- 1Univ. Littoral Côte d’Opale, CNRS, Univ. Lille, IRD, UMR 8187 - LOG - Laboratoire d’Océanologie et de Géosciences, Wimereux, France
- 2Univ. Bordeaux, CNRS, UMR 5805 EPOC, Station Marine d’Arcachon, Arcachon, France
- 3Univ. Bretagne Occidentale, CNRS, IRD, OSU-IUEM, UAR 3113, Plouzané, France
- 4Univ. Bordeaux, CNRS, UMR 5805 EPOC, Pessac, France
- 5CNRS, IRD, CP53, Unité biologie des organismes et écosystèmes aquatiques (BOREA), Muséum National D’Histoire Naturelle, Sorbonne Université, Université de Caen Normandie, Université des Antilles, Paris, France
- 6Sorbonne Université, CNRS, FR2424, Station Biologique de Roscoff, Roscoff, France
- 7IRD, UAR191, Instrumentation, Moyens Analytiques, Observatoires en Géophysique et Océanographie (IMAGO), Technopôle de Brest-Iroise, Plouzané, France
- 8Univ. Bordeaux, CNRS, OASU, UAR 2567 POREA, Pessac, France
- 9OSU Réunion, Université de La Réunion, Saint-Denis, France
- 10La Rochelle Université, CNRS, UMR 7266 LIENSs, 2 rue Olympe de Gouges, La Rochelle, France
- 11Sorbonne Université, Laboratoire d’Océanographie de Villefranche sur mer (LOV) CNRS UMR 7093, Villefranche sur Mer, France
- 12MNHN, station marine de Dinard, CRESCO, Dinard, France
- 13Univ. Montpellier, UMR 5243, Laboratoire Géosciences Montpellier, Montpellier, France
- 14Plateforme d’Ecologie Marine Expérimentale MEDIMEER, OSU OREME (Univ de Montpellier, CNRS, IRD, INRAE), Station Marine de Sète, Sète, France
- 15Sorbonne Université, CNRS, UMR 7144 AD2M, Station Biologique de Roscoff, Roscoff, France
- 16Sorbonne Université, CNRS, OSU STAMAR - UAR2017, Paris, France
- 17CAPENA, Centre pour l’Aquaculture, la Pêche et l’Environnement de Nouvelle-Aquitaine, Bayonne, France
- 18Normandie Université, Université de Caen Normandie, Caen, France
- 19Centre de Recherches en Environnement Côtier (CREC) - Station Marine de l’Université de Caen Normandie, Luc sur mer, France
- 20Laboratoire de Biologie des Organismes et Ecosystèmes Aquatiques (BOREA) – Université de Caen Normandie, MNHN, SU, UA, CNRS UMR 8067, IRD 207, Caen, France
- 21Sorbonne Université, Paris 06, CNRS, UMR7621 LOMIC, Observatoire Océanologique, Banyuls sur Mer, France
- 22Aix Marseille Univ, CNRS/IN2P3, CPPM, Marseille, France
- 23Sorbonne Université, IMEV FR 7093 OSU STAMAR, Villefranche sur Mer, France
- 24GIPREB, Syndicat Mixte, Berre-l’Etang, France
- 25Univ Brest, CNRS, IRD, Ifremer, LEMAR, Plouzané, France
- 26Laboratoire de Biologie des Organismes et Ecosystèmes Aquatiques (BOREA), MNHN, SU, CNRS, EPHE, UA, Paris, France
- 27Aix Marseille Univ, Université de Toulon, CNRS, IRD, MIO, Marseille, France
- 28Sorbonne Université, CNRS, FR3724, Observatoire Océanologique, Banyuls sur Mer, France
- 29Univ. Pau & Pays Adour, CNRS, E2S UPPA, Institut des Sciences Analytiques et de Physico-Chimie pour l’Environnement et les Matériaux – MIRA, UMR5254, Anglet, France
- 30Ifremer, LITTORAL, Laboratoire Environnement et Ressources des Pertuis Charentais (LER/PC), La Tremblade, France
- 31MARBEC (Marine Biodiversity, Exploitation and Conservation), Univ Montpellier, CNRS, Ifremer, IRD, Montpellier, France
- 32Environment Department, Faroe Marine Resarch Institute, Tórshavn, Faroe Islands
- 33Department of Marine Resources and Energy, Tokyo University of Marine Science and Technology, Tokyo, Japan
- 34Department of Zoology and Entomology, Rhodes University, Grahamstown, South Africa
Introduction: While crucial to ensuring the production of accurate and high-quality data—and to avoid erroneous conclusions—data quality control (QC) in environmental monitoring datasets is still poorly documented.
Methods: With a focus on annual inter-laboratory comparison (ILC) exercises performed in the context of the French coastal monitoring SOMLIT network, we share here a pragmatic approach to QC, which allows the calculation of systematic and random errors, measurement uncertainty, and individual performance. After an overview of the different QC actions applied to fulfill requirements for quality and competence, we report equipment, accommodation, design of the ILC exercises, and statistical methodology specially adapted to small environmental networks (<20 laboratories) and multivariate datasets. Finally, the expanded uncertainty of measurement for 20 environmental variables routinely measured by SOMLIT from discrete sampling—including Essential Ocean Variables—is provided.
Results, Discussion, Conclusion: The examination of the temporal variations (2001–2021) in the repeatability, reproducibility, and trueness of the SOMLIT network over time confirms the essential role of ILC exercises as a tool for the continuous improvement of data quality in environmental monitoring datasets.
Introduction
In the context of global change, the number of studies that include meta-analytical combinations of environmental data increased at a fast pace in the past decades (e.g., Duarte et al., 2009; Harvey et al., 2013; Talarmin et al., 2016; McCrackin et al., 2017; Carstensen and Duarte, 2019; Lheureux et al., 2021; Lheureux et al., 2023). In these studies, it is assumed that the data were of similar quality and reliability, which is not necessarily true: measurement processes are all subject to systematic (i.e., bias) and random (i.e., variations) errors of various types, such as the choice of the method, ambient conditions, or the working practices of analysts (Taverniers et al., 2004; de Boer, 2016). At a time when high-quality scientific journals require a comprehensive documentation of data quality control (QC) procedures for publication (de Boer, 2016), and because erroneous conclusions may arise from studies using inappropriate methods, it is important to increase the confidence levels of data QC, which is often poorly documented in environmental monitoring datasets.
By using the 20 years of experience of the French coastal monitoring SOMLIT network (https://www.SOMLIT.fr/), the goal of our paper is to share a pragmatic approach to QC that focuses on annual inter-laboratory comparison (ILC) exercises as an indication of measurement uncertainty. The SOMLIT network currently includes 12 laboratories and 22 permanent sampling stations distributed along the French littoral (Figure 1) and covers a wide range of environmental and trophic conditions (Goberville et al., 2010; Liénart et al., 2017; Liénart et al., 2018; Lheureux et al., 2021). Implemented by CNRS (Centre National de la Recherche Scientifique) in 1997 to increase our scientific knowledge of the responses of marine coastal ecosystems to natural and human-induced influences, the SOMLIT network is part of the national research infrastructure ILICO (Infrastructure de recherche LIttorale et CÔtière; Cocquempot et al., 2019). Common standard operating protocols are used and all data are continuously routinely quality-controlled since 2006 by means of robust quality assurance procedures guided by the ISO-17025 standard (ISO-17025, 2005, then ISO-17025, 2017).
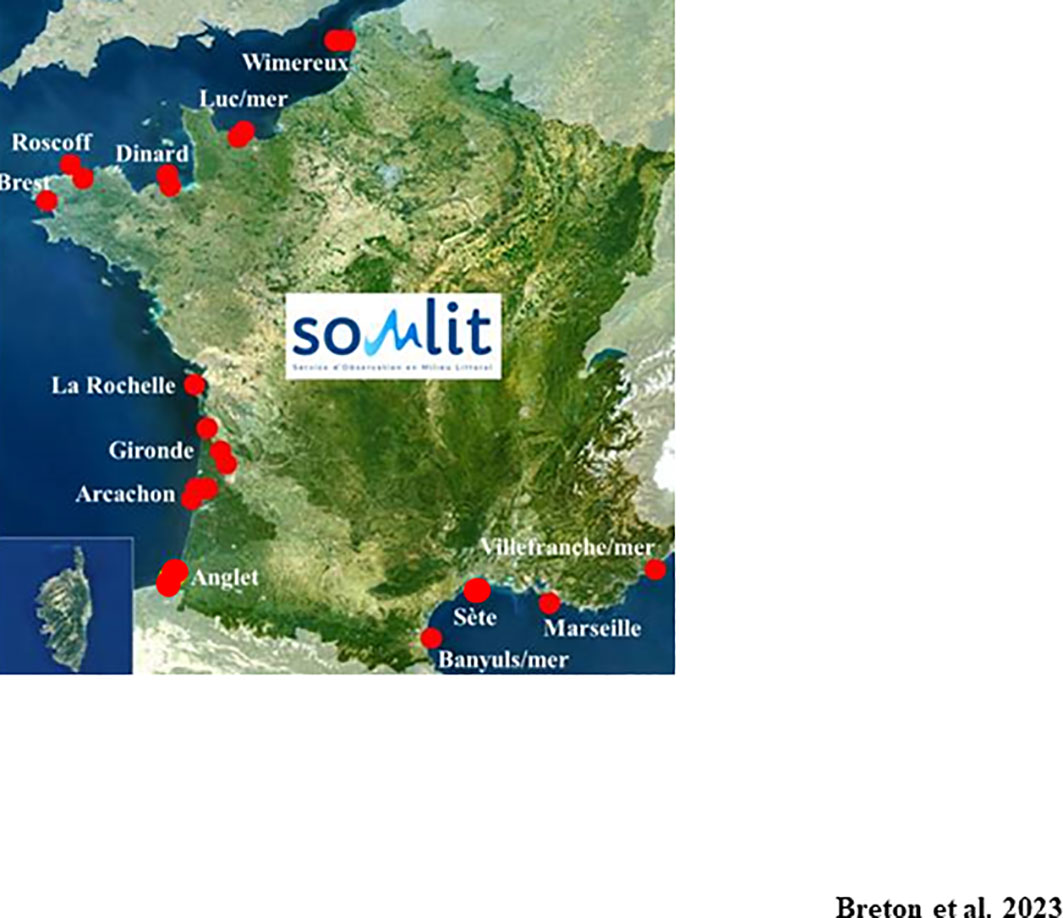
Figure 1 Location of the sites and stations belonging to the French coastal monitoring network SOMLIT (the logotype is in the center of the map).
After an overview of the different QC actions implemented by the SOMLIT to fulfill requirements for quality and competence, we report equipment, accommodation, design of the ILC exercises and statistical methodology to assess individual performance specifically adapted to small (<20 laboratories) environmental networks and multivariate datasets, based on the ISO-13528 standard (ISO-13528, 2005), Héberger and Kollár-Hunek (2011), and procedures for measurement uncertainty assessment. Finally, we provide an expanded uncertainty of measurement for 20 environmental variables routinely measured by SOMLIT from discrete sampling (Table 1), including Essential Ocean Variables (Miloslavich et al., 2018).
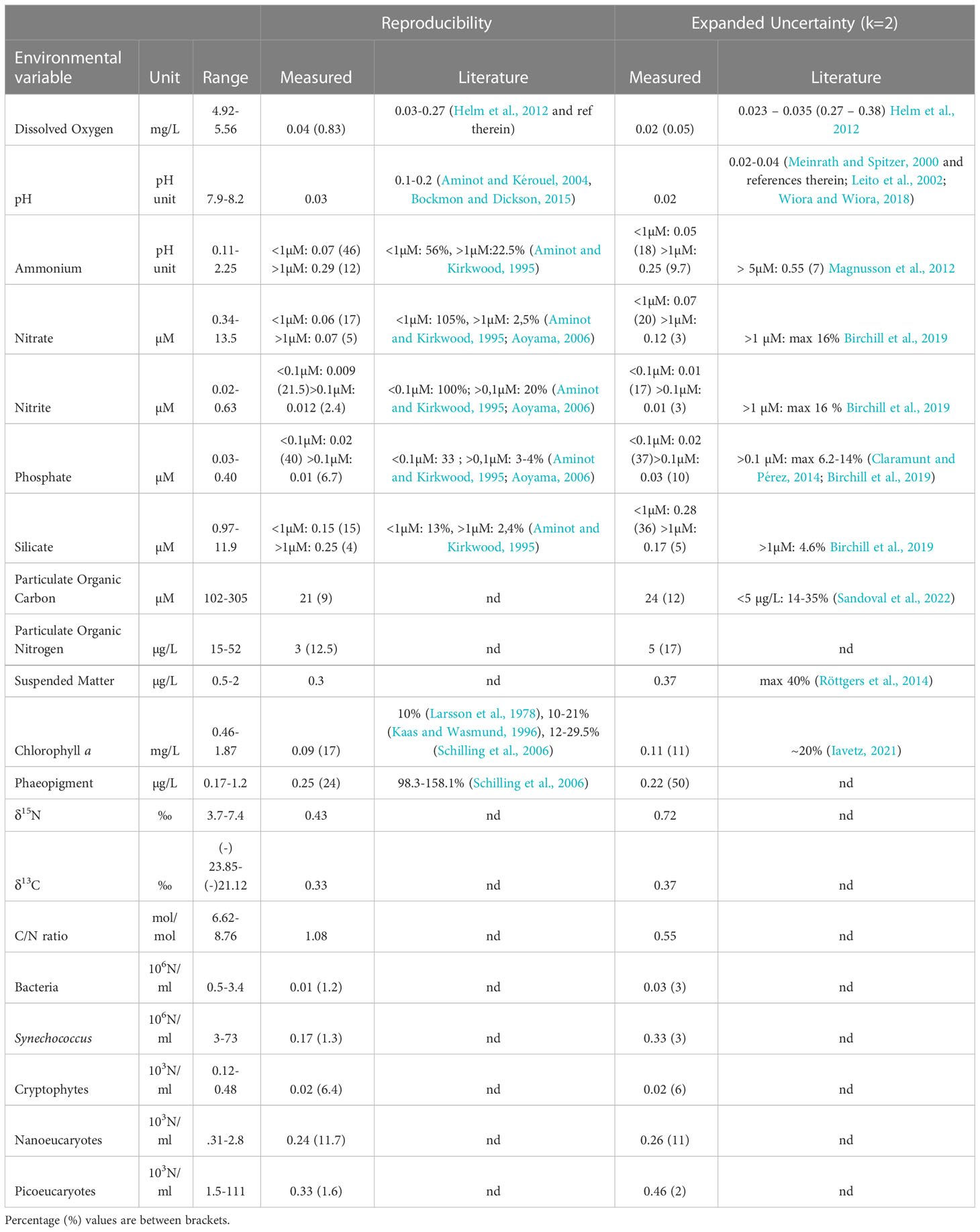
Table 1 Range, reproducibility, and expanded uncertainty of the environmental variables measured during inter-laboratory comparison exercises of the SOMLIT network over the last 6 years.
Data quality control overview
Data QC within the SOMLIT network is composed of five actions (Figure 2). Within-laboratory control is continuously performed by each laboratory on the basis of control charts (Figure 3; Table S1) and the Nordtest TR 569 principles (Nordtest TR 569, 2007): control charts ensure the quality of produced data according to statistical or reference criteria—when available—and represent an essential step for the calculation of uncertainty. Control charts may also be performed (1) to objectively compare different analytical methods, (2) for new method validation, (3) to qualify an inexperienced analyst, or (4) to ensure stable ambient conditions (e.g., temperature and hygrometry) for measurements. There are two kinds of control charts: X-charts and R-charts for controlling systematic errors and random variations, respectively (Nordtest TR 569, 2007). The setting control limits used to build the control charts used by the SOMLIT network are given in Table S1.
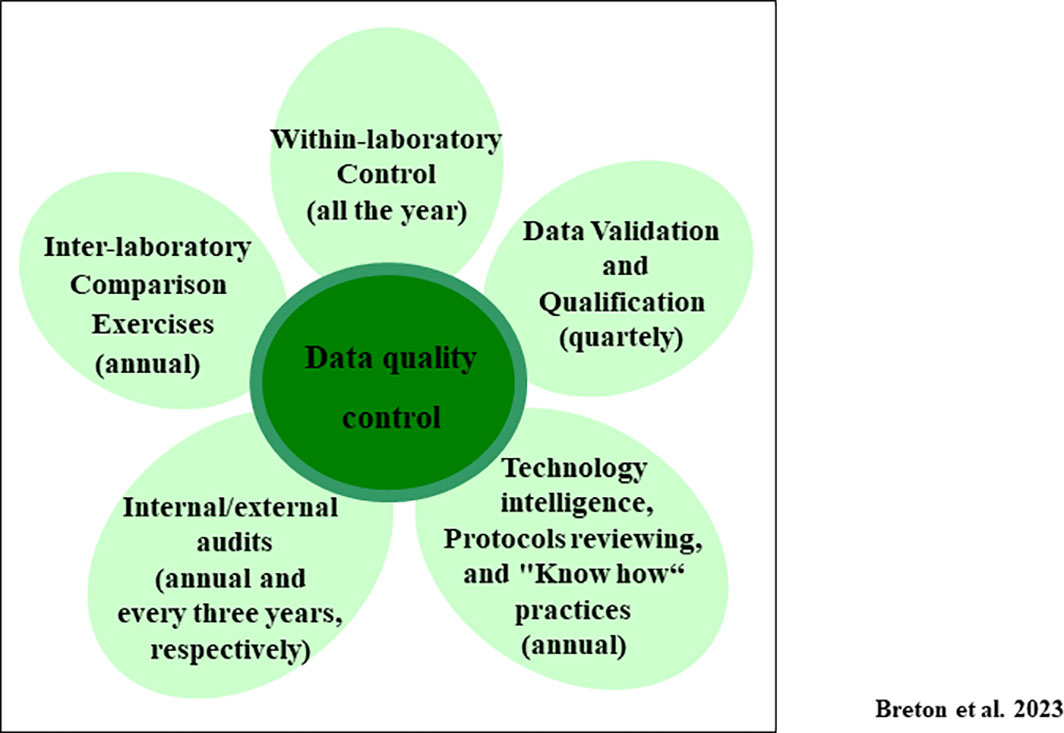
Figure 2 Scheme of the different actions for data quality control implemented in the SOMLIT network and their frequency based on ISO 17025 (2017).
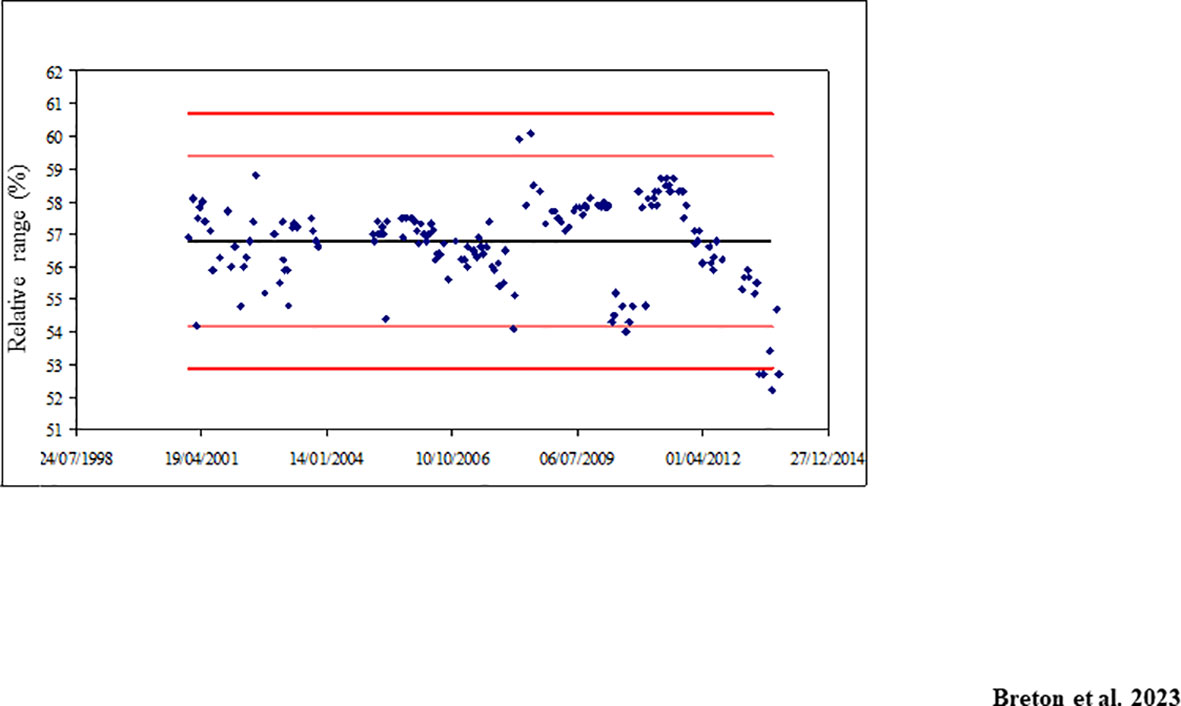
Figure 3 Within-laboratory control: example of control chart based on Nordtest TR 569 (2007) for ensuring that the data are of good quality and the measurement process is statistically stable. Black line: the central line; thin red line: the warming limits; and thick red line: the action limits. The data value is considered in control if it is within the warming limits or if the value is between the warming and action limits but the two previous ones are within the warming limits. In this case, data are considered of good quality. The data value is considered outside control if the data value is outside action limits or between the warming and action limits and at least one of the two previous values are between warming and action limits too. In this case, measurement analysis must be made again once the problems are resolved if possible. The data value is considered in control but must be scrupulously monitored if the last 7 previous values exhibit a trend or 10 of the last 11 control values are on the same side and far from the central line.
SOMLIT data are qualified on a trimonthly basis using QC flags (see Table 2), which are derived from the World Ocean Circulation Experiment (WOCE; https://exchange-format.readthedocs.io/en/latest/quality.html#woce-bottle-quality-codes). QC flags include two levels. Level 1 ensures interoperability, but the codification is simplified to provide QC flags easily understandable by end-users. Level 2 is detailed in order to answer to the needs of data producers; it corresponds to internal QC according to the ISO 17025 standard (ISO-17025, 2017). Internal and external audits guided by the ISO 17025 standard (ISO-17025, 2017) are made on an annual and triennial basis, respectively, for ensuring laboratory quality management and technical requirements. We provide an example of the report in Figure 4.
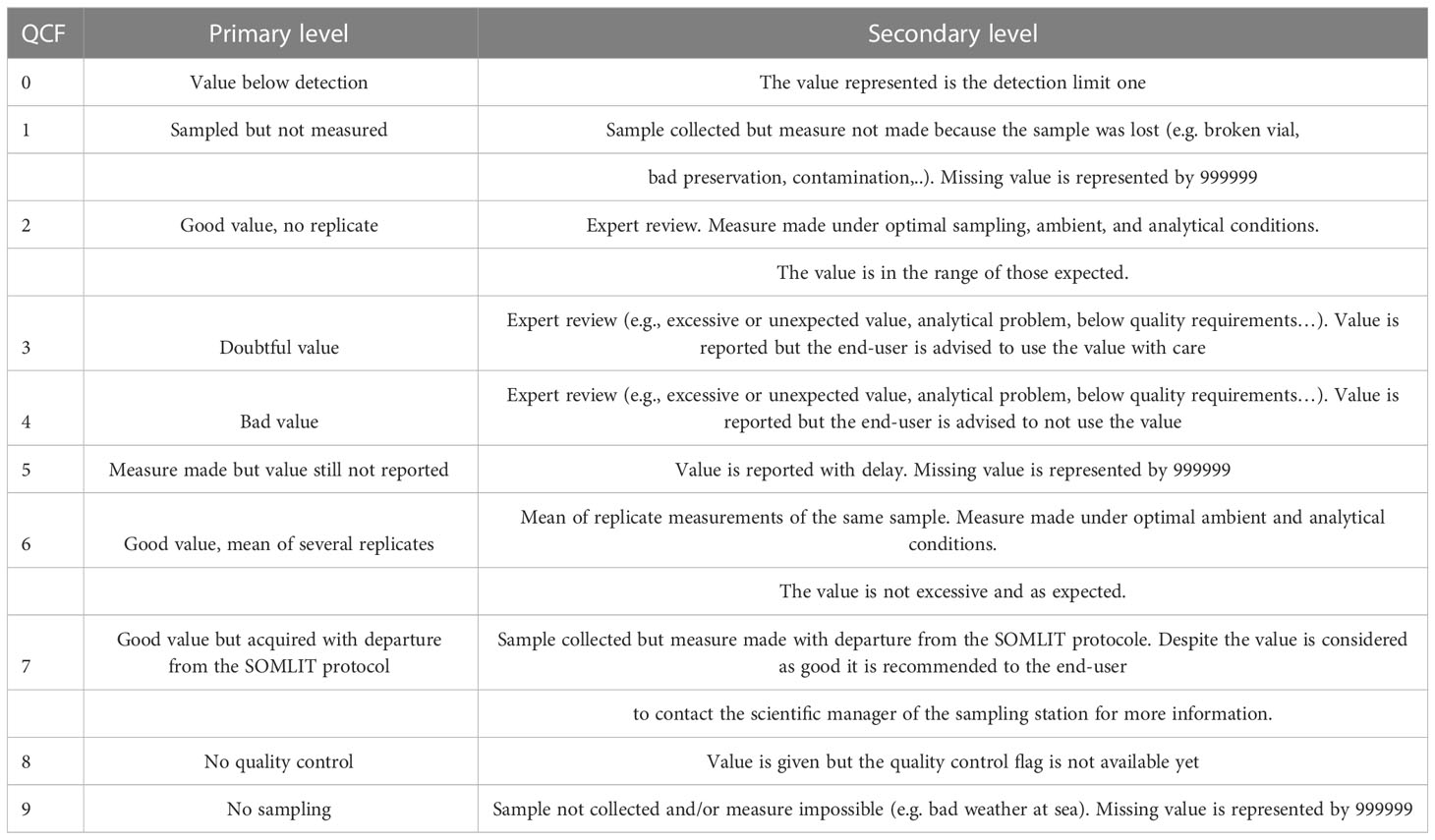
Table 2 Primary and secondary level quality control flags (QCF) used by the SOMLIT network for the data qualification step.
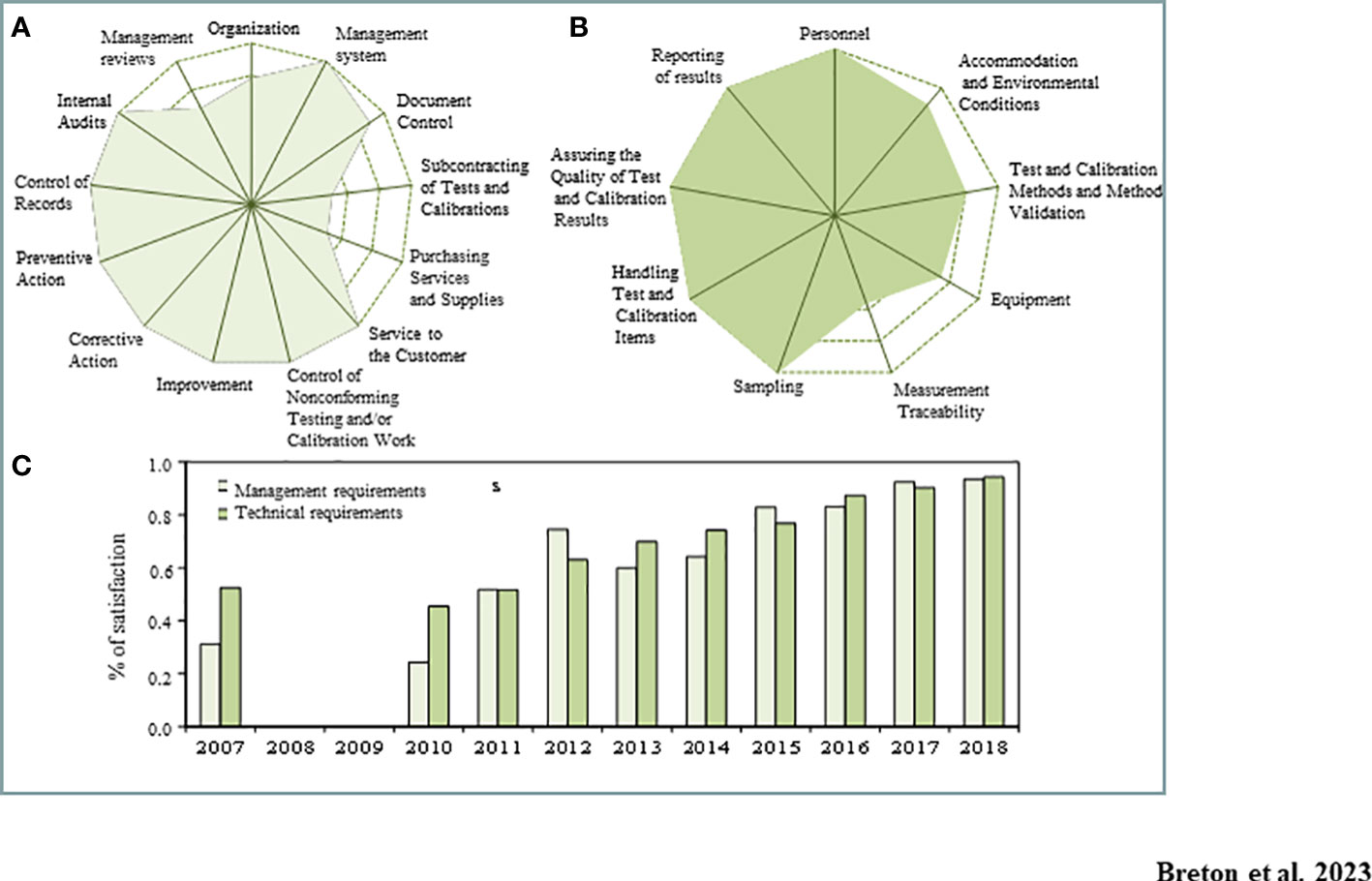
Figure 4 Internal/external audits of the SOMLIT network guided by the ISO 17025 standard (ISO-13528, 2005) to ensure the integrity of data and minimize errors: example of reports provided by one SOMLIT laboratory. (A, B) Management and technical requirements, respectively. (C) Temporal variations of the percentage (%) of satisfaction to meet the requirements.
ILCs are undertaken annually at the end of summer in one of the SOMLIT laboratories (Figure 1). To strengthen the robustness of comparisons, we alternate among different hosting laboratories, with sampling stations in the oligo- to ultra-oligotrophic Mediterranean Sea, oligo- to mesotrophic Atlantic waters, and the meso-eutrophic English Channel (Table S2). ILC allows best practices to be shared among participants from different laboratories, to evaluate data reliability (uncertainty calculation), and to assess the performance of individual operators from each laboratory. If issues are identified, ILC provides a forum to help participants to improve their practices and to correct their errors. In the following sections, we describe the methodology used during ILC and show how efforts to fulfill quality requirements have helped to improve the trueness and reproducibility of SOMLIT data over time.
Design of ILC exercises and sample testing preparation
Although the SOMLIT program routinely measures 25 environmental variables, only the 22 variables subjected to discrete sampling (Table 1) were considered each year. Figure 5 describes the different steps involved in the ILC exercises. Given that stable reference material is lacking for most of the environmental variables measured by the SOMLIT network, performance in total measurement was evaluated by robust analysis, using measurement data from real natural seawater (see below).
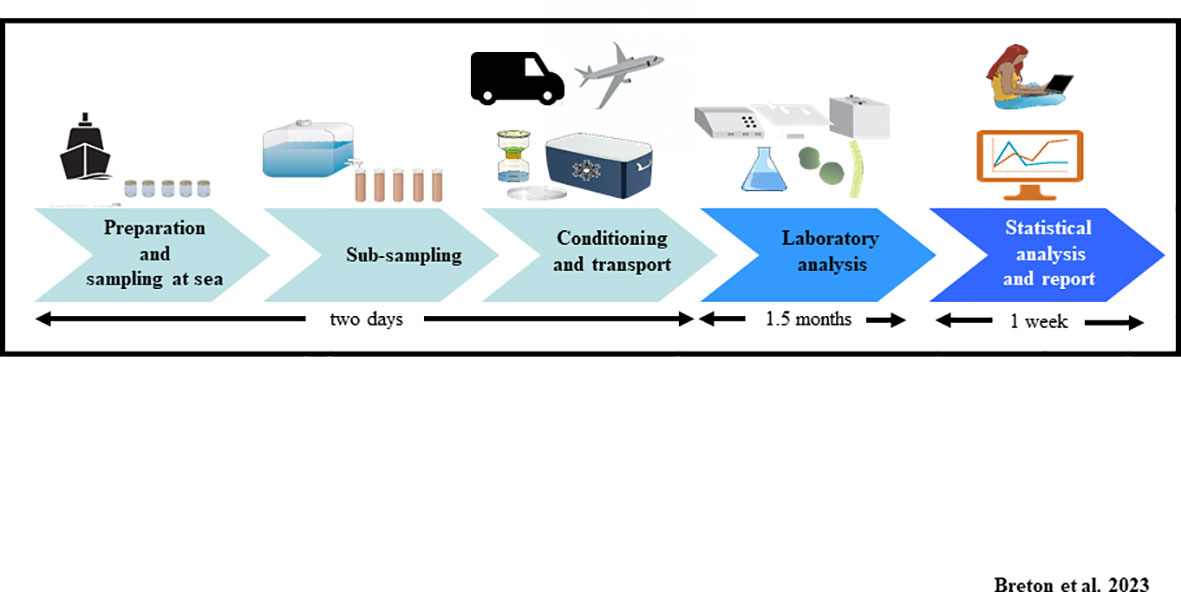
Figure 5 Scheme of the different steps of the annual ILC exercises implemented by the SOMLIT network since 2001.
Sampling at sea
Sampling at sea was carried out onboard the research vessel of the hosting laboratory, using Niskin bottles or a pump. Approximately 2–3 m3 of seawater was collected with ten 50-L tanks. The sampling was performed a few hours (less than 1 day) before the start of the ILC exercise.
Sub-sampling and conditioning
Back to the host laboratory, seawater was transferred to a large-volume stainless-steel tank equipped with 15 petcock assemblies (Figure S1) and gently and continuously mixed with a rotating blade system inside (80 min at approximately 10 rpm). Mixing was stopped during sub-sampling. For the dissolved matter analysis, samples were first successively sub-sampled in 5 replicates in the following order: dissolved oxygen (hereafter O2), pH, ammonium (), nitrate (), nitrite (), phosphate (), and silicate [Si(OH)4]. Conditioning and storage were the same as conducted routinely (Table S3). To reduce as far as possible any sampling inhomogeneity between participants, samples for each variable were sub-sampled at the same time by the different participants. Before filling, vials were rinsed three times with sampling seawater. Samples were also successively sub-sampled for particulate matter analysis: pico- and nanoplankton, chlorophyll a (Chla) and phaeopigments, particulate organic carbon (POC), particulate organic nitrogen (PON), suspended particulate matter (SPM), and δ13C and δ15N.
Processing and storage during transport and back to home laboratory (Figure 5) were carried out following the methodology given in Table S3. Each year, the Quality Manager allows laboratory participants to perform analyses within 1 to 3 months, depending on the variables. The list of analytical techniques for each environmental variable is given in Table S3. Finally, the Quality Manager collects replicate measurement results for each environmental variable and from each participating laboratory, as well as information on the date of analysis and potential problems that may have occurred during sub-sampling, processing, storage, and/or analysis.
Once the performance scores in repeatability and trueness are obtained for the different participating laboratories, and after calculation of the annual reproducibility of the SOMLIT network (see below), a quality report is written by the Quality Manager and sent to each participating laboratory. In the case of “questionable” performance during two consecutive ILC exercises or “unsatisfactory” performance in the current ILC exercise, the laboratory in question must take corrective actions, such as to redo a calibration, to improve dim light conditions for chlorophyll a analysis, in order to achieve satisfactory performance and/or reproducibility in accordance with other participating laboratories.
Calculation of systematic and random errors and performance
The different steps of the statistical methodology used to assess the total measurement accuracy (trueness and precision) for the 22 environmental variables during ILC exercises, as well as the overall performance of the participating laboratory, are described in Figure 6. The methodology has been adapted for small (<20 participating laboratories) networks, using the algorithm A mentioned in the ISO-13528 standard (see the freeware using Microsoft Excel© in Table 1 of the Supplementary Materials) for non-parametric data, and the user-friendly macro created by Héberger and Kollár-Hunek (2011) for both non-parametric and multivariate datasets. The application of the latter approach allows a better appraisal of proficiency testing (PT) in the case of multivariate monitoring in comparison with the ISO-13528 standard (ISO-13528, 2005, see Medina-Pastor et al., 2010; Stoyke et al., 2012): it ranks the performance of the different laboratories on the basis of data of different units and explores overall improvement of performance over time.
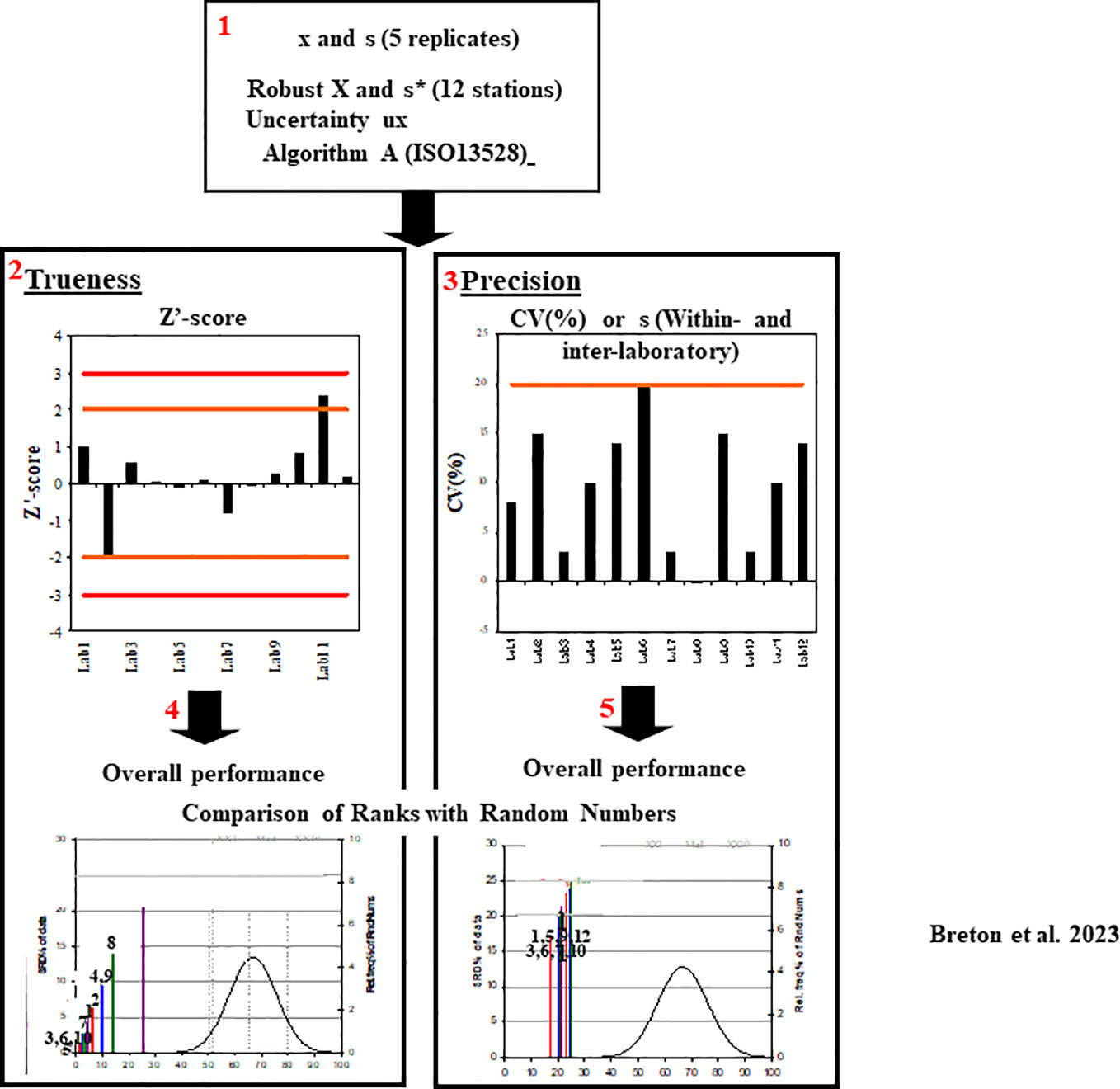
Figure 6 Annual ILC exercises implemented by the SOMLIT network: the different steps in the statistical methodology used to assess the total measurement accuracy of the 22 environmental variables and the participating laboratory performance. Step 1: Calculation of the mean (x) and the standard deviation (s) of replicates as well as that of the robust mean (X), standard deviation (s*), and uncertainty (ux) of total measurement of the measurand, by applying the algorithm A. Steps 2 and 3: Calculation of the individual deviations (z′-score) to the SOMLIT robust for assessing the trueness (systematic bias) and precision (random variations). Steps 4 and 5: Calculation of the final rank of each participating laboratory for the overall performance for trueness and precision (Héberger and Kollár-Hunek, 2011).
Robust statistics are used without outlier exclusion before calculation (Analytical Methods Committee, 1989). For each environmental variable, performance in trueness of total measurement (i.e., from sampling to data reporting) was assessed by calculating a z′-score according to Equation 1 (ISO-13528, 2005):
where xi is the mean value of five replicates obtained by the participating laboratories i, x* and are the robust average and the robust standard deviation of the different xi, respectively, and ux is the uncertainty of total measurement of the measurand, as mentioned in Equation 2:
where p is the number of participating laboratories at the ILC exercise. The robust average and robust standard deviation were calculated using the algorithm A (see the freeware using Microsoft Excel© in Table 1 of the Supplementary Materials) of ISO-13528 (2005). z′-score was chosen against z-score because of the small size of the SOMLIT network and because the uncertainty of the assigned value was considered to be not negligible given that most environmental variables never met the following inequality (Equation 3):
According to ISO-13528:
● |z′| ≤ 2 was considered as “satisfactory”
● 2< |z′|< 3 was considered as “questionable”
● |z′| ≥ 3 was considered as “unsatisfactory”
For each laboratory, performance in repeatability was assessed by comparing the standard deviation of five replicate measurements with the limit of acceptable precision given in the literature, when available (see Table S4). Reproducibility (between-laboratory precision) was evaluated by calculating the robust standard deviation x* of the different reported mean values of the measurand using the algorithm A. To classify the different participating laboratories according to their respective overall performance in trueness and repeatability, we performed a Sum of Ranking Difference (SRD, see the Supplementary Materials for file format) test, a non-parametric multivariate technique recommended when the number of participating laboratories is less than 13 (Héberger and Kollár-Hunek, 2011). While SRD has multiple applications (e.g., Andrić and Héberger, 2015; Kalivas et al., 2015; Sziklai and Héberger, 2020), it allows us to analyze similarities between laboratories by ranking (columns of the input matrix relative to trueness and replicability targets, across the measurement of several environmental variables, and rows of the input matrix). The closer to zero the SRD value, the better the overall performance of the laboratory. The SRD test was validated by a random test called Comparison of Ranks with Random Numbers (CRRN) by ensuring that no participating laboratory is randomly ranked (the black curve is the cumulative distribution function of the random SRD values; Figure 6). The method is described in detail in Héberger and Kollár-Hunek (2011). A freeware for the calculation of SRD-CRNN with Microsoft Excel© is available at http://aki.ttk.mta.hu/srd. We chose the zero value for trueness targets and the reproducibility targets given in Table 3.
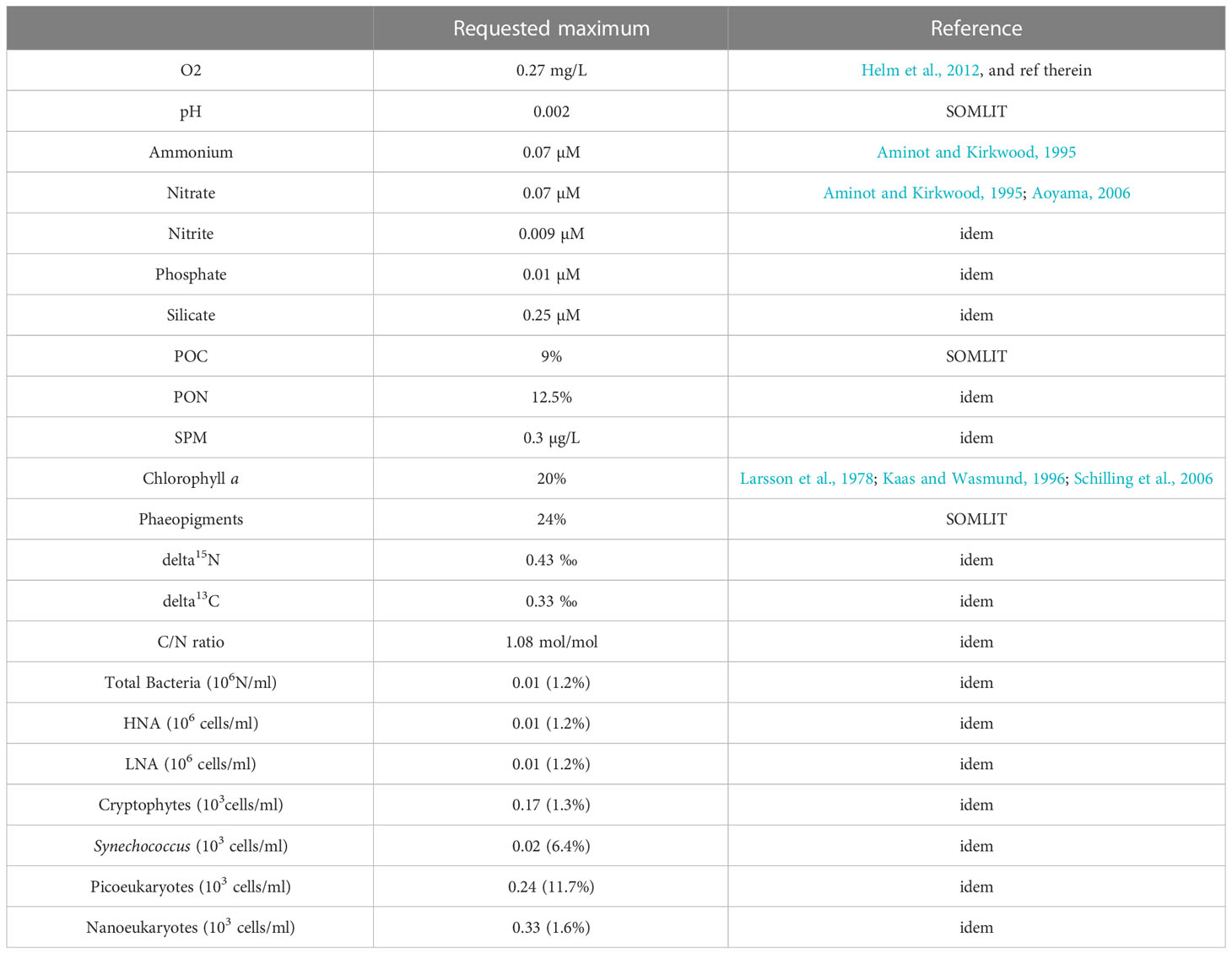
Table 3 Reproducibility targets and reference used to calculate the overall performance of the participating laboratories to the inter-laboratory comparison exercises of the SOMLIT network.
Figure 7 shows the inter-annual (2001–2021) variations of the within-laboratory and between-laboratory reproducibility (Figures 7A, B)—the opposite of the robust coefficient of variation s*—as well as the trueness—the bias is measured as the absolute difference between the inter-laboratory median value and the robust mean—obtained during the ILC exercises. We here demonstrate how efforts made to fulfill quality requirements for trueness and reproducibility have contributed to improve the quality of SOMLIT data over time, confirming the essential role of ILC exercises as a tool for continuous improvement of long-term monitoring networks.
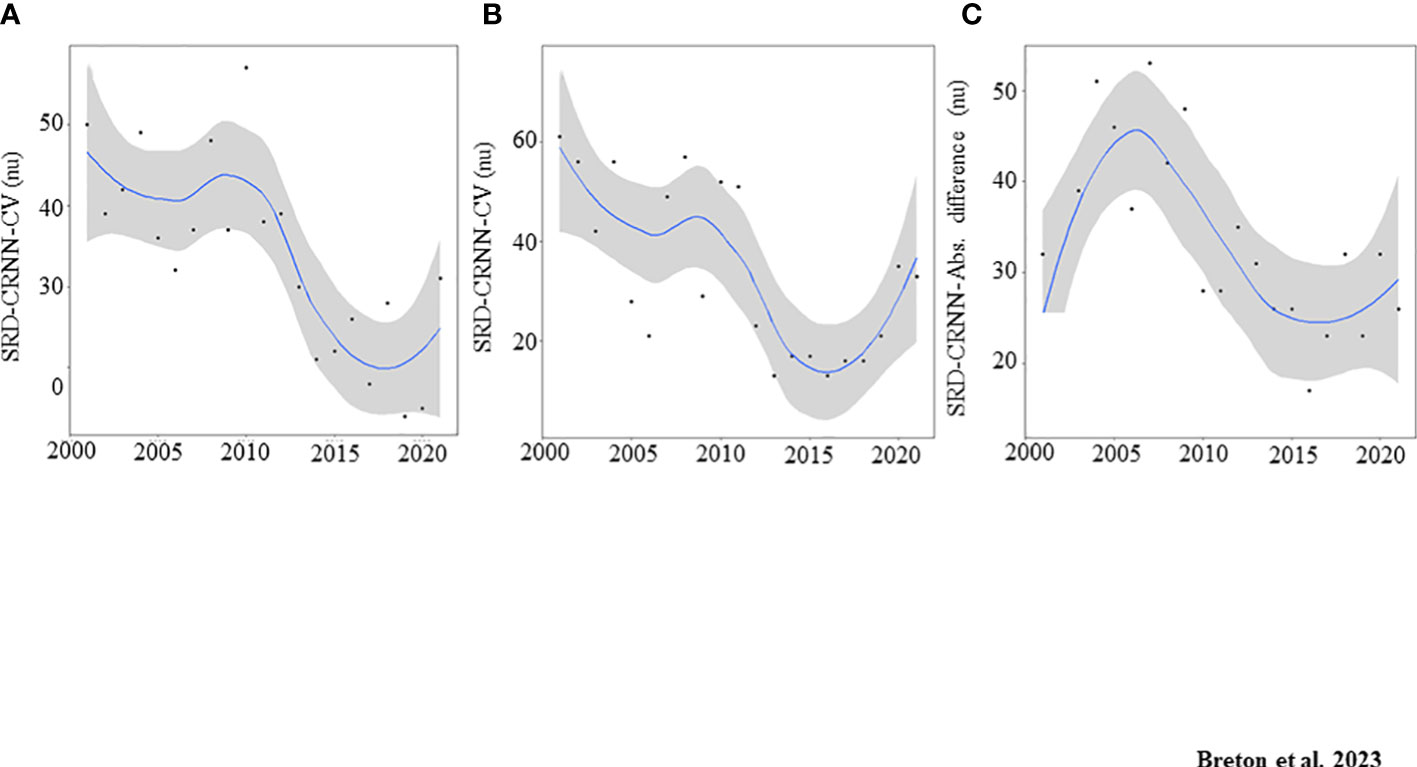
Figure 7 Long-term variations in (A) within-laboratory reproducibility (SRD-CRNN-CV, %), (B) between-laboratory reproducibility (the opposite of the robust coefficient of variation; SRD-CRNN-CV, %), and (C) trueness (bias measured as absolute difference between the inter-laboratory median value and the robust mean; SRD-CRNN-Abs. difference, nu) obtained during the inter-laboratory comparison exercises of the SOMLIT network over the period 2001–2021. The solid blue line and ribbon represent Locally Estimated Scatterplot Smoothing (LOESS) smoothing and the 95% confidence interval. SRD-CRNN, sum of ranking differences and comparison of ranks by random numbers; CV, coefficient of variation; nu, no unit.
Estimation of total measurement uncertainty (U) of the SOMLIT network
Two approaches exist for the evaluation of measurement uncertainty. The first is based on a physical model relating the measuring process at all steps, using the propagation law of uncertainty (GUM, 1993). The second relies on a statistical model that uses data from within-laboratory control and/or ILC exercises or PT exercises (ISO-13528, 2005; Ferretti, 2011; Magnusson et al., 2012; de Boer, 2016). The statistical model is often favored, however, and was chosen by the SOMLIT network both for its less apparent mathematical complexity and for the lack of knowledge about the processes that need to be measured for the physical model (e.g., firm statistical background). For that purpose, we used the data from ILC exercises from the last 6 years. Note that the values we obtained are an approximation of the total measurement uncertainty, given that sampling uncertainty was never investigated.
Total measurement uncertainty of the SOMLIT network (U, in ± Unit and/or in %) was calculated following Equation 4 (Magnusson et al., 2012):
where uRW is the precision uncertainty and b is the bias uncertainty obtained from Equations 5 and 6, respectively:
with σι and xi being the median and mean, respectively, of the repeatability values obtained by the different laboratories during one ILC exercise for one measure, sbias being the standard deviation of the different median values of b for at least six different ILC exercises, n being the number of ILC exercises used for uncertainty calculation, and ux being the median value of the uncertainty of the assigned values calculated for at least six ILC exercises. U is the expanded uncertainty at a confidence interval of 95% (k = 2).
The resulting expanded uncertainty values of the total measurement of the 22 environmental variables monitored by the SOMLIT network given in Table 1 (see also Table S3) are either below or very close to those found in the literature, when available.
Conclusion
In this paper, we presented the “road map” and the statistical methodology for the ILC exercises performed by the SOMLIT network, a framework especially adapted to small (<20 participating laboratories) and multivariate environmental datasets. By applying this framework to data acquired during 21 years, we showed that the SOMLIT program provided reliable data, and that the data quality increased over time thanks to the implementation of ILCs. Given the paucity of uncertainty values of the measurement of environmental variables—such as measured by the SOMLIT network—in the literature, our study stresses the importance of implementing ILC exercises in order to improve the quality of data representative of long-term environmental monitoring. We also highlight general acceptance limits in the measurement of these environmental variables.
Data availability statement
The raw data supporting the conclusions of this article will be made available by the authors, without undue reservation.
Author contributions
Conceptualization and methodology: EBr, NS, PR-M, BS, TC, SF, ACai, VD, YA, GGr, EGr, AG, OJ, VL, EMac, DM, FM, SM, DP, PP, FR-J, IS, P-GS, and NG. Data collection and curation: EBr, NS, PR-M, TC, SF, SAl, FA, SAu, EBe, HB, LB, SB, ACau, J-BC, LCh, RC’h, M-AC, LCo, OC, MC, HL, GD, JD, AD, MD, CE, SG, EGr, AG, JG, KG, GGu, VG, MH, OJ, PL, NLab, ML, VL, CLa, JL, NLac, BL, EL, DL, CLe, CLi, EMac, EMar, BMa, SM, LMor, LMou, AN, SN, RP, DP, FP, PP, FR-J, PRIM, CS, ES, RVa, FVi, FVo, LZ, and NG. Formal analysis: EBR and ALh. Project administration, funding acquisition, and resources: EBr, NS, PR-M, BS, TC, HA, CB, YB, SB, PCl, PCo, OC, ACai, YA, GGr, EGr, AG, MC, JF, MG, OJ, JL, LL, ALe, SL’H, YL, SM, FM, BMo, LMou, SN, PP, PRIM, ES, IS, LS, VV, RVu, P-GS, and NG. Writing—original draft preparation and writing—review and editing: EBr, NS, PR-M, EGo, ALh, VD, BL, BMo, PRIM, P-GS, FV, and NG. All authors contributed to the article and approved the submitted version.
Funding
This research received no specific grant from any funding agency.
Acknowledgments
The authors thank all the past participants involved in the inter-laboratory comparison exercises, especially Louise Oriol and Nicole Degros who greatly contributed to the implementation of the first exercises and the continuous improvement of SOMLIT data quality up to their retirement, as well as our enthusiastic and retired colleague Jean-Paul Lehodey and the numerous persons who contributed to the smooth running of the SOMLIT network: C. Arnaud, L. Beaugeard, G. Beaugrand, M. Breret, Y. Brizard, A. Brouquier, H. Derriennic, C. Desnos, V. Ferrer, M. Ferreri, L. Giannecchini, F. Guyon, G. Izabel, J-L Jung, V. Kempf, C. Larvor, S. Marro, M-L Pedrotti, Mireille Pujo-Pay, C. Rouzier, S. Soriano, E. Thiébaut, and H. Violette. The authors would also like to thank the different laboratories we visited over the last two decades for providing space and facilities, as well as the different captains and crew members of the research vessels of the marine stations and of the French National Fleet (Flotte Océanographique Française, FOF).
Conflict of interest
The authors declare that the research was conducted in the absence of any commercial or financial relationships that could be construed as a potential conflict of interest.
Publisher’s note
All claims expressed in this article are solely those of the authors and do not necessarily represent those of their affiliated organizations, or those of the publisher, the editors and the reviewers. Any product that may be evaluated in this article, or claim that may be made by its manufacturer, is not guaranteed or endorsed by the publisher.
Supplementary material
The Supplementary Material for this article can be found online at: https://www.frontiersin.org/articles/10.3389/fmars.2023.1135446/full#supplementary-material
References
Aminot A., Kérouel R. (2004). Hydrologie des écosystèmes marins: paramètres et analyses (Brest: Ifremer).
Aminot A., Kirkwood D. S. (1995). Report on the results of the fifth 1CES study for in seawater Vol. 213. Eds. Aminot A., Kirkwood D. S. (Copenhagen, Denmark:Coop. Res. Rep.), 41–65.
Analytical Methods Committee (1989). Robust statistics–how not to reject outliers–part 2. Inter-laboratory trials. Analyst 114, 1699–1705. doi: 10.1039/AN9891401699
Andrić F., Héberger K. (2015). Chromatographic and computational assessment of lipophilicity using sum of ranking differences and generalized pair-correlation. J. @ Chromatogr. A 1380, 130–138. doi: 10.1016/j.chroma.2014.12.073
Aoyama M. (2006). 2003 intercomparison exercise for reference material for nutrients in seawater in a seawater matrix. Tech. Rep. Meteorological Res. Institute (50), 91. doi: 10.11483/mritechrepo.50
Birchill A. J., Clinton-Bailey G., Hanz R., Mawji E., Cariou T., White C., et al. (2019). Realistic measurement uncertainties for marine macronutrient measurements conducted using gas segmented flow and Lab-on-Chip techniques Vol. 200 (Elsevier: Talanta), 228–235. doi: 10.1016/j.talanta.2019.03.032ff.ffhal-02182171f
Bockmon E. E., Dickson A. G. (2015). An inter-laboratory comparison assessing the quality of seawater carbon dioxide measurements. Mar. Chem. 171, 36–43. doi: 10.1016/j.marchem.2015.02.002
Carstensen J., Duarte C. M. (2019). Drivers of pH variability in coastal ecosystems. Environ. Sci. Technol. 53, 4020–4029. doi: 10.1021/acs.est.8b03655
Claramunt I., Pérez L. (2014). Estimation of measurement uncertainty in the determination of orthophosphates in seawater by continuous flow analysis (CFA). Accreditation Qual. Assur. 19, 205–212. doi: 10.1007/s00769-014-1051-x
Cocquempot L., Delacourt C., Paillet J., Riou P., Aucan J., Ballu V., et al. (2019). Coastal ocean and nearshore observation: a French case study. Front. Mar. Sci. 6. doi: 10.3389/fmars.2019.00324
de Boer J. (2016). Quality in scientific research – in commemoration of dr. David wells. Chemosphere 154, A1–A2. doi: 10.1016/j.chemosphere.2016.04.034
Duarte C. M., Conley D. J., Carstensen J., Sánchez-Camacho M. (2009). Return to neverland: shifting baselines affect eutrophication restoration targets. Estuaries Coasts 32, 29–36. doi: 10.1007/s12237-008-9111-2
Ferretti M. (2011). Quality assurance: a vital need in ecological monitoring. CAB Rev; 6, 1–14. doi: 10.1079/PAVSNNR20116011
Goberville E., Beaugrand G., Sautour B., Tréguer P., SOMLIT Team (2010). Climate-driven changes in coastal marine systems of western Europe. Mar. Ecol. Prog. Ser. 408, 129–148. doi: 10.3354/meps08564
GUM (1993). Guide to the Expression of Uncertainty in Measurement, International Organization for Standardization (ISO). 1993.
Harvey B. P., Gwynn-Jones D., Moore P. J. (2013). Meta-analysis reveals complex marine biological responses to the interactive effects of ocean acidification and warming. Ecol. Evol. 3, 1016–1030. doi: 10.1002/ece3.516
Héberger K., Kollár-Hunek K. K. (2011). Sum of ranking differences for method discrimination and its validation: comparison of ranks with random numbers. J. Chemometr. 25, 151. doi: 10.1002/cem.1320
Helm I., Jalukse L., Leito I. (2012). A highly accurate method for determination of dissolved oxygen: gravimetric winkler method. Analytica chimica Acta 741, 21–31. doi: 10.1016/j.aca.2012.06.049
Iavetz R. (2021). Proficiency test final report AQA 21-05 chlorophyll a in water (Sydney, Australia: National Measurement Institute. Australian Gorvenment), 44. Available at: https://www.industry.gov.au/sites/default/files/2021-11/aqa-21-05-final-report.pdf.
ISO-13528 (2005). Statistical methods for use in proficiency testing by inter-laboratory comparisons.
Kaas H., Wasmund N. (1996). Report on comparability of chlrophyll data. in: report of the ICES/Helcom workshop on quality assurance of pelagic biological measurements in the Baltic Sea (« annex 4 »).
Kalivas J. H., Héberger K., Andries E. (2015). ). sum of ranking differences (SRD) to ensemble multivariate calibration model merits for tuning parameter selection and comparing calibration methods. Anal. Chim. Acta 869, 21–33. doi: 10.1016/j.aca.2014.12.056
Larsson U., Norling L., Carlberg S., Lööf S., Tolstoy A., v. Bröckl K., et al. (1978). Intercalibration of methods for chlorophyll measurements in the Baltic Sea, merentutkimuslait. Julk./Havsforskningsinst. Skr. 243, 63–76.
Leito I., Strauss L., Koort E., Viljar P. (2002). Estimation of uncertainty in routine pH measurement. Accred Qual Assur. 7, 242–249. doi: 10.1007/s00769-002-0470-2
Lheureux A., David V., Del Amo Y., Soudant D., Auby I., Bozec Y., et al. (2023). Trajectories of nutrients concentrations and ratios in the French coastal ecosystems: 20 years of changes in relation with large-scale and local drivers. Sci. Total Environ. 857, 159619. doi: 10.1016/j.scitotenv.2022.159619
Lheureux A., Savoye N., Del Amo Y., Goberville E., Bozec Y., Breton E., et al. (2021). Bi-decadal variability in physico-biogeochemical characteristics of the temperate coastal ecosystems: from large-scale to local drivers. Mar. Ecol. Prog. Ser. 660, 19–35. doi: 10.3354/meps13577
Liénart C., Savoye N., Bozec Y., Breton E., Conan P., David V., et al. (2017). Dynamics of particulate organic matter composition in coastal systems: a spatio-temporal study at multi-systems scale. Prog. Oceanography 156, 221–239. doi: 10.1016/j.pocean.2017.03.001
Liénart C., Savoye N., David V., Ramond P., Rodriguez Tress P., Hanquiez V., et al. (2018). Dynamics of particulate organic matter composition in coastal systems: forcing to the spatio-temporal variability at multi-systems scale. Prog. Oceanography 162, 271–289. doi: 10.1016/j.pocean.2018.02.026
Magnusson B., Näykki T., Hovind H., Krysell M., Sahlin E. (2012) Handbook for calculation of measurement uncertainty in environmental laboratories. NORDTEST report TR 537. Available at: http://www.nordtest.info.
McCrackin M. L., Jones H. P., Jones P. C., Moreno-Mateos D. (2017). Recovery of lakes and coastal marine ecosystems from eutrophication: a global meta-analysis. Limnol. Oceanogr. 62, 507–518. doi: 10.1002/lno.10441
Medina-Pastor P., Mezcua M., Rodríguez-Torreblanca C., Fernández-Alba A. R. (2010). Laboratory assessment by combined z score values in proficiency tests: experience gained through the European union proficiency tests for pesticide residues in fruits and vegetables. Analytical Bioanalytical Chem. 397, 3061–3070. doi: 10.1007/s00216-010-3877-3
Meinrath G., Spitzer P. (2000). Uncertainities in determination of pH. Microchimica Acta A 135, 155–168. doi: 10.1007/s006040070005
Miloslavich P., Bax N. J., Simmons S. E., Klein E., Appeltans W., Aburto-Oropeza O., et al. (2018). Essential ocean variables for global sustained observations of biodiversity and ecosystem changes. Glob. Change Biol. 24, 2416–2433. doi: 10.1111/gcb.14108
Nordtest TR 569 (2007). Handbook of internal quality control for chemical analysis. technical report, Hovind, NIVA, Norway, Bertil Magnusson, SP, Sweden, Mikael Krysell et Ulla Lund, Eurofins A/S, Danemark, Irma Mäkinen, SYKE, Finland. Available at: http://www.nordtest.info/wp/wp-content/uploads/2012/01/nt-tr-569_ed4_en-internal-quality-controll-handbook-for-chemical-laboratories.pdf
Röttgers R., Heymann K., Krasemann H. (2014). Suspended matter concentrations in coastal waters: methodological improvements to quantify individual measurement uncertainty. Estuarine Coast. Shelf Sci. 151, 148–155. doi: 10.1016/j.ecss.2014.10.010
Sandoval SP, Dall'Olmo G., Haines K., Rasse R., Ross J. (2006). Uncertainties of particulate organic carbon concentrations in the mesopelagic zone of the Atlantic ocean. Open Res. Europe 1, 43
Schilling P., Powilleit M., Uhlig S. (2006). Chlorophyll-a determination: results of an inter-laboratory comparison. Accreditation Qual. Assur. 11, 462–469. doi: 10.1007/s00769-006-0158-0
Stoyke M., Radeck W., Gowik P. (2012). Anthelmintics in bovine milk and muscle: inter-laboratory studies among EU national reference laboratories accred. Qual. Assur. 17, 405–412. doi: 10.1007/s00769-012-0919-x
Sziklai B. R., Héberger K. (2020). Apportionment and districting by sum of ranking differences. PloS One 15 (3), e0229209. doi: 10.1371/journal.pone.0229209
Talarmin A., Lomas M. W., Bozec Y., Savoye N., Frigstad H., Karl D. M., et al. (2016). Seasonal and long-term changes in elemental concentrations and ratios of marine particulate organic matter. glob. biogeochem. Cycles 30, 1699–1711. doi: 10.1002/2016GB005409
Taverniers I., De Loose M., Van Bockstaele E. (2004). Trends in quality in the analytical laboratory. i. traceability and measurement uncertainty of analytical results. TrAC Trends Analytical Chem. 23, 480–490. doi: 10.1016/S0165-9936(04)00733-2
Keywords: environmental monitoring network, data quality control, inter-laboratory comparison exercises, measurement uncertainty, analyst performance, multivariate dataset
Citation: Breton E, Savoye N, Rimmelin-Maury P, Sautour B, Goberville E, Lheureux A, Cariou T, Ferreira S, Agogué H, Alliouane S, Aubert F, Aubin S, Berthebaud E, Blayac H, Blondel L, Boulart C, Bozec Y, Bureau S, Caillo A, Cauvin A, Cazes J-B, Chasselin L, Claquin P, Conan P, Cordier M-A, Costes L, Crec’hriou R, Crispi O, Crouvoisier M, David V, Del Amo Y, De Lary H, Delebecq G, Devesa J, Domeau A, Durozier M, Emery C, Feunteun E, Fauchot J, Gentilhomme V, Geslin S, Giraud M, Grangeré K, Grégori G, Grossteffan E, Gueux A, Guillaudeau J, Guillou G, Harrewyn M, Jolly O, Jude-Lemeilleur F, Labatut P, Labourdette N, Lachaussée N, Lafont M, Lagadec V, Lambert C, Lamoureux J, Lanceleur L, Lebreton B, Lecuyer E, Lemeille D, Leredde Y, Leroux C, Leynaert A, L’Helguen S, Liénart C, Macé E, Maria E, Marie B, Marie D, Mas S, Mendes F, Mornet L, Mostajir B, Mousseau L, Nowaczyk A, Nunige S, Parra R, Paulin T, Pecqueur D, Petit F, Pineau P, Raimbault P, Rigaut-Jalabert F, Salmeron C, Salter I, Sauriau P-G, Seuront L, Sultan E, Valdès R, Vantrepotte V, Vidussi F, Voron F, Vuillemin R, Zudaire L and Garcia N (2023) Data quality control considerations in multivariate environmental monitoring: experience of the French coastal network SOMLIT. Front. Mar. Sci. 10:1135446. doi: 10.3389/fmars.2023.1135446
Received: 31 December 2022; Accepted: 27 January 2023;
Published: 26 April 2023.
Edited by:
Rachel Przeslawski, New South Wales Department of Primary Industries, AustraliaReviewed by:
Paul Van Ruth, University of Tasmania, AustraliaMark Bushnell, National Ocean Service (NOAA), United States
Copyright © 2023 Breton, Savoye, Rimmelin-Maury, Sautour, Goberville, Lheureux, Cariou, Ferreira, Agogué, Alliouane, Aubert, Aubin, Berthebaud, Blayac, Blondel, Boulart, Bozec, Bureau, Caillo, Cauvin, Cazes, Chasselin, Claquin, Conan, Cordier, Costes, Crec’hriou, Crispi, Crouvoisier, David, Del Amo, De Lary, Delebecq, Devesa, Domeau, Durozier, Emery, Feunteun, Fauchot, Gentilhomme, Geslin, Giraud, Grangeré, Grégori, Grossteffan, Gueux, Guillaudeau, Guillou, Harrewyn, Jolly, Jude-Lemeilleur, Labatut, Labourdette, Lachaussée, Lafont, Lagadec, Lambert, Lamoureux, Lanceleur, Lebreton, Lecuyer, Lemeille, Leredde, Leroux, Leynaert, L’Helguen, Liénart, Macé, Maria, Marie, Marie, Mas, Mendes, Mornet, Mostajir, Mousseau, Nowaczyk, Nunige, Parra, Paulin, Pecqueur, Petit, Pineau, Raimbault, Rigaut-Jalabert, Salmeron, Salter, Sauriau, Seuront, Sultan, Valdès, Vantrepotte, Vidussi, Voron, Vuillemin, Zudaire and Garcia. This is an open-access article distributed under the terms of the Creative Commons Attribution License (CC BY). The use, distribution or reproduction in other forums is permitted, provided the original author(s) and the copyright owner(s) are credited and that the original publication in this journal is cited, in accordance with accepted academic practice. No use, distribution or reproduction is permitted which does not comply with these terms.
*Correspondence: Elsa Breton, ZWxzYS5icmV0b25AdW5pdi1saXR0b3JhbC5mcg==