- 1Department of Biological and Environmental Science, University of Jyväskylä, Jyväskylä, Finland
- 2Fisheries and Aquatic Production, Flanders Research Institute for Agriculture, Fisheries and Food (ILVO), Oostende, Belgium
Marine populations often show considerable variation in their productivity, including regime shifts. Of special interest are prolonged shifts to low recruitment and low abundance which occur in many fish populations despite reductions in fishing pressure. One of the possible causes for the lack of recovery has been suggested to be the Allee effect (depensation). Nonetheless, both regime shifts and the Allee effect are empirically emerging patterns but provide no explanation about the underlying mechanisms. Environmental forcing, on the other hand, is known to induce population fluctuations and has also been suggested as one of the primary challenges for recovery. In the present study, we build upon recently developed Bayesian change-point models to explore the contribution of food and climate as external drivers in recruitment regime shifts, while accounting for density-dependent mechanisms (compensation and depensation). Food availability is approximated by the copepod community. Temperature is included as a climatic driver. Three demersal fish populations in the Irish Sea are studied: Atlantic cod (Gadus morhua), whiting (Merlangius merlangus) and common sole (Solea solea). We demonstrate that, while spawning stock biomass undoubtedly impacts recruitment, abiotic and biotic drivers can have substantial additional impacts, which can explain regime shifts in recruitment dynamics or low recruitment at low population abundances. Our results stress the importance of environmental forcing to capture variability in fish recruitment.
1 Introduction
Fluctuations of population abundances are induced by stochastic processes related to demographic dynamics and environmental forcing (Rouyer et al., 2012). Empirical evidence shows that selective fishing amplifies these population fluctuations due to size-selective mortality and rejuvenation of the population structure (Hsieh et al., 2006; Anderson et al., 2008). Fish populations experiencing higher levels of variability in fishing are more responsive to temperature effects in their size structure (Tu et al., 2018), which suggests that fishing exacerbates the sensitivity of populations to climate-induced changes (Perry et al., 2010; Gamelon et al., 2019). Indeed, despite a general pattern of increasing population abundances following the release of a long history of excessive fishing pressure (Hilborn et al., 2020), the rebuilding of populations has only been partially successful (Zimmermann and Werner, 2019). Failure to account for environmental forcing in fisheries management has been suggested as one of the primary challenges to address this lack of recovery (Memarzadeh et al., 2019; Bastardie et al., 2021). The link between environmental drivers and population growth has been extensively demonstrated by studies investigating the causes of slow or absent recovery of collapsed Atlantic cod (Gadus morhua) populations (Beaugrand et al., 2003; Olsen et al., 2011; Sguotti et al., 2019a; Möllmann et al., 2021; Winter et al., 2023).
Two meta-analyses of over 200 fish populations worldwide showed that the ability to recover was best explained solely by population abundance in only a small number of populations, while the majority of populations were affected by productivity regime shifts, either with or without direct relationship to population abundance (Vert-Pre et al., 2013; Szuwalski et al., 2015). Populations rebuild through somatic growth and reproduction, whereby reproduction generally gets attributed a larger impact (Zimmermann et al., 2018; Stawitz and Essington, 2019). Reproduction is accounted for in fisheries management by the assumed relationship between the population biomass of spawning fish (Spawning Stock Biomass or SSB) and the number of recruits, i.e., young fish entering the fished population or stock. Recent meta-analyses of over 70 Northeast Atlantic fish populations demonstrated significant density-dependent recruitment in two thirds of the analyzed populations (Zimmermann et al., 2018; Rindorf et al., 2022), demonstrating that population abundance cannot be ignored when examining recruitment dynamics. Recruitment mostly operates through compensation, implying increased per capita population growth rates at low abundances (Hilborn et al., 2014; Rindorf et al., 2022). The inverse, depensation or the Allee effect, was found in fewer occasions, but debate on its importance is ongoing, as declined [per capita] population growth rates may act as an inhibitory force in rebuilding populations at very low abundances (e.g., Neuenhoff et al., 2018; Perälä et al., 2022; Winter et al., 2023).
The relationship between the population biomass of spawning fish and the number of recruits are modelled using Stock-Recruitment (SR) models, which were traditionally time-invariant and did not take temporal productivity changes into account (Beverton and Holt, 1957). If the recruitment dynamics are nonetheless non-stationary and experience regime shifts, then recruitment predictions derived using stationary SR models are misguiding and may lead to unintentional overfishing (Möllmann et al., 2021), particularly in the light of global climate change (Punt et al., 2014). Substantial scientific progress to improve our understanding of environmental forcing on SR relationships was achieved over the last decades (e.g. Kell et al., 2005; Dorner et al., 2013; Punt et al., 2014), and pursuing these research avenues is further encouraged to this day (Kjesbu et al., 2023). Our forecasting capacities using environmentally mediated SR models, however, remain generally insufficient to improve tactical management advice (Stige et al., 2013; Subbey et al., 2014; King et al., 2015; Haltuch et al., 2019; Maunder and Thorson, 2019; Uriarte et al., 2023). Fisheries management advice in the Northeast Atlantic (NEA), for instance, showed that environmentally driven recruitment is implemented in none of the 230 studied stock assessments, and in only 11 out of 102 short-term forecasts (Trenkel et al., 2023). Tactical operational management advice therefore uses alternative approaches to account for changes in productivity (Collie et al., 2021; Silvar-Viladomiu et al., 2021; Trenkel et al., 2023). These alternative approaches are required when the available data are insufficient to estimate SR models (Conn et al., 2010; Nesslage and Wilberg, 2019), but are also used in data-rich assessments by focusing on empirical methods without knowing the underlying ecological mechanism. Examples are the Peterman’s productivity method (Peterman et al., 2000; Silvar-Viladomiu et al., 2022a), state-space models whereby variation in productivity is stochastically assessed over time (e.g. Nishijima et al., 2021; Stock and Miller, 2021; Su, 2023), and the truncation of the recruitment time series to recent productivity conditions (Van Deurs et al., 2021; Silvar-Viladomiu et al., 2022b). Environmentally mediated SR models were, contrastingly, used in most of the scientific advice for rebuilding plans in the NEA (24 out of 30, Trenkel et al., 2023). Indeed, the need for continual mechanistic insights at broader level is recognized and required to inform strategic management in the longer term (Silvar-Viladomiu et al., 2022a; Kjesbu et al., 2023), for instance for the evaluation of climate-adaptive management measures (e.g. Holsman et al., 2020; Kühn et al., 2023).
Dynamic detection of regime shifts in real marine ecosystems and reliable predictions of recruitment are, however, notoriously difficult, even though incremental progress is being made (e.g. Nishijima et al., 2021; Kühn et al., 2021; Mazur et al., 2022). Early warning signals for critical transitions, at which the ecosystem shifts abruptly to an alternative productivity state, are being developed (Scheffer et al., 2009). These include elevated non-linearity and/or changes in the variance of population parameters as indicators of shifting dynamics (Dakos et al., 2017; Sguotti et al., 2019b). Bayesian Online Change-Point Detection (BOCPD) algorithms is one of the techniques that can successfully detect how SR parameter shifts over time (Perälä et al., 2017; Tirronen et al., 2022), and provides a promising method to account for regime shifts in recruitment dynamics. Concurrent time series analysis of environmental forcing variables and population productivity parameters have suggested coinciding regime shifts of environmental and climatic variables with population productivity (Thomson et al., 2010; Perälä et al., 2020), but despite their suggested importance (Sguotti et al., 2019b; Möllmann et al., 2021), the contribution of these environmental variables to regime shifts in SR parameters remains underexplored in BOCPD models.
In the present study, we build upon recently developed BOCPD models to explore the contribution of food and climate as external drivers in recruitment regime shifts, while accounting for density-dependent mechanisms (compensation and depensation). Food availability is approximated by the copepod community. Temperature is included as a climatic driver. Recruitment variation is considered annually at the population level and is partitioned between the variation that relates to spawning biomass and the variation that relates to environmental conditions. The focus on population-level SR models aims to facilitate integration in future stock assessments, management strategy evaluations and fisheries management (Maunder and Thorson, 2019). Recruitment variability can have a myriad of causes, including internal regulating factors such as population age structure (e.g. Hixon et al., 2013; Fogarty and O'brien, 2016) and external factors such as trophic interactions, hydrodynamics and environmental drivers (see Houde, 2016; Houde et al., 2022 for a review of drivers affecting early life stages). The increasing rate of climate-induced changes also manifests itself through a plethora of drivers of fish productivity, such as water temperatures, ocean acidification and shifts in hydrodynamics at individual, behavioral, population and ecosystem levels (Pörtner and Peck, 2010; Koenigstein et al., 2016). This study followed the suggestion by Rijnsdorp et al. (2009) that changes in the pelagic egg or larval stage and habitat alteration are key determinants of climate-induced recruitment success. Sea surface temperature is selected as a covariate for the pelagic phase, because pelagic egg and larval development and mortality are strongly temperature dependent (Gibson et al., 2015), as is the termination of the pelagic larval phase (Lacroix et al., 2018). Larval development may be further compromised by starvation and predation of which this study focused on larval prey availability only (Arevalo et al., 2023). Habitat alternation effects on recruits or juveniles manifest through a wealth of environmental drivers such as food abundance and quality, predators, river plumes, temperature and human pressures such as eutrophication and contamination (e.g. Brown et al., 2018). This study focused on sea bottom temperature as one of the primary habitat-related drivers that is affected by climate change (Henderson, 2019; Vaz et al., 2019), and because the onset of spawning is also driven by sea bottom temperatures (Gerritsen et al., 2003; Armstrong et al., 2004; Fincham et al., 2013).
Three demersal fish populations in the Irish Sea were selected as case study examples: Atlantic cod, whiting (Merlangius merlangus) and common sole (Solea solea). These commercially fished populations represent good candidates for depensation dynamics, as they met the threshold criterion defined by Hutchings (2014), whereby SSB was below 0.26 SSBMSY during some years of the observed time series. The Irish Sea has undergone regime shifts during the eighties whereby the exact year of abrupt changes depend on the considered species. Jellyfish abundance increased gradually since 1970 with frequent outbreaks in the eighties. Copepod biomass showed a step decrease in 1985, while phytoplankton showed a step increase in 1989 (Lynam et al., 2011). Across the entire North Atlantic region, calanoid copepod assemblages showed large-scale biogeographical shifts between 1958 and 2005, whereby assemblages generally shifted northwards following the movement of the 10°C isotherm (Beaugrand et al., 2009). Bayesian network analysis confirmed these zooplankton regime shifts in the Irish Sea (Mitchell et al., 2021). Zooplankton assemblages consisted of two separate ecological networks in the seventies, comprising a network of sub-arctic and temperate oceanic species such as Calanus finmarchicus and Acartia spp., and another network of warm temperate and shelf sea species such as Calanus helgolandicus, Pseudocalanus spp. and Temora longicornis. The eighties were characterized by a transitional period with significantly higher dependence on environmental factors. In the nineties there was an increased connectivity between all zooplankton networks, which provided a broader prey spectrum for generalist zooplanktonic predators such as the jellyfish Aurelia aurita (Mitchell et al., 2021) and fish larvae. Fish population biomass of spawning cod, whiting and sole decreased subsequently to levels that were too low to support a sustainable fishery (ICES, 2019; ICES, 2022a; ICES, 2022b). The finfish fishery collapsed despite consecutive years of reduced fishing pressure and management efforts (Bentley et al., 2020). The objective of the present study is to investigate whether the lack of recovery and reduced recruitment of the three studied fish populations was due to the interaction of low population abundances and environmental forcing. To this end, we search for regime shifts in the density-dependent recruitment dynamics and then explore whether those can be explained by environmental covariates.
2 Materials and methods
2.1 Bayesian change-point model
We modelled recruitment by a Bayesian change-point model that consists of a predictive model for recruitment and an unknown number of change points at which the parameters of the recruitment model change (Tirronen et al., 2022). The recruitment model included spawning stock biomass (SSB) and environmental factors as explanatory variables. Specifically, the relationship between the number of recruits, adjusted to be for age 0 and denoted by , and the corresponding values of SSB, denoted by , was modelled by nonlinear SR models. The impact of environmental variables on recruitment was modelled by including separate environmental coefficients to this relationship following the formulation by Hilborn and Walters (1992).
To estimate the relationship between recruitment, SSB and environmental factors and possible changes in this relationship on empirical data, we applied BOCPD (Adams and MacKay, 2007). The method processes data in a sequential manner, updating estimates for the parameter values and computing posterior probabilities for a change point after each data point. In the following, , , denotes the run length at time , i.e., the time elapsed since the last change point. We assumed that the run lengths form a Markov chain. Moreover, denotes the approximation of the model parameters at time , inferred from the run length . For BOCPD, the change point model was defined by the following distributions:
● the underlying predictive model for recruitment, where represent the measured values of environmental factors affecting the cohort of year
● the joint prior distribution for the model parameters,
● the change point prior,
● the initial run length,
2.2 Underlying predictive recruitment model
When modelling the relationship between recruits, SSB and environmental variables, we allowed depensation and regarded the impact of environmental variables as density-independent. The relationship between mean recruitment, denoted by and SSB was modelled by two different SR models: the sigmoidal Beverton-Holt (SBH) and Saila-Lorda (SL) models (Needle, 2001; Saila et al., 1988; Iles, 1994), hereafter called the plain SR models. The plain SR models were extended (Hilborn and Walters, 1992) to include a coefficient describing the impact of environmental factors ( omitted for simplicity of notation):
where is the plain SR model and
extends the plain SR models with the impact of environmental factors on recruitment (hereafter called extended SR models), the parameter describing the magnitude of the impact of each specific environmental variable .
The SBH model reads as
where is the asymptotic maximum number of recruits, is the SSB that produces half of and the parameter controls the type of the S-R relationship, producing compensatory recruitment when and depensatory recruitment when . The SL model read as:
Above, is the maximum number of recruits, produced when , and denotes the depensation parameter as for SBH. While for SBH, recruitment monotonically increases with increasing SSB to , the SL model is increasing when SSB and decreasing when SSB , representing overcompensation.
To consider variation from the mean relationship, we modelled the actual number of recruits, , as lognormally distributed around the mean SR relationship. Specifically, the logarithm of was modelled as a normally distributed random variable,
where is a variance parameter.
In our analysis, we considered models with one or two environmental variables as well as the plain SR models without environmental factors. Overall, the underlying predictive recruitment model had from four to six parameters, depending on the number of environmental variables. For two environmental variables, for SBH and for SL.
2.3 Priors
We assumed the parameters of the underlying predictive model to be a priori independent and used weakly informative priors for them, similar to the ones used in previous studies (Perälä et al., 2017; Tirronen et al., 2022). With such priors, the parameter values were constrained to realistic ones but any particular values in the chosen ranges were not favored. However, as high uncertainty in the priors may lead to high uncertainty in the posterior distributions of the model parameters and obscure changes in the parameters, we wanted to avoid priors with unnecessarily wide supports.
For the (asymptotic) maximum number of recruits, and (for SBH and SL, respectively), we used the uniform distribution as a prior, with and . Similarly, for and we used , with and . The prior distribution of the depensation parameter, was set to a mixture of two uniform distributions, that gives equal prior probabilities for depensatory and compensatory recruitment. The support of the distribution was bounded from below by to keep the value of strictly positive. The priors of the coefficients of environmental variables, , were initially set to but we also tested narrower supports within the range. For the results, we considered the supports to be wide enough, not restricting parameter inference. Moreover, the prior of the variance parameter, was set to As such, the upper boundary of was higher than the coefficients of variation in the studied recruit time series ( for cod, for common sole and for whiting). As for , the support of the distribution was bounded from below by to keep the value of strictly positive. However, since the lower boundary of may have a considerable impact on change-point detection (Tirronen et al., 2022), as it defines the amount of scatter that is required around the mean curve, we tested also higher values for the lower boundary (hereafter called regularization): for cod and common sole, and for whiting.
The change point prior was defined using a constant hazard function (Adams and MacKay, 2007),
, that gives as the prior probability of a change point. We set a high prior probability for a change point by fitting the model to the data sets with and assumed that a change point occurred before the first data point, i.e., .
2.4 Bayesian inference
To estimate the parameters of our nonlinear recruitment model, we combined the BOCPD method with simulation-based filtering (Liu and West, 2001; Perälä et al., 2017). In previous studies (Perälä et al., 2017; Tirronen et al., 2022), the method has been extensively validated for SR models. All the change-point analyses using BOCPD were run in MATLAB Release 2018b (The Mathworks, Inc., Natick, Massachusetts, United States).
BOCPD starts a new run at each time point, updates estimates for the parameter values in the existing runs and computes posterior probabilities of the run lengths (Adams and MacKay, 2007; S1.1 in Supplementary Material). Following Perälä et al. (2017), we used the run length probabilities obtained by filtering to compute smoothed run length probabilities, i.e., run length probabilities in retrospect, given the whole data (S1.2). The smoothed run length probabilities are less sensitive to single outliers than the filtered ones, and we used the smoothed probabilities to divide the data into segments. In this, we determined the most likely segmentation (MLS) of each data set by maximizing the product of the smoothed run length probabilities over all possible segmentations (Perälä et al., 2017; S1.2). We set the maximum number of segments to five and considered only segments that consisted of at least three years, except at the beginning and at the end of the time series, since the data cannot inform when the first segment started or the last one ended.
For parameter estimates, we considered the posterior distributions of the model parameters at the end of the inferred segments so that all the data within a segment would contribute to the segment-specific estimates. The medians of such posterior distributions of the parameters and are denoted by (omitting component-specific notations) and respectively. For evidence of depensation, we considered whether the posterior probability of depensation and were high ( and clearly above one) and whether there were data at low abundance (Tirronen et al., 2022).
When there are no missing data, BOCPD enables computing the model evidence (Adams and MacKay, 2007), or its counterpart, the negative log marginal likelihood (nlml; S1.3), which can be used for model comparison. Within this approach, the best fitting model is the one with the lowest nlml. However, nlml may considerably vary along with the priors used and the time range of the data. Because of this, we used it only for comparison of models with the same time range and same priors, particularly in terms of the variance parameter . Although we were interested in the highest evidence among all our models, we also considered alternative models for recruitment despite their overall rank. As such, we especially used nlml to guide ranking and selection among models with similar predictors.
The simulation-based filtering makes BOCPD a stochastic method, and we addressed variation in the results caused by the stochasticity by fitting the models to the data using three different random samples in the filter. In this, we found that samples in the filter produced estimates with sufficiently low variation for models with one environmental factor at maximum, while was enough for models with two environmental factors.
2.5 Empirical case study data
Population and recruitment data were taken from the Stock Assessment Database of the International Council for the Exploration of the Sea (ICES, 2021), which are based on estimated stock assessment outputs. All assessments were categorized as data-rich ICES stocks (i.e. Category 1 stocks, with full analytical assessment). Cod and whiting stocks were assessed using an Age-Structured Assessment Programme (ASAP) with commercial landings and multiple survey indices as input. The sole stock assessment was based on age-based analytical assessment (XSA) using commercial landings data and one survey index. Full details are provided in ICES (2018: 122-182) for cod, ICES (2021: 1285-1352) for whiting and ICES (2021: 1013-1081) for sole. The time series ranged from 1968 (cod age 0), 1980 (whiting age 0) and 1970 (sole, age 2) until 2018 and were sufficiently long (≥35 years) to examine the effect of productivity regime shifts on recruitment.
Environmentally-driven productivity changes were examined using i) sea bottom temperature (SBT) and sea surface temperature (SST) as proxy for climate-induced changes and ii) a copepod-based indicator as proxy for food availability during larval life stages. Annual SBT data were averaged from CTD (conductivity, temperature and depth) data in the ICES Dataset on Ocean HydroChemistry (ICES, 2022c). SBT was estimated at different depth levels (6, 17, 27, 37 and 47 m). SST was estimated from the modelled HadISST 1° latitude-longitude grid product (Rayner et al., 2003) by annual temperature means over the spawning grounds and seasons, between January and June (Fox et al., 2000; Ellis et al., 2012; Burns et al., 2019). Prey of fish larvae is dominated by copepods (Pepin, 2022), with a gradual shift from naupliar (larval) stages of copepods for first-feeding fish larvae to copepodite (juvenile) stages and eventually to crustaceans and/or fish when they grow larger (Pepin, 2022). Copepod abundances were derived from the Continuous Plankton Recorder (CPR) data (Richardson et al., 2006; Johns, 2022), and they were also limited by the spawning season. Data were spatially restricted around 53.5° northern latitude because missing records before the mid-1980s would bias the copepod abundances (Figure S1). Annual composition was constructed by applying standardized Principal Component Analysis on log-transformed abundances (PCA, using years x taxa). The first principal component (PC) explained 21.5%, the second 11.3% of the total variability (Figure S2). Over half of the taxa contributions to PC1 is based on young Calanus (stage I-IV) (11.6%), Para-Pseudocalanus (13.3%), Temora longicornis (11.3%) and Acartia (10.5%) species. Unidentified Centropages species (22.5%), Centropages typicus (17.5%) and Candacia armata (12.9%) are the main contributors to PC2 (Figures S3-S4). In our analyses, we considered both the raw PC time series as well as smoothed ones (Figure S5). The PC data had missing values in 1988. To be able to compute the model evidence, imputation was done using the average of the smoothed PC values in 1987 and 1989 (Table S2).
In the analyses, all the environmental time series were rescaled to have a mean of zero and a standard deviation of one. Environmental variables for cod and whiting were included without a time lag, as both species are recruited at age 0. Environmental variables hypothesized to affect the larval stages of common sole were included with a time lag of two years, while SBT was averaged over the entire juvenile period (Table S1).
All empirical data preparation were made using the R software (R Core Team, 2022) and additional R packages: ‘icesSAG’ (Millar et al., 2022) to access the ICES Stock Assessment Database, ‘FactoMineR’ (Lê et al., 2008), ‘factoextra’ (Kassambara and Mundt, 2020) for PCA and ‘MARSS’ (Holmes et al., 2012; Holmes et al., 2021) for smoothed PC time trends.
3 Results
For all three populations studied, the results suggested that the most prominent shifts from high to low recruitment could be explained by changes in sea temperature or food availability (Figures 1–6). The strongest evidence of environmental factors explaining recruitment was found for cod (Tables 1–3). Among the studied populations, there were differences in the environmental factors that could have explained their recruitment. Nonetheless, for all three populations, while the results suggested dependence between recruitment and either the PC1 or the PC2 trend, the raw PC time series were not found to predict recruitment.
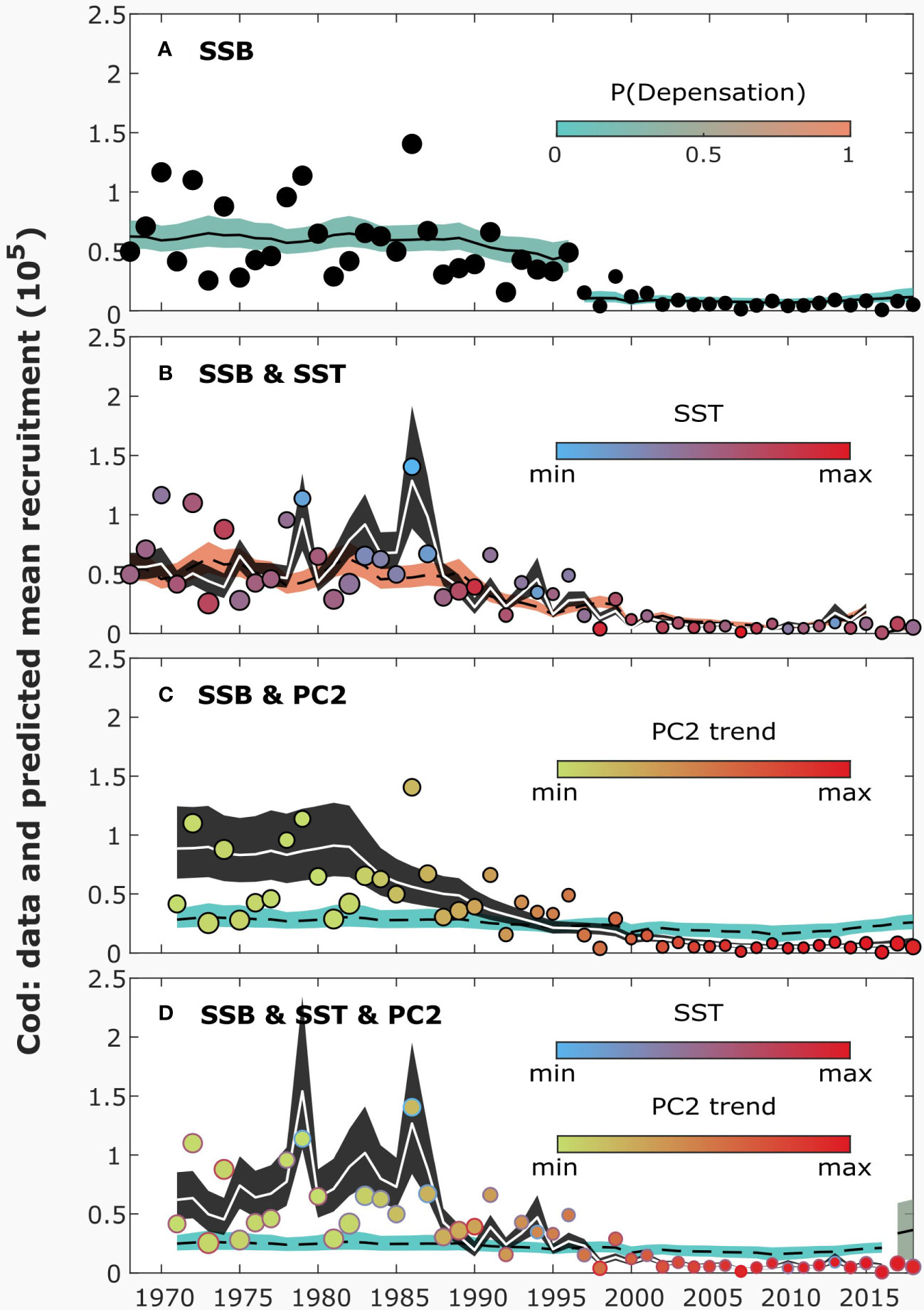
Figure 1 The data and predicted mean recruitment by different models for cod. The plain sigmoidal Beverton-Holt (SBH; equation 3; A) model includes only spawning stock biomass (SSB) as an explanatory variable. The extended SBH models (equation 1) include also sea-surface temperature (SST; B), plankton (smoothed PC2; C) or both of them (D). The colored areas (A-D) illustrate the 90% credible intervals of the plain SBH (equation 3) model over time, the colors scaled to the posterior probability of depensation in each inferred segment. The black areas (B-D) correspond to the 90% credible intervals of the extended recruitment models (equation 1). The position of dots (A-D) represents the number of recruits in each year, while their size is scaled to the value of SSB and their color (B-D) to the value of environmental variables. The outline color (D) refers to upper environmental driver (SST), the filled color to lower driver (PC2 trend). The solid lines show the medians of the posterior distributions. For the results, no regularization was used.
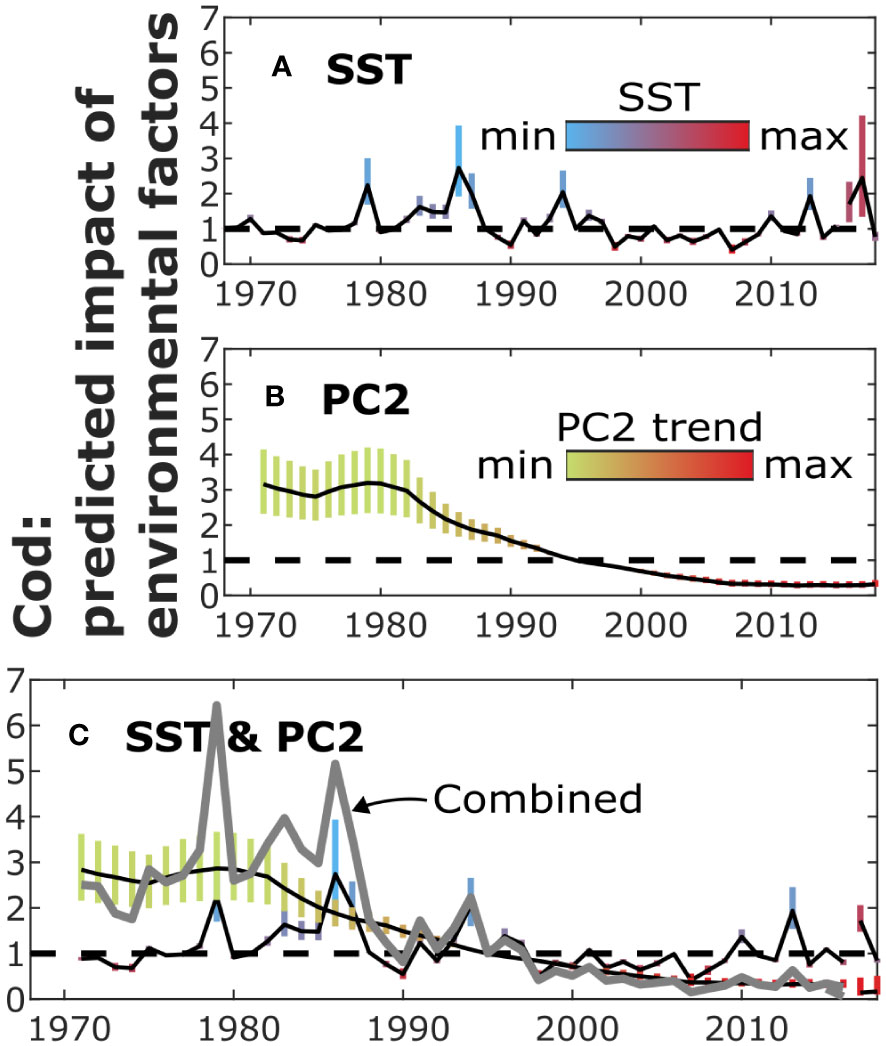
Figure 2 The predicted impact of environmental variables on recruitment by different models (Figure 1) for cod. The colored bars illustrate the 90% credible intervals of the impact of SST (A) and PC2 (B) as well as their combined impact (C) on recruitment (equation 2). The colors show the magnitude of the environmental variables themselves. The solid lines show the medians of the posterior distributions. Specifically, the grey line (C) illustrates the posterior median of the combined impact of SST and PC2.
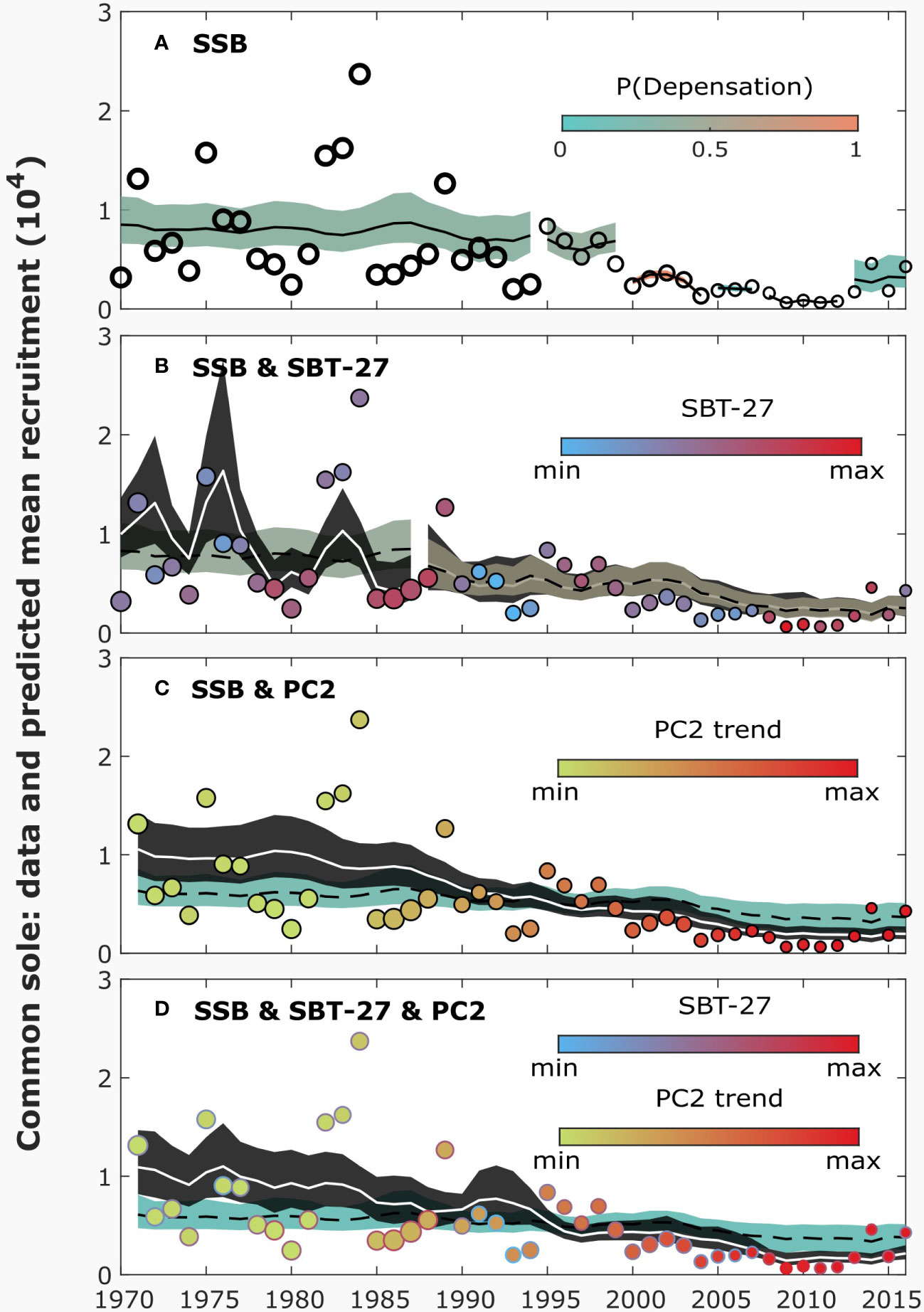
Figure 3 The data and predicted mean recruitment by different models for sole, illustrated similarly as in Figure 1. The plain sigmoidal Beverton-Holt (SBH; equation 3; A) model includes only spawning stock biomass (SSB) as an explanatory variable. The extended SBH models (equation 1) include also the sea-bottom temperature at the depth of 27 meters (SBT-27; B), plankton (smoothed PC2; C) or both of them (D). For the results, no regularization was used.
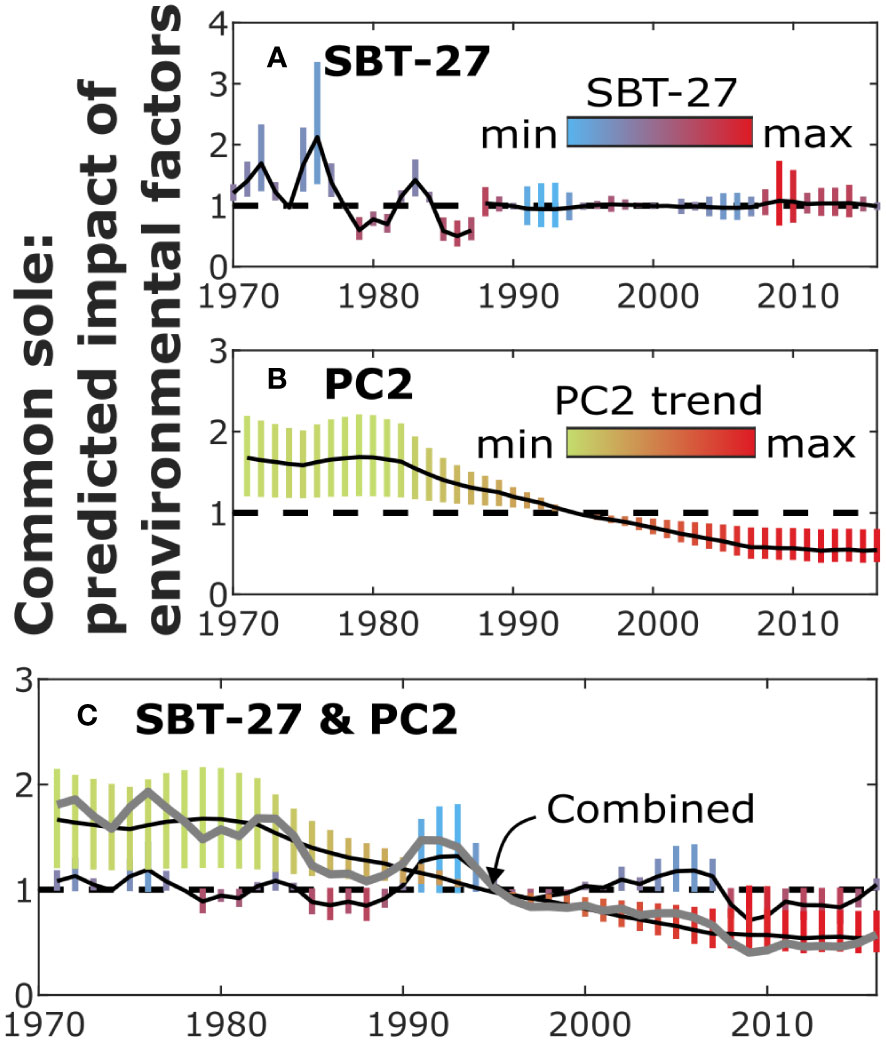
Figure 4 The predicted impact of environmental variables on recruitment by different models (Figure 3) for sole. The figure illustrates the impact of SBT-27 (A) and PC2 (B) as well as their combined impact (C) on recruitment, similarly as in Figure 2.
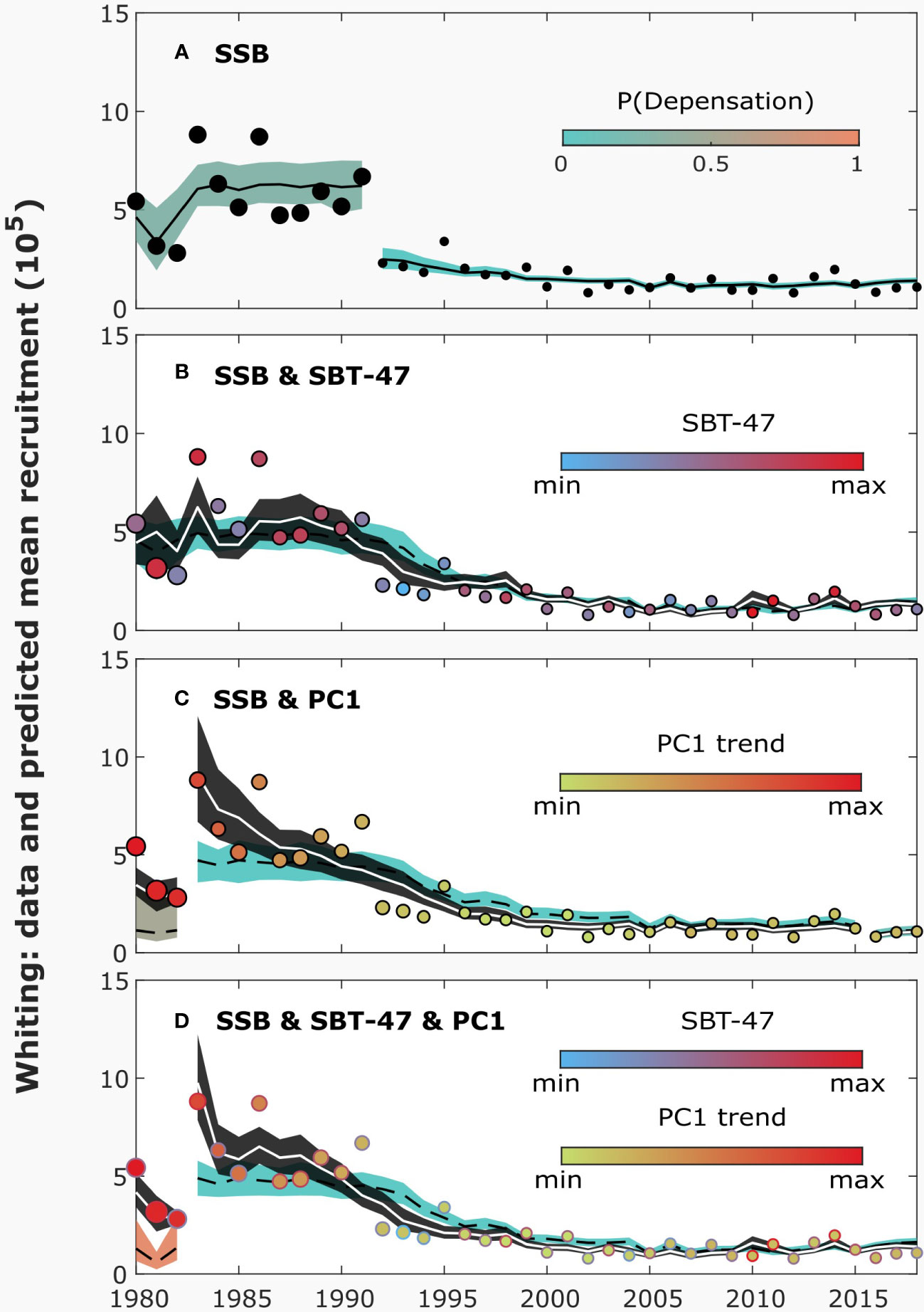
Figure 5 The data and predicted mean recruitment by different models for whiting, illustrated similarly as in Figure 1. The plain Saila-Lorda (SL; equation 4; A) model includes only spawning stock biomass (SSB) as an explanatory variable. The extended SL models (equation 1) include also the sea-bottom temperature at the depth of 47 meters (SBT-47; B), plankton (smoothed PC1; C) or both of them (D). For the results, no regularization was used.
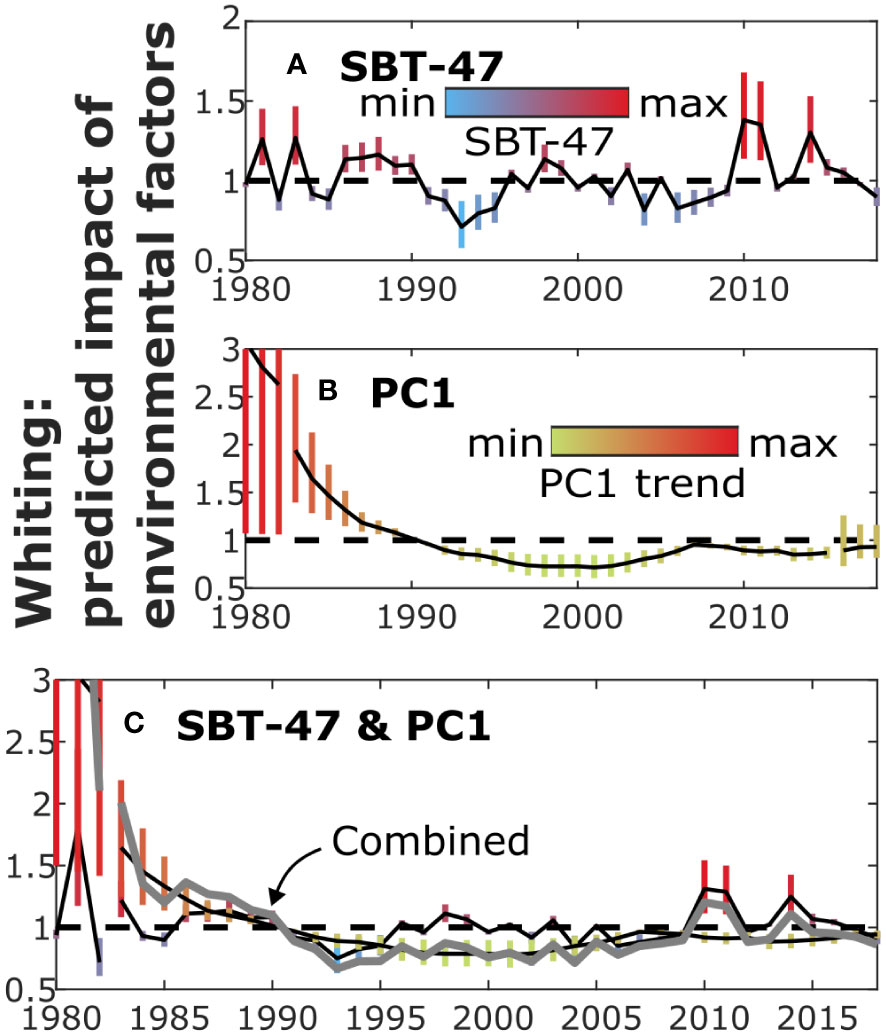
Figure 6 The predicted impact of environmental variables on recruitment by different models (Figure 5) for whiting. The figure illustrates the impact of SBT-47 (A) and PC1 (B) as well as their combined impact (C) on recruitment, similarly as in Figure 2.
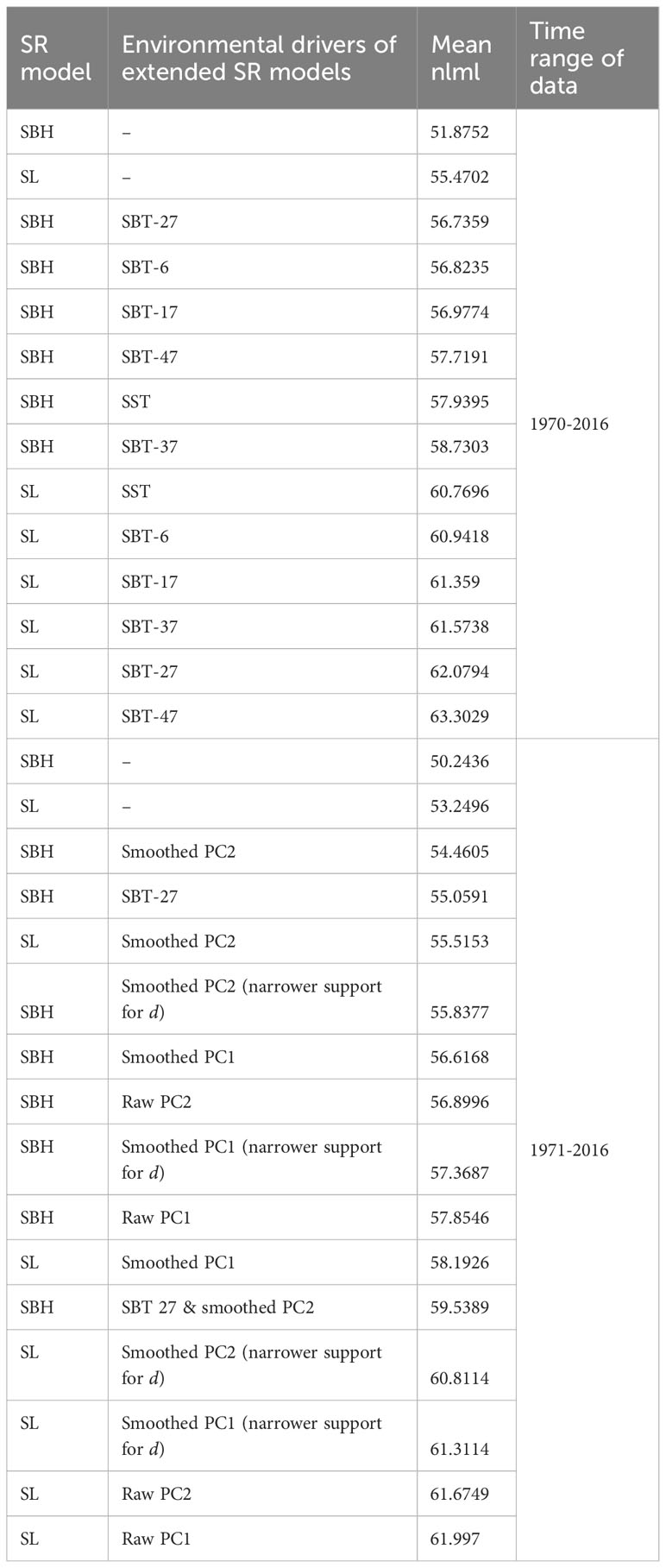
Table 2 Plain and extended SR models for common sole without regularization (see Table 1 for abbreviations).
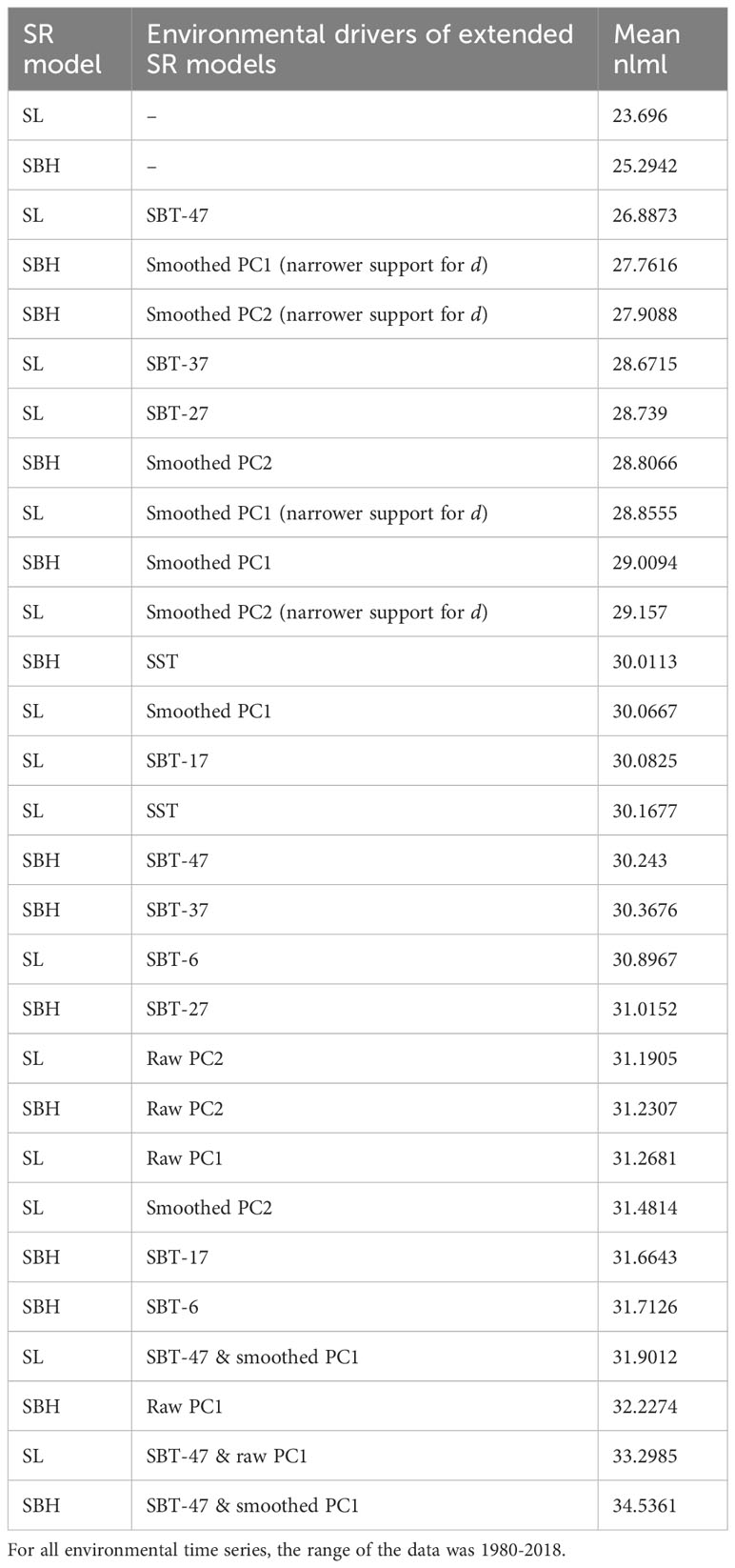
Table 3 Plain and extended SR models for whiting without regularization (see Table 1 for abbreviations).
While regularization may prevent overfitting of the plain SR models, it was found to diminish the impact of environmental variables, possibly distorting parameter estimation (Sections S3.1-S3.2, S4.1-S4.2 and S5.1-S5.2 in Supplementary Material). Thus, in the following, we focus on results without regularization, especially for models with environmental variables.
3.1 Atlantic cod
According to the obtained nlml values, the best fitting SR model for cod was SBH, with or without environmental variables (Table 1). Yet, both of the plain SR models suggested a change-point in 1996-1997 (Figure S8 in Supplementary Material), when the number of cod recruits experienced a downward shift, accompanied by a decrease in SSB (Figure 1A). The plain SBH model suggested that the asymptotic maximum number of recruits shifted to a lower level, accompanied by a minor change in and increased variation from mean recruitment (Figure S14a).
However, for cod, the results clearly suggested that changing environmental conditions (colored bars in Figure 2) explained the changes in recruitment. The strongest evidence for explaining recruitment was found for SST and the PC2 trend. SST yielded higher model evidence than PC2, although the difference was small (; Table 1). The extended SBH model including both SST and smoothed PC2 obtained the highest model evidence.
When environmental variables were included in the model, the results suggested considerably different recruitment dynamics than with the plain SR model. When SST was included in the model, evidence for a change point in 1996-1997 vanished. The results suggested that the recruitment of cod was inversely dependent on SST (Figures 1B, 2A; for most of the period). Starting around 1997, there was a period of approximately ten years with relatively high SST values, on average, when compared to the rest of the period. The results also suggested that a change in recruitment occurred in 2016, but as the segment consisted only of two or three years, the parameter inference remained uncertain.
Evidence of change points decreased also when the PC2 trend was included in the SBH model. While the filtered run length probabilities were high in 1997 (Figure S16a), only one of the samples used in model fitting yielded evidence for a change point in 1997 after smoothing. The results suggested that the recruitment of cod was negatively dependent on the PC2 trend (Figures 1C, 2B; among the best fitting models that included only the PC2 trend, = -0.9). In the smoothed PC2 data, values before and after 1995 were, respectively, lower and higher than the average of the studied period.
When both SST and the PC2 trend were included in the SBH model, the results were similar for each environmental factor. Nonetheless, the impact of PC2 was inferred weaker (Figures 1D, 2C; for most of the period) than for the model including only PC2. Evidence for a change point at the end of the period (2017) was obtained, as for the model including only SST, but there were only two years of data for parameter inference.
For cod, the results did not yield strong evidence for depensation. When SST was included in the model, the posterior probability of depensation was one during the whole period, but the second segment consisted only of three years and, in the first segment (1968-2015), was low (), yielding more evidence for compensatory recruitment than for depensation. Nonetheless, during the studied period, the recruitment of cod was at its lowest in 2016, accompanied by low SSB (Figure 1A).
3.2 Common sole
Of the plain SR models for common sole, SBH obtained higher model evidence than SL (Table 2). However, for SBH, MLS consisted of considerably many change points at the end of the time series (Figure 3A). Regularization decreased the number of change points, yielding evidence only to the years 2000 and 2013 and no considerable difference in nlml between SBH and SL (Figures S19, S21). The inferred change points reflected the shifts in the SR data from 1995 onwards (Figure 3A). In 1995, SSB shifted to a lower level, accompanied by a decrease in average recruitment in 2000. In 2008, SSB (and recruitment) shifted to a lower level still but, in 2013, recruitment partly recovered.
The evidence of environmental factors explaining recruitment was weaker for common sole than for cod, as the plain SR models obtained the highest model evidence (Table 2). Nonetheless, the results suggested that the recruitment of common sole was negatively dependent on the PC2 trend, although the relationship was weaker than for cod (Figures 3C, 4B; Figure S20; for SBH). Among the best fitting models, evidence of change points vanished when PC2 was included in the SBH model, similarly as for cod.
Moreover, although models including sea temperature were not ranked first according to nlml, the predicted impact of SBT-27 suggested an inverse relationship between recruitment and the sea temperature (Figures 3B, 4A). Some evidence of similar impact was also found for SBT-6 and SBT-17 (Figures S24b, c). When SBT-27 was included in the SBH model, the number of inferred change points decreased considerably, yielding evidence only to 1988. Interestingly, the results suggested that the dependence of recruitment on SBT-27 had vanished in 1988 ( switched from -0.6 to 0). The results suggested a similar effect for SBT-17 and SBT-6. Although the SBH model including PC2 obtained higher model evidence than the one including SBT-27, the difference was not considerable ( for mean nlml).
When both SBT-27 and the PC2 trend were included in the model, the predicted impact of PC2 did not change, when change points obtained no evidence (Figures 3D, 4C). In such a case, the impact of SBT-27 was inferred weak (). When the change point in 1988 gained support, the predicted impact of SBT-27 remained similar but the results suggested that the impact of PC2 shifted from positive to negative in 1988 ( switched from 0.2 to -0.5). In addition, a change in the parameters was inferred in 1973 but the first segment consisted of only two years. Overall, the combined impact of SBT-27 and PC2 remained uncertain.
Depensation evidence was found for the plain SBH model between 2000-2012, with and without regularization (the posterior probability of depensation was 0.9 and with regularization), although recruitment was not inferred depensatory for the whole period in the latter case. Similar results were obtained for SL. Both SSB and the number of recruits were low during the suggested depensation period. Nonetheless, when SBT-27 or the PC2 trend was included in the model, evidence of depensation vanished.
3.3 Whiting
For whiting, model evidence was highest for the plain SL model, with a change point in 1992 (Table 3, Figure S36). The parameter estimates suggested that the change occurred mostly in the maximum number of recruits, accompanied by smaller changes in other parameters (Figure S42a). In the SR data of whiting, there was a considerable downward shift in the number of recruits and SSB in 1992 (Figure 5A).
As for common sole, the evidence of environmental variables explaining recruitment was not strong for whiting (Table 3). Highest evidence of environmental factors explaining recruitment was obtained for SBT-47. The relationship between recruits and SBT-47 was inferred positive (Figures 5B, 6A; was 0.1-0.2). No evidence for a change point was found, when SBT-47 was included in the SR model. Similar, but more uncertain, impacts on recruitment were suggested for SBT-27 and SBT-37 (Figures S40d-e). These sea-bottom temperatures were at their lowest in 1993 during the period.
Highest model evidence among plankton variables was obtained, when the PC1 trend was included in the SBH model. Although model evidence was lower for SL than for SBH in this case, the highest evidence was obtained by using PC1 also for SL. The relationship between recruitment and the PC1 trend was inferred positive for both SR models (Figures 5C, 6B; was 0.3-0.4 for most the period). The inclusion of PC1 yielded to some evidence of change points, but they were located at the beginning and end of the time series (1983 and 2016) so that a change point in 1992 was not supported. In the smoothed PC1 data, values before and after 1990 were, respectively, higher and lower than the average of the studied period.
When both SBT-47 and the PC1 trend were included in the SR models, the predicted environmental impacts did not change considerably (Figures 5D, 6C; was 0.1 and 0.3 for most of the period, respectively). In this case, evidence of a change point in 1983 was still obtained.
For whiting, no evidence of depensation was found. Although the posterior probability of depensation was high for some models, the suggested depensation segments lied within 1980-1991, during which SSB and the number of recruits were not low.
4 Discussion
Regime shifts and compensation/depensation are properties that emerge from population dynamics. In other words, they are empirically detectable patterns but provide no explanation about the underlying causal mechanisms. In the present study we demonstrated that the inclusion of climate and food abundance related covariates confounded these patterns, which can be seen through the disappearance of statistical support for regime shift and depensation. In general, lower recruitment was linked to reduced food availability for the recruits (positively related to the smoothed PC1 trend and inversely to the smoothed PC2) and was further affected by temperatures. These results are in line with previous studies that provide evidence about the importance of environmental drivers on recruitment (Möllmann et al., 2021; Winter et al., 2023). While spawning stock biomass undoubtedly impacts recruitment and, albeit our study cannot rule out or estimate the role of other potential environmental covariates, our study suggests that temperature and prey abundance may have substantial impacts on recruitment and that these drivers can explain regime shifts in recruitment dynamics or low recruitment at low population abundances (i.e., the presence of Allee effects).
In our Irish Sea study system low population levels eventually resulted in the collapse of the finfish fishery (Bentley et al., 2020), and the flatfish beam trawl fishery temporarily collapsed when the landings of their primary target species plumetted from over 1000 tonnes of sole (2001-2003) to a historic low of<100 tonnes (2014-2018) (ICES, 2022b). The Irish Sea fisheries shifted target assemblages from demersal fish to invertebrate fisheries, following the global trend towards invertebrate fisheries (Anderson et al., 2011). Failure to account for a shift to low recruitment patterns in fish population could be one of the causes for the collapse of the socio-ecological system (Thurstan and Roberts, 2010; Britten et al., 2016). The lacking recovery of Irish Sea fish populations and its related management failure (Kelly et al., 2006) has led to a scientific endeavour to incorporate ecosystem considerations into single species stock assessments, such as the results from the Irish Sea Ecopath with Ecosim model (Bentley et al., 2021). This Irish Sea case study follows the wider ranging trend whereby ecosystem considerations are slowly being incorporated into the existing advisory and management frameworks of single species stock assessments (Link, 2002; Howell et al., 2021; Trenkel et al., 2023), and which is particularly relevant in the face of forestalling climate driven collapses (Holsman et al., 2020). This pragmatic and incremental approach is envisaged as a valuable step forward, although Thorpe et al. (2021) suggested that its implementation requires further scrutiny, such as resolving the discrepancies between ecosystem and single species models. Our study intends to boost the increased uptake of ecosystem considerations in operational fisheries management by investigating why the fishing mortality reference points in Bentley et al. (2021) required environmental mediation, and this is, more precisely, addressed by investigating how environmental mediation affected stock-recruitment relationships of three key single species populations. One of the pathways to assess recovery failure by reduced recruitment performance was already captured in modelling approaches of Tirronen et al. (2022) by considering dynamic changes in the type of recruitment, i.e., temporary Allee effects. The present study takes a next step by extending these models to include environmental factors.
The results of the present study should nonetheless be viewed critically, as several uncertainties are, as usual, involved with complex data and complex models. Population and recruitment data were based on modelled outputs from stock assessments rather than ‘raw’ survey observations, following standard practice in similar recent studies (e.g. Zimmermann et al., 2019; Brosset et al., 2020; Tirronen et al., 2022; Bell et al., 2023; Ma et al., 2023). Although these data represent the best available source of integrated information across the entire population, and are subject to regular quality control, given their use in management advice (Szuwalski et al., 2019; Silvar-Viladomiu et al., 2022a), we acknowledge criticism in using modelled outputs as ‘data’ in analyses (Brooks and Deroba, 2015) and call for caution by suggesting the use of our study results as one of the multiple lines of evidence (Thorson et al., 2015; see discussion on case study species below). Extending SR data with environmental variables can be dangerous practice, as it may be easy to find spurious correlations (Hilborn and Walters, 1992; Subbey et al., 2014). We re-iterate Bentley et al. (2021) by recognizing our limited forecasting ability, as environmental forcing variables cannot be used to infer causal relationships. The environmental drivers in our analysis, however, coincide with the prominent shifts and overall SR dynamics of the studied populations, rather than being associated with a few data points. Moreover, although extending SR models by environmental factors can lead to overfitting, the inclusion of environmental data can also prevent overfitting by nonstationary SR models, as shifts in recruitment may get explained by changes in environmental factors. Another point of potential criticism is that, when the number of parameters increases in BOCPD, this may yield to increased uncertainty in parameter estimates and change point inference which may, ultimately, obscure change points. To mitigate this, we aimed to use as informative prior distributions for the model parameters as were reasonable. It should also be noticed that, although we presented only the results according to the most likely segmentation of the data sets, BOCPD provides the posterior probability of a change point for each year and enables addressing alternative segmentations. Lastly, we call for caution in directly incorporating the environmental drivers of the SR models in operational management advice, as concerns have been raised about the species-specific nature of the environmental mediaton of SR models (see below for more details on our case study species) and about the non-stationary nature of these relationships. Moreover, the inclusion of appropriate environmental drivers further depends on the availability of relevant long-term data series. Our study focused on the inclusion of environmental drivers in BOCPD models, which requires a broader framework if it is to be used for fisheries management advice. To this end, we refer to a non-exhaustive list of reviews on good practices (e.g. Crone et al., 2019; Maunder and Thorson, 2019; Punt, 2023) and on the pros and cons of using environmental forcing in SR models (Subbey et al., 2014; King et al., 2015; Haltuch et al., 2019; Sharma et al., 2019; Silvar-Viladomiu et al., 2022a).
In our case study, the use of environmental variables had the strongest evidence for cod SR models. When environmental forcing was ignored, the cod time series was segmented into two periods (prior and post 1997) which corroborates the findings of Bentley et al. (2020); Bentley et al. (2021). When environmental forcing variables were included, our time series was best represented by one prolonged period (ignoring the last few years where parameter estimation was uncertain). The regime shift was explained away by including temperature and food as environment-mediating variables. Those results are in line with findings of cod recruiment models in the North Sea, which substantially improved when food and climate were added as environmental covariates (Olsen et al., 2011). Cod recruitment levels were low when food abundance was low but increased with increasing prey availability. Under high food abundance, cod recruitment levels ceased to increase at higher temperatures. The North Sea cod model explained 45 per cent of the total variance when food and climate were included, whereas only 10 per cent of the variance was explained by the traditional Beverton-Holt models (Olsen et al., 2011).
The importance of prey availability for controlling early life stages has led to several recruitment hypotheses highlighting prey abundance as a critical constraint of larval production, such as the critical period hypothesis by Hjort (1914), and the match-mismatch hypothesis by Cushing (1990) (Houde et al., 2022). The mismatch of timing schedules and levels of zooplankton production was identified as an important determinant of low recruitment levels for several fish species (Peck et al., 2012). Prey preferences of fish larvae are primarily (but not exclusively) characterized by their size. Cod and whiting have a similar prey size selection and exhibit pronounced preferences for Calanus species, even when abundances of Calanus species are low such as in the Irish Sea (Heath, 1992; Rowlands et al., 2006; de Figueiredo et al., 2007; Rowlands et al., 2008). The synchronous seasonality of high abundances of Calanus finmarchicus and young gadoids was hypothesized to support recruitment of cod and whiting in the Irish Sea and to coincide with the oscillations in the NAO (Nash and Geffen, 2004). Cod larvae preferentially select Calanus species, but also prey upon Para/Pseudocalanus and Temora species (Pepin, 2022). Other planktonic prey such as Oithona and Centropages species are often found in disproportionally low amounts in cod larvae in comparison to their environmental incidence, which implies that cod larvae defer from predating those species (Heath and Lough, 2007). These prey selection patterns explain why the increasing trends of the smoothed PC2 (with a higher prevalence of Centropages species) nullifies the change point in the cod SR model without environmental predictors. The increasing prevalence of Centropages species and a reduction in abundance of Calanus species coincided with a reduction in cod recruitment. It appears that the timing of prey availability and first-feeding cod larvae is not only correlated (Nash and Geffen, 2004) but also coincident. Neuheimer et al. (2018) suggested that the temperature-induced variation in spawning peak (up to 86 days across Atlantic cod populations) is related to the local adaptation of parental cod, which are matching their spawning time to environmental conditions in order to match the abundance of first-feeding larvae with the expected occurrence of prey abundance.
For whiting, the evidence for using environmental variables in the SR models was, however, not strong. Whereas our study corroborate a regime shift from high to low whiting recruitment in the early 1990s and whereas inclusion of environmental covariates nullified this regime shift, model evidence was higher for whiting models without environmental forcing variables. Environmental mediation of whiting recruitment was suggested to follow similar mechanisms as for cod (Lauerburg et al., 2018; Bentley et al., 2021). The diet composition of whiting larvae is indeed largely similar as that of cod larvae throughout their larval development (Rowlands et al., 2008), but low whiting recruitment has also been linked to other factors such as forage fish abundance and predation mortality in the North Sea and the interplay of temperature-induced climatic changes and benthic prey availability in the Bristol Channel (Floeter et al., 2005; Lauerburg et al., 2018; Henderson, 2019). Other differences between cod and whiting recruits include settlement duration, which occurred in a single pulse of one month for cod in the North Sea whereas whiting settled over a more protracted period (Bastrikin et al., 2014). Settlement in the nursery areas depends on the timing and location of adult spawning and the developments of egg and larval stages, which are both strongly mediated by temperature (Kjesbu, 1994; Gerritsen et al., 2003; Armstrong et al., 2004; Geffen et al., 2006).
Indeed, while density dependence and prey availability are highly cited as drivers of fish productivity, temperature is the most cited factor affecting recruitment (Savina-Rolland et al., 2022) and suggested as a primary driver of changes in fish productivity (Rijnsdorp et al., 2009; Pörtner and Peck, 2010; Koenigstein et al., 2016), supporting the inclusion of temperature as an appropriate candidate forcing variable to explain recruitment variability. Temperature correlated well with cod and whiting recruitment in earlier Irish Sea studies (Planque and Fox, 1998; Beggs et al., 2014; Bentley et al., 2020) and with sole recruitment in the North Sea and Bristol Channel, albeit not in the Irish Sea (Rijnsdorp et al., 1992; Henderson and Seaby, 2005; Akimova et al., 2016). Temperature affects the early life stages of fish in a myriad of ways, and is manifested through both physical and biological processes (Houde, 2016). Direct biological temperature effects relate to fishes’ ectotherm nature, implying that physiological changes are instantly affected by temperature (growth, metabolism, reproduction) (e.g. McBride et al., 2015). Indirect temperature effects relate to hydrodynamics and their physical environment (Ohlberger, 2013; Houde, 2016) and to changes in the food web (Cushing, 1990; Beaugrand et al., 2003; Olsen et al., 2011).
Temperature effects have differential effects on our case study species, as their dome-shaped thermal optima differ. When thermal ranges were prorated by their spatial distribution, they varied between 3 and 12°C for cod, 9 and 13°C for whiting and 10 and 19°C for sole (Bentley et al., 2019). Cod is a boreal species with its southern range edge to the south of the Irish Sea, while the Irish Sea is in the center of the distributional range of whiting (Baudron et al., 2020). Sole is at its northern distributional edge (Hermant et al., 2010). Species at the edge of their latitudinal ranges are potentially more susceptible to climate-induced changes beyond their preferred thermal ranges, the ‘species range hypothesis’ (Neuheimer et al., 2011), and particularly so for spawners and embryos (Dahlke et al., 2020). Indeed, cod recruitment has shown a highly significant and pronounced correlation with temperature signals across a latitudinal gradient, whereby warmer years increased cod recruitment in cold waters and colder years favored cod recruitment in warmer waters (Planque and Fox, 1998; Brander, 2000; Pörtner et al., 2001). The pattern of recruitment variation along latitudinal gradients was confirmed for gadoids (Leggett and Frank, 1997), but the species range hypothesis was rejected as a generic pattern for flatfishes (Leggett and Frank, 1997; Philippart et al., 1998) even though it was apparent for sole. Sole spawning and recruitment starts earlier in southernmost areas (Vaz et al., 2019) and has been shown to exhibit an increased variability at its northern distributional ranges, as demonstrated by a higher coefficient of variation for recruitment and growth in the Irish Sea and the North Sea as opposed to its southern population in the Bay of Biscay (Rijnsdorp et al., 1992; Leggett and Frank, 1997). Several studies suggested that sole recruitment variability was predominantly generated during the pelagic egg and larval stages and the variability of larval supply to the nursery areas (van der Veer et al., 2000; Gibson et al., 2015). These findings motivated the inclusion of environmental forcing variables in the pelagic stage, and temperature as main temporal driver during the benthic stage. Our results, however, suggest that sole recruitment was only partially explained by the inclusion of copepod prey and temperature. One potential reason is that not only copepod prey contribute to the diet of sole larvae, but also the larvae of polychaetes and bivalves (Last, 1978; Fonds, 1979; Lagardère et al., 1999). Another plausible reason is that sole only recruits at the age of two years in the Irish Sea, in contrast to whiting and cod (recruitment age of zero). The longer pre-recruit window extends the period of environmental mediation, and complicates the inclusion of the most crucial environmental variables (Haltuch et al., 2019; Brosset et al., 2020). Habitat-related processes during its spatially concentrated benthic juvenile stage were expected to dampen rather than to generate recruitment variability (van der Veer et al., 2000), but occasionally the opposite is true, e.g. during cold and strongly varying winter temperatures (van der Veer et al., 2000), or due to human-induced habitat degradation (Brown et al., 2018).
Overall, we conclude that BOCPD models can successfully identify regime shifts and compensatory or depensatory mechanisms. When regime shifts of fish recruitment are concurrent with environmental regime shifts, and nullified by inclusion of environmental forcing variables in the SR models, then we suggest that recruitment is likely impaired by selected environmental drivers. The low whiting and sole recruitment could only be partially explained by planktonic prey and temperature, which suggests that an expanded selection of environmental drivers is required. The tenfold reduction in Irish Sea cod recruitment of the late nineties was not satisfactorily explained by depensatory mechanisms alone but was convincingly nullified by increasing temperatures and reduced planktonic prey. This finding corroborates with similar findings in the North Sea (Olsen et al., 2011), and warrants further testing of cod SR models that account for food and temperature mediation. The present study hereby demonstrates how the formerly identified regime shifts of several ecosystem constituents in the Irish Sea have also affected the recruitment of fish populations. Retrospective BOCPD time series analysis provides a useful tool to this end. BOCPD helps identifying ecosystem shifts to low productive states, and the possible environmental drivers that affect the altered SR relationships.
Data availability statement
The datasets presented in this study can be found in online repositories. The names of the repository/repositories and accession number(s) can be found below: https://doi.org/10.5061/dryad.0k6djhb3m.
Author contributions
MT, JD and AK conceived the research idea. MT and JD designed the methodology. JD collected the data, MT analyzed the data with inputs from JD. All authors contributed to the article and approved the submitted version.
Funding
The contribution of JD was conducted in the framework of the H2020 project SEAwise (Societal challenges, GA number 101000318). This study was funded by the Academy of Finland (project grant 317495 to AK). This project has also received funding from the European Research Council (ERC) under the European Union’s Horizon 2020 research and innovation programme (grant agreement No 770884).
Acknowledgments
We are grateful for the support by David Johns on CPR survey data. Early ideation was supported by conceptual discussions with David Reid, Jacob Bentley and Anna Rindorf. We thank the associate editor and peer reviewers for their feedback.
Conflict of interest
The authors declare that the research was conducted in the absence of any commercial or financial relationships that could be construed as a potential conflict of interest.
Publisher’s note
All claims expressed in this article are solely those of the authors and do not necessarily represent those of their affiliated organizations, or those of the publisher, the editors and the reviewers. Any product that may be evaluated in this article, or claim that may be made by its manufacturer, is not guaranteed or endorsed by the publisher.
Supplementary material
The Supplementary Material for this article can be found online at: https://www.frontiersin.org/articles/10.3389/fmars.2023.1167354/full#supplementary-material
References
Adams R. P., MacKay D. J. C. (2007). Bayesian online changepoint detection. Technical report (University of Cambridge, Cambridge, UK).
Akimova A., Núñez-Riboni I., Kempf A., Taylor M. H. (2016). Spatially-resolved influence of temperature and salinity on stock and recruitment variability of commercially important fishes in the North Sea. PloS One 11, e0161917. doi: 10.1371/journal.pone.0161917
Anderson C. N. K., Hsieh C. H., Sandin S. A., Hewitt R., Hollowed A., Beddington J., et al. (2008). Why fishing magnifies fluctuations in fish abundance. Nature 452, 835–839. doi: 10.1038/nature06851
Anderson S. C., Mills Flemming J., Watson R., Lotze H. K. (2011). Rapid global expansion of invertebrate fisheries: trends, drivers, and ecosystem effects. PloS One 6, e14735. doi: 10.1371/journal.pone.0014735
Arevalo E., Cabral H. N., Villeneuve B., Possémé C., Lepage M. (2023). Fish larvae dynamics in temperate estuaries: A review on processes, patterns and factors that determine recruitment. Fish Fisheries 24, 466–487. doi: 10.1111/faf.12740
Armstrong M. J., Gerritsen H. D., Allen M., Mccurdy W. J., Peel J. (2004). Variability in maturity and growth in a heavily exploited stock: cod (Gadus morhua L.) in the Irish Sea. ICES J. Mar. Sci. 61, 98–112. doi: 10.1016/j.icesjms.2003.10.005
Bastardie F., Brown E. J., Andonegi E., Arthur R., Beukhof E., Depestele J., et al. (2021). A review characterizing 25 ecosystem challenges to be addressed by an ecosystem approach to fisheries management in Europe. Front. Mar. Sci. 7. doi: 10.3389/fmars.2020.629186
Bastrikin D. K., Gallego A., Millar C. P., Priede I. G., Jones E. G. (2014). Settlement length and temporal settlement patterns of juvenile cod (Gadus morhua), haddock (Melanogrammus aeglefinus), and whiting (Merlangius merlangus) in a northern North Sea coastal nursery area. ICES J. Mar. Sci. 71, 2101–2113. doi: 10.1093/icesjms/fsu029
Baudron A. R., Brunel T., Blanchet M.-A., Hidalgo M., Chust G., Brown E. J., et al. (2020). Changing fish distributions challenge the effective management of European fisheries. Ecography 43, 494–505. doi: 10.1111/ecog.04864
Beaugrand G., Brander K. M., Alistair Lindley J., Souissi S., Reid P. C. (2003). Plankton effect on cod recruitment in the North Sea. Nature 426, 661–664. doi: 10.1038/nature02164
Beaugrand G., Luczak C., Edwards M. (2009). Rapid biogeographical plankton shifts in the North Atlantic Ocean. Global Change Biol. 15, 1790–1803. doi: 10.1111/j.1365-2486.2009.01848.x
Beggs S. E., Cardinale M., Gowen R. J., Bartolino V. (2014). Linking cod (Gadus morhua) and climate: investigating variability in Irish Sea cod recruitment. Fisheries Oceanogr. 23, 54–64. doi: 10.1111/fog.12043
Bell R. J., Tableau A., Collie J. S. (2023). Changes in the productivity of US West Coast fish stocks. Fisheries Res. 264, 106712. doi: 10.1016/j.fishres.2023.106712
Bentley J. W., Lundy M. G., Howell D., Beggs S. E., Bundy A., De Castro F., et al. (2021). Refining fisheries advice with stock-specific ecosystem information. Front. Mar. Sci. 8. doi: 10.3389/fmars.2021.602072
Bentley J. W., Serpetti N., Fox C. J., Heymans J. J., Reid D. G. (2020). Retrospective analysis of the influence of environmental drivers on commercial stocks and fishing opportunities in the Irish Sea. Fisheries Oceanogr. 29, 415–435. doi: 10.1111/fog.12486
Bentley J. W., Serpetti N., Fox C., Reid D., Heymans J. J. (2019). Modelling the food web in the Irish Sea in the context of a depleted commercial fish community. Part 2: ICES Ecopath with Ecosim Key Run. Report no 297 (Oban, UK: Scottish Association for Marine Science).
Beverton R. J. H., Holt S. J. (1957). On the dynamics of exploited fish populations (Springer, Dordrecht, NL).
Brander K. (2000). Effects of environmental variability on growth and recruitment in cod (Gadus morhua) using a comparative approach. Oceanologica Acta 23, 485–496. doi: 10.1016/S0399-1784(00)00133-X
Britten G. L., Dowd M., Worm B. (2016). Changing recruitment capacity in global fish stocks. Proc. Natl. Acad. Sci. 113, 134–139. doi: 10.1073/pnas.1504709112
Brooks E. N., Deroba J. J. (2015). When “data” are not data: the pitfalls of post hoc analyses that use stock assessment model output. Can. J. Fisheries Aquat. Sci. 72, 634–641. doi: 10.1139/cjfas-2014-0231
Brosset P., Smith A. D., Plourde S., Castonguay M., Lehoux C., Van Beveren E. (2020). A fine-scale multi-step approach to understand fish recruitment variability. Sci. Rep. 10, 16064. doi: 10.1038/s41598-020-73025-z
Brown E. J., Vasconcelos R. P., Wennhage H., Bergström U., Støttrup J. G., Van De Wolfshaar K., et al. (2018). Conflicts in the coastal zone: human impacts on commercially important fish species utilizing coastal habitat. ICES J. Mar. Sci. 75, 1203–1213. doi: 10.1093/icesjms/fsx237
Burns N. M., Bailey D. M., Wright P. J. (2019). A method to improve fishing selectivity through age targeted fishing using life stage distribution modelling. PloS One 14, e0214459. doi: 10.1371/journal.pone.0214459
Collie J. S., Bell R. J., Collie S. B., Minto C. (2021). Harvest strategies for climate-resilient fisheries. ICES J. Mar. Sci. 78, 2774–2783. doi: 10.1093/icesjms/fsab152
Conn P. B., Williams E. H., Shertzer K. W. (2010). When can we reliably estimate the productivity of fish stocks? Can. J. Fisheries Aquat. Sci. 67, 511–523. doi: 10.1139/F09-194
Crone P. R., Maunder M. N., Lee H., Piner K. R. (2019). Good practices for including environmental data to inform spawner-recruit dynamics in integrated stock assessments: Small pelagic species case study. Fisheries Res. 217, 122–132. doi: 10.1016/j.fishres.2018.12.026
Cushing D. H. (1990). “Plankton Production and Year-class Strength in Fish Populations: an Update of the Match/Mismatch Hypothesis,” in Advances in Marine Biology. Eds. Blaxter J. H. S., Southward A. J. (Academic Press, Copenhagen, San Diego, California, USA), 249–293.
Dahlke F. T., Wohlrab S., Butzin M., Pörtner H.-O. (2020). Thermal bottlenecks in the life cycle define climate vulnerability of fish. Science 369, 65–70. doi: 10.1126/science.aaz3658
Dakos V., Glaser S. M., C.-h. and Sugihara G. (2017). Elevated nonlinearity as an indicator of shifts in the dynamics of populations under stress. J. R. Soc. Interface 14, 20160845. doi: 10.1098/rsif.2016.0845
de Figueiredo G. M., Nash R. D. M., Montagnes D. J. S. (2007). Do protozoa contribute significantly to the diet of larval fish in the Irish Sea? J. Mar. Biol. Assoc. United Kingdom 87, 843–850. doi: 10.1017/S002531540705713X
Dorner B., Holt K. R., Peterman R. M., Jordan C., Larsen D. P., Olsen A. R., et al. (2013). Evaluating alternative methods for monitoring and estimating responses of salmon productivity in the North Pacific to future climatic change and other processes: A simulation study. Fisheries Res. 147, 10–23. doi: 10.1016/j.fishres.2013.03.017
Ellis J. R., Milligan S. P., Readdy L., Taylor N., Brown M. J. (2012). Spawning and nursery grounds of selected fish species in UK waters (Cefas, Lowestoft (UK), 56.
Fincham J. I., Rijnsdorp A. D., Engelhard G. H. (2013). Shifts in the timing of spawning in sole linked to warming sea temperatures. J. Sea Res. 75, 69–76. doi: 10.1016/j.seares.2012.07.004
Floeter J., Schrum C., Temming A. (2005). Recruitment of North Sea whiting (Merlangius merlangus (L.)): the interplay of bottom-up and top-down processes (Hamburg: Universität Hamburg).
Fogarty M. J., O'brien L. (2016). “Recruitment in Marine Fish Populations,” in Fish Reproductive Biology. Wiley, West Sussex, UK, 9–49.
Fonds M. (1979). Laboratory observations on the influence of temperature and salinity on development of the eggs and growth of the larvae of Solea solea (Pisces). Mar. Ecol. Prog. Ser. 1, 91–99. doi: 10.3354/meps001091
Fox C. J., O’Brien C. M., Dickey-Collas M., Nash R. D. M. (2000). Patterns in the spawning of cod (Gadus morhua L.), sole (Solea solea L.) and plaice (Pleuronectes platessa L.) in the Irish Sea as determined by generalized additive modelling. Fisheries Oceanogr. 9, 33–49. doi: 10.1046/j.1365-2419.2000.00120.x
Gamelon M., Sandercock B. K., Sæther B.-E. (2019). Does harvesting amplify environmentally induced population fluctuations over time in marine and terrestrial species? J. Appl. Ecol. 56, 2186–2194. doi: 10.1111/1365-2664.13466
Geffen A. J., Fox C. J., Nash R. D. M. (2006). Temperature-dependent development rates of cod Gadus morhua eggs. J. Fish Biol. 69, 1060–1080. doi: 10.1111/j.1095-8649.2006.01181.x
Gerritsen H. D., Armstrong M. J., Allen M., Mccurdy W. J., Peel J. A. D. (2003). Variability in maturity and growth in a heavily exploited stock: whiting (Merlangius merlangus L.) in the Irish Sea. J. Sea Res. 49, 69–82. doi: 10.1016/S1385-1101(02)00197-1
Gibson R. N., Nash R. D. M., Geffen A. J., van der Veer H. W. (2015). Flatfishes Biology and Exploitation (West Sussex, UK: Wiley Blackwell).
Haltuch M. A., Brooks E. N., Brodziak J., Devine J. A., Johnson K. F., Klibansky N., et al. (2019). Unraveling the recruitment problem: A review of environmentally-informed forecasting and management strategy evaluation. Fisheries Res. 217, 198–216. doi: 10.1016/j.fishres.2018.12.016
Heath M. R. (1992). “Field Investigations of the Early Life Stages of Marine Fish,” in Advances in Marine Biology. Eds. Blaxter J. H. S., Southward A. J. (Academic Press, San Diego, California, USA), 1–174.
Heath M. R., Lough R. G. (2007). A synthesis of large-scale patterns in the planktonic prey of larval and juvenile cod (Gadus morhua). Fisheries Oceanogr. 16, 169–185. doi: 10.1111/j.1365-2419.2006.00423.x
Henderson P. A. (2019). A long-term study of whiting, Merlangius merlangus (L) recruitment and population regulation in the Severn Estuary, UK. J. Sea Res. 155, 101825. doi: 10.1016/j.seares.2019.101825
Henderson P. A., Seaby R. M. (2005). The role of climate in determining the temporal variation in abundance, recruitment and growth of sole Solea solea in the Bristol Channel. J. Mar. Biol. Assoc. United Kingdom 85, 197–204. doi: 10.1017/S0025315405011069h
Hermant M., Lobry J., Bonhommeau S., Poulard J.-C., Le Pape O. (2010). Impact of warming on abundance and occurrence of flatfish populations in the Bay of Biscay (France). J. Sea Res. 64, 45–53. doi: 10.1016/j.seares.2009.07.001
Hilborn R., Amoroso R. O., Anderson C. M., Baum J. K., Branch T. A., Costello C., et al. (2020). Effective fisheries management instrumental in improving fish stock status. Proc. Natl. Acad. Sci. 117, 2218–2224. doi: 10.1073/pnas.1909726116
Hilborn R., Hively D. J., Jensen O. P., Branch T. A. (2014). The dynamics of fish populations at low abundance and prospects for rebuilding and recovery. J. Mar. Sci. 71, 2141–2151. doi: 10.1093/icesjms/fsu035
Hilborn R., Walters C. J. (1992). Quantitative fisheries stock assessment, choice, dynamics and uncertainty (London: Chapman and Hall). doi: 10.1007/978-1-4615-3598-0
Hixon M. A., Johnson D. W., Sogard S. M. (2013). BOFFFFs: on the importance of conserving old-growth age structure in fishery populations. ICES J. Mar. Sci. 71, 2171–2185. doi: 10.1093/icesjms/fst200
Hjort J. (1914). “Fluctuations in the Great fisheries of Northern Europe viewed in the light of biological research,” in Rapport et procès-verbaux (Copenhagen (DK: Conseil Permanent International Pour l'Exploration de la mer (ICES).
Holmes E. E., Scheuerell M. D., Ward E. J. (2021). Analysis of multivariate time-series using the MARSS package. Version 3.11.4 (2725 Montlake Blvd E., Seattle, WA 98112: NOAA Fisheries, Northwest Fisheries Science Center). doi: 10.5281/zenodo.5781847
Holmes E. E., Ward E. J., Wills K. (2012). MARSS: multivariate autoregressive state-space models for analyzing time-series data. R J. 4, 11–19. doi: 10.32614/RJ-2012-002
Holsman K. K., Haynie A. C., Hollowed A. B., Reum J. C. P., Aydin K., Hermann A. J., et al. (2020). Ecosystem-based fisheries management forestalls climate-driven collapse. Nat. Commun. 11, 4579. doi: 10.1038/s41467-020-18300-3
Houde E. D. (2016). “Recruitment Variability,” in Fish Reproductive Biology. Eds. Jakobsen T., Fogarty M. J., Megrey B. A., Moksness E. (Wiley-Blackwell, West Sussex, UK), 98–187.
Houde E. D., Able K. W., Strydom N. A., Wolanski E., Arula T. (2022). “Reproduction, Ontogeny and Recruitment,” in Fish and Fisheries in Estuaries. Eds. Whitfield A. K., Able K. W., Blaber S. J. M., Elliott M. (Wiley-Blackwell, West Sussex, UK), 60–187.
Howell D., Schueller A. M., Bentley J. W., Buchheister A., Chagaris D., Cieri M., et al. (2021). Combining ecosystem and single-species modeling to provide ecosystem-based fisheries management advice within current management systems. Front. Mar. Sci. 7. doi: 10.3389/fmars.2020.607831
Hsieh C. H., Reiss C. S., Hunter J. R., Beddington J. R., May R. M., Sugihara G. (2006). Fishing elevates variability in the abundance of exploited species. Nature 443, 859–862. doi: 10.1038/nature05232
Hutchings J. A. (2014). Renaissance of a caveat: Allee effects in marine fish. ICES J. Mar. Sci. 71, 2152–2157. doi: 10.1093/icesjms/fst179
ICES (2019). Whiting (Merlangius merlangus) in Division 7.a (Irish Sea) (ICES Advice: Recurrent Advice, Copenhagen, DK).
ICES (2021). ICES Data Portal, Stock Assessment Database, Extracted November 10, 2021 (ICES, Copenhagen, Denmark). Available at: http://standardgraphs.ices.dk.
ICES (2022a). Cod (Gadus morhua) in Division 7.a (Irish Sea) (ICES Advice: Recurrent Advice, Copenhagen, DK).
ICES (2022b). Sole (Solea solea) in Division 7.a (Irish Sea) (ICES Advice: Recurrent Advice, Copenhagen, DK).
ICES (2022c). ICES Data Portal, Dataset on Ocean HydroChemistry, Extracted February 3, 2022 (Copenhagen: ICES). Available at: http://ocean.ices.dk.
Iles T. C. (1994). A review of stock-recruitment relationships with reference to flatfish populations. Netherlands Journal of Sea Research 32, 399–420.
Johns D. (2022). Marine Biological Association of the UK (MBA) (2022): Irish Sea CPR updated dataset Mar 2022. The Archive for Marine Species and Habitats Data (DASSH) Marine Biological Association of the UK (MBA), Plymouth, UK. doi: 10.17031/1819
Kassambara A., Mundt F. (2020). Factoextra: Extract and Visualize the Results of Multivariate Data Analyses (R package version 1.0.7). Available at: https://CRAN.R-project.org/package=factoextra.
Kell L. T., Pilling G. M., O'brien C. M. (2005). Implications of climate change for the management of North Sea cod (Gadus morhua). ICES J. Mar. Sci. 62, 1483–1491. doi: 10.1016/j.icesjms.2005.05.006
Kelly C. J., Codling E. A., Rogan E. (2006). The Irish Sea cod recovery plan: some lessons learned. ICES J. Mar. Sci. 63, 600–610. doi: 10.1016/j.icesjms.2005.12.001
King J. R., Mcfarlane G. A., Punt A. E. (2015). Shifts in fisheries management: adapting to regime shifts. Philos. Trans. R. Soc. B: Biol. Sci. 370:20130277. doi: 10.1098/rstb.2013.0277
Kjesbu O. S. (1994). Time of start of spawning in Atlantic cod (Gadus morhua) females in relation to vitellogenic oocyte diameter, temperature, fish length and condition. J. Fish Biol. 45, 719–735. doi: 10.1111/j.1095-8649.1994.tb00939.x
Kjesbu O. S., Tiedemann I., Alix M., Thorsen A., Sundby S. (2023). Half a century of high-latitude fisheries oceanography research on the “recruitment problem” in Northeast Arctic cod (Gadus morhua). ICES J. Mar. Sci. 80, 1179–1201. doi: 10.1093/icesjms/fsad073
Koenigstein S., Mark F. C., Gößling-Reisemann S., Reuter H., Poertner H.-O. (2016). Modelling climate change impacts on marine fish populations: process-based integration of ocean warming, acidification and other environmental drivers. Fish Fisheries 17, 972–1004. doi: 10.1111/faf.12155
Kühn B., Kempf A., Brunel T., Cole H., Mathis M., Sys K., et al. (2023). Adding to the mix – Challenges of mixed-fisheries management in the North Sea under climate change and technical interactions. Fisheries Manage. Ecol. 30, 360–377. doi: 10.1111/fme.12629
Kühn B., Taylor M. H., Kempf A. (2021). Using machine learning to link spatiotemporal information to biological processes in the ocean: a case study for North Sea cod recruitment. Mar. Ecol. Prog. Ser. 664, 1–22. doi: 10.3354/meps13689
Lacroix G., Barbut L., Volckaert F. (2018). Complex effect of projected sea temperature and wind change on flatfish dispersal. Global Change Biol. 24, 85–100. doi: 10.1111/gcb.13915
Lagardère F., Amara R., Joassard L. (1999). “Vertical distribution and feeding activity of metamorphosing sole, Solea solea, before immigration to the Bay of Vilaine nursery (northern Bay of Biscay, France),” in When do fishes become juveniles? Eds. Copp G. H., Kováč V., Hensel K. (Dordrecht: Springer, Netherlands), 213–228.
Last J. M. (1978). The food of four species of pleuronectiform larvae in the eastern English Channel and southern North Sea. Mar. Biol. 45, 359–368. doi: 10.1007/BF00391822
Lauerburg R., Temming A., Pinnegar J. K., Kotterba P., Sell A. F., Kempf A., et al. (2018). Forage fish control population dynamics of North Sea whiting Merlangius merlangus. Mar. Ecol. Prog. Ser. 594, 213–230. doi: 10.3354/meps12533
Lê S., Josse J., Husson F. (2008). FactoMineR: A package for multivariate analysis. J. Stat. Software 25 (1), 1–18. doi: 10.18637/jss.v025.i01
Leggett W. C., Frank K. T. (1997). A comparative analysis of recruitment variability in North Atlantic flatfishes — testing the species range hypothesis. J. Sea Res. 37, 281–299. doi: 10.1016/S1385-1101(97)00023-3
Link J. S. (2002). Ecological considerations in fisheries management: when does it matter? Fisheries 27, 10–17. doi: 10.1577/1548-8446(2002)027<0010:ECIFM>2.0.CO;2
Liu J., West M. (2001). “Combined parameter and state estimation in simulation-based filtering,” in Sequential Monte Carlo methods in practice. Springer New York,: NY, USA, 197–223.
Lynam C. P., Lilley M. K. S., Bastian T., Doyle T. K., Beggs S. E., Hays G. C. (2011). Have jellyfish in the Irish Sea benefited from climate change and overfishing? Global Change Biol. 17, 767–782. doi: 10.1111/j.1365-2486.2010.02352.x
Ma S., Fu C., Li J., Sun P., Liu Y., Ye Z., et al. (2023). Non-stationary effects of multiple drivers on the dynamics of Japanese sardine (Sardinops melanostictus, Clupeidae). Fish Fisheries 24, 40–55. doi: 10.1111/faf.12708
Maunder M. N., Thorson J. T. (2019). Modeling temporal variation in recruitment in fisheries stock assessment: A review of theory and practice. Fisheries Res. 217, 71–86. doi: 10.1016/j.fishres.2018.12.014
Mazur M. D., Tanaka K. R., Shank B., Chang J.-H., Hodgdon C. T., Reardon K. M., et al. (2022). Incorporating spatial heterogeneity and environmental impacts into stock-recruitment relationships for Gulf of Maine lobster. ICES J. Mar. Sci. 79, 362–372. doi: 10.1093/icesjms/fsab266
McBride R. S., Somarakis S., Fitzhugh G. R., Albert A., Yaragina N. A., Wuenschel M. J., et al. (2015). Energy acquisition and allocation to egg production in relation to fish reproductive strategies. Fish Fisheries 16, 23–57. doi: 10.1111/faf.12043
Memarzadeh M., Britten G. L., Worm B., Boettiger C. (2019). Rebuilding global fisheries under uncertainty. Proc. Natl. Acad. Sci. 116, 15985–15990. doi: 10.1073/pnas.1902657116
Millar C., Large S., Magnusson A., Pinto C. (2022). icesSAG: Stock Assessment Graphs Database Web Services (R package version 1.4.0). Available at: https://CRAN.R-project.org/package=icesSAG.
Mitchell E. G., Wallace M. I., Smith V. A., Wiesenthal A. A., Brierley A. S. (2021). Bayesian Network Analysis reveals resilience of the jellyfish Aurelia aurita to an Irish Sea regime shift. Sci. Rep. 11, 3707. doi: 10.1038/s41598-021-82825-w
Möllmann C., Cormon X., Funk S., Otto S. A., Schmidt J. O., Schwermer H., et al. (2021). Tipping point realized in cod fishery. Sci. Rep. 11, 14259. doi: 10.1038/s41598-021-93843-z
Nash R. D. M., Geffen A. J. (2004). Seasonal and interannual variation in abundance of Calanus finmarchicus (Gunnerus) and Calanus helgolandicus (Claus) in inshore waters (west coast of the Isle of Man) in the central Irish Sea. J. Plankton Res. 26, 265–273. doi: 10.1093/plankt/fbh029
Needle C. L. (2001). Recruitment models: diagnosis and prognosis. Reviews in Fish Biology and Fisheries 21, 95–111
Nesslage G. M., Wilberg M. J. (2019). A performance evaluation of surplus production models with time-varying intrinsic growth in dynamic ecosystems. Can. J. Fisheries Aquat. Sci. 76, 2245–2255. doi: 10.1139/cjfas-2018-0292
Neuenhoff R. D., Swain D. P., Cox S. P., McAllister M. K., Trites A. W., Walters C. J., et al. (2018). Continued decline of a collapsed population of Atlantic cod (Gadus morhua) due to predation-driven Allee effects. Can. J. Fisheries Aquat. Sci. 76, 168–184. doi: 10.1139/cjfas-2017-0190
Neuheimer A. B., Mackenzie B. R., Payne M. R. (2018). Temperature-dependent adaptation allows fish to meet their food across their species' range. Sci. Adv. 4, eaar4349. doi: 10.1126/sciadv.aar4349
Neuheimer A. B., Thresher R. E., Lyle J. M., Semmens J. M. (2011). Tolerance limit for fish growth exceeded by warming waters. Nat. Climate Change 1, 110–113. doi: 10.1038/nclimate1084
Nishijima S., Kubota H., Kaga T., Okamoto S., Miyahara H., Okamura H. (2021). State-space modeling clarifies productivity regime shifts of Japanese flying squid. Population Ecol. 63, 27–40. doi: 10.1002/1438-390X.12062
Ohlberger J. (2013). Climate warming and ectotherm body size – from individual physiology to community ecology. Funct. Ecol. 27, 991–1001. doi: 10.1111/1365-2435.12098
Olsen E. M., Ottersen G., Llope M., Chan K.-S., Beaugrand G., Stenseth N. C. (2011). Spawning stock and recruitment in North Sea cod shaped by food and climate. Proc. R. Soc. B: Biol. Sci. 278, 504–510. doi: 10.1098/rspb.2010.1465
Peck M. A., Huebert K. B., Llopiz J. K. (2012). “Chapter 3 - Intrinsic and Extrinsic Factors Driving Match–Mismatch Dynamics During the Early Life History of Marine Fishes,” in Advances in Ecological Research. Eds. Woodward G., Jacob U., O'Gorman E. J. (Academic Press, San Diego, California, USA), 177–302.
Pepin P. (2022). Feeding by larval fish: how taxonomy, body length, mouth size, and behaviour contribute to differences among individuals and species from a coastal ecosystem. ICES J. Mar. Sci 80(1), 91–106. doi: 10.1093/icesjms/fsac215
Perälä T., Hutchings J. A., Kuparinen A. (2022). Allee effects and the Allee-effect zone in northwest Atlantic cod. Biol. Lett. 18, 20210439. doi: 10.1098/rsbl.2021.0439
Perälä T., Olsen E. M., Hutchings J. A. (2020). Disentangling conditional effects of multiple regime shifts on Atlantic cod productivity. PloS One 15, e0237414. doi: 10.1371/journal.pone.0237414
Perälä T. A., Swain D. P., Kuparinen A. (2017). Examining nonstationarity in the recruitment dynamics of fishes using Bayesian change point analysis. Can. J. Fisheries Aquat. Sci. 74, 751–765. doi: 10.1139/cjfas-2016-0177
Perry R. I., Cury P., Brander K., Jennings S., Möllmann C., Planque B. (2010). Sensitivity of marine systems to climate and fishing: Concepts, issues and management responses. J. Mar. Syst. 79, 427–435. doi: 10.1016/j.jmarsys.2008.12.017
Peterman R. M., Pyper B. J., Grout J. A. (2000). Comparison of parameter estimation methods for detecting climate-induced changes in productivity of Pacific salmon (Oncorhynchus spp.). Can. J. Fisheries Aquat. Sci. 57, 181–191. doi: 10.1139/f99-204
Philippart C. J. M., Henderson P. A., Johannessen T., Rijnsdorp A. D., Rogers S. I. (1998). Latitudinal variation in fish recruits in Northwest Europe. J. Sea Res. 39, 69–77. doi: 10.1016/S1385-1101(97)00016-6
Planque B., Fox C. J. (1998). Interannual variability in temperature and the recruitment of Irish Sea cod. Mar. Ecol. Prog. Ser. 172, 101–105. doi: 10.3354/meps172101
Pörtner H. O., Berdal B., Blust R., Brix O., Colosimo A., De Wachter B., et al. (2001). Climate induced temperature effects on growth performance, fecundity and recruitment in marine fish: developing a hypothesis for cause and effect relationships in Atlantic cod (Gadus morhua) and common eelpout (Zoarces viviparus). Continental Shelf Res. 21, 1975–1997. doi: 10.1016/S0278-4343(01)00038-3
Pörtner H. O., Peck M. A. (2010). Climate change effects on fishes and fisheries: towards a cause-and-effect understanding. J. Fish Biol. 77, 1745–1779. doi: 10.1111/j.1095-8649.2010.02783.x
Punt A. E. (2023). Those who fail to learn from history are condemned to repeat it: A perspective on current stock assessment good practices and the consequences of not following them. Fisheries Res. 261, 106642. doi: 10.1016/j.fishres.2023.106642
Punt A. E., A'mar T., Bond N. A., Butterworth D. S., De Moor C. L., De Oliveira J., et al. (2014). Fisheries management under climate and environmental uncertainty: control rules and performance simulation. ICES J. Mar. Sci. 71, 2208–2220. doi: 10.1093/icesjms/fst057
Rayner N. A., Parker D. E., Horton E. B., Folland C. K., Alexander L. V., Rowell D. P., et al. (2003). Global analyses of sea surface temperature, sea ice, and night marine air temperature since the late nineteenth century. J. Geophysical Res.: Atmospheres 108 (14), 4407. doi: 10.1029/2002JD002670
R Core Team. (2022). R: A language and environment for statistical computing. R Foundation for Statistical Computing: Vienna, Austria. https:///www.R-project.org/
Richardson A., Walne A., John A., Jonas T., Lindley J., Sims D., et al. (2006). Using continuous plankton recorder data. Prog. Oceanogr. 68, 27–74. doi: 10.1016/j.pocean.2005.09.011
Rijnsdorp A. D., Peck M. A., Engelhard G. H., Möllmann C., Pinnegar J. K. (2009). Resolving the effect of climate change on fish populations. ICES J. Mar. Sci. 66, 1570–1583. doi: 10.1093/icesjms/fsp056
Rijnsdorp A. D., Van Beek F. A., Flatman S., Millner R. M., Riley J. D., Giret M., et al. (1992). Recruitment of sole stocks, Solea solea (L.), in the Northeast Atlantic. Netherlands J. Sea Res. 29, 173–192. doi: 10.1016/0077-7579(92)90018-A
Rindorf A., van Deurs M., Howell D., Andonegi E., Berger A., Bogstad B., et al. (2022). Strength and consistency of density dependence in marine fish productivity. Fish Fisheries 23, 812–828. doi: 10.1111/faf.12650
Rouyer T., Sadykov A., Ohlberger J., Stenseth N. C. (2012). Does increasing mortality change the response of fish populations to environmental fluctuations? Ecol. Lett. 15, 658–665. doi: 10.1111/j.1461-0248.2012.01781.x
Rowlands W. L., Dickey-Collas M., Geffen A. J., Nash R. D. M. (2006). Gape morphology of cod Gadus morhua L., haddock Melanogrammus aeglefinus (L.) and whiting Merlangius merlangus (L.) through metamorphosis from larvae to juveniles in the western Irish Sea. J. Fish Biol. 69, 1379–1395. doi: 10.1111/j.1095-8649.2006.01200.x
Rowlands W. L., Dickey-Collas M., Geffen A. J., Nash R. D. M. (2008). Diet overlap and prey selection through metamorphosis in Irish Sea cod (Gadus morhua), haddock (Melanogrammus aeglefinus), and whiting (Merlangius merlangus). Can. J. Fisheries Aquat. Sci. 65, 1297–1306. doi: 10.1139/F08-041
Saila S. B., Recksiek C. W., Prager M. H. (1988). Basic fishery scienceprograms: a compendium of microcomputer programs, and manual of operation. Elsevier Science Publishers, Amsterdam . 230 p.
Savina-Rolland M., Rindorf A., Brown E. J., Neuenfeldt S., Van Deurs M., Carbonara P., et al. (2022). SEAwise Report on the key drivers of stock productivity and future environmental scenarios (Technical University of Denmark, Copenhagen, DK). doi: 10.11583/DTU.21269295
Scheffer M., Bascompte J., Brock W. A., Brovkin V., Carpenter S. R., Dakos V., et al. (2009). Early-warning signals for critical transitions. Nature 461, 53–59. doi: 10.1038/nature08227
Sguotti C., Otto S. A., Cormon X., Werner K. M., Deyle E., Sugihara G., et al. (2019b). Non-linearity in stock–recruitment relationships of Atlantic cod: insights from a multi-model approach. ICES J. Mar. Sci. 77, 1492–1502. doi: 10.1093/icesjms/fsz113
Sguotti C., Otto Saskia A., Frelat R., Langbehn Tom J., Ryberg Marie P., Lindegren M., et al. (2019a). Catastrophic dynamics limit Atlantic cod recovery. Proc. R. Soc. B: Biol. Sci. 286, 20182877. doi: 10.1098/rspb.2018.2877
Sharma R., Porch C. E., Babcock E. A., Maunder M. N., Punt A. E. (2019). Recruitment: Theory, estimation, and application in fishery stock assessment models. Fisheries Res. 217, 1–4. doi: 10.1016/j.fishres.2019.03.015
Silvar-Viladomiu P., Batts L., Minto C., Miller D., Lordan C. (2022b). An empirical review of ICES reference points. ICES J. Mar. Sci. 79, 2563–2578. doi: 10.1093/icesjms/fsac194
Silvar-Viladomiu P., Minto C., Brophy D., Reid D. G. (2022a). Peterman's productivity method for estimating dynamic reference points in changing ecosystems. ICES J. Mar. Sci 79(4), 1034–1047. doi: 10.1093/icesjms/fsac035
Silvar-Viladomiu P., Minto C., Halouani G., Batts L., Brophy D., Lordan C., et al. (2021). Moving reference point goalposts and implications for fisheries sustainability. Fish Fisheries 22, 1345–1358. doi: 10.1111/faf.12591
Stawitz C. C., Essington T. E. (2019). Somatic growth contributes to population variation in marine fishes. J. Anim. Ecol. 88, 315–329. doi: 10.1111/1365-2656.12921
Stige L. C., Hunsicker M. E., Bailey K. M., Yaragina N. A., Hunt G. L. Jr. (2013). Predicting fish recruitment from juvenile abundance and environmental indices. Mar. Ecol. Prog. Ser. 480, 245–261. doi: 10.3354/meps10246
Stock B. C., Miller T. J. (2021). The Woods Hole Assessment Model (WHAM): A general state-space assessment framework that incorporates time- and age-varying processes via random effects and links to environmental covariates. Fisheries Res. 240, 105967. doi: 10.1016/j.fishres.2021.105967
Su Z. (2023). Evaluation of management performance of a new state-space model for pink salmon (Oncorhynchus gorbuscha) stock–recruitment analysis. Can. J. Fisheries Aquat. Sci. 80, 1268–1288. doi: 10.1139/cjfas-2022-0262
Subbey S., Devine J. A., Schaarschmidt U., Nash R. D. M. (2014). Modelling and forecasting stock–recruitment: current and future perspectives. ICES J. Mar. Sci. 71, 2307–2322. doi: 10.1093/icesjms/fsu148
Szuwalski C. S., Britten G. L., Licandeo R., Amoroso R. O., Hilborn R., Walters C. (2019). Global forage fish recruitment dynamics: A comparison of methods, time-variation, and reverse causality. Fisheries Res. 214, 56–64. doi: 10.1016/j.fishres.2019.01.007
Szuwalski C. S., Vert-Pre K. A., Punt A. E., Branch T. A., Hilborn R. (2015). Examining common assumptions about recruitment: a meta-analysis of recruitment dynamics for worldwide marine fisheries. Fish Fisheries 16, 633–648. doi: 10.1111/faf.12083
Thomson J. R., Kimmerer W. J., Brown L. R., Newman K. B., Nally R. M., Bennett W. A., et al. (2010). Bayesian change point analysis of abundance trends for pelagic fishes in the upper San Francisco Estuary. Ecol. Appl. 20, 1431–1448. doi: 10.1890/09-0998.1
Thorpe R. B., Spence M. A., Dolder P. J., Nash R. D. M. (2021). Commentary: combining ecosystem and single-species modeling to provide ecosystem-based fisheries management advice within current management systems. Front. Mar. Sci. 8. doi: 10.3389/fmars.2021.707841
Thorson J. T., Cope J. M., Kleisner K. M., Samhouri J. F., Shelton A. O., Ward E. J. (2015). Giants' shoulders 15 years later: lessons, challenges and guidelines in fisheries meta-analysis. Fish Fisheries 16, 342–361. doi: 10.1111/faf.12061
Thurstan R. H., Roberts C. M. (2010). Ecological meltdown in the firth of clyde, scotland: two centuries of change in a coastal marine ecosystem. PloS One 5, e11767. doi: 10.1371/journal.pone.0011767
Tirronen M., Perälä T., Kuparinen A. (2022). Temporary Allee effects among non-stationary recruitment dynamics in depleted gadid and flatfish populations. Fish Fisheries 23, 392–406. doi: 10.1111/faf.12623
Trenkel V. M., Ojaveer H., Miller D. C. M., Dickey-Collas M. (2023). The rationale for heterogeneous inclusion of ecosystem trends and variability in ICES fishing opportunities advice. Mar. Ecol. Prog. Ser. 704, 81–97. doi: 10.3354/meps14227
Tu C.-Y., Chen K.-T., Hsieh C.-h. (2018). Fishing and temperature effects on the size structure of exploited fish stocks. Sci. Rep. 8, 7132. doi: 10.1038/s41598-018-25403-x
Uriarte A., Ibaibarriaga L., Sánchez-Maroño S., Abaunza P., Andrés M., Duhamel E., et al. (2023). Lessons learnt on the management of short-lived fish from the Bay of Biscay anchovy case study: Satisfying fishery needs and sustainability under recruitment uncertainty. Mar. Policy 150, 105512. doi: 10.1016/j.marpol.2023.105512
Van Deurs M., Brooks M. E., Lindegren M., Henriksen O., Rindorf A. (2021). Biomass limit reference points are sensitive to estimation method, time-series length and stock development. Fish and Fisheries 22, 18–30
van der Veer H. W., Berghahn R., Miller J. M., Rijnsdorp A. D. (2000). Recruitment in flatfish, with special emphasis on North Atlantic species: Progress made by the Flatfish Symposia. ICES J. Mar. Sci. 57, 202–215. doi: 10.1006/jmsc.1999.0523
Vaz ANishi. C., Scarcella G., Pardal M. A., Martinho F. (2019). Water temperature gradients drive early life-history patterns of the common sole (Solea solea L.) in the Northeast Atlantic and Mediterranean. Aquat. Ecol. 53, 281–294. doi: 10.1007/s10452-019-09688-2
Vert-Pre K. A., Amoroso R. O., Jensen O. P., Hilborn R. (2013). Frequency and intensity of productivity regime shifts in marine fish stocks. Proc. Natl. Acad. Sci. 110, 1779–1784. doi: 10.1073/pnas.1214879110
Winter A. M., Vasilyeva N., Vladimirov A. (2023). Spawner weight and ocean temperature drive Allee effect dynamics in Atlantic cod, Gadus morhua: inherent and emergent density regulation. EGUsphere 2023, 1–54. doi: 10.5194/bg-20-3683-2023
Zimmermann F., Claireaux M., Enberg K. (2019). Common trends in recruitment dynamics of north-east Atlantic fish stocks and their links to environment, ecology and management. Fish Fisheries 20, 518–536. doi: 10.1111/faf.12360
Zimmermann F., Ricard D., Heino M. (2018). Density regulation in Northeast Atlantic fish populations: Density dependence is stronger in recruitment than in somatic growth. J. Anim. Ecol. 87, 672–681. doi: 10.1111/1365-2656.12800
Keywords: Allee effect, environmental forcing, non-linear recruitment dynamics, regime shifts, population recovery
Citation: Tirronen M, Depestele J and Kuparinen A (2023) Can regime shifts in reproduction be explained by changing climate and food availability? Front. Mar. Sci. 10:1167354. doi: 10.3389/fmars.2023.1167354
Received: 16 February 2023; Accepted: 25 September 2023;
Published: 11 October 2023.
Edited by:
Priscilla Licandro, Stazione Zoologica Anton Dohrn, ItalyReviewed by:
Xiujuan Shan, Chinese Academy of Fishery Sciences (CAFS), ChinaGenevieve Nesslage, University of Maryland, College Park, United States
Copyright © 2023 Tirronen, Depestele and Kuparinen. This is an open-access article distributed under the terms of the Creative Commons Attribution License (CC BY). The use, distribution or reproduction in other forums is permitted, provided the original author(s) and the copyright owner(s) are credited and that the original publication in this journal is cited, in accordance with accepted academic practice. No use, distribution or reproduction is permitted which does not comply with these terms.
*Correspondence: Maria Tirronen, bWFyaWEuai5lLnRpcnJvbmVuQGp5dS5maQ==
†These authors have contributed equally to this work