Early Detection of Sepsis With Machine Learning Techniques: A Brief Clinical Perspective
- 1Infectious Diseases Unit, San Martino Policlinico Hospital – IRCCS for Oncology and Neurosciences, Genoa, Italy
- 2Department of Health Sciences (DISSAL), University of Genoa, Genoa, Italy
- 3Department of Informatics Bioengineering, Robotics, and Systems Engineering (DIBRIS), University of Genoa, Genoa, Italy
- 4Anaesthesia and Intensive Care, San Martino Policlinico Hospital – IRCCS for Oncology and Neurosciences, Genoa, Italy
- 5Department of Surgical Sciences and Integrated Diagnostics (DISC), University of Genoa, Genoa, Italy
Sepsis is a major cause of death worldwide. Over the past years, prediction of clinically relevant events through machine learning models has gained particular attention. In the present perspective, we provide a brief, clinician-oriented vision on the following relevant aspects concerning the use of machine learning predictive models for the early detection of sepsis in the daily practice: (i) the controversy of sepsis definition and its influence on the development of prediction models; (ii) the choice and availability of input features; (iii) the measure of the model performance, the output, and their usefulness in the clinical practice. The increasing involvement of artificial intelligence and machine learning in health care cannot be disregarded, despite important pitfalls that should be always carefully taken into consideration. In the long run, a rigorous multidisciplinary approach to enrich our understanding in the application of machine learning techniques for the early recognition of sepsis may show potential to augment medical decision-making when facing this heterogeneous and complex syndrome.
Introduction
Sepsis, defined as a life-threatening organ dysfunction caused by a dysregulated host response to infection, is a major cause of death worldwide (1–5).
Sepsis is a complex and evolving concept: (i) complex, because it involves two different actors (the infection and the host response) and their relative contribution to the organ damage may vary across patients and over time in the same patient (6, 7); (ii) evolving, because various definitions have been developed and adopted over the last years, reflecting the complexity of the elusive concept to be defined. Although the evolution of the concept of sepsis could reflect a positive trajectory aimed to provide more precise definitions for both clinical and research purposes, the long-lasting process of defining sepsis has led to the use of different terminology and definitions across research studies (8, 9). As a consequence, this has reduced the comparability of study results, and also hindered a smooth development of predictive models of sepsis. Nonetheless, the development of good predictive models of sepsis remains a timely topic, since it would help clinicians to readily identify patients at higher risk of (or likely to already have) sepsis, thereby allowing close monitoring and/or early treatment (thereby potentially reducing mortality) (10).
Prediction of sepsis through the use of machine learning models has gained particular attention over the past few years (11–17). In the present perspective, we provide a brief clinician-oriented vision on some relevant aspects concerning the use of machine learning predictive models for the prediction of sepsis in the daily practice. In particular, although predictive models may be developed for either prediction sensu stricto or early detection (i.e., as early diagnostic tools), we will mainly focus on the ability of these tools to early detect patients with sepsis.
Methods
On 25 July 2020, we performed a PubMed search using the following key words: “sepsis” AND “machine learning.” Reference lists and abstracts of the pre-screened 189 studies were manually checked, using an iterative approach, and selected for potential eligibility. Selected full texts and pertinent references were further reviewed, with the ultimate decision on inclusion being based upon authors' judgment. Of note, additional searches through PubMed and Google Scholar performed on 23 December 2020 led to the evaluation of 18 additional full texts for potential inclusion. The final text was structured in the following sections: (i) brief introduction on prediction of clinical events through machine learning models and the need for a multidisciplinary approach; (ii) the controversy of sepsis definition and its influence on the development of prediction models; (iii) the choice and availability of input features; (iv) the measure of the model performance, the output, and their usefulness for clinicians; (v) limitations and conclusions.
Brief Introduction on Prediction of Clinical Events Through Machine Learning Models and the Need for a Multidisciplinary Approach
Through machine learning algorithms, computers are conferred the ability to learn from data (18, 19). Conceptually, machine learning, which is a branch of artificial intelligence, is different from standard computer expert systems for helping clinicians in daily decision making (20). Indeed, the latter are explicitly programmed to perform a given task, whereas machine learning algorithms are more generally programmed to find out associations. For example, in supervised learning, an outcome (e.g., sepsis), is predicted through calculations starting from input features (e.g., patient demographic and clinical characteristics). Notably, the outcome and the input of machine learning algorithms may also represent the dependent and independent variables of classical statistical predictive models. Not surprisingly, there is an important conceptual overlap between classical statistics and machine learning techniques (21). A difference in their continuum may rely on the fact that some machine learning algorithms could be able to find out composite features not easy or impossible to be defined by humans. In turn, this may improve the accuracy of prediction (22). However, this also fuels the issue of the interpretability of the model, with computations that may become less transparent and not completely explainable once results are produced (23). In turn, this may hamper recognition of biases, thereby leading to additional ethical and legal implications connected to the use of machine learning techniques in healthcare (24–26).
In addition, it should be also taken into consideration that the field of machine learning is closely connected with the concept of “big data,” since, at least in general, the possibility of including complex features in prediction models requires far larger samples than those usually employed in classical prediction studies (22, 27). Therefore, the possible future availability of large datasets of medical data from electronic medical records (EMR), laboratory databases, and vital signs monitors will inevitably require a multidisciplinary approach. This would guarantee standardized extraction of data, data security, interpretability or sufficient explanation of employed machine learning models, extrapolation of useful outputs from a clinical perspective, and full compliance to all the most updated ethical and legal laws and regulations. This will also apply to the prediction of sepsis, both for research studies and for real-time prediction at the bedside in the daily clinical practice.
The Controversy of Sepsis Definition and Its Influence on the Development of Prediction Models
According to the recent Sepsis-3 criteria, sepsis is formally defined as an acute increase of ≥2 points in the Sequential Organ Failure Assessment (SOFA) score, consequent to a suspected or proven infection (1). Increases in the SOFA score, detected through monitoring of laboratory and clinical parameters, reflect possible impairments in cardiovascular, respiratory, renal, hepatic, coagulation, and neurological systems (28). This is in line with the novel definition of sepsis as a life-threatening and progressive organ dysfunction. Previously, sepsis was defined as the presence of a systemic inflammatory response syndrome (SIRS) caused by a proven or suspected infection and based on laboratory and clinical parameters (white blood cell count, respiratory rate, heart rate, and body temperature) that could be altered before manifestation of sepsis-related organ dysfunction (29). However, this previous, more general definition, lacked specificity, since SIRS may be present in several non-infectious conditions. On the other hand, it has very high sensitivity because of the large denominator of patients with SIRS (although some patients with organ dysfunction due to infection may not present with SIRS, and a few cases may still be missed) (30). Overall, the novel definition offers a better performance than the previous one for identifying septic patients at higher risk of mortality in intensive care units (ICU) (1). However, a better prediction of mortality does not inherently reflect the best timing for intervention. For example, it still remains unclear the optimal timing for starting antimicrobial therapy. More specifically, while it is intuitive to initiate antimicrobials if sepsis is first recognized at the time of organ dysfunction (according to the novel sepsis definition), what if the patient is already suspected to have sepsis before developing any organ dysfunction (according to previous sepsis definitions)? Can we wait, or should we initiate antimicrobials immediately? Notably, there is not a high-level evidence-based response to this question yet, and the choice is usually made upon clinical judgment at the patient's bedside, on a case-by-case basis.
However, this is hardly reproducible for defining what should be predicted by both classical statistics and machine learning models to support clinicians in the administration of antimicrobials at the best time to reduce mortality of sepsis, and, at the same time, without administering antimicrobials indiscriminately to all patients with SIRS but no sepsis, in line with antimicrobial stewardship principles. Prediction of sepsis through machine learning techniques is not exempt from this unresolved controversy, as reflected by the various definitions of sepsis employed in the different models available in the literature (see Table 1) (85). Against this backdrop, it is worth noting that the possible solution of using expert physicians' judgement for labeling sepsis cases as gold standard for model training may not resolve the issue because of suboptimal agreement across physicians (86). A reasonable alternative strategy explored by some authors may be the use of unsupervised machine learning techniques (i.e., by recognizing patterns in the data without a labeled outcome as in supervised learning) for the identification of novel phenotypes of sepsis based on clinical and laboratory values (77, 87, 88). This could help in the identification of specific subgroups of patients to be included in dedicated studies (preferably randomized clinical trials) to assess the impact of early antimicrobial therapy (77, 89, 90). Furthermore, this would allow to use the trial outcome as a measure of a posteriori accuracy of sepsis classification based on the phenotypes identified with machine learning techniques.
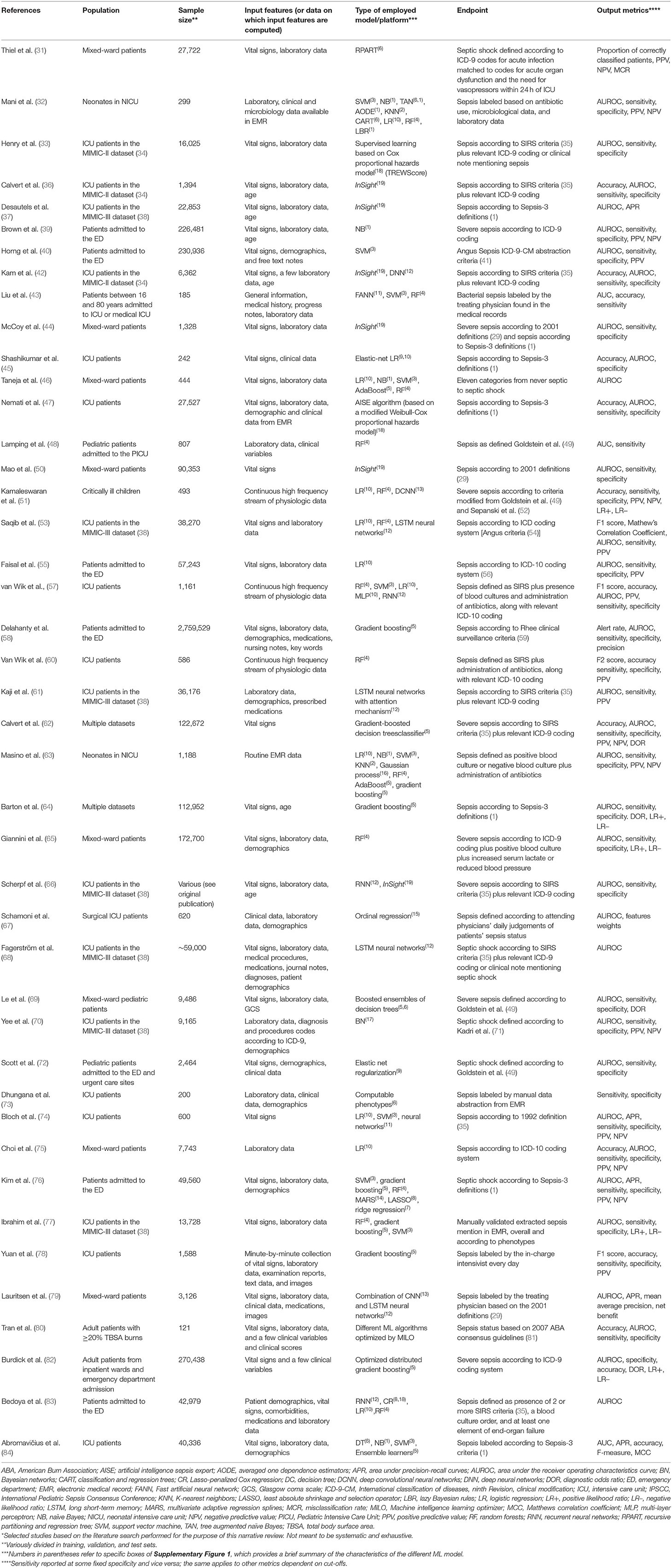
Table 1. Characteristics of selected observational studies on the use of machine learning for the prediction/early diagnosis of sepsis*.
The Choice and Availability of Input Features
The increasing use of EMR implies an immediate availability of an electronic form of relevant clinical and laboratory data, that support the development and real-time use of right-aligned predictive models for the early detection of sepsis (91–93). Indeed, these models may continuously update their prediction of sepsis by utilizing the unceasing stream of electronic data from the EMR and/or vital signs monitors (57, 74, 85, 92, 94). A good predictive model may thus allow one to correctly classify in real time a true case of sepsis in the controversial gray area in between the previous and novel sepsis definitions. Amongst others, two important questions need to be addressed: (i) which and what minimum number of input features should be used for developing a good predictive model for the early detection of sepsis? (ii) once a good predictive model is developed, would an early antimicrobial treatment improve the prognosis of these patients?
Intuitively, there can be no answer to the latter question without first developing a good predictive model. Therefore, the appropriate selection of input features remains paramount. In this regard, it is still unclear which between a parsimonious (even of a few vital and/or laboratory parameters) and an expanded (for example, considering also information from unstructured physicians' free text in EMR notes) selection of input features could be preferable, and where precisely is any possible desirable middle in between these two extremes. In the literature, there are encouraging experiences with the use of a few vital and/or laboratory parameters for the prediction of severe sepsis according to previous definitions, with also a positive impact on survival having been registered in a small but randomized single center clinical trial and in some observational studies (44, 95–97). However, the possibility of employing input features relying on patients' clinical data and medical histories remains attractive if only for attempting a concomitant prediction of etiology (e.g., of multidrug-resistant causative agent based on a combination of previous microbiological isolates and risk factors for multidrug-resistant infections, such as previous antibiotic use), that may impact appropriateness of empirical antimicrobial therapy while waiting for blood cultures results (21). Notably, this paves the way to some important issues regarding the automated extraction of unstructured data from EMR. Examples are the need for standardization of extraction, sufficient accuracy of automated extraction, internal/external validation, and continuous re-calibration of automated extraction over time. Furthermore, besides these technical aspects, the presence of missing data [which is inherent in EMR, since they are not an instrument designed for research purposes (50)] and their potential impact on the uncertainty of prediction should be properly handled.
It should also be considered that a different amount of information is expected between ICU patients (more closely monitored clinically and through laboratory tests) and patients in other wards. Therefore, besides inherent differences in their characteristics, also the different amount of available data may influence both the selection of the best set of features and the predictive performance of models developed for ICU patients and models developed for other populations (e.g., to be used in the emergency department), that may be not interchangeable. Finally, the choice of the input features could also be dictated by the local availability of the necessary infrastructures. For example, lack of validation or ability to properly extract clinical data from the local EMR may only allow the use of laboratory values for building predictive models (manual extraction of additional clinical data from EMR may be unfeasible or extremely time-consuming if large samples are concerned).
The Measure of the Model Performance, the Output, and Their Usefulness for Clinicians
As shown in Table 1, the model performance is frequently measured in term of area under the receiver operating characteristics curve (AUROC), which provide an aggregate measure of performance. Strictly speaking, the AUROC identifies how well predictions are classified considering both the number of true positive and true negative, i.e., how many true cases of sepsis or true cases of not sepsis have been correctly classified. However, this measure may not inherently reflect the clinical usefulness of the algorithm. Indeed, the global value of AUROC is independent of the chosen cut-off, whereas, in the setting of sepsis, the major interest for comparing models may be in the portion of the curve that maximizes sensitivity (in order not to miss true cases and not to delay a potentially life-saving antimicrobial treatment). This would allow, once a low risk of missing true cases is determined, to compare models in terms of specificity (aiming at reducing the number of false positive cases treated with antimicrobials, thereby reducing useless toxicity and resistance selection). In any case, the definitive proof of usefulness of any classification model should be provided by randomized clinical trials comparing sepsis management based on its use vs. standard identification of sepsis with respect to a clinically relevant primary endpoint (e.g., short-term mortality). Finally, it should be kept in mind that the present perspective was aimed to highlight some issues that may hamper the comparability and extrapolation of results of available models, and not to primarily assess their performance, which is not reported in detail. Nonetheless, we think it may be of interest to highlight the wide heterogeneity of model results. For example, the best AUROC for the prediction of sepsis ranged from 0.68 to 0.99 in a recent systematic review, whereas sensitivity and specificity were inconsistently reported to allow proper comparison (92).
Another important aspect is the output of the model. While sepsis is the outcome (or dependent variable), the output of the model may be different according to the type of model (for a brief summary of the technical characteristics of different ML models employed in available studies of sepsis prediction, see Supplementary Figure 1) (98). For example, the output of logistic regression (but also, for example, of a sigmoid function-based output layer a of neural network), intended for a single patient, is his/her probability of experiencing the outcome (in this case having sepsis or not), based on his/her input features and the calculations of the trained predictive algorithm. Let's say, for example, that the algorithm calculates for a novel given patient, based on their clinical and laboratory data, a 40% probability of having sepsis. In terms of usefulness, this probability provided to doctors may be a clinically understandable value that can be easily weighted in the balance when deciding whether or not to administer antimicrobials, possibly also improving acceptance of the implementation of machine learning-based sepsis alerts in daily practice (99). However, clinicians should also be aware of the limitations of the model, in order to further improve an appropriate understanding and use of the output. In this regard, there are no standardized directives on the optimal way to present model pitfalls to clinicians together with the model output, although “model facts” labels have started to be proposed (100).
Limitations and Conclusions
Given the narrative (and not systematic) nature of this brief narrative perspective, some original works may have not been included, a fact that, together with the lack of an in-depth description of computational aspects of the different algorithms, may represent major limitations of the present paper. Nonetheless, our intention was to provide a brief perspective for clinicians by addressing some topics of clinical interest concerning the application of machine learning techniques for the early detection of sepsis in the daily clinical practice. For this reason, we feel an extended focus on technical aspects would have been beyond the scope of the present manuscript.
In conclusion, the use of predictive tools based on machine learning may support medical decision-making by providing novel elements to improve the correct and early identification of patients with sepsis. Although at the present time it cannot be said yet whether or not this will ultimately improve patient survival and relevant antimicrobial stewardship outcomes, the increasing involvement of artificial intelligence and machine learning in health care cannot be ignored. In the long run, a rigorous multidisciplinary approach to enrich our understanding of the application of machine learning techniques to the early recognition of sepsis may be worth the trip and truly augment medical decision-making when facing this heterogeneous and complex syndrome.
Data Availability Statement
The original contributions presented in the study are included in the article/Supplementary Material, further inquiries can be directed to the corresponding author.
Author Contributions
DG made substantial contributions to the study concept and design, first drafting of the manuscript, and critical revision of the manuscript for important intellectual content. AS, MG, PP, SM, LC, CR, LB, and MB made substantial contributions to the study concept and design and critical revision of the manuscript for important intellectual content. FD, AV, and FB made substantial contributions to the literature review and critical revision of the manuscript for important intellectual content. All authors read and approved the final manuscript.
Conflict of Interest
The authors declare that the research was conducted in the absence of any commercial or financial relationships that could be construed as a potential conflict of interest.
Supplementary Material
The Supplementary Material for this article can be found online at: https://www.frontiersin.org/articles/10.3389/fmed.2021.617486/full#supplementary-material
References
1. Singer M, Deutschman CS, Seymour CW, Shankar-Hari M, Annane D, Bauer M, et al. The third international consensus definitions for sepsis and septic shock (Sepsis-3). JAMA. (2016) 315:801–10. doi: 10.1001/jama.2016.0287
2. Stevenson EK, Rubenstein AR, Radin GT, Seylemez Wiener R, Walkey AJ. Two decades of mortality trends among patients with severe sepsis: a comparative meta-analysis*. Crit. Care Med. (2014) 42:625–31. doi: 10.1097/CCM.0000000000000026
3. Fleischmann C, Scherag A, Adhikari NK, Hartog CS, Tsaganos T, Schattmann P, et al. Assessment of global incidence and mortality of hospital-treated sepsis. Current estimates and limitations. Am. J. Respir. Crit. Care Med. (2016) 193:259–72. doi: 10.1164/rccm.201504-0781OC
4. Vincent JL, Sakr Y, Singer M, Loeches IM, Machado FR, Marshall JC, et al. Prevalence and outcomes of infection among patients in intensive care units in 2017. JAMA. (2020) 323:1478–87. doi: 10.1001/jama.2020.2717
5. Fleischmann-Struzek C, Mellhammar L, Rose N, Cassini A, Rudd KE, Schlattmann P, et al. Incidence and mortality of hospital- and ICU-treated sepsis: results from an updated and expanded systematic review and meta-analysis. Intensive Care Med. (2020) 46:1552–62. doi: 10.1007/s00134-020-06151-x
6. Delano MJ, Ward PA. The immune system's role in sepsis progression, resolution, and long-term outcome. Immunol. Rev. (2016) 274:330–53. doi: 10.1111/imr.12499
7. Iskander KN, Osuchowski MF, Stearns-Kurosawa DJ, Kurosawa S, Stepien D, Valentine C, Remick DG. Sepsis: multiple abnormalities, heterogeneous responses, and evolving understanding. Physiol. Rev. (2013) 93:1247–88. doi: 10.1152/physrev.00037.2012
8. Carneiro AH, Povoa P, Gomes JA. Dear Sepsis-3, we are sorry to say that we don't like you. Rev. Bras. Ter. Intensiva. (2017) 29:4–8. doi: 10.5935/0103-507X.20170002
9. Marshall JC. Sepsis-3: what is the meaning of a definition? Crit. Care Med. (2016) 44:1459–60. doi: 10.1097/CCM.0000000000001983
10. Seymour CW, Gesten F, Prescott HC, Friedrich ME, Iwashyna TJ, Phillips GS, et al. Time to treatment and mortality during mandated emergency care for sepsis. N. Engl. J. Med. (2017) 376:2235–44. doi: 10.1056/NEJMoa1703058
11. Teng AK, Wilcox AB. A review of predictive analytics solutions for sepsis patients. Appl. Clin. Inform. (2020) 11:387–98. doi: 10.1055/s-0040-1710525
12. Mlodzinski E, Stone DJ, Celi LA. Machine learning for pulmonary and critical care medicine: a narrative review. Pulm. Ther. (2020) 6:67–77. doi: 10.1007/s41030-020-00110-z
13. Medic G, Kosaner Kliess M, Atallah L, Weichert J, Panda S, Postma M, et al. Evidence-based clinical decision support systems for the prediction and detection of three disease states in critical care: a systematic literature review. F1000Res. (2019) 8:1728. doi: 10.12688/f1000research.20498.1
14. Schinkel M, Paranjape K, Nannan Panday RS, Skyttberg N, Nanayakkara PWB. Clinical applications of artificial intelligence in sepsis: a narrative review. Comput. Biol. Med. (2019) 115:103488. doi: 10.1016/j.compbiomed.2019.103488
15. Islam MM, Nasrin T, Walther BA, Wu CC, Yang HC, Li YC. Prediction of sepsis patients using machine learning approach: a meta-analysis. Comput. Methods Programs Biomed. (2019) 170:1–9. doi: 10.1016/j.cmpb.2018.12.027
16. Vellido A, Ribas V, Morales C, Sanmartin AR, Ruiz Rodriguez JC. Machine learning in critical care: state-of-the-art and a sepsis case study. Biomed. Eng. Online. (2018) 17(Suppl. 1):135. doi: 10.1186/s12938-018-0569-2
17. Liu VX, Walkey AJ. Machine learning and sepsis: on the road to revolution. Crit. Care Med. (2017) 45:1946–7. doi: 10.1097/CCM.0000000000002673
18. Peiffer-Smadja N, Rawson TM, Ahmad R, Buchard A, Georgiou P, Lescure FX, et al. Machine learning for clinical decision support in infectious diseases: a narrative review of current applications. Clin. Microbiol. Infect. (2020) 26:584–95. doi: 10.1016/j.cmi.2019.09.009
19. Tobore I, Li J, Yuhang L, Al-Handarish Y, Kandwal A, Nie Z, et al. Deep learning intervention for health care challenges: some biomedical domain considerations. JMIR Mhealth Uhealth. (2019) 7:e11966. doi: 10.2196/11966
20. Rajkomar A, Dean J, Kohane I. Machine learning in medicine. N. Engl. J. Med. (2019) 380:1347–58. doi: 10.1056/NEJMra1814259
21. Giacobbe DR, Mora S, Giacomini M, Bassetti M. Machine learning and multidrug-resistant gram-negative bacteria: an interesting combination for current and future research. Antibiotics. (2020) 9:54. doi: 10.3390/antibiotics9020054
22. Beam AL, Kohane IS. Big data and machine learning in health care. JAMA. (2018) 319:1317–8. doi: 10.1001/jama.2017.18391
23. Rudin C. Stop explaining black box machine learning models for high stakes decisions and use interpretable models instead. Nat. Mach. Intell. (2019) 1:206–15. doi: 10.1038/s42256-019-0048-x
24. de Miguel I, Sanz B, Lazcoz G. Machine learning in the EU health care context: exploring the ethical, legal and social issues. Inf. Commun. Soc. (2020) 23:1139–53. doi: 10.1080/1369118X.2020.1719185
25. Gerke S, Minssen T, Cohen G. Ethical and legal challenges of artificial intelligence-driven healthcare. Artif. Intell. Healthcare. (2020) 2020:295–336. doi: 10.1016/B978-0-12-818438-7.00012-5
26. Johnson SLJ. AI, machine learning, and ethics in health care. J. Leg Med. (2019) 39:427–41. doi: 10.1080/01947648.2019.1690604
27. Ngiam KY, Khor IW. Big data and machine learning algorithms for health-care delivery. Lancet Oncol. (2019) 20:e262–73. doi: 10.1016/S1470-2045(19)30149-4
28. Vincent JL, Moreno R, Takala J, Willatts S, De Mendonça A, Bruining H, et al. The SOFA (Sepsis-related Organ Failure Assessment) score to describe organ dysfunction/failure. Intensive Care Med. (1996) 22:707–10. doi: 10.1007/BF01709751
29. Levy MM, Fink MP, Marshall JC, Abraham E, Angus D, Cook D, et al. SCCM/ESICM/ACCP/ATS/SIS international sepsis definitions conference. Intensive Care Med. (2003) 29:530–8. doi: 10.1007/s00134-003-1662-x
30. Kaukonen KM, Bailey M, Pilcher D, Cooper DJ, Bellomo R. Systemic inflammatory response syndrome criteria in defining severe sepsis. N. Engl. J. Med. (2015) 372:1629–38. doi: 10.1056/NEJMoa1415236
31. Thiel SW, Rosini JM, Shannon W, Doherty JA, Micek ST, Kollef MH. Early prediction of septic shock in hospitalized patients. J. Hosp. Med. (2010) 5:19–25. doi: 10.1002/jhm.530
32. Mani S, Ozdas A, Aliferis C, Varol HA, Chen Q, Carnevale R, et al. Medical decision support using machine learning for early detection of late-onset neonatal sepsis. J. Am. Med. Inform. Assoc. (2014) 21:326–36. doi: 10.1136/amiajnl-2013-001854
33. Henry KE, Hager DN, Pronovost PJ, Saria S. A targeted real-time early warning score (TREWScore) for septic shock. Sci. Transl. Med. (2015) 7:299ra122. doi: 10.1126/scitranslmed.aab3719
34. Lee J, Scott DJ, Villarroel M, Clifford GD, Saeed M, Mark RG. Open-access MIMIC-II database for intensive care research. Annu. Int. Conf. IEEE Eng. Med. Biol. Soc. (2011) 2011:8315–8. doi: 10.1109/IEMBS.2011.6092050
35. American College of Chest Physicians/Society of Critical Care Medicine Consensus Conference. Definitions for sepsis and organ failure and guidelines for the use of innovative therapies in sepsis. Crit. Care Med. (1992) 20:864–74. doi: 10.1097/00003246-199206000-00025
36. Calvert JS, Price DA, Chettipally UK, Barton CW, Feldman MD, Hoffman JL, et al. A computational approach to early sepsis detection. Comput. Biol. Med. (2016) 74:69–73. doi: 10.1016/j.compbiomed.2016.05.003
37. Desautels T, Calvert J, Hoffman J, Jay M, Kerem Y, Shieh L, et al. Prediction of sepsis in the intensive care unit with minimal electronic health record data: a machine learning approach. JMIR Med. Inform. (2016) 4:e28. doi: 10.2196/medinform.5909
38. Johnson AE, Pollard TJ, Shen L, Lehman LH, Feng M, Ghassemi M, et al. MIMIC-III, a freely accessible critical care database. Sci. Data. (2016) 3:160035. doi: 10.1038/sdata.2016.35
39. Brown SM, Jones J, Kuttler KG, Keddington RK, Allen TL, Haug P. Prospective evaluation of an automated method to identify patients with severe sepsis or septic shock in the emergency department. BMC Emerg. Med. (2016) 16:31. doi: 10.1186/s12873-016-0095-0
40. Horng S, Sontag DA, Halpern Y, Jernite Y, Shapiro NI, Nathanson LA. Creating an automated trigger for sepsis clinical decision support at emergency department triage using machine learning. PLoS ONE. (2017) 12:e0174708. doi: 10.1371/journal.pone.0174708
41. Angus DC, Linde-Zwirble WT, Lidicker J, Clermont G, Carcillo J, Pinsky MR. Epidemiology of severe sepsis in the United States: analysis of incidence, outcome, and associated costs of care. Crit. Care Med. (2001) 29:1303–10. doi: 10.1097/00003246-200107000-00002
42. Kam HJ, Kim HY. Learning representations for the early detection of sepsis with deep neural networks. Comput. Biol. Med. (2017) 89:248–55. doi: 10.1016/j.compbiomed.2017.08.015
43. Liu Y, Choi KS. Using machine learning to diagnose bacterial sepsis in the critically ill patients. In: H. Chen, D. Zeng, E. Karahanna, I. Bardhan, editors. Smart Health. ICSH 2017. Lecture Notes in Computer Science. Cham: Springer (2017). p. 223–33. doi: 10.1007/978-3-319-67964-8_22
44. Shimabukuro DW, Barton CW, Feldman MD, Mataraso SJ, Das R. Effect of a machine learning-based severe sepsis prediction algorithm on patient survival and hospital length of stay: a randomised clinical trial. BMJ Open Respir Res. (2017) 4:e000234. doi: 10.1136/bmjresp-2017-000234
45. Shashikumar SP, Stanley MD, Sadiq I, Li Q, Holder A, Clifford GD, Nemati S. Early sepsis detection in critical care patients using multiscale blood pressure and heart rate dynamics. J. Electrocardiol. (2017) 50:739–43. doi: 10.1016/j.jelectrocard.2017.08.013
46. Taneja I, Reddy B, Damhorst G, Zhao SD, Hassan U, Price Z, et al. Combining biomarkers with EMR data to identify patients in different phases of sepsis. Sci. Rep. (2017) 7:10800. doi: 10.1038/s41598-017-09766-1
47. Nemati S, Holder A, Razmi F, Stanley MD, Clifford GD, Buchman TG. An interpretable machine learning model for accurate prediction of sepsis in the ICU. Crit. Care Med. (2018) 46:547–53. doi: 10.1097/CCM.0000000000002936
48. Lamping F, Jack T, Rübsamen N, Sasse M, Beerbaum P, Mikolajczyk RT, et al. Development and validation of a diagnostic model for early differentiation of sepsis and non-infectious SIRS in critically ill children – a data-driven approach using machine-learning algorithms. BMC Pediatr. (2018) 18:112. doi: 10.1186/s12887-018-1082-2
49. Goldstein B, Giroir B, Randolph A. International pediatric sepsis consensus conference: definitions for sepsis and organ dysfunction in pediatrics. Pediatr. Crit. Care Med. (2005) 6:2–8. doi: 10.1097/01.PCC.0000149131.72248.E6
50. Paxton C, Niculescu-Mizil A, Saria S. Developing predictive models using electronic medical records: challenges and pitfalls. AMIA Annu. Symp. Proc. (2013) 2013:1109–15.
51. Kamaleswaran R, Akbilgic O, Hallman MA, West AN, Davis RL, Shah SH. Applying artificial intelligence to identify physiomarkers predicting severe sepsis in the PICU. Pediatr. Crit. Care Med. (2018) 19:e495–503. doi: 10.1097/PCC.0000000000001666
52. Sepanski RJ, Godambe SA, Mangum CD, Bovat CS, Zaritsky AL, Shah SH. Designing a pediatric severe sepsis screening tool. Front. Pediatr. (2014) 2:56. doi: 10.3389/fped.2014.00056
53. Saqib M, Sha Y, Wang MD. Early prediction of sepsis in EMR records using traditional ML techniques and deep learning LSTM networks. Annu. Int. Conf. IEEE Eng. Med. Biol. Soc. (2018) 2018:4038–41. doi: 10.1109/EMBC.2018.8513254
54. Iwashyna TJ, Odden A, Rohde J, Bonham C, Kuhn L, Malani P, et al. Identifying patients with severe sepsis using administrative claims: patient-level validation of the angus implementation of the international consensus conference definition of severe sepsis. Med. Care. (2014) 52:e39–43. doi: 10.1097/MLR.0b013e318268ac86
55. Faisal M, Scally A, Richardson D, Beatson K, Howes R, Speed K, Mohmmed MA. Development and external validation of an automated computer-aided risk score for predicting sepsis in emergency medical admissions using the patient's first electronically recorded vital signs and blood test results. Crit. Care Med. (2018) 46:612–8. doi: 10.1097/CCM.0000000000002967
56. Jolley RJ, Quan H, Jette N, Sawka KJ, Diep L, Goliath J, et al. Validation and optimisation of an ICD-10-coded case definition for sepsis using administrative health data. BMJ Open. (2015) 5:e009487. doi: 10.1136/bmjopen-2015-009487
57. Rush B, Celi LA, Stone DJ. Applying machine learning to continuously monitored physiological data. J. Clin. Monit. Comput. (2019) 33:887–93. doi: 10.1007/s10877-018-0219-z
58. Delahanty RJ, Alvarez J, Flynn LM, Sherwin RL, Jones SS. Development and evaluation of a machine learning model for the early identification of patients at risk for sepsis. Ann. Emerg. Med. (2019) 73:334–44. doi: 10.1016/j.annemergmed.2018.11.036
59. Rhee C, Dantes R, Epstein L, Murphy DJ, Seymour CW, Iwashyna TI, et al. Incidence and trends of sepsis in US hospitals using clinical vs claims data, 2009-2014. JAMA. (2017) 318:1241–9. doi: 10.1001/jama.2017.13836
60. van Wyk F, Khojandi A, Kamaleswaran R. Improving prediction performance using hierarchical analysis of real-time data: a sepsis case study. IEEE J. Biomed. Health Inform. (2019) 23:978–86. doi: 10.1109/JBHI.2019.2894570
61. Kaji DA, Zech JR, Kim JS, Cho SK, Dangayach NS, Costa AB, Oermann EK. An attention based deep learning model of clinical events in the intensive care unit. PLoS ONE. (2019) 14:e0211057. doi: 10.1371/journal.pone.0211057
62. Calvert J, Saber N, Hoffman J, Das R. Machine-learning-based laboratory developed test for the diagnosis of sepsis in high-risk patients. Diagnostics. (2019) 9:20. doi: 10.3390/diagnostics9010020
63. Masino AJ, Harris MC, Forsyth D, Ostapenko S, Srinivasan L, Bonafide CP, et al. Machine learning models for early sepsis recognition in the neonatal intensive care unit using readily available electronic health record data. PLoS ONE. (2019) 14:e0212665. doi: 10.1371/journal.pone.0212665
64. Barton C, Chettipally U, Zhou Y, Jiang Z, Lynn-Palevsky A, Le S, et al. Evaluation of a machine learning algorithm for up to 48-hour advance prediction of sepsis using six vital signs. Comput. Biol. Med. (2019) 109:79–84. doi: 10.1016/j.compbiomed.2019.04.027
65. Giannini HM, Ginestra JC, Chivers C, Draugelis M, Hanish A, Schweickert WD, et al. A machine learning algorithm to predict severe sepsis and septic shock: development, implementation, and impact on clinical practice. Crit. Care Med. (2019) 47:1485–92. doi: 10.1097/CCM.0000000000003891
66. Scherpf M, Grasser F, Malberg H, Zaunseder S. Predicting sepsis with a recurrent neural network using the MIMIC III database. Comput. Biol. Med. (2019) 113:103395. doi: 10.1016/j.compbiomed.2019.103395
67. Schamoni S, Lindner HA, Schneider-Lindner V, Thiel M, Riezler S. Leveraging implicit expert knowledge for non-circular machine learning in sepsis prediction. Artif. Intell. Med. (2019) 100:101725. doi: 10.1016/j.artmed.2019.101725
68. Fagerstrom J, Bang M, Wilhelms D, Chew MS. LiSep LSTM: a machine learning algorithm for early detection of septic shock. Sci. Rep. (2019) 9:15132. doi: 10.1038/s41598-019-51219-4
69. Le S, Hoffman J, Barton C, Fitzgerald JC, Allen A, Pellegrini E, et al. Pediatric severe sepsis prediction using machine learning. Front. Pediatr. (2019) 7:413. doi: 10.3389/fped.2019.00413
70. Yee CR, Narain NR, Akmaev VR, Vamulapalli V. A data-driven approach to predicting septic shock in the intensive care unit. Biomed. Inform. Insights. (2019) 11:1178222619885147. doi: 10.1177/1178222619885147
71. Kadri SS, Rhee C, Strich JR, Morales MK, Hohmann S, Menchaca J. Estimating ten-year trends in septic shock incidence and mortality in United States academic medical centers using clinical data. Chest. (2017) 151:278–85. doi: 10.1016/j.chest.2016.07.010
72. Scott HF, Colborn KL, Sevick CJ, Bajaj L, Kissoon N, Deakyne Davies SJ, Kempe A. Development and validation of a predictive model of the risk of pediatric septic shock using data known at the time of hospital arrival. J. Pediatr. (2020) 217:145–51.e6. doi: 10.1016/j.jpeds.2019.09.079
73. Dhungana P, Serafim LP, Ruiz AL, Bruns D, Weister TJ, Smischney NJ, et al. Machine learning in data abstraction: a computable phenotype for sepsis and septic shock diagnosis in the intensive care unit. World J. Crit. Care Med. (2019) 8:120–6. doi: 10.5492/wjccm.v8.i7.120
74. van Wyk F, Khojandi A, Mohammed A, Begoli E, Davis RL, Kamaleswaran. A minimal set of physiomarkers in continuous high frequency data streams predict adult sepsis onset earlier. Int. J. Med. Inform. (2019) 122:55–62. doi: 10.1016/j.ijmedinf.2018.12.002
75. Choi JS, Trinh TX, Ha J, Yang MS, Lee Y, Kim YE, et al. Implementation of complementary model using optimal combination of hematological parameters for sepsis screening in patients with fever. Sci. Rep. (2020) 10:273. doi: 10.1038/s41598-019-57107-1
76. Kim J, Chang H, Kim D, Jang DH, Park I, Kim K. Machine learning for prediction of septic shock at initial triage in emergency department. J. Crit. Care. (2020) 55:163–70. doi: 10.1016/j.jcrc.2019.09.024
77. Seymour CW, Kennedy JN, Wang S, Chang CH, Elliott CF, Xu Z, et al. Derivation, validation, and potential treatment implications of novel clinical phenotypes for sepsis. JAMA. (2019) 321:2003–17. doi: 10.1001/jama.2019.5791
78. Yuan KC, Tsai LW, Lee KH, Cheng YW, Hsu SC, Lo YS, et al. The development an artificial intelligence algorithm for early sepsis diagnosis in the intensive care unit. Int. J. Med. Inform. (2020) 141:104176. doi: 10.1016/j.ijmedinf.2020.104176
79. Lauritsen SM, Kalor ME, Kongsgaard EL, Lauritsen KM, Jørgensen MJ, Lange J, et al. Early detection of sepsis utilizing deep learning on electronic health record event sequences. Artif. Intell. Med. (2020) 104:101820. doi: 10.1016/j.artmed.2020.101820
80. Tran NK, Albahra S, Pham TN, Holmes JHt, Greenhalgh D, Palmieri TL, et al. Novel application of an automated-machine learning development tool for predicting burn sepsis: proof of concept. Sci. Rep. (2020) 10:12354. doi: 10.1038/s41598-020-69433-w
81. Greenhalgh DG, Saffle JR, Holmes JHT, Gamelli RL, Palmieri TL, Horton JW, et al. American Burn Association consensus conference to define sepsis and infection in burns. J. Burn Care Res. (2007) 28:776–90. doi: 10.1097/BCR.0b013e3181599bc9
82. Burdick H, Pino E, Gabel-Comeau D, Gu C, Roberts J, Le S, et al. Validation of a machine learning algorithm for early severe sepsis prediction: a retrospective study predicting severe sepsis up to 48 h in advance using a diverse dataset from 461 US hospitals. BMC Med. Inform. Decis. Mak. (2020) 20:276. doi: 10.1186/s12911-020-01284-x
83. Bedoya AD, Futoma J, Clement ME, Corey K, Brajer N, Lin A, et al. Machine learning for early detection of sepsis: an internal and temporal validation study. JAMIA Open. (2020) 3:252–60. doi: 10.1093/jamiaopen/ooaa006
84. Abromavičius V, Plonis D, Tarasevičius D, Serackis A. Two-stage monitoring of patients in intensive care unit for sepsis prediction using non-overfitted machine learning models. Electronics. (2020) 9:1133. doi: 10.3390/electronics9071133
85. Saria S, Henry KE. Too many definitions of sepsis: can machine learning leverage the electronic health record to increase accuracy and bring consensus? Crit. Care Med. (2020) 48:137–41. doi: 10.1097/CCM.0000000000004144
86. Lopansri BK, Miller RR III, Burke JP, Levy M, Opal S, Rothman RE, et al. Physician agreement on the diagnosis of sepsis in the intensive care unit: estimation of concordance and analysis of underlying factors in a multicenter cohort. J. Intensive Care. (2019) 7:13. doi: 10.1186/s40560-019-0368-2
87. Ibrahim ZM, Wu H, Hamoud A, Hamoud A, Stappen L, Dobson RJB, Agarossi A. On classifying sepsis heterogeneity in the ICU: insight using machine learning. J. Am. Med. Inform. Assoc. (2020) 27:437–43. doi: 10.1093/jamia/ocz211
88. Guilamet MCV, Bernauer M, Micek ST, Kollef MH. Cluster analysis to define distinct clinical phenotypes among septic patients with bloodstream infections. Medicine. (2019) 98:e15276. doi: 10.1097/MD.0000000000015276
89. Fohner AE, Greene JD, Lawson BL, Chen JH, Kipnis P, Escobar GJ, et al. Assessing clinical heterogeneity in sepsis through treatment patterns and machine learning. J. Am. Med. Inform. Assoc. (2019) 26:1466–77. doi: 10.1093/jamia/ocz106
90. Knaus WA, Marks RD. New phenotypes for sepsis: the promise and problem of applying machine learning and artificial intelligence in clinical research. JAMA. (2019) 321:1981–82. doi: 10.1001/jama.2019.5794
91. Luz CF, Vollmer M, Decruyenaere J, Nijsten MW, Glasner C, Sinha B. Machine learning in infection management using routine electronic health records: tools, techniques, and reporting of future technologies. Clin. Microbiol. Infect. (2020) 26:1291–9. doi: 10.1016/j.cmi.2020.02.003
92. Fleuren LM, Klausch TLT, Zwager CL, Schoonmade LJ, Guo T, Roggeveen LF, et al. Machine learning for the prediction of sepsis: a systematic review and meta-analysis of diagnostic test accuracy. Intensive Care Med. (2020) 46:383–400. doi: 10.1007/s00134-019-05872-y
93. Ruppel H, Liu V. To catch a killer: electronic sepsis alert tools reaching a fever pitch? BMJ Qual. Saf . (2019) 28:693–6. doi: 10.1136/bmjqs-2019-009463
94. Bloch E, Rotem T, Cohen J, Singer P, Aperstein Y. Machine learning models for analysis of vital signs dynamics: a case for sepsis onset prediction. J. Healthc Eng. (2019) 2019:5930379. doi: 10.1155/2019/5930379
95. Burdick H, Pino E, Gabel-Comeau D, McCoy A, Gu C, Roberts J, et al. Effect of a sepsis prediction algorithm on patient mortality, length of stay and readmission: a prospective multicentre clinical outcomes evaluation of real-world patient data from US hospitals. BMJ Health Care Inform. (2020) 27:e100109. doi: 10.1136/bmjhci-2019-100109
96. McCoy A, Das R. Reducing patient mortality, length of stay and readmissions through machine learning-based sepsis prediction in the emergency department, intensive care unit and hospital floor units. BMJ Open Qual. (2017) 6:e000158. doi: 10.1136/bmjoq-2017-000158
97. Mao Q, Jay M, Hoffman JL, Calvert J, Barton EC, Shimabukuro D, et al. Multicentre validation of a sepsis prediction algorithm using only vital sign data in the emergency department, general ward and ICU. BMJ Open. (2018) 8:e017833. doi: 10.1136/bmjopen-2017-017833
98. Zaki M, Wagner M Jr. Data Mining and Machine Learning: Fundamental Concepts and algorithms. 2nd ed. Cambridge: Cambridge University Press (2020). doi: 10.1017/9781108564175
99. Ginestra JC, Giannini HM, Schweickert WD, Meadows L, Lynch MJ, Pavan K. Clinician perception of a machine learning-based early warning system designed to predict severe sepsis and septic shock. Crit. Care Med. (2019) 47:1477–84. doi: 10.1097/CCM.0000000000003803
Keywords: sepsis, machine learning, artificial intelligence, early diagnosis, supervised learning, unsupervised learning
Citation: Giacobbe DR, Signori A, Del Puente F, Mora S, Carmisciano L, Briano F, Vena A, Ball L, Robba C, Pelosi P, Giacomini M and Bassetti M (2021) Early Detection of Sepsis With Machine Learning Techniques: A Brief Clinical Perspective. Front. Med. 8:617486. doi: 10.3389/fmed.2021.617486
Received: 14 October 2020; Accepted: 19 January 2021;
Published: 12 February 2021.
Edited by:
Marc Jean Struelens, Université libre de Bruxelles, BelgiumReviewed by:
Alex Soriano, Hospital Clínic de Barcelona, SpainAlice Picciarella, Policlinico Casilino, Italy
Copyright © 2021 Giacobbe, Signori, Del Puente, Mora, Carmisciano, Briano, Vena, Ball, Robba, Pelosi, Giacomini and Bassetti. This is an open-access article distributed under the terms of the Creative Commons Attribution License (CC BY). The use, distribution or reproduction in other forums is permitted, provided the original author(s) and the copyright owner(s) are credited and that the original publication in this journal is cited, in accordance with accepted academic practice. No use, distribution or reproduction is permitted which does not comply with these terms.
*Correspondence: Daniele Roberto Giacobbe, daniele.roberto.giacobbe@gmail.com