidal. Ecol. Evol. 3, 1356–1373. doi: 10.1002/ece3.541
Kairo J. G., Bosire J., Langat J., Kirui B., Koedam N. (2009). Allometry and biomass distribution in replanted mangrove plantations at gazi bay, Kenya. Aquat Conserv. Mar. Freshw. Ecosyst. 19, S63–S69. doi: 10.1002/aqc.1046
Kerr J. (2017). Introduction to energy and climate: Developing a sustainable environment, 1st ed (CRC Press). doi: 10.1201/9781315151885
Lanuru M., Mashoreng S., Amri K. (2018). Using site-selection model to identify suitable sites for seagrass transplantation in the west coast of South Sulawesi. J. Phys. Conf. Ser. 979. doi: 10.1088/1742-6596/979/1/012007
Marion S. R., Orth R. J. (2010). Innovative Techniques for Large-scale Seagrass Restoration Using Zostera marina (eelgrass) Seeds. Restor. Ecol. 18, 514–526.
Matthiopoulos J., Fieberg J., Aarts G. (2020). Species-habitat associations: Spatial data, predictive models, and ecological insights (University of Minnesota Digital Conservancy: University of Minnesota Libraries Publishing). doi: 10.24926/2020.081320
Maxwell P. S., Ekl J. S., Van Katwijk M. M., Brien K. R. O. (2016). The fundamental role of ecological feedback mechanisms for the adaptive management of seagrass ecosystems – a review. Biological Reviews 92 (3), 1521–1538. doi: 10.1111/brv.12294
McDonald A. M., Prado P., Heck K. L., Fourqurean J. W., Frankovich T. A., Dunton K. H., et al. (2016). Seagrass growth, reproductive, and morphological plasticity across environmental gradients over a large spatial scale. Aquat Bot. 134, 87–96. doi: 10.1016/j.aquabot.2016.07.007
Nordlund L. M., Koch E. W., Barbier E. B., Creed J. C. (2016). Seagrass ecosystem services and their variability across genera and geographical regions. PloS One 11, 1–23. doi: 10.1371/journal.pone.0163091
Nyström Sandman A., Wikström S. A., Blomqvist M., Kautsky H., Isaeus M. (2013). Scale-dependent influence of environmental variables on species distribution: A case study on five coastal benthic species in the Baltic Sea. Ecography (Cop) 36, 354–363. doi: 10.1111/j.1600-0587.2012.07053.x
Orth R. J., Lefcheck J. S., McGlathery K. S., Aoki L., Luckenbach M. W., Moore K. A., et al. (2020). Restoration of seagrass habitat leads to rapid recovery of coastal ecosystem services. Sci. Adv. 6, eabc6434. doi: 10.1126/sciadv.abc6434
Paquit J. C., Pampolina N. M., Tiburan C. L., Manalo M. M. Q. (2017). Maxent modeling of the habitat distribution of the critically endangered pterocarpus indicus willd. forma indicus in Mindanao, Philippines. J. Biodivers Environ. Sci. 10, 112–122.
Peralta G., Brun F. G., Pérez-Lloréns J. L., Bouma T. J. (2006). Direct effects of current velocity on the growth, morphometry and architecture of seagrasses: A case study on zostera noltii. Mar. Ecol. Prog. Ser. 327, 135–142. doi: 10.3354/meps327135
Reynolds L. K., Waycott M., McGlathery K. J., Orth R. J. (2016). Ecosystem services returned through seagrass restoration. Restor. Ecol. 24, 583–588. doi: 10.1111/rec.12360
Robinson N. M., Nelson W. A., Costello M. J., Sutherland J. E., Lundquist C. J. (2017). A systematic review of marine-based species distribution models (SDMs) with recommendations for best practice. Front. Mar. Sci. 4, 1–11. doi: 10.3389/fmars.2017.00421
Röhr M. E., Holmer M., Baum J. K., Björk M., Chin D., Chalifour L., et al. (2018). Blue carbon storage capacity of temperate eelgrass (Zostera marina) meadows. Global Biogeochem Cycles 32, 1457–1475. doi: 10.1029/2018GB005941
Shafer D. J., Swannack T. M., Saltus C., Kaldy J. E., Davis A. (2016). Development and validation of a habitat suitability model for the non-indigenous seagrass zostera japonica in north america. Manag Biol. Invasions 7, 141–155. doi: 10.3391/mbi.2016.7.2.02
Sillero N., Barbosa A. M. (2021). Common mistakes in ecological niche models. Int. J. Geogr. Inf Sci. 35, 213–226. doi: 10.1080/13658816.2020.1798968
Singer A., Millat G., Staneva J., Kröncke I. (2017). Modelling benthic macrofauna and seagrass distribution patterns in a north Sea tidal basin in response to 2050 climatic and environmental scenarios. Estuar. Coast. Shelf Sci. 188, 99–108. doi: 10.1016/j.ecss.2017.02.003
Spivak A. C., Sanderman J., Bowen J. L., Canuel E. A., Hopkinson C. S. (2019). Global-change controls on soil-carbon accumulation and loss in coastal vegetated ecosystems. Nat. Geosci 12, 685–692. doi: 10.1038/s41561-019-0435-2
Stafford R., Chamberlain B., Clavey L., Gillingham P. K., McKain S., Morecroft M. D., et al. (2021). Nature-based solutions for climate change in the UK: A report by the British ecological society. Br. Ecol. Soc. 190.
Tan Y. M., Dalby O., Kendrick G. A., Statton J., Sinclair E. A., Fraser M. W., et al. (2020). Seagrass Restoration Is Possible: Insights and Lessons From Australia and New Zealand. Front. Mar. Sci. 7. doi: 10.3389/fmars.2020.00617
Tyberghein L., Verbruggen H., Pauly K., Troupin C., Mineur F., De Clerck O. (2012). Bio-ORACLE: A global environmental dataset for marine species distribution modelling. Glob Ecol. Biogeogr. 21, 272–281. doi: 10.1111/j.1466-8238.2011.00656.x
Unsworth R. K. F., Bertelli C. M., Cullen-Unsworth L. C., Esteban N., Jones B. L., Lilley R., et al. (2019a). Sowing the seeds of seagrass recovery using hessian bags. Front. Ecol. Evol. 7, 1–7. doi: 10.3389/fevo.2019.00311
Unsworth R. K. F., McKenzie L. J., Collier C. J., Cullen-Unsworth L. C., Duarte C. M., Eklöf J. S., et al. (2019b). Global challenges for seagrass conservation. Ambio 48, 801–815. doi: 10.1007/s13280-018-1115-y
Valle M., Borja Á, Chust G., Galparsoro I., Garmendia J. M. (2011). Modelling suitable estuarine habitats for zostera noltii, using ecological niche factor analysis and bathymetric LiDAR. Estuar. Coast. Shelf Sci. 94, 144–154. doi: 10.1016/j.ecss.2011.05.031
Valle M., Chust G., del Campo A., Wisz M. S., Olsen S. M., Garmendia J. M., et al. (2014). Projecting future distribution of the seagrass zostera noltii under global warming and sea level rise. Biol. Conserv. 170, 74–85. doi: 10.1016/j.biocon.2013.12.017
Valle M., van Katwijk M. M., de Jong D. J., Bouma T. J., Schipper A. M., Chust G., et al. (2013). Comparing the performance of species distribution models of zostera marina: Implications for conservation. J. Sea Res. 83, 56–64. doi: 10.1016/j.seares.2013.03.002
van Katwijk M. M., Thorhaug A., Marbà N., Orth R. J., Duarte C. M., Kendrick G. A., et al. (2016). Global analysis of seagrass restoration: The importance of large-scale planting. J. Appl. Ecol. 53, 567–578. doi: 10.1111/1365-2664.12562
Verbruggen H., Tyberghein L., Belton G. S., Mineur F., Jueterbock A., Hoarau G., et al. (2013). Improving transferability of introduced species’ distribution models: New tools to forecast the spread of a highly invasive seaweed. PloS One 8, 1–13. doi: 10.1371/journal.pone.0068337
Verbruggen H., Tyberghein L., Pauly K., Vlaeminck C., Van Nieuwenhuyze K., Kooistra W. H. C. F., et al. (2009). Macroecology meets macroevolution: Evolutionary niche dynamics in the seaweed halimeda. Glob Ecol. Biogeogr 18, 393–405. doi: 10.1111/j.1466-8238.2009.00463.x
Vinagre C., Fonseca V., Cabral H., Costa M. J. (2006). Habitat suitability index models for the juvenile soles, solea solea and solea senegalensis, in the tagus estuary: Defining variables for species management. Fish Res. 82, 140–149. doi: 10.1016/j.fishres.2006.07.011
Waycott M., Duarte C. M., Carruthers T. J. B., Orth R. J., Dennison W. C., Olyarnik S., et al. (2009). Accelerating loss of seagrasses across the globe threatens coastal ecosystems. PNAS 1, 12377–12381. doi: 10.1073/pnas.0905620106
Wesselmann M., Chefaoui R. M., Marbà N., Serrao E. A., Duarte C. M. (2021). Warming threatens to propel the expansion of the exotic seagrass halophila stipulacea. Front. Mar. Sci. 8, 1–14. doi: 10.3389/fmars.2021.759676
Keywords: habitat suitability modelling (HSM), seagrass, macrophyte, restoration, ensemble, Maxent (maximum entropy)
Citation: Bertelli CM, Stokes HJ, Bull JC and Unsworth RKF (2022) The use of habitat suitability modelling for seagrass: A review. Front. Mar. Sci. 9:997831. doi: 10.3389/fmars.2022.997831
Received: 19 July 2022; Accepted: 10 October 2022;
Published: 26 October 2022.
Edited by:
Junyu He, Zhejiang University, ChinaReviewed by:
Kathryn Margaret McMahon, Edith Cowan University, AustraliaAmrit Kumar Mishra, The University of Hong Kong, Hong Kong SAR, China
Copyright © 2022 Bertelli, Stokes, Bull and Unsworth. This is an open-access article distributed under the terms of the Creative Commons Attribution License (CC BY). The use, distribution or reproduction in other forums is permitted, provided the original author(s) and the copyright owner(s) are credited and that the original publication in this journal is cited, in accordance with accepted academic practice. No use, distribution or reproduction is permitted which does not comply with these terms.
*Correspondence: Chiara M. Bertelli, Yy5tLmJlcnRlbGxpQHN3YW5zZWEuYWMudWs=
†These authors have contributed equally to this work and share first authorship