Overestimation of microbial community respiration caused by nitrification, and the identification of keystone groups associated with respiration
- 1Institute of Marine Science and Technology, Shandong University, Qingdao, China
- 2Center Tech Tianjin Chemical Research and Design Institute Co., Ltd., Tianjin, China
- 3Southern Marine Science and Engineering Guangdong Laboratory, Zhuhai, China
- 4Fujian Key Laboratory of Marine Carbon Sequestration, Xiamen University, Xiamen, China
Instruction: Microbial community respiration (MCR) strongly controls the fate of organic carbon in the ocean. The balance between MCR and primary production strongly determines whether the ocean is a net sink or source of CO2 to the atmosphere. Thus, it is necessary to estimate MCR to better understand the role of oceans in the global carbon cycle. Methods based on apparent oxygen utilization (AOU) are predominant while electron transport system (ETS) assay gets increasing attention. Although methods get developed, few studies on MCR have been performed on a seasonal cycle. Because MCR is strongly associated with the temperature which changes along with the succession of seasons, it is urgent to study the MCR on a seasonal cycle.
Methods: Thus, we measured MCR using in vivo tetrazolium salt 2-(p-iodophenyl)-3-(p-nitrophenyl)-5-phenyltetrazolium chloride (INT) reduction rates (ETS) and oxygen-optode methods (AOU) simultaneously we measured the MCR based on AOU and ETS methods simultaneously from November 2020 to November 2021 in Aoshan Bay, China.
Results: The highest AOU appeared in autumn, followed by summer, spring, and winter, whereas the highest ETS activity appeared in summer, followed by spring, autumn and winter. The seasonal trend of MCR estimated from AOU and ETS were not consistent, and further analysis indicated that oxygen consumption induced by nitrification caused the overestimation of MCR in autumn evaluated from AOU.
Discussion: Microbial groups that were strongly correlated with MCR estimated by ETS had the ability to degrade various substrates and could get energy directly from light. It should be careful to notice the deviation of assumed organic carbon demand based on ETS caused by the alternation of day and night. Furthermore, the pattern of bacterial groups associated with year-round MCR was distinct from season-specific MCR. This study raised a warning for caution when estimating MCR based on AOU and it was better to fully take the photoheterotrophy into account when assuming organic carbon remineralization based on ETS.
1 Introduction
The metabolic activity of oceans is dominated by microorganisms; they play pivotal roles in mineralizing aquatic organic carbon, thus the overall patterns of oceanic carbon flux are largely controlled by microbes (Wang, 2018). One-half of the primary production of the biosphere occurs in the ocean (Kulk et al., 2020; Crockford et al., 2023) and a large fraction of it becomes dissolved (dissolved organic matter, DOM) (Dittmar et al., 2021). This dissolved part of the oceanic primary production is almost exclusively accessible to bacteria and archaea (Dittmar et al., 2021; Love et al., 2021). The assimilated carbon is respired or used to increase bacterial biomass, with the former accounting for 60-99% (Reinthaler and Herndl, 2005; Alonso-Sáez et al., 2007). The fate of carbon mediated by microbes strongly determines whether the ocean is net heterotrophic or autotrophic (Hansell et al., 2004; Reinthaler and Herndl, 2005). The trophic state of the ocean decides whether the ocean is a net source or net sink of CO2 to the atmosphere, thus impacting the global climate (Holden et al., 2018; Friedlingstein et al., 2022). The primary productivity of phytoplankton and the mineralization of microorganisms affect the carbonate system. (Carla et al., 2022). It is necessary to estimate the organic carbon respiration by microbes to better understand the role of the ocean in the global carbon cycle. The amount of organic carbon respired to inorganic carbon can be quantified by microbial community respiration (MCR), thus it is a fundamental parameter in estimating the importance of bacteria in organic carbon remineralization (Guo et al., 2022).
The coastal ocean connects different carbon pools (terrestrial, atmosphere, open ocean, and sediment) and constitutes one of the most biogeochemically active zones on Earth. The general view is that the global coastal ocean is a net sink for atmospheric CO2 (Laruelle et al., 2014; Gruber, 2015), taking up -0.45 Pg C year−1 (Borges et al., 2005) to -0.21 Pg C year−1 (Laruelle et al., 2010) of CO2 from the atmosphere. This is largely induced by high primary production in these regions (Borges et al., 2005; Hernández-León et al., 2020). Although the coastal ocean covers only 7% of the global ocean, it contributes approximately 30% of the oceanic primary production (Gattuso et al., 1998). The balance between primary production and microbial respiration determines the roles of the coastal ocean in the global carbon cycle. Although MCR is reported as being strongly associated with temperature (García et al., 2023), which changes along with seasons, large fractions of previous studies on MCR have been performed within a certain time frame, with few studies being performed on a seasonal cycle. In this study, we measured MCR covering a full season cycle in Aoshan Bay to determine the seasonal dynamics of MCR and other environmental variables.
Major available approaches in MCR measurement can roughly be divided into two categories: (I) based on the measurement of the decline of a reactant (typically oxygen) or increase of a product (typically CO2); and (II) based on the detection of the activity of enzymatic indicators (electron transport system (ETS) assay). Methods based on apparent oxygen utilization (AOU) are predominant, and 91% of the observation data of MCR are estimated with the Winkler method (Del Giorgio and Williams, 2005), which is the typical method for monitoring dynamics of oxygen concentration (Carpenter, 1965). Studies indicated that the bacterial respiration rate obtained by the Winkler method is overestimated, largely caused by the deficiencies of the method itself (Aranguren-Gassis et al., 2012; Martínez-García et al., 2013; García-Martín et al., 2019). With the development of technology, increasing methods to monitor the change of dissolved oxygen concentration have been developed, for example, the optodes sensor method (Warkentin et al., 2007). It is reported that the optodes method is better than conventional procedures (Warkentin et al., 2007). The deficiencies of the methodology are few, so does the MCR estimated based on oxygen consumption reflect the real MCR in the ocean? Thanks to accurate MCR being fundamental to estimating the carbon flow in marine food webs and the role of oceans in the global carbon cycle, we measured the MCR based on the in vivo INT method and optodes method continuously from November 2020 to November 2021 in Aoshan Bay, China (120°72’E, 36°36’N).
2 Methods and materials
2.1 Environmental variables determination
The temperature and salinity were recorded with Multiparameter Sonde (YSI EXO, YSI Inc.). The concentration of dissolved oxygen (DO) was measured using the Winkler method (Carpenter, 1965). The pH value of seawater was measured using a pH meter (StarA211, Thermo Fisher Scientific). For nutrient (ammonium, nitrite, nitrate, silicate, and phosphate) analysis, seawater was prefiltered with a 0.45 µm pore-size filter (Millipore) and then stored in 15 mL tubes. Samples were measured using a Skalar SAN++ (AutoAnalyzer3, Seal Analytical) at Shandong University (precision: ± 0.1μmol/kg).
To measure the concentration of total organic carbon (TOC), 30 mL of seawater was transferred to 40 mL vials (CNW). All TOC samples were acidified to a pH value of 2 to 3 with 85% phosphoric acid to remove inorganic carbon and were then stored at -20 °C until analyses. The concentration of TOC was detected using an organic carbon analyzer (TOC-L CPH/CON, Shimadzu) following the high-temperature combustion method (Hansell and Carlson, 2001). Seawater used for fluorescent dissolved organic matter (FDOM) analysis was filtered through precombusted (450 °C, 6 h) 0.7 µm pore size GF/F membranes. The filtrate was stored in 40 mL precombusted (450 °C, 6 h) glass vials (CNW) at -20 °C in the dark until analysis. Absorbance and excitation-emission matrix (EEM) fluorescence spectra were recorded simultaneously using the Yvon Horiba Aqualog system (Aqualog-UV-800-C, HORIBA INSTRUMENTS INC). Excitation was scanned from 250 to 500 nm at 5 nm steps, and emission was recorded from 300 to 600 nm at 2 nm intervals. Fluorescence spectra were normalized to the area of the water Raman peak when the excitation was at 350 nm (Lawaetz and Stedmon, 2009). Three PARAFAC components (C1-C3) were identified and were compared to the OpenFluor database (Murphy et al., 2014). Two fluorescence-related parameters, biological index (BIX) and humification index (HIX), were calculated (Hansen et al., 2016). Detailed information on the calculation of HIX and BIX is provided in the Supplementary Material.
To detect the concentration of total chlorophyll a (Chl a), 1 L of seawater was filtered through 0.22 µm pore-size polycarbonate filters (47 mm diameter, Whatman). Filtered samples were stored in 15 mL tubes and subsequently transferred to -80 °C until analysis. The concentration of Chl a was determined using the 90% acetone method. First, tubes were filled with 4 mL 90% acetone and the filter was immersed in the acetone for 12 h at 4 °C. Then, the tube was centrifugated for 10 min at 4000 rpm at 18 °C. After that, the absorbance of acetone containing Chl a was measured using a spectrophotometer (UV-2700, Shimadzu) with selected wavelengths from 500-750 nm. Finally, the concentration (ug·mL-1) of Chl a was calculated using the following equation: Chl a=11.47×(A664-A750)-0.40×(A630-A750), where A represents the value of absorbance at special wavelength (Jeffrey and Humphrey, 1975; Wellburn, 1994).
To measure microbial cell abundance, 2 mL triplicate seawater samples were collected and fixed by adding glutaraldehyde (final concentration of 0.5%) for 20 min in the dark at room temperature. Then the samples were fast-frozen in liquid nitrogen and stored at -80 °C until analysis. Cell abundance was measured using a flow cytometer (Accuri C6, Becton and Dickinson) following a previously established protocol (Marie et al., 1997).
2.2 Molecular analysis
To measure bacterial community structure, 1 L of seawater was prefiltered through a 20-µm-pore-size bolting cloth and then filtered through 0.22-µm-pore-size polycarbonate membranes, and the membranes were stored in 2 mL RNAase-free tube at -80 °C until DNA extraction. PowerSoil Kit (MoBio®) was used to extract DNA according to the manufacturer’s protocol. The bacterial V3–V4 regions of 16S rRNA genes were amplified using primers 338F: 5’-ACTCCTACGGGAGGCAGCA-3’ and 806R: 5’-GGACTACNNGGGTATCTAAT-3’ (Guo et al., 2018). Amplicons were purified using an AxyPrep DNA Gel Extraction Kit (Axygen Biosciences) and then sequenced on an Illumina HiSeq 2500 platform.
Bioinformatic analysis was conducted following previous protocols (Zhang et al., 2021). Briefly, FLASH was used to merge paired-end clean reads. Then, the QIIME2 pipeline was used to denoise merged reads and remove chimera. Clean and non-chimeric sequences were further clustered into amplicon sequence variants (ASVs). To assign taxonomy, ASVs were checked against the SILVA database (v138).
2.3 Microbial community respiration rate determination
Seawater samples were collected from the surface using CTD. To elucidate how MCR varied over time at the surface of Aoshan Bay, we measured MCR using in vivo tetrazolium salt 2-(p-iodophenyl)-3-(p-nitrophenyl)-5-phenyltetrazolium chloride (INT) reduction rates (Martínez-García et al., 2009) and oxygen-optode methods (Jiao et al., 2021) simultaneously. Rates of MCR were estimated from the formation of INT-formazan (INT-F), the production of the reduction of the INT by electron transport system (ETS) dehydrogenase enzymes, following the protocol described by Martínez‐García (Martínez-García et al., 2009) with some modifications. The optodes sensor can directly record the change in oxygen concentration and reflect the apparent oxygen consumption in the bottle. Briefly, three replicates were immediately fixed by adding formaldehyde (2% v/v final concentration) and used as dead controls.
Samples (three dead controls and three live controls, 200 mL of each) were spiked with a solution of INT (with a final concentration of 0.2 mM), meanwhile, the optodes sensors (OXSPS TROXSP5, Pyroscience, Germany) were stuck inside the incubation bottle. It should be noted that the control should be put in the dark for 20 minutes before adding INT. Then, samples were incubated in the dark for 1 h (Supplementary Figure 3) in temperature-controlled (± 0.1 °C of the in situ temperature) incubation chambers. After incubation, formaldehyde (2% v/v final concentration) was added to the live samples, and the samples were kept in the dark for 20 minutes. Finally, all samples were filtered through 0.22 μm pore size polycarbonate membrane filters (47 mm diameter) at pressure < 0.02 MPa, and all filters were placed into cryovials. Then, 1.6 mL propanol was added to all cryovials, and INT-F was extracted by initially sonicating the cryovials for approximately 30 min at 50 °C using an ultrasonic bath. Propanol containing the INT-F was then transferred into microfuge vials and centrifuged. Finally, the concentration of INT-F was determined by absorption spectrophotometry (UV-2700, Shimadzu spectrophotometer, Japan) at 485 nm. The optode was calibrated before every measurement and the signal was measured periodically, every 10 min. Oxygen consumption rates for each sample were obtained from the slope of the linear regressions. Detailed information on respiration rate estimation is provided in the Supplementary Material.
2.4 Statistical analyses
NMDS analysis was conducted in R using the metaMDS function in the vegan package. NMDS was performed on the relative abundance of ASVs in order to focus on relative changes in microbial community compositions.
To link MCR with physical-chemical variables and biogeochemical processes, partial least squares path modeling (PLS-PM) was performed. Latent variables, namely, nutrients and α-diversity, included more than one manifest variable, with nutrients including ammonium and nitrite, and α-diversity including Simpson, ACE, and Pielous. The model was constructed using the plspm package in R. Variables with loadings < 0.7 were removed and the remaining variables were used to perform the final PLS-PM structure equation (Gao et al., 2019). The prediction performance of models was assessed by the goodness of fit (GOF) and R2 value.
The maximal information coefficient (MIC) can capture diverse relationships between two pairs of variables (Reshef et al., 2011). The dataset consisted of 22 microbial samples and 18 environmental variables. MIC analyses were run using B=0.6, CV=0.5, and statistically significant relationships with MIC≥0.4 (Logares et al., 2020) were considered. A precomputed p-value was used to assess MIC significance (Reshef et al., 2011).
The correlation among different species at the order level was calculated in R. Only robust (correlation coefficient ≥ 0.6 or ≤ -0.6) and statistically significant (p < 0.05) correlations were considered, and networks were visualized using Cytoscape v3.9.1. The fast greedy modularity optimization was used to find modules of highly correlated taxa (Clauset et al., 2004). Groups of ASVs in a module are highly connected among themselves, whereas they have fewer connections with ASVs outside the module. Positive correlations indicate the relative abundance of ASV changes along the same trend, whereas negative correlations signify the relative abundance of ASV changes in the opposite direction. We used the mantel test to calculate the correlation between different modules with in vivo INT reduction rate, and significant modules were chosen to do further analysis. Spearman correlation between species within the chosen module and the in vivo INT reduction rate were assessed in R. This was helpful in finding microbial groups that played pivotal roles in determining MCR.
3 Results
3.1 Environmental variables
The variation in season nearshore environment in Aoshan Bay causes a number of changes, including changes in temperature, DO, Chl a, nutrients, microbial community compositions, organic matter, and MCR. Sampling took place from November 2020 through November 2021.
The temperature fluctuated along with the season and the ranking of the four seasons was summer, spring, autumn, and winter (Supplementary Figure 2A). The same trend was observed in cell abundance (Supplementary Figure 2C). For DO, the situation was the opposite: DO was lower in summer than in other seasons (Supplementary Figure 2D). The value of pH ranged from 7.9 to 8.3 (Supplementary Figure 2C). The concentration of Chl a was increasing with time and peaked in summer, but decreased after summer (Supplementary Figure 2F). The coastal ocean was strongly impacted by freshwater input, thus the salinity drastically changed (Supplementary Figure 2B). Salinity was lower in autumn than in other seasons (Supplementary Figure 2B). The changes in DIN and nitrifiers are shown together in the following section to better explain the dynamics of the nitrogen cycle.
3.2 Microbial community respiration and FDOM
The MCR was estimated using in vivo INT reduction rates and oxygen consumption rates simultaneously. The INT reduction rate ranged from 0.022 ( ± 0.004) to 0.441 ( ± 0.045) μmol INTF L-1 h-1 and the oxygen consumption rate ranged from 0.057 ( ± 0.044) to 9.840 ( ± 0.857) μmol O2 L-1 h-1 (Figure 1). Mean MCR was 0.124 μmol INTF L-1 h-1 and 1.544 μmol O2 L-1 h-1, respectively. The INT reduction rate was increasing from winter to summer and the same trend was true for the oxygen consumption rate (Figure 1). When it shifted from summer to autumn, the INT reduction rate decreased while the oxygen consumption rate still increased (Figure 1). Thus, the MCR was the highest in summer based on ETS but the highest in autumn based on oxygen consumption rate (Figure 1). According to the season, four different periods of respiration rates were defined: spring, summer, autumn, and winter. The INT reduction rate was summer (0.251 μmol INTF L-1 h-1) > spring (0.104 μmol INTF L-1 h-1) > autumn (0.077 μmol INTF L-1 h-1) > winter (0.050 μmol INTF L-1 h-1), whereas the oxygen consumption rate was autumn (3.262 μmol O2 L-1 h-1) > winter (1.405 μmol O2 L-1 h-1) > summer (1.150 μmol O2 L-1 h-1) > spring (0.724 μmol O2 L-1 h-1). These results reflected the divergence of MCR estimated with different methods.
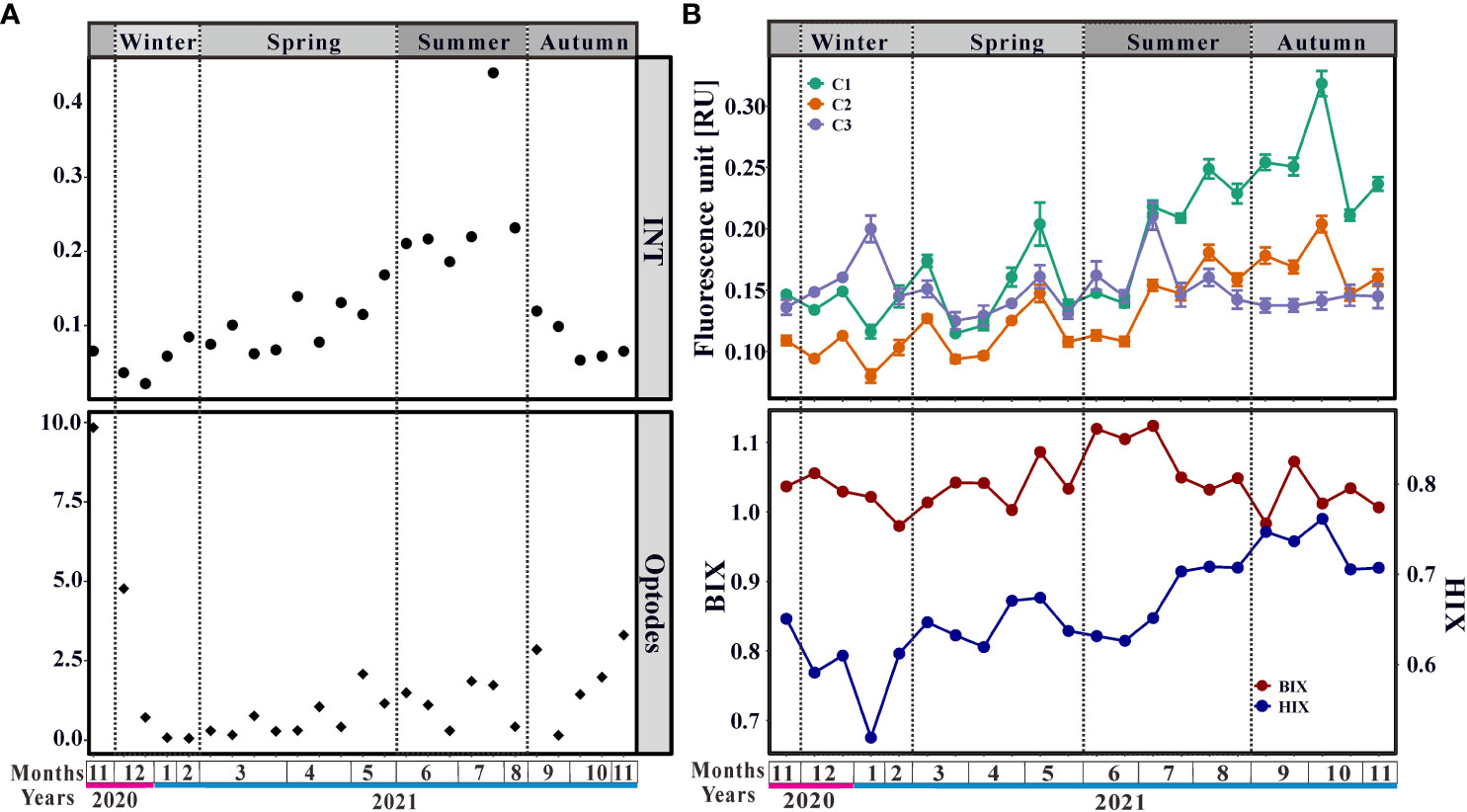
Figure 1 Changes of (A) INT reduction rates (μmol INTF L-1 h-1) and oxygen consumption rates (μmol O2 L-1 h-1), and (B) components of Fluorescent Dissolved Organic Matter (FDOM).
The PARAFAC model decomposed three components (C1, C2, and C3), which were statistically validated using the split-half validation (Supplementary Figure 4). These components were confirmed with the OpenFluor database (Murphy et al., 2014). C1 (Maxex/em=250(310)/398 nm) and C2 (Maxex/em=250(360)/480 nm) were characterized as humic-like components, and C3 (Maxex/em=275/323 nm) exhibited protein-like component properties. C1 and C2 had similar variations in that fluorescence intensity increased over time and peaked in autumn (Figure 1B). In contrast, the fluctuation of C3 was more slight and a peak appeared in winter (Figure 1B). Besides, HIX increased with time and peaked in autumn. These results indicated that refractory humic-like components accumulated in autumn. The differences in BIX in winter, spring, and autumn were slight, and BIX in summer was higher than in other seasons (Figure 1B).
3.3 Microbial community dynamics
At the phylum level, communities were dominated by Proteobacteria (58.3%), Bacteroidota (20.1%), and Actinobacteriota (8.6%) (Figure 2A), while at the order level, communities were dominated by Rhodobacterales (32.8%), Flavobacteriales (17.6%), Actinomarinales (6.3%), Alteromonadales (4.0%), Burkholderiales (3.4%), and Oceanospirillales (3.3%) (Figure 2B). The relative abundance of Proteobacteria reached a peak in late January, lasted until March, and then decreased (Figure 2A). Actinobacteriota was higher in autumn than in other seasons (Figure 2A). It should be noted that the relative abundance of Cyanobacteria peaked in August and then decreased with time (Figure 2A). Differences among seasons were little for Bacteroidota (Figure 2A). Although no hypoxia occurred in Aoshan Bay (Supplementary Figure 2D), the sulfate-reducing bacteria Desulfobacterota was abundant in groups throughout the year (Figure 2A), being highest in spring and lowest in winter (Figure 2A).
Rhodobacterales (33%), Flavobacteriales (18%), Actinomarinales (6%), and Alteromonadales (4%) were predominant throughout the year at the order level (Figure 2B). Oceanospirillales were significantly higher in summer than in other seasons and the same was true for Chitinophagales and Vibrionales (Figure 2B). Actinomarinales accounted for a larger proportion in autumn than in other seasons (Figure 2B). Alteromonadales were higher in winter and summer than in spring and autumn (Figure 2B). As for Burkholderiales, the relative abundance was higher in the first (winter and spring) half of the year than in the second (summer and autumn) half (Figure 2B). SAR11_clade was clearly higher in later spring than in other periods (Figure 2B). Similar to Cyanobacteria, the bloom of synechococcales appeared in late summer and decreased with time (Figure 2B).
Dissolved inorganic nitrogen (DIN) concentration changed along with the seasons (Figure 3A). Ammonium, nitrite, and nitrate were highest in autumn as compared to the rest of the year (Figure 3A). The concentration of ammonium changed little, being low before August and then increasing, causing ammonium accumulation in autumn (Figure 3A). Although the concentration of nitrite fluctuated with each month, it was higher in autumn than in other seasons (Figure 3A). Nitrate concentration increased in winter and early spring, but then dropped, with the lowest value appearing in summer (Figure 3A). The concentration of nitrate increased from July onward, peaking in autumn (Figure 3A). Nitrifiers were widespread in the coastal ocean and four species were found in Aoshan Bay (Figure 3B). In winter and spring, ammonium-oxidizing bacteria (AOB) belonging to Nitrosococcales were clearly dominant (Figure 3B). Nitrosomonadaceae, another group affiliated to AOB, dominated the nitrifying bacteria in summer (Figure 3B). Nitrite-oxidizing bacteria (NOB), Nitrospinales and Nitrospirales, were higher in autumn than in other seasons (Figure 3B). Bloom of Nitrospirales was also observed in the second half of spring (Figure 3B). In summary, AOB was the dominant nitrifier in Aoshan Bay except in autumn, during which the relative abundance of AOB was not clearly higher than that of NOB (Figure 3B).
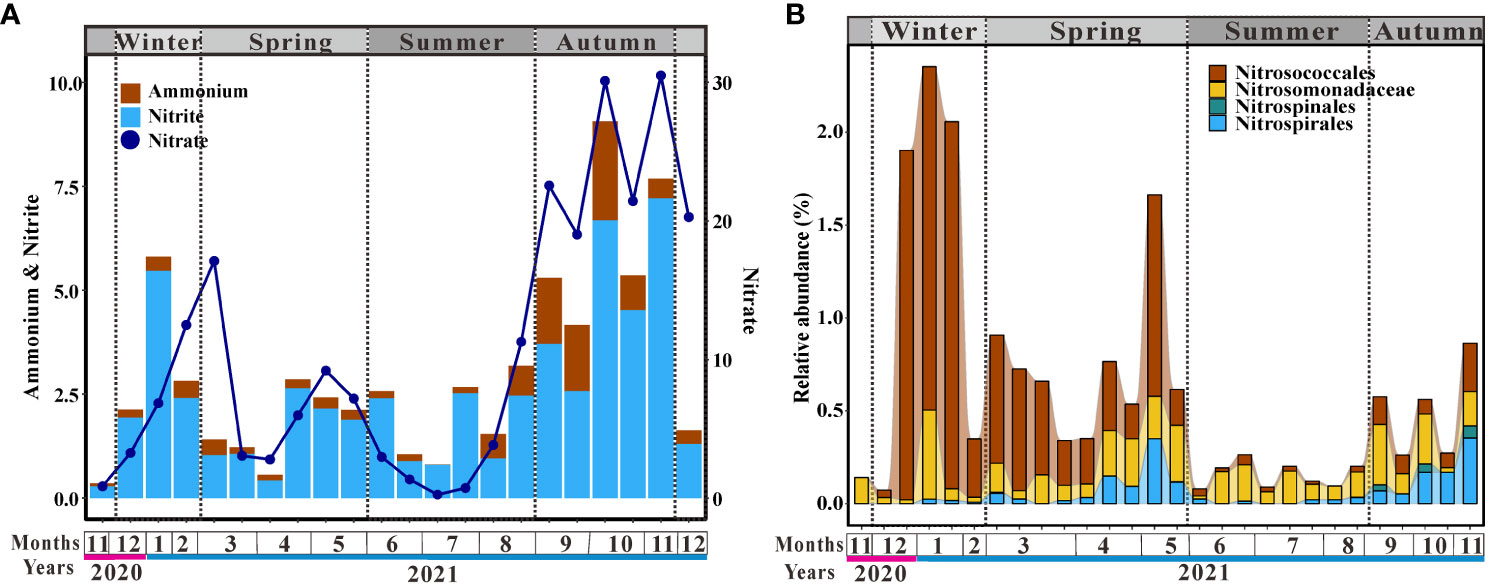
Figure 3 Changes of (A) dissolved inorganic nitrogen and (B) relative abundance of major nitrifiers.
3.4 Links between physical-chemical parameters and microbial activities
MCR was well explained by our block variables (R2 = 0.86) and provided a good fit to our data (GOF of 0.62; Figure 4A). Temperature showed the largest effect on MCR via direct (path coefficient = 0.38) and indirect (path coefficient = 0.37) effects. There were strong positive correlations between TOC and MCR, and a similar trend was observed between cell abundance and MCR (Figure 4A). It was meaningful to notice the negative effect of nutrients on DO (Figure 4A).
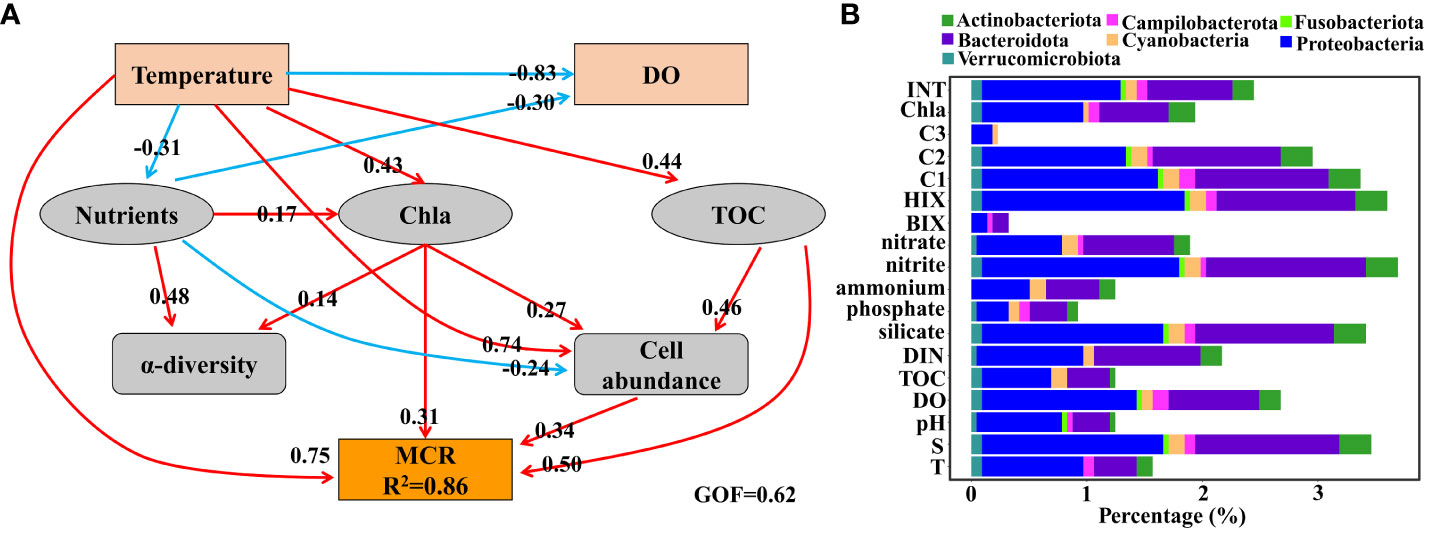
Figure 4 (A) Partial least squares path analysis for microbial community respiration (MCR), showing the relationships between selected physical-chemical parameters, characteristics of microbes, and MCR. GOF, goodness of fit; DO, dissolved oxygen; TOC, total organic carbon; Chl a, chlorophyll (a) Red and blue lines represent positive and negative correlations, respectively. For simplicity, only effects that were not less than 0.1 are shown in the plot. Meanwhile, the effects of oxygen on other parameters are also not shown in the plot as a result of no hypoxia in the sampling field. (B) The percentage of ASVs significantly correlated with various environmental parameters (based on maximal information coefficient). Information on the taxonomy of ASVs is shown in different colors.
To investigate how individual species responded to physical-chemical parameters, MIC was used to determine the correlation of single species with multiple abiotic environmental variables (Figure 4B). Nitrite was the variable with the highest number of associated species (3.7%), followed by HIX (3.6%), salinity (3.5%), silicate (3.4%), C1 (3.4%) and C2 (3.0%) components of FDOM, DO (2.7%), ETS (2.5%), and DIN (2.2%) (Figure 4B). The remaining variables displayed associations with individual species that were of less than 2% (Figure 4B). Proteobacteria featured a greater proportion of individual species associations with almost all physical-chemical parameters than other groups (Figure 4B). Except for Proteobacteria, species affiliated with Bacteroidota were associated dominantly with almost all environmental variables (Figure 4B). Species significantly related to INT belonged to Actinobacteriota (0.18%), Proteobacteria (1.20%), Fusobacteriota (0.05%), Cyanobacteria (0.09%), Campilobacterota (0.09%), Bacteroidota (0.74%), and Verrucomicrobiota (0.09%) (Figure 4B).
3.5 Keystone microbial groups linking MCR
The NMDS plot calculated from the bacterial communities on the basis of the Bray-Curtis index displayed that samples collected during the same season preferred to cluster together and microbial dynamics were significantly related to seasons (Supplementary Figure 4). Furthermore, MIC displayed that 2.5% of ASVs significantly associated with INT that represented the intensity of MCR (Figure 4B). Because much information is lost at lower taxonomic levels, network and mantel tests were conducted to detect microorganisms strongly determining MCR at the order level. Five major modules based on the co-occurrence of groups (hereafter referred to as Modules 1-5) were identified (Figure 5A). Among them, Module 1 significantly positively correlated with the in vivo INT reduction rate (Mantel test, p<0.05). Within Module 1, by correlating the Spearman correlation coefficient between module membership with MCR assessed by the in vivo INT reduction rate method, we identified orders that were significantly correlated with MCR. Results showed that 14 orders were significantly correlated to MCR throughout the year (Figure 5B). Three orders were strongly associated with MCR in spring, while there were six in summer, zero in autumn, and one in winter (Figure 5B).
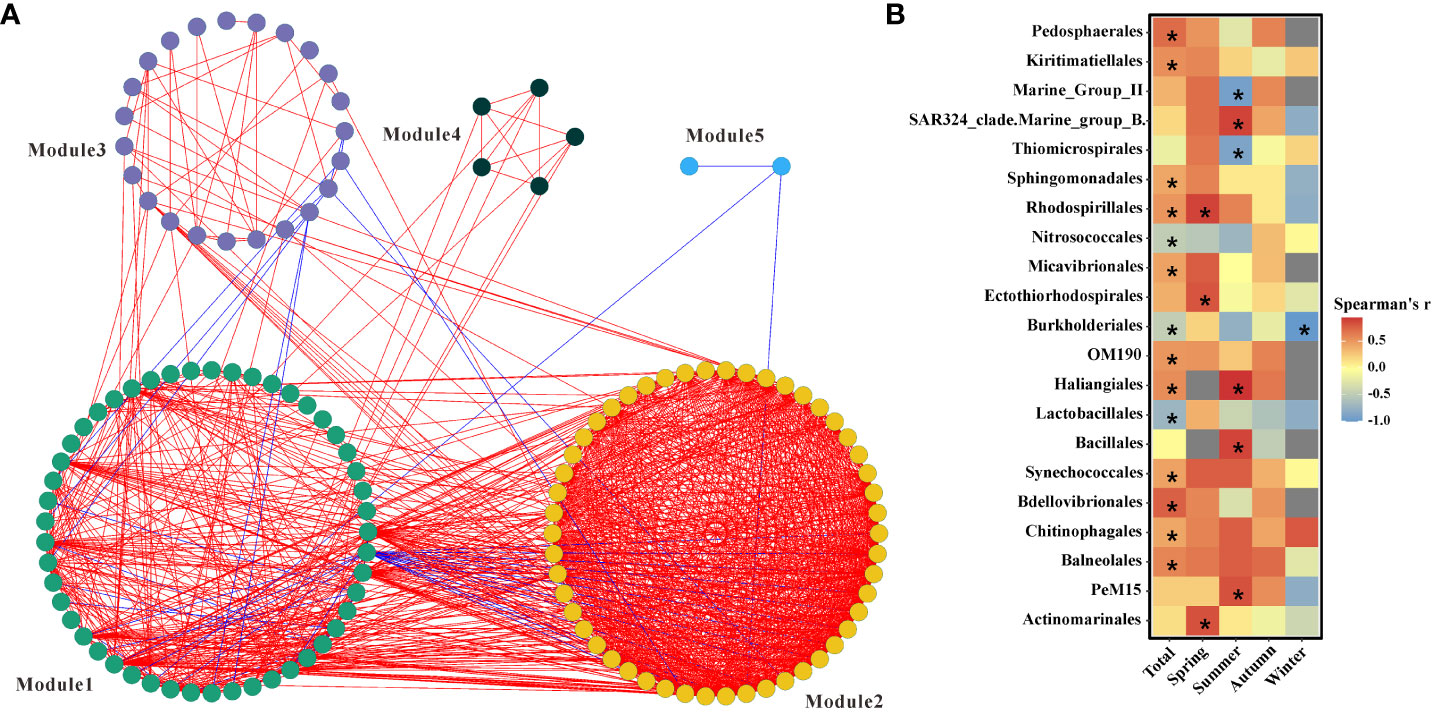
Figure 5 (A) Network interactions between microbial groups. Red and blue lines indicate positive and negative interactions between microbial groups, respectively. (B) significant correlations between microbial groups involved in module 1 and the in vivo INT reduction rate.
4 Discussion
4.1 The divergence of MCR estimated based on in vivo INT reduction rate and oxygen consumption rate
It is worth noting that the peak of MCR appeared in summer based on the in vivo INT reduction rate, and in autumn based on the optodes method, (Figure 1A). Studies prove that MCR is strongly impacted by characteristics and concentration of organic matter (Cleveland et al., 2007; Alonso-Sáez et al., 2008; Chin et al., 2023). In Aoshan Bay, changes in FDOM indicated that the humic-like components accumulated in autumn (Figure 1B). In general, humic-like components are recalcitrant to microbial degradation and accumulate in field and incubation experiments (Tanaka et al., 2014; Zheng et al., 2019). The relationship between MCR and the concentration of organic carbon is generally significantly positive (Alonso-Sáez et al., 2008), and the results of PLS-PM supported this (Figure 4A). It can be inferred that MCR should be lower in autumn than in summer because the organic matter is more refractory, meanwhile, the concentration of it is lower in autumn than in summer. Furthermore, temperature is another important environmental factor determining MCR, which has been supported in our study (Figure 4A), and generally MCR is positively correlated with temperature and peaks in summer (Lucea et al., 2005; Apple et al., 2006; Smith et al., 2021). Changes of organic carbon pool and temperature supported that MCR should be highest in summer than in other seasons. The change of the in vivo INT reduction rate was in accordance with this view, however, this was not true to the oxygen consumption rate (Figure 1A). This implied that MCR constituted less proportion of total oxygen consumption in autumn and there were other processes causing oxygen depletion.
Nitrifiers are universal in oceans (Karner et al., 2001; Wuchter et al., 2006; Bayer et al., 2022) and nitrification is an important oxygen sink. There are many studies exploring the role of nitrification in oxygen consumption. A study in the Changjiang River plume indicated that oxygen demands of nitrification accounted for 0.32 to 318% of the community respiration rate (Hsiao et al., 2014). Studies based on other estuaries or fjords found that nitrification can constitute 20-30% and even up to 64% of the total oxygen consumption (Lipschultz et al., 1986; Berounsky and Nixon, 1993; Pakulski et al., 1995; Dai et al., 2008; Grundle and Juniper, 2011). A recent study supported that nitrification was universal in the ocean and was largely determined by its substrates (Tang et al., 2023). The negative effect of nutrients on DO in Aoshan Bay was in accordance with previous studies (Figure 4A). All these studies prove that nitrification plays a crucial role in oxygen depletion in coastal oceans, and the estimation of MCR based on AOU in the eutrophic coastal ocean was uncertain.
The change of DIN showed that substrates of nitrification, ammonium, and nitrite, were significantly higher in autumn than in other seasons (Figure 3A). A previous study indicated that the nitrification rate was positively correlated with ammonium concentration (Hsiao et al., 2014). This suggested that the nitrification rate would be higher in autumn than in other seasons. Results of MIC displayed that the proportion of ASVs associated with nitrite was the largest (Figure 4B), which further supported that nitrification played a pivotal role in Aoshan Bay. The relative abundance of nitrifiers, including Nitrospinales and Nitrospirales, was clearly higher in autumn than in other seasons (Figure 3B). There were little differences in Nitrosomonadaceae levels in the different seasons (Figure 3B). However, Nitrosococcales were higher in winter than in other seasons (Figure 3B). Nitrosococcales and Nitrosomonadaceae are AOB (Prosser et al., 2014; Ward et al., 2021), while Nitrospinales and Nitrospirales are NOB (Jing et al., 2022). Considering that the concentration of nitrite was higher than ammonium in winter (Figure 3B), although the relative abundance of AOB was highest in winter (Figure 3A), the nitrification rate was low. The same situation was true in spring. Nitrification is not as important as MCR in oxygen depletion in summer, since nitrifiers are less competitive than phytoplankton for ammonium and nitrite in this season (Hampel et al., 2018; Zakem et al., 2018). Nitrification was a more important oxygen sink in autumn than in other seasons in Aoshan Bay. This explains the difference between the in vivo INT reduction rate and oxygen consumption rate estimated by the optodes method in autumn. The oxygen consumption rate could not accurately reflect MCR because nitrification constituted a large proportion of total oxygen consumption in autumn.
As discussed above, nitrification contributes to biological oxygen demand and causes discrepancies in estimating MCR based on the apparent oxygen consumption rate in Aoshan Bay. Besides, reduced substrates, such as HS-, Fe2+, and methane, also contribute to oxygen demand (Friedrich et al., 2014; Fennel and Testa, 2019). It had been reported that the difference of MCR evaluated by AOU and in vivo INT could be used to estimate the activities of aerobic chemoautotrophy, which played an important role in maintaining the aphotic ocean system with carbon and energy scarcity (Li X et al., 2023). One of the potential ways to solve this problem is to estimate MCR and the concentration of key reduced elements (e.g., ammonium, nitrite, and sulfide) simultaneously. The dynamics of nutrients might be used to calibrate the MCR rate.
Whether the ocean is a net sink or a source of CO2 to the atmosphere is essential for understanding the role of the ocean in mitigating climate change. The balance between MCR and primary production strongly determines the air-sea CO2 flux, thus numerous researchers focus on MCR. The change of AOU was widely used to estimate MCR in coastal oceans that receive excessive nutrients from the land (Carol et al., 2023). Both aerobic respiration and chemoautotrophy are sensitive to substrate concentration (Kache et al., 2021). The results of this study support the idea that MCR based on AOU was overestimated, especially in eutrophic coastal oceans. These areas are predominant for primary production and microbial respiration, accompanied by active chemoautotrophic processes. It has been reported that intense nitrification plays a vital role in oxygen consumption in estuarine areas (Lu et al., 2020). In other regions with particularly high nutrient concentrations, the rate of aerobic chemoautotrophic processes even exceeds that of respiration (Hsiao et al., 2014). Therefore, relying solely on AOU for estimating MCR may not be accurate enough. It could be inferred that the amount of atmospheric carbon absorbed by the ocean was underestimated in previous studies. It is better to measure MCR based on AOU and in vivo INT simultaneously to obtain more accurate values of MCR in eutrophic ocean zones. It is necessary to update the database of MCR, especially those measured based on AOU in coastal areas and continental shelf sea.
4.2 Keystone groups significantly associated with MCR
As discussed above, the MCR assessed by AOU was not always accurate, thus values estimated by INT were chosen for performing further analysis. Microbial community compositions changed with the change in seasons (Gilbert et al., 2012; Hu et al., 2023) and the results of NMDS were consistent with this view (Supplementary Figure5). It can be inferred that the species significantly associated with MCR were different according to the seasons. MIC showed that large proportions of ASVs (Figure 4B) were significantly correlated with INT and were used to estimate MCR. Considering the complex interactions among different species, they were first separated into modules. The result of the mantel test indicated that only module 1 had a significant correlation with the in vivo INT reduction rate. The relative abundance of module 1 was less than 20% from January to April, whereas it was more than 25% in other months. The pattern of bacterial groups associated with year-round MCR is distinct from season-specific MCR (Figure 5B). These keystone groups could roughly be divided into four categories.
The first clade was associated with products of photoautotrophs. Pedosphaerales have been reported to be the rhizosphere microbiota of many plants (Walters et al., 2018; Yurgel et al., 2018), and their relative abundance was higher from May to September than in any other months. In the study field, green tide caused by the macroalgae Ulva prolifera has occurred yearly since 2007 and started in May (Cao et al., 2019). Pedosphaerales, tightly associated with eukaryotic hosts, rapidly responded to the green tide and played an important role in transforming the large amount of organic matter produced by the green tide. Thus, it was a key group in determining MCR. It has been reported that OM190 was tightly associated with macroalgae (Bengtsson and Øvreås, 2010; Bondoso et al., 2017) and the relative abundance of Thiomicrospirales strongly correlated with the diatom abundance (Liu et al., 2019). A study in the estuary found that Bacillales was a major β-glucosidase producer and this enzyme was fundamental in mediating the degradation of carbohydrates (Eswaran and Khandeparker, 2019). A further study indicated that Bacillales accounted for the highest proportions of microbial communities in the seagrass meadows (Jiang et al., 2015).
The advantage or characteristic of the second clade was that they contained genes involved in degrading complex compounds that were recalcitrant to other microorganisms. A study indicated that species involved in Kiritimatiellales are enriched in sulfatases and play an important role in degrading highly sulfate polysaccharides (Van Vliet et al., 2019). It was reported that groups involved in the bacterial order Sphingomonadales have the ability to degrade various hydrocarbons, including a wide range of aromatic compounds (Kertesz et al., 2019). In general, aromatic compounds are not optimal substrates and accumulate in the field (Medeiros et al., 2015) and incubation experiments (Zheng et al., 2019). The capacity to utilize aromatic compounds provides Sphingomonadales more chances to survive. Species in the order Chitinophagales can degrade complex carbohydrates including chitin (Hou et al., 2021). Although many bacteria accumulate intracellular glycogen as an energy source, only a few bacteria are able to utilize exogenous glycogen. It has been reported that members involved in the order Balneolales encoded the genes for pullulanase, which facilitated the depolymerization and exogenous utilization of glycogen (Ataeian et al., 2022).
The third clade included groups that were able to get energy, all or part of it, from light. Synechococcales are an important primary producer (Singh and Bhadury, 2018). The common characteristic of Rhodospirillales is that they contain photosynthetic pigments and fill a phototrophic niche (King et al., 2010; Pfennig and Trüper, 2019). Recent studies found that groups affiliated with Ectothiorhodospirales are purple sulfur bacteria (Oren, 2014) that are capable of photosynthesis. The high activity and in situ cell numbers of Actinomarinales in coastal sands suggested it was a keystone heterotroph for carbon mineralization (Miksch et al., 2021). Another study further suggested that Actinomarinales might be photoheterotrophs with rhodopsin and heliorhodopsin (López-Pérez et al., 2020). A previous study indicated that the SAR324 clade displayed plasticity energy-related metabolic pathways and was presumed to be photoheterotrophy (Boeuf et al., 2021). Proteorhodopsin-based phototrophy might constitute an important source of energy and cause deviation of measured MCR following assumed organic carbon remineralization between day and night (Munson-McGee et al., 2022). Microbial community composition should be fully taken into account and this might be an effective way to assess variations caused by variables among lineages.
Groups affiliated to the fourth clade were dominated by predators and organisms at higher trophic levels. The order Micavibrionales, assigned to the Bdellovibrio and like organisms (BALOs), is obligate predatory bacteria (Davidov et al., 2006). A recent study demonstrated that the abundance of Micavibrionales was likely linked to phytoplankton dynamics (Ezzedine et al., 2020). Similarly, Bdellovibrionales is also an important predatory bacteria (Beck et al., 2005), and a study about BALOs indicated that the rates of assimilating carbon of predatory bacteria were 211% higher than that of non-predatory bacteria (Hungate et al., 2021). Little is known about the bacterial order Haliangiales. It is involved in Myxococcota that feed on the other microbes they encounter (Whitworth et al., 2021).
All species discussed above were positively associated with MCR, while there were three species, namely, Nitrosococcales, Burkholderiales, and Lactobacillales, that demonstrated a negative correlation with MCR throughout the year (Figure 5B), partly because they were strongly negatively associated with MCR in special seasons. Nitrosococcales are an important ammonia-oxidizing bacteria (Ward et al., 2021) and were positively associated with MCR in autumn (Figure 5B). This further supports that nitrifiers play a vital role in the cycle of elements in autumn. However, this group showed a negative correlation with MCR especially in summer, when photoautotrophs dominated the ecosystem. Burkholderiales and Lactobacillales showing the highest negative correlations to MCR in winter might be caused by the low temperature (De Vrieze et al., 2015; Levy-Booth et al., 2021). It had been reported that blooms of Marine_group_II coincided with decreases in Chl a in the Santa Barbara Channel (Murray et al., 1999). This partly explained the significant negative correlation between Marine_group_II and MCR in summer. Thiomicrospirales are important sulfur-oxidizing bacteria (Lecoeuvre et al., 2021) and significantly negatively correlated to MCR in summer when photoautotrophic microorganisms dominated the food web.
Although some keystone groups were rare, previous studies documented that rare microorganisms played a pivotal role in many biogeochemical processes (Banerjee et al., 2018). Overall, the common characteristic of these crucial groups that strongly impacted MCR is the capacity to effectively get energy and utilize various substrates. The total amount of energy is conserved according to the first law of thermodynamics. Under this situation, the stronger capability of getting more energy is beneficial to their survival.
5 Conclusion
Although it is a common view that MCR plays a fundamental role in shaping the oceanic carbon cycle, few studies have been performed on a seasonal cycle. Activities of microorganisms are strongly associated with the season, however, a single study on MCR cannot reflect the impacts of environmental parameters along with seasons on MCR. To better understand the roles of the ocean in the global carbon cycle, we measured MCR throughout the whole year based on AOU and ETS in Aoshan Bay. The seasonal trends of MCR based on AOU and ETS were not consistent (Figure 6). The MCR peaked in summer based on ETS, while it peaked in autumn based on AOU. Further analysis proved that nitrification caused the overestimation of MCR based on AOU. Although previous studies supported that nitrification was an important oxygen sink, they did not explicitly illustrate the effects of nitrification on MCR estimation based on AOU. This study proved that the overestimation of MCR was caused by nitrification based on AOU, which might affect the estimation of the flow of carbon through marine plankton food webs. To calibrate MCR, it is better to monitor the dynamics of reduced nutrients when using AOU. Based on the results of the in vivo INT reduction rate, groups strongly associated with MCR were identified. These keystone groups were capable of utilizing various substrates or getting energy directly from light. Caution is necessary when assuming organic carbon remineralization based on MCR inferred from ETS, and, in future research, attention should be paid to the deviation caused by photoheterotrophy.
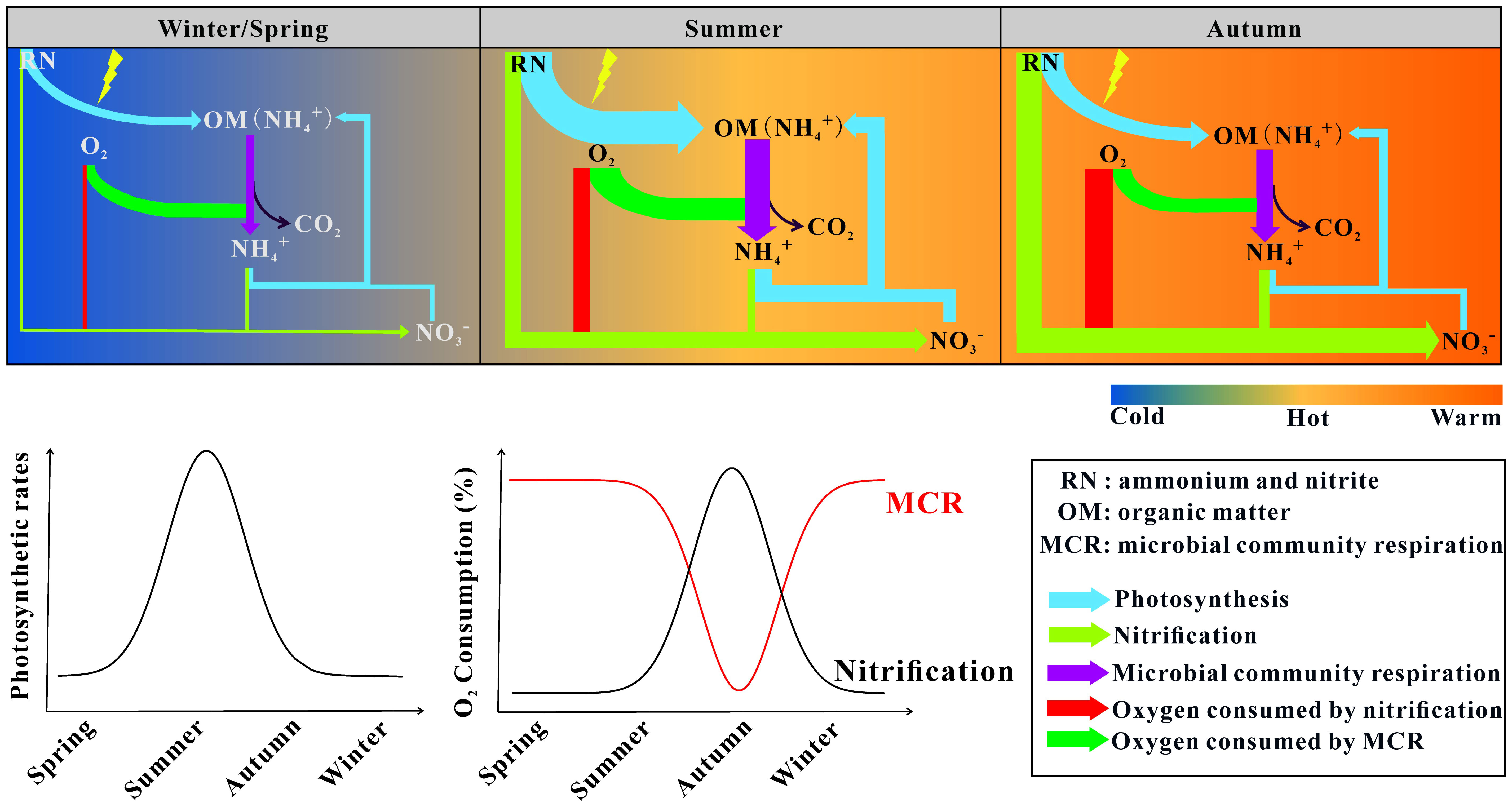
Figure 6 | Major biogeochemical processes involved in oxygen consumption. The width of the arrows represents the flux of nitrogen and oxygen.
Data availability statement
The raw data supporting the conclusions of this article will be made available by the authors, without undue reservation.
Author contributions
LZ: Formal Analysis, Writing – original draft. WZ: Writing – review & editing. YW: Writing – review & editing. YL: Writing – review & editing. JC: Writing – review & editing. BL: Writing – review & editing. BS: Writing – review & editing. HS: Methodology, Writing – review & editing.
Funding
The author(s) declare financial support was received for the research, authorship, and/or publication of this article. This work was funded by Southern Marine Science and Engineering Guangdong Laboratory (Zhuhai) (SML2020SP004), the Key Research and Development Program of Shandong Province (2020ZLYS04), the National Key Research and Development Program of China (2018YFA0605800), the National Natural Science Foundation of China (42188102), and the Opening Foundation of Fujian Key Laboratory of Marine Carbon Sequestration Research Fund, Xiamen University (FKLMCS2023002).
Acknowledgments
We sincerely thank TingYat Lee for his assistance in editing the original manuscript. We also thank Xiao Chen, Hongwei Ren, and Xianrui Song for their support during this experiment.
Conflict of interest
Authors WZ and BL were employed by the company Center Tech Tianjin Chemical Research and Design Institute Co., Ltd.
The remaining authors declare that the research was conducted in the absence of any commercial or financial relationships that could be construed as a potential conflict of interest.
Publisher’s note
All claims expressed in this article are solely those of the authors and do not necessarily represent those of their affiliated organizations, or those of the publisher, the editors and the reviewers. Any product that may be evaluated in this article, or claim that may be made by its manufacturer, is not guaranteed or endorsed by the publisher.
Supplementary material
The Supplementary Material for this article can be found online at: https://www.frontiersin.org/articles/10.3389/fmars.2023.1331680/full#supplementary-material.
References
Alonso-Sáez L., Gasol J. M., Arístegui J., Vilas J. C., Vaqué D., Duarte C. M., et al. (2007). Large-scale variability in surface bacterial carbon demand and growth efficiency in the subtropical northeast Atlantic Ocean. Limnol. Oceanogr. 52 (2), 533–546. doi: 10.4319/lo.2007.52.2.0533
Alonso-Sáez L., Vázquez-Domínguez E., Cardelus C., Pinhassi J., Sala M. M., Lekunberri I., et al. (2008). Factors controlling the year-round variability in carbon flux through bacteria in a coastal marine system. Ecosystems 11 (3), 397–409. doi: 10.1007/s10021-008-9129-0
Apple J. K., Del Giorgio P., Michael Kemp W. (2006). Temperature regulation of bacterial production, respiration, and growth efficiency in a temperate salt-marsh estuary. Aquat. microbial ecologyaquat Microb. Ecol. . 43 (3), 243–254. doi: 10.3354/AME043243
Aranguren-Gassis M., Teira E., Serret P., Martínez-García S., Fernández E. (2012). Potential overestimation of bacterial respiration rates in oligotrophic plankton communities. Mar. Ecol. Prog. Ser. 453, 1–10. doi: 10.3354/meps09707
Ataeian M., Liu Y., Kouris A., Hawley A. K., Strous M. (2022). Ecological interactions of cyanobacteria and heterotrophs enhances the robustness of cyanobacterial consortium for carbon sequestration. Front. Microbiol. 13. doi: 10.3389/fmicb.2022.780346
Banerjee S., Schlaeppi K., van der Heijden M. (2018). Keystone taxa as drivers of microbiome structure and functioning. Nat. Rev. Microbiol. 16 (9), 567–576. doi: 10.1038/s41579-018-0024-1
Bayer B., McBeain K., Carlson C., Santoro A. (2022). Carbon content, carbon fixation yield and dissolved organic carbon release from diverse marine nitrifiers. Limnology Oceanography. 68, 84-96. doi: 10.1002/lno.12252
Beck S., Schwudke D., Appel B., Linscheid M., Strauch E. (2005). Characterization of outer membrane protein fractions of Bdellovibrionales. FEMS Microbiol. Letters. 243 (1), 211–217. doi: 10.1016/j.femsle.2004.12.006
Bengtsson M. M., Øvreås L. (2010). Planctomycetes dominate biofilms on surfaces of the kelp Laminaria hyperborea. BMC Microbiol. 10 (1), 1–12. doi: 10.1186/1471-2180-10-261
Berounsky V. M., Nixon S. W. (1993). Rates of nitrification along an estuarine gradient in Narragansett Bay. Estuaries Coasts. 16 (4), 718–730. doi: 10.2307/1352430
Boeuf D., Eppley J. M., Mende D. R., Malmstrom R. R., Woyke T., DeLong E. F. (2021). Metapangenomics reveals depth-dependent shifts in metabolic potential for the ubiquitous marine bacterial SAR324 lineage. Microbiome. 9 (1), 1–18. doi: 10.1186/s40168-021-01119-5
Bondoso J., Godoy-Vitorino F., Balague V., Gasol J. M., Harder J., Lage O. M. (2017). Epiphytic Planctomycetes communities associated with three main groups of macroalgae. FEMS Microbiol. Ecology. 93 (3), fiw255. doi: 10.1093/femsec/fiw255
Borges A. V., Delille B., Frankignoulle M. (2005). Budgeting sinks and sources of CO2 in the coastal ocean: Diversity of ecosystems counts. Geophysical Res. Letters. 32 (14). doi: 10.1029/2005GL023053
Cao Y., Wu Y., Fang Z., Cui X., Liang J., Song X. (2019). Spatiotemporal patterns and morphological characteristics of Ulva prolifera distribution in the Yellow Sea, China in 2016–2018. Remote Sensing. 11 (4), 445. doi: 10.3390/rs11040445
Carla F. B., Denis P., Lucía, Ricardo I., Valeria S., Rubén M., et al. (2022). Physical and biological effects on the carbonate system during summer in the Northern Argentine Continental Shelf (Southwestern Atlantic). J. Mar. Syst. 237, 103828. doi: 10.1016/j.jmarsys.2022.103828
Carol E., Galliari M. J., Santucci L., Nuñez F., Faleschini M. (2023). Assessment of groundwater-driven dissolved nutrient inputs to coastal wetlands associated with marsh-coastal lagoons systems of the littoral of the outer Río de la Plata estuary. Sci. Total Environ. 885, 163942. doi: 10.1016/j.scitotenv.2023.163942
Carpenter J. H. (1965). The Chesapeake Bay Institute technique for the Winkler dissolved oxygen method. Limnology Oceanography. 10 (1), 141–143. doi: 10.4319/lo.1965.10.1.0141
Chin M., Lau S., Midot F., Jee M., Lo M., Sangok F., et al. (2023). Root exclusion method for separating soil respiration components: Review and methodological considerations. Pedosphere. 33, 683–699. doi: 10.1016/j.pedsph.2023.01.015
Clauset A., Newman M. E., Moore C. J. (2004). Finding community structure in very large networks. Phys. Rev. E .70 (6), 66111. doi: 10.1103/PhysRevE.70.066111
Cleveland C. C., Nemergut D. R., Schmidt S. K., Townsend A. R. (2007). Increases in soil respiration following labile carbon additions linked to rapid shifts in soil microbial community composition. Biogeochemistry. 82 (3), 229–240. doi: 10.1007/s10533-006-9065-z
Crockford P., Halevy I., Milo R., Bar On Y., Ward L. M. (2023). The geologic history of primary productivity. Curr. Biol. 33, 4741-4750. doi: 10.1016/j.cub.2023.09.040
Dai M., Wang L., Guo X., Zhai W., Li Q., He B., et al. (2008). Nitrification and inorganic nitrogen distribution in a large perturbed river/estuarine system: the Pearl River Estuary, China. Biogeosciences .5 (5), 1227–1244. doi: 10.5194/bg-5-1227-2008
Davidov Y., Huchon D., Koval S. F., Jurkevitch E. (2006). A new α-proteobacterial clade of Bdellovibrio-like predators: implications for the mitochondrial endosymbiotic theory. Environ. Microbiol. 8 (12), 2179–2188. doi: 10.1111/j.1462-2920.2006.01101.x
De Vrieze J., Saunders A. M., He Y., Fang J., Nielsen P. H., Verstraete W., et al. (2015). Ammonia and temperature determine potential clustering in the anaerobic digestion microbiome. Water Res. 75, 312–323. doi: 10.1016/j.watres.2015.02.025
Del Giorgio P., Williams P. (2005). Respiration in aquatic ecosystems. OUP Oxford. 1, 1–17. doi: 10.1093/acprof:oso/9780198527084.003.0001
Dittmar T., Lennartz S. T., Buck-Wiese H., Hansell D., Santinelli C., Vanniet C., et al. (2021). Enigmatic persistence of dissolved organic matter in the ocean. Nat. Rev. Earth Environ. 2, 570–583. doi: 10.1038/s43017-021-00183-7
Eswaran R., Khandeparker L. (2019). Seasonal variation in β-glucosidase-producing culturable bacterial diversity in a monsoon-influenced tropical estuary. Environ. Monit. Assessment. 191 (11), 1–11. doi: 10.1007/s10661-019-7818-0
Ezzedine J. A., Jacas L., Desdevises Y., Jacquet S. (2020). Bdellovibrio and like organisms in Lake Geneva: an unseen elephant in the room? Front. Microbiol. 11. doi: 10.3389/fmicb.2020.00098
Fennel K., Testa J. M. (2019). Biogeochemical controls on coastal hypoxia. Annu. Rev. Mar. Sci. 11 (1), 105–130. doi: 10.1146/annurev-marine-010318-095138
Friedlingstein P., Jones M. W., O'Sullivan M., Andrew R. M., Bakker D. C., Hauck J., et al. (2022). Global carbon budget 2021. Earth System Sci. Data. 14 (4), 1917–2005. doi: 10.5194/essd-14-1917-2022
Friedrich J., Janssen F., Aleynik D., Bange H. W., Boltacheva N., Çagatay M., et al. (2014). Investigating hypoxia in aquatic environments: diverse approaches to addressing a complex phenomenon. Biogeosciences. 11 (4), 1215–1259. doi: 10.5194/bg-11-1215-2014
Gao X., Chen H., Govaert L., Wang W., Yang J. (2019). Responses of zooplankton body size and community trophic structure to temperature change in a subtropical reservoir. Ecol. Evolution. 9 (22), 12544–12555. doi: 10.1002/ece3.5718
García F. C., Clegg T., O’Neill D. B., Warfield R., Pawar S., Yvon-Durocher G. (2023). The temperature dependence of microbial community respiration is amplified by changes in species interactions. Nat. Microbiol. 8 (2), 272–283. doi: 10.1038/s41564-022-01283-w
García-Martín E. E., Daniels C. J., Davidson K., Lozano J., Mayers K. M., McNeill S., et al. (2019). Plankton community respiration and bacterial metabolism in a North Atlantic Shelf Sea during spring bloom development (April 2015). Prog. In Oceanography. 177, 101873. doi: 10.1016/j.pocean.2017.11.002
Gattuso J.-P., Frankignoulle M., Wollast R. (1998). Carbon and carbonate metabolism in coastal aquatic ecosystems. Annu. Rev. Ecol. Systematics. 29, 405–434. doi: 10.1146/annurev.ecolsys.29.1.405
Gilbert J. A., Steele J. A., Caporaso J. G., Steinbrück L., Reeder J., Temperton B., et al. (2012). Defining seasonal marine microbial community dynamics. ISME J. 6 (2), 298–308. doi: 10.1038/ismej.2011.107
Gruber N. J. N. (2015). Carbon at the coastal interface. 517(7533). Nature. 517, 148–149. doi: 10.1038/nature14082
Grundle D. S., Juniper S. K. (2011). Nitrification from the lower euphotic zone to the sub-oxic waters of a highly productive British Columbia fjord. Mar. Chem. 126 (1-4), 173–181. doi: 10.1016/j.marchem.2011.06.001
Guo C., Ke Y., Chen B., Zhang S., Liu H. (2022). Making comparable measurements of bacterial respiration and production in the subtropical coastal waters. Mar. Life Sci. Technology. 4 (3), 414–427. doi: 10.1007/s42995-022-00133-2
Guo Y., Zhao Y., Zhu T., Li J., Feng Y., Zhao H., et al. (2018). A metabolomic view of how low nitrogen strength favors anammox biomass yield and nitrogen removal capability. Water Res. 143, 387–398. doi: 10.1016/j.watres.2018.06.052
Hampel J. J., McCarthy M. J., Gardner W. S., Zhang L., Xu H., Zhu G., et al. (2018). Nitrification and ammonium dynamics in Taihu Lake, China: seasonal competition for ammonium between nitrifiers and cyanobacteria. Biogeosciences. 15 (3), 733–748. doi: 10.5194/bg-15-733-2018
Hansell D. A., Carlson C. A. (2001). Biogeochemistry of total organic carbon and nitrogen in the Sargasso Sea: control by convective overturn. Deep Sea Res. Part II Topical Stud. Oceanography. 48 (8-9), 1649–1667. doi: 10.1016/S0967-0645(00)00153-3
Hansell D. A., Ducklow H. W., Macdonald A. M., O-Neil Baringer M. (2004). Metabolic poise in the North Atlantic Ocean diagnosed from organic matter transports. Limnology Oceanography. 49 (4), 1084–1094. doi: 10.4319/lo.2004.49.4.1084
Hansen A. M., Kraus T. E., Pellerin B. A., Fleck J. A., Downing B. D., Bergamaschi B. A. (2016). Optical properties of dissolved organic matter (DOM): Effects of biological and photolytic degradation. Limnology Oceanography. 61 (3), 1015–1032. doi: 10.1002/lno.10270
Hernández-León S., Koppelmann R., Fraile-Nuez E., et al. (2020). Large deep-sea zooplankton biomass mirrors primary production in the global ocean. Nat. Communication. 11, 6048. doi: 10.1038/s41467-020-19875-7
Holden P. B., Edwards N. R., Ridgwell A., Wilkinson R., Fraedrich K., Lunkeit F., et al. (2018). Climate–carbon cycle uncertainties and the Paris Agreement. Nat. Climate Change. 8 (7), 609–613. doi: 10.1038/s41558-018-0197-7
Hou Y., Li B., Feng G., Zhang C., He J., Li H., et al. (2021). Responses of bacterial communities and organic matter degradation in surface sediment to Macrobrachium nipponense bioturbation. Sci. Total Environment. 759, 143534. doi: 10.1016/j.scitotenv.2020.143534
Hsiao S.-Y., Hsu T.-C., Liu J.-w., Xie X., Zhang Y., Lin J., et al. (2014). Nitrification and its oxygen consumption along the turbid Chang Jiang River plume. Biogeosciences .11 (7), 2083–2098. doi: 10.5194/bg-11-2083-2014
Hu W., Zheng N., Zhang Y., Bartlam M., Wang Y. (2023). Spatiotemporal dynamics of high and low nucleic acid-content bacterial communities in Chinese coastal seawater: assembly process, co-occurrence relationship and the ecological functions. Front. Microbiol. 14. doi: 10.3389/fmicb.2023.1219655
Hungate B. A., Marks J. C., Power M. E., Schwartz E., van Groenigen K. J., Blazewicz S. J., et al. (2021). The functional significance of bacterial predators. mBio. 12 (2), e00466–e00421. doi: 10.1128/mBio.00466-21
Jeffrey S., Humphrey G. (1975). New spectrophotometric equations for determining chlorophylls a, b, c1 and c2 in higher plants, algae and natural phytoplankton. Biochem. Physiol. Pflanz 167, 191–194. doi: 10.1016/S0015-3796(17)30778-3
Jiang Y. F., Ling J., Wang Y. S., Chen B., Zhang Y. Y., Dong J. D. (2015). Cultivation-dependent analysis of the microbial diversity associated with the seagrass meadows in Xincun Bay, South China Sea. Ecotoxicology. 24 (7), 1540–1547. doi: 10.1007/s10646-015-1519-4
Jiao N., Liu J., Edwards B., Lv Z., Cai R., Liu Y., et al. (2021). Correcting a major error in assessing organic carbon pollution in natural waters. Sci. Advances. 7 (16), eabc7318. doi: 10.1126/sciadv.abc7318
Jing H., Xiao X., Zhang Y., Li Z., Jian H., Luo Y., et al. (2022). Composition and ecological roles of the core microbiome along the abyssal-hadal transition zone sediments of the mariana trench. Microbiol. Spectrum. 10 (3). doi: 10.1128/spectrum.01988-21
Kache S., Bartl I., Wäge-Recchioni J., Voss M. (2021). Influence of organic particle addition on nitrification rates and ammonium oxidiser abundances in Baltic seawater. Mar. Ecol. Prog. Ser. 674, 59–72. doi: 10.3354/meps13797
Karner M. B., DeLong E. F., Karl D. M. (2001). Archaeal dominance in the mesopelagic zone of the Pacific Ocean. Nature. 409 (6819), 507. doi: 10.1038/35054051
Kertesz M. A., Kawasaki A., Stolz A. (2019). Aerobic hydrocarbon-degrading alphaproteobacteria: Sphingomonadales. Taxonomy Genomics Ecophysiology Hydrocarbon-Degrading Microbe. chapter 4, 105–124. doi: 10.1007/978-3-030-14796-9_9
King A. J., Freeman K. R., McCormick K. F., Lynch R. C., Lozupone C., Knight R., et al. (2010). Biogeography and habitat modelling of high-alpine bacteria. Nat. Commun. 1 (1), 1–6. doi: 10.1038/ncomms1055
Kulk G., Platt T., Dingle J., Jackson T., Jönsson B. F., Bouman H. A., et al. (2020). Primary production, an index of climate change in the ocean: satellite-based estimates over two decades. Remote Sens. 12 5, 826. doi: 10.3390/rs12050826
Laruelle G. G., Dürr H. H., Slomp C. P., Borges A. (2010). Evaluation of sinks and sources of CO2 in the global coastal ocean using a spatially-explicit typology of estuaries and continental shelves. Geophysical Res. Letters. 37 (15), L0567. doi: 10.1029/2010GL043691
Laruelle G. G., Lauerwald R., Pfeil B., Regnier P. (2014). Regionalized global budget of the CO2 exchange at the air-water interface in continental shelf seas. Global Biogeochemical Cycles. 28 (11), 1199–1214. doi: 10.1002/2014GB004832
Lawaetz A. J., Stedmon C. A. (2009). Fluorescence intensity calibration using the Raman scatter peak of water. Appl. Spectroscopy. 63 (8), 936–940. doi: 10.1366/000370209788964548
Lecoeuvre A., Ménez B., Cannat M., Chavagnac V., Gérard E. J. (2021). Microbial ecology of the newly discovered serpentinite-hosted Old City hydrothermal field (southwest Indian ridge). ISME J. 15 (3), 818–832. doi: 10.1038/s41396-020-00816-7
Levy-Booth D. J., Hashimi A., Roccor R., Liu L.-Y., Renneckar S., Eltis L. D., et al. (2021). Genomics and metatranscriptomics of biogeochemical cycling and degradation of lignin-derived aromatic compounds in thermal swamp sediment. ISME J. 15 (3), 879–893. doi: 10.1038/s41396-020-00820-x
Li X., Zhao X., Dang H., Zhang C., Fernández-Urruzola I., Liu Z., et al. (2023). High variability in organic carbon sources and microbial activities in the hadopelagic waters. Limnology Oceanography 68, 1704–1718. doi: 10.1002/lno.12379
Lipschultz F., Wofsy S. C., Fox L. E. (1986). Nitrogen metabolism of the eutrophic Delaware River ecosystem. Limnology Oceanography. 31 (4), 701–716. doi: 10.4319/lo.1986.31.4.0701
Liu Y., Debeljak P., Rembauville M., Blain S., Obernosterer I. (2019). Diatoms shape the biogeography of heterotrophic prokaryotes in early spring in the Southern Ocean. Environ. Microbiol. 21 (4), 1452–1465. doi: 10.1111/1462-2920.14579
Logares R., Deutschmann I. M., Junger P. C., Giner C. R., Krabberød A. K., Schmidt T. S., et al. (2020). Disentangling the mechanisms shaping the surface ocean microbiota. Microbiome. 8 (1), 1–17. doi: 10.1186/s40168-020-00827-8
López-Pérez M., Haro-Moreno J. M., Iranzo J., Rodriguez-Valera F. (2020). Genomes of the “Candidatus Actinomarinales” order: highly streamlined marine epipelagic actinobacteria. mSystems. 5 (6), e01041–e01020. doi: 10.1128/mSystems.01041-20
Love C., Arrington E., Gosselin K., Reddy C., Mooy B., Nelson R., et al. (2021). Microbial production and consumption of hydrocarbons in the global ocean. Nat. Microbiol. 6, 489–498. doi: 10.1038/s41564-020-00859-8
Lu Y., Cheung S., Chen L., Kao S.-J., Xia X., Gan J., et al. (2020). New insight to niche partitioning and ecological function of ammonia oxidizing archaea in subtropical estuarine ecosystem. Biogeosciences 17, 6017–6032. doi: 10.5194/bg-17-6017-2020,2020
Lucea A., Duarte C. M., Agustí S., Kennedy H. (2005). Nutrient dynamics and ecosystem metabolism in the Bay of Blanes (NW Mediterranean). Biogeochemistry. 73 (2), 303–323. doi: 10.1007/s10533-004-0059-4
Marie D., Partensky F., Jacquet S., Vaulot D. (1997). Enumeration and cell cycle analysis of natural populations of marine picoplankton by flow cytometry using the nucleic acid stain SYBR Green I. Appl. Environ. Microbiol. 63 (1), 186–193. doi: 10.1128/AEM.63.1.186-193.1997
Martínez-García S., Fernández E., Aranguren-Gassis M., Teira E. (2009). In vivo electron transport system activity: a method to estimate respiration in natural marine microbial planktonic communities. Limnology Oceanography Methods 7 (6), 459–469. doi: 10.4319/lom.2009.7.459
Martínez-García S., Fernández E., del Valle D. A., Karl D. M., Teira E. (2013). Experimental assessment of marine bacterial respiration. Aquat. Microbial Ecology. 70 (3), 189–205. doi: 10.3354/ame01644
Medeiros P. M., Seidel M., Powers L. C., Dittmar T., Hansell D. A., Miller W. L. (2015). Dissolved organic matter composition and photochemical transformations in the northern North Pacific Ocean. Geophysical Res. Letters. 42 (3), 863–870. doi: 10.1002/2014GL062663
Miksch S., Meiners M., Meyerdierks A., Probandt D., Wegener G., Titschack J., et al. (2021). Bacterial communities in temperate and polar coastal sands are seasonally stable. ISME Commun. 1 (1), 1–11. doi: 10.1038/s43705-021-00028-w
Munson-McGee J. H., Lindsay M. R., Sintes E., Brown J. M., D’Angelo T., Brown J., et al. (2022). Decoupling of respiration rates and abundance in marine prokaryoplankton. Nature. 612, 1–7. doi: 10.1038/s41586-022-05505-3
Murphy K. R., Stedmon C. A., Wenig P., Bro R. (2014). OpenFluor–an online spectral library of auto-fluorescence by organic compounds in the environment. Analytical Methods 6 (3), 658–661. doi: 10.1039/c3ay41935e
Murray A., Blakis A., Massana R., Strawzewski S., Passow U., Alldredge A., et al. (1999). A time series assessment of planktonic archaeal variability in the Santa Barbara Channel. Aquat. Microbial Ecology. 20 (2), 129–145. doi: 10.3354/ame020129
Oren A. J. T. P. (2014). The family ectothiorhodospiraceae. Prokaryotes. chapter 9, 199–222. doi: 10.1007/978-3-642-38922-1_248
Pakulski J. D., Benner R., Amon R., Eadie B., Whitledge T. (1995). Community metabolism and nutrient cycling in the Mississippi River plume: evidence for intense nitrification at intermediate salinities. Mar. Ecol. Prog. Ser. 117, 207–218. doi: 10.3354/meps117207
Pfennig N., Trüper H. G. (2019). The rhodospirillales (Phototrophic or photosynthetic bacteria). Handb. Microbiol. chapter 3, 14-24. doi: 10.1201/9781351072939
Prosser J. I., Head I. M., Stein L. Y. (2014). ). The family nitrosomonadaceae in The Prokaryotes: Alphaproteobacteria and Betaproteobacteria. Springer Berlin/Heidelberg. chapter 8, 901–918. doi: 10.1007/978-3-642-30197-1_372
Reinthaler T., Herndl G. (2005). Seasonal dynamics of bacterial growth efficiencies in relation to phytoplankton in the southern North Sea. Environ. Sci. .39 (1), 7–16. doi: 10.3354/AME039007
Reshef D. N., Reshef Y. A., Finucane H. K., Grossman S. R., McVean G., Turnbaugh P. J., et al. (2011). Detecting novel associations in large data sets. Science. 334 (6062), 1518–1524. doi: 10.1126/science.120543
Singh T., Bhadury P. (2018). Distribution patterns of marine planktonic cyanobacterial assemblages in transitional marine habitats using 16S rRNA phylogeny. Phycological Res. 66 (3), 189–198. doi: 10.1111/pre.12224
Smith T., Clegg T., Bell T., Pawar S. (2021). Systematic variation in the temperature dependence of bacterial carbon use efficiency. Ecol. Letters. 24. 2123-2133. doi: 10.1111/ele.13840
Tanaka K., Kuma K., Hamasaki K., Yamashita Y. (2014). Accumulation of humic-like fluorescent dissolved organic matter in the Japan Sea. Sci. Rep. 4 (1), 1–7. doi: 10.1038/srep05292
Tang W., Ward B., Beman M., Bristow L., Clark D., Fawcett S. (2023). Database of nitrification and nitrifiers in the global ocean. Earth System Sci. Data Discussions. 2023, 1–66. doi: 10.5194/essd-2023-194
Van Vliet D. M., Palakawong Na Ayudthaya S., Diop S., Villanueva L., Stams A. J., Sánchez-Andrea I. (2019). Anaerobic degradation of sulfated polysaccharides by two novel Kiritimatiellales strains isolated from Black Sea sediment. Front. Microbiol. 10. doi: 10.3389/fmicb.2019.00253
Walters W. A., Jin Z., Youngblut N., Wallace J. G., Sutter J., Zhang W., et al. (2018). Large-scale replicated field study of maize rhizosphere identifies heritable microbes. Proc. Natl. Acad. Sci. 115 (28), 7368–7373. doi: 10.1073/pnas.1800918115
Wang L. (2018). Corrigendum to Microbial control of carbon cycle in the ocean. Natl. Sci. Review. 5, 442. doi: 10.1093/nsr/nwy047
Ward L., Johnston D., Shih P. (2021). Phanerozoic radiation of ammonia oxidizing bacteria. Sci. Rep. 11 (1), 1–9. doi: 10.1038/s41598-021-81718-2
Warkentin M., Freese H. M., Karsten U., Schumann R. (2007). New and fast method to quantify respiration rates of bacterial and plankton communities in freshwater ecosystems by using optical oxygen sensor spots. Appl. Environ. Microbiol. 73 (21), 6722–6729. doi: 10.1128/AEM.00405-07
Wellburn A. R. (1994). The spectral determination of chlorophylls a and b, as well as total carotenoids, using various solvents with spectropho-tometers of different resolution. J. Plant Physiol. 144, 307–313. doi: 10.1016/S0176-1617(11)81192-2
Whitworth D. E., Sydney N., Radford E. (2021). Myxobacterial genomics and post-genomics: A review of genome biology, genome sequences and related 'Omics studies. Microorganisms . 9 (10), 2143. doi: 10.3390/microorganisms9102143
Wuchter C., Abbas B., Coolen M. J., Herfort L., van Bleijswijk J., Timmers P., et al. (2006). Archaeal nitrification in the ocean. Proc. Natl. Acad. Sci. 103 (33), 12317–12322. doi: 10.1073/pnas.0600756103
Yurgel S. N., Douglas G. M., Dusault A., Percival D., Langille M. (2018). Dissecting community structure in wild blueberry root and soil microbiome. Front. Microbiol. 9. doi: 10.3389/fmicb.2018.01187
Zakem E. J., Al-Haj A., Church M. J., van Dijken G. L., Dutkiewicz S., Foster S. Q., et al. (2018). Ecological control of nitrite in the upper ocean. Nat. Commun. 9 (1), 1–13. doi: 10.1038/s41467-018-03553-w
Zhang L., Chen M., Chen X., Wang J., Zhang Y., Xiao X., et al. (2021). Nitrifiers drive successions of particulate organic matter and microbial community composition in a starved macrocosm. Environ. Int. 157, 106776. doi: 10.1016/j.envint.2021.106776
Keywords: microbial community respiration, apparent oxygen utilization, electron transport system assay, photoheterotrophy, coastal ocean
Citation: Zhang L, Zhou W, Wang Y, Liu Y, Chen J, Li B, Su B and Song H (2024) Overestimation of microbial community respiration caused by nitrification, and the identification of keystone groups associated with respiration. Front. Mar. Sci. 10:1331680. doi: 10.3389/fmars.2023.1331680
Received: 01 November 2023; Accepted: 28 December 2023;
Published: 29 January 2024.
Edited by:
Junfu Dong, Shandong University, ChinaReviewed by:
Zhuo-Yi Zhu, Shanghai Jiao Tong University, ChinaXiangbin Ran, Ministry of Natural Resources, China
Hanzhi Lin, Science Systems and Applications, Inc., United States
Copyright © 2024 Zhang, Zhou, Wang, Liu, Chen, Li, Su and Song. This is an open-access article distributed under the terms of the Creative Commons Attribution License (CC BY). The use, distribution or reproduction in other forums is permitted, provided the original author(s) and the copyright owner(s) are credited and that the original publication in this journal is cited, in accordance with accepted academic practice. No use, distribution or reproduction is permitted which does not comply with these terms.
*Correspondence: Hui Song, songhui2018@foxmail.com