- 1Division of Physical Chemistry, Department of Chemistry, Lund University, Lund, Sweden
- 2Division of Biochemistry and Structural Biology, Department of Chemistry, Lund University, Lund, Sweden
- 3Center for Neutron Research, National Institute of Standards and Technology, Gaithersburg, MD, United States
- 4Chemical and Biomolecular Engineering Department, University of Delaware, Newark, DE, United States
- 5Institut Laue-Langevin, Grenoble, France
- 6ISIS Neutron and Muon Source, Harwell Oxford, Didcot, United Kingdom
- 7Max IV Laboratory, Lund University, Lund, Sweden
- 8Life Sciences Group, Institut Laue-Langevin, Grenoble, France
- 9European Spallation Source, Lund, Sweden
The dense accumulation of α-Synuclein fibrils in neurons is considered to be strongly associated with Parkinson’s disease. These intracellular inclusions, called Lewy bodies, also contain significant amounts of lipids. To better understand such accumulations, it should be important to study α-Synuclein fibril formation under conditions where the fibrils lump together, mimicking what is observed in Lewy bodies. In the present study, we have therefore investigated the overall structural arrangements of α-synuclein fibrils, formed under mildly acidic conditions, pH = 5.5, in pure buffer or in the presence of various model membrane systems, by means of small-angle neutron scattering (SANS). At this pH, α-synuclein fibrils are colloidally unstable and aggregate further into dense clusters. SANS intensities show a power law dependence on the scattering vector, q, indicating that the clusters can be described as mass fractal aggregates. The experimentally observed fractal dimension was d = 2.6 ± 0.3. We further show that this fractal dimension can be reproduced using a simple model of rigid-rod clusters. The effect of dominatingly attractive fibril-fibril interactions is discussed within the context of fibril clustering in Lewy body formation.
1 Introduction
Amyloids are protein-rich fibrillar aggregates that possess a characteristic β-sheet structure (Serpell, Berriman et al., 2000; Jahn, Makin et al., 2010). Their presence constitutes the hallmark for several related neurodegenerative diseases, including Parkinson’s, Alzheimer’s disease, and type II diabetes (Spillantini and Goedert 2000; Ghiso and Frangione 2002). The association of amyloid fibrils with various diseases has led to extensive research in the field of amyloid fibrils (Chiti and Dobson 2006; Eisenberg and Jucker 2012; Iadanza, Jackson et al., 2018; Ke, Zhou et al., 2020). Despite extensive studies, the link between the amyloid fibril formation and pathology is still unclear in several of these diseases, and therapies are just starting to emerge (Tanzi 2021).
The morphology and composition of the amyloid deposits vary among different diseases and may also vary for the same disease (Tycko 2015). Therefore, understanding the structural and chemical properties of the amyloid aggregates is highly relevant as the structural features of the amyloid deposits may carry information on the process and conditions that lead to their formation and may serve as a basis for therapeutic discoveries. This motivates detailed and systematic investigations of amyloid deposits formed under different conditions.
One protein that has received much interest in amyloid-related research is α-Synuclein, αS, associated with a group of overlapping neurodegenerative disorders called α-synucleinopathies (Spillantini and Goedert 2000; Visanji, Lang et al., 2019), comprising Parkinson’s disease, dementia with Lewy bodies and multiple system atrophy. Both Parkinson’s disease and dementia with Lewy bodies are characterized by intercellular inclusion bodies, known as Lewy bodies (Shults 2006). The demonstration that the main component of Lewy bodies is a β-sheet-rich, fibrillar form of αS (Shults 2006; Araki, Yagi et al., 2019; Lashuel 2020), has motivated extensive studies of αS fibrils (Waxman and Giasson 2009; Alam, Bousset et al., 2019; Guerrero-Ferreira, Kovacik et al., 2020). It has also been shown that Lewy bodies contain membrane lipids (Lashuel 2020; Mahul-Mellier, Burtscher et al., 2020), which has motivated detailed studies on interaction between αS and lipids (Pfefferkorn, Jiang et al., 2012; Andreasen, Lorenzen et al., 2015; Iyer and Claessens 2019; Lashuel 2020), covering systems where the protein is present in the monomeric state (Jain, Bhasne et al., 2013; Fusco, De Simone et al., 2014; Fusco, Pape et al., 2016; Hannestad, Rocha et al., 2020), during the aggregation (Jiang, de Messieres et al., 2013; Galvagnion, Brown et al., 2016; Gaspar, Pallbo et al., 2018) as well as in the final amyloid aggregates (Hellstrand et al., 2013b; Galvagnion, Topgaard et al., 2019; Gaspar, Idini et al., 2021).
Amyloid fibrils often have very large aspect ratios, L/D > 100 (length over cross-section diameter). Considering that fibrils are sufficiently charged to be colloidally stable, the large aspect ratios allow fibrils to form an overlapping network in solution above a critical volume fraction (overlap concentration)
In the case of αS, it has been shown that besides a long-range electrostatic repulsion, fibril-fibril interactions are also characterized by a short-range attractive interaction, presumably due to hydrophobic patches on the fibril surface (Semerdzhiev, Lindhoud et al., 2018; Pogostin, Linse et al., 2019). As the protein charge depends on the solution pH, the effective fibril-fibril interaction is hence pH dependent, and shifts from dominatingly repulsive to dominatingly attractive in the vicinity of the isoelectric point (pI ≈ 4.8 (Croke, Patil et al., 2011)) (Pogostin, Linse et al., 2019). Pogostin et al. (Pogostin, Linse et al., 2019) investigated αS fibril structure and interactions in pH range 5.5–7.5 and they found that the fibril structure, including its radius of 5.2 nm, was independent of the pH while the fibril-fibril interactions gradually switched from repulsive to attractive with decreasing pH. At pH = 5.5, the system no longer shows the property of a gel, indicating that the fibril network collapses into clusters.
The reason why these inclusions form in vivo are still not understood. We note, however, that such accumulations of aS fibrils, together with some other components, including lipids and other protein, are typically consequences of attractive interactions, suggesting that it could be of particular interest to study the behavior of αS fibrils under conditions when they are not colloidally stable. We achieved attractive, colloidally unstable αS fibrils under mildly acidic pH, close to the αS pI. In this study, we present a small angle neutron scattering (SANS) study of attractive αS fibrils formed at pH = 5.5, in pure buffer but also in the presence of different model lipid membrane systems. Small angle scattering is an ideal tool to study the arrangement of colloids on the 1–100 nm length scale (Glatter 2018), which includes amyloid fibrils (Ricci, Spinozzi et al., 2016), as it is non-destructive and experiments can be performed directly in a solution state.
2 Materials and Methods
2.1 α-Synuclein
Human αS was expressed in E. coli and purified as previously described in (Grey, Linse et al., 2011).
E. coli cell pellet containing matchout deuterated αS was prepared in the Deuteration Laboratory of the Institut Laue-Langevin (ILL) in Grenoble, France as described by (Hellstrand et al., 2013a). A high cell density fed-batch culture using 85% deuterated Enfors minimal medium was carried out with computer-controlled temperature at 30°C and pO2 at 30% saturation (Haertlein, Moulin et al., 2016). The degree of deuteration was 75%. Deuterated αS monomers were isolated as described above.
2.2 Vesicle Preparation
The lipids used in this study were the phospholipids 1,2-dioleoylysn-glycero-3-phosphocholine (DOPC), 1,2-dioleoyl-sn-glycero-3-phospho-l-serine (DOPS), 1-palmitoyl-2-oleoyl-glycero-3-phosphocholine and (POPC), 1-palimtoyl-2-oleoyl-sn-glycero-3-phosho-l-serine (POPS), 1,2-dimyristoyl-sn-glycero-3-phosphocholine (DMPC), 1,2-dimyristoyl-sn-glycero-3-phospho-l-serine (DMPS), and the ganglioside lipids GM1 and GM3 from ovine brain. All lipids were obtained from Avanti Polar Lipids (Alabaster, AL, Unites States). In the preparation of mixed lipid vesicles, lipids were weighted and mixed with the desired proportion (PC:other 9:1). The powder was dissolved in chloroform:methanol (3:1 volume ratio) mixture. The solvent was evaporated under a stream of
Vesicles were formed either via sonication or extrusion. The sonication was performed for 15 min, 10 s on/off duty at 75% amplitude on ice. The lipid dispersions were centrifuged for 10 min at 1361 rad/s in order to pellet any contaminating particles from the sonicator tip. The supernatant was collected and used as the vesicle dispersion. Extruded vesicles were prepared using a 100 nm pore size filters with 21 passes in total.
2.3 Samples
In the present study, we analyze and discuss scattering data from fibrils formed at different conditions, in the presence of model membranes with various lipid compositions, obtained at different neutron scattering facilities. For simplicity, samples are numerically labelled and are described in the Table 1, grouped together according to the scattering facility at which the samples were measured, as the sample preparation was different for each facility. A more detailed description of the sample preparation and the SANS experimental conditions is provided in the following text. The buffer used for all samples was a 10 mM MES buffer at pH = 5.5.
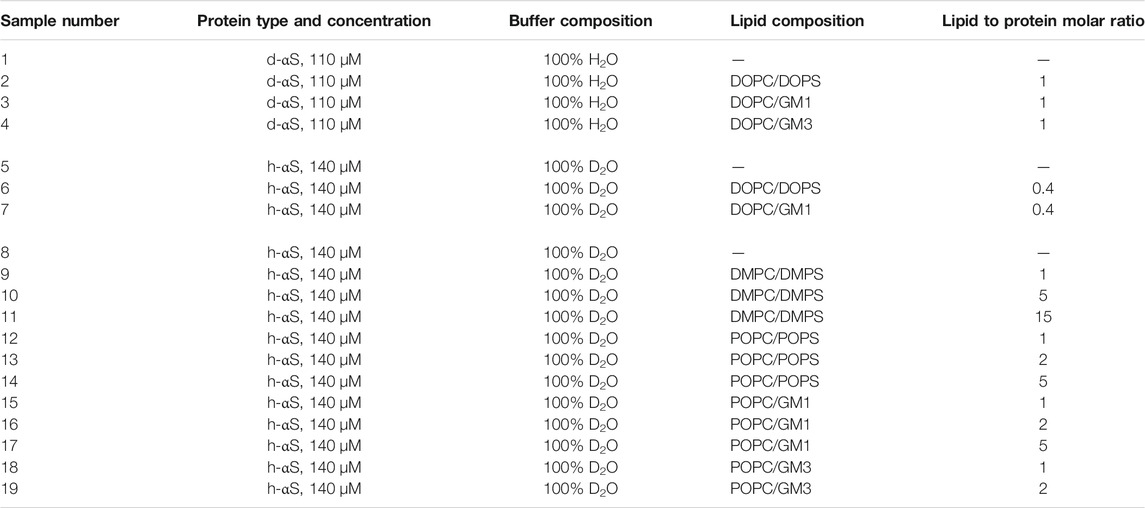
TABLE 1. Summary of samples investigated. The table shows the protein and lipid concentration, lipid composition in model membranes and deuteration level of the buffer used in the scattering experiment (M = mol/L).
2.3.1 Samples 1–4
Samples 1–4 were composed of deuterated αS, alone or in the presence of protonated lipids, in 100% H2O buffer. The monomeric αS protein was incubated alone (sample 1) or mixed with a dispersion of sonicated vesicles: DOPC:DOPS (sample 2), DOPC:GM1 (sample 3), or DOPC:GM3 (sample 4). The protein and lipid concentrations were both 110
After 72 h incubation the samples were centrifuged at 6,720 rcf for 2 min. The supernatant was separated from the sedimented fibrils and discarded. The separation of supernatant from the sediment was done to minimize the impact on the scattering profile of lipid residues that were not part of the aggregates and hence did not sediment during the centrifugation. Fibrils were then freeze-dried before transportation to the experimental site, where they were re-hydrated with buffer.
2.3.2 Samples 5–7
Samples 5–7 were composed of protonated αS, alone or in the presence of protonated lipids, in 100% D2O buffer. The monomeric αS protein was incubated alone (sample 5) or mixed with a dispersion of sonicated vesicles: DOPC:DOPS (sample 6), or DOPC:GM1 (sample 7) in H2O buffer. The protein concentration was 140
2.3.3 Samples 8–19
Samples 8–19 were composed of protonated αS, alone or mixed with protonated lipids, in 100% D2O buffer. The monomeric αS protein was incubated alone (sample 8) or mixed with a dispersion of extruded vesicles DMPC:DMPS (samples 9–11), POPC:DOPS (samples 12–14), POPC:GM1 (samples 15–17), or POPC:GM3 (samples 18 and 19). The protein concentration was 140
After the incubation period, a 4-step washing procedure was performed on samples 9–19 prior to the SANS experiments. This was done in order to wash away lipids from the sample allowing to record only fibril scattering. The first step of the procedure is centrifugation at 15,615 rcf for 15 min, which resulted in a formation of a dense pellet. The supernatant above the formed pellet was removed in the second step of the procedure. Afterwards, the pellet was resuspended in the same amount of buffer as had been removed in the second step. The fourth step of the procedure involves redispersing the pellet by shaking and gentle vortexing. This procedure was repeated five times.
2.4 Small Angle Neutron Scattering Experiments
Small angle neutron scattering (SANS) experiments were carried out at three different facilities. Below we describe the experimental procedures for each set of experiments.
Samples 1–4 were measured at the D22 beam line located at Institut Laue-Langevin (ILL) in Grenoble France. Three different sample-to-detector distances, 17.6, 5.6, and 1.4 m, with collimation lengths of 17.6, 5.6, and 2.8 m, respectively, were combined. The neutron wavelength was 6.0 Å with the wavelength spread of 10%. Detector patterns were reduced using Grasp software (C. Dewhurst), including thickness and background, as well as direct flux normalization to obtain scattered intensity in absolute units. Scattering curves obtained at the different sample-to-detector distances were combined giving a total q-range comprised between 0.002 and 0.6 Å−1, where q is the wave vector transfer. Samples were measured in single stopper cylindrical cells 120-QS Hellma quartz cuvettes with a 1 mm path length. Measurements were taken at 37°C with the use of a rotating rack to prevent the sedimentation of the fibrils.
Samples 5–7 were measured at the LOQ beamline located at the ISIS Neutron and Muon Source, Chilton, United Kingdom. Samples were measured in single stopper cylindrical cells 120-QS Hellma quartz cuvettes with a 1 and 2 mm path length. A fixed sample-to-detector distance (4 m) combined with a white beam and time-of-flight detection provided a q range of 0.009–0.25 Å−1. The raw scattering data collected at the LOQ instrument in ISIS were corrected for the efficiency and spatial linearity of the detectors, the sample transmission and the background scattering using the instrument dedicated software Mantid (https://www.mantidproject.org/) and the standard procedure indicated in the software guide. Data were then converted into scattered intensity data I(Q). These data were then placed on an absolute scale (cm−1) by comparison with the scattering profile collected from a calibration standard, constituted of a solid blend of hydrogenous and perdeuterated polystyrene which has been measured with the same instrument configuration as per established procedures (Wignall and Bates 1987). Measurements were performed at 37°C with the aid of a rotating rack in order to prevent the sedimentation of the fibrils.
Samples 8–19 were measured at NG7 SANS instrument located at NIST Center for Neutron Research, Gaithersburg, MD, United States. Measurements were performed at four sample to-detector distances (1, 4, 13, and 15.3 m with lenses), and a neutron wavelength of 6.0 Å (sample-to-detector distances of 1, 4, and 13 m) and 8.1 Å (15.3 m with lenses), to obtain a q range spanning from
3 Results and Discussion
3.1 SANS Studies of αS Fibrils
The current work explores, with the use of SANS, the structural organization of αS fibrils at pH 5.5, which is close to their isoelectric point. In these conditions, the fibrils are not colloidally stable, but precipitate out of solution by aggregating into clusters that are prone to sediment (Pogostin, Linse et al., 2019). A total of 19 different samples, for simplicity labeled from 1 to 19, were investigated.
Figure 1 shows SANS patterns, I(q), acquired from all 19 samples probed. The data are shifted with an arbitrary scale for better representation. The data on the absolute scale are shown in Supplementary Figure S1. As can be observed from Figure 1, the scattering patterns from the different samples are strikingly similar. They show a power law scattering,
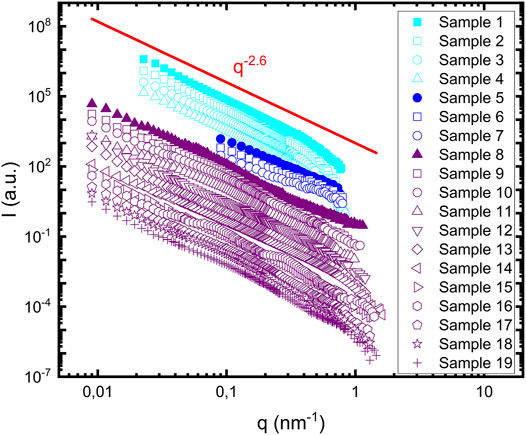
FIGURE 1. Scattering profiles of 19 samples summarized in Table 1. Samples containing lipids are represented with open symbols. Samples 1–4 are shown in cyan, samples five to seven are shown in blue and samples 8–19 are shown in purple. Samples containing protein alone are represented with filled symbols. The red line represents the power law dependence of the scattering profile, with a power value equal to 2.6.
αS fibrils have the shape of homogeneous cylinders, with a radius
3.2 Modeling of Fibril Clusters and Their Scattering
In order to better understand the fibril cluster organization, we have constructed fibril clusters using a simple fibril model. The approach by which individual fibrils are connected, is inspired by the FJC model. From the constructed fibril clusters, we calculate the corresponding scattering function, i.e., the cluster formfactor. Below, we present the model in detail and the way for calculating the scattering. As this is a new approach for describing rod clusters, that also may be used to analyze other rigid rod assemblies, we also analyze the model itself in some detail. To assess the present approach we analyze the model scattering function by comparing it with analytical Beaucage model (Hammouda 2010) of fractal objects.
In the present cluster model, individual fibrils were modeled as infinitesimally thin rods, represented by a straight line, of total Nmon point scatterers, referred to as monomers, that are separated by a distance dmon, as schematically illustrated in Figure 2A. Thus, the fibril length, L, is given by
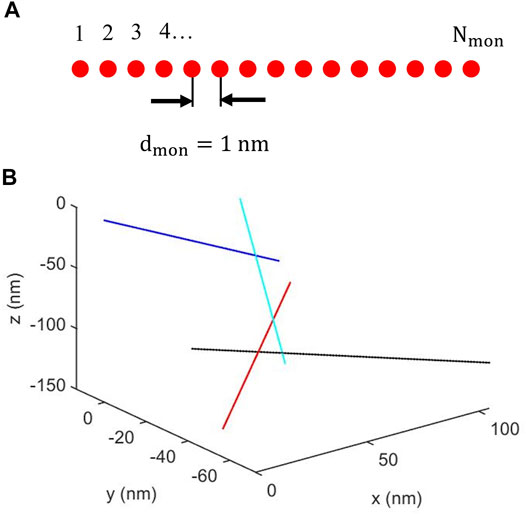
FIGURE 2. (A) Schematic illustration of a fibril composed of a linear array of Nmon identical monomers equally spaced with a separation dmon. (B) Example of cluster of four fibrils. For clarity, the fibrils are represented with different colors. Fibril #1 (blue) shares a monomer position with fibril #2 (cyan). Fibril #2 also shares a monomer position with fibril #3 (black), that in addition shares a monomer position with fibril #4 (red).
The spherically averaged scattering intensity, Pc(q), from the cluster, was then calculated from the spherically averaged Debye scattering equation (Farrow and Billinge 2009)
Here,
Figure 3 displays the scattering pattern
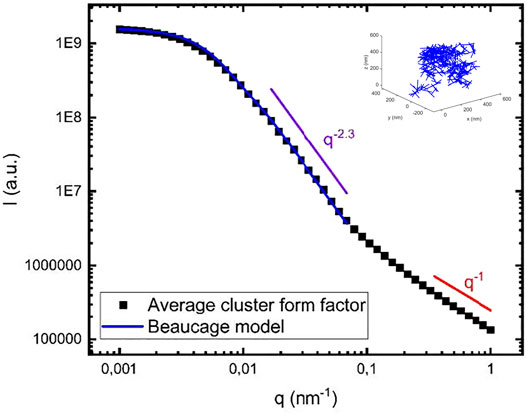
FIGURE 3. Calculated cluster form factor obtained from averaging over 20 different clusters, each containing 400 fibrils (black squares). As an example, one of the modeled clusters is shown in the inset. The blue line is a calculated scattering curve using the Beaucage model (see text) with
The model cluster involve two characteristic length scales, the overall cluster radius of gyration,
In Figure 3, the simulated scattering curve is also compared with the analytical Beaucage model (Hammouda 2010). Beaucage model describes fractal objects, and it has been used to describe amyloid fractals formed by amyloid-β, a protein involved in Alzheimer’s disease (Festa et al., 2019a; Festa et al., 2019b). The model describes a low q Guinier regime, followed by a Porod regime with a power law
Here, erf(x) is the error function and the so called Porod scale factor,
where
Experimentally we observe
and the fractal dimension
With Nmon = 100 and dmon = 1 nm, we obtain <l> = 33 nm. With this value of <l> and N = Nfib = 400 in Eq. 4 we obtain
Within this simple cluster model, reducing the effective step length l, by connecting the fibril segments randomly reduces
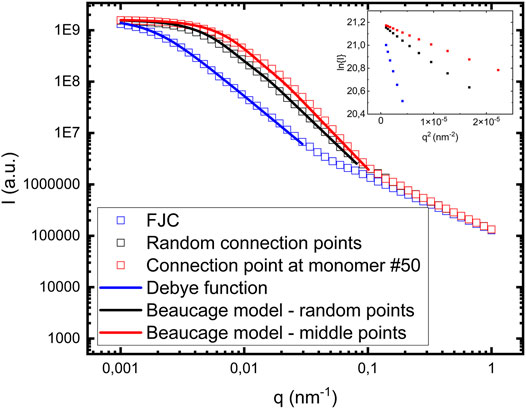
FIGURE 4. Comparison between different calculated cluster form factors: i) FJC model (blue empty squares), ii) random connection points (black empty squares), and iii) each fibril having one connection point at monomer #50 (red empty squares). The blue solid line in i) correspond to a model calculation using the Debye function (Eq. 6). The black and the red solid lines correspond to model calculations using the Beaucage model (Eqs 2 and 3). Rg decreases and d increases from i) to iii). The inset shows Guinier plots ln(I) vs q2 for the low q data, from which Rg also can be determined.
In Figure 4 we are also comparing with the FJC model, where fibrils are connected end-to-end. For ideal chains, like FJC chains, the form factor was derived by Debye (Debye 1947) and is consistent with d = 2
where x = qRg. Shown in Figure 4 as a solid blue line is a calculated model form factor PFJC(q) (Eq. 6) using Rg = 800 nm. This is in good agreement with Rg = 808 nm, calculated from Eq. 4.
As seen in Figure 4, Rg decreases and d increases as the distance between connection points in a fibril with neighboring fibrils is decreasing. Shown as an inset in Figure 4 are Guinier plots,
With the calculations presented above we demonstrate that within the simple model used, it is possible to construct fibril clusters having different fractal dimensions, including the values that we observe experimentally. Our experimentally observed value 2.6 is similar, but slightly larger than what is typically found for rod clusters (
In the experimental scattering patterns (Figure 1) we essentially observe only a single power law dependence of the scattered intensity
The model that we have used in Figures 3, 4 assumes infinitely thin fibrils. The mesh size of such clusters is approximately equal to 10 nm. To further illustrate the effect of a finite size cylinder, we have extended calculation, and we are showing them in the Supplementary Figure S5.
3.3 αS Clusters in Biology
αS fibrils are a major component of Lewy bodies, a pathological feature of Parkinson’s disease (Shults 2006; Araki, Yagi et al., 2019; Lashuel 2020). They are micrometre sized intracellular inclusions in the substantia nigra, that also contain lipids, membranous organelles, as well as other proteins (Lashuel 2020; Mahul-Mellier, Burtscher et al., 2020). Agglomerates and clusters of this kind are typically consequences of dominating attractive interactions, and recent work has indicated that the accumulations of various species through an effective liquid-liquid phase separation may be effective in various biological functions (Hyman, Weber et al., 2014). Colloidal interactions in the living cell, e.g., protein-protein interactions and protein membrane interactions are typically weakly repulsive, because essentially all colloidal aggregates and macromolecules carry a net negative charge. This ensures the colloidal stability of the living cell (Wennerström, Vallina Estrada et al., 2020). An interesting question concerns the origin of, and the reason for, the effective attractive interaction resulting in the accumulation of αS fibrils, and other components, that lead to the formation of Lewy bodies. Here, in combination with a previous work (Pogostin, Linse et al., 2019), we have shown that a pH drop from neutral to mildly acidic conditions (pH = 5.5) is sufficient to switch fibril-fibril interactions from being predominantly repulsive to become predominantly attractive resulting in a dense clustering of αS fibrils. At the same time, the rate of αS fibril formation is significantly increased at mildly acidic pH (pH = 5.5) due to strongly enhanced secondary nucleation (Cohen, Linse et al., 2013).
Mildly acidic pH is indeed found in some cellular compartments such as lysosomes and also in endosomes (Demaurex 2002; Hu, Dammer et al., 2015). Attractive fibril-fibril interactions may also result from cleavage of the acidic C-terminus, which up-shifts the isoelectric point, or from increased salt screening of long-range electrostatic repulsion. The use of mildly acidic pH to induce fibril clustering likely mimics these three cases and may provide a route towards the study of fibril organization in Lewy bodies.
3.4 Summary and Conclusion
Dispersions of αS fibrils formed at pH 5.5 behave significantly different compared to those formed at slightly higher pH where a stable fibril hydrogel network can be formed. (Pogostin et al., 2019). At pH 5.5 the formed αS fibrils are colloidally unstable and aggregate further into clusters. Inspired by the fact that Lewy bodies appear to contain accumulations of αS fibrils, indicating effectively attractive fibril-fibril interactions, we have here investigated αS fibrils clusters at pH = 5.5 in more detail. SANS experiments performed on 19 different samples show strikingly similar result. The SANS intensities show an extended power law dependence on the scattering vector, q, that is consistent with that the clusters can be described as mass fractals, with a fractal dimension d ≈ 2.6. To further conform this conclusion, we have developed a simple model of rigid rod clusters, that was found to be able to reproduce the experimentally observed fractal dimension. The simple cluster model is closely related to the classical FJC model of polymers, that may also serve as a reference case with d = 2.
Data Availability Statement
The raw data supporting the conclusions of this article will be made available by the authors, without undue reservation.
Author Contributions
MD, II, AJ, ES, SL, and UO designed research. MD, II, and VL performed experiments with the help of YL, AM, and AT. MH and JMD produced deuterated protein. MD and UO performed computer modeling and data analysis. MD, II, ES, SL, and UO wrote the paper with input from all other co-authors.
Funding
This work was partially supported by Swedish Foundation for Strategic Research through the national Graduate School SwedNessESS (GSn15–0008), the Swedish Research Council VR (SL 2015-00143; SL, ES, AJ 2014-03972) and the Knut and Alice Wallenberg Foundation grant (ES, SL, UO 2016.0074).
Conflict of Interest
The authors declare that the research was conducted in the absence of any commercial or financial relationships that could be construed as a potential conflict of interest.
Publisher’s Note
All claims expressed in this article are solely those of the authors and do not necessarily represent those of their affiliated organizations, or those of the publisher, the editors and the reviewers. Any product that may be evaluated in this article, or claim that may be made by its manufacturer, is not guaranteed or endorsed by the publisher.
Acknowledgments
MD wishes to thank the NIST Center for Neutron Research, for its kind hospitality during an extended stay at the facility. Certain commercial equipment, instruments, or software are identified in this paper to foster understanding. Such identification does not imply recommendation or endorsement by the National Institute of Standards and Technology, nor does it imply that the materials or equipment identified are necessarily the best available for the purpose.
Supplementary Material
The Supplementary Material for this article can be found online at: https://www.frontiersin.org/articles/10.3389/fmolb.2021.768004/full#supplementary-material
References
Alam, P., Bousset, L., Melki, R., and Otzen, D. E. (2019). α‐synuclein Oligomers and Fibrils: a Spectrum of Species, a Spectrum of Toxicities. J. Neurochem. 150 (5), 522–534. doi:10.1111/jnc.14808
Andreasen, M., Lorenzen, N., and Otzen, D. (2015). Interactions between Misfolded Protein Oligomers and Membranes: A central Topic in Neurodegenerative Diseases? Biochim. Biophys. Acta (Bba) - Biomembranes 1848 (9), 1897–1907. doi:10.1016/j.bbamem.2015.01.018
Araki, K., Yagi, N., Aoyama, K., Choong, C.-J., Hayakawa, H., Fujimura, H., et al. (2019). Parkinson's Disease Is a Type of Amyloidosis Featuring Accumulation of Amyloid Fibrils of α-synuclein. Proc. Natl. Acad. Sci. USA 116 (36), 17963–17969. doi:10.1073/pnas.1906124116
Chiti, F., and Dobson, C. M. (2006). Protein Misfolding, Functional Amyloid, and Human Disease. Annu. Rev. Biochem. 75, 333–366. doi:10.1146/annurev.biochem.75.101304.123901
Cohen, S. I. A., Linse, S., Luheshi, L. M., Hellstrand, E., White, D. A., Rajah, L., et al. (2013). Proliferation of Amyloid- 42 Aggregates Occurs through a Secondary Nucleation Mechanism. Proc. Natl. Acad. Sci. 110 (24), 9758–9763. doi:10.1073/pnas.1218402110
Croke, R. L., Patil, S. M., Quevreaux, J., Kendall, D. A., and Alexandrescu, A. T. (2011). NMR Determination of pKa Values in α-synuclein. Protein Sci. 20 (2), 256–269. doi:10.1002/pro.556
Debye, P. (1947). Molecular-weight Determination by Light Scattering. J. Phys. Chem. 51 (1), 18–32. doi:10.1021/j150451a002
Demaurex, N. (2002). pH Homeostasis of Cellular Organelles. Physiology 17 (1), 1–5. doi:10.1152/physiologyonline.2002.17.1.1
Eisenberg, D., and Jucker, M. (2012). The Amyloid State of Proteins in Human Diseases. Cell 148 (6), 1188–1203. doi:10.1016/j.cell.2012.02.022
Evans, D. F., and Wennerström, H. (1999). The Colloidal Domain: Where Physics, Chemistry, Biology, and Technology Meet. 2nd Edition. New Jersey, United States: Wiley VCH.
Farrow, C. L., and Billinge, S. J. L. (2009). Relationship between the Atomic Pair Distribution Function and Small-Angle Scattering: Implications for Modeling of Nanoparticles. Acta Cryst. Sect A. 65 (3), 232–239. doi:10.1107/s0108767309009714
Festa, G., Mallamace, F., Sancesario, G. M., Corsaro, C., Mallamace, D., Fazio, E., et al. (2019a). Aggregation States of Aβ1-40, Aβ1-42 and Aβp3-42 Amyloid Beta Peptides: A SANS Study. Ijms 20 (17), 4126. doi:10.3390/ijms20174126
Festa, G., Sancesario, G., Corsaro, C., Longo, S., Mallamace, D., Fazio, E., et al. (2019b). SANS Study of Amyloid β1−40: Unfolded Monomers in DMSO, Multidimensional Aggregates in Water Medium. Physica A: Stat. Mech. its Appl. 517, 385–391. doi:10.1016/j.physa.2018.11.027
Frohm, B., Denizio, J. E., Lee, D. S. M., Gentile, L., Olsson, U., Malm, J., et al. (2015). A Peptide from Human Semenogelin I Self-Assembles into a pH-Responsive Hydrogel. Soft Matter 11 (2), 414–421. doi:10.1039/c4sm01793e
Fusco, G., De Simone, A., Gopinath, T., Vostrikov, V., Vendruscolo, M., Dobson, C. M., et al. (2014). Direct Observation of the Three Regions in α-synuclein that Determine its Membrane-Bound Behaviour. Nat. Commun. 5, 3827. doi:10.1038/ncomms4827
Fusco, G., Pape, T., Stephens, A. D., Mahou, P., Costa, A. R., Kaminski, C. F., et al. (2016). Structural Basis of Synaptic Vesicle Assembly Promoted by α-synuclein. Nat. Commun. 7, 12563. doi:10.1038/ncomms12563
Galvagnion, C., Brown, J. W. P., Ouberai, M. M., Flagmeier, P., Vendruscolo, M., Buell, A. K., et al. (2016). Chemical Properties of Lipids Strongly Affect the Kinetics of the Membrane-Induced Aggregation of α-synuclein. Proc. Natl. Acad. Sci. USA 113 (26), 7065–7070. doi:10.1073/pnas.1601899113
Galvagnion, C., Topgaard, D., Makasewicz, K., Buell, A. K., Linse, S., Sparr, E., et al. (2019). Lipid Dynamics and Phase Transition within α-Synuclein Amyloid Fibrils. J. Phys. Chem. Lett. 10 (24), 7872–7877. doi:10.1021/acs.jpclett.9b03005
Gaspar, R., Idini, I., Carlström, G., Linse, S., and Sparr, E. (2021). Transient Lipid-Protein Structures and Selective Ganglioside Uptake during α-Synuclein-Lipid Co-aggregation. Front. Cel Dev. Biol. 9, 622764. doi:10.3389/fcell.2021.622764
Gaspar, R., Pallbo, J., Weininger, U., Linse, S., and Sparr, E. (2018). Ganglioside Lipids Accelerate Alpha-Synuclein Amyloid Formation. Biochim. Biophys. Acta Proteins Proteom, 1866(10):1062-1072. doi:10.1016/j.bbapap.2018.07.004
Ghiso, J., and Frangione, B. (2002). Amyloidosis and Alzheimer's Disease. Adv. Drug Deliv. Rev. 54, 1539–1551. doi:10.1016/s0169-409x(02)00149-7
Glatter, O. (2018). Scattering Methods and Their Application in Colloid and Interface Science. Elsevier, Amsterdam, Netherlands. doi:10.1016/C2016-0-04640-5
Glinka, C. J., Barker, J. G., Hammouda, B., Krueger, S., Moyer, J. J., and Orts, W. J. (1998). The 30 M Small-Angle Neutron Scattering Instruments at the National Institute of Standards and Technology. J. Appl. Cryst. 31, 430–445. doi:10.1107/s0021889897017020
Grey, M., Linse, S., Nilsson, H., Brundin, P., and Sparr, E. (2011). Membrane Interaction of α-Synuclein in Different Aggregation States. J. Parkinsons Dis. 1 (4), 359–371. doi:10.3233/jpd-2011-11067
Guerrero-Ferreira, R., Kovacik, L., Ni, D., and Stahlberg, H. (2020). New Insights on the Structure of Alpha-Synuclein Fibrils Using Cryo-Electron Microscopy. Curr. Opin. Neurobiol. 61, 89–95. doi:10.1016/j.conb.2020.01.014
Haertlein, M., Moulin, M., Devos, J. M., Laux, V., Dunne, O., and Trevor Forsyth, V. (2016). Biomolecular Deuteration for Neutron Structural Biology and Dynamics. Methods Enzymol. 566, 113–157. doi:10.1016/bs.mie.2015.11.001
Hammouda, B. (2010). Analysis of the Beaucage Model. J. Appl. Cryst. 43 (6), 1474–1478. doi:10.1107/s0021889810033856
Hannestad, J. K., Rocha, S., Agnarsson, B., Zhdanov, V. P., Wittung-Stafshede, P., and Höök, F. (2020). Single-vesicle Imaging Reveals Lipid-Selective and Stepwise Membrane Disruption by Monomeric α-synuclein. Proc. Natl. Acad. Sci. U S A. 117 (25), 14178–14186. doi:10.1073/pnas.1914670117
Hellstrand, E., Grey, M., Ainalem, M.-L., Ankner, J., Forsyth, V. T., Fragneto, G., et al. (2013a). Adsorption of α-Synuclein to Supported Lipid Bilayers: Positioning and Role of Electrostatics. ACS Chem. Neurosci. 4 (10), 1339–1351. doi:10.1021/cn400066t
Hellstrand, E., Nowacka, A., Topgaard, D., Linse, S., and Sparr, E. (2013b). Membrane Lipid Co-aggregation with α-Synuclein Fibrils. PLoS One 8 (10), e77235. doi:10.1371/journal.pone.0077235
Hu, Y. B., Dammer, E. B., Ren, R. J., and Wang, G. (2015). The Endosomal-Lysosomal System: from Acidification and Cargo Sorting to Neurodegeneration. Transl. Neurodegener. 4 (1), 18. doi:10.1186/s40035-015-0041-1
Hyman, A. A., Weber, C. A., and Jülicher, F. (2014). Liquid-Liquid Phase Separation in Biology. Annu. Rev. Cel Dev. Biol. 30 (1), 39–58. doi:10.1146/annurev-cellbio-100913-013325
Iadanza, M. G., Jackson, M. P., Hewitt, E. W., Ranson, N. A., and Radford, S. E. (2018). A new era for Understanding Amyloid Structures and Disease. Nat. Rev. Mol. Cel Biol. 19 (12), 755–773. doi:10.1038/s41580-018-0060-8
Iyer, A., and Claessens, M. M. A. E. (2019). Disruptive Membrane Interactions of Alpha-Synuclein Aggregates. Biochim. Biophys. Acta (Bba) - Proteins Proteomics 1867 (5), 468–482. doi:10.1016/j.bbapap.2018.10.006
Jahn, T. R., Makin, O. S., Morris, K. L., Marshall, K. E., Tian, P., Sikorski, P., et al. (2010). The Common Architecture of Cross-β Amyloid. J. Mol. Biol. 395, 717–727. doi:10.1016/j.jmb.2009.09.039
Jain, N., Bhasne, K., Hemaswasthi, M., and Mukhopadhyay, S. (2013). Structural and Dynamical Insights into the Membrane-Bound α-Synuclein. PLoS ONE 8 (12), e83752. doi:10.1371/journal.pone.0083752
Jiang, Z., de Messieres, M., and Lee, J. C. (2013). Membrane Remodeling by α-Synuclein and Effects on Amyloid Formation. J. Am. Chem. Soc. 135 (43), 15970–15973. doi:10.1021/ja405993r
Ke, P. C., Zhou, R., Serpell, L. C., Riek, R., Knowles, T. P. J., Lashuel, H. A., et al. (2020). Half a century of Amyloids: Past, Present and Future. Chem. Soc. Rev. 49 (15), 5473–5509. doi:10.1039/c9cs00199a
Kline, S. R. (2006). Reduction and Analysis of SANS and USANS Data Using IGOR Pro. J. Appl. Cryst. 39, 895–900. doi:10.1107/s0021889806035059
Lashuel, H. A. (2020). Do Lewy Bodies Contain Alpha-Synuclein Fibrils? and Does it Matter? A Brief History and Critical Analysis of Recent Reports. Neurobiol. Dis. 141, 104876. doi:10.1016/j.nbd.2020.104876
Lazzari, S., Nicoud, L., Jaquet, B., Lattuada, M., and Morbidelli, M. (2016). Fractal-like Structures in Colloid Science. Adv. Colloid Interf. Sci. 235, 1–13. doi:10.1016/j.cis.2016.05.002
Mahul-Mellier, A.-L., Burtscher, J., Maharjan, N., Weerens, L., Croisier, M., Kuttler, F., et al. (2020). The Process of Lewy Body Formation, rather Than Simply α-synuclein Fibrillization, Is One of the Major Drivers of Neurodegeneration. Proc. Natl. Acad. Sci. USA 117 (9), 4971–4982. doi:10.1073/pnas.1913904117
Mohraz, A., Moler, D. B., Ziff, R. M., and Solomon, M. J. (2004). Effect of Monomer Geometry on the Fractal Structure of Colloidal Rod Aggregates. Phys. Rev. Lett. 92 (15), 155503. doi:10.1103/PhysRevLett.92.155503
Murphy, R. P., Hatch, H. W., Mahynski, N. A., Shen, V. K., and Wagner, N. J. (2020). Dynamic Arrest of Adhesive Hard Rod Dispersions. Soft Matter 16 (5), 1279–1286. doi:10.1039/c9sm01877h
Pedersen, J. S. (1997). Analysis of Small-Angle Scattering Data from Colloids and Polymer Solutions: Modeling and Least-Squares Fitting. Adv. Colloid Interf. Sci. 70, 171–210. doi:10.1016/s0001-8686(97)00312-6
Pfefferkorn, C. M., Jiang, Z., and Lee, J. C. (2012). Biophysics of α-synuclein Membrane Interactions. Biochim. Biophys. Acta (Bba) - Biomembranes 1818 (2), 162–171. doi:10.1016/j.bbamem.2011.07.032
Pogostin, B. H., Linse, S., and Olsson, U. (2019). Fibril Charge Affects α-Synuclein Hydrogel Rheological Properties. Langmuir 35 (50), 16536–16544. doi:10.1021/acs.langmuir.9b02516
Ricci, C., Spinozzi, F., Mariani, P., and Grazia Ortore, M. (2016). Protein Amyloidogenesis Investigated by Small Angle Scattering. Cpd 22 (26), 3937–3949. doi:10.2174/1381612822666160519113237
Rubinstein, M., and Colby, R. H. (2003). Polymer Physics. Oxford University Press, Oxford, United Kingdom.
Semerdzhiev, S. A., Lindhoud, S., Stefanovic, A., Subramaniam, V., Van Der Schoot, P., and Claessens, M. M. A. E. (2018). Hydrophobic-Interaction-Induced Stiffening of α-Synuclein Fibril Networks. Phys. Rev. Lett. 120 (20), 208102. doi:10.1103/PhysRevLett.120.208102
Serpell, L. C., Berriman, J., Jakes, R., Goedert, M., and Crowther, R. A. (2000). Fiber Diffraction of Synthetic Alpha -synuclein Filaments Shows Amyloid-like Cross-Beta Conformation. Proc. Natl. Acad. Sci. 97 (9), 4897–4902. doi:10.1073/pnas.97.9.4897
Shults, C. W. (2006). Lewy Bodies. Proc. Natl. Acad. Sci. 103 (6), 1661–1668. doi:10.1073/pnas.0509567103
Solomon, M. J., and Spicer, P. T. (2010). Microstructural Regimes of Colloidal Rod Suspensions, Gels, and Glasses. Soft Matter 6 (7). doi:10.1039/b918281k
Spillantini, M. G., and Goedert, M. (2000). The Alpha-Synucleinopathies: Parkinson's Disease, Dementia with Lewy Bodies, and Multiple System Atrophy. Ann. N. Y. Acad. Sci. 920 (1), 16–27. doi:10.1111/j.1749-6632.2000.tb06900.x
Tanzi, R. E. (2021). FDA Approval of Aduhelm Paves a New Path for Alzheimer’s Disease. ACS Chem. Neurosci. 12(15):2714-2715. doi:10.1021/acschemneuro.1c00394
Tycko, R. (2015). Amyloid Polymorphism: Structural Basis and Neurobiological Relevance. Neuron 86 (3), 632–645. doi:10.1016/j.neuron.2015.03.017
Visanji, N. P., Lang, A. E., and Kovacs, G. G. (2019). Beyond the Synucleinopathies: Alpha Synuclein as a Driving Force in Neurodegenerative Comorbidities. Transl. Neurodegener. 8 (1), 28. doi:10.1186/s40035-019-0172-x
Waxman, E. A., and Giasson, B. I. (2009). Molecular Mechanisms of α-synuclein Neurodegeneration. Biochim. Biophys. Acta (Bba) - Mol. Basis Dis. 1792 (7), 616–624. doi:10.1016/j.bbadis.2008.09.013
Weitz, D. A., Huang, J. S., Lin, M. Y., and Sung, J. (1985). Limits of the Fractal Dimension for Irreversible Kinetic Aggregation of Gold Colloids. Phys. Rev. Lett. 54 (13), 1416–1419. doi:10.1103/physrevlett.54.1416
Wennerström, H., Vallina Estrada, E., Danielsson, J., and Oliveberg, M. (2020). Colloidal Stability of the Living Cell. Proc. Natl. Acad. Sci. USA 117 (19), 10113–10121. doi:10.1073/pnas.1914599117
Keywords: alpha-synuclein, amyloid fibril, fractal cluster, Lewy bodies (LB), small-angle neutron scattering (SANS), rigid-rod cluster modeling
Citation: Dubackic M, Idini I, Lattanzi V, Liu Y, Martel A, Terry A, Haertlein M, Devos JM, Jackson A, Sparr E, Linse S and Olsson U (2021) On the Cluster Formation of α-Synuclein Fibrils. Front. Mol. Biosci. 8:768004. doi: 10.3389/fmolb.2021.768004
Received: 31 August 2021; Accepted: 30 September 2021;
Published: 19 October 2021.
Edited by:
Giorgio Giardina, Sapienza University of Rome, ItalyReviewed by:
Henrich Frielinghaus, Helmholtz Association of German Research Centers (HZ), GermanyWilliam Heller, Oak Ridge National Laboratory, United States
Carmelo Corsaro, University of Messina, Italy
Copyright © 2021 Dubackic, Idini, Lattanzi, Liu, Martel, Terry, Haertlein, Devos, Jackson, Sparr, Linse and Olsson. This is an open-access article distributed under the terms of the Creative Commons Attribution License (CC BY). The use, distribution or reproduction in other forums is permitted, provided the original author(s) and the copyright owner(s) are credited and that the original publication in this journal is cited, in accordance with accepted academic practice. No use, distribution or reproduction is permitted which does not comply with these terms.
*Correspondence: Marija Dubackic, bWFyaWphLmR1YmFja2ljQGZrZW0xLmx1LnNl, bWFqdXNrYWRAaG90bWFpbC5jb20=
†These authors share first authorship