- Fisheries Acoustic Research Laboratory, University of Washington, School of Aquatic and Fishery Sciences, Seattle, WA, United States
Tidal turbines are one source of marine renewable energy but development of tidal power is hampered by uncertainties in fish-turbine interaction impacts. Current knowledge gaps exist in efforts to quantify risks, as empirical data and modeling studies have characterized components of fish approach and interaction with turbines, but a comprehensive model that quantifies conditional occurrence probabilities of fish approaching and then interacting with a turbine in sequential steps is lacking. We combined empirical acoustic density measurements of Pacific herring (Clupea pallasii) and when data limited, published probabilities in an impact probability model that includes approach, entrainment, interactions, and avoidance of fish with axial or cross-flow tidal turbines. Interaction impacts include fish collisions with stationary turbine components, blade strikes by rotating blades, and/or a collision followed by a blade strike. Impact probabilities for collision followed by a blade strike were lowest with estimates ranging from 0.0000242 to 0.0678, and highest for blade strike ranging from 0.000261 to 0.40. Maximum probabilities occurred for a cross-flow turbine at night with no active or passive avoidance. Estimates were lowest when probabilities were conditional on sequential events, and when active and passive avoidance was included for an axial-flow turbine during the day. As expected, conditional probabilities were typically lower than analogous independent events and literature values. Estimating impact probabilities for Pacific herring in Admiralty Inlet, Washington, United States for two device types illustrates utilization of existing data and simultaneously identifies data gaps needed to fully calculate empirical-based probabilities for any site-species combination.
1 Introduction
Tidal turbines are a potential Marine Renewable Energy (MRE) source that can be deployed in high flow current regions (Pelc and Fujita, 2002). Tidal energy technology has been deployed but widespread adoption is hampered, in part, by concerns of aquatic animal impacts (Copping and Hemery, 2020). Primary concerns include collisions with stationary turbine components and/or strikes from rotating blades that could inhibit growth or affect survival of fish, seabirds, or marine mammals (Hemery et al., 2021). Knowledge gaps and inadequate empirical data on animal-device interactions necessitate obtaining, quantifying, and interpreting physical and biological data that can be used to discern the influence of animal-turbine encounters (e.g., Copping and Hemery, 2020), collisions (e.g., Müller et al., 2023), and blade strikes (e.g., Castro-Santos and Haro, 2015; Courtney et al., 2022). Unverified perceptions of mortality from animal-device interactions can impede development of monitoring regulations for tidal turbine sites. Comprehensive baseline and post-installation monitoring data on animal-tidal device interactions are not available, resulting in uncertainty among regulators who are cautious when permitting full-scale MRE sites (Copping et al., 2020a).
Encounter and collision rates between aquatic animals and tidal turbines are not well quantified due to limited opportunities and appropriate technologies to observe, measure, and characterize interactions (Fox et al., 2018; Courtney et al., 2022; Bender et al., 2023). Worldwide, there have been few (Copping et al., 2021) acoustic and optical technologies deployed to monitor tidal energy sites (e.g., Williamson et al., 2017; Staines et al., 2022). Even though stationary acoustic multibeam and multi-frequency echosounders are available, their deployment is often limited due to operational constraints including limited detection of weaker targets (Williamson et al., 2017). An approach that supplements empirical measures when animal behavior and hydrodynamic data are limited is the use of models to estimate the probability of animal-device interactions (Buenau et al., 2022). These studies include fish swimming trajectories during approach (Shen et al., 2016) or interaction (e.g., Viehman and Zydlewski, 2015; Bevelhimer et al., 2017) with tidal turbines. There remains a need for a comprehensive model that quantifies probabilities as fish approach and potentially interact with a hydrokinetic turbine.
To fully estimate potential encounter and interaction risks that influence MRE monitoring requirements and operational regulations, additional risk factors should be incorporated into a conditional, encounter-impact probability model. Current empirical observations and many encounter models lack active and passive avoidance behaviors of fish as they approach and interact with a device. Collision with stationary components of a device is another factor that is not commonly separated from blade strikes in published models. Collisions with stationary structures (Müller et al., 2023) could disorient fish (Courtney et al., 2022) and potentially lead to a blade strike.
This study develops a conditional probabilistic model that quantifies encounter and interactions of fish with tidal turbines. The encounter-impact model estimates probabilities of approach, encounter, collision with stationary components, blade strike by rotating blades, and sequential collision and blade strike using acoustic data from Admiralty Inlet, Washington, United States, and literature values when empirical data are lacking. Existing data gaps are identified along with appropriate next steps for model application. This encounter-impact model is designed to be generic and can be applied to any potential tidal energy project site.
2 Methods
2.1 Model description
The encounter-impact model computes occurrence probabilities for individual model components, and conditional probabilities of fish approaching and potentially interacting with a tidal turbine in sequential steps (Figure 1).
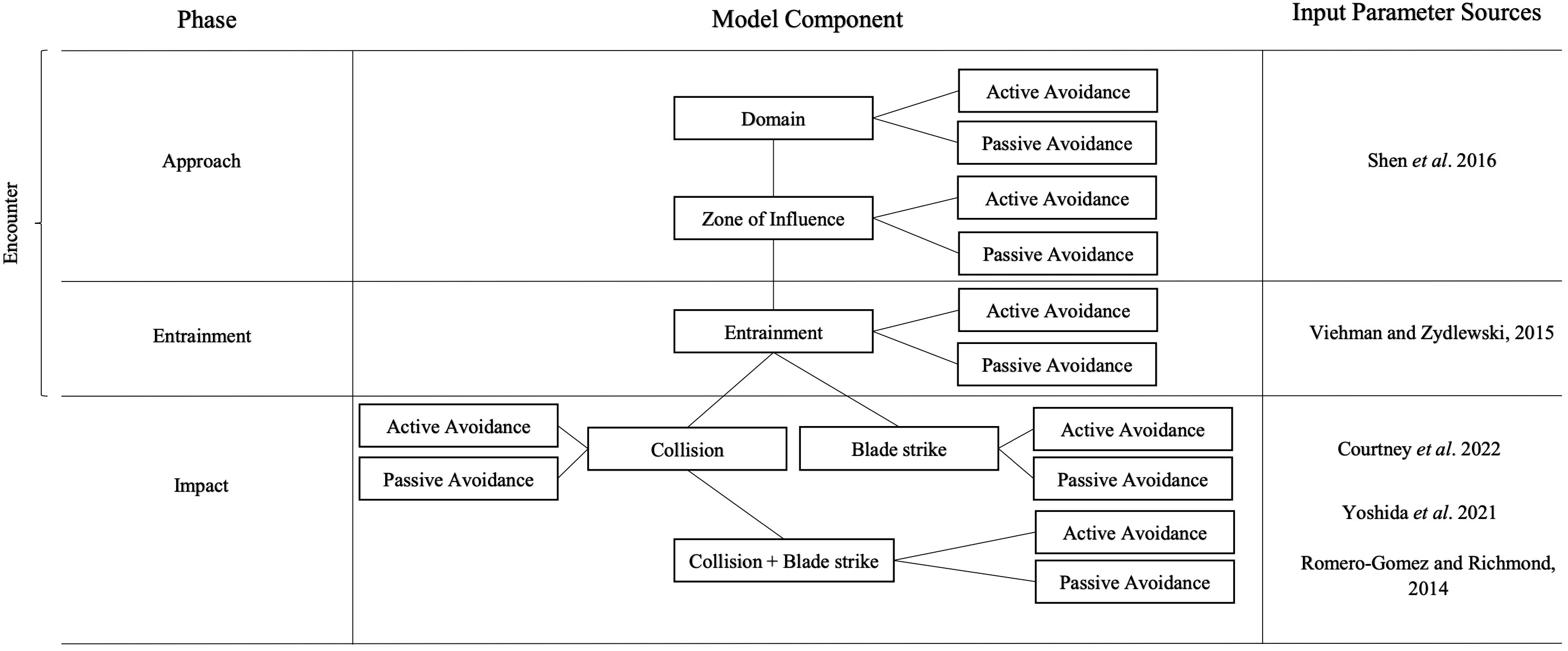
Figure 1 A schematic of the empirical encounter-impact probability model. The left column identifies the model phase, the center column details model components, and the right column identifies literature used to extract parameter values that are used in corresponding model components.
The approach phase quantifies when an animal enters the vicinity of an MRE device and includes the model domain, zone of influence, and estimates of active or passive avoidance. The model domain is comprised of the study area and estimates the probability of whether a fish is present within a site. If fish are present, then the domain model component is assigned a probability value of 1 (Table 1). As an empirical analog, Shen et al. (2016) used mobile hydroacoustics to track fish approaching a cross-flow tidal turbine and observed responses to a turbine by fish, measured using change in swimming direction, at distances over a hundred meters (Shen et al., 2016). We define the zone of influence as the reaction distance between an animal and the turbine. In this model, the zone of influence is set to Shen et al.'s (2016) 140 m upstream from an axial or cross-flow tidal turbine (Figures 2A, B). A vertical height of 25 m above the seafloor is used to represent approximately twice the vertical footprint of a proposed turbine in Admiralty Inlet (Jacques, 2014) and is within Shen et al.'s (2016) range of water depths (25 m at low tide to 32 m at high tide) at their study site. The probability of being within the zone of influence is dependent on the device’s shape and size, water depth, range of tidal current speeds, and fish swimming ability. The probability of being in the zone of influence is defined as the probability of a fish being within the domain multiplied by the complement of an individual avoiding the device (Table 1).
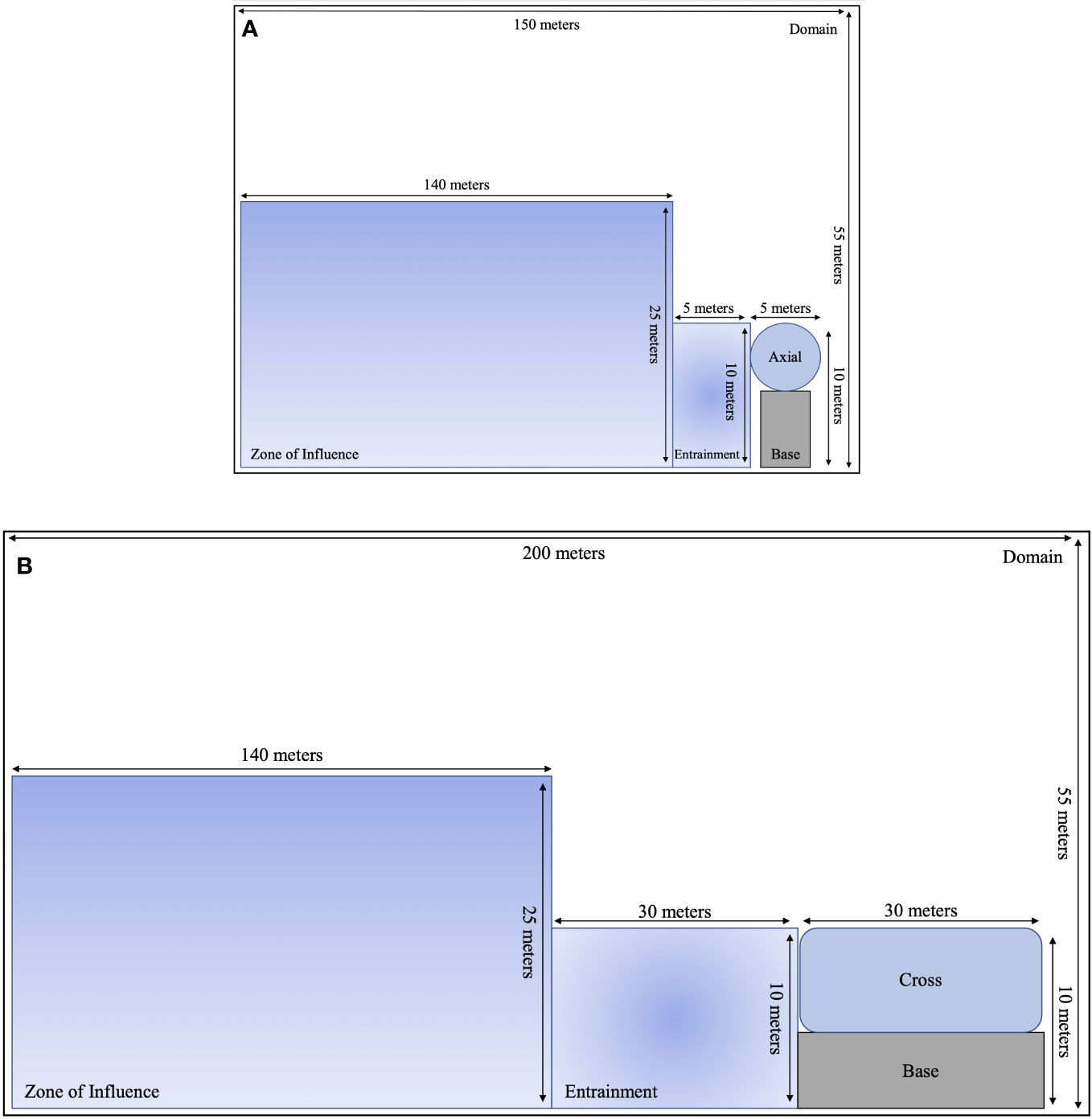
Figure 2 A two-dimensional schematic showing dimensions of the encounter-impact model components for (A) axial and (B) cross-flow turbines.
Entrainment occurs when a fish is within the area adjacent to the device, normal to the device face. If an animal continues its current trajectory with no avoidance, it will collide with the turbine base or enter the turbine. The turbine base and entry area are half the vertical height of the turbine (Figures 2A, B). Areal dimensions of the cross-flow turbine base and turbine entrance are both 30 m by 10 m. Areal dimensions of the axial-flow turbine base and turbine entrance are 5 m by 10 m. The probability of entrainment is defined as the probability of a fish being within the zone of influence multiplied by the probability of 1 minus avoiding the device given that the individual is within the zone of influence (Table 1). Avoidance calculations are detailed below.
Interactions between a fish and a tidal turbine are composed of collisions and/or blade strikes. We define collision as physical contact between an animal and the turbine base or a non-moving device component (e.g., Müller et al., 2023). We define blade strike as contact between an animal and a rotating blade (e.g., Castro-Santos and Haro, 2015; Courtney et al., 2022). In the model, collision and blade strike are treated as potential sequential events, where fish can collide with a turbine support structure and then be struck by a rotating blade. There is inconsistent use of these terms in the literature. A singular term, collision (e.g., Copping and Hemery, 2020), has been used to describe interactions between an animal and a device. However, the terms collision and strike have also been used interchangeably when characterizing interactions between animals and turbine blades (e.g., Hammar et al., 2013; Yoshida et al., 2020; Yoshida et al., 2021), and omitting animal contact with stationary turbine structures. This may not be a trivial oversight as turbine dimensions can exceed 15 to 20 m in length and width (cf. Viehman and Zydlewski, 2015; Shen et al., 2016; Courtney et al., 2022), which provides large surface areas for fish to collide with a turbine base or non-rotating structures when avoidance is not possible.
Impact is defined as one or more interactions between a fish and a device through collision and/or blade strike (e.g., Yoshida et al., 2021; Courtney et al., 2022). Since blade strike constitutes the greatest risk to fish and are a concern among researchers and regulators (Copping et al., 2020a), experimental and field data are used to calculate probability estimates, with published values emphasizing blade strikes. In cases where empirical data are lacking, the impact phase of the model incorporates laboratory and simulation model data (Romero-Gomez and Richmond, 2014) that align with our encounter-impact collision and blade strike model components.
Impact probabilities are calculated for each model subcomponent and overall potential impact (Table 1). All impact probabilities also depend on whether an animal is present within the entrainment area. The occurrence probability of collision with a turbine is calculated as the probability of entrainment multiplied by the probability of collision given that a fish is entrained. The probability of blade strike is defined as the probability of entrainment multiplied by the probability of a blade strike given that a fish has entered the device. Lastly, the probability of collision and blade strike is defined as the probability of entrainment, multiplied by the probability of collision, multiplied by the probability of blade strike given that a fish collided with the device. The overall probability of impact is calculated as the sum of the three potential interaction events: collision, blade strike, and collision and blade strike.
All phases of the encounter-impact model include active and passive avoidance (Figure 1). Avoidance is defined as a change in a fish’s trajectory in response to tidal devices. In behavioral studies, fish have been shown to actively avoid predation and navigate around obstacles, even at long distances (e.g., Utne, 1997; Muirhead and Sprules, 2003; Zhang et al., 2017; Berry et al., 2019; Bender et al., 2023; Müller et al., 2023). Tidal flow speeds often surpass fish swimming capabilities (cf. Okubo, 1987; He, 1993), potentially leading to passive transport through the water and passage around or through MRE devices. Therefore, the definition of avoidance is expanded to a fish’s response and movement away from a device and/or its avoidance due to hydrodynamic forces (Copping and Hemery, 2020). We define the threshold between active and passive avoidance using the ratio of swimming capability to tidal flow. Average Pacific herring (Clupea pallasii) fork length from Admiralty Inlet net samples is used to estimate swimming speed using Okubo's (1987) locomotion equation:
where Ss is swimming speed (ms-1), and L is fish length (m). Active locomotion is assumed when the ratio of swimming speed to tidal flow is greater than 1 body length per second (bls-1) (He, 1993). Passive locomotion occurs when the tidal speed exceeds 1 bls-1, in this study 0.155 ms-1.
2.2 Tidal turbine dimensions
For this study, representative axial and cross-flow tidal turbine devices are used in calculations of encounter and impact probabilities. Tidal turbine dimensions used are based on an axial-flow Verdant Power Kinetic Hydropower System (KHPS) (Bevelhimer et al., 2017) (Figure 2A) and a cross-flow Ocean Renewable Power Company (ORPC) TidGen Power System (Shen et al., 2016) (Figure 2B). Verdant Power KHPS turbine characteristics include a three-bladed, single-rotor turbine. The height of the device is approximately 10 m, with a rotor-swept area of 5 m in diameter, defining an area of 5 m by 10 m. The TidGen device is 31.2 m long and 9.5 m high with foils (i.e., rotating blades) 6.7 - 9.5 m above the seafloor, defining an area of 30 m by 10 m.
2.3 Empirical data description
Data used to estimate occurrence probabilities were collected in 2011 (Horne et al., 2013) at a site in Admiralty Inlet, Puget Sound, Washington chosen by the Snohomish Public Utility District for potential deployment of two Open Hydro (https://www.emec.org.uk/about-us/our-tidal-clients/open-hydro/) turbines. The proposed site is approximately 750 m off Admiralty Head at a depth of 55 m mean tide height. Data were collected using a Simrad EK-60 echosounder operating at 120 kHz, an autonomous bottom-deployed 1MHz Nortek AWAC acoustic doppler current profiler (ADCP), and a midwater trawl deployed from a mobile surface vessel. Acoustic and fish surveys were conducted from May 2 to May 13 and June 3 to June 14, 2011, during day and night for a combined total transect length of 28 km (Jacques, 2014). 324 parallel transects (0.7 to 1.5 km long) extending northwest and southeast of the proposed turbine location, were spaced 0.5 km apart (see Horne et al., 2013 for survey details). The ADCP, deployed from May 9 until June 10, 2011, collected concurrent tide state and tidal velocity measurements for 12 minutes every two hours (Jacques, 2014).
A Marinovich midwater trawl, a 6 m x 6 m box trawl fished with 4.6 m x 6.5 m steel V-doors, was used to capture samples to quantify species composition and length-frequencies of the fish community. Among captured species, Pacific herring represented 32% of the total catch by number. In this study, all acoustic backscatter is attributed to Pacific herring in acoustic density calculations. The average length of Pacific herring caught in the midwater trawl was 0.155 m and is used in all acoustic and swimming speed calculations. Given analogous fish lengths and time of year, the target strength conversion equation for Pacific herring from Thomas et al. (2002): 26.2·log10(Lcm) - 72.5 is used to transform acoustic-derived densities (m2 m-3) to fish densities (fish m-2).
2.4 Factors contributing to model component probabilities
Since no turbine was deployed during data collection, the Admiralty Inlet dataset provides the flexibility to analyze acoustic fish densities and distributions using multiple turbine types and light regimes represented by time of day. To observe how acoustic densities varied with light fluctuations, probabilities of occurrence for each model component during day and night are calculated for each turbine type. Fish densities are estimated by dividing each surveyed transect in horizontal 140 m, 30 m, or 5 m bins (corresponding to turbine type, Figures 2A, B) and then grouping bins to match the size of each model component. This approach also ensures that each bin along every transect can be used as a location for sequential model components or a device.
Probability estimates in the encounter-impact model are also influenced by active and passive avoidance. The zone of influence in the model uses three avoidance scenarios in probability estimates. The first scenario assumes fish are unable to avoid the turbine within the zone of influence. In the second scenario, fish can avoid the turbine within the zone of influence using active and passive avoidance. Active avoidance rates are estimated from the Admiralty Inlet dataset by discounting abundance estimates of fish within model components by Shen et al.'s (2016) avoidance rate of 0.372. Passive avoidance rates are estimated by tabulating fish observations swimming around or above model components, assuming that avoidance will occur to the side or above a device. The proportion of time passive avoidance occurs is determined by the tidal cycle – when tidal flow speeds surpass fish swimming speeds. The third scenario uses Shen et al.'s (2016) active avoidance rate of 0.372 without incorporating passive avoidance. When an avoidance rate from Admiralty Inlet or Shen et al. (2016) is incorporated into the model, estimates of fish impact are calculated using conditional probabilities from sequential model components. This approach evaluates a fish’s ability to avoid a device across model components and provides insight into the likelihood of impact for each model phase and overall encounters with tidal turbines. Conversely, when an avoidance rate is not included, calculated impact probabilities are not dependent on sequential model components and analogous to rates in published studies.
2.5 Estimating empirical probabilities
Occurrence of fish during day and night is determined by enumerating acoustic abundance estimates detected within bins along each mobile survey transect, aligned with areas of each model component (Figures 2A, B). Abundances along binned cells are summed to estimate total abundance for each transect. Probabilities of occurrence for each model component are determined by dividing the number of fish detected within each cell of each model component by total fish abundance.
Since no fish-turbine interaction measurements are available from Admiralty Inlet, encounter and impact published values are used in model calculations. At this time, there are no published probability estimates of collisions between fish and stationary tidal structures or collisions followed by blade strikes. Collision probabilities are estimated by calculating the complement of published blade strike probabilities and discounting by length-dependent swimming speed and time of day avoidance rates published in Viehman and Zydlewski (2015).
Blade strike probabilities are taken from field measurements (Courtney et al., 2022), laboratory experiments (Yoshida et al., 2021), and calculated using a blade-strike model (Romero-Gomez and Richmond, 2014):
where P(strike) is the probability of a blade strike, n is the number of blades, N is a fixed rotation rate [i.e., 0.357 s-1 for a cross-flow turbine (Viehman and Zydlewski, 2015) and 0.667 s-1 for an axial-flow turbine (Bevelhimer et al., 2017)], L is fish length (m), α represents the fish approach angle perpendicular to the blade plane (), and U is tidal velocity (ms-1). Blade strike probabilities are estimated using Equation (2) for tidal velocities observed in Admiralty Inlet that ranged from 1.0 ms-1 to 3.0 ms-1 (Horne et al., 2013) in increments of 0.2 ms-1. Incremental changes in tidal velocities depict the progression of a tidal cycle, yielding a range of strike probabilities in response to periodic flow conditions. The encounter-impact model also uses blade strike rates from Courtney et al. (2022) (0.13) and Yoshida et al. (2021) (0.02 – 0.05) in blade strike calculations. Inclusion of these rates in the blade strike model component compensates for limited data availability and introduces a range of probability estimates that incorporate turbine design, time of day, and device avoidance.
The sequential occurrence probability of collision and blade strike is determined by multiplying collision and published blade strike probability estimates. Probabilities of collision, blade strike, and collision and blade strike are discounted by avoidance rates in model calculations. Overall impact probabilities are calculated by summing estimated probabilities of each impact subcomponent (Table 1).
3 Results
Probabilities of occurrence for each component of the encounter-impact model are influenced by turbine type, time of day, and avoidance. Probabilities of occurrence for the zone of influence range between 0.0636 to 0.0649 for both turbine types (Tables S1A–D, Supplementary Material). Entrainment probabilities range between 0.00245 to 0.0408 for an axial-flow turbine (Tables S1A, B, Supplementary Material) and 0.0118 to 0.0408 for a cross-flow turbine (Tables S1C, D, Supplementary Material). Probabilities of impact depend on occurrences of collision, blade strike, or sequential collision and blade strike. Collision probabilities between fish and tidal devices span three orders of magnitude from 0.000364 to 0.324 for both turbine types, with similar probabilities of blade strike ranging between 0.000261 to 0.40 for both turbine types (Tables S1A–D, Supplementary Material). As expected, probabilities of collision and blade strike are lower than either single event impact, ranging between 0.0000242 to 0.0678 for both turbine types (Tables S1A–D, Supplementary Material). Overall impact probabilities, a summation of subcomponents, for the two turbine types are nearly identical ranging between 0.00110 to 0.666 for an axial-flow turbine and 0.00110 to 0.689 for a cross-flow turbine (Table 2).
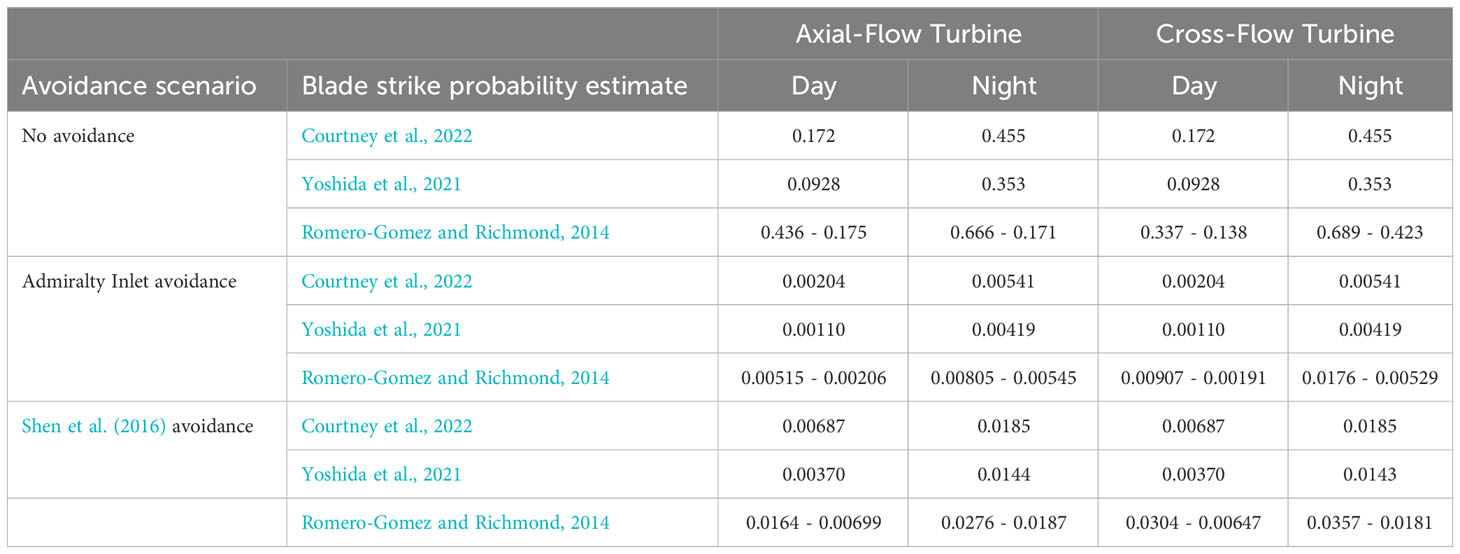
Table 2 Impact probability estimates for axial and cross-flow turbines for avoidance scenarios using alternate blade strike probability estimates.
When comparing occurrence probabilities for each model component, estimates are higher at night than during the day for both turbine types averaging between 0.00194 to 0.100 (Tables S1A–D, Supplementary Material). Blade strike occurrence probabilities are the only model component that have higher average probabilities during the day than at night, with an average 0.00238 difference for the axial-flow turbine (Tables S1A, B, Supplementary Material), and an average 0.00237 difference for the cross-flow turbine (Tables S1C, D, Supplementary Material). When comparing overall impact probabilities in light regimes, probabilities are higher at night than during the day for both turbine types, with probability variations ranging over three orders of magnitude (Table 2). Turbine design influences impact probabilities, with an axial-flow turbine exhibiting the lowest risk of impact across factors and avoidance scenarios (Table 2).
As expected, probabilities of occurrence for each model component are higher when no avoidance is included and model components are not conditioned on preceding events in model calculations (Tables S1A–D, Supplementary Material). Probabilities of occurrence are lowest when Admiralty Inlet avoidance rates are applied, reflecting the inclusion of conditional probabilities in model calculations. Probabilities of occurrence for the zone of influence are similar across all avoidance scenarios for both turbine types ranging between 0.0636 and 0.0649 (Tables S1A–D, Supplementary Material). Probabilities of occurrence for entrainment are higher when Shen et al.'s 2016 avoidance rate (0.0408) is applied to the model for both turbine types (Tables S1B, D, Supplementary Material). Probabilities of impact are highest by two to three orders of magnitude when no avoidance is included for a cross-flow turbine (Table 2). Collision probabilities (0.324), blade strike probabilities (0.40), and sequential collision and blade strike probabilities (0.0678) are all highest for both turbine types when subcomponents are modeled with no avoidance (Tables S1A–D, Supplementary Material). Minimum and maximum probability values are similar between subcomponents and overall impact estimates, with larger values occurring when no avoidance is applied and lowest when avoidance rates from Admiralty Inlet were used in model calculations (Table 2).
Conditional probability estimates from this study are both lower and higher than other published values (Table 3). Shen et al. (2016) and Bangley et al. (2022) observed order of magnitude higher probabilities of fish approach and encounter with a tidal turbine than average approach estimates in this study. Similarly, Viehman and Zydlewski (2015) report order of magnitude higher average probabilities of entrainment at night with a 0.290 probability estimate difference between day and night calculations. Band et al. (2016) observed order of magnitude higher probabilities of collision for Harbor seals with turbine rotors when compared to results of this study. In contrast, Wilson et al.'s (2006) non-conditional encounter probabilities for Pacific herring were two orders of magnitude lower than those estimated in this study.
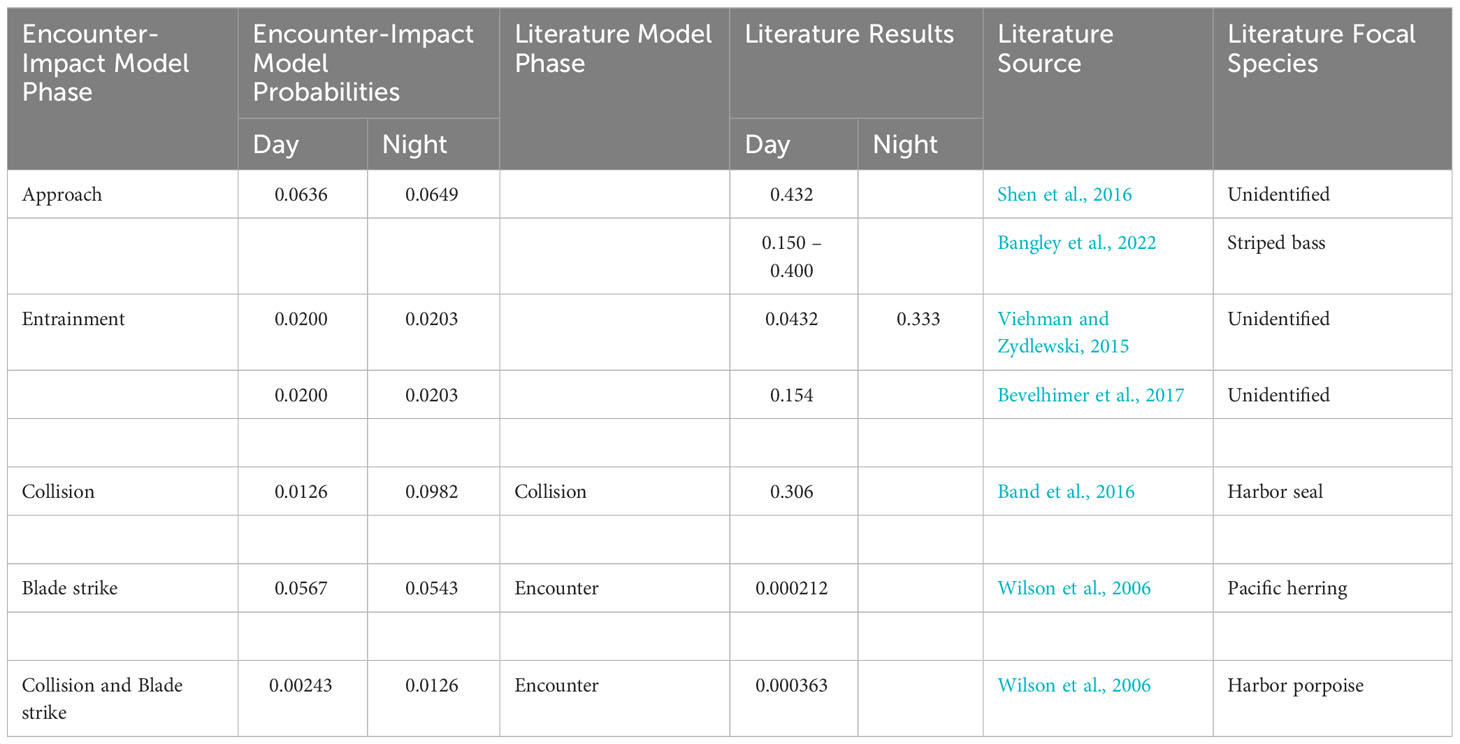
Table 3 Comparison of average occurrence probabilities for each phase of the encounter-impact model to published literature values.
Regardless of the combination of factors, probabilities of fish-turbine encounters and impact range from a minimum of 0.0000242 to a maximum of 0.324. Overall impacts ranged from a minimum of 0.00110 to a maximum of 0.689. Probability values are particularly low when conditioned on fish occurring within a turbine’s zone of influence, where subsequent entrainment may lead to an impact. All highest probability values occur at night with no avoidance in calculations for a cross-flow turbine.
4 Discussion
Occurrence probability estimates of fish-turbine interactions are influenced by model component, device surface area, and turbine design. The concept of a zone of influence, demonstrated by Shen et al. (2016) and Grippo et al. (2020), represents a large range in which fish can respond to turbine presence. Larger turbine designs, exemplified by the 30 m by 10 m silhouette of the ORPC TidGen cross-flow turbine, also present a large surface area for potential collisions and interactions with a device. The cross-flow turbine is approximately six times longer than the Verdant Power KHPS axial-flow turbine. Greater cross-flow impact probability estimates and congruent empirical blade strike estimates from Courtney et al. (2022) demonstrate high probabilities of entrainment and collision associated with cross-flow turbines, attributable to the large size of the device. Design characteristics of turbines also affect the entrainment model component, as entrainment dimensions scale with turbine size.
Light and dark cycles have limited influence on empirical data-based variations in occurrence and impact probabilities. A slight increase in probability values is observed for model estimates based on night empirical data compared to those sampled during the day. Fish behavior in light and dark conditions provides insight on fish-turbine detection distances where field (Viehman and Zydlewski, 2015; Viehman et al., 2015; Williamson et al., 2019) and experimental (Yoshida et al., 2021) studies found that light intensity affects fish distribution in the presence of MRE devices. Williamson et al. (2019) observed a 2.63 times greater increase in fish aggregation rates around turbine structures at night compared to day, supporting previous studies that show greater probabilities of turbine entry for fish at night (Viehman and Zydlewski, 2015). Viehman et al. (2015) reported that fish are more evenly distributed at night, even at dynamic tidal turbine sites, demonstrating the persistence of fish in dark conditions where turbines are present.
Variations in model component and impact probability estimates are contingent on turbine type, light conditions, avoidance scenarios, and blade strike probabilities. Occurrence probabilities for the zone of influence are unaffected by avoidance scenarios, with slight elevation in nighttime estimates, as probabilities are directly extracted from the Admiralty Inlet empirical data that had no turbine present at the site. Probabilities of entrainment modeled with no avoidance are similar or an order of magnitude lower for the smaller axial-flow turbine compared to the cross-flow turbine. Analogous encounter rate studies (Bangley et al., 2022; Sanderson et al., 2023) using acoustic telemetry tags found encounter rate probabilities an order of magnitude higher compared to the conditional encounter probability estimates. Impact probabilities in model scenarios with no avoidance (e.g., Wilson et al., 2006) result in higher values by one to two orders of magnitude compared to impact subcomponents that include avoidance. It is worth noting that fish-turbine encounter probabilities reported by Wilson et al. (2006) are based on Pacific herring but are not conditional and do not incorporate active or passive avoidance. Comparing blade strike probabilities derived from literature values, Yoshida et al.'s (2021) probabilities result in the lowest overall impact probability estimates when combined with an avoidance scenario. These lower probability values are attributed to a lower turbine blade rotational speed to fish swimming speed ratio, resulting in greater avoidance and lower blade strike rates. In contrast, impact probabilities are highest when using blade strike probabilities from the Romero-Gomez and Richmond (2014) blade strike model that does not include fish avoidance, despite model probabilities decreasing as flow speeds increase. In combination, our probability estimates demonstrate that avoidance is an important factor influencing impact probability estimates, both as a scenario within the conditional model and experimentally with fish and a turbine present.
Additional empirical data are needed to quantify collision rates with stationary structures and blade strikes. Data that track individual fish through turbine encounters supports probability estimates of avoidance, collision with turbine structures, and the combination of collision followed by blade strike. Long-range fish trajectory data can be used to quantify active and passive turbine avoidance behaviors through each step of a sequential encounter model and be used in conditional probability of occurrence calculations. The lack of collision and sequential collision and blade strike data or suitable published values necessitated modification of blade strike rates for model subcomponent calculations. Parameter values for these impact subcomponents are derived by multiplying blade strike probabilities from the literature using Shen et al.'s (2016) avoidance rate of 0.372. The use of published blade strike probabilities in calculation of collision probability estimates may have increased collision probabilities. To illustrate by example, Courtney et al. (2022) observed greater blade strike occurrences compared to other studies that found no blade strikes in natural environments (e.g., Hammar et al., 2013; Viehman and Zydlewski, 2015; Shen et al., 2016; Bevelhimer et al., 2017 Romero-Gomez and Richmond's, 2014) blade-strike model, parameterized for Pacific herring in Admiralty Inlet, does not include avoidance, which increased blade strike estimates compared to field (Hammar et al., 2013; Courtney et al., 2022; Bender et al., 2023) and experimental (Zhang et al., 2017; Berry et al., 2019; Yoshida et al., 2020; Yoshida et al., 2021; Müller et al., 2023) studies. Probabilities calculated using blade strike rates from Courtney et al. (2022) and Romero-Gomez and Richmond (2014) resulted in overall impact probability values that range one to two orders of magnitude higher than estimates derived from Yoshida et al.'s (2021) blade strike estimates. The lack of data or published probability values for collisions and blade strikes, that also include avoidance rates, illustrate a current knowledge gap. Availability of animal-turbine interaction datasets facilitates validation of probability estimates and will allow resource managers to quantify injury and mortality of aquatic animals including species of special status such as the threatened Puget Sound Chinook salmon (Oncorhynchus tshawytscha) (Hall et al., 2018).
Empirical data from a demonstration tidal turbine site are used in this study but sample conditions may affect probability estimates. For example, fish densities derived from the Admiralty Inlet acoustic dataset are categorized as Pacific herring. Representing a mixed fish community by a single species in the conversion of acoustic backscatter measurements to density and abundance estimates is potentially biased, but any biases in the data are assumed constant and low. Pacific herring are used to represent pelagic, schooling fish that are common constituents of any fish community at a MRE site. The encounter-impact model does not explicitly include diel vertical migration, a factor that may affect probability estimates (e.g., Rossington and Benson, 2020), but did include day and night periods to reflect differences in vertical distributions. We did not include crepuscular changes in vertical distributions, which represent brief periods (<3% total) during a 24-hour cycle. Williamson et al. (2019) examined day/night fish aggregation vertical distributions in proximity to turbine structures and observed lower school heights at night compared to those during the day, suggesting that vertical migration can potentially influence probability estimates for specific times during a day, but also emphasizing our limited understanding of fish behavior in proximity of tidal devices. At the time of data collection there were no hydrokinetic devices deployed in Admiralty Inlet. Fish density data used in probability calculations lack information on fish-turbine interactions, necessitating the use of published avoidance, collision, and blade strike values. The absence of a turbine at the data collection site also precludes the ability to measure indirect or delayed impacts of animal-device interactions. Indirect or delayed impact examples include hydraulic shear stress (Hammar et al., 2015) and/or barotrauma (Copping et al., 2020b) that may lead to additional fish injury and/or mortality. Conditional probability values are calculated using empirical acoustic transect data along sequential steps in the encounter-impact model. The data serve as a series of spatiotemporal snapshots of fish distributions but do not explicitly include individual fish trajectories as they pass through a model domain.
The encounter-impact model provides a robust framework for estimating impact probabilities for both individual fish and specific turbines. The model is not structured to estimate encounter or impact probabilities for fish populations or for arrays of tidal turbines. Models such as Hammar et al.'s (2015) population collision risk model and the Exposure Time Population Model (ETPM) from Grant et al. (2014) estimate impacts of animal-tidal turbine interactions on populations encountering and interacting with a device. Hammar et al.'s (2015) model incorporates a component for tidal turbine array passage and co-occurrence, depicting fish approaching and being at the same depth as rotor blades during turbine operation. This component is used to estimate probabilities of a fish population passing through a tidal turbine array and potentially encountering rotor-swept areas of any turbine within the site. The ETPM estimates collision risk for diving birds interacting with MRE devices by evaluating mortality rates that would lead to population-level impacts, which could be adapted to estimate fish and aquatic mammal interaction and mortality rates. The challenge remains to scale high resolution, individual animal-device interactions to populations or species in a single model that accurately estimates impacts of MRE arrays on aquatic communities.
Our model combines analyses of empirical data from Admiralty Inlet with literature values to estimate probabilities of device encounters and impacts on fish. Numeric models can also be used to estimate values for unknown variables such as fish approach and turbine interaction that identify data gaps in the MRE research portfolio (Buenau et al., 2022). An alternate approach to address challenges associated with incomplete empirical data is agent-based modeling. Agent-based models represent populations using individual agents (i.e., organisms) containing unique traits and interact with other agents and their environment (DeAngelis and Mooij, 2005). Within a specified domain, agent-based models are initialized with parameter values and behavioral rules (e.g., aggregation, avoidance) formulated using field observations, existing datasets, or published values (Murphy et al., 2020). When applied to MRE, agent-based models can include individual and aggregative behaviors in response to hydrokinetic devices (e.g., Goodwin et al., 2004) that are then used to quantify impact probabilities for individuals (e.g., Rossington and Benson, 2020) or populations (Hammar et al., 2015). The combination of empirical data with numerical models is a formidable tool to assess fish interactions with MRE devices and is essential for informed regulatory decision-making, conservation strategies, and sustainable development of the MRE blue economy.
5 Conclusion
MRE is an applied science and engineering field that requires foundational and operational understanding through increased research and environmental monitoring. One area of uncertainty is characterizing fish avoidance behaviors, including reaction distances to MRE devices. This knowledge gap hinders permitting/consenting and subsequent development of MRE projects worldwide. To facilitate progress from demonstration projects to commercial-scale sites, it is essential to implement effective risk management strategies, comprehensive environmental monitoring, and regulatory frameworks that provide clear standards for operation of all MRE sectors including tidal energy (Inger et al., 2009). The encounter-impact empirical model in this study estimates probabilities of occurrence for sequential stages of fish interactions with tidal turbines and can be adapted for any species, location, and device. The comprehensive yet flexible structure of this model serves as a starting point to quantify encounter and impact risks and to further discussion on impact uncertainty.
Data availability statement
The raw data supporting the conclusions of this article will be made available by the authors, without undue reservation.
Ethics statement
Only remotely sensed, acoustic data were collected in the original study by the University of Washington. Species composition and length data from net catches were originally collected by and under the authority of the Washington State Department of Fish and Wildlife (WDFW). We received data that were used to choose the type species and calculate an average length for conversion of acoustic backscatter (i.e., reflected energy) measurements to fish density and abundance estimates.
Author contributions
JP: Conceptualization, Data curation, Formal Analysis, Investigation, Methodology, Software, Visualization, Writing – original draft, Writing – review & editing. JH: Conceptualization, Funding acquisition, Investigation, Methodology, Project administration, Resources, Supervision, Validation, Writing – review & editing.
Funding
The author(s) declare financial support was received for the research, authorship, and/or publication of this article. This work was supported by a Department of Energy grant (DE-EE-0006816.0000) to the ALFA project with additional financial support of a Graduate Fellowship from the School of Aquatic and Fishery Sciences, University of Washington, Seattle, Washington. This publication is partially funded by the Cooperative Institute for Climate, Ocean, & Ecosystem Studies (CICOES) under NOAA Cooperative Agreement NA20OAR4320271, Contribution No. 2023-1300.
Acknowledgments
We thank the captain and crew of the RV Centennial along with the science crew who collected and processed the Admiralty Inlet acoustic and fish catch data: Dale Jacques, Sandra Parker-Stetter, Hannah Linder, and Jennifer Nomura.
Conflict of interest
The authors declare that the research was conducted in the absence of any commercial or financial relationships that could be construed as a potential conflict of interest.
Publisher’s note
All claims expressed in this article are solely those of the authors and do not necessarily represent those of their affiliated organizations, or those of the publisher, the editors and the reviewers. Any product that may be evaluated in this article, or claim that may be made by its manufacturer, is not guaranteed or endorsed by the publisher.
Supplementary material
The Supplementary Material for this article can be found online at: https://www.frontiersin.org/articles/10.3389/fmars.2023.1270428/full#supplementary-material
References
Band B., Sparling C., Thompson D., Onoufriou J., San Martin E., West N. (2016). Refining estimates of collision risk for harbour seals and tidal turbines. Scott. Mar. Freshwater Sci. 7 (17), 123. doi: 10.7489/1786-1
Bangley C. W., Hasselman D. J., Flemming J. M., Whoriskey F. G., Culina J., Enders L., et al. (2022). Modeling the probability of overlap between marine fish distributions and marine renewable energy infrastructure using acoustic telemetry data. Front. Mar. Sci. 9. doi: 10.3389/fmars.2022.851757
Bender A., Langhamer O., Francisco F., Forslund J., Hammar L., Sundberg J., et al. (2023). Imaging-sonar observations of salmonid interactions with a vertical axis instream turbine. River Res. Appl. 39 (8), 1578–1589. doi: 10.1002/rra.4171
Berry M., Sundberg J., Francisco F. (2019). Salmonid response to a vertical axis hydrokinetic turbine in a stream aquarium. In 13th European Wave and Tidal Energy Conference (EWTEC).
Bevelhimer M., Scherelis C., Colby J., Adonizio M. A. (2017). Hydroacoustic assessment of behavioral responses by fish passing near an operating tidal turbine in the east river, new york’. Trans. Am. Fisheries Soc. 146 (5), 1028–1042. doi: 10.1080/00028487.2017.1339637
Buenau K. E., Garavelli L., Hemery L. G., García Medina G. (2022). A review of modeling approaches for understanding and monitoring the environmental effects of marine renewable energy. J. Mar. Sci. Eng. 10 (1), 94. doi: 10.3390/jmse10010094
Castro-Santos T., Haro A. (2015). Survival and behavioral effects of exposure to a hydrokinetic turbine on juvenile atlantic salmon and adult american shad. Estuaries Coasts 38 (1), 203–214. doi: 10.1007/s12237-013-9680-6
Copping A. E., Freeman M. C., Gorton A. M., Hemery L. G. (2020a). Risk retirement—Decreasing uncertainty and informing consenting processes for marine renewable energy development. J. Mar. Sci. Eng. 8 (3), 172. doi: 10.3390/jmse8030172
Copping A., Hemery L. (2020). OES-environmental 2020 state of the Science report: Environmental effects of marine renewable energy development around the world. Report for ocean energy systems (OES). PNNL–29976, 1632878. doi: 10.2172/1632878
Copping A., Hemery L., Overhus D., Garavelli L., Freeman M., Whiting J., et al. (2020b). Potential environmental effects of marine renewable energy development-the state of the science. J. Mar. Sci. Eng. 8 (11), 879–897. doi: 10.3390/jmse8110879
Copping A. E., Hemery L. G., Viehman H., Seitz A. C., Staines G. J., Hasselman D. J. (2021). ‘Are fish in danger? A review of environmental effects of marine renewable energy on fishes’. Biol. Conserv. 262, 109297. doi: 10.1016/j.biocon.2021.109297
Courtney M. B., Flanigan A. J., Hostetter M., Seitz A. C. (2022). Characterizing sockeye salmon smolt interactions with a hydrokinetic turbine in the kvichak river, alaska. North Am. J. Fisheries Manage. 42 (4), 1054–1065. doi: 10.1002/nafm.10806
DeAngelis D. L., Mooij W. M. (2005). Individual-based modeling of ecological and evolutionary processes. Annu. Rev. Ecology Evolution Systematics 36, 147–168. doi: 10.1146/annurev.ecolsys.36.102003.152644
Fox C. J., Benjamins S., Masden E. A., Miller R. (2018). Challenges and opportunities in monitoring the impacts of tidal-stream energy devices on marine vertebrates. Renewable Sustain. Energy Rev. 81, 1926–1938. doi: 10.1016/j.rser.2017.06.004
Goodwin R. A., Anderson J. J., Nestler J. M. (2004). ‘Decoding 3-d movement patterns of fish in response to hydrodynamics and water quality for forecast simulation’, in Hydroinformatics. World Sci. Publishing Company 2, 254–261. doi: 10.1142/9789812702838_0031
Grant M. C., Trinder M., Harding N. J. (2014). “A diving bird collision risk assessment framework for tidal turbines,” in Scottish natural heritage commissioned report no. 773. Available at: https://tethys.pnnl.gov/sites/default/files/publications/SNH-2014.pdf.
Grippo M., Zydlewski G., Shen H., Goodwin R. A. (2020). Behavioral responses of fish to a current-based hydrokinetic turbine under mutliple operational conditions. Environ. Monit. Assess. 192 (10), 645. doi: 10.1007/s10661-020-08596-5
Hall J. E., Greene C. M., Stefankiv O., Anderson J. H., Timpane-Padgham B., Beechie T. J., et al. (2018). ‘Large river habitat complexity and productivity of Puget Sound Chinook salmon. PloS One 13 (11), e0205127. doi: 10.1371/journal.pone.0205127
Hammar L., Andersson S., Eggertsen L., Haglund J., Gullström M., Ehnberg J., et al. (2013). Hydrokinetic turbine effects on fish swimming behaviour. PloS One 8 (12), e84141. doi: 10.1371/journal.pone.0084141
Hammar L., Eggertsen L., Andersson S., Ehnberg J., Arvidsson R., Gullström M., et al. (2015). A probabilistic model for hydrokinetic turbine collision risks: exploring impacts on fish. PloS One 10 (3), e0117756. doi: 10.1371/journal.pone.0117756
He (1993). Swimming speeds of marine fish in relation to fishing gears. ICES Mar. Sci. Symp 196, 183–189.
Hemery L. G., Copping A. E., Overhus D. M. (2021). Biological consequences of marine energy development on marine animals. Energies 14 (24), 8460. doi: 10.3390/en14248460
Horne J. K., Jacques D. A., Parker-Stetter S. L., Linder H. L., Nomura J. M. (2013). Evaluating Acoustic Technologies to Monitor Aquatic Organisms at Renewable Energy Sites Final Report (U.S. Dept. of the Interior, Bureau of Ocean Energy Management. BOEM), 2014–2057. Available at: https://espis.boem.gov/final%20reports/5415.pdf.
Inger R., Attrill M. J., Bearhop S., Broderick A. C., James Grecian W., Hodgson D. J., et al. (2009). Marine renewable energy: potential benefits to biodiversity? An urgent call for research. J. Appl. Ecol. 46 (6), 1145–1153. doi: 10.1111/j.1365-2664.2009.01697.x
Jacques D. A. (2014) Describing and Comparing Variability of Fish and Macrozooplankton Density at Marine Hydrokinetic Energy Sites. Available at: https://digital.lib.washington.edu:443/researchworks/handle/1773/27479.
Muirhead J., Sprules W. G. (2003). Reaction distance of Bythotrephes longimanus, encounter rate and index of prey risk for Harp Lake, Ontario. Freshw. Biol. 48 (1), 135–146. doi: 10.1046/j.1365-2427.2003.00986.x
Müller S., Muhawenimana V., Sonnino-Sorisio G., Wilson C. A. M. E., Cable J., Ouro (2023). ‘Fish response to the presence of hydrokinetic turbines as a sustainable energy solution’. Sci. Rep. 13 (1), 7459. doi: 10.1038/s41598-023-33000-w
Murphy K. J., Ciuti S., Kane A. (2020). An introduction to agent-based models as an accessible surrogate to field-based research and teaching. Ecol. Evol. 10 (22), 12482–12498. doi: 10.1002/ece3.6848
Pelc R., Fujita R. M. (2002). Renewable energy from the ocean. Mar. Policy 26 (6), 471–479. doi: 10.1016/S0308-597X(02)00045-3
Romero-Gomez, Richmond M. C. (2014). Simulating blade-strike on fish passing through marine hydrokinetic turbines. Renewable Energy 71, 401–413. doi: 10.1016/j.renene.2014.05.051
Rossington K., Benson T. (2020). An agent-based model to predict fish collisions with tidal stream turbines. Renewable Energy 151, 1220–1229. doi: 10.1016/j.renene.2019.11.127
Sanderson B. G., Bangley C. W., McGarry L., Hasselman D. J. (2023). Measuring detection efficiency of high-residency acoustic signals for estimating probability of fish–turbine encounter in a fast-flowing tidal passage’. J. Mar. Sci. Eng. 11 (6), 1172. doi: 10.3390/jmse11061172
Shen H., Zydlewski G. B., Viehman H. A., Staines G. (2016). Estimating the probability of fish encountering a marine hydrokinetic device. Renewable Energy 97, 746–756. doi: 10.1016/j.renene.2016.06.026
Staines G. J., Mueller R. P., Seitz A. C., Evans M. D., O’Byrne P. W., Wosnik M. (2022). Capabilities of an acoustic camera to inform fish collision risk with current energy converter turbines. J. Mar. Sci. Eng. 10 (4), 483. doi: 10.3390/jmse10040483
Thomas G. L., Kirsch J., Thorne R. E. (2002). Ex situ target strength measurements of pacific herring and pacific sand lance. North Am. J. Fisheries Manage. 22 (4), 1136–1145. doi: 10.1577/1548-8675(2002)022<1136:ESTSMO>2.0.CO;2
Utne A. C. W. (1997). The effect of turbidity and illumination on the reaction distance and search time of the marine planktivore Gobiusculus flavescens. J. Fish Biol. 50 (5), 926–938. doi: 10.1111/j.1095-8649.1997.tb01619.x
Viehman H. A., Zydlewski G. B. (2015). Fish interactions with a commercial-scale tidal energy device in the natural environment. Estuaries Coasts 38 (1), 241–252. doi: 10.1007/s12237-014-9767-8
Viehman H. A., Zydlewski G. B., McCleave J. D., Staines G. J. (2015). Using hydroacoustics to understand fish presence and vertical distribution in a tidally dynamic region targeted for energy extraction. Estuaries Coasts 38 (1), 215–226. doi: 10.1007/s12237-014-9776-7
Williamson B. J., Fraser S., Blondel P., Bell P. S., Waggitt J. J., Scott B. E. (2017). Multisensor acoustic tracking of fish and seabird behavior around tidal turbine structures in scotland. IEEE J. Oceanic Eng. 42 (4), 948–965. doi: 10.1109/JOE.2016.2637179
Williamson B., Fraser S., Williamson L., Nikora V., Scott B. (2019). Predictable changes in fish school characteristics due to a tidal turbine support structure. Renewable Energy 141, 1092–1102. doi: 10.1016/j.renene.2019.04.065
Wilson B., Batty R., Daunt F., Carter C. (2006). “Collision risks between marine renewable energy devices and mammals, fish and diving birds,” in Report to the scottish executive. Available at: https://tethys.pnnl.gov/publications/collision-risks-between-marine-renewable-energy-devices-mammals-fish-diving-birds.
Yoshida T., Furuichi D., Williamson B. J., Zhou J., Dong S., Li Q., et al. (2021). Experimental study of fish behavior near a tidal turbine model under dark conditions. J. Mar. Sci. Technol 27, 541–548. doi: 10.1007/s00773-021-00850-w
Yoshida T., Zhou J., Park S., Muto H., Kitazawa D. (2020). ‘Use of a model turbine to investigate the high striking risk of fish with tidal and oceanic current turbine blades under slow rotational speed’. Sustain. Energy Technol. Assessments 37, 100634. doi: 10.1016/j.seta.2020.100634
Keywords: collision risk, empirical modeling, encounter, environmental impact, fish, hydrokinetic turbines, marine renewable energy, tidal energy
Citation: Peraza JI and Horne JK (2023) Quantifying conditional probabilities of fish-turbine encounters and impacts. Front. Mar. Sci. 10:1270428. doi: 10.3389/fmars.2023.1270428
Received: 31 July 2023; Accepted: 23 October 2023;
Published: 09 November 2023.
Edited by:
Shengjie Rui, Norwegian Geotechnical Institute (NGI), NorwayReviewed by:
Lysel Garavelli, Pacific Northwest National Laboratory (DOE), United StatesKanmin Shen, PowerChina Huadong Engineering Corporation Limited, China
Hang Xu, Zhejiang University, China, in collaboration with reviewer KS
Copyright © 2023 Peraza and Horne. This is an open-access article distributed under the terms of the Creative Commons Attribution License (CC BY). The use, distribution or reproduction in other forums is permitted, provided the original author(s) and the copyright owner(s) are credited and that the original publication in this journal is cited, in accordance with accepted academic practice. No use, distribution or reproduction is permitted which does not comply with these terms.
*Correspondence: Jezella I. Peraza, amV6cGVyQHV3LmVkdQ==