- 1The First Clinical Medical School, China Medical University, Shenyang, China
- 2The Second Clinical Medical School, China Medical University, Shenyang, China
- 3Emory College of Arts and Sciences, Emory University, Atlanta, GA, United States
- 4The Fourth Clinical Medical School, China Medical University, Shenyang, China
- 5School of Intelligent Medicine, China Medical University, Shenyang, China
Introduction: Machine learning technology has demonstrated significant potential in glaucoma research, particularly in early diagnosis, predicting disease progression, evaluating treatment responses, and developing personalized treatment strategies. The application of machine learning not only enhances the understanding of the pathological mechanism of glaucoma and optimizes the diagnostic process but also provides patients with accurate medical services.
Methods: This study aimed to describe the current state of research, highlight directions for further development, and identify potential trends for improvement. This review was conducted following the scoping review of the Preferred Reporting Items for Systematic Reviews and Meta-Analyses (PRISMA) extension to showcase advancements in the application of machine learning in glaucoma research and treatment.
Results: We employed a comprehensive search strategy to retrieve literature from the Web of Science Core Collection database, ultimately including 3,581 articles in the analysis. Through data analysis, we identified current research hotspots, noted differences in researchers' attitudes and opinions, and predicted potential future development trends.
Discussion: We divided the research topics into six categories, clearly identifying “eye diseases”, “retinal fundus imaging” and “risk factors” as the key terms for the development of this field. These findings signify the promising prospects of machine learning, particularly when integrated with multimodal technologies and large language models, to enhance the diagnosis and treatment of glaucoma.
1 Introduction
Glaucoma, characterized by retinal nerve fiber layer defects and concomitant visual field (VF) damage, is considered one of the leading causes of irreversible visual impairment worldwide (1). Glaucoma is estimated to affect more than 110 million individuals over the next two decades (2, 3). Therefore, adopting novel diagnostic techniques to explore glaucoma pathogenesis and facilitate early detection protocols is of significant clinical relevance (4, 5).
In 2016, a significant shift occurred in the application of machine learning in the realm of ophthalmology with the publication of articles focusing on its applications in diabetic retinopathy (DR) screening (6, 7). Subsequently, the integration of medical imaging with machine learning technology has been applied to disease diagnosis, and researchers have developed numerous automated ophthalmic diagnostic systems (8–11). Deep learning (DL) algorithms, which extract features from images and represent them in layers, have shown considerable promise in distinguishing between glaucomatous and non-glaucomatous patterns (12, 13). Moreover, the DL model performed well in the fundus image analysis of glaucoma, with a diagnostic accuracy of 95.59% (14). These algorithms are critical for screening, diagnostic decision-making, and disease prognosis (15). The emergence of machine learning-based multimodal imaging technologies is poised to have a transformative impact on medical management and therapeutic strategies for glaucoma in the coming years (16).
In recent years, the growing body of literature on machine learning applications in glaucoma research has attracted significant scholarly interest, reflecting the dynamic evolution of the field and the imperative for systematic evaluation. During this period, a comprehensive assessment and review of existing knowledge can be performed using methods such as statistical analysis of publication volume, collaboration across countries, regions, or institutions, analysis of the sources and characteristics of cited publications, and identification of high-frequency and emerging keywords. These approaches can clarify the current research hotspots in advancing glaucoma diagnosis and treatment with machine learning and predict future development directions. Currently, advancements in machine learning within the field of glaucoma are mostly fragmented, with a lack of comprehensive and forward-looking reviews. Therefore, we conducted an assessment and scoping review of relevant articles to assess and summarize the existing knowledge about the application of machine learning in glaucoma research. We aimed to clarify the role of machine learning in advancing glaucoma research and identify key research directions through a visual approach, considering the development trends in this field (17, 18). Our report may provide some references for ophthalmologists and medical engineering researchers to comprehensively explore this field and embrace machine learning applications in glaucoma.
2 Methods
2.1 Scoping review
For this scoping review, we selected the Web of Science Core Collection database, as it offers comprehensive subject coverage and high-quality literature, providing a wide range of influential, representative, and accurate literature on the research topic. We developed a search strategy that covers a wide range of themes, which, unlike systematic reviews, does not rely solely on the quality of evidence as the only measure. This approach allows for better acquisition of researchers' perspectives and attitudes within the broad scope of the study. We also extracted and identified multiple characteristics of the retrieved literature, including country, institution, references, journals, subject categories, and keywords. This enables our research to gain a more comprehensive understanding of the development status within the field, thereby making more accurate predictions about development trends.
2.2 Data retrieval
We developed an accurate and comprehensive search query using the following search terms: (TS= ((“Machine Learning” OR “Artificial Intelligence” OR “Deep Learning” OR “Support Vector Machine*” OR “Linear Regression” OR “Logistic Regression” OR “decision tree” OR “random forest” OR “K-Nearest Neighbors” OR” Naive Bayes” OR “Naive Bayes Model” OR “Convolutional Neural Network*” OR “Recurrent Neural Network*” OR “XGBoost” OR “Fully Convolutional Network*” OR “Generative Adversarial Network” OR “Reinforcement Learning” OR “Back Propagation” OR “Fully Neural Network” OR “Recursive Neural Network” OR “Auto encoder” OR “Deep Belief Network” OR “Restricted Boltzmann machine” OR “Transformers” OR “Graph Convolution Networks” OR “k-means” OR “Ada boost” OR “Markov chain” OR “Natural Language Processing” OR “Generative Pre-trained Transformer” OR “Bidirectional Encoder Representations from Transformers” OR “LLM”) AND (“Glaucoma”))), the literature type= “Article”. A literature search was conducted from 2000 to 2024.
2.3 Inclusion and exclusion verification
In terms of publication time, to study the latest advancements in machine learning within the field of glaucoma, we focused on relatively recent literature, excluding studies published before 2000. Regarding article types, we selected “articles” to ascertain the perspectives and attitudes of relevant researchers, as these formats are less influenced by external opinions during the writing process and can clearly express personal viewpoints. We excluded evidence types such as “conferences”, “article reviews”, “letters”, and “news”. For search topics, we extracted keywords from the research questions and, based on these, obtained clusters of related words, which were used as search terms to ensure a comprehensive and objective search of the topic keywords. To ensure that the articles found aligned with our research theme, two researchers independently verified the retrieved data.
2.4 Data feature recognition
We extracted various metadata from the retrieved publications, including article titles, abstracts, authorship, institution, country/region, journal, keywords, and cited references, to create a connected network of 3,581 articles with 80,657 references.
2.5 Data classification analysis
Data analysis was performed from multiple perspectives, including publication year, country/region, institution, journal, cited literature, high-frequency keywords, and emerging keywords. This approach allowed us to study the characteristics of researchers in terms of changes in word preference, selection of publication journals, and shifts in word frequency, thereby identifying current research hotspots and variations in researchers' attitudes and viewpoints (19).
2.6 Summary and visualization presentation
We converted the data into easily recognizable charts or images and conducted predictive research on the development direction of researchers' views and attitudes toward the subject. Based on the knowledge obtained from data classification analysis, we predicted future trends and potential research directions in this field.
2.7 Patient consent
This study was a scoping review, and the clinical data used were derived from public databases; therefore, informed consent from patients could not be obtained.
3 Results
3.1 Distribution of articles by publication years
We retrieved a total of 3,581 published articles. As shown in Figure 1A, the bars represent the total annual number of published studies, illustrating the publication trend from 2000 to 2024. Notably, the number of published articles has steadily increased, at a slow pace from 2000 to 2016, followed by a significant rise in recent years. Over the last 4 years, this number has accounted for more than 11% of the total number of published articles. These findings demonstrate that the boom in machine learning technology and its application in glaucoma is gradually becoming the focus of attention and entering a stage of rapid development. The number of publications in 2024 was significantly lower than in 2023. This is because the information we retrieved on 1 April 2024, only included literature published before that date and did not encompass publications released after 1 April 2024.
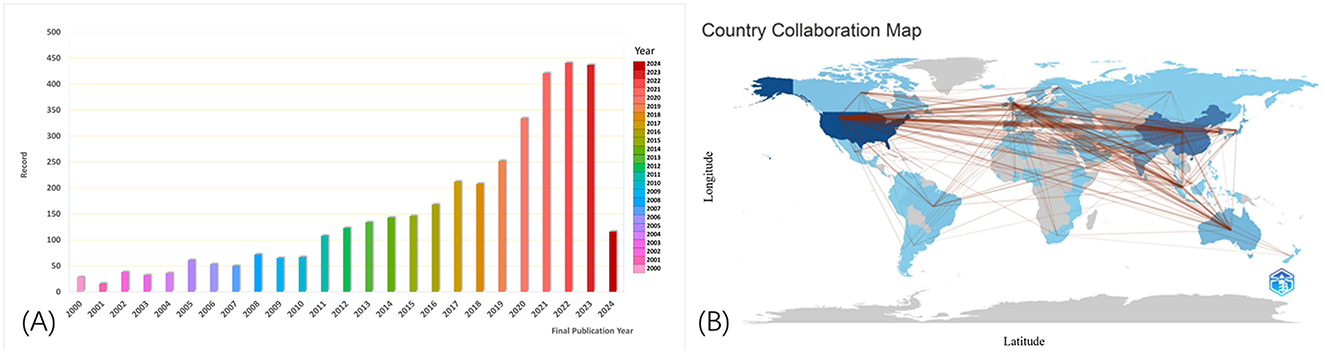
Figure 1. Numbers of published articles from 2000 to 2024 (A). The cooperation of countries or regions that contributed to publications (B). Collaboration between countries provides a strong incentive for progress in scientific studies. The lines represent the collaboration between different countries, with broader lines indicating more collaboration and communication.
3.2 Analysis of countries/regions, institutions, and journals
We analyzed the literature within the reference range, which included publications from 102 countries and 3,343 institutes. The country that has contributed the most to the literature is the United States, followed by China, South Korea, England, and India, which rank second to fifth, respectively. Most of the influential research institutions are located in the United States and the United Kingdom, indicating that these countries and institutions have invested more research efforts in this field and should be given attention. However, it is worth mentioning that the research center with the highest number of published articles is the University of California system (300 articles), highlighting its prominent role and significant impact in the field of machine learning applications for glaucoma.
As shown in Figure 1B made by the bibliometrix package in R software shows how articles were distributed in the various contributing countries and areas. Collaboration between countries is a strong incentive for progress in scientific studies. The lines refer to the work between different countries. The broader lines indicate more collaboration and communication.
3.3 References analyses
References are key indicators, as frequently cited documents can significantly influence their research areas. Table 1 lists the top 10 most-cited articles in this field, which were cited more than 470 times out of 80,657 articles. The paper published in the Journal of the American Medical Association by Ting, DSW, Wong, TY, et al., which has been cited 1,369 times, ranks first (20). This article is the most frequently cited within this field of research.
3.4 High-frequency keyword analyses
We conducted a systematic extraction of keywords from 3,581 articles, and the top 25 with highest occurrences are listed (Table 2). While identifying thematic areas in fields of investigation, a closer examination of these keywords used in the papers revealed that 25 keywords occurred at least 125 times.
We used the VOSviewer to analyze the relationships between high-frequency keywords in the literature and performed visual processing. From the perspective of high-frequency keywords, six popular research topics were summarized: (1) correlation between optic disc morphology development in glaucoma and age; (2) relationship between risk factors, such as elevated intraocular pressure and open-angle glaucoma; (3) association and variations in the prevalence and risk of eye diseases across different populations; (4) differentiation of ocular injuries using optical coherence tomography (OCT); (5) progression of normal-tension glaucoma; and (6) research on the diagnosis, classification, and image segmentation of DR (Figure 2).
3.5 Burst keywords analyses
Keywords reflect current research interests, whereas burst keywords highlight emerging trends and leading edges in research. We used CiteSpace to analyze trends over time in hotspot shifts, making use of the most common keywords showing the most robust citation bursts (17). Among these were eye diseases (2021–2024), retinal fundus images (2022–2024), and risk factors (2022–2024). The most concentrated studies in these hotspots began in 2016 and continue to the present. These burst keywords emerged around 2020 or 2021 and continued to be a research hotspot until 2024. The shift in keywords reflects the change in the research focus of researchers in the field and the development process of the field. As shown in Figure 3, in the field of glaucoma research facilitated by machine learning technology, the keywords published in the literature have transitioned from terms such as linear regression model, glaucomatous eyes, multivariate analysis, axial length, spherical equivalent, and normal eyes, to eye diseases (2021–2024), retinal fundus images (2022–2024), and risk factors (2022–2024). This indicates that retinal fundus imaging technology is becoming popular, and the study of risk factors for eye diseases is becoming increasingly important (Figure 3).
4 Discussion
4.1 General data
By examining the selected articles, a total of 3,581 Science Citation Index Expanded (SCIE) papers on the application of machine learning in glaucoma were published from 2000 to 2024, demonstrating a rapid growth trend. The US contributed the largest number of articles, 1,141 (32.1 %), followed by China, 664 (18.5%). Among the top 10 leading institutions, four were based in the US and three in the UK, highlighting the significant role of researchers from these countries in advancing the field. Ophthalmology, which was the published journal most referred to (16,334 times), thereby greatly promoting the study of machine learning in glaucoma. Additionally, we looked into the 10 most-cited published articles, and the top paper was by Ting et al. (20) and published in the Journal of the American Medical Association, which had 1,369 citations. This may indicate that the paper could be a foundational or pivotal work in the field, providing essential evidence for ongoing research. These frequently acknowledged studies may provide valuable insight into this field of research.
4.2 The current research characteristics
The intricate and multidimensional interplay within the knowledge domain of machine learning in glaucoma warrants further examination to elucidate its conceptual structure. By analyzing the frequency and co-occurrence of high-frequency keywords, we can identify the thematic composition of research patterns and provide insights into future developments within the industry. We have summarized the research hotspots of machine learning in the field of glaucoma, which can be divided into six different perspectives.
4.2.1 Correlation between the development of optic disc morphology in glaucoma and age
Machine learning technology has made significant progress in exploring the relationship between optic disc morphology and age in glaucoma. Initially, Omodaka et al. used “machine learning based on quantified eye parameters” to classify the shape of the optic disc in glaucoma, using age as one of the most important distinguishing features (21). With advancements in DL technology, researchers have discovered a positive correlation between aging and optic disc parameters, particularly an increase in the vertical cup-to-plate ratio, which is a key risk factor for glaucoma (22). By integrating machine learning with genomic data, models have identified genetic loci linked to the vertical cup-to-plate ratio, underscoring aging's pivotal role in glaucoma pathogenesis, while demonstrating robust predictive performance for glaucoma subtypes (AUCs: 0.74 for POAG, 0.73 for HTG, and 0.76 for NTG) in independent validation cohorts (23). These studies not only deepen the understanding of the pathological mechanisms of glaucoma but also provide valuable insights for early diagnosis and personalized treatment.
4.2.2 Relationship between risk factors, such as elevated intraocular pressure and open-angle glaucoma
In the study of open-angle glaucoma (OAG), the application of machine learning techniques identified multiple risk factors associated with disease progression. These include the rate of optic nerve fiber layer thinning, the association between smoking and disease progression, and the association between intraocular pressure and OAG risk (24–26). Additionally, machine learning technology identified abnormal cardiac contractile potential in patients with OAG, providing a new perspective on the systemic effects of OAG (27). Moreover, machine learning demonstrated the potential for the early prediction of OAG through the analysis of electronic health records, identifying ocular hypertension as a key indicator. This highlighted the crucial role of machine learning in early diagnosis (28). Machine learning models can utilize clinical characteristics to predict the risk of ocular hypertension following silicone oil tamponade (29), achieving a highest accuracy of 0.7944 with the gradient-boosted decision trees model. These findings underscore the key role of machine learning technology in the early diagnosis and management of glaucoma.
4.2.3 Association and differences in the risk and prevalence of eye diseases among different populations
Machine learning technology has significantly contributed to the diagnosis, genetic research, screening, management, and epidemiology of glaucoma. The application of DL technology has facilitated the early identification of metabolic risk factors, which may aid in the early detection of glaucoma risk (27). Machine learning has made significant advancements in automatically evaluating optic disc parameters, greatly improving diagnostic efficiency and accuracy through convolutional neural network (CNN) models. Additionally, machine learning-assisted genome-wide association studies have revealed a large number of genetic loci associated with glaucoma, offering new insights into the disease's genetic background (30). Machine learning technology has improved screening and detection capabilities for primary angle-closure glaucoma (31, 32). In patients with Parkinson's disease, machine learning technology has been utilized to assess visual dysfunction, further expanding its application in the medical field (33). These advancements not only improve research efficiency and accuracy but also provide new tools and methods for the early detection, intervention, and treatment of glaucoma.
4.2.4 Differentiation of ocular injuries using OCT
The combination of machine learning techniques with OCT has revolutionized the study of the thickness assessment of the nerve fiber layer and its correlation with damage in the VF. Research has progressed from simple correlation analyses to the use of neural networks and DL algorithms to improve diagnostic accuracy (34, 35). Following the unsupervised AI for identifying the pattern of nerve fiber layer thickness associated with VF loss from OCT images, the weakly supervised DL enabled the automatic segmentation of single retinal ganglion cells in adaptive optical OCT images (36, 37). These advancements not only improve the accuracy of glaucoma diagnosis but also provide powerful tools for the early detection and personalized treatment of eye diseases.
4.2.5 Progression of normal-tension glaucoma
Machine learning techniques are playing an increasingly vital role in the diagnosis, classification, progression prediction, and risk assessment of normal-tension glaucoma (NTG). With DL systems, researchers have utilized pre-trained models such as EfficientNet-b0 to achieve high accuracy (AUC = 0.98) and specificity (0.94) in NTG screening and classification (38). By integrating retinal images with clinical data, DL models have effectively predicted both the likelihood and timing of NTG conversion in normotensive glaucoma suspects, demonstrating exceptional performance (AUC = 0.994) (39). Additionally, machine learning classifiers showed superior capability in predicting NTG progression (AUC = 0.881) among young myopic patients using baseline clinical parameters alone (40). Furthermore, the combination of machine-learning algorithms with OCT angiography has provided a new perspective for NTG diagnosis and severity classification (41). A prospective cohort study on retinal vascular caliber, utilizing DL analysis, has provided a novel approach to assess factors associated with NTG progression risk (42).
4.2.6 Research on the diagnosis, classification, and image segmentation of DR
The integration of DL and image processing technologies significantly improves the accuracy and efficiency of DR diagnosis. For example, a model combining pre-processing techniques and CNN demonstrated excellent performance in identifying DR, achieving an accuracy of up to 98.56% (43, 44). Further research on the depth of the preliminary training CNN model, such as EfficientNet-B7, has enabled effective multi-class classification of DR, achieving an accuracy of over 96% (45). Additionally, multi-scale residual attention networks have outperformed traditional models in retinal vascular segmentation, improving segmentation accuracy (46). Feature fusion technology, integrating wavelet packet transforms and intensity-hue-saturation features, has used a Support Vector Machine for classification to further enhance the classification performance for eye diseases (47). These studies not only promote the early diagnosis of the DR but also provide new perspectives and methods for treatment.
4.3 Hotspots and frontiers in research
Keywords focus on contemporary research concepts or issues while representing emerging trends and the leading edge of research. This shift in keywords reflects the evolution of the research topics. In this study, we identified hot keywords that cover the research frontiers of the subject of interest. These include eye diseases (2021–2024), retinal fundus images (2022–2024), and risk factors (2022–2024).
4.3.1 Eye diseases (2021–2024)
The application of machine learning in the field of eye disease diagnosis is gradually revolutionizing traditional medical methods, not only improving the speed and accuracy of diagnosis but also providing new perspectives on disease management and treatment.
DL techniques, particularly CNN, have demonstrated high efficiency in identifying eye diseases, such as glaucoma and DR, by analyzing medical images (48). Furthermore, by integrating multimodal data, including retinal images with OCT, machine learning can provide a more comprehensive analysis of the disease and enhance diagnostic accuracy (49).
The advantages of machine learning technology are also reflected in the processing of unbalanced datasets. By modifying the loss functions, the machine learning model can effectively improve eye disease detection performance, even in unbalanced data or in the presence of outliers (50). The CNN model optimized using the pollen optimization algorithm has improved the accuracy of diagnosing diseases, such as glaucoma and cataracts in the classification of eye diseases, thereby facilitating the early detection of ophthalmic conditions (51). Furthermore, its combination with a long short-term memory network has effectively reduced errors in functional VF tests (52).
In the context of explaining the database used for training and the automated training of machine learning models, the use of generative adversarial networks for the synthesis of fundus images can meet the current limitations of the shortage of medical images and the high costs of image annotation for the rapid training of relevant machine learning diagnostic models. Integrating this with the transparency and systematization of machine learning diagnostic attention mechanisms can enhance the credibility of machine learning diagnostics, particularly in the field of ophthalmic imaging and diagnostics, where these advancements have broad applications (53). Another key application of machine learning is predicting disease progression and evaluating treatment effects, enabling personalized medical recommendations to patients. The DL model utilizes segmented OCT images to extract parameters for predicting functional VF test values. The integration of multimodal inputs can predict the progression of glaucoma. A machine learning model can also simulate glaucoma progression based on historical patient data, revealing the disease development patterns in different patients (52).
4.3.2 Retinal fundus image (2022–2024)
The value of retinal fundus imaging as a core tool in the diagnosis of eye diseases has been significantly enhanced by DL technology. DL models, particularly CNNs, have been widely applied to the automated analysis of retinal fundus images, thereby improving the detection and diagnosis of major retinal diseases, such as glaucoma and DR. For example, a study integrating CNN with a visual converter significantly improved the performance of the classification of retinopathy (54). Additionally, using transfer learning and fine-tuning techniques, these models can be further optimized for specific retinal fundus image classification tasks after pre-training on public datasets such as ImageNet, achieving superior diagnostic accuracy.
Innovations in DL models continue to advance. For example, an attention-based fully CNN has been proposed for the precise segmentation of retinal blood vessels (55). This demonstrates that DL techniques can not only accelerate diagnosis but also enhance the accuracy of diagnosis by focusing on key regions within the image. Some studies have focused on image enhancement and pre-processing techniques to improve the quality of retinal fundus images, further improving model performance.
Despite the significant potential of DL in the diagnosis of eye diseases, researchers have highlighted several challenges that must be addressed. The diversity and representativeness of datasets, the ability to generalize models, and the requirement for clinical validation are current research priorities. For instance, one study combined 22 publicly available datasets to address the issue of unbalanced classes and improve the medical effectiveness of a model (56). Additionally, methods of multimodal data fusion are also being explored, integrating retinal fundus images with other imaging modalities, such as OCT, to provide a more comprehensive assessment of the disease.
4.3.3 Risk factors (2022–2024)
Machine learning is playing an increasingly vital role in glaucoma risk factor analysis, early diagnosis, disease progression prediction, and patient adherence to treatment. Machine learning models are of great significance in the field of disease risk alerts, owing to their outstanding data processing and image recognition capabilities.
Through DL and multimodal recognition, machine learning can process complex medical data and identify key risk factors, such as video disc images (57). Furthermore, machine learning models can analyze surgical data to predict surgical outcomes, aiding clinical decision-making (5, 58).
The predictive power of machine learning is particularly prominent in the early detection and monitoring of glaucoma. For example, the DL model automatically evaluates the ultrasound images of biological microscopes, identifies relevant biological risk factors, and assists in the early detection of glaucoma (59). Simultaneously, by analyzing clinical records through natural language processing, machine learning can detect disease progression and predict the requirement for surgical intervention, thereby avoiding surgical risks (60).
To improve patient treatment adherence, machine learning analyzes individual differences and provides customized treatment recommendations to physicians, thereby increasing the likelihood of patients following their prescribed treatment plans (61).
The application of machine learning in glaucoma not only enhances the understanding of risk factors for glaucoma but also optimizes the diagnostic process and provides more precise medical interventions for patients.
4.4 Future perspectives
The integration of multimodal technology into glaucoma research offers significant potential for personalized treatment and enhanced patient outcomes. While single-mode OCT data already enables high-precision diagnosis, the combination of multimodal data may further enhance model generalization (15). Li et al. developed a multimodal DL framework that combines clinical data, VF measurements, and OCT images of 86 patients with glaucoma. This framework prognosticated glaucoma progression in VF at 12 months with an optimal area under the curve of 0.83 (62). Notably, the integration of multimodal images enabled the model to forecast vision loss with greater precision and at earlier onset. Another study introduced a new multimodal neural network designed to create a comprehensive dataset for glaucoma diagnosis and classification. To address the shortage of training data, transfer learning has also been integrated to overcome data scarcity, enabling a more effective representation of high-dimensional glaucoma-related information and enhancing model performance in multimodal analysis (52). Future studies need to further integrate multi-modal data and expand sample diversity to improve the generalization ability of the model. Bibliometric research also shows that interdisciplinary collaboration, such as computer engineering and ophthalmology, is the key to promoting the implementation of AI diagnostic systems (63).
The application of large language models (LLMs) in glaucoma management has progressed significantly, enabling rapid clinical note analysis and the extraction of critical data, such as family history, which is essential for predicting the requirement for surgical intervention. Studies indicate that LLMs achieved over 80% accuracy in predicting the probability of surgery within 1 year, thereby aiding targeted therapeutic planning to preserve visual acuity. While not infallible, they have demonstrated greater precision than traditional assessment methods in certain cases (64). A recent cross-sectional study comparing the diagnostic efficacy of an LLM chatbot (Generative Pre-trained Transformer-4) with that of glaucoma and retinal specialists has demonstrated the superior performance of LLMs in medical accuracy and response comprehensiveness, as evaluated using a Likert scale with statistical validation. These findings indicated that LLMs have the potential to enhance ophthalmological diagnostics and be integrated into clinical practice, provided they undergo further refinement and extensive patient testing. The ongoing development and validation of LLMs are essential to ensure their reliability and effectiveness in real-world settings, ultimately aiming to improve glaucoma patient care and outcomes (65).
Vision transformers (ViTs) are emerging as a breakthrough in glaucoma diagnosis, addressing challenges such as data scarcity and subtle feature discrimination. Tohye et al. propose CA-ViTs, integrating Conditional Variational GAN (CVGAN) for data augmentation and contour-guided optic disc/cup extraction, achieving 93% accuracy in multi-class classification on the SMDC dataset. This framework outperforms CNNs and vanilla ViTs (p < 0.01), demonstrating ViTs' potential for precise severity grading (66). Future studies include leveraging diffusion models and multi-modal data fusion to enhance diagnostic robustness.
4.5 Limitation
This study has some limitations. The reliance on SCIE-indexed publications may exclude relevant studies from non-indexed regional journals or preprints. Future studies should incorporate a broader range of databases, including non-English and regional sources, to provide a more comprehensive perspective on global research trends. AI, genetics, and personalized medicine could refine predictive models and treatment strategies.
This study acknowledges certain limitations in machine learning applications for glaucoma. First, the interpretability of complex models, such as deep learning, remains challenging, potentially hindering clinical trust. Second, the current generalization of machine learning models in glaucoma research is still limited by data standardization issues, such as color and resolution differences between different imaging devices (67). Third, biases in training data, such as fundus photography studies, mainly focus on specific populations, so it is especially important to be avoided (68). Addressing these limitations requires future efforts in model transparency, diverse dataset curation, and rigorous cross-validation.
5 Conclusion
A comprehensive, systematic, and impartial study was conducted on articles published on the application of machine learning in glaucoma research from 2000 to 1 April 2024, along with a scope review. Notably, there has been a significant surge in scholarly output post-2016, with the United States, China, England, and South Korea emerging as leading contributors to this evolving field. The thematic categorization of research has divided the multifaceted focus into six pivotal areas, with “Eye diseases”, “Retinal fundus image,” and “Risk factors” emerging as the developing directions in this field. The study's findings highlighted the broad prospects of machine learning in improving the diagnosis and treatment of glaucoma, emphasizing the requirement for ongoing research, interdisciplinary integration, and cross-disciplinary collaboration to enhance patient care and prioritize the prevention of risk factors.
Data availability statement
The original contributions presented in the study are included in the article/Supplementary material, further inquiries can be directed to the corresponding author.
Author contributions
TF: Conceptualization, Data curation, Funding acquisition, Methodology, Project administration, Supervision, Writing – review & editing. JZ: Visualization, Writing – original draft. BT: Visualization, Writing – original draft. MT: Resources, Software, Writing – original draft. XS: Investigation, Writing – original draft. JL: Formal analysis, Writing – original draft.
Funding
The author(s) declare that financial support was received for the research and/or publication of this article. This study was funded by “Medical and health big data analysis and decision-making platform based on artificial intelligence” (2900021010-CMU-010).
Acknowledgments
The authors thank the Web of Science database for its data support, which enabled us to summarize and analyze the most cutting-edge scientific research results.
Conflict of interest
The authors declare that the research was conducted in the absence of any commercial or financial relationships that could be construed as a potential conflict of interest.
Generative AI statement
The author(s) declare that no Gen AI was used in the creation of this manuscript.
Publisher's note
All claims expressed in this article are solely those of the authors and do not necessarily represent those of their affiliated organizations, or those of the publisher, the editors and the reviewers. Any product that may be evaluated in this article, or claim that may be made by its manufacturer, is not guaranteed or endorsed by the publisher.
Supplementary material
The Supplementary Material for this article can be found online at: https://www.frontiersin.org/articles/10.3389/fmed.2025.1573329/full#supplementary-material
References
1. Wu JH, Moghimi S, Nishida T, Mahmoudinezhad G, Zangwill LM, Weinreb RN. Detection and agreement of event-based OCT and OCTA analysis for glaucoma progression. Eye. (2024) 38:973–9. doi: 10.1038/s41433-023-02817-0
2. Pesudovs K, Lansingh VC, Kempen JH, Tapply I, Fernandes AG, Cicinelli MV, et al. Global estimates on the number of people blind or visually impaired by cataract: a meta-analysis from 2000 to 2020. Eye. (2024) 38:2156–72. doi: 10.1038/s41433-024-02961-1
3. McLaughlin DE, Semrov A, Munshi H, Patel AJ, Rahi J, Grajewski AL, et al. The impact of childhood glaucoma on psychosocial functioning and quality of life: a review of the literature. Eye. (2023) 37:3157–73. doi: 10.1038/s41433-023-02492-1
4. Tham Y-C, Li X, Wong TY, Quigley HA, Aung T, Cheng C-Y. Global prevalence of glaucoma and projections of glaucoma burden through 2040: a systematic review and meta-analysis. Ophthalmology. (2014) 121:2081–90. doi: 10.1016/j.ophtha.2014.05.013
5. Lee SY, Lee DY, Ahn J. Evaluation of machine learning approach for surgical results of Ahmed valve implantation in patients with glaucoma. BMC Ophthalmol. (2024) 24:11. doi: 10.1186/s12886-024-03510-w
6. Abràmoff MD, Lou YY, Erginay A, Clarida W, Amelon R, Folk JC, et al. Improved automated detection of diabetic retinopathy on a publicly available dataset through integration of deep learning. Invest Ophthalmol Vis Sci. (2016) 57:5200–6. doi: 10.1167/iovs.16-19964
7. Gulshan V, Peng L, Coram M, Stumpe MC, Wu D, Narayanaswamy A, et al. Development and validation of a deep learning algorithm for detection of diabetic retinopathy in retinal fundus photographs. JAMA-J Am Med Assoc. (2016) 316:2402–10. doi: 10.1001/jama.2016.17216
8. Nuzzi R, Boscia G, Marolo P, Ricardi F. The impact of artificial intelligence and deep learning in eye diseases: a review. Front Med. (2021) 8:11. doi: 10.3389/fmed.2021.710329
9. Schmidt-Erfurth U, Sadeghipour A, Gerendas BS, Waldstein SM, Bogunovic H. Artificial intelligence in retina. Prog Retin Eye Res. (2018) 67:1–29. doi: 10.1016/j.preteyeres.2018.07.004
10. Vujosevic S, Aldington SJ, Silva P, Hernández C, Scanlon P, Peto T, et al. Screening for diabetic retinopathy: new perspectives and challenges. Lancet Diabetes Endocrinol. (2020) 8:337–47. doi: 10.1016/S2213-8587(19)30411-5
11. Ji YK, Chen N, Liu S, Yan ZP, Qian H, Zhu SJ, et al. Research progress of artificial intelligence image analysis in systemic disease-related ophthalmopathy. Dis Markers. (2022) 2022:10. doi: 10.1155/2022/3406890
12. Thomas PBM, Chan T, Nixon T, Muthusamy B, White A. Feasibility of simple machine learning approaches to support detection of non-glaucomatous visual fields in future automated glaucoma clinics. Eye. (2019) 33:1133–9. doi: 10.1038/s41433-019-0386-2
13. Andreou PA, Wickremasinghe SS, Asaria RH, Tay E, Franks WA. A comparison of HRT II and GDx imaging for glaucoma detection in a primary care eye clinic setting. Eye. (2007) 21:1050–5. doi: 10.1038/sj.eye.6702394
14. Zhu SJ, Lu B, Wang CH, Wu MN, Zheng B, Jiang Q, et al. Screening of common retinal diseases using six-category models based on efficientnet. Front Med-Lausanne. (2022) 9:9. doi: 10.3389/fmed.2022.808402
15. Escamez CSF, Giral EM, Martinez SP, Fernandez NT. High interpretable machine learning classifier for early glaucoma diagnosis. Int J Ophthalmol. (2021) 14:393–8. doi: 10.18240/ijo.2021.03.10
16. Girard MJA, Schmetterer L. Artificial intelligence and deep learning in glaucoma: current state and future prospects. In:Bagetta G, Nucci C, , editors. Glaucoma: A Neurodegenerative Disease of the Retina and Beyond - Pt B. Progress in Brain Research. Amsterdam: Elsevier (2020). p. 37–64.
17. Wang RY, Zuo GX, Li KK, Li WT, Xuan ZQ, Han YZ, et al. Systematic bibliometric and visualized analysis of research hotspots and trends on the application of artificial intelligence in diabetic retinopathy. Front Endocrinol. (2022) 13:13. doi: 10.3389/fendo.2022.1036426
18. Mirzania D, Thompson AC, Muir KW. Applications of deep learning in detection of glaucoma: a systematic review. Eur J Ophthalmol. (2021) 31:1618–42. doi: 10.1177/1120672120977346
19. Kokol P. Synthetic knowledge synthesis in hospital libraries. J Hosp Librariansh. (2024) 24:10–7. doi: 10.1080/15323269.2023.2291282
20. Ting DSW, Cheung CYL, Lim G, Tan GSW, Quang ND, Gan A, et al. Development and validation of a deep learning system for diabetic retinopathy and related eye diseases using retinal images from multiethnic populations with diabetes. JAMA-J Am Med Assoc. (2017) 318:2211–23. doi: 10.1001/jama.2017.18152
21. Omodaka K, An G, Tsuda S, Shiga Y, Takada N, Kikawa T, et al. Classification of optic disc shape in glaucoma using machine learning based on quantified ocular parameters. PLoS ONE. (2017) 12:e0190012. doi: 10.1371/journal.pone.0190012
22. Shin J, Kang MS, Park K, Lee JS. Association between metabolic risk factors and optic disc cupping identified by deep learning method. PLoS One. (2020) 15:11. doi: 10.1371/journal.pone.0239071
23. Alipanahi B, Hormozdiari F, Behsaz B, Cosentino J, McCaw ZR, Schorsch E, et al. Large-scale machine-learning-based phenotyping significantly improves genomic discovery for optic nerve head morphology. Am J Hum Genet. (2021) 108:1217–30. doi: 10.1016/j.ajhg.2021.05.004
24. Lee EJ, Kim TW, Weinreb RN, Park KH, Kim SH, Kim DM. β-Zone parapapillary atrophy and the rate of retinal nerve fiber layer thinning in glaucoma. Invest Ophthalmol Vis Sci. (2011) 52:4422–7. doi: 10.1167/iovs.10-6818
25. Wang DG, Huang YQ, Huang CK, Wu PF, Lin JW, Zheng YQ, et al. Association analysis of cigarette smoking with onset of primary open-angle glaucoma and glaucoma-related biometric parameters. BMC Ophthalmol. (2012) 12:5. doi: 10.1186/1471-2415-12-59
26. Topouzis F, Wilson MR, Harris A, Founti P, Yu F, Anastasopoulos E, et al. Association of open-angle glaucoma with perfusion pressure status in the thessaloniki eye study. Am J Ophthalmol. (2013) 155:843–51. doi: 10.1016/j.ajo.2012.12.007
27. Suzuki Y, Kiyosawa M. Cardiac hypertrophy may be a risk factor for the development and severity of glaucoma. Biomedicines. (2022) 10:11. doi: 10.3390/biomedicines10030677
28. Raju M, Shanmugam KP, Shyu CR. Application of machine learning predictive models for early detection of glaucoma using real world data. Appl Sci-Basel. (2023) 13:12. doi: 10.3390/app13042445
29. Fan W, Zhang CH, Ge LX, Su N, Chen JQ, Song SY, et al. Prediction model for elevated intraocular pressure risk after silicone oil filling based on clinical features. Front Med. (2024) 10:9. doi: 10.3389/fmed.2023.1340198
30. Han XK, Steven K, Qassim A, Marshall HN, Bean C, Tremeer M, et al. Automated Al labeling of optic nerve head enables insights into cross-ancestry glaucoma risk and genetic discovery in >280,000 images from UKB and CLSA. Am J Hum Genet. (2021) 108:1204–16. doi: 10.1016/j.ajhg.2021.05.005
31. Niu WR, Dong CQ, Zhang X, Feng YF, Yuan F. Ocular biometric characteristics of chinese with history of acute angle closure. J Ophthalmol. (2018) 2018:6. doi: 10.1155/2018/5835791
32. Chou CC, Shih PJ, Wang CY, Jou TS, Chen JP, Wang IJ. Corvis biomechanical factor facilitates the detection of primary angle closure glaucoma. Transl Vis Sci Technol. (2022) 11:8. doi: 10.1167/tvst.11.10.7
33. Hamedani AG, Willis AW. Self-reported visual dysfunction in parkinson disease: the survey of health, ageing and retirement in Europe. Eur J Neurol. (2020) 27:484–9. doi: 10.1111/ene.14092
34. El Beltagi TA, Bowd C, Boden C, Amini P, Sample PA, Zangwill LM, et al. Retinal nerve fiber layer thickness measured with optical coherence tomography is related to visual function in glaucomatous eyes. Ophthalmology. (2003) 110:2185–91. doi: 10.1016/S0161-6420(03)00860-1
35. (44)) Park K, Kim J, Lee J. The relationship between bruch's membrane opening-minimum rim width and retinal nerve fiber layer thickness and a new index using a neural network. Transl Vis Sci Technol. (2018) 7:17. doi: 10.1167/tvst.7.4.14
36. Wang MY, Shen LQ, Pasquale LR, Wang H, Li D, Choi EY, et al. An artificial intelligence approach to assess spatial patterns of retinal nerve fiber layer thickness maps in glaucoma. Transl Vis Sci Technol. (2020) 9:12. doi: 10.1167/tvst.9.9.41
37. Soltanian-Zadeh S, Kurokawa K, Liu ZL, Zhang FR, Saeedi O, Hammer DX, et al. Weakly supervised individual ganglion cell segmentation from adaptive optics OCT images for glaucomatous damage assessment. Optica. (2021) 8:642–51. doi: 10.1364/OPTICA.418274
38. Hung KH, Kao YC, Tang YH, Chen YT, Wang CH, Wang YC, et al. Application of a deep learning system in glaucoma screening and further classification with colour fundus photographs: a case control study. BMC Ophthalmol. (2022) 22:12. doi: 10.1186/s12886-022-02730-2
39. Ha A, Sun S, Kim YK, Jeoung JW, Kim HC, Park KH. Deep-learning-based prediction of glaucoma conversion in normotensive glaucoma suspects. Br J Ophthalmol. (2024) 108:927–32. doi: 10.1136/bjo-2022-323167
40. Lee J, Kim YK, Jeoung JW, Ha A, Kim YW, Park KH. Machine learning classifiers-based prediction of normal-tension glaucoma progression in young myopic patients. Jpn J Ophthalmol. (2020) 64:68–76. doi: 10.1007/s10384-019-00706-2
41. De Jesus DA, Brea LS, Breda JB, Fokkinga E, Ederveen V, Borren N, et al. Octa multilayer and multisector peripapillary microvascular modeling for diagnosing and staging of glaucoma. Transl Vis Sci Technol. (2020) 9:22. doi: 10.1167/tvst.9.2.58
42. Lin TPH, Hui HYH, Ling AN, Chan PP, Shen RY, Wong MOM, et al. Risk of normal tension glaucoma progression from automated baseline retinal-vessel caliber analysis: a prospective cohort study. Am J Ophthalmol. (2023) 247:111–20. doi: 10.1016/j.ajo.2022.09.015
43. Mayya V, Kamath SS, Kulkarni U, Surya DK, Acharya UR. An empirical study of preprocessing techniques with convolutional neural networks for accurate detection of chronic ocular diseases using fundus images. Appl Intell. (2023) 53:1548–66. doi: 10.1007/s10489-022-03490-8
44. Murugan R, Roy P, Singh U. An abnormality detection of retinal fundus images by deep convolutional neural networks. Multimed Tools Appl. (2020) 79:24949–67. doi: 10.1007/s11042-020-09217-6
45. Vadduri M, Kuppusamy P. Enhancing ocular healthcare: deep learning-based multi-class diabetic eye disease segmentation and classification. IEEE Access. (2023) 11:137881–98. doi: 10.1109/ACCESS.2023.3339574
46. Jiang Y, Yao HX, Wu C, Liu WH. A multi-scale residual attention network for retinal vessel segmentation. Symmetry-Basel. (2021) 13:16. doi: 10.3390/sym13101820
47. Keerthiveena B, Esakkirajan S, Selvakumar K, Yogesh T. Computer-aided diagnosis of retinal diseases using multidomain feature fusion. Int J Imaging Syst Technol. (2020) 30:367–79. doi: 10.1002/ima.22379
48. Bali A, Mansotra V. Analysis of deep learning techniques for prediction of eye diseases: a systematic review. Arch Comput Method Eng. (2024) 31:487–520. doi: 10.1007/s11831-023-09989-8
49. Thakoor KA, Koorathota SC, Hood DC, Sajda P. Robust and interpretable convolutional neural networks to detect glaucoma in optical coherence tomography images. IEEE Trans Biomed Eng. (2021) 68:2456–66. doi: 10.1109/TBME.2020.3043215
50. Luo X, Li JY, Chen MJ, Yang X, Li XJ. Ophthalmic disease detection via deep learning with a novel mixture loss function. IEEE J Biomed Health Inform. (2021) 25:3332–9. doi: 10.1109/JBHI.2021.3083605
51. Rathakrishnan N, Raja D. Optimized convolutional neural network-based comprehensive early diagnosis method for multiple eye disease recognition. J Electron Imaging. (2022) 31:21. doi: 10.1117/1.JEI.31.4.043016
52. Hussain S, Chua J, Wong D, Lo J, Kadziauskiene A, Asoklis R, et al. Predicting glaucoma progression using deep learning framework guided by generative algorithm. Sci Rep. (2023) 13:14. doi: 10.1038/s41598-023-46253-2
53. Li T, Bo W, Hu CY, Kang H, Liu HR, Wang K, et al. Applications of deep learning in fundus images: a review. Med Image Anal. (2021) 69:32. doi: 10.1016/j.media.2021.101971
54. Lian J, Liu TY. Lesion identification in fundus images via convolutional neural network-vision transformer. Biomed Signal Process Control. (2024) 88:9. doi: 10.1016/j.bspc.2023.105607
55. Gobinath C, Gopinath MP. Attention aware fully convolutional deep learning model for retinal blood vessel segmentation. J Intell Fuzzy Syst. (2023) 44:6413–23. doi: 10.3233/JIFS-224229
56. Chlopowiec AR, Karanowski K, Skrzypczak T, Grzesiuk M, Chlopowiec AB, Tabakov M. Counteracting data bias and class imbalance-towards a useful and reliable retinal disease recognition system. Diagnostics. (2023) 13:16. doi: 10.21203/rs.3.rs-2391402/v1
57. Kamal MS, Dey N, Chowdhury L, Hasan SI, Santosh KC. Explainable AI for glaucoma prediction analysis to understand risk factors in treatment planning. IEEE Trans Instrum Meas. (2022) 71:9. doi: 10.1109/TIM.2022.3171613
58. Ul Banna H, Zanabli A, McMillan B, Lehmann M, Gupta S, Gerbo M, et al. Evaluation of machine learning algorithms for trabeculectomy outcome prediction in patients with glaucoma. Sci Rep. (2022) 12:11. doi: 10.1038/s41598-022-06438-7
59. Jiang WY, Yan YL, Cheng SM, Wan SS, Huang LY, Zheng HM, et al. Deep learning-based model for automatic assessment of anterior angle chamber in ultrasound biomicroscopy. Ultrasound Med Biol. (2023) 49:2497–509. doi: 10.1016/j.ultrasmedbio.2023.08.013
60. Hu W, Wang SY. Predicting glaucoma progression requiring surgery using clinical free-text notes and transfer learning with transformers. Transl Vis Sci Technol. (2022) 11:10. doi: 10.1167/tvst.11.3.37
61. Karimi A, Stanik A, Kozitza C, Chen AY. Integrating deep learning with electronic health records for early glaucoma detection: a multi-dimensional machine learning approach. Bioengineering-Basel. (2024) 11:20. doi: 10.3390/bioengineering11060577
62. Li Y, Han YJ Li ZH, Zhong Y, Guo ZF. A Transfer learning-based multimodal neural network combining metadata and multiple medical images for glaucoma type diagnosis. Sci Rep. (2023) 13:13. doi: 10.1038/s41598-022-27045-6
63. Zhao JQ, Lu Y, Zhu SJ, Li KR, Jiang Q, Yang WH. Systematic bibliometric and visualized analysis of research hotspots and trends on the application of artificial intelligence in ophthalmic disease diagnosis. Front Pharmacol. (2022) 13:12. doi: 10.3389/fphar.2022.930520
64. Jin K, Yuan L, Wu HK, Grzybowski A, Ye J. Exploring large language model for next generation of artificial intelligence in ophthalmology. Front Med. (2023) 10:9. doi: 10.3389/fmed.2023.1291404
65. Huang AS, Hirabayashi K, Barna L, Parikh D, Pasquale LR. Assessment of a large language model's responses to questions and cases about glaucoma and retina management. JAMA Ophthalmol. (2024) 142:371–5. doi: 10.1001/jamaophthalmol.2023.6917
66. Tohye TG, Qin Z. Al-antari MA, Ukwuoma CC, Lonseko ZM, Gu YH. Ca-ViT: contour-guided and augmented vision transformers to enhance glaucoma classification using fundus images. Bioengineering. (2024) 11:23. doi: 10.3390/bioengineering11090887
67. Liu YH, Li LY, Liu SJ, Gao LX, Tang Y, Li ZH, et al. Artificial intelligence in the anterior segment of eye diseases. Int J Ophthalmol. (2024) 17:1743–51. doi: 10.18240/ijo.2024.09.23
68. Murtagh P, Greene G, O'Brien C. Current applications of machine learning in the screening and diagnosis of glaucoma: a systematic review and meta-analysis. Int J Ophthalmol-Chi. (2020) 13:149–62. doi: 10.18240/ijo.2020.01.22
69. Abràmoff MD, Lavin PT, Birch M, Shah N, Folk JC. Pivotal trial of an autonomous AI-based diagnostic system for detection of diabetic retinopathy in primary care offices. NPJ Digit Med. (2018) 1:8. doi: 10.1038/s41746-018-0040-6
70. Fu HZ, Cheng J, Xu YW, Wong DWK, Liu J, Cao XC. Joint optic disc and cup segmentation based on multi-label deep network and polar transformation. IEEE Trans Med Imaging. (2018) 37:1597–605. doi: 10.1109/TMI.2018.2791488
71. Jia YL, Wei E, Wang XG, Zhang XB, Morrison JC, Parikh M, et al. Optical coherence tomography angiography of optic disc perfusion in glaucoma. Ophthalmology. (2014) 121:1322–32. doi: 10.1016/j.ophtha.2014.01.021
72. Nouri-Mahdavi K, Hoffman D, Coleman AL, Liu G, Li G, Gaasterland D, et al. Predictive factors for glaucomatous visual field progression in the advanced glaucoma intervention study. Ophthalmology. (2004) 111:1627–35. doi: 10.1016/j.ophtha.2004.02.017
73. Budenz DL, Anderson DR, Varma R, Schuman J, Cantor L, Savell J, et al. Determinants of normal retinal nerve fiber layer thickness measured by stratus OCT. Ophthalmology. (2007) 114:1046–52. doi: 10.1016/j.ophtha.2006.08.046
74. Liu L, Jia YL, Takusagawa HL, Pechauer AD, Edmunds B, Lombardi L, et al. Optical coherence tomography angiography of the peripapillary retina in glaucoma. JAMA Ophthalmol. (2015) 133:1045–52. doi: 10.1001/jamaophthalmol.2015.2225
75. Li ZX, He YF, Keel S, Meng W, Chang RT, He MG. Efficacy of a deep learning system for detecting glaucomatous optic neuropathy based on color fundus photographs. Ophthalmology. (2018) 125:1199–206. doi: 10.1016/j.ophtha.2018.01.023
76. Leung EW, Medeiros FA, Weinreb RN. Prevalence of ocular surface disease in glaucoma patients. J Glaucoma. (2008) 17:350–5. doi: 10.1097/IJG.0b013e31815c5f4f
Keywords: machine learning, glaucoma diagnosis, deep learning, multimodal imaging, ophthalmic research
Citation: Zhang J, Tian B, Tian M, Si X, Li J and Fan T (2025) A scoping review of advancements in machine learning for glaucoma: current trends and future direction. Front. Med. 12:1573329. doi: 10.3389/fmed.2025.1573329
Received: 08 February 2025; Accepted: 03 April 2025;
Published: 24 April 2025.
Edited by:
Weihua Yang, Southern Medical University, ChinaReviewed by:
Liang Zhao, Kyoto University, JapanMurugesan Raju, University of Missouri, United States
Copyright © 2025 Zhang, Tian, Tian, Si, Li and Fan. This is an open-access article distributed under the terms of the Creative Commons Attribution License (CC BY). The use, distribution or reproduction in other forums is permitted, provided the original author(s) and the copyright owner(s) are credited and that the original publication in this journal is cited, in accordance with accepted academic practice. No use, distribution or reproduction is permitted which does not comply with these terms.
*Correspondence: Ting Fan, dGZhbkBjbXUuZWR1LmNu
†These authors have contributed equally to this work and share first authorship