Acoustic indices respond to specific marine mammal vocalizations and sources of anthropogenic noise
- 1Ocean Science Analytics, San Diego, CA, United States
- 2Hopkins Marine Station, Stanford University, Pacific Grove, CA, United States
- 3Environmental Assessment Services, Limited Liability Company (LLC) Contracted to Southwest Fisheries Science Center, Richland, WA, United States
Using passive acoustic methods for biodiversity conservation and effective ecosystem monitoring is hindered by laborious, human-mediated processes of accurately identifying biologic and anthropogenic sounds within large datasets. Soundscape ecology provides a potential means of addressing this need through the use of automated acoustic-based biodiversity indices, which show promise in representing biodiversity in terrestrial environments. However, the direct relationship between specific underwater sounds and acoustic index measurements are largely unexplored. Using passive acoustic data collected from three broadband hydrophones within the Ocean Observatories Initiative’s cabled arrays in the Pacific northwest, we identified periods of vocalizing marine mammals and sources of anthropogenic noise. Automated calculations of seven acoustic indices were compared across biologic and anthropogenic sound type and call parameters. Although several index measurements did not vary significantly, the Acoustic Complexity Index (ACI) measurements increased in response to echolocation clicks from sperm whales (Physeter macrocephalus) and burst pulses originating from unidentified delphinid species. Measurements of the Bioacoustic Index (BI) decreased dramatically in response to sperm whale echolocation clicks, a more obvious trend when loud clicks were parsed from moderate and quiet clicks. Correlations coefficient and confidence interval values between ACI and BI measurements and call characteristics from sperm whales indicate a moderate to strong relationship, which was not found in correlations with delphinid calls. A generalized linear mixed-effect model indicated multiple species and sound types contribute significantly to the variation of several index measurements. Noise generated by passing ships consistently resulted in decreased values for the Normalized Difference Soundscape Index (NDSI) and Total Entropy (H) as compared to quiet periods and periods with vocalizing marine mammals. These findings provide information on the relationship between several acoustic indices and specific underwater sounds produced by marine mammals and anthropogenic sources. This ground-truthing endeavor expands the understanding of acoustic indices and their potential use as a tool for conservation and ecosystem health management purposes.
1 Introduction
Biodiversity conservation is increasingly considered an essential element to maintaining ecosystem health and function (Tittensor et al., 2019; Sala et al., 2021). Studies have utilized various ecological indicators to describe the overall condition or health of marine ecosystems, and inform management and conservation practices (Halpern et al., 2014; Loomis et al., 2014; Miloslavich et al., 2018). Ecological indicators are biological, chemical and physical measures of key components in a specific environment, wherein the collective use of these indicators provides a means of evaluating and managing an ecosystem (Rombouts et al., 2013; Queirós et al., 2016). However, to effectively employ this holistic method, accurate representative indicators must be selected. One of the more challenging indicators to identify are biological indicators, which are either direct or indirect measures of the biodiversity of marine species (Holt & Miller, 2011; Parmar et al., 2016).
Marine mammals are important ecological contributors in ocean ecosystems and studies regarding their keystone predator status have shown they significantly impact prey abundance and thus ecosystem health (Moore, 2008; Benoit-Bird et al., 2013; Moore et al., 2014; Moore & Kuletz, 2019). Establishing a baseline understanding of the typical seasonal and inter-annual occurrence of these large megafauna within a region is important for monitoring ecosystem response to climate change or renewable technology infrastructure (Laidre et al., 2008; Van Parijs et al., 2021). Yet continuously collecting visual observations of marine mammals within a region is impractical due to the high cost of aerial or vessel surveys along with inclement, seasonal weather (Moore et al., 2018). This prevents resource managers from continuously collecting direct measures (visual observations) of marine mammal biodiversity within marine ecosystem.
Passive acoustic monitoring using long-term recorders is a well-vetted tool that provides an indirect measure of marine mammal temporal and spatial occurrence (Wiggins & Hildebrand, 2007; Lammers et al., 2008; Aniceto et al., 2022). Continuous acoustic monitoring is becoming more prevalent and dependable as a conservation approach, leading to recommendations for longer term acoustic studies during offshore wind development operations and incorporation of hydrophones at long-term monitoring sites (Trowbridge et al., 2019; Van Parijs et al., 2021). Although data collection has become more feasible and cost effective, large acoustic datasets are often met with substantial processing delays due to the need for human-mediated annotation of underwater sounds by an experienced analyst (Gibb et al., 2019). Underwater sounds are differentiated into one of three broad categories: biophony (biological sources of sound such as marine mammals), geophony (geophysical sources of sounds such as earthquakes), and anthrophony (human-produced sources of sound such as passing ships and sonar) as coined by Krause (2008). Due to the variability in the types of sounds that can occur in underwater environments, processing of acoustic data for biodiversity purposes requires that a wide range of sounds can be accurately detected and differentiated. Although machine learning has expedited the review process, most methods remain either semi-automated and reliant on an experienced acoustic analyst or are limited to detecting specific, stereotyped calls (Browning et al., 2017). To achieve more timely processing of passive acoustic data and inform ecosystem monitoring efforts, techniques for the efficient assessment of continuously collected data are required.
Soundscape ecology, or the study of acoustic relationships between organisms and their environment, is increasingly used as a tool for evaluating biodiversity and biological monitoring (Pijanowski et al., 2011; Sueur et al., 2014; Duarte et al., 2015; Merchant et al., 2015; Risch & Parks, 2017). Pijanowski et al. (2011) highlighted the importance of soundscape ecology and the ability to compute acoustic indices for the purposes of biodiversity measurement and assessment of the pervasiveness of anthropogenic noise. Sueur et al. (2014) went on to further elaborate on α indices that evaluate the quality and context of sounds within terrestrial acoustic environments using either amplitude, sound complexity level, or the overall contribution to a soundscape. Important aspects of this suite of metrics include the low costs associated with generating measurements, and the rapid ability to determine the occurrence of biological activity independent of manual annotation. Recently, these terrestrial-based mathematical tools were assessed for their applicability to marine environments (Erbe et al., 2015; Bertucci et al., 2016; Haver et al., 2018). Fish and coral reef ecology studies lead the charge in utilizing soundscape metrics (Rice et al., 2017; Elise et al., 2019a; Elise et al., 2019b; Benocci et al., 2022). Most of these studies occur in shallow waters (<30 m) along the coast, or within coral reefs or estuaries and focus on sounds within a 0-8,000 Hz frequency band (Pieretti and Danovaro, 2020). While there is some indication that α acoustic indices may be associated with periods of increased biological sound activity, their suitability in underwater environments requires a greater understanding of how specific biophony, geophony and anthrophony influence these measurements in marine environments.
Although limited in number, a handful of studies have explored the relationship between acoustic indices and specific biological sounds. Parks et al (2014) study explored the connection between several acoustic indices and baleen whale calls, finding a potential response in the noise compensated entropy index to periods of vocal activityRoca and Van Opzeeland (2020) machine learning assessment of marine mammal biodiversity between pelagic and on-shelf acoustic environments successfully identified periods of increased diversity using a combination of 23 measurements. Their efforts elucidate the potential for certain indices or a combination of indices to be associated with marine mammal biodiversity. Benocci et al. (2022) further explored the influence of sounds from an artificial tank environment on acoustic indices and despite the occurrence of mechanical and anthropic origin sounds, found consistent responses to fish sounds which could be useful in natural environments. However, other studies found limitations and inconsistencies in the relationship between call type or rate and index measurements (Parsons et al., 2016; Bohnenstiehl et al., 2018). Staaterman et al. (2017) paired the calculation of several acoustic indices with visual fish surveys in four habitat types and found these metrics were not clear indicators of fish biodiversity, recommending the needs for further development and ground-truthing of these measurements. Mooney et al. (2020) suggest that understanding the sources responsible for the variability in acoustic indices is an important element to their utilization. Therefore, additional studies are needed to establish a better understanding of these potentially useful indirect biological indicators.
In this study, we explore the relationship between several acoustic indices and underwater sounds detected at three sites along one of the Ocean Observatories Initiative’s monitoring regions situated in the dynamic California Current Ecosystem. Pieretti and Danovaro (2020) point out that most marine studies involving these metrics evaluate sounds produced by the community as a whole, as opposed to specifically focusing on an individual species. Our objective in this effort is to address the need for understanding what influences these measurements by determining the trends of seven acoustic indices in association with species-specific sounds. Furthermore, we evaluate recordings from multiple sites within the same ecosystem to account for differences attributable to instrument noise, and ambient noise across and along the slope. The intent of this ground-truthing effort is to provide novel understanding of how acoustic indices consistently respond to select underwater sounds.
2 Materials and methods
The coastal waters off Newport, Oregon are part of the California Current Ecosystem and are highly productive largely due to a combination of wind-driven upwelling and river plume supplied nitrate to the adjacent waters (Huyer et al., 2005; Hickey et al., 2010). These distinctive oceanographic processes in conjunction with a burgeoning fishing industry led to the selection of this site for monitoring by the Ocean Observatories Initiative (OOI) (Trowbridge et al., 2019). The OOI consists of a series of ocean observation networks that provide ecosystem monitoring data from hundreds of instruments which will continue collecting data for the next 20 years. The Coastal Endurance and Continental Margin Cabled Arrays are strategically situated within this region and consists of cross- and along-shelf broadband icListen hydrophones deployed and maintained by the OOI (Figure 1). The recorders on the shelf at 80 m (HYDBBA 106) and the slope at ~580 m (HYDBBA 105) are part of the Coastal Endurance Array. The recorder on the base of the slope on a 200 m shallow profiler mooring (HYDBBA 103) is part of the Cabled Continental Marin Array. Passive acoustic data from all three recorders include a monitoring bandwidth of 32 kHz (64 kHz sampling rate) and have been continuously recording since 2016. The Ocean Sonics icListen HF have a monitoring bandwidth of 10 Hz to 200 kHz but are limited to a 64 kHz sampling rate by the data transfer hardware and process. We evaluated one month of data from each recorder: January 2017 for HYDBBA 103 and 106, and April 2018 for HYDBBA 105. We had previously annotated these months of data and there were limitations to the overlap in datasets across all three recorders that resulted in their use in this study. Annotation of this data was unfunded and we utilized what data had been processed to date. Although the data from HYDBBA 103 and 106 were collected in the same month of 2017, they are separated by almost 90 kilometers, which for most species is too great a distance to simultaneously detect sounds from the same group of animals.
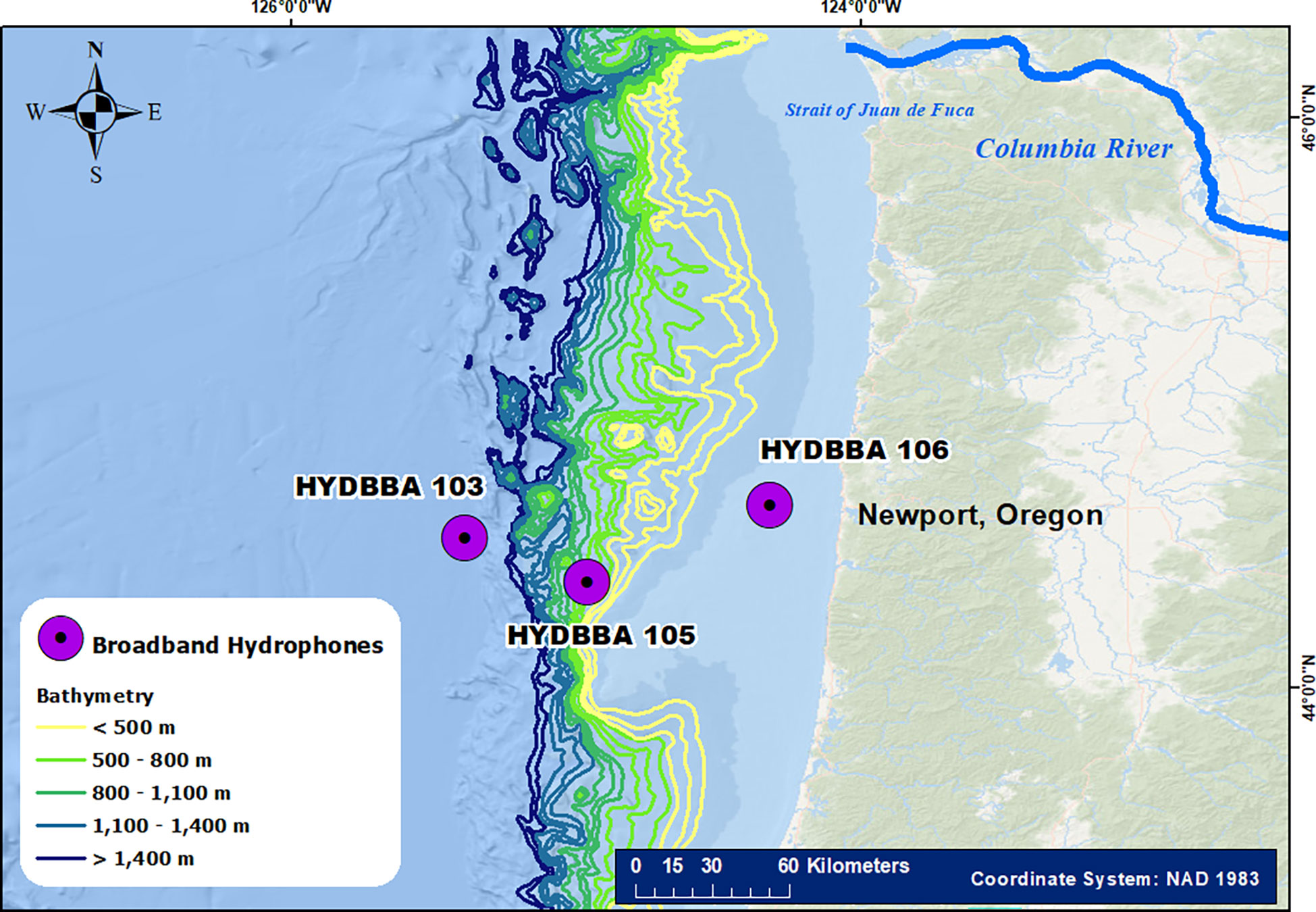
Figure 1 Map of the study area showing the location of the three recorders on the Ocean Observatories Initiative cabled arrays. The recorders on the shelf at 80 m (HYDBBA 106) and the slope at ~580 m (HYDBBA 105) are part of the Coastal Endurance array. The recorder on the base of the slope on a 200 m shallow profiler mooring (HYDBBA 103) is part of the Cabled Continental Marin array. Recorders are located 30 km to 60 km from each other and offshore from Newport, Oregon. Bathymetric lines indicate increasing depth across the slope with darker colors.
2.1 Annotation of acoustic events
We reviewed the three months of data to determine periods of vocalizing marine mammal species and anthropogenic sounds. The raw audio (.wav files) acoustic dataset was processed in PAMGuard (version 2.01.05; Gillespie et al., 2009) using a two-stage process. PAMGuard is a widely used, open access software program that includes automated and semi-automated modules for the detection and localization of marine mammals. PAMGuard is a modular program that allows the user to define what display, detection, localizations and noise monitoring modules they wish to use in an analysis. The first stage of processing occurred in PAMGuard’s standard mode and involved automated detection of calls from several marine mammal species using a combination of the in-built click and whistle and moan detector modules. Settings for the click detector included standard settings and a series of click classifiers to differentiate delphinid and sperm whale echolocation clicks. Two click classifiers were used for the detection of echolocation clicks from sperm whales to differentiate between “regular” and “slow” clicks (Weilgart & Whitehead, 1988; Barlow & Taylor, 2005). We wanted to make sure both click types were detectable in the processing. The “slow” click classifier consisted of a detection/test band of 500 Hz – 8 kHz, and peak frequency range of 500 Hz – 4 kHz. The “regular” click classifier consisted of a detection/test band of 1 – 12 kHz and peak frequency range of 5-12 kHz. All tonal and burst pulse type of calls were detected using the whistle and moan detector. Frequency range was adjusted to detect all potential sounds within the available bandwidth, requiring true positives to be determined in the secondary stage (Gillespie et al., 2009; Gillespie et al., 2013). Default settings for noise and thresholding were retained.
The second stage of processing involved using PAMGuard’s ViewerMode for the post-automation annotation of the automated detections. Annotation was performed by an experienced bioacoustic analyst and involved discrimination of detections into appropriate “acoustic events.” An acoustic event was defined as a bout of calls from a biological source that had no more than 30 minutes gap between calls from the same species for whistles or burst pulse calls, and no more than 60 minutes gap between bouts for calls from sperm whales and baleen whale species. This selection of time interval is mostly just used as a means of organizing data that are “likely” from the same individual or group, given their potential to remain detected in the area for a certain amount of time. We were not however using this information for any density or abundance determination, so the organization is mostly just useful for data management purposes. Calls attributable to marine mammals were assigned to “acoustic events” according to species or species group (e.g., delphinid species, fin whale). Annotations were made by using the Detection Grouper module in PAMGuard, which allows a user to selected detected calls and assign those calls to a group which can then have custom information associated with the group. The assignment of calls to a group relies on an analyst identifying a period of calls within the data, and then boxing calls to assign to the group. Any calls that were ambiguous or unidentifiable simply received an “unidentified sound” or “unidentified cetacean” assignment. Any detections that were attributable to ambient or instrument noise (false positives) were disregarded, so that only annotations of true events were included in further processing. Annotations were intermittently reviewed by a second, expert bioacoustic analyst to ensure species identification were accurate.
Processing data for all potential calls within the same processing run can be computationally intensive. To increase efficiency of the annotation process, three analysis iterations were completed so the analyst could focus on classifying calls from a subset of possible species/species groups. The very low frequency run (10-200 Hz) focused on calls from baleen whales such as fin and blue whales. The low frequency run (200 Hz – 2 kHz) focused on slightly higher frequency calls, such as those from humpback whales. The mid-high frequency run (2 kHz – 32 kHz) was used to annotate calls from odontocetes, such as sperm whales and delphinids. During annotation, several modules are used to expedite the review of automated detections and parse out true detections from noise This specifically included the Long-term Spectral Average module which allows average spectrum measurements over periods of several seconds (10 seconds in this study) and a clip generator that organizes clips of detections for rapid review. Acoustic event annotations were not used to identify individuals or specific groups within the data, as an animal may have moved past the recorder and returned; acoustic events were solely used to assist in the data management of acoustic encounters.
Periods of passing ship often triggered false positives on the click detector. These false positives were reliable for documenting these underwater sounds and were annotated as “Passing ship” acoustic events. Ships were only annotated in this way if they exhibited the same ship event structure observed in the long-term spectral average display by other studies (Ragland et al., 2022). Mid-frequency active sonar was annotated by selecting contours from the whistle and moan detector. No other sources of anthropogenic noise (e.g., echosounders) were annotated in this analysis.
2.2 Extraction of call characteristics
Acoustic event-based information including the species identification, the count of calls (number of calls per audio file, per species), and select additional parameters were extracted using the R-based package ‘PAMpal’ (v0.13.0; Sakai et al., 2020). PAMpal extracts information from the binary files and database generated by PAMGuard, and allows users to calculate parameters from detected calls and summarize this information in user selected time intervals. A call or detection count was summed for each five-minute wav file, and multiple acoustic events occurring within the same file were summarized independently. Additionally, select parameters calculated from the “contours” (which are the detections made by the whistle and moan detector) included the mean call amplitude (dB), mean call bandwidth (Hz), and mean frequency (Hz) determined for each 5-minute wav file. For echolocation clicks, we extracted the total number of clicks as well as average measures of mean click amplitude (dBPP - a relative measure of intensity/amplitude calculated as 20 * log10 of the difference between the maximum and minimum value of the waveform), mean 10 dB bandwidth (kHz), and mean peak frequency (kHz) for each five-minute wav file associated with sperm whale acoustic events. We did not differentiate between "slow" and "regular" clicks for parameter measurement extraction, so data from both click categories were combined for analyses. Additionally, we extracted occurrence information (e.g., labeled a five-minute wav file as containing a type of sound) for anthropogenic sounds such as passing ships and mid-frequency active sonar.
Output from R calculations of acoustic indices were merged with summaries of information in five-minute files from marine mammal and anthropogenic acoustic events. We assigned details of call types to indicate if each five-minute wav files contained contours from a single event, from overlapping (different species) events, contours and clicks from single or overlapping events, or periods where biophony and anthrophony were not detected. When correlating call characteristics to indices, we excluded all five-minute wav files with multiple species. For all analysis and graphs comparing index measurements with a specific sound type, only data containing calls from that specific species/species group or anthropogenic noise source were included. We did not include periods where calls or sounds overlapped. We are confident that all possible sounds from marine mammals and anthropogenic noise were annotated in each dataset, meaning the “Vocalizations absent” category can only have included ambient and instrument noise or potentially noise from geophony. We did not exclude periods with rain or storms from this “Vocalizations absent” category as we wanted the natural variability of ocean ambient noise to be reflected in those periods without marine mammal or anthropogenic sounds. Finally, prior to performing statistical tests and descriptive graphics, we determined the average call count from the data specific to each recorder for all marine mammal species and excluded all five-minute wav files that contained fewer calls than the mean call count value across all acoustic events. This was done to ensure that data used in the statistical tests and comparative figures included a sufficient number of calls to relate to quiet periods without vocalizations.
2.3 Statistical analysis
The statistical analyses included in our study were identified due to the presumed potential for pseudoreplication, or observations that could include temporal or spatial dependence. As posed by Alcocer et al. (2022), the data had the large potential for temporal autocorrelation given successive five-minute periods were identified as individual data points. To account for this issue we incorporated statistical measures that are robust to the non-independence, as demonstrated by Moreno-Gómez et al. (2019) in a study of acoustic indices and bird and anuran richness.
Evaluating the Relevance of Species to Index Measurement Variation: Determining the effect of species or anthropogenic sound source on acoustic indices was an important element to this study. Boxplots grouped by recorder and reported by species/species group or sound type were initially created. We then assessed the contribution of species to the acoustic index measurements by fitting a per-index generalized linear mixed-effects model using the ‘lme4’ R package (Bates et al., 2015). Given the size of this dataset and the variability in detected sounds on each recorder, as well as the difference in month for one of the recorders, each index was fitted with a single model that examined species as a fixed effect and used recorder as a random effect. As we did not produce separate models to compare in this analysis, we do not report AIC values or assess the best fit model.
Correlating Index Measurements to Call Characteristics: We examined the relationship between each of several acoustic indices and characteristics from species where a notable difference from periods without vocalizations were observed in the descriptive figures. This was performed using a Spearman correlation test to determine a correlation coefficient (“ρ”) to measure the strength and direction of the variables' relationship using the spearmanRho function of the ‘rcompanion’ package in R (Mangiafico, 2016). The nonparametric Spearman correlation was used due to all index measurements exhibiting a non-normal distribution. To mitigate issues that could potentially arise due to data dependence for each recorder, we performed a bootstrap routine with 1,000 iterations. Bootstrapped correlation coefficient measurements and associated confidence intervals were calculated for all extracted call measurements.
A summary of sample sizes were reported for all call types and all recorders. All statistical analysis was performed using the native stats package in R (v4.2.2; R Core Team, 2022) and figures were generated using the ‘ggplot2’ package in R.
2.4 Acoustic indices
2.4.1 Description of indices
The OOI collects data in five-minute files, so for data management purposes, we used this time interval for calculation of acoustic indices and acoustic event summarization. Given a recent publication on the guidelines for use of acoustic indices in environmental research by Bradfer‐Lawrence (2019) used 10 min continuous recordings as part of the dataset, we determined five-minutes represented an acceptable recording duration. For each of the five-minute raw audio (.wav files), the following acoustic indices were calculated: Acoustic Complexity Index, Acoustic Diversity Index, Acoustic Evenness Index, Bioacoustic Index, Normalized Difference Soundscape Index, Number of Peaks, and Total Entropy (termed “Acoustic Entropy Index” in other studies).
The Acoustic Complexity Index (ACI) is an algorithm developed by Farina (2019) to provide a fast and direct quantification of biological sounds based on intensity. It is the most commonly assessed acoustic index in marine environments (Pieretti and Danovaro, 2020; Minello et al., 2021). ACI calculates the differences in amplitude in adjacent time samples for each frequency bin, then adds together the difference, thus representing a measure of complexity of the acoustic environment. The concept behind this metric is that biological sounds are more variable with respect to intensity than anthropogenic sounds, and several studies have demonstrated ACI use for evaluating species diversity (Pieretti et al, 2017; Davies et al., 2020). Greater diversity in calls from terrestrial species is linked to larger ACI values, although irregular noise from wind and rain can increase this metric as well. ACI was first applied to an underwater environment by McWilliam and Hawkins (2013) who identified a strong association with biological sounds. Subsequent marine-based studies report that ACI is a promising biodiversity metric as summarized most recently by Minello et al. (2021). However, other studies have reported that this metric varies greatly in association with underwater sounds (Kaplan et al., 2015; Bohnenstiehl et al., 2018; Bolgan et al., 2018).
The Acoustic Diversity Index (AD) and the Acoustic Evenness Index (AE) are complementary measures that are have an inverse relationship with the data (Villanueva-Rivera et al., 2011). The AD applies the Shannon Diversity Index (a mathematical method to measure the diversity of species in a community) to the relative proportion of signal occurring in each 1 kHz frequency band (Shannon, 1948). Higher values are associated with greater amplitude evenness among frequency bands over time, and greater numbers of vocalizing species in terrestrial studies. Lower values reflect prevalence of a contribution to a narrow band region exclusively. Conversely, AE measures the evenness of the acoustic activity by estimating the Gini coefficient on the signal in each 1 kHz band. Saturated soundscapes or acoustically rich habitats can decrease this metric. These two metrics are encountered less frequently in marine studies.
The Bioacoustic Index (BI) is a long-standing metric used in terrestrial acoustic monitoring but less prevalent in studies of marine environments. The BI was originally developed by Boelman et al. (2007) to determine avian abundance and increases in the BI measurement corresponds to increased measures of avian diversity. The Number of Peaks (NP) index tally’s the number of major spectral peaks in the data. In terrestrial environments, this value increases when habitats have a higher number of species or with certain types of single species sounds (Gasc et al., 2013). To our knowledge this metric has not been evaluated for marine-based studies.
The Normalized Difference Soundscape Index (NDSI) compares energy in two bands to provide an estimate of anthropogenic disturbance by finding the ratio of an anthrophony frequency band to a biophony frequency band (Boelman et al., 2007; Kasten et al., 2012). NDSI has been utilized in terrestrial acoustic studies, but to our knowledge has not been assessed for underwater environments (Bradfer-Lawrence et al., 2020; Ross et al., 2021). The NDSI index relies on the consistent nature of anthropogenic noise in separate frequency bands from biophony. In a terrestrial scenario where anthrophony occurs within a band of 1-2 kHz and biophony is expected to occur within 2-8 kHz, increased NDSI measurements would indicate higher biophony activity and low anthropogenic noise. We selected an anthrophony band of 10 Hz-1 kHz (despite the knowledge that some calls from large baleen whales existing in this band) and a biophony band of 1-32 kHz. We proceeded with these settings for anthrophony as most of the energy from vessel traffic occurs within this first 1,000 Hz bandwidth (Hatch et al., 2008; McKenna et al., 2012; Merchant et al., 2012). Calls from species such as blue, fin and humpback whales also occur within this bandwidth, so we anticipated that calculations associated with these sounds would be in discriminable from noise and would decrease NDSI measurements. Our anticipation was this metric would only be useful for those calls existing above 1 kHz.
Finally, the Total Entropy (H) metric combines a temporal diversity index and a frequency diversity index, which are generated by applying the Shannon diversity index to the amplitude envelope (Shannon, 1948; Sueur et al., 2008). Increased values are associated with greater evenness of the amplitude among frequency bands over time, or with soundscapes containing near-silent recordings. This metric is more prevalent in marine studies and in the literature is referred to as either the spectral entropy or the temporal entropy (Parks et al., 2014; Elise et al., 2019b).
2.4.2 Calculation of indices
Acoustic index measurements and statistical analysis were performed using R 4.0.5 (R Core Team, 2021). Measurements of ACI, AD, AE, BI, and NDSI were calculated from five-minute duration raw audio.wav files using the ‘soundecology’ package (v1.3.3; Villanueva-Rivera et al., 2018). Measurements of NP and H were calculated using the ‘seewave’ package (v2.2.0; Sueur et al, 2008). All indices were calculated using a Fast Fourier Transform (FFT) of 512, a minimum frequency of 10 Hz and a maximum frequency of 32 kHz (with the exception of the bands indicated for NDSI). The AD and AE calculations had a frequency step of 100 Hz, which was decidedafter sensitivity testing with several step sizes. We also used sensitivity testing to determine an appropriate dbFS threshold for each recorder to use with AD and AE calculations. These values are dependent on characteristics of the hydrophone and acoustic environment, necessitating this testing. We ultimately used a value of -50 dbFS for HYDBBA 103 and 106, and a value of -100 dbFS for HYDBBA 105. Given we are interested in persistent patters across recorders and not discrete values, we did not anticipate this difference would result in any problems in the interpretation.
3 Results
3.1 Acoustic detections
Marine mammals, passing ships, and sonar activity were identified in the dataset and the number of five-minute audio files containing each sound category varied for each sound category and recorder (Table 1). Differences in recording coverage during each month varied, resulting in differences in the total sample size of five-minute files for each site (shelf = 6,410 files, slope = 4,725 files, base of the slope = 7,101 files). Periods of vocalizing marine mammals occurred less frequently at the base of the slope (HYDBBA 103) as compared to the slope (HYDBBA 105) and the shelf (HYDBBA 106). Calls from delphinid species (not identifiable to species level), humpback whales (Megaptera novaeangliae) and fin whales (Balaenoptera physalus) were detected at all three sites. Delphinid calls predominantly consisted of burst pulses and could not be classified to species as they could have been produced from one of several species and visual validation was unavailable. Echolocation clicks from sperm whales (Physeter macrocephalus) were detected on the slope and at the base of the slope. Blue whale (Balaenoptera musculus) A and B calls and mid-frequency active sonar were detected on the recorder located on the shelf. Possible fish sounds were only detected at the base of the slope. Passing ships were detected at the slope and shelf sites. Within each set of recordings, all five-minute files where vocalizations were absent were included in the comparison (4188 – 6943 files). The combined dataset including information on marine mammal occurrence, call measurement and acoustic indices can be found in Supplementary Table 1.
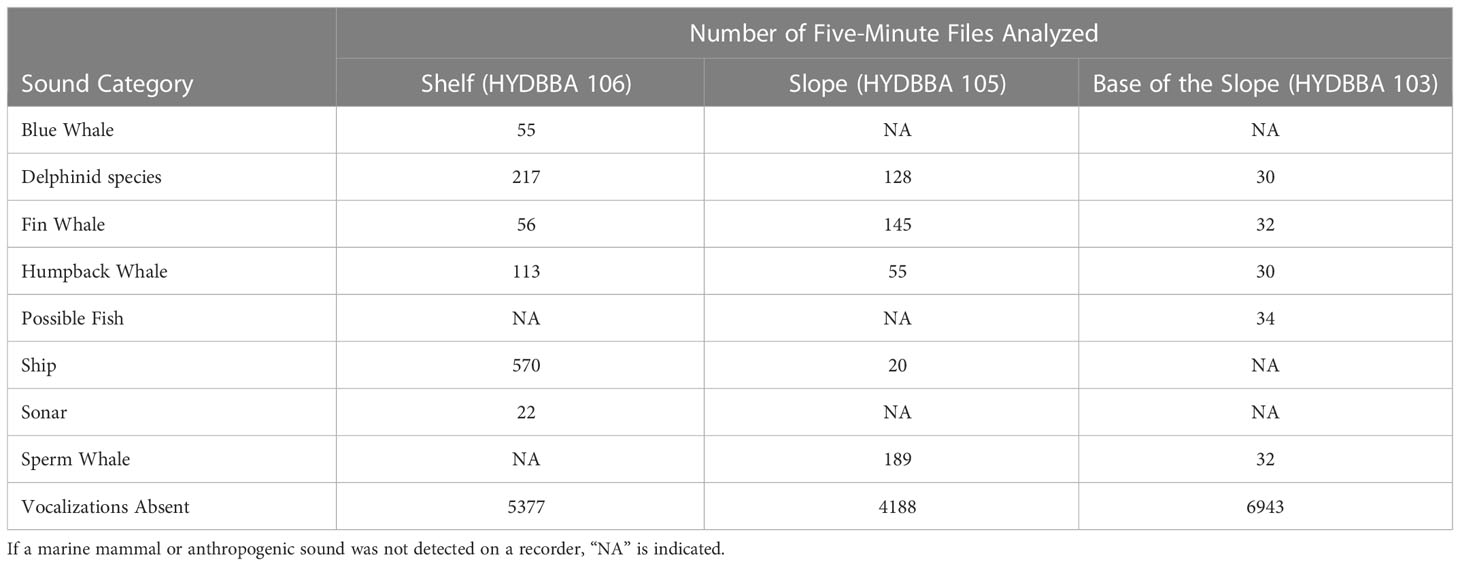
Table 1 Summary of five-minute file sample sizes used in the analysis for each recorder by sound category.
3.2 Biophony effects
The acoustic index measurements varied in association with calls produced by several marine mammal species and anthropogenic noise sources at each of the OOI recorder sites (Figures 2-5; Supplementary Figures 1, 2). ACI measurements from five-minute wav files notably increased during periods of vocalizing delphinid species and sperm whales (Figure 2). AD and AE resulted in complementary, opposite measurements and are thus summarized collectively. These indices responded inconsistently to different marine mammal calls, and were more obscure in terms of the measurement response (Table 2; Supplementary Figures 1, 2). BI measurements decreased in associationwith to sperm whale echolocation clicks for both the recorders (Figure 3). Humpback whales had a smaller significant effect on BI at all three sites exhibiting a slightly higher distribution of values than delphinids, fin whales and blue whales. The NDSI varied for all marine mammal groups across the three recorders (Figure 4). Humpback whale calls resulted in a reduction ofNDSI measurement on the slope and at the base of the slope, but were insignificant on the shelf. There was a notable reduction in the measurements associated with the possible fish category at the base of the slope. The NP and H measurements also exhibited variable responses to calls from marine mammals (Supplementary Figure 3 and Figure 5, respectively). Although differences in NP measurements were noted for several species, the increase or decrease in measurements as compared to periods without vocalizations was inconsistent at each recorder and displayed no clear trend in the data. The H measurements increased in association with blue whale or delphinid calls and decreased in connection to the possible fish sounds (Figure 5).
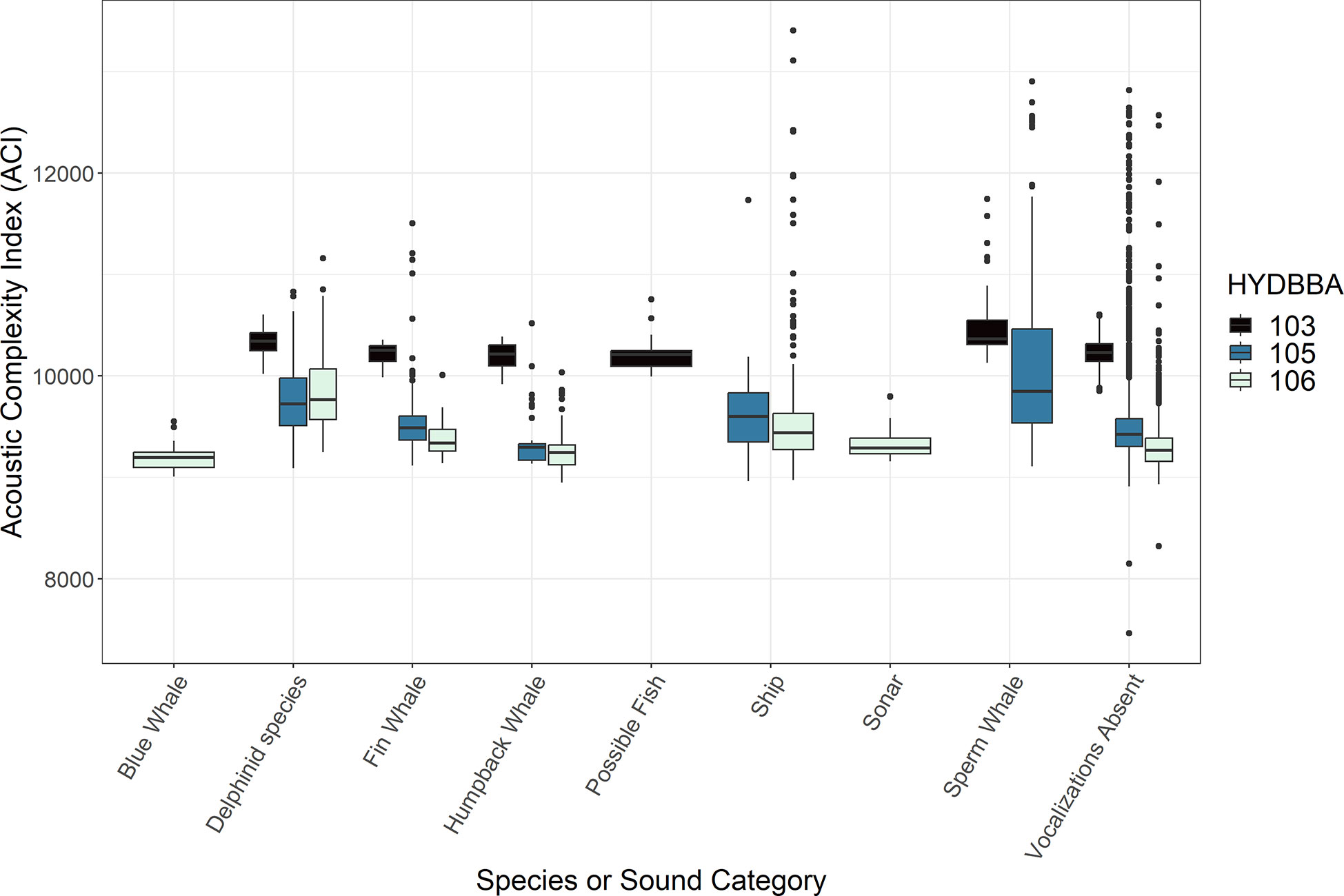
Figure 2 Box plots representing measurements of Acoustic Complexity Index (ACI) associated with underwater sounds. Data are organized by recorders located on the shelf (106), the slope (105) and at the base of the slope (103). Marine mammal species and human-made sounds vary for each recorder resulting in some sound types with only one or two box plots. All recorders include a plot for data that contained no annotated sound (labeled ”Vocalizations Absent“).
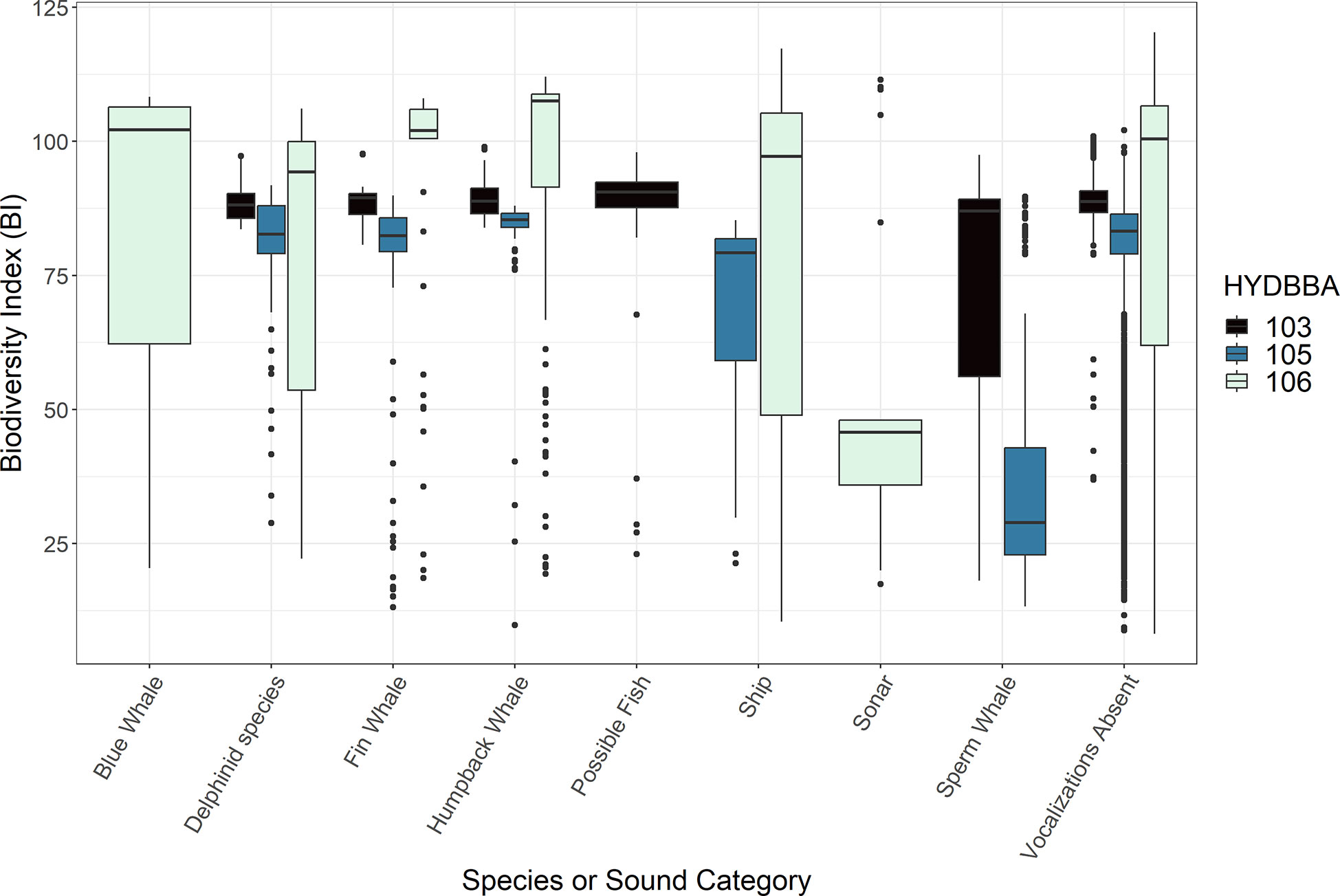
Figure 3 Box plots representing measurements of Bioacoustic Index (BI) associated with underwater sounds. Data are organized by recorders located on the shelf (106), the slope (105) and at the base of the slope (103). Marine mammal species and human-made sounds vary for each recorder resulting in some sound types with only one or two box plots. All recorders include a plot for data that contained no annotated sound (labeled ”Vocalizations Absent“).
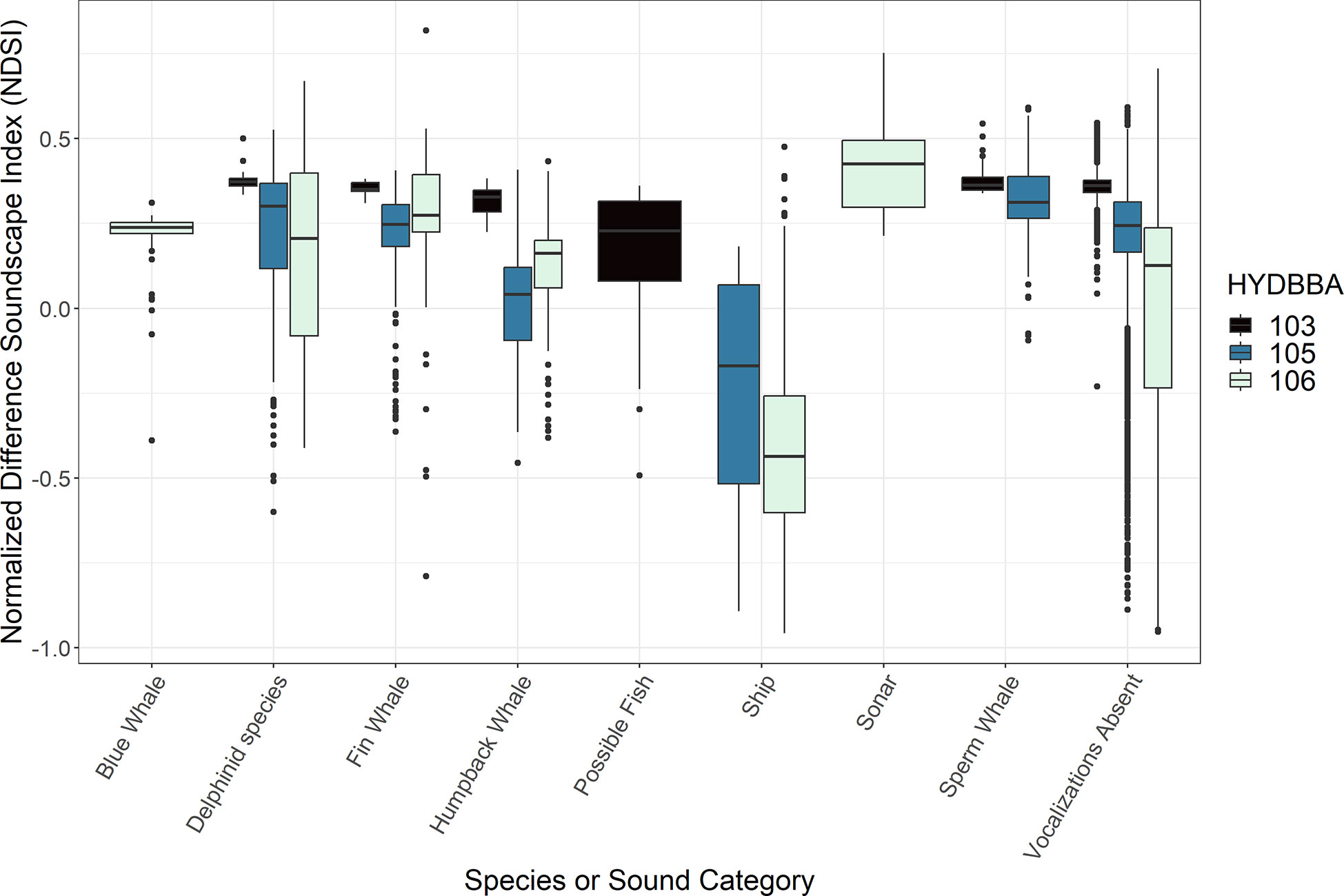
Figure 4 Box plots representing measurements of Normalized Difference Soundscape Index (NDSI) associated with underwater sounds. Data are organized by recorders located on the shelf (106), the slope (105) and at the base of the slope (103). Marine mammal species and human-made sounds vary for each recorder resulting in some sound types with only one or two box plots. All recorders include a plot for data that contained no annotated sound (labeled ”Vocalizations Absent“).
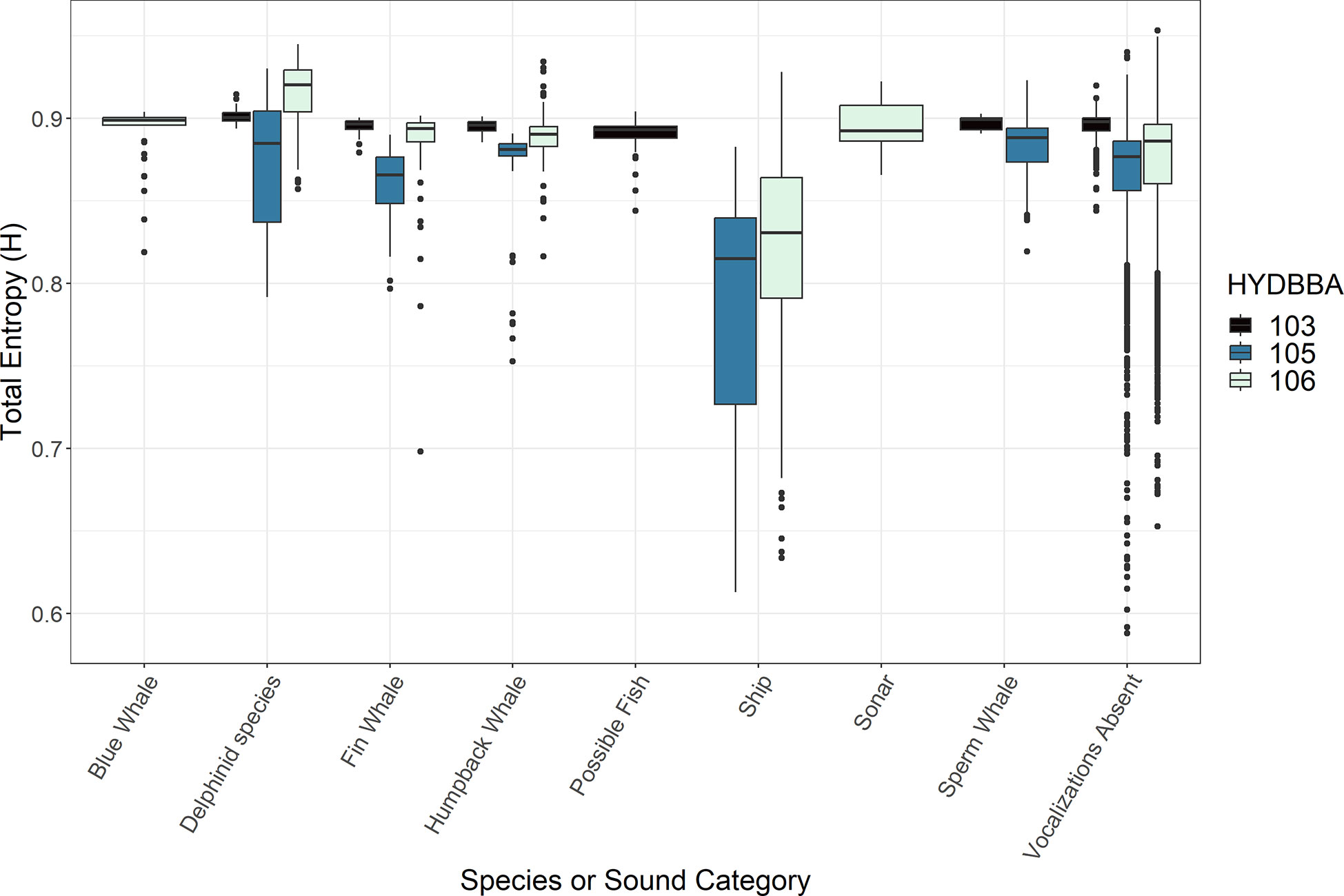
Figure 5 Box plots representing measurements of Total Entropy (H) associated with underwater sounds. Data are organized by recorders located on the shelf (106), the slope (105) and at the base of the slope (105). Marine mammal species and human-made sounds vary for each recorder resulting in some sound types with only one or two box plots. All recorders include a plot for data that contained no annotated sound (labeled ”Vocalizations Absent“).
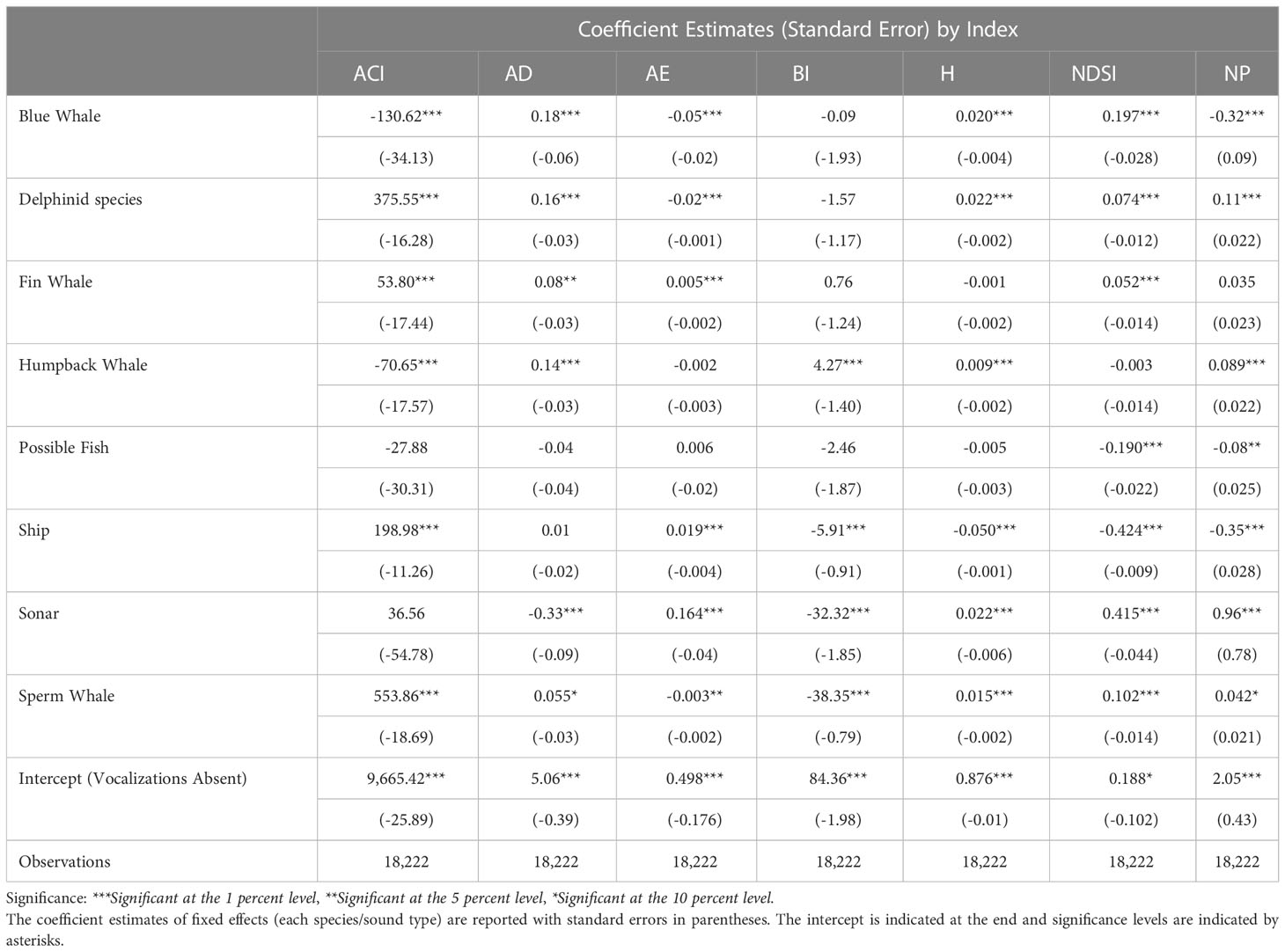
Table 2 Generalized linear mixed effects model evaluating the effects of species on each index incorporating recorder as a random effect.
The results of the generalized linear mixed-effects models provide additional evidence of the contribution of each species or species groups to the variability of the dependent variable (each index; Table 2). For ACI, all categories but “Possible Fish” and “Sonar” indicate they significantly contribute as a predictor variable in the model. Delphinid species and sperm whales demonstrate a higher likelihood of increasing this metric. For AD and AE, although blue whales, delphinid species, fin whales, and sperm whales indicate a statistically significant effect on the model, the coefficient estimate is relatively low for these indices. Only humpback whales and sperm whales significantly contribute to the variation for BI, with the sperm whales resulting in a large negative coefficient. The model for H model does indicate significant differences between many species, but they have a lower likelihood of increasing or decreasing this measurement. All but humpback whales have the potential to predict the NDSI variable, although blue whales are more likely to increase this value while possible fish are more likely to decrease it. Finally, the NP measurements are significantly predicted by all species but fin whales, although the likelihood of this impact is nominal.
3.3 Call characteristic correlations
In assessing the difference in ACI, BI and NDSI measurements for periods with sperm whale clicks and periods without vocalizations, we observed an incremental differentiation when qualitatively parsing data into categories of loud, moderate, and quiet echolocation clicks (Figure 6). The BI measurements were dramatically reduced as compared to periods without vocalizations for loud and moderate clicks. To a lesser extent, the same trend was observed for the ACI and NDSI values particularly for those echolocation clicks that were qualitatively classified as “loud” by the analyst.
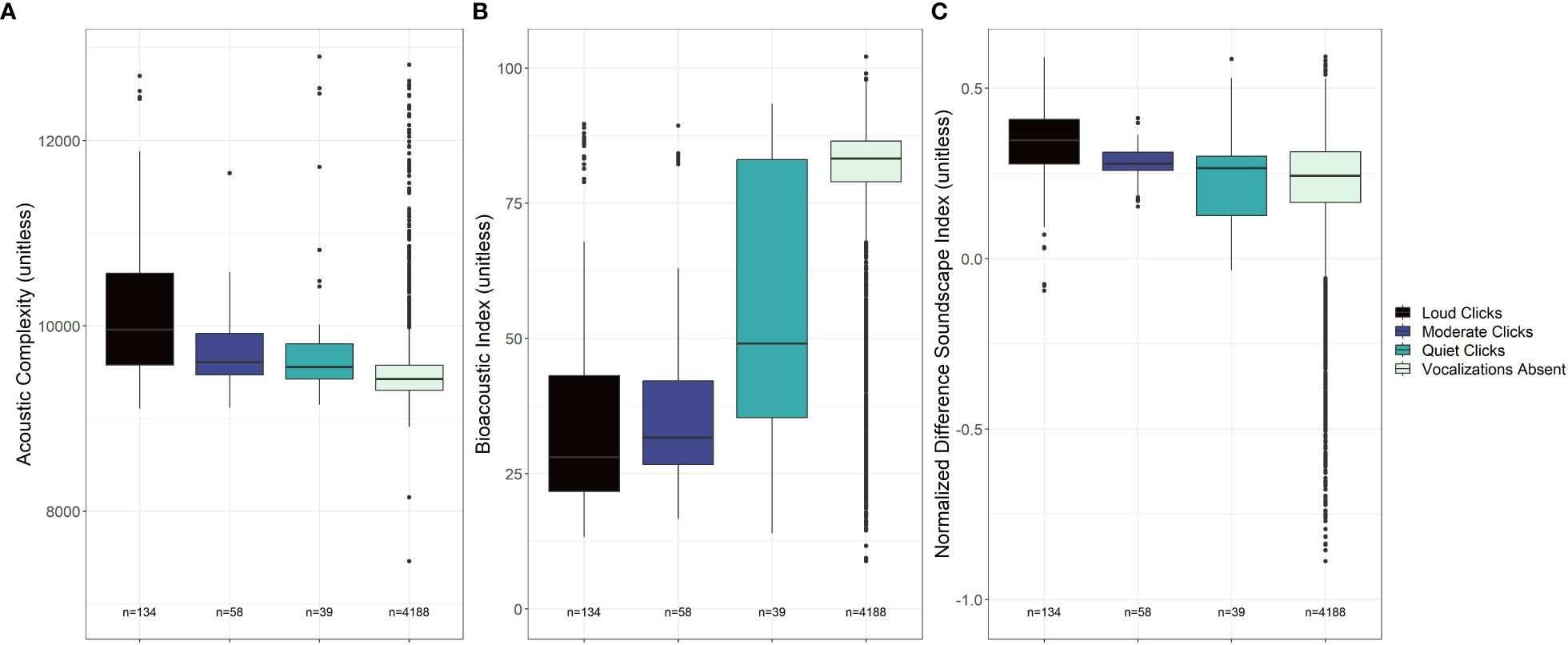
Figure 6 Box plots of measurements from three acoustic indices for sperm whale clicks parsed out by amplitude quality. Qualitative groups of clicks include ”Loud clicks,“ ”Moderate clicks,“ and ”Quiet clicks.“ Periods lacking any echolocation clicks (labeled ”Vocalizations Absent“) are also included for reference in all three figures. Sample sizes for each click quality level and period lacking vocalizations are indicated. The acoustic indices in this figure include the Acoustic Complexity Index, the Bioacoustic Index, and the Normalized Difference Soundscape Index.
We explored the correlation of call characteristics of sperm whale for both ACI and BI (Figure 7). For delphinid calls we only evaluated data that contained “contour” detections of burst pulses, and did not include measurements of echolocation clicks in the correlation comparison. Annotated echolocation clicks from delphinids resulted in too low of a sample size to evaluate independently, and burst pulses make up the majority of the annotations for the delphinids in this region. Although additional significant relationships were found for other species and indices, these two indices provided consistent trends in the data distribution from multiple recorders. Figure 7 indicates the Spearman correlation (ρ) and bootstrapped confidence intervals for each sperm whale call characteristic and ACI and BI. The 10 dB bandwidth (ρ103 = 0.41, ρ105 = 0.37), click amplitude (ρ103 = 0.69, ρ105 = 0.51), peak frequency (ρ103 = 0.63, ρ105 = 0.30), and Total Clicks (number of clicks per 5-min file) (ρ103 = 0.47, ρ105 = 0.61), for ACI values of sperm whale clicks had a moderate to strong, positive correlation with ACI at both sites. Confidence intervals were greater for the recorder at the base of the slope due to smaller sample size, but were consistent with the trends of the slope recorder (105) (Figure 7). For the BI measurements, the 10 dB bandwidth (ρ103 = -0.33, ρ105 = -0.43), click amplitude (ρ103 = -0. 39, ρ105 = -0.65), peak frequency (ρ103 = -0.37, ρ105 = -0.43), and Total Clicks (number of clicks per 5-min file) (ρ103 = -0.34, ρ105 = -0.55) all indicate a moderate to strong negative correlation with sperm whale clicks. Of these metrics, the click amplitude and total number of clicks per 5-min file suggest the strongest influence.
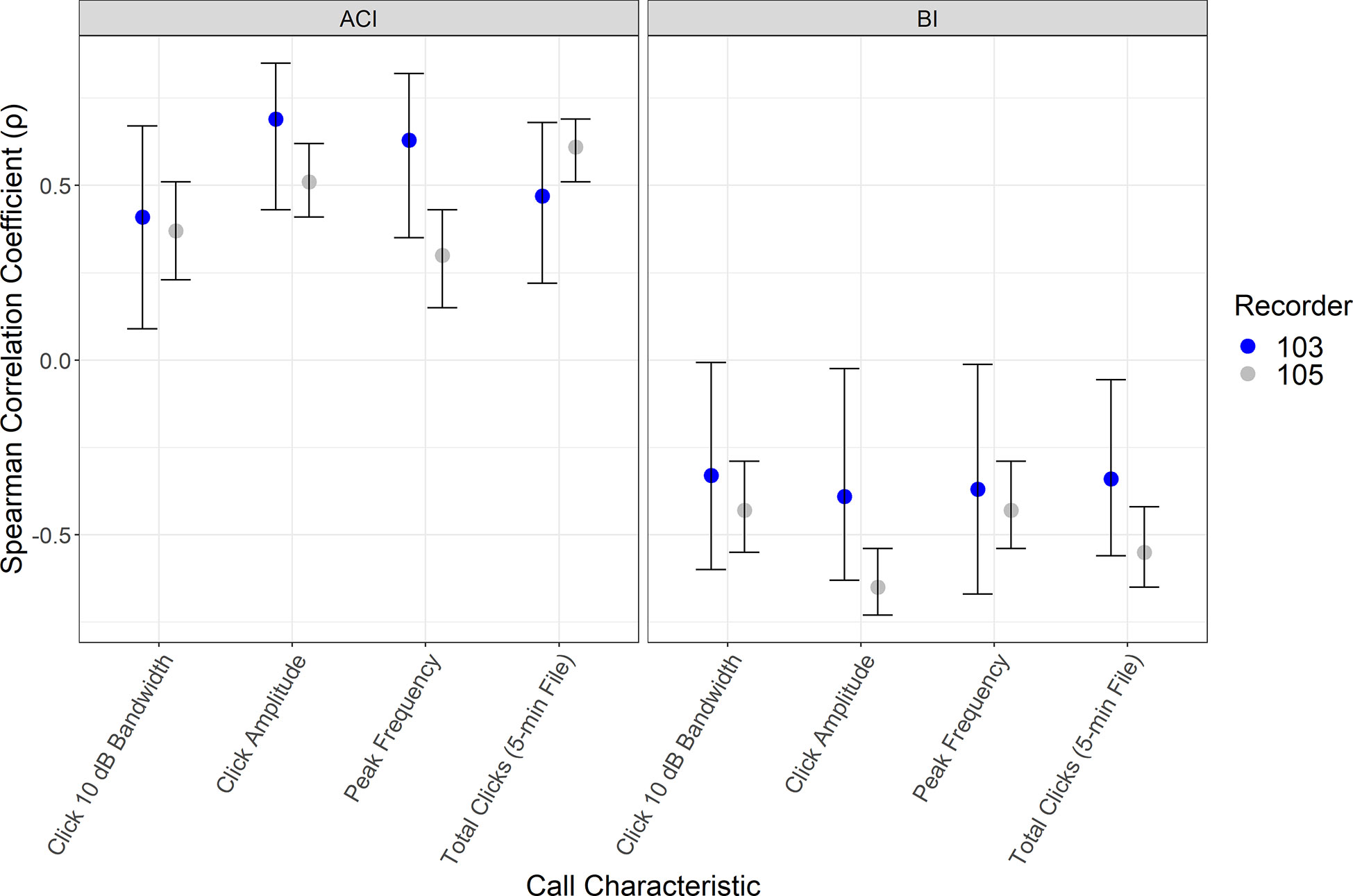
Figure 7 Results of the Spearman correlation coefficient (ρ) and bootstrapped confidence intervals comparing call characteristics of sperm whales to Acoustic Complexity Index and Bioacoustic Index. Mean measurements calculated per 5-minute interval of sperm whale echolocation clicks characteristics including 10 dB bandwidth, click amplitude, peak frequency, and total number of clicks (per 5-min file) are reported for the recorder on the base of the slope (103) and the slope (105).
For delphinids, inconsistency across in the correlation results, closer to zero correlation values for some call parameters, and wide confidence intervals do not suggest any specific influence on ACI (Figure 8). These results were unexpected given the increase in ACI observed from the descriptive figures. Supplementary Tables 2 and 3 include the correlation coefficient values and confidence intervals calculated in this analysis.
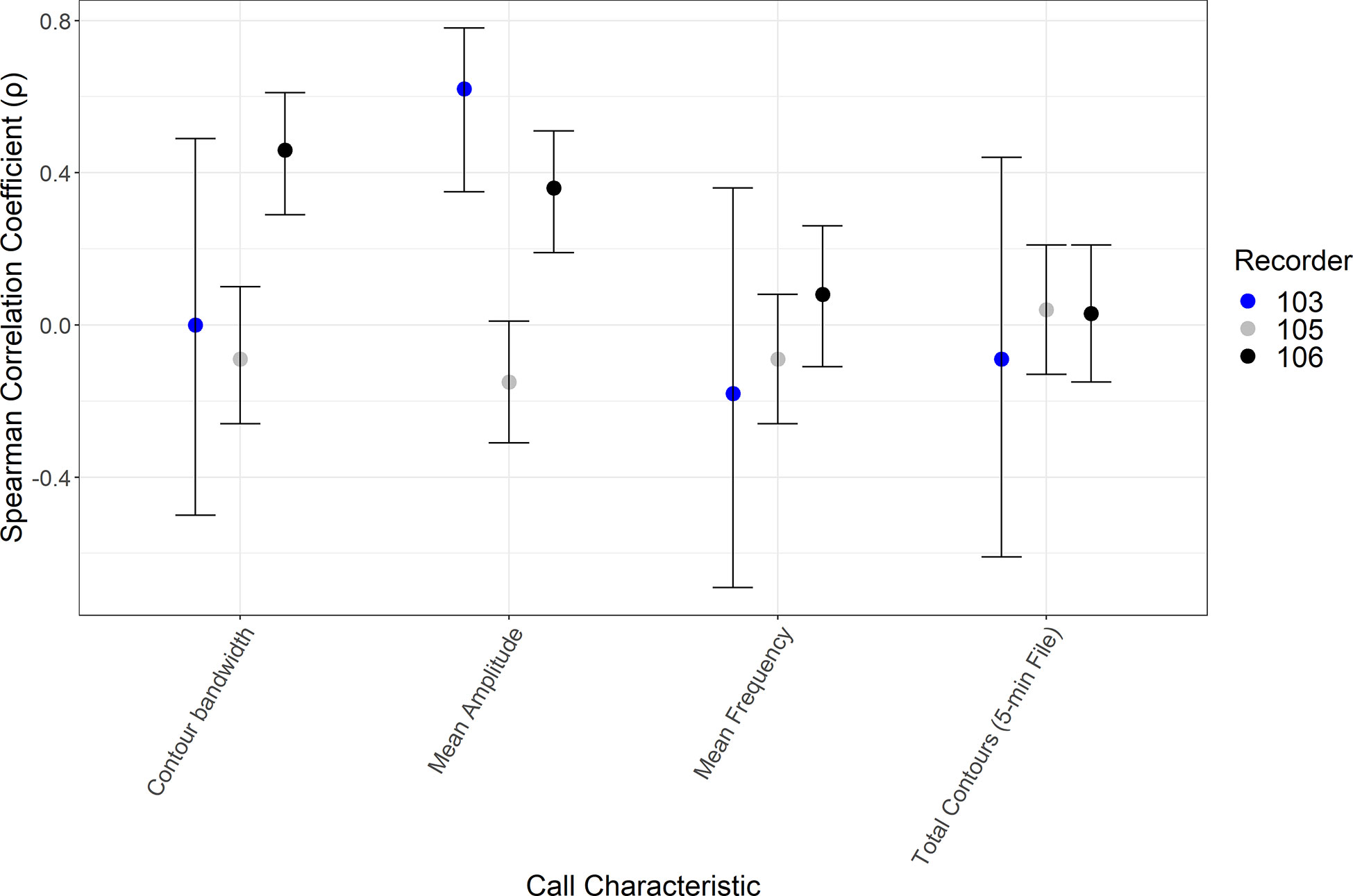
Figure 8 Results of the Spearman correlation coefficient (ρ) and bootstrapped confidence intervals comparing call characteristics of delphinid species to Acoustic Complexity Index. Characteristics of burst pulsed calls from delphinid species including mean measurements (per five-minute file) of contour bandwidth, call amplitude, mean frequency and total number of calls are reported for the recorders at the base of the slope (103), the slope (105), and the shelf (106).
3.4 Anthrophony effects
Annotated anthrophony consisted of ships on the shelf and the slope and mid-frequency active sonar pings at the shelf. Five-minute files containing noise from passing ships resulted in an increase of ACI as compared to periods without vocalizations (Figure 2). ACI did not change significantly during periods with sonar, although sample size was low (Table 1). Sonar was noticeably lower for AD and higher for AE Supplementary Figures 1, 2). Similar to sperm whale observations, sonar was associated with reduced measurement of BI in comparison to periods where vocalizations were absent Figure 3). Ships had a negligible significant difference from quiet periods for BI, but again were noticeably different from quiet periods on the slope.
The NDSI measurements were greatly reduced for passing ships as compared to periods lacking vocalizations at the shelf and slope recorders (Figure 4). For sonar, large increased difference in NDSI was observed as compared to periods where vocalizations were absent A similar trend was observed for the NP measurements for both passing ships and sonar (Supplementary Figure 3). Sonar periods are associated with increased measurements of H as compared to periods without vocalizations, however passing ships were dramatically reduced from quiet periods on the shelf as well as along the slope (Figure 5).
The results of the generalized linear mixed-effects models for passing ships and sonar indicate interesting influences on several index variables (Table 2). Passing ships have a small likelihood of increasing ACI, but a larger likelihood of decreasing BI and decreasing BI and NDSI. The sonar sound category had a large positive effect on AE and NDSI, and had a large negative coefficient relating to BI. The likelihood of sonar and passive ships increasing or decreasing NDSI respectively is far greater than the intercept (Vocalizations Absent).
4 Discussion
In this study we were able to explore how several soundscape metrics vary in response to underwater sounds from marine mammals and anthropogenic noise. The aim of this effort was to address the consistent recommendations from the terrestrial and marine acoustic ecologists by exploring how acoustic indices respond to marine mammals sounds (Mooney et al., 2020; Pieretti and Danovaro, 2020). Unexpectedly, we also uncovered information relating to anthrophony and acoustic indices. Collectively, these results provide novel information to the field of acoustic ecology.
Biodiversity is not explicitly reflected by any single acoustic index measurements, unlike some results from terrestrial studies. For instance, Dröge et al. (2021) found a positive correlation between the Acoustic Diversity Index and the Acoustic Entropy and avian species richness. However, their study also indicated a significantly negative response in the Acoustic Complexity Index, which is contrary to results from other studies of avian species diversity (Towsey et al., 2014). Our results suggest a dynamic relationship between underwater sounds and acoustic indices, and one that is not driven by any one sound characteristic. Minello et al. (2021) suggested that soniferous species may not all have equal footing as acoustic bioindicator species for ecosystem health monitoring. Our results certainly reflect this sentiment, as was seen in the relationship between sperm whales and delphinids with ACI and BI. Further observations, limitations and applications of this knowledge are outlined below.
4.1 Strong responses to underwater sounds
Index measurements are recorder dependent making them incomparable in terms of exact values, but this study results in several trends in the response of some of these measurements to calls from marine mammals and anthropogenic noise sources. We therefore focus our interpretation of these results on sound categories that exhibited a consistent, strong effect on the measurements of a select set of indices. Given the difference in instrument and ambient noise on the shelf, slope, and base of the slope recorders, these robust trends are anticipated to persist on different instruments located in similar acoustic environments:
1. Sperm whale echolocation clicks influence two indices with the increase in ACI and decrease in BI displayed a gradual shift in values when click quality was parsed out strong, loud clicks diverging most significantly from the periods lacking vocalizations which could be due to a combined contribution of click amplitude, 10 dB bandwidth, peak frequency and the quantity of calls produced. To a lesser extent, sperm whale clicks also resulted in increased NDSI measurements. These trends were observed consistently for both the slope and the base of the slope site. We believe information regarding the combination of ACI, BI and NDSI associated with clicks from sperm whales could serve as a means of predicting their occurrence within long-term acoustic data. However, one note of caution relates to the combination of bandwidth and index parameter settings as research suggests that fluctuation of ACI values can occur with use of finer scale frequency resolution (Bohnenstiehl et al., 2018).
2. The burst pulse type calls produced by delphinid acoustic groups in this region increased measurements of ACI regardless of ambient conditions of the recorder, although none of the call characteristics evaluated in this study appeared to be exclusively responsible for this shift in the measurements. We found it particularly interesting that, similar to results observed in relation to peak chorusing of fish by Staaterman et al. (2017), an increase in the number of calls, particularly at the acoustically intense recorder site at the shelf had almost no correlation to ACI measurements. While low sample size of five-minute files prevented us from evaluating the correlation of ACI measurements with echolocation click characteristics, clicks in combination with burst pulses could be responsible for increases in ACI and should be further explored. Should an index exclusive approach to evaluating a dataset were to be employed, burst pulses could be differentiated from sperm whales through measurements of the BI, as the measurements for delphinids were similar to periods without vocalizations. Although other metrics do show some possible trends as well (e.g., increased measurements of H at all recorders), the magnitude of this significant difference varied too much to make it a reliable indicator of delphinids.
3. Passing ships exhibited a strong, consistent influence on both the NDSI and H measurements (Figure 4, 5). For both indices, measurements were greatly reduced in comparison to periods with and without detections from other sound categories. The measurements of H are consistent with Parks et al. (2014) regarding their association with low frequency baleen whale calls, but to our knowledge the drastic decrease in this metric during periods of passing ships is a new finding. The only potential overlap in downward trending NDSI measurement would be with the possible fish sounds, however ships were not detected on the recorder at the base of the slope, so could not be compared. The frequency band used to define anthrophony in this index was 10-1000 Hz, which we expected to be negatively impacted by biophony vocalizing within this bandwidth. Differentiation of ships from humpback whale calls could be accomplished in a scenario where indices are combined because humpback whale calls had no influence on H measurements. Assessing the combination of NDSI and H in long term recordings could be a valuable method for detecting passing ships. However, an evaluation of the degree of NDSI and H response with varying distance from recorder or type of ship should be performed, as it was not done so within this study.
We did observe additional trends in the data that could be used as a further means of rapidly determining periods of biophony and anthrophony in a dataset, however the data were either limited to one site (e.g., blue whales) or require a complex machine learning approach to discriminate which combination of indices can provide meaningful information. Based on the efforts of this study, we suggest that sperm whales and delphinid species could serve as representative acoustic indicators in coastal ecosystem monitoring efforts that incorporate acoustic indices.
Anthropogenic sources of noise in this dataset provided information beyond the well-established relationship between intensity indices such as sound pressure level and ship traffic. Additionally, we found sonar to have a significant influence on several indices, however sample size of five-minute files containing sonar were low (n=22) and only occurred at the shelf recorder. A larger dataset may elucidate trends in this anthropogenic noise source. Although noise filtering was suggested by several studies as a means of focusing on biodiversity of calling species, we suggest this might mask the effects of louder signals on index measurement response.
Additionally, we recommend evaluating the effects of call type and rate of a variety of fish species to better understand their relationship with acoustic indices. Armed with a more robust understanding of key influential sources of biophony, marine ecologists can more effectively determine those combinations of acoustic indices that contribute to understanding biodiversity in ocean environments.
4.2 Study limitations
To our knowledge, this study is the first to compare acoustic indices across large bandwidth (10 Hz to 32 kHz) data for assessing multiple types of underwater sounds. However, the integration across such a large bandwidth may be marginalizing the low frequency calls from baleen whales within these acoustic indices results. It is likely that incorporating a series of calculations exclusively on a lower bandwidth could result a larger response from acoustic indices, as was evident in Parks et al. (2014). We suggest that bandwidth possibly impacts acoustic index associations as it pertains to both low frequency calling animals and cases where bandwidths are much greater than the 32 kHz of this study. We could not evaluate calls from species that produce vocalizations above the 32 kHz bandwidth, such as beaked whales and harbour porpoise. Given the influence on measurements for several indices in response to sperm whale echolocation clicks, evaluating other echolocating species would be beneficial. Another caveat is that some species did not produce vocalizations at each recorder site, limiting our ability to compare between different acoustic recorder environments.
The results are further limited as they were collected in the same region of the northwest Pacific Ocean and used data from the same type of instrumentation. However, the variability in the recorders in terms of instrument noise from the hydrophone or co-located sensors and acoustic environment provided more confidence in those persistent trends mentioned above. Additional analysis of data from a different region and using different instrumentation would assist in further validation of these results.
4.3 Large-scale monitoring applications
The concept of utilizing acoustic indices to characterize the biophony diversity found in acoustic environments is not novel, nor is the acknowledgement that further research is required to improve the reliability of these measurements as indicators (Sueur et al., 2014). Roca and Van Opzeeland (2020) have already tested incorporating a suite of acoustic index measurements in machine learning methods for monitoring acoustic environments using large acoustic datasets. We anticipate this work will contribute to current efforts seeking to incorporate acoustic indices in autonomous, machine learning methods for continuous environmental monitoring (Sethi et al., 2019; Williams et al., 2022).
Implementing meaningful large-scale ecosystem health monitoring has additional challenges. Recommendations from the acoustic community suggest that fluctuations in oceanographic measurements associated with indicator species may elucidate periods of ecological disturbances (Pieretti and Danovaro, 2020; Minello et al., 2021). To truly assess this would require the co-location of additional physical and chemical oceanographic data as is the case with the OOI cabled array sensors. Mooney et al. (2020), indicated the need to record over sufficient timescales to observe deviations from typical seasonal and interannual variability in a marine habitat. Therefore, combining acoustic indices as indirect, biological indicators in concert with physical and chemical monitoring over long time periods, such as is possible with the OOI Coastal Endurance and Continental Margin arrays would be an ideal scenario for accurately monitoring ecosystem health. In this context, acoustic indices could provide a solution to the issue of underutilized passive acoustic data and contribute to biodiversity conservation within marine ecosystems.
Data availability statement
The original contributions presented in the study are included in the article/Supplementary Material. Further inquiries can be directed to the corresponding author.
Author contributions
EF conceived the study and coordinated the project effort. EF and HC contributed to the annotation of acoustic events within the dataset. TS contributed to the call extraction of the analysis used to link each type of sound to the acoustic indices. EF conducted the analysis of acoustic indices. All authors contributed to the article and approved the submitted version.
Funding
This project was made possible through in-kind donation of time from all manuscript authors. The data was obtained from the publicly available, National Science Foundation supported Ocean Observatories Initiative’s network of hydrophones along the Coastal Endurance and Cabled Arrays off of Newport, Oregon.
Acknowledgments
We would like to express our thanks and gratitude to the National Science Foundation funded Ocean Observatories Initiative (OOI) for the provision of the data used in this analysis to the general public. The tireless efforts of Orest Kawka from the University of Washington in responding to our many questions regarding the acoustic data collection were greatly appreciated. Craig Risien of Oregon State University was a consistent and informative contact regarding OOI resources. Finally, we would like to extend a special thanks to the OOI Facilities Board for their support of our work and provision of the Larry P. Atkinson Travel Fellowship to present results of this study at the Society for Marine Mammalogy 2022 conference. We are specifically appreciative of Annette DeSilva of the OOI Facilities Board and Darlene Crew Trist of Woods Hole Oceanographic Institution for their promotion of our related efforts and support of analytical endeavors.
Conflict of interest
Author TS was employed by LLC Contracted to Southwest Fisheries Science Center, Richland.
The remaining authors declare that the research was conducted in the absence of any commercial or financial relationships that could be construed as a potential conflict of interest.
Publisher’s note
All claims expressed in this article are solely those of the authors and do not necessarily represent those of their affiliated organizations, or those of the publisher, the editors and the reviewers. Any product that may be evaluated in this article, or claim that may be made by its manufacturer, is not guaranteed or endorsed by the publisher.
Supplementary material
The Supplementary Material for this article can be found online at: https://www.frontiersin.org/articles/10.3389/fmars.2023.1025464/full#supplementary-material
Supplementary Table 1 | Summary of all combined annotations and acoustic indices results used to perform analysis from three recorders. This version includes all periods where more than one species overlapped in a five-minute period (which were discounted in the analytical portion). This dataset also includes only those observations where the call count was at or greater than the mean call counter across all detections (by species).
Supplementary Table 2 | Spearman correlation coefficient and confidence interval results for delphinid call characteristics at three recorders.
Supplementary Table 3 | Spearman correlation coefficient and confidence interval results for sperm whale call characteristics for two indices and at two recorders.
Supplementary Figure 1 | Box plots representing measurements of The Number of Frequency Peaks (NP) associated with underwater sounds. Data are organized by recorders located on the shelf (106), the slope (105) and at the base of the slope (103). Marine mammal species and human-made sounds vary for each recorder resulting in some sound types with only one or two box plots. All recorders include a plot for data that contained no annotated sound (labeled “Vocalizations Absent”).
Supplementary Figure 2 | Box plots representing measurements of The Number of Frequency Peaks (NP) associated with underwater sounds. Data are organized by recorders located on the shelf (106), the slope (105) and at the base of the slope (103). Marine mammal species and human-made sounds vary for each recorder resulting in some sound types with only one or two box plots. All recorders include a plot for data that contained no annotated sound (labeled “Vocalizations Absent”).
Supplementary Figure 3 | Box plots representing measurements of Acoustic Evenness (AE) associated with underwater sounds. Data are organized by recorders located on the shelf (106), the slope (105) and at the base of the slope (103). Marine mammal species and human-made sounds vary for each recorder resulting in some sound types with only one or two box plots. All recorders include a plot for data that contained no annotated sound (labeled “Vocalizations Absent”).
References
Alcocer I., Lima H., Sugai L. S. M., Llusia D. (2022). Acoustic indices as proxies for biodiversity: a meta-analysis. Biol. Rev. 97 (6), 2209–2236. doi: 10.1111/brv.12890
Aniceto A. S., Ferguson E. L., Pedersen G., Tarroux A., Primicerio R. (2022). Temporal patterns in the soundscape of a Norwegian gateway to the Arctic. Sci. Rep. 12 (1), 1–15. doi: 10.1038/s41598-022-11183-y
Barlow J., Taylor B. L. (2005). Estimates of sperm whale abundance in the northeastern temperate pacific from a combined acoustic and visual survey. Mar. Mammal Sci. 21 (3), 429–445. doi: 10.1111/j.1748-7692.2005.tb01242.x
Bates D., Mächler M., Bolker B., Walker S. (2015). Fitting linear mixed-effects models using lme4. J. Stat. Software 67 (1), 1–48. doi: 10.18637/jss.v067.i01
Benocci R., Asnaghi E., Bisceglie A., Lavorano S., Galli P., Roman H. E., et al. (2022). Method for assessing the soundscape in a marine artificial environment. Sustainability 14 (16), 10359. doi: 10.3390/su141610359
Benoit-Bird K. J., Battaile B. C., Heppell S. A., Hoover B., Irons D., Jones N., et al. (2013). Prey patch patterns predict habitat use by top marine predators with diverse foraging strategies. PLoS One 8 (1), e53348. doi: 10.1371/journal.pone.0053348
Bertucci F., Parmentier E., Lecellier G., Hawkins A. D., Lecchini D. (2016). Acoustic indices provide information on the status of coral reefs: An example from moorea island in the south pacific. Sci. Rep. 6 (1), 1–9. doi: 10.1038/srep33326
Boelman N. T., Asner G. P., Hart P. J., Martin R. E. (2007). Multi-trophic invasion resistance in Hawaii: bioacoustics, field surveys, and airborne remote sensing. Ecol. Appl. 17 (8), 2137–2144. doi: 10.1890/07-0004.1
Bohnenstiehl D. R., Lyon R. P., Caretti O. N., Ricci S. W., Eggleston D. B. (2018). Investigating the utility of ecoacoustic metrics in marine soundscapes. J. Ecoacoustics 2 (2), 1. doi: 10.22261/JEA.R1156L
Bolgan M., Amorim M. C. P., Fonseca P. J., Di Iorio L., Parmentier E. (2018). Acoustic complexity of vocal fish communities: A field and controlled validation. Sci. Rep. 8 (1), 1–11. doi: 10.1038/s41598-018-28771-6
Bradfer-Lawrence T., Bunnefeld N., Gardner N., Willis S. G., Dent D. H. (2020). Rapid assessment of avian species richness and abundance using acoustic indices. Ecol. Indic. 115, 106400. doi: 10.1016/j.ecolind.2020.106400
Browning E., Gibb R., Glover-Kapfer P., Jones K. E. (2017). Passive acoustic monitoring in ecology and conservation. Woking, UK, WWF-UK: WWF Conservation Technology Series 1 (2), 76. doi: 10.25607/OBP-876
Davies B. F., Attrill M. J., Holmes L., Rees A., Witt M. J., Sheehan E. V. (2020). Acoustic complexity index to assess benthic biodiversity of a partially protected area in the southwest of the UK. Ecol. Indic. 111, 106019. doi: 10.1016/j.ecolind.2019.106019
Dröge S., Martin D. A., Andriafanomezantsoa R., Burivalova Z., Fulgence T. R., Osen K., et al. (2021). Listening to a changing landscape: Acoustic indices reflect bird species richness and plot-scale vegetation structure across different land-use types in north-eastern Madagascar. Ecol. Indic. 120, 106929. doi: 10.1016/j.ecolind.2020.106929
Duarte M. H. L., Sousa-Lima R. S., Young R. J., Farina A., Vasconcelos M., Rodrigues M., et al. (2015). The impact of noise from open-cast mining on Atlantic forest biophony. Biol. Conservation 191, 623–631. doi: 10.1016/j.biocon.2015.08.006
Elise S., Bailly A., Urbina-Barreto I., Mou-Tham G., Chiroleu F., Vigliola L., et al. (2019a). An optimised passive acoustic sampling scheme to discriminate among coral reefs’ ecological states. Ecol. Indic. 107, 105627. doi: 10.1016/j.ecolind.2019.105627
Elise S., Urbina-Barreto I., Pinel R., Mahamadaly V., Bureau S., Penin L., et al. (2019b). Assessing key ecosystem functions through soundscapes: A new perspective from coral reefs. Ecol. Indic. 107, 105623. doi: 10.1016/j.ecolind.2019.105623
Erbe C., Verma A., McCauley R., Gavrilov A., Parnum I. (2015). The marine soundscape of the Perth canyon. Prog. Oceanography 137, 38–51. doi: 10.1016/j.pocean.2015.05.015
Farina A. (2019). Ecoacoustics: A quantitative approach to investigate the ecological role of environmental sounds. Mathematics 7 (1), 21. doi: 10.3390/math7010021
Gasc A., Sueur J., Pavoine S., Pellens R., Grandcolas P. (2013). Biodiversity sampling using a global acoustic approach: Contrasting sites with microendemics in new Caledonia. PLoS One 8 (5), e65311. doi: 10.1371/journal.pone.0065311
Gibb R., Browning E., Glover-Kapfer P., Jones K. E. (2019). Emerging opportunities and challenges for passive acoustics in ecological assessment and monitoring. Methods Ecol. Evol. 10 (2), 169–185. doi: 10.1111/2041-210X.13101
Gillespie D., Caillat M., Gordon J., White P. (2013). Automatic detection and classification of odontocete whistles. J. Acoustical Soc. America 134 (3), 2427–2437. doi: 10.1121/1.4816555
Gillespie D., Mellinger D. K., Gordon J., McLaren D., Redmond P., McHugh R., et al. (2009). PAMGUARD: Semiautomated, open source software for real-time acoustic detection and localization of cetaceans. J. Acoustical Soc. America 125 (4), 2547–2547. doi: 10.1121/1.4808713
Halpern B. S., Longo C., Scarborough C., Hardy D., Best B. D., Doney S. C., et al. (2014). Assessing the health of the US West coast with a regional-scale application of the ocean health index. PLoS One 9 (6), e98995. doi: 10.1371/journal.pone.0098995
Hatch L., Clark C., Merrick R., Van Parijs S., Ponirakis D., Schwehr K., et al. (2008). Characterizing the relative contributions of large vessels to total ocean noise fields: a case study using the Gerry e. studds stellwagen bank national marine sanctuary. Environ. Manage. 42 (5), 735–752. doi: 10.1007/s00267-008-9169-4
Haver S. M., Gedamke J., Hatch L. T., Dziak R. P., Van Parijs S., McKenna M. F., et al. (2018). Monitoring long-term soundscape trends in US waters: the NOAA/NPS ocean noise reference station network. Mar. Policy 90, 6–13. doi: 10.1016/j.marpol.2018.01.023
Hickey B. M., Kudela R. M., Nash J. D., Bruland K. W., Peterson W. T., MacCready P., et al. (2010). River influences on shelf ecosystems: Introduction and synthesis. J. Geophysical Research: Oceans 115 (C2). doi: 10.1029/2009JC005452
Huyer A., Fleischbein J. H., Keister J., Kosro P. M., Perlin N., Smith R. L., et al. (2005). Two coastal upwelling domains in the northern California current system. J. Mar. Res. 63 (5), 901–929. doi: 10.1357/002224005774464238
Kaplan M. B., Mooney T. A., Partan J., Solow A. R. (2015). Coral reef species assemblages are associated with ambient soundscapes. Mar. Ecol. Prog. Series 533, 93–107. doi: 10.3354/meps11382
Kasten E. P., Gage S. H., Fox J., Joo W. (2012). The remote environmental assessment laboratory's acoustic library: An archive for studying soundscape ecology. Ecol. Inf. 12, 50–67. doi: 10.1016/j.ecoinf.2012.08.001
Krause B. (2008). Anatomy of the soundscape: evolving perspectives. J. Audio Eng. Soc. 56 (1/2), 73–80.
Laidre K. L., Stirling I., Lowry L. F., Wiig Ø., Heide-Jørgensen M. P., Ferguson S. H. (2008). Quantifying the sensitivity of Arctic marine mammals to climate-induced habitat change. Ecol. Appl. 18 (sp2), S97–S125. doi: 10.1890/06-0546.1
Lammers M. O., Brainard R. E., Au W. W., Mooney T. A., Wong K. B. (2008). An ecological acoustic recorder (EAR) for long-term monitoring of biological and anthropogenic sounds on coral reefs and other marine habitats. J. Acoustical Soc. America 123 (3), 1720–1728. doi: 10.1121/1.2836780
Loomis D. K., Ortner P. B., Kelble C. R., Paterson S. K. (2014). Developing integrated ecosystem indices. Ecol. Indic. 44, 57–62. doi: 10.1016/j.ecolind.2014.02.032
Mangiafico S. S. (2016). Summary and Analysis of Extension Program Evaluation in R, version 1.20.01. rcompanion.org/handbook/.
McKenna M. F., Ross D., Wiggins S. M., Hildebrand J. A. (2012). Underwater radiated noise from modern commercial ships. J. Acoustical Soc. America 131 (1), 92–103. doi: 10.1121/1.3664100
McWilliam J. N., Hawkins A. D. (2013). A comparison of inshore marine soundscapes. J. Exp. Mar. Biol. Ecol. 446, 166–176. doi: 10.1016/j.jembe.2013.05.012
Merchant N. D., Fristrup K. M., Johnson M. P., Tyack P. L., Witt M. J., Blondel P., et al. (2015). Measuring acoustic habitats. Methods Ecol. Evol. 6 (3), 257–265. doi: 10.1111/2041-210X.12330
Merchant N. D., Witt M. J., Blondel P., Godley B. J., Smith G. H. (2012). Assessing sound exposure from shipping in coastal waters using a single hydrophone and automatic identification system (AIS) data. Mar. pollut. Bull. 64 (7), 1320–1329. doi: 10.1016/j.marpolbul.2012.05.004
Miloslavich P., Bax N. J., Simmons S. E., Klein E., Appeltans W., Aburto-Oropeza O., et al. (2018). Essential ocean variables for global sustained observations of biodiversity and ecosystem changes. Global Change Biol. 24 (6), 2416–2433. doi: 10.1111/gcb.14108
Minello M., Calado L., Xavier F. C. (2021). Ecoacoustic indices in marine ecosystems: a review on recent developments, challenges, and future directions. ICES J. Mar. Sci. 78 (9), 3066–3074. doi: 10.1093/icesjms/fsab193
Mooney T. A., Di Iorio L., Lammers M., Lin T. H., Nedelec S. L., Parsons M., et al. (2020). Listening forward: approaching marine biodiversity assessments using acoustic methods. R. Soc. Open Sci. 7 (8), 201287. doi: 10.1098/rsos.201287
Moore S. E. (2008). Marine mammals as ecosystem sentinels. J. Mammalogy 89 (3), 534–540. doi: 10.1644/07-MAMM-S-312R1.1
Moore J. E., Forney K. A., Weller D. W. (2018). Surveys. Encyclopedia Mar. Mammals, 960–963. doi: 10.1016/B978-0-12-804327-1.00253-3
Moore S. E., Kuletz K. J. (2019). Marine birds and mammals as ecosystem sentinels in and near distributed biological observatory regions: An abbreviated review of published accounts and recommendations for integration to ocean observatories. Deep Sea Res. Part II: Topical Stud. Oceanography 162, 211–217. doi: 10.1016/j.dsr2.2018.09.004
Moore S. E., Logerwell E., Eisner L., Farley E. V., Harwood L. A., Kuletz K., et al. (2014). “Marine fishes, birds and mammals as sentinels of ecosystem variability and reorganization in the pacific Arctic region,” in The pacific arctic region (Dordrecht: Springer), 337–392. doi: 10.1007/978-94-017-8863-2_11
Moreno-Gómez F. N., Bartheld J., Silva-Escobar A. A., Briones R., Márquez R., Penna M. (2019). Evaluating acoustic indices in the valdivian rainforest, a biodiversity hotspot in south America. Ecol. Indic. 103, 1–8. doi: 10.1016/j.ecolind.2019.03.024
Parks S. E., Miksis-Olds J. L., Denes S. L. (2014). Assessing marine ecosystem acoustic diversity across ocean basins. Ecol. Inf. 21, 81–88. doi: 10.1016/j.ecoinf.2013.11.003
Parmar T. K., Rawtani D., Agrawal Y. K. (2016). Bioindicators: the natural indicator of environmental pollution. Front. Life Sci. 9 (2), 110–118. doi: 10.1080/21553769.2016.1162753
Parsons M., Erbe C., McCauley R., McWilliam J., Marley S., Gavrilov A., et al. (2016). “Long-term monitoring of soundscapes and deciphering a usable index: Examples of fish choruses from Australia,” in Proceedings of meetings on acoustics 4ENAL, vol. 27. (Dublin, Ireland: Acoustical Society of America), 010023. doi: 10.1121/2.0000286
Pieretti N., Danovaro R. (2020). Acoustic indexes for marine biodiversity trends and ecosystem health. Philos. Trans. R. Soc. B 375 (1814), 20190447. doi: 10.1098/rstb.2019.0447
Pieretti N., Martire M. L., Farina A., Danovaro R. (2017). Marine soundscape as an additional biodiversity monitoring tool: A case study from the Adriatic Sea (Mediterranean Sea). Ecol. Indic. 83, 13–20. doi: 10.1016/j.ecolind.2017.07.011
Pijanowski B. C., Villanueva-Rivera L. J., Dumyahn S. L., Farina A., Krause B. L., Napoletano B. M., et al. (2011). Soundscape ecology: The science of sound in the landscape. BioScience 61 (3), 203–216. doi: 10.1525/bio.2011.61.3.6
Queirós A. M., Strong J. A., Mazik K., Carstensen J., Bruun J., Somerfield P. J., et al. (2016). An objective framework to test the quality of candidate indicators of good environmental status. Front. Mar. Sci. 3. doi: 10.3389/fmars.2016.00073
Ragland J., Abadi S., Sabra K. (2022). Long-term noise interferometry analysis in the northeast pacific ocean. J. Acoustical Soc. America 151 (1), 194–204. doi: 10.1121/10.0009232
R Core Team. (2021). R: A language and environment for statistical computing (Vienna, Austria: R Foundation for Statistical Computing). Available at: https://www.R-project.org/.
R Core Team. (2022). R: A language and environment for statistical computing (Vienna, Austria: R Foundation for Statistical Computing). Available at: https://www.R-project.org/.
Rice A. N., Soldevilla M. S., Quinlan J. A. (2017). Nocturnal patterns in fish chorusing off the coasts of Georgia and eastern Florida. Bull. Mar. Sci. 93 (2), 455–474. doi: 10.5343/bms.2016.1043
Risch D., Parks S. E. (2017). “Biodiversity assessment and environmental monitoring in freshwater and marine biomes using ecoacoustics,” in Ecoacoustics. the ecological role of sounds. Eds. Farina A., Gage S. H. (Oxford, UK: Wiley), 145–168.
Roca I. T., Van Opzeeland I. (2020). Using acoustic metrics to characterize underwater acoustic biodiversity in the southern ocean. Remote Sens. Ecol. Conserv. 6 (3), 262–273. doi: 10.1002/rse2.129
Rombouts I., Beaugrand G., Artigas L. F., Dauvin J. C., Gevaert F., Goberville E., et al. (2013). Evaluating marine ecosystem health: case studies of indicators using direct observations and modelling methods. Ecol. Indic. 24, 353–365. doi: 10.1016/j.ecolind.2012.07.001
Ross S. R. J., Friedman N. R., Yoshimura M., Yoshida T., Donohue I., Economo E. P. (2021). Utility of acoustic indices for ecological monitoring in complex sonic environments. Ecol. Indic. 121, 107114. doi: 10.1016/j.ecolind.2020.107114
Sakai T., Barlow J., Griffiths E., Oswald M, Baumann-Pickering S., Oswald J., et al. (2020). R package ‘PAMpal’. doi: 10.1121/1.2743157
Sala E., Mayorga J., Bradley D., Cabral R. B., Atwood T. B., Auber A., et al. (2021). Protecting the global ocean for biodiversity, food and climate. Nature 592 (7854), 397–402. doi: 10.1038/s41586-021-03371-z
Sethi S. S., Jones N. S., Fulcher B. D., Picinali L., Clink D. J., Klinck H., et al. (2019). Combining machine learning and a universal acoustic feature-set yields efficient automated monitoring of ecosystems. BioRxiv, 865980. doi: 10.1101/865980
Shannon C. E. (1948). A mathematical theory of communication. Bell system Tech. J. 27 (3), 379–423. doi: 10.1002/j.1538-7305.1948.tb01338.x
Staaterman E., Ogburn M. B., Altieri A. H., Brandl S. J., Whippo R., Seemann J., et al. (2017). Bioacoustic measurements complement visual biodiversity surveys: preliminary evidence from four shallow marine habitats. Mar. Ecol. Prog. Ser. 575, 207–215. doi: 10.3354/meps12188
Sueur J., Aubin T., Simonis C. (2008). Seewave, a free modular tool for sound analysis and synthesis. Bioacoustics 18 (2), 213–226. doi: 10.1080/09524622.2008.9753600
Sueur J., Farina A., Gasc A., Pieretti N., Pavoine S. (2014). Acoustic indices for biodiversity assessment and landscape investigation. Acta Acustica united Acustica 100 (4), 772–781. doi: 10.3813/AAA.918757
The jamovi project (2021) Jamovi (Version 2.2.5). Available at: https://www.jamovi.org.
Tittensor D. P., Beger M., Boerder K., Boyce D. G., Cavanagh R. D., Cosandey-Godin A., et al. (2019). Integrating climate adaptation and biodiversity conservation in the global ocean. Sci. Adv. 5 (11), eaay9969. doi: 10.1126/sciadv.aay9969
Towsey M., Wimmer J., Williamson I., Roe P. (2014). The use of acoustic indices to determine avian species richness in audio-recordings of the environment. Ecol. Inf. 21, 110–119. doi: 10.1016/j.ecoinf.2013.11.007
Trowbridge J., Weller R., Kelley D., Dever E., Plueddemann A., Barth J. A., et al. (2019). The ocean observatories initiative. Front. Mar. Sci. 6. doi: 10.3389/fmars.2019.00074
Van Parijs S. M., Baker K., Carduner J., Daly J., Davis G. E., Esch C., et al. (2021). NOAA And BOEM minimum recommendations for use of passive acoustic listening systems in offshore wind energy development monitoring and mitigation programs. Front. Mar. Sci. 8. doi: 10.3389/fmars.2021.760840
Villanueva-Rivera L. J., Pijanowski B. C., Doucette J., Pekin B. (2011). A primer of acoustic analysis for landscape ecologists. Landscape Ecol. 26 (9), 1233–1246. doi: 10.1007/s10980-011-9636-9
Villanueva-Rivera L. J., Pijanowski B. C., Villanueva-Rivera M. L. J. (2018). Package ‘soundecology’. R Package version 1 (3), 3.
Weilgart L. S., Whitehead H. (1988). Distinctive vocalizations from mature male sperm whales (Physeter macrocephalus). Can. J. Zoology 66 (9), 1931–1937. doi: 10.1139/z88-282
Wiggins S. M., Hildebrand J. A. (2007). “High-frequency acoustic recording package (HARP) for broad-band, long-term marine mammal monitoring,” in 2007 symposium on underwater technology and workshop on scientific use of submarine cables and related technologies (Tokyo, Japan: IEEE), 551–557. doi: 10.1109/UT.2007.370760
Keywords: acoustic indices, bioacoustics, marine mammals, soundscape, anthropogenic noise
Citation: Ferguson EL, Clayton HM and Sakai T (2023) Acoustic indices respond to specific marine mammal vocalizations and sources of anthropogenic noise. Front. Mar. Sci. 10:1025464. doi: 10.3389/fmars.2023.1025464
Received: 22 August 2022; Accepted: 24 January 2023;
Published: 09 February 2023.
Edited by:
Guillermo Luna-Jorquera, Universidad Católica del Norte, ChileReviewed by:
Felipe N. Moreno-Gómez, Universidad Católica del Maule, ChileGiovanni Zambon, University of Milano-Bicocca, Italy
Copyright © 2023 Ferguson, Clayton and Sakai. This is an open-access article distributed under the terms of the Creative Commons Attribution License (CC BY). The use, distribution or reproduction in other forums is permitted, provided the original author(s) and the copyright owner(s) are credited and that the original publication in this journal is cited, in accordance with accepted academic practice. No use, distribution or reproduction is permitted which does not comply with these terms.
*Correspondence: Elizabeth L. Ferguson, eferguson@oceanscienceanalytics.com