- 1State Key Laboratory of Nonlinear Mechanics (LNM) and Beijing Key Laboratory of Engineered Construction and Mechanobiology, Institute of Mechanics, Chinese Academy of Sciences, Beijing, China
- 2School of Engineering Science, University of Chinese Academy of Sciences, Beijing, China
- 3College of Mechanical Engineering, Zhejiang University of Technology, Hangzhou, China
Cell-cell adhesion and the adhesion of cells to extracellular matrix are mediated by the specific binding of receptors on the cell membrane to their cognate ligands on the opposing surface. The adhesion receptors can exhibit affinity for nanoscale lipid clusters that form in the cell membrane. Experimental studies of such adhesion systems often involve a cell adhering either to a solid surface with immobile ligands or a supported lipid bilayer with mobile ligands. A central question in these cell-substrate adhesions is how the mobility of the ligands physically affects their binding to the adhesion receptors and thereby the behavior of the nanoscale lipid clusters associated with the receptors. Using a statistical mechanical model and Monte Carlo simulations for the adhesion of cells to substrates with ligands, we find that, for mobile ligands, binding to adhesion receptors can promote the formation of mesoscale lipid domains, which in turn enhances the receptor-ligand binding. However, in the case of immobile ligands, the receptor-ligand binding and the tendency for the nanoscale lipid clusters to further coalesce depend on the distribution of the ligands on the substrate. Our findings help to explain why different adhesion experiments for identifying the interplay between receptor-ligand binding and heterogeneities in cell membranes led to contradictory results.
1 Introduction
Cell-cell adhesion and the adhesion of cells to the extracellular matrix (ECM), mediated by the specific binding of receptors and ligands anchored to the apposing surfaces, governs numerous biological processes such as signal transduction, immune responses, cell locomotion, tissue formation, as well as cancer invasion and metastasis (Weikl and Lipowsky, 2004; Krobath et al., 2009; van der Merwe and Dushek, 2011; Benham-Pyle et al., 2015; Chang et al., 2020; Li and Song, 2020; Romani et al., 2021). In cell-cell adhesion, both receptors and ligands are laterally mobile, whereas in cell-ECM adhesion, mobile receptors on the cell membranes often bind immobile ligands presented on the apposing surface (Sackmann and Smith, 2014). The key quantity to characterizing the receptor-ligand binding is the equilibrium constant
The cell adhesion receptors can be associated with nanoscale lipid clusters in the cell membranes (Shao et al., 2015; Su et al., 2016; Stone et al., 2017). These clusters enriched in cholesterol and sphingolipids are specialized liquid-ordered membrane microdomains and termed as lipid rafts. They can be stabilized and made to coalesce, forming platforms that function in membrane signaling (Lingwood and Simons, 2010; Sezgin et al., 2017). Cell-cell adhesion experiments show that the adhesion of immune cells to the antigen presenting cells leads to the accumulation of lipid rafts and reorganization of signaling proteins at the immunological synapse, which in turn facilitate the receptor-ligand mediated antigen presentation and immune cell activation (Anderson and Roche, 2015). Disrupting the rafts in T cell membrane via cholesterol depletion directly reduces the binding constant K of the T cell receptor (TCR) and peptide-major histocompatibility complexes (pMHC) (Huang et al., 2010). Experiments of mimetic systems indicate that the adhesion of giant vesicles decorated with biotin to streptavidin-functionalized supported bilayers stabilizes raft heterogeneity in both isolated plasma membrane vesicles and synthetic vesicles, leading to protein aggregation within the adhesion zone. Destabilizing raft and protein heterogeneities in vesicles adversely affects the biotin-streptavidin mediated stable adhesion (Zhao et al., 2013). For the adhesion of cells to substrates with immobile ligands mimicking cell-ECM adhesion, experimental studies led to contradictory results regarding the interplay between receptor-ligand binding and coalescence of raft domains (Gaus et al., 2006; Mitchell et al., 2009; Evani and Ramasubramanian, 2016; Son et al., 2017). For example, Son et al. (Son et al., 2017) found that integrin-mediated adhesion of human aortic endothelial cells to a substrate facilitates raft domain formation and integrin clustering, which increase the cell-substrate adhesion. Conversely, Evani and Ramasubramanian (Evani and Ramasubramanian, 2016) observed that the adhesion of infected monocytes to the microchannels coated with E-selectin is enhanced due to the increased uniformity of lipid raft and CD44 distribution. These studies raise the question of how the mobility of the ligands physically affects their binding to the cell adhesion receptors and thereby the behavior of the nanoscale lipid clusters associated with the receptors.
In this paper, we use methods of classical statistical mechanics and Monte Carlo (MC) simulations to study the interplay between receptor-ligand binding and raft domain formation for two typical experimental systems of cell adhesion, where multicomponent membranes adhere to a supported planar bilayer with mobile ligands, or to a planar substrate with immobile ligands, as illustrated in Figure 1. We find that, in a biologically relevant range of model parameters, the interplay between receptor-ligand binding and raft domain formation depends strongly on the ligand mobility. In the former adhesion system with mobile ligands, the receptor-ligand binding can enhance the coalescence of the rafts associated with the receptors by means of membrane-mediated attraction between the receptor-ligand complexes. The raft coalescence in turn facilitates the formation of additional receptor-ligand complexes due to the less loss in the configurational entropy of the membrane. This suggests that the receptor-ligand binding and raft domain formation in cell-supported bilayer adhesion system are mutually beneficial. In the latter adhesion system with immobile ligands, the interplay depends sensitively on the distribution of the ligands immobilized on the substrate. Our results show that for ligands uniformly or randomly immobilized on the substrate, the receptor-ligand binding disfavors the aggregation of rafts, whereas for ligands immobilized in the form of clusters on the substrate, receptor-ligand binding and raft aggregation can cooperate.
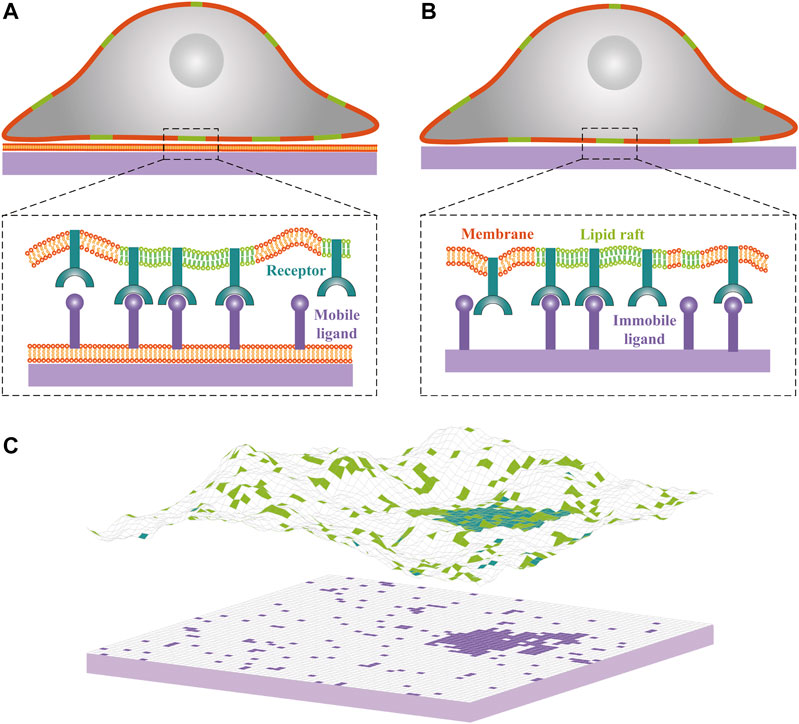
FIGURE 1. Cartoons of cell-substrate adhesion with (A) mobile ligands anchored to a lipid bilayer supported by a planar substrate and (B) immobile ligands directly attached to a planar substrate. Lipid rafts in the cell membrane are shown in green, cell adhesion receptors in blue, and ligands in purple. (C) Snapshot from Monte Carlo simulations of one membrane that adheres to a planar supported bilayer. Lipid rafts, receptors and ligands are indicated by square patches with the same color code as in panels (A) and (B). Each receptor or ligand occupies a single patch. The adhesion receptors exhibit weak affinity for lipid rafts, as reflected by accumulation of the blue patches within the green patches. One receptor-ligand complex forms only if the receptor and ligand are opposite each other and if the distance between receptor and ligand patches is within receptor-ligand binding range. Lipid rafts have a tendency to coalesce partially because of their hydrophobic mismatch with the membrane matrix.
2 Model and Methods
We employ a statistical-mechanical model that has been widely used to study both the dynamics (Bahrami and Weikl, 2018; Knezevic et al., 2018) and equilibrium behavior (Weikl et al., 2016; Li et al., 2018b) of cell adhesion. In our model of cell-substrate adhesion, the cell membrane, supported bilayer, as well as rigid planar substrate are represented by two-dimensional square lattices of size a, as shown in Figure 1C. The system bending energy can be written in a discretized form as (Li et al., 2020; Li et al., 2018a)
which governs the elastic deformation of the cell membrane. Here κ is the bending rigidity of cell membrane,
We set the lattice size
where the square-well binding potential
In our model, only the cell membrane contains lipid raft. Each raft patch is represented by a square lattice of size
To consider the tendency for lipid rafts to coalesce due to their hydrophobic mismatch with the membrane matrix, we assume a contact energy u for nearest-neighbor raft patches. The total raft-raft contact energy is then described by (Li et al., 2017b)
which sums over all pairs of nearest-neighbor raft patches i and j.
We employ the Metropolis MC method to simulate the adhesion systems with total energy
We have simulated the adhering membrane with an area of
We also develop a mean field (MF) theory to study the phase behavior of adhesion system with planar supported membrane containing mobile ligand molecules, as detailed in the Supporting Information (SI). Briefly, we first introduce the grand-canonical Hamiltonian, as given by Supplementary Eq. S1 in SI. Then we treat the raft-raft interaction in a MF manner and derive the approximate free energy of the system by considering the contact probability
3 Results
3.1 Adhesion of Cell Membrane to the Planar Supported Membrane with Mobile Ligands
Before exploring the interplay between receptor-ligand binding and raft domain formation, we first consider a limiting case of rigid and planar membranes (
Consider now the adhesion systems with mobile receptors that have affinity for lipid rafts in the cell membrane and mobile ligands anchored to a planar supported bilayer as illustrated in Figure 1A. To first explore the effect of the receptor-ligand binding on the phase behavior, we perform a series of MC simulations with the receptor concentration
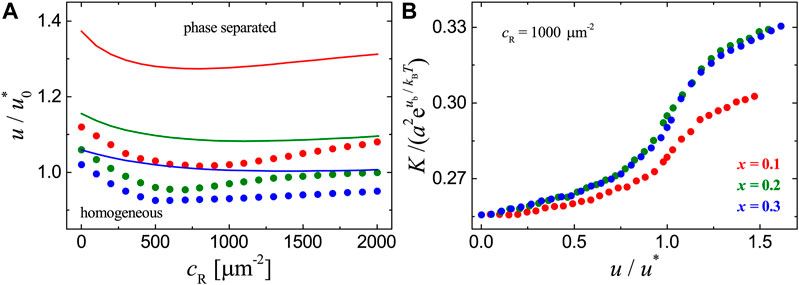
FIGURE 2. Results for the adhesion systems with mobile ligands and receptor-ligand binding strength
To clearly show how the raft domain formation affects the receptor-ligand binding, we plot the binding constant K measured in MC simulations as a function of raft-raft contact energy u in Figure 2B. For each adhesion system with fixed concentration
3.2 Adhesion of Cell Membrane to the Substrate with Immobile Ligands
We now turn to the cell-substrate adhesion systems with ligands immobilized on the flat substrate as illustrated in Figure 1B. We consider three types of distributions for the immobile ligands, namely, (i) in clusters, (ii) uniform, and (iii) random. For the first type of ligand distribution, we perform MC simulations with raft area fraction
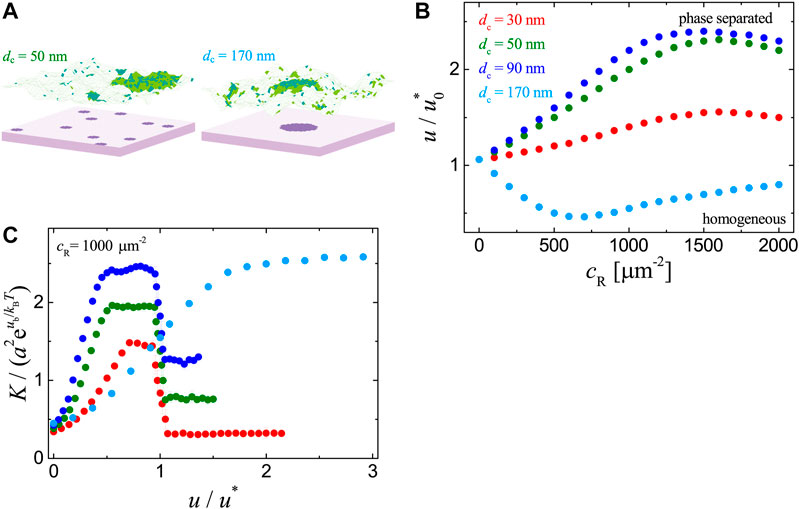
FIGURE 3. Results for the adhesion systems with immobile ligands in clusters as obtained from MC simulations with
We further analyze the dependence of receptor-ligand binding constant K on the raft-raft contact energy u. As illustrated in Figure 3C, in the case of one single ligand cluster (
We then consider the adhesion systems with ligands uniformly or randomly immobilized on the substrate. We’ve performed MC simulations for the two cases with ligand concentration
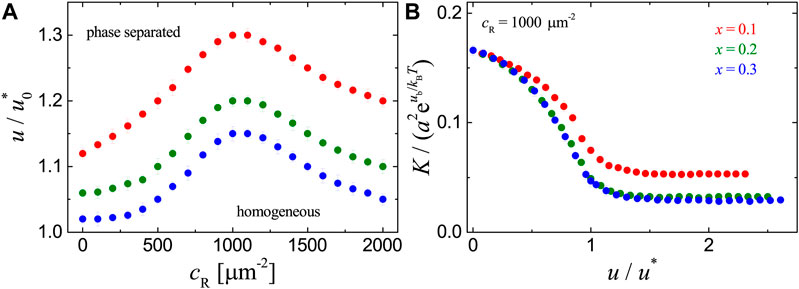
FIGURE 4. Results for the adhesion systems with ligands immobilized uniformly on the substrate as obtained from MC simulations with
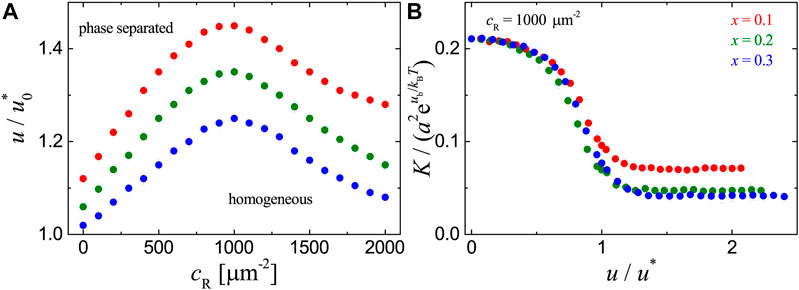
FIGURE 5. Results for the adhesion systems with ligands immobilized randomly on the substrate as obtained from MC simulations with
As mentioned in Introduction, there is a discrepancy regarding the interplay between receptor-ligand binding and lipid raft coalescence in adhesion experiments with mobile (Huang et al., 2010; Anderson and Roche, 2015) and immobile ligands (Gaus et al., 2006; Mitchell et al., 2009; Evani and Ramasubramanian, 2016; Son et al., 2017). Our results suggest that the discrepancy may be caused by the mobility of ligands in cell-substrate adhesion. Consistent with the experiments with mobile ligands, we find that the receptor-ligand binding and raft domain formation are mutually beneficial. For cell-substrate adhesion with immobile ligands, we find that the distribution of ligands immobilized on the substrate is an important factor. Depending on the ligand distribution, the receptor-ligand binding and raft coalescence can be mutually beneficial or not. Experimentally, the immobilization of ligand proteins on the substrate depends on temperature, pH, buffer composition, ionic strength, as well as the properties of protein and substrate (Rabe et al., 2011). Different conditions can lead to different distributions of ligands on the substrate, which affects the interplay between receptor-ligand binding and raft domain formation.
4 Conclusion
We’ve investigated cell-substrate adhesion mediated by the binding of cell adhesion receptors to ligands that are either anchored to a substrate-supported planar lipid bilayer or directly immobilized on the flat substrate by means of MC simulations and MF calculations based on a classical statistical mechanical model. The model takes into account shape fluctuations of the cell membrane and weak coupling of adhesion receptors to lipid rafts within the cell membrane. We focused on how the ligand mobility plays a role in the interplay between the receptor-ligand binding and raft domain formation. In the biologically relevant range of model parameters, we find that, for the adhesion systems with mobile ligands, the receptor-ligand binding promotes the coalescence of raft domains, which in turn enhances the binding. The lateral attraction between receptor-ligand complexes induced by the membrane shape fluctuations is responsible for the positive correlation.
In the adhesion systems with immobile ligands, we find that the interplay between receptor-ligand binding and raft domain formation depends on the distribution of ligands. For uniformly or randomly immobilized ligands, their binding to raft-associated receptors disfavors the aggregation of rafts and vice versa. For substrate-immobilized ligands in clusters, the receptor-ligand binding and raft domain formation can be mutually beneficial or not depending on whether there exists one single or multiple clusters of immobile ligands. The interplay can be understood by considering the loss in membrane configurational entropy and the raft translational entropy upon binding. Our findings not only reveal that ligand mobility and distribution are important physical factors in cell-substrate adhesion, but also help to explain the seemingly contradictory results regarding the interplay between R-L binding and raft heterogeneities in cell-substrate adhesion experiments (Gaus et al., 2006; Mitchell et al., 2009; Huang et al., 2010; Anderson and Roche, 2015; Evani and Ramasubramanian, 2016; Son et al., 2017), where the mobility and distribution of ligands on the substrate should be carefully taken into account.
Data Availability Statement
The original contributions presented in the study are included in the article/Supplementary Material, further inquiries can be directed to the corresponding authors.
Author Contributions
YS, HW and FS designed the research; LL performed computer simulations and analytical calculations; XW and HW assisted with data analysis and interpretation; LL, YS, HW and FS analyzed and interpreted the results, and wrote the manuscript.
Funding
This work was supported by the NSFC Basic Science Center Program for “Multiscale Problems in Nonlinear Mechanics” (11988102), the Youth Innovation Promotion Association CAS, the National Natural Science Foundation of China (11902327 and 11972041), and the Strategic Priority Research Program of the Chinese Academy of Sciences (XDB22040102).
Conflict of Interest
The authors declare that the research was conducted in the absence of any commercial or financial relationships that could be construed as a potential conflict of interest.
The handling editor declared a past co-authorship with one of the authors LL.
Acknowledgments
LL thanks Shouqin Lü (Institute of Mechanics, Chinese Academy of Sciences) for insightful discussions.
Supplementary Material
The Supplementary Material for this article can be found online at: https://www.frontiersin.org/articles/10.3389/fmolb.2021.655662/full#supplementary-material.
References
Anderson, H. A., and Roche, P. A. (2015). MHC Class II association with lipid rafts on the antigen presenting cell surface. BBA-Mol. Cell Res. 1853, 775–780. doi:10.1016/j.bbamcr.2014.09.019
Bahrami, A. H., and Weikl, T. R. (2018). Curvature-mediated assembly of janus nanoparticles on membrane vesicles. Nano Lett. 18, 1259–1263. doi:10.1021/acs.nanolett.7b04855
Bell, G. (1978). Models for the specific adhesion of cells to cells. Science 200, 618–627. doi:10.1126/science.347575
Benham-Pyle, B. W., Pruitt, B. L., and Nelson, W. J. (2015). Mechanical strain induces E-cadherin-dependent Yap1 and -catenin activation to drive cell cycle entrys. Science 348, 1024–1027. doi:10.1126/science.aaa4559
Chang, Y. C., Wu, J. W., Wang, C. W., and Jang, A. C. C. (2020). Hippo signaling-mediated mechanotransduction in cell movement and cancer metastasis. Front. Mol. Biosci. 6, 157. doi:10.3389/fmolb.2019.00157
Doan, T. L. L., Guerardel, Y., Loubiere, P., Mercier-Bonin, M., and Dague, E. (2011). Measuring kinetic dissociation/association constants between Lactococcus lactis bacteria and mucins using living cell probes. Biophys. J. 101, 2843–2853. doi:10.1016/j.bpj.2011.10.034
Evani, S. J., and Ramasubramanian, K. (2016). Biophysical regulation of chlamydia pneumoniae-infected monocyte recruitment to atherosclerotic foci. Sci. Rep. 6, 19058. doi:10.1038/srep19058
Fallahi-Sichani, M., and Linderman, J. J. (2009). Lipid raft-mediated regulation of G-protein coupled receptor signaling by ligands which influence receptor dimerization: a computational study. PloS One 4, e6604. doi:10.1371/journal.pone.0006604
Fenz, S. F., Bihr, T., Schmidt, D., Merkel, R., Seifert, U., Sengupta, K., et al. (2017). Membrane fluctuations mediate lateral interaction between cadherin bonds. Nat. Phys. 13, 906–913. doi:10.1038/nphys4138
Foster, L. J., de Hoog, C. L., and Mann, M. (2003). Unbiased quantitative proteomics of lipid rafts reveals high specificity for signaling factors. Proc. Natl. Acad. Sci. U.S.A. 100, 5813–5818. doi:10.1073/pnas.0631608100
Gambin, Y., Lopez-Esparza, R., Reffay, M., Sierecki, E., Gov, N. S., Genest, M., et al. (2006). Lateral mobility of proteins in liquid membranes revisited. Proc. Natl. Acad. Sci. U. S. A. 103, 2098–2102. doi:10.1073/pnas.0511026103
Hodges, K., Le Lay, S., Balasubramanian, N., and Schwartz, M. A. (2006). Integrin-mediated adhesion regulates membrane order. J. Cell Biol. 174, 725–734. doi:10.1083/jcb.200603034
Hu, J., Lipowsky, R., and Weikl, T. R. (2013). Binding constants of membrane-anchored receptors and ligands depend strongly on the nanoscale roughness of membranes. Proc. Natl. Acad. Sci. U.S.A. 110, 15283–15288. doi:10.1073/pnas.1305766110
Huang, J., Zarnitsyna, V. I., Liu, B., Edwards, L. J., Jiang, N., et al. (2010). The kinetics of two-dimensional TCR and pMHC interactions determine T-cell responsiveness. Nature 464, 932–936. doi:10.1038/nature08944
Knezevic, M., Jiang, H. D., and Wang, S. S. (2018). Active tuning of synaptic patterns enhances immune discrimination. Nano Lett. 121, 238101. doi:10.1103/PhysRevLett.121.238101
Krobath, H., Rozycki, B., Lipowsky, R., and Weikl, T. R. (2009). Binding cooperativity of membrane adhesion receptors. Soft Matt. 5, 3354–3361. doi:10.1039/b902036e
Li, L., Hu, J., Li, L., and Song, F. (2019). Binding constant of membrane-anchored receptors and ligands that induce membrane curvatures. Soft Matter 15, 3507–3514. doi:10.1039/c8sm02504e
Li, L., Hu, J., Różycki, B., and Song, F. (2020). Intercellular receptor-ligand binding and thermal fluctuations facilitate receptor aggregation in adhering membranes. Nano Lett. 20, 722–728. doi:10.1021/acs.nanolett.9b04596
Li, L., Hu, J., Shi, X., Shao, Y., and Song, F. (2017a). Lipid rafts enhance the binding constant of membrane-anchored receptors and ligands. Soft Matter 13, 4294–4304. doi:10.1039/c7sm00572e
Li, L., Hu, J., Xu, G., and Song, F. (2018a). Binding constant of cell adhesion receptors and substrate-immobilized ligands depends on the distribution of ligands. Phys. Rev. E 97, 012405. doi:10.1103/physreve.97.012405
Li, L., Shao, Y., Li, W., and Song, F. (2018b). Entropic pressure between fluctuating membranes in multilayer systems. Sci. China: Phys. Mech. Astron. 61, 128711. doi:10.1007/s11433-018-9264-x
Li, L., and Song, F. (2020). Biomechanical research into lamina cribrosa in glaucoma. Natl. Sci. Rev. 7, 1277–1279. doi:10.1093/nsr/nwaa063
Li, L., Xu, G., and Song, F. (2017b). Impact of lipid rafts on the T-cell-receptor and peptide-major-histocompatibility-complex interactions under different measurement conditions. Phys. Rev. E 95, 012403. doi:10.1103/physreve.95.012403
Limozin, L., Bongrand, P., and Robert, P. (2016). A rough energy landscape to describe surface-linked antibody and antigen bond formation. Sci. Rep. 6, 35193. doi:10.1038/srep35193
Lingwood, D., and Simons, K. (2010). Lipid rafts as a membrane-organizing principle. Science 327, 46–50. doi:10.1126/science.1174621
Mitchell, J. S., Brown, W. S., Woodside, D. G., Vanderslice, P., and McIntyre, B. W. (2009). Clustering T‐cell GM1 lipid rafts increases cellular resistance to shear on fibronectin through changes in integrin affinity and cytoskeletal dynamics. Immunol. Cell Biol. 87, 324–336. doi:10.1038/icb.2008.103
Montroll, E. W., Potts, R. B., and Ward, J. C. (1963). Correlations and spontaneous magnetization of the two-dimensional ising model. J. Math. Phys. 4, 308–322. doi:10.1063/1.1703955
O’Donoghue, G. P., Pielak, R. M., Smoligovets, A. A., Lin, J. J., and Groves, J. T. (2013). Direct single molecule measurement of TCR triggering by agonist pMHC in living primary T cells. eLife 2, e00778. doi:10.7554/eLife.00778
Rabe, M., Verdes, D., and Seeger, S. (2011). Understanding protein adsorption phenomena at solid surfaces. Adv. Colloid Interfac. 162, 87–106. doi:10.1016/j.cis.2010.12.007
Ramadurai, S., Holt, A., Krasnikov, V., van den Bogaart, G., Killian, J. A., and Poolman, B. (2009). Lateral diffusion of membrane proteins. J. Am. Chem. Soc. 131, 12650–12656. doi:10.1021/ja902853g
Romani, P., Valcarcel-Jimenez, L., Frezza, C., and Dupont, S. (2021). Crosstalk between mechanotransduction and metabolism. Nat. Rev. Mol. Cell Bio. 22, 22–38. doi:10.1038/s41580-020-00306-w
Rozycki, B., Lipowsky, R., and Weikl, T. R. (2010). Segregation of receptorligand complexes in cell adhesion zones: phase diagrams and the role of thermal membrane roughness. New J. Phys. 12, 095003. doi:10.1088/1367-2630/12/9/095003
Sackmann, E., and Smith, A.-S. (2014). Physics of cell adhesion: some lessons from cell-mimetic systems. Soft Matt. 10, 1644–1659. doi:10.1039/c3sm51910d
Sezgin, E., Levental, I., Mayor, S., and Eggeling, C. (2017). The mystery of membrane organization: composition, regulation and roles of lipid rafts. Nat. Rev. Mol. Cell Biol. 18, 361–374. doi:10.1038/nrm.2017.16
Shao, B., Yago, T., Setiadi, H., Wang, Y., Mehta-D’souza, P., Fu, J., et al. (2015). O-glycans direct selectin ligands to lipid rafts on leukocytes. Proc. Natl. Acad. Sci. U.S.A. 112, 8661–8666. doi:10.1073/pnas.1507712112
Simons, K., and Toomre, D. (2000). Lipid rafts and signal transduction. Nat. Rev. Mol. Cell Biol. 1, 31–39. doi:10.1038/35036052
Son, S. Y., Moroney, G. R., and Butler, P. G. (2017). -integrin-mediated adhesion is lipid-bilayer dependent. Biophys. J. 113, 1080–1092. doi:10.1016/j.bpj.2017.07.010
Speck, T., Reister, E., and Seifert, U. (2010). Specific adhesion of membranes: mapping to an effective bond lattice gas. Phys. Rev. E 82, 021923. doi:10.1103/physreve.82.021923
Steinkühler, J., Różycki, B., Alvey, C., Lipowsky, R., Weikl, T. R., Dimova, R., et al. (2019). Membrane fluctuations and acidosis regulate cooperative binding of ‘marker of self protein’ CD47 with the macrophage checkpoint receptor SIRPα. J. Cell Sci. 132, jcs216770. doi:10.1242/jcs.216770
Stone, M. B., Shelby, S. A., Nunez, M. F., Wisser, K., and Veatch, S. L. (2017). Protein sorting by lipid phase-like domains supports emergent signaling function in B lymphocyte plasma membranes. Elife 6, e19891. doi:10.7554/elife.19891
Su, X., Ditlev, J. A., Hui, E., Xing, W., Banjade, S., Okrut, J., et al. (2016). Phase separation of signaling molecules promotes T cell receptor signal transduction. Science 352, 595–599. doi:10.1126/science.aad9964
Tsourkas, P. K., Longo, M. L., and Raychaudhuri, S. (2008). Monte Carlo study of single molecule diffusion can elucidate the mechanism of B cell synapse formation. Biophys. J. 95, 1118–1125. doi:10.1529/biophysj.107.122564
van der Merwe, P. A., and Dushek, O. (2011). Mechanisms for t cell receptor triggering. Nat. Rev. Immunol. 11, 47–55. doi:10.1038/nri2887
Weikl, T. R., Hu, J., Xu, G.-K., and Lipowsky, R. (2016). Binding equilibrium and kinetics of membrane-anchored receptors and ligands in cell adhesion: insights from computational model systems and theory. Cell Adhes. Migr. 10, 576–589. doi:10.1080/19336918.2016.1180487
Weikl, T. R., and Lipowsky, R. (2004). Pattern formation during T-cell adhesion. Biophys. J. 87, 3665–3678. doi:10.1529/biophysj.104.045609
Xu, G.-K., Hu, J., Lipowsky, R., and Weikl, T. R. (2015). Binding constants of membrane-anchored receptors and ligands: a general theory corroborated by monte carlo simulations. J. Chem. Phys. 143, 243136. doi:10.1063/1.4936134
Zhao, J., Wu, J., and Veatch, S. L. (2013). Adhesion stabilizes robust lipid heterogeneity in supercritical membranes at physiological temperature. Biophys. J. 104, 825–834. doi:10.1016/j.bpj.2012.12.047
Keywords: cell adhesion, receptor-ligand binding, nanoscale lipid cluster, ligand mobility, phase separation, binding constant
Citation: Li L, Wang X, Wu H, Shao Y, Wu H and Song F (2021) Interplay Between Receptor-Ligand Binding and Lipid Domain Formation Depends on the Mobility of Ligands in Cell-Substrate Adhesion. Front. Mol. Biosci. 8:655662. doi: 10.3389/fmolb.2021.655662
Received: 19 January 2021; Accepted: 17 February 2021;
Published: 12 April 2021.
Edited by:
Bartosz Rozycki, Institute of Physics, Polish Academy of Sciences, PolandReviewed by:
Guang-Kui Xu, Xi’an Jiaotong University, ChinaJiuling Wang, University of South Carolina, United States
Yunfeng Chen, The Scripps Research Institute, United States
Copyright © 2021 Li, Wang, Wu, Shao, Wu and Song. This is an open-access article distributed under the terms of the Creative Commons Attribution License (CC BY). The use, distribution or reproduction in other forums is permitted, provided the original author(s) and the copyright owner(s) are credited and that the original publication in this journal is cited, in accordance with accepted academic practice. No use, distribution or reproduction is permitted which does not comply with these terms.
*Correspondence: Yingfeng Shao, c2hhb3lmQGxubS5pbWVjaC5hYy5jbg==; Huaping Wu, d3VodWFwaW5nQGdtYWlsLmNvbQ==; Fan Song, c29uZ2ZAbG5tLmltZWNoLmFjLmNu